Questions and Answers of Data Science
VerifiedAdded on 2022/08/27
|7
|688
|22
AI Summary
Contribute Materials
Your contribution can guide someone’s learning journey. Share your
documents today.
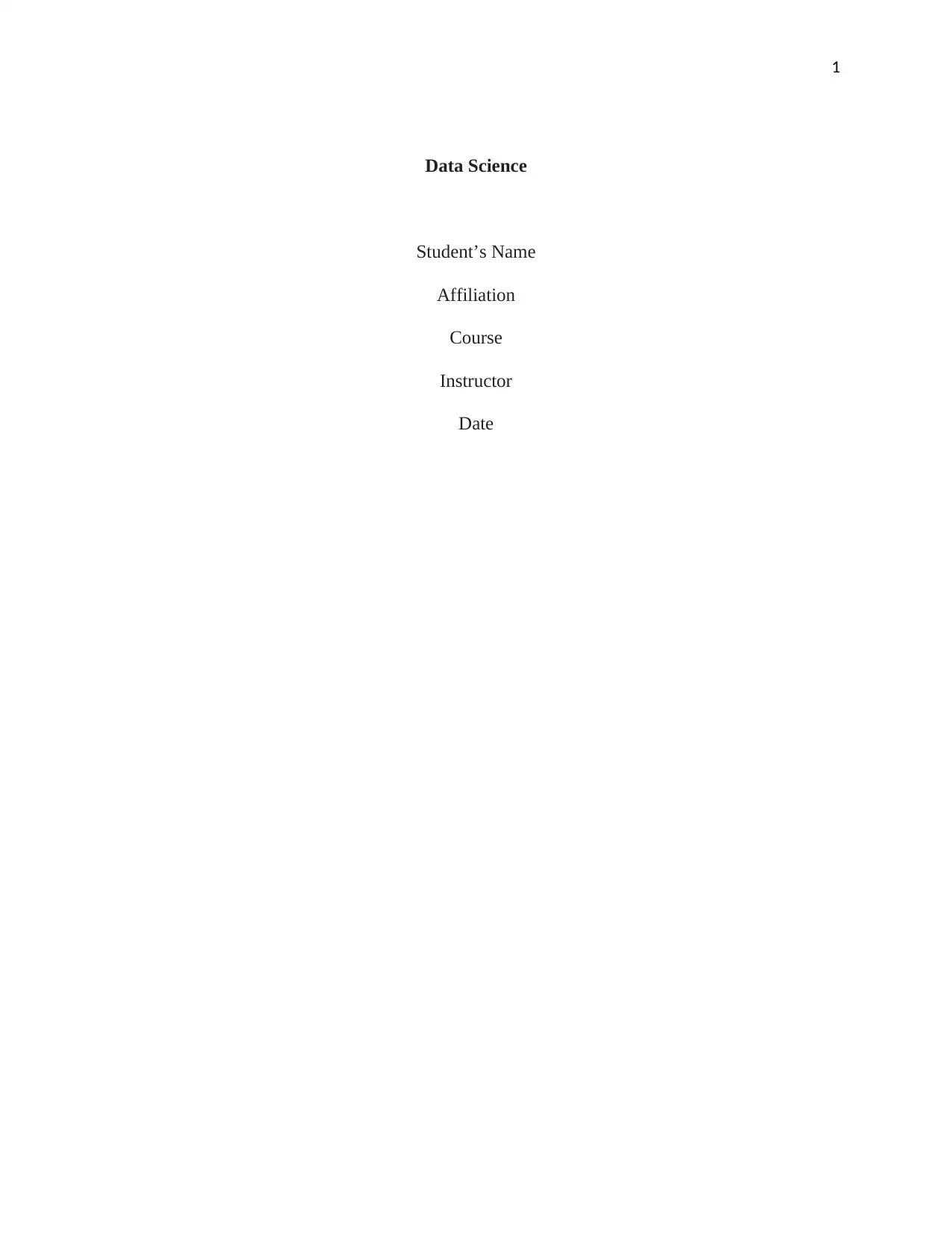
1
Data Science
Student’s Name
Affiliation
Course
Instructor
Date
Data Science
Student’s Name
Affiliation
Course
Instructor
Date
Secure Best Marks with AI Grader
Need help grading? Try our AI Grader for instant feedback on your assignments.
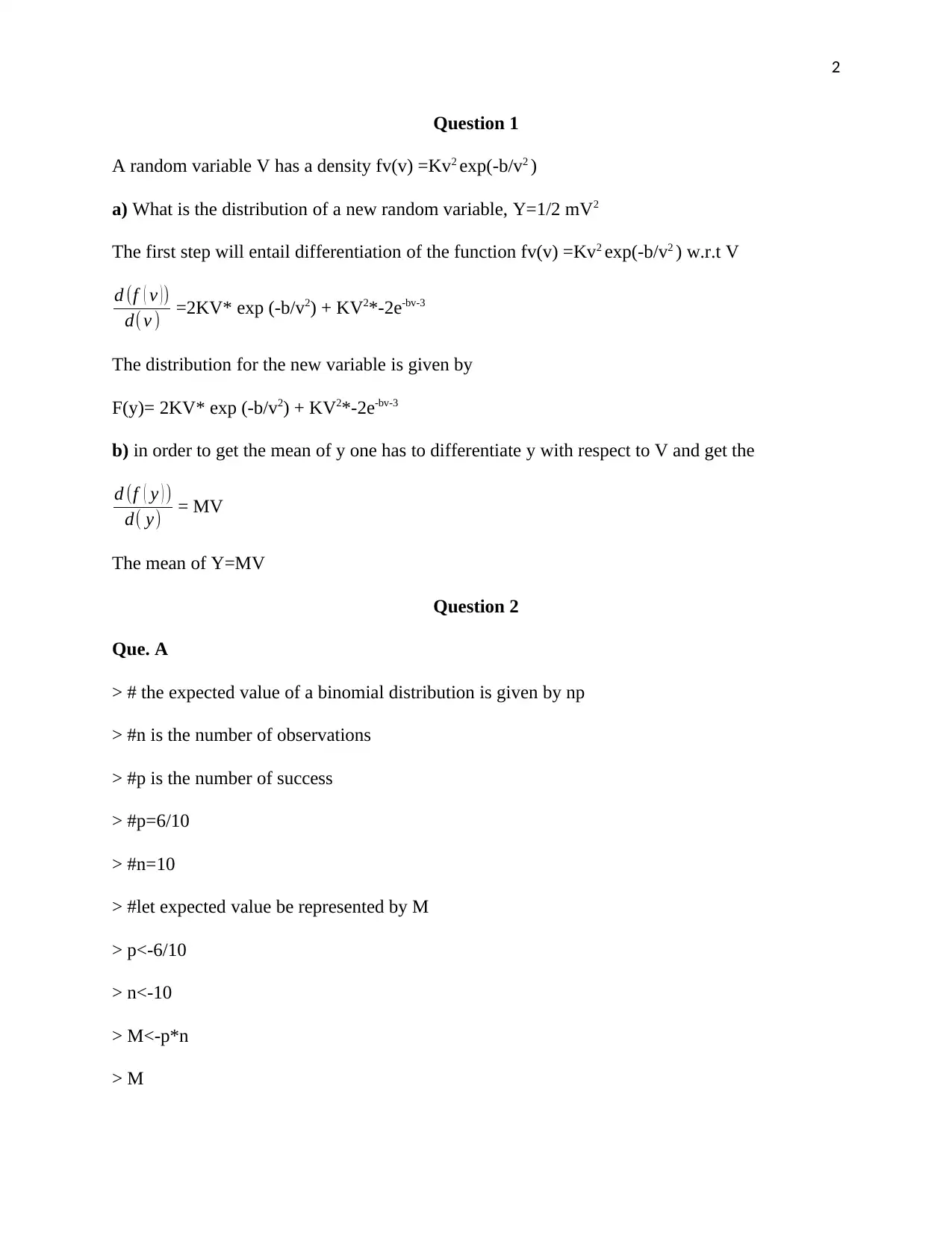
2
Question 1
A random variable V has a density fv(v) =Kv2 exp(-b/v2 )
a) What is the distribution of a new random variable, Y=1/2 mV2
The first step will entail differentiation of the function fv(v) =Kv2 exp(-b/v2 ) w.r.t V
d (f ( v ))
d(v ) =2KV* exp (-b/v2) + KV2*-2e-bv-3
The distribution for the new variable is given by
F(y)= 2KV* exp (-b/v2) + KV2*-2e-bv-3
b) in order to get the mean of y one has to differentiate y with respect to V and get the
d (f ( y ) )
d( y) = MV
The mean of Y=MV
Question 2
Que. A
> # the expected value of a binomial distribution is given by np
> #n is the number of observations
> #p is the number of success
> #p=6/10
> #n=10
> #let expected value be represented by M
> p<-6/10
> n<-10
> M<-p*n
> M
Question 1
A random variable V has a density fv(v) =Kv2 exp(-b/v2 )
a) What is the distribution of a new random variable, Y=1/2 mV2
The first step will entail differentiation of the function fv(v) =Kv2 exp(-b/v2 ) w.r.t V
d (f ( v ))
d(v ) =2KV* exp (-b/v2) + KV2*-2e-bv-3
The distribution for the new variable is given by
F(y)= 2KV* exp (-b/v2) + KV2*-2e-bv-3
b) in order to get the mean of y one has to differentiate y with respect to V and get the
d (f ( y ) )
d( y) = MV
The mean of Y=MV
Question 2
Que. A
> # the expected value of a binomial distribution is given by np
> #n is the number of observations
> #p is the number of success
> #p=6/10
> #n=10
> #let expected value be represented by M
> p<-6/10
> n<-10
> M<-p*n
> M
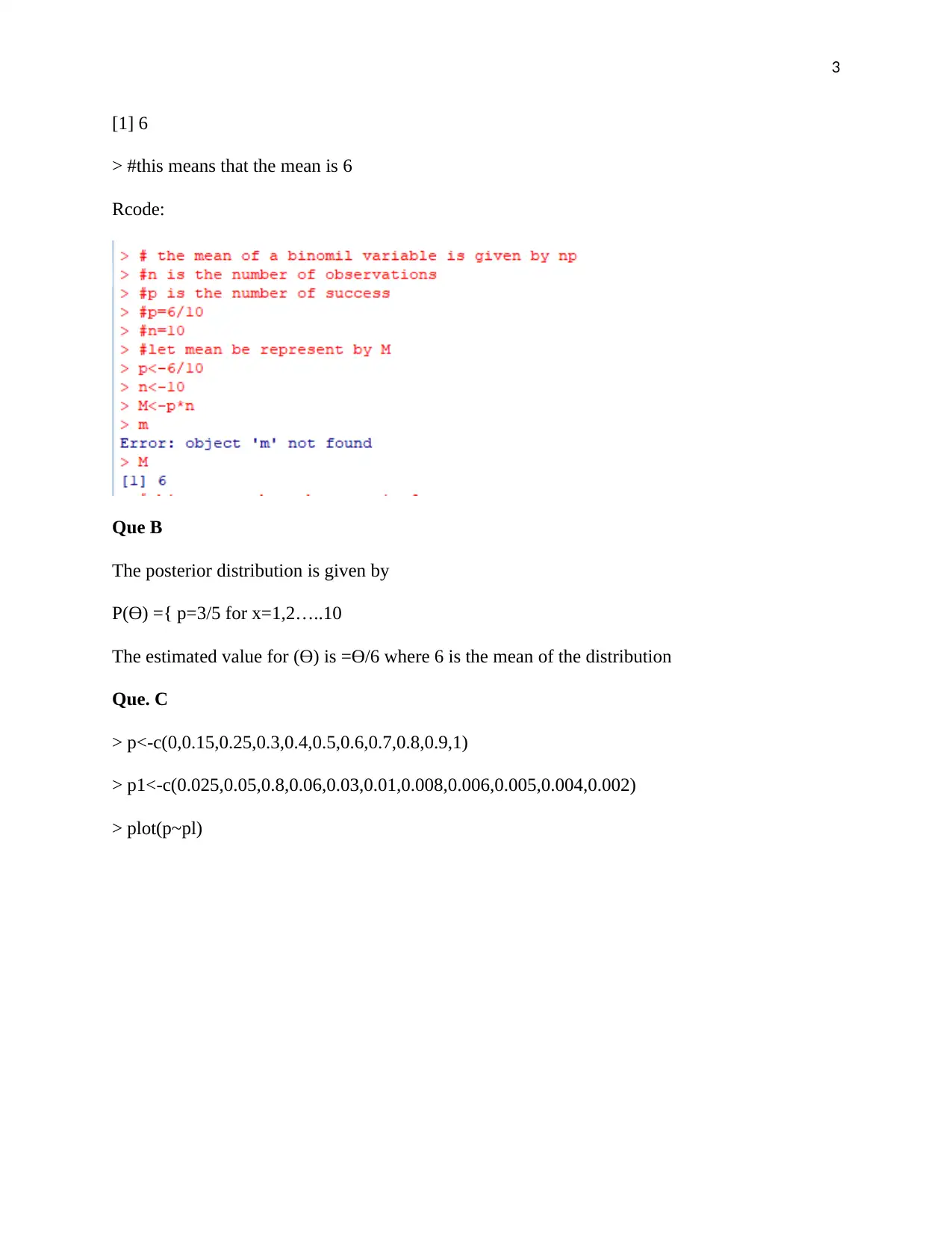
3
[1] 6
> #this means that the mean is 6
Rcode:
Que B
The posterior distribution is given by
P(Ɵ) ={ p=3/5 for x=1,2…..10
The estimated value for (Ɵ) is =Ɵ/6 where 6 is the mean of the distribution
Que. C
> p<-c(0,0.15,0.25,0.3,0.4,0.5,0.6,0.7,0.8,0.9,1)
> p1<-c(0.025,0.05,0.8,0.06,0.03,0.01,0.008,0.006,0.005,0.004,0.002)
> plot(p~pl)
[1] 6
> #this means that the mean is 6
Rcode:
Que B
The posterior distribution is given by
P(Ɵ) ={ p=3/5 for x=1,2…..10
The estimated value for (Ɵ) is =Ɵ/6 where 6 is the mean of the distribution
Que. C
> p<-c(0,0.15,0.25,0.3,0.4,0.5,0.6,0.7,0.8,0.9,1)
> p1<-c(0.025,0.05,0.8,0.06,0.03,0.01,0.008,0.006,0.005,0.004,0.002)
> plot(p~pl)
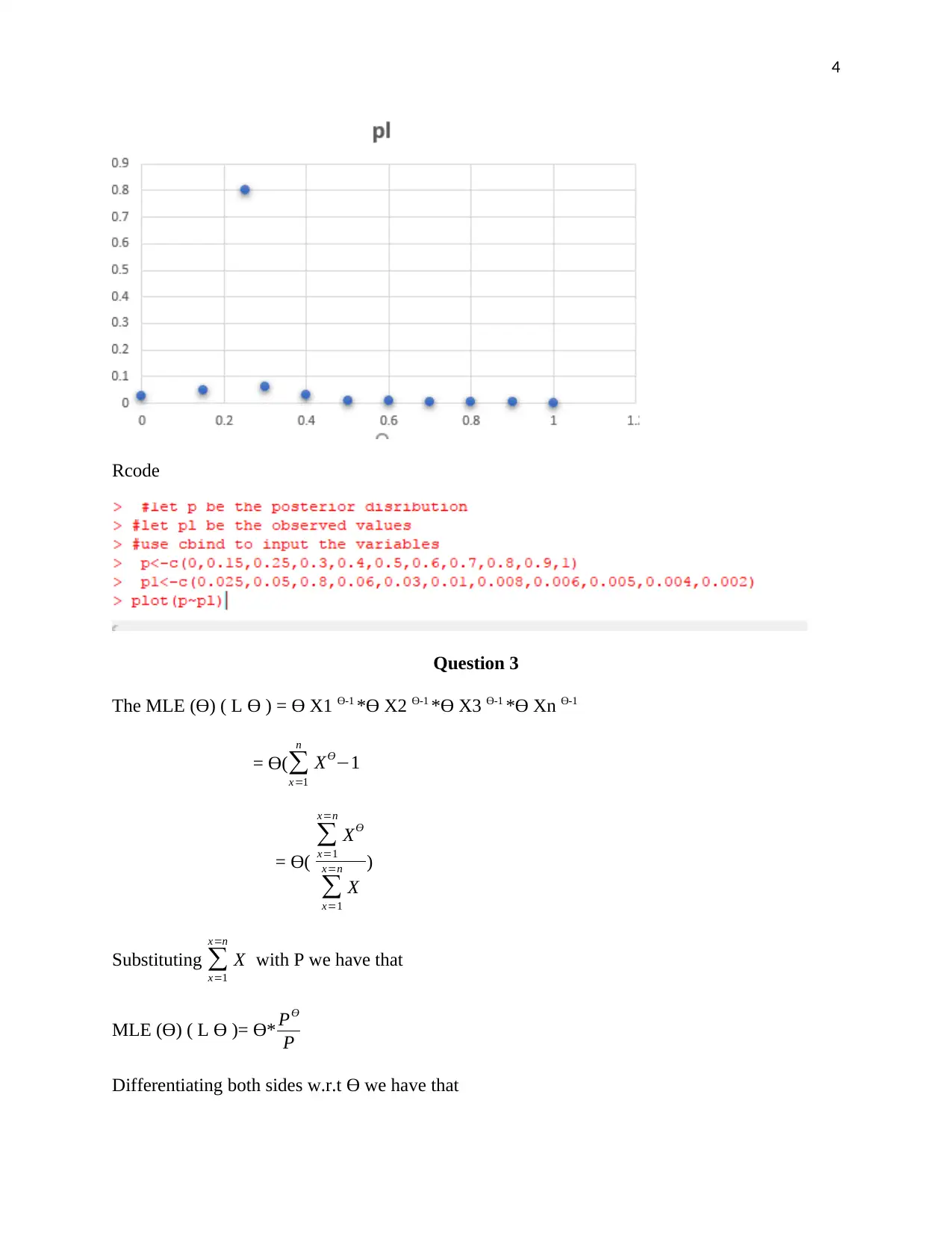
4
Rcode
Question 3
The MLE (Ɵ) ( L Ɵ ) = Ɵ X1 Ɵ-1 *Ɵ X2 Ɵ-1 *Ɵ X3 Ɵ-1 *Ɵ Xn Ɵ-1
= Ɵ(∑
x=1
n
XƟ−1
= Ɵ(
∑
x=1
x=n
XƟ
∑
x=1
x=n
X
)
Substituting ∑
x=1
x=n
X with P we have that
MLE (Ɵ) ( L Ɵ )= Ɵ* PƟ
P
Differentiating both sides w.r.t Ɵ we have that
Rcode
Question 3
The MLE (Ɵ) ( L Ɵ ) = Ɵ X1 Ɵ-1 *Ɵ X2 Ɵ-1 *Ɵ X3 Ɵ-1 *Ɵ Xn Ɵ-1
= Ɵ(∑
x=1
n
XƟ−1
= Ɵ(
∑
x=1
x=n
XƟ
∑
x=1
x=n
X
)
Substituting ∑
x=1
x=n
X with P we have that
MLE (Ɵ) ( L Ɵ )= Ɵ* PƟ
P
Differentiating both sides w.r.t Ɵ we have that
Secure Best Marks with AI Grader
Need help grading? Try our AI Grader for instant feedback on your assignments.
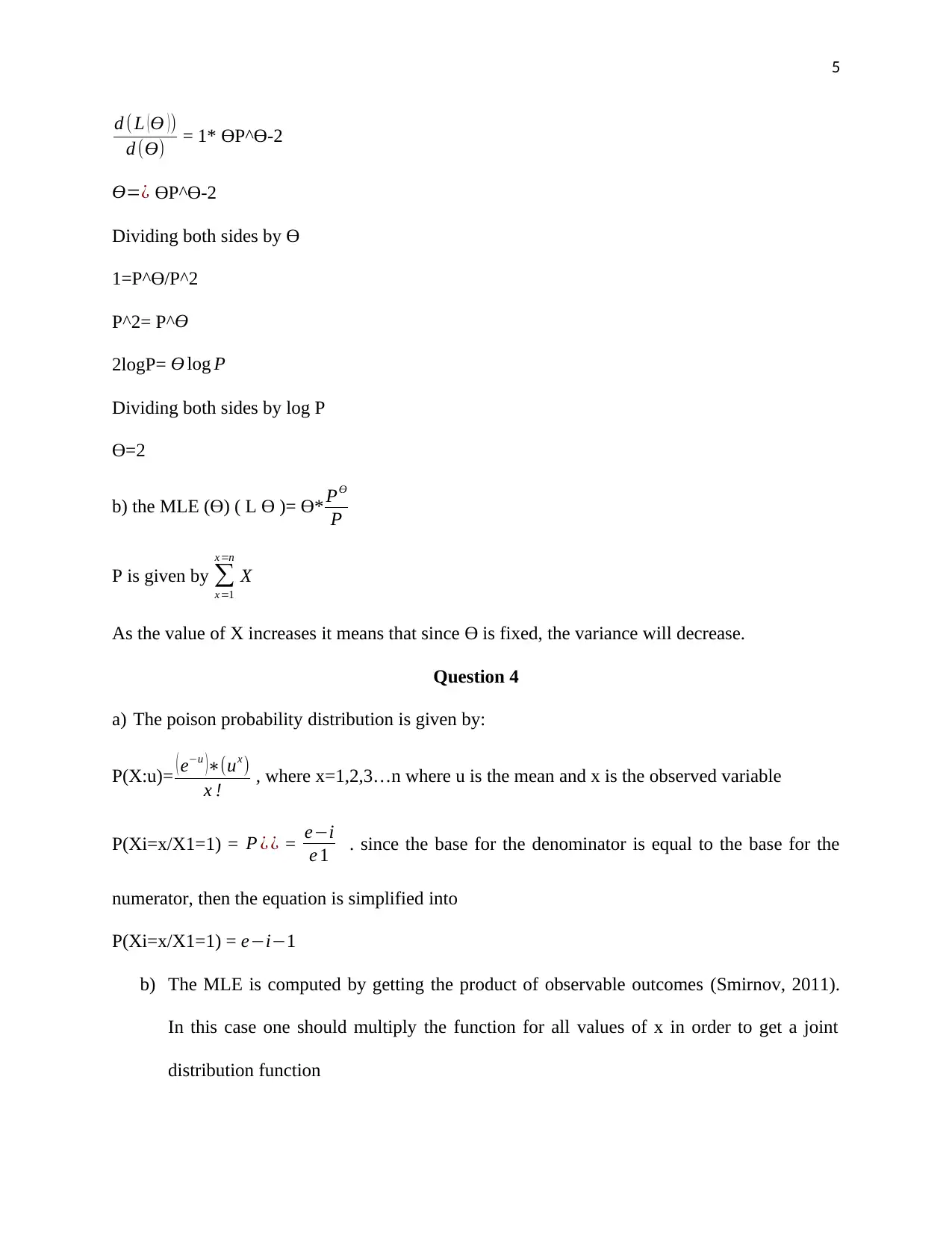
5
d (L (Ɵ ))
d (Ɵ) = 1* ƟP^Ɵ-2
Ɵ=¿ ƟP^Ɵ-2
Dividing both sides by Ɵ
1=P^Ɵ/P^2
P^2= P^Ɵ
2logP= Ɵ log P
Dividing both sides by log P
Ɵ=2
b) the MLE (Ɵ) ( L Ɵ )= Ɵ* PƟ
P
P is given by ∑
x=1
x=n
X
As the value of X increases it means that since Ɵ is fixed, the variance will decrease.
Question 4
a) The poison probability distribution is given by:
P(X:u)= ( e−u )∗(ux)
x ! , where x=1,2,3…n where u is the mean and x is the observed variable
P(Xi=x/X1=1) = P ¿ ¿ = e−i
e 1 . since the base for the denominator is equal to the base for the
numerator, then the equation is simplified into
P(Xi=x/X1=1) = e−i−1
b) The MLE is computed by getting the product of observable outcomes (Smirnov, 2011).
In this case one should multiply the function for all values of x in order to get a joint
distribution function
d (L (Ɵ ))
d (Ɵ) = 1* ƟP^Ɵ-2
Ɵ=¿ ƟP^Ɵ-2
Dividing both sides by Ɵ
1=P^Ɵ/P^2
P^2= P^Ɵ
2logP= Ɵ log P
Dividing both sides by log P
Ɵ=2
b) the MLE (Ɵ) ( L Ɵ )= Ɵ* PƟ
P
P is given by ∑
x=1
x=n
X
As the value of X increases it means that since Ɵ is fixed, the variance will decrease.
Question 4
a) The poison probability distribution is given by:
P(X:u)= ( e−u )∗(ux)
x ! , where x=1,2,3…n where u is the mean and x is the observed variable
P(Xi=x/X1=1) = P ¿ ¿ = e−i
e 1 . since the base for the denominator is equal to the base for the
numerator, then the equation is simplified into
P(Xi=x/X1=1) = e−i−1
b) The MLE is computed by getting the product of observable outcomes (Smirnov, 2011).
In this case one should multiply the function for all values of x in order to get a joint
distribution function
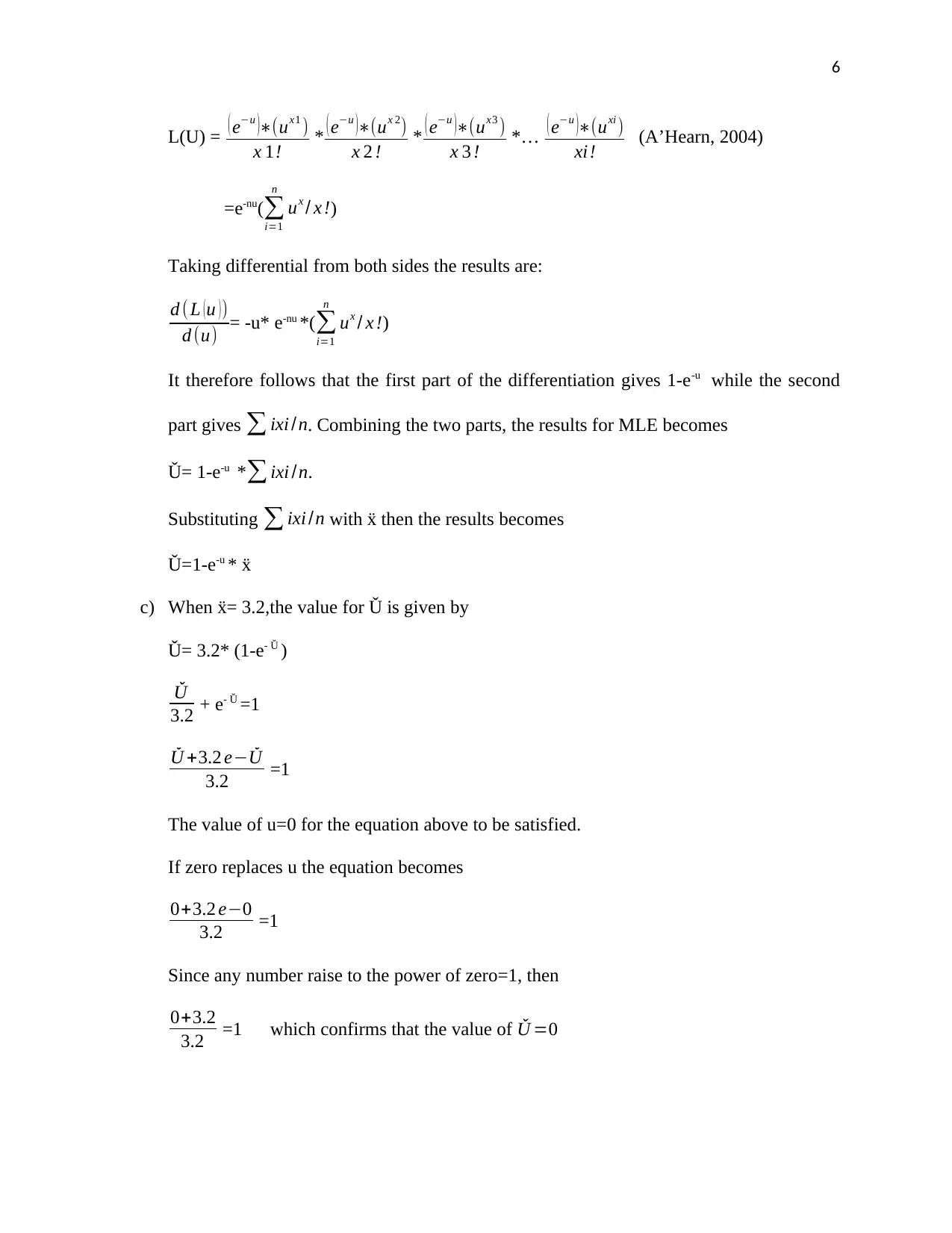
6
L(U) = ( e−u )∗(ux1 )
x 1! * ( e−u )∗(ux 2)
x 2 ! * ( e−u )∗(ux3 )
x 3 ! *… ( e−u )∗(uxi )
xi! (A’Hearn, 2004)
=e-nu(∑
i=1
n
ux / x !)
Taking differential from both sides the results are:
d (L (u ))
d (u) = -u* e-nu *(∑
i=1
n
ux / x !)
It therefore follows that the first part of the differentiation gives 1-e-u while the second
part gives ∑ ixi /n. Combining the two parts, the results for MLE becomes
Ǔ= 1-e-u *∑ ixi /n.
Substituting ∑ ixi /n with ẍ then the results becomes
Ǔ=1-e-u * ẍ
c) When ẍ= 3.2,the value for Ǔ is given by
Ǔ= 3.2* (1-e- Ǔ )
Ǔ
3.2 + e- Ǔ =1
Ǔ +3.2 e−Ǔ
3.2 =1
The value of u=0 for the equation above to be satisfied.
If zero replaces u the equation becomes
0+3.2 e−0
3.2 =1
Since any number raise to the power of zero=1, then
0+3.2
3.2 =1 which confirms that the value of Ǔ =0
L(U) = ( e−u )∗(ux1 )
x 1! * ( e−u )∗(ux 2)
x 2 ! * ( e−u )∗(ux3 )
x 3 ! *… ( e−u )∗(uxi )
xi! (A’Hearn, 2004)
=e-nu(∑
i=1
n
ux / x !)
Taking differential from both sides the results are:
d (L (u ))
d (u) = -u* e-nu *(∑
i=1
n
ux / x !)
It therefore follows that the first part of the differentiation gives 1-e-u while the second
part gives ∑ ixi /n. Combining the two parts, the results for MLE becomes
Ǔ= 1-e-u *∑ ixi /n.
Substituting ∑ ixi /n with ẍ then the results becomes
Ǔ=1-e-u * ẍ
c) When ẍ= 3.2,the value for Ǔ is given by
Ǔ= 3.2* (1-e- Ǔ )
Ǔ
3.2 + e- Ǔ =1
Ǔ +3.2 e−Ǔ
3.2 =1
The value of u=0 for the equation above to be satisfied.
If zero replaces u the equation becomes
0+3.2 e−0
3.2 =1
Since any number raise to the power of zero=1, then
0+3.2
3.2 =1 which confirms that the value of Ǔ =0
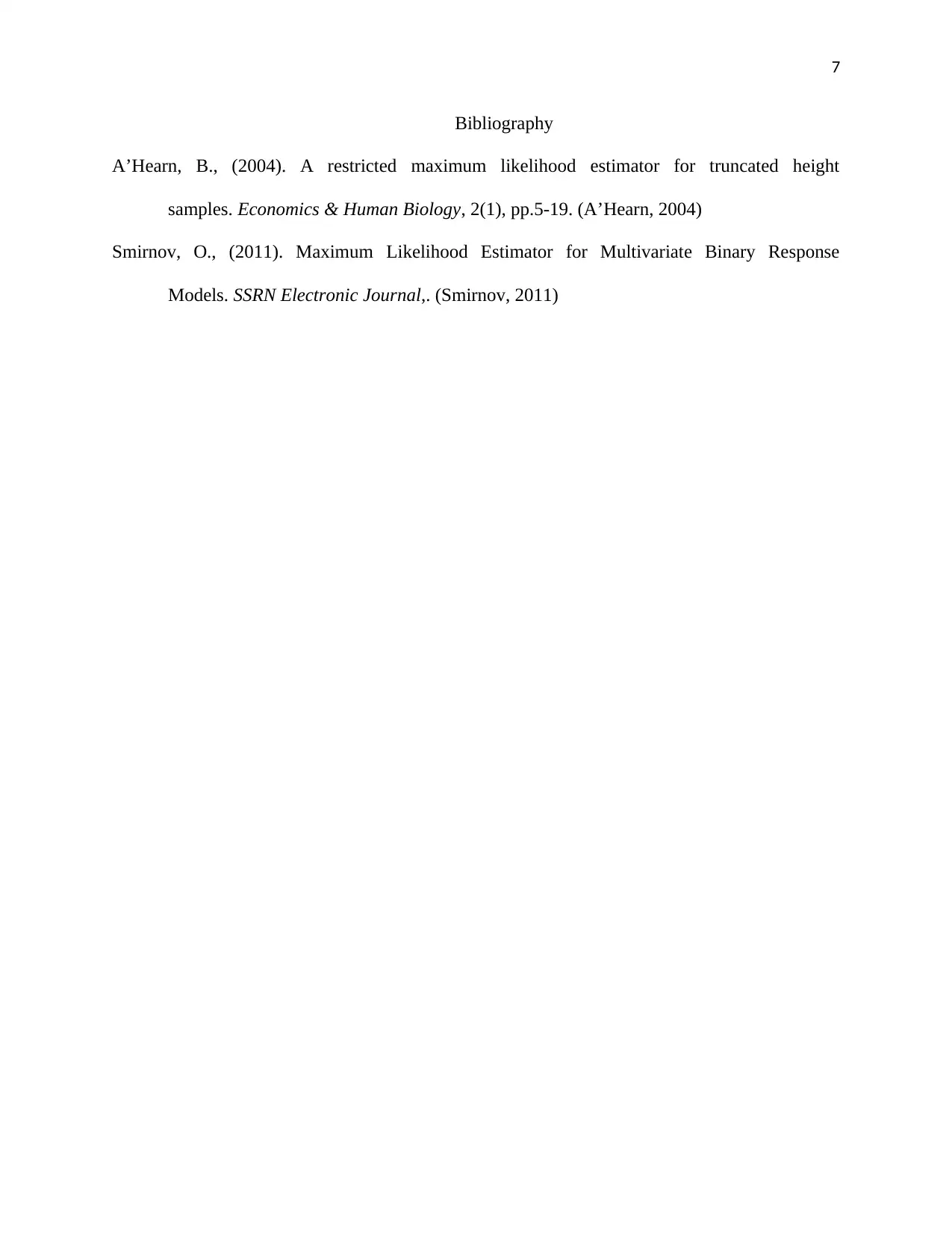
7
Bibliography
A’Hearn, B., (2004). A restricted maximum likelihood estimator for truncated height
samples. Economics & Human Biology, 2(1), pp.5-19. (A’Hearn, 2004)
Smirnov, O., (2011). Maximum Likelihood Estimator for Multivariate Binary Response
Models. SSRN Electronic Journal,. (Smirnov, 2011)
Bibliography
A’Hearn, B., (2004). A restricted maximum likelihood estimator for truncated height
samples. Economics & Human Biology, 2(1), pp.5-19. (A’Hearn, 2004)
Smirnov, O., (2011). Maximum Likelihood Estimator for Multivariate Binary Response
Models. SSRN Electronic Journal,. (Smirnov, 2011)
1 out of 7
Related Documents

Your All-in-One AI-Powered Toolkit for Academic Success.
+13062052269
info@desklib.com
Available 24*7 on WhatsApp / Email
Unlock your academic potential
© 2024 | Zucol Services PVT LTD | All rights reserved.