Accounting and Decision Support Tools
VerifiedAdded on 2023/04/08
|11
|1512
|308
AI Summary
This document discusses various topics related to accounting and decision support tools, including expected value, sales of loaves, four machines, control limits, and hypothesis testing. It provides explanations, calculations, and probabilities for each topic.
Contribute Materials
Your contribution can guide someone’s learning journey. Share your
documents today.
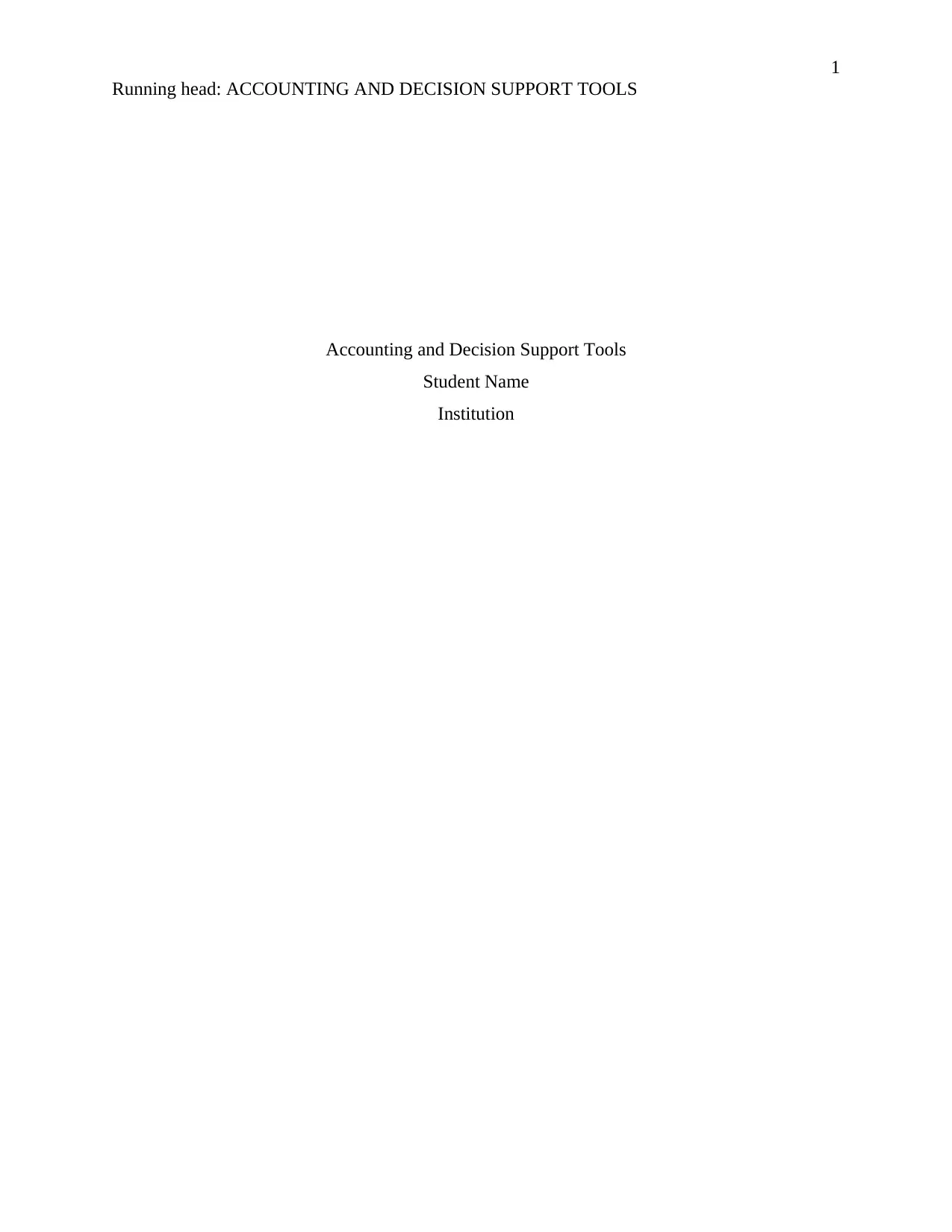
1
Running head: ACCOUNTING AND DECISION SUPPORT TOOLS
Accounting and Decision Support Tools
Student Name
Institution
Running head: ACCOUNTING AND DECISION SUPPORT TOOLS
Accounting and Decision Support Tools
Student Name
Institution
Secure Best Marks with AI Grader
Need help grading? Try our AI Grader for instant feedback on your assignments.
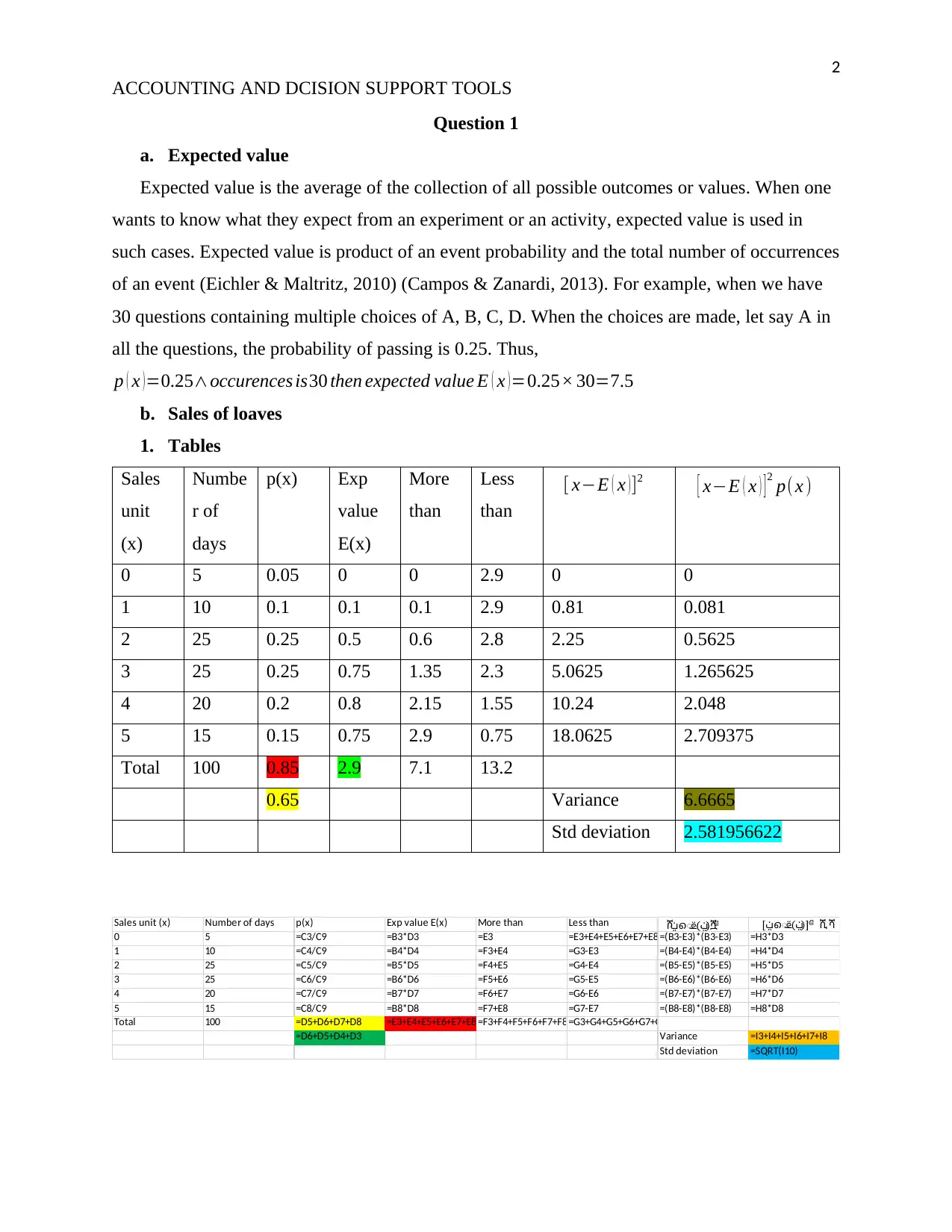
2
ACCOUNTING AND DCISION SUPPORT TOOLS
Question 1
a. Expected value
Expected value is the average of the collection of all possible outcomes or values. When one
wants to know what they expect from an experiment or an activity, expected value is used in
such cases. Expected value is product of an event probability and the total number of occurrences
of an event (Eichler & Maltritz, 2010) (Campos & Zanardi, 2013). For example, when we have
30 questions containing multiple choices of A, B, C, D. When the choices are made, let say A in
all the questions, the probability of passing is 0.25. Thus,
p ( x )=0.25∧occurences is30 then expected value E ( x )=0.25× 30=7.5
b. Sales of loaves
1. Tables
Sales
unit
(x)
Numbe
r of
days
p(x) Exp
value
E(x)
More
than
Less
than
0 5 0.05 0 0 2.9 0 0
1 10 0.1 0.1 0.1 2.9 0.81 0.081
2 25 0.25 0.5 0.6 2.8 2.25 0.5625
3 25 0.25 0.75 1.35 2.3 5.0625 1.265625
4 20 0.2 0.8 2.15 1.55 10.24 2.048
5 15 0.15 0.75 2.9 0.75 18.0625 2.709375
Total 100 0.85 2.9 7.1 13.2
0.65 Variance 6.6665
Std deviation 2.581956622
Sales unit (x) Number of days p(x) Exp value E(x) More than Less than
0 5 =C3/C9 =B3*D3 =E3 =E3+E4+E5+E6+E7+E8 =(B3-E3)*(B3-E3) =H3*D3
1 10 =C4/C9 =B4*D4 =F3+E4 =G3-E3 =(B4-E4)*(B4-E4) =H4*D4
2 25 =C5/C9 =B5*D5 =F4+E5 =G4-E4 =(B5-E5)*(B5-E5) =H5*D5
3 25 =C6/C9 =B6*D6 =F5+E6 =G5-E5 =(B6-E6)*(B6-E6) =H6*D6
4 20 =C7/C9 =B7*D7 =F6+E7 =G6-E6 =(B7-E7)*(B7-E7) =H7*D7
5 15 =C8/C9 =B8*D8 =F7+E8 =G7-E7 =(B8-E8)*(B8-E8) =H8*D8
Total 100 =D5+D6+D7+D8 =E3+E4+E5+E6+E7+E8 =F3+F4+F5+F6+F7+F8 =G3+G4+G5+G6+G7+G8
=D6+D5+D4+D3 Variance =I3+I4+I5+I6+I7+I8
Std deviation =SQRT(I10)
ሾݔെܧ ݔ ሿଶ ݔെܧ ݔ ଶ ሺ ሻ
[ x−E ( x ) ]2
[ x−E ( x ) ]2
p(x )
ACCOUNTING AND DCISION SUPPORT TOOLS
Question 1
a. Expected value
Expected value is the average of the collection of all possible outcomes or values. When one
wants to know what they expect from an experiment or an activity, expected value is used in
such cases. Expected value is product of an event probability and the total number of occurrences
of an event (Eichler & Maltritz, 2010) (Campos & Zanardi, 2013). For example, when we have
30 questions containing multiple choices of A, B, C, D. When the choices are made, let say A in
all the questions, the probability of passing is 0.25. Thus,
p ( x )=0.25∧occurences is30 then expected value E ( x )=0.25× 30=7.5
b. Sales of loaves
1. Tables
Sales
unit
(x)
Numbe
r of
days
p(x) Exp
value
E(x)
More
than
Less
than
0 5 0.05 0 0 2.9 0 0
1 10 0.1 0.1 0.1 2.9 0.81 0.081
2 25 0.25 0.5 0.6 2.8 2.25 0.5625
3 25 0.25 0.75 1.35 2.3 5.0625 1.265625
4 20 0.2 0.8 2.15 1.55 10.24 2.048
5 15 0.15 0.75 2.9 0.75 18.0625 2.709375
Total 100 0.85 2.9 7.1 13.2
0.65 Variance 6.6665
Std deviation 2.581956622
Sales unit (x) Number of days p(x) Exp value E(x) More than Less than
0 5 =C3/C9 =B3*D3 =E3 =E3+E4+E5+E6+E7+E8 =(B3-E3)*(B3-E3) =H3*D3
1 10 =C4/C9 =B4*D4 =F3+E4 =G3-E3 =(B4-E4)*(B4-E4) =H4*D4
2 25 =C5/C9 =B5*D5 =F4+E5 =G4-E4 =(B5-E5)*(B5-E5) =H5*D5
3 25 =C6/C9 =B6*D6 =F5+E6 =G5-E5 =(B6-E6)*(B6-E6) =H6*D6
4 20 =C7/C9 =B7*D7 =F6+E7 =G6-E6 =(B7-E7)*(B7-E7) =H7*D7
5 15 =C8/C9 =B8*D8 =F7+E8 =G7-E7 =(B8-E8)*(B8-E8) =H8*D8
Total 100 =D5+D6+D7+D8 =E3+E4+E5+E6+E7+E8 =F3+F4+F5+F6+F7+F8 =G3+G4+G5+G6+G7+G8
=D6+D5+D4+D3 Variance =I3+I4+I5+I6+I7+I8
Std deviation =SQRT(I10)
ሾݔെܧ ݔ ሿଶ ݔെܧ ݔ ଶ ሺ ሻ
[ x−E ( x ) ]2
[ x−E ( x ) ]2
p(x )
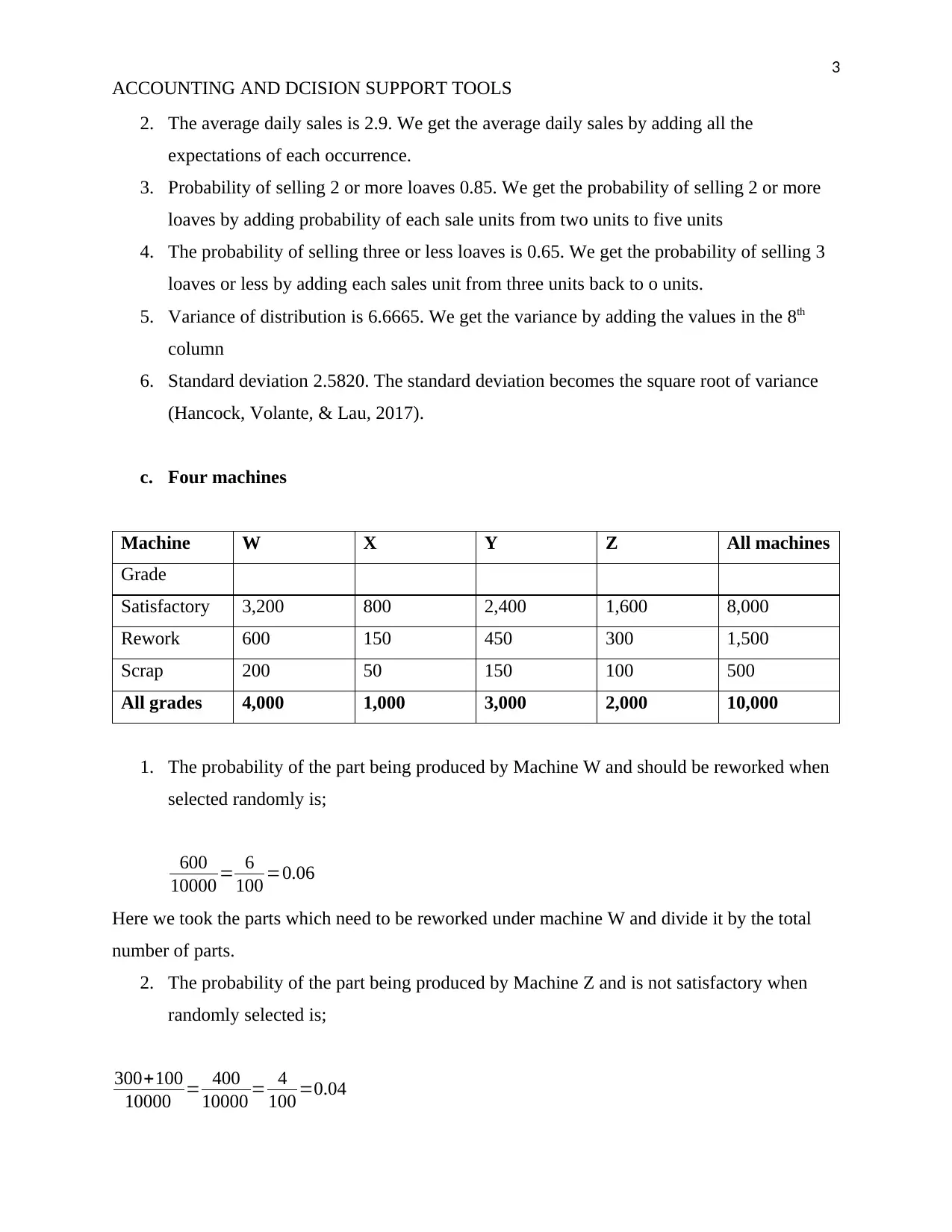
3
ACCOUNTING AND DCISION SUPPORT TOOLS
2. The average daily sales is 2.9. We get the average daily sales by adding all the
expectations of each occurrence.
3. Probability of selling 2 or more loaves 0.85. We get the probability of selling 2 or more
loaves by adding probability of each sale units from two units to five units
4. The probability of selling three or less loaves is 0.65. We get the probability of selling 3
loaves or less by adding each sales unit from three units back to o units.
5. Variance of distribution is 6.6665. We get the variance by adding the values in the 8th
column
6. Standard deviation 2.5820. The standard deviation becomes the square root of variance
(Hancock, Volante, & Lau, 2017).
c. Four machines
Machine W X Y Z All machines
Grade
Satisfactory 3,200 800 2,400 1,600 8,000
Rework 600 150 450 300 1,500
Scrap 200 50 150 100 500
All grades 4,000 1,000 3,000 2,000 10,000
1. The probability of the part being produced by Machine W and should be reworked when
selected randomly is;
600
10000 = 6
100 =0.06
Here we took the parts which need to be reworked under machine W and divide it by the total
number of parts.
2. The probability of the part being produced by Machine Z and is not satisfactory when
randomly selected is;
300+100
10000 = 400
10000 = 4
100 =0.04
ACCOUNTING AND DCISION SUPPORT TOOLS
2. The average daily sales is 2.9. We get the average daily sales by adding all the
expectations of each occurrence.
3. Probability of selling 2 or more loaves 0.85. We get the probability of selling 2 or more
loaves by adding probability of each sale units from two units to five units
4. The probability of selling three or less loaves is 0.65. We get the probability of selling 3
loaves or less by adding each sales unit from three units back to o units.
5. Variance of distribution is 6.6665. We get the variance by adding the values in the 8th
column
6. Standard deviation 2.5820. The standard deviation becomes the square root of variance
(Hancock, Volante, & Lau, 2017).
c. Four machines
Machine W X Y Z All machines
Grade
Satisfactory 3,200 800 2,400 1,600 8,000
Rework 600 150 450 300 1,500
Scrap 200 50 150 100 500
All grades 4,000 1,000 3,000 2,000 10,000
1. The probability of the part being produced by Machine W and should be reworked when
selected randomly is;
600
10000 = 6
100 =0.06
Here we took the parts which need to be reworked under machine W and divide it by the total
number of parts.
2. The probability of the part being produced by Machine Z and is not satisfactory when
randomly selected is;
300+100
10000 = 400
10000 = 4
100 =0.04
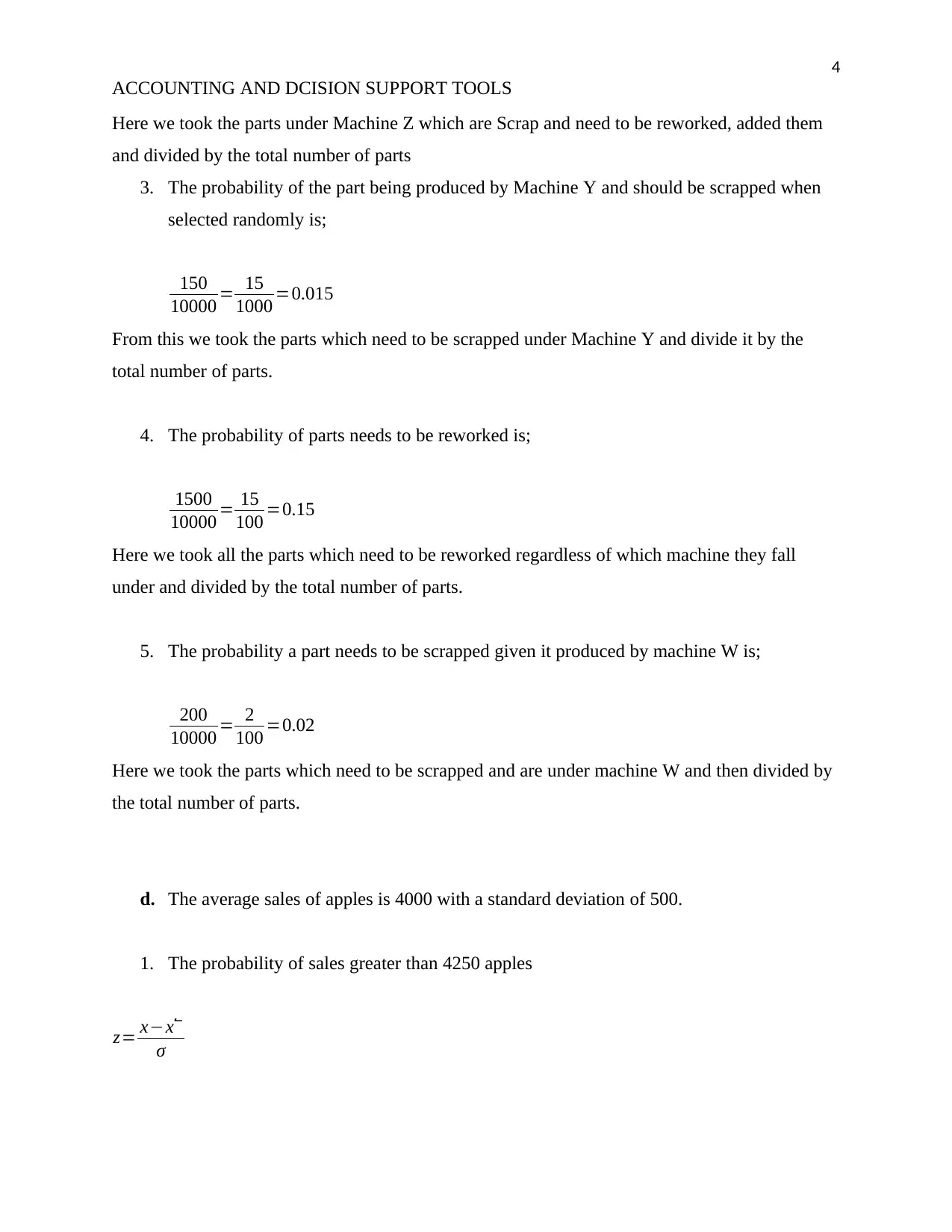
4
ACCOUNTING AND DCISION SUPPORT TOOLS
Here we took the parts under Machine Z which are Scrap and need to be reworked, added them
and divided by the total number of parts
3. The probability of the part being produced by Machine Y and should be scrapped when
selected randomly is;
150
10000 = 15
1000 =0.015
From this we took the parts which need to be scrapped under Machine Y and divide it by the
total number of parts.
4. The probability of parts needs to be reworked is;
1500
10000 = 15
100 =0.15
Here we took all the parts which need to be reworked regardless of which machine they fall
under and divided by the total number of parts.
5. The probability a part needs to be scrapped given it produced by machine W is;
200
10000 = 2
100 =0.02
Here we took the parts which need to be scrapped and are under machine W and then divided by
the total number of parts.
d. The average sales of apples is 4000 with a standard deviation of 500.
1. The probability of sales greater than 4250 apples
z= x−x՟
σ
ACCOUNTING AND DCISION SUPPORT TOOLS
Here we took the parts under Machine Z which are Scrap and need to be reworked, added them
and divided by the total number of parts
3. The probability of the part being produced by Machine Y and should be scrapped when
selected randomly is;
150
10000 = 15
1000 =0.015
From this we took the parts which need to be scrapped under Machine Y and divide it by the
total number of parts.
4. The probability of parts needs to be reworked is;
1500
10000 = 15
100 =0.15
Here we took all the parts which need to be reworked regardless of which machine they fall
under and divided by the total number of parts.
5. The probability a part needs to be scrapped given it produced by machine W is;
200
10000 = 2
100 =0.02
Here we took the parts which need to be scrapped and are under machine W and then divided by
the total number of parts.
d. The average sales of apples is 4000 with a standard deviation of 500.
1. The probability of sales greater than 4250 apples
z= x−x՟
σ
Secure Best Marks with AI Grader
Need help grading? Try our AI Grader for instant feedback on your assignments.
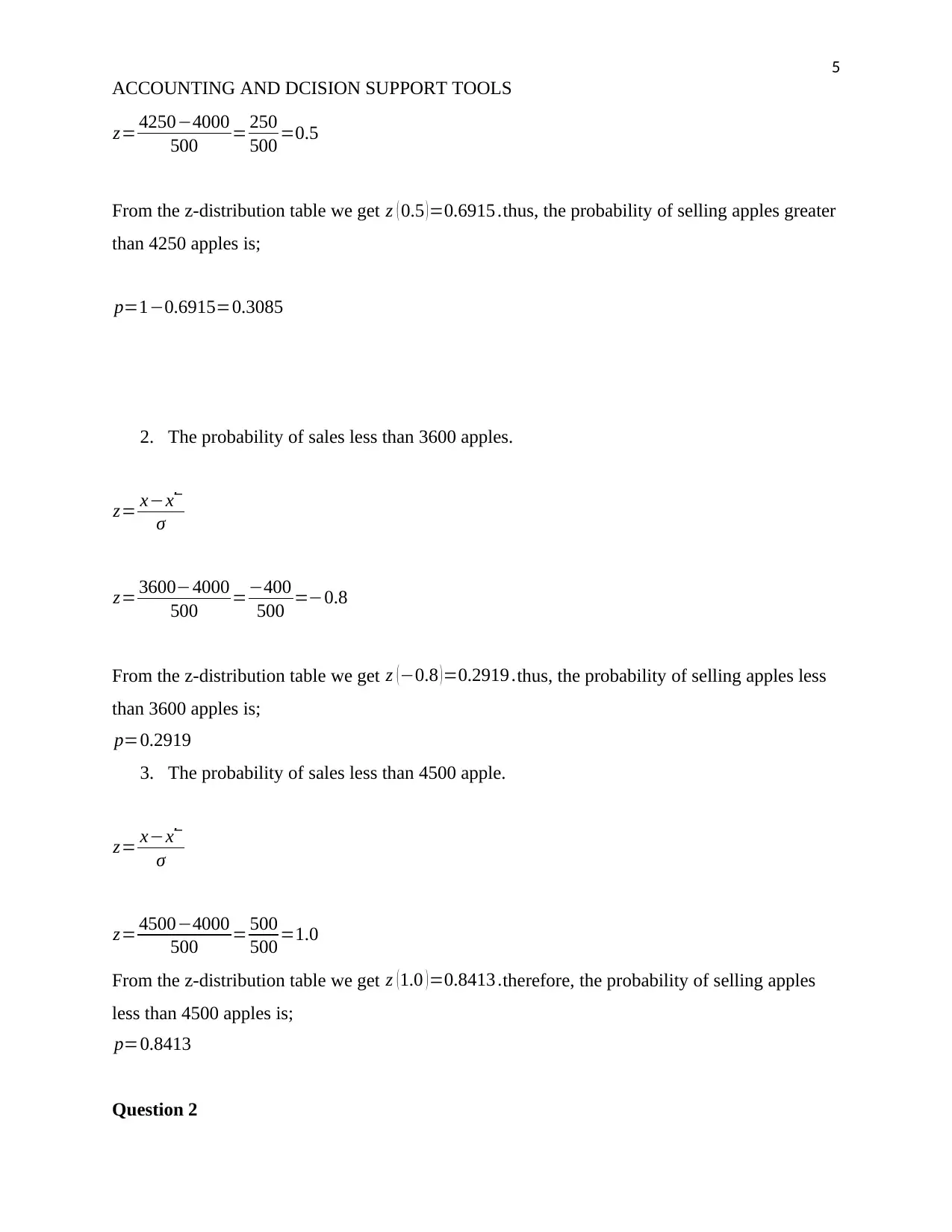
5
ACCOUNTING AND DCISION SUPPORT TOOLS
z= 4250−4000
500 = 250
500 =0.5
From the z-distribution table we get z ( 0.5 )=0.6915 .thus, the probability of selling apples greater
than 4250 apples is;
p=1−0.6915=0.3085
2. The probability of sales less than 3600 apples.
z= x−x՟
σ
z= 3600−4000
500 =−400
500 =−0.8
From the z-distribution table we get z (−0.8 )=0.2919 .thus, the probability of selling apples less
than 3600 apples is;
p=0.2919
3. The probability of sales less than 4500 apple.
z= x−x՟
σ
z= 4500−4000
500 = 500
500 =1.0
From the z-distribution table we get z ( 1.0 ) =0.8413 .therefore, the probability of selling apples
less than 4500 apples is;
p=0.8413
Question 2
ACCOUNTING AND DCISION SUPPORT TOOLS
z= 4250−4000
500 = 250
500 =0.5
From the z-distribution table we get z ( 0.5 )=0.6915 .thus, the probability of selling apples greater
than 4250 apples is;
p=1−0.6915=0.3085
2. The probability of sales less than 3600 apples.
z= x−x՟
σ
z= 3600−4000
500 =−400
500 =−0.8
From the z-distribution table we get z (−0.8 )=0.2919 .thus, the probability of selling apples less
than 3600 apples is;
p=0.2919
3. The probability of sales less than 4500 apple.
z= x−x՟
σ
z= 4500−4000
500 = 500
500 =1.0
From the z-distribution table we get z ( 1.0 ) =0.8413 .therefore, the probability of selling apples
less than 4500 apples is;
p=0.8413
Question 2
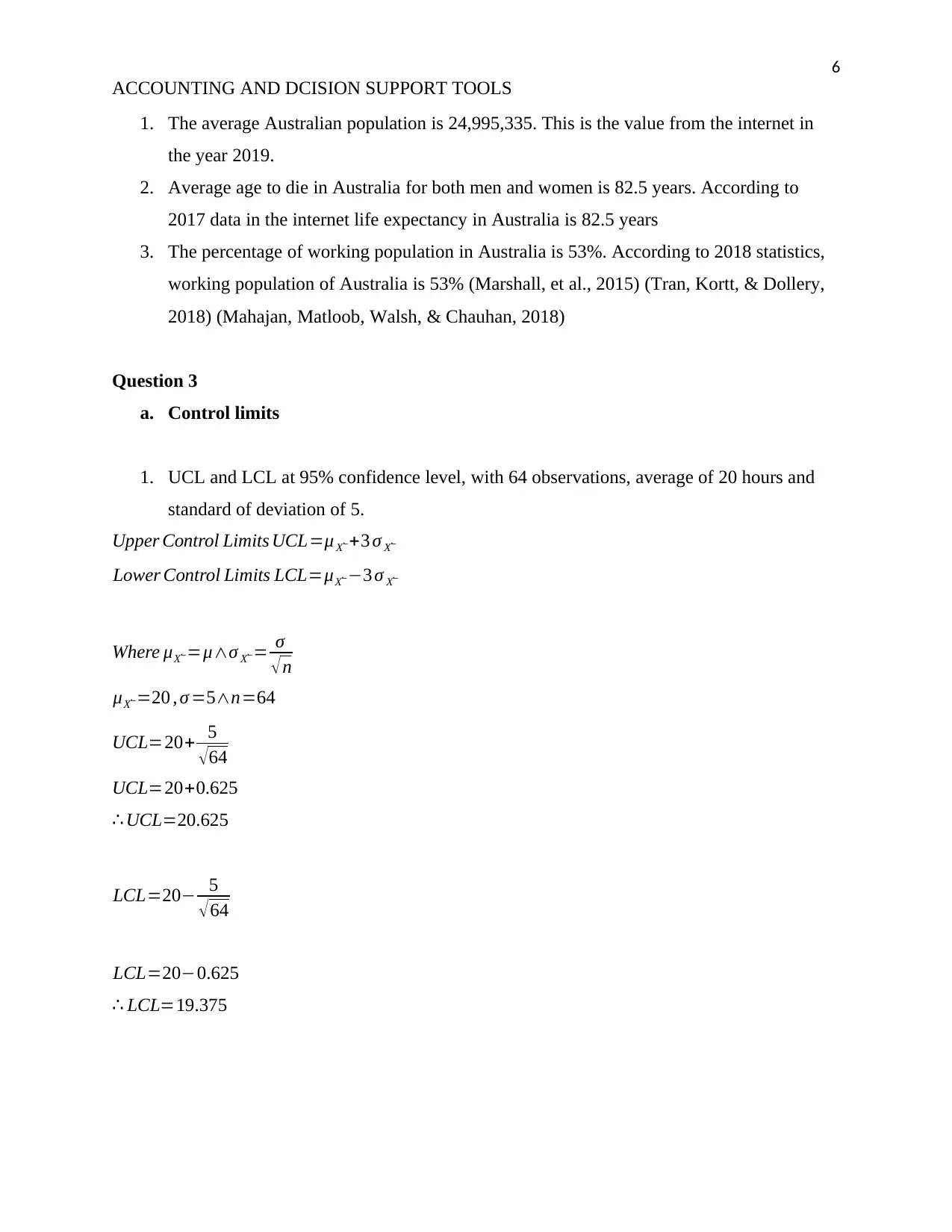
6
ACCOUNTING AND DCISION SUPPORT TOOLS
1. The average Australian population is 24,995,335. This is the value from the internet in
the year 2019.
2. Average age to die in Australia for both men and women is 82.5 years. According to
2017 data in the internet life expectancy in Australia is 82.5 years
3. The percentage of working population in Australia is 53%. According to 2018 statistics,
working population of Australia is 53% (Marshall, et al., 2015) (Tran, Kortt, & Dollery,
2018) (Mahajan, Matloob, Walsh, & Chauhan, 2018)
Question 3
a. Control limits
1. UCL and LCL at 95% confidence level, with 64 observations, average of 20 hours and
standard of deviation of 5.
Upper Control Limits UCL=μ X՟+3 σ X՟
Lower Control Limits LCL=μX՟−3 σ X՟
Where μX՟=μ∧σ X՟= σ
√ n
μX՟=20 , σ =5∧n=64
UCL=20+ 5
√64
UCL=20+0.625
∴ UCL=20.625
LCL=20− 5
√ 64
LCL=20−0.625
∴ LCL=19.375
ACCOUNTING AND DCISION SUPPORT TOOLS
1. The average Australian population is 24,995,335. This is the value from the internet in
the year 2019.
2. Average age to die in Australia for both men and women is 82.5 years. According to
2017 data in the internet life expectancy in Australia is 82.5 years
3. The percentage of working population in Australia is 53%. According to 2018 statistics,
working population of Australia is 53% (Marshall, et al., 2015) (Tran, Kortt, & Dollery,
2018) (Mahajan, Matloob, Walsh, & Chauhan, 2018)
Question 3
a. Control limits
1. UCL and LCL at 95% confidence level, with 64 observations, average of 20 hours and
standard of deviation of 5.
Upper Control Limits UCL=μ X՟+3 σ X՟
Lower Control Limits LCL=μX՟−3 σ X՟
Where μX՟=μ∧σ X՟= σ
√ n
μX՟=20 , σ =5∧n=64
UCL=20+ 5
√64
UCL=20+0.625
∴ UCL=20.625
LCL=20− 5
√ 64
LCL=20−0.625
∴ LCL=19.375
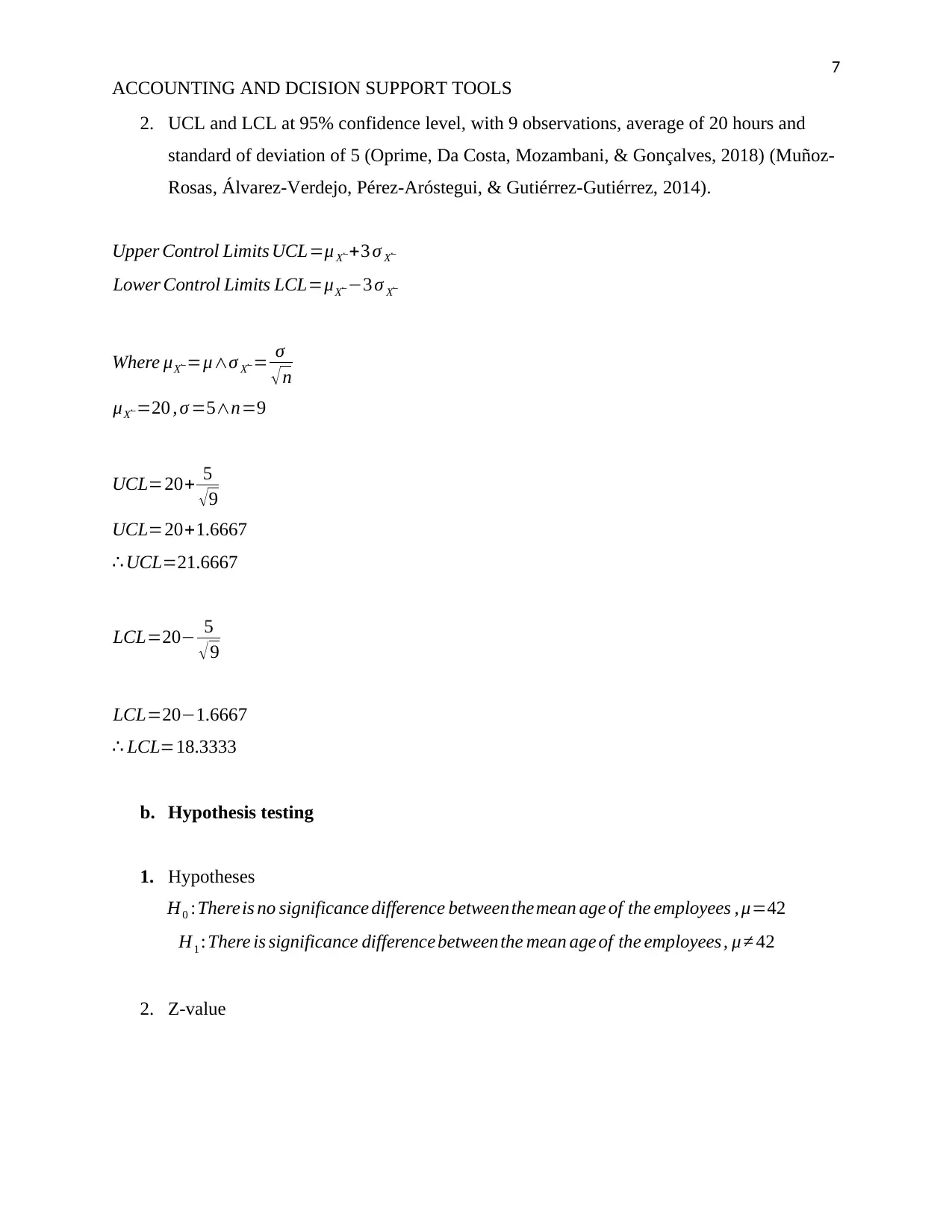
7
ACCOUNTING AND DCISION SUPPORT TOOLS
2. UCL and LCL at 95% confidence level, with 9 observations, average of 20 hours and
standard of deviation of 5 (Oprime, Da Costa, Mozambani, & Gonçalves, 2018) (Muñoz-
Rosas, Álvarez-Verdejo, Pérez-Aróstegui, & Gutiérrez-Gutiérrez, 2014).
Upper Control Limits UCL=μ X՟+3 σ X՟
Lower Control Limits LCL=μX՟−3 σ X՟
Where μX՟=μ∧σ X՟= σ
√ n
μX՟=20 , σ =5∧n=9
UCL=20+ 5
√9
UCL=20+1.6667
∴ UCL=21.6667
LCL=20− 5
√9
LCL=20−1.6667
∴ LCL=18.3333
b. Hypothesis testing
1. Hypotheses
H0 :Thereis no significance difference between themean age of the employees , μ=42
H1 :There is significance difference between the mean age of the employees , μ ≠ 42
2. Z-value
ACCOUNTING AND DCISION SUPPORT TOOLS
2. UCL and LCL at 95% confidence level, with 9 observations, average of 20 hours and
standard of deviation of 5 (Oprime, Da Costa, Mozambani, & Gonçalves, 2018) (Muñoz-
Rosas, Álvarez-Verdejo, Pérez-Aróstegui, & Gutiérrez-Gutiérrez, 2014).
Upper Control Limits UCL=μ X՟+3 σ X՟
Lower Control Limits LCL=μX՟−3 σ X՟
Where μX՟=μ∧σ X՟= σ
√ n
μX՟=20 , σ =5∧n=9
UCL=20+ 5
√9
UCL=20+1.6667
∴ UCL=21.6667
LCL=20− 5
√9
LCL=20−1.6667
∴ LCL=18.3333
b. Hypothesis testing
1. Hypotheses
H0 :Thereis no significance difference between themean age of the employees , μ=42
H1 :There is significance difference between the mean age of the employees , μ ≠ 42
2. Z-value
Paraphrase This Document
Need a fresh take? Get an instant paraphrase of this document with our AI Paraphraser
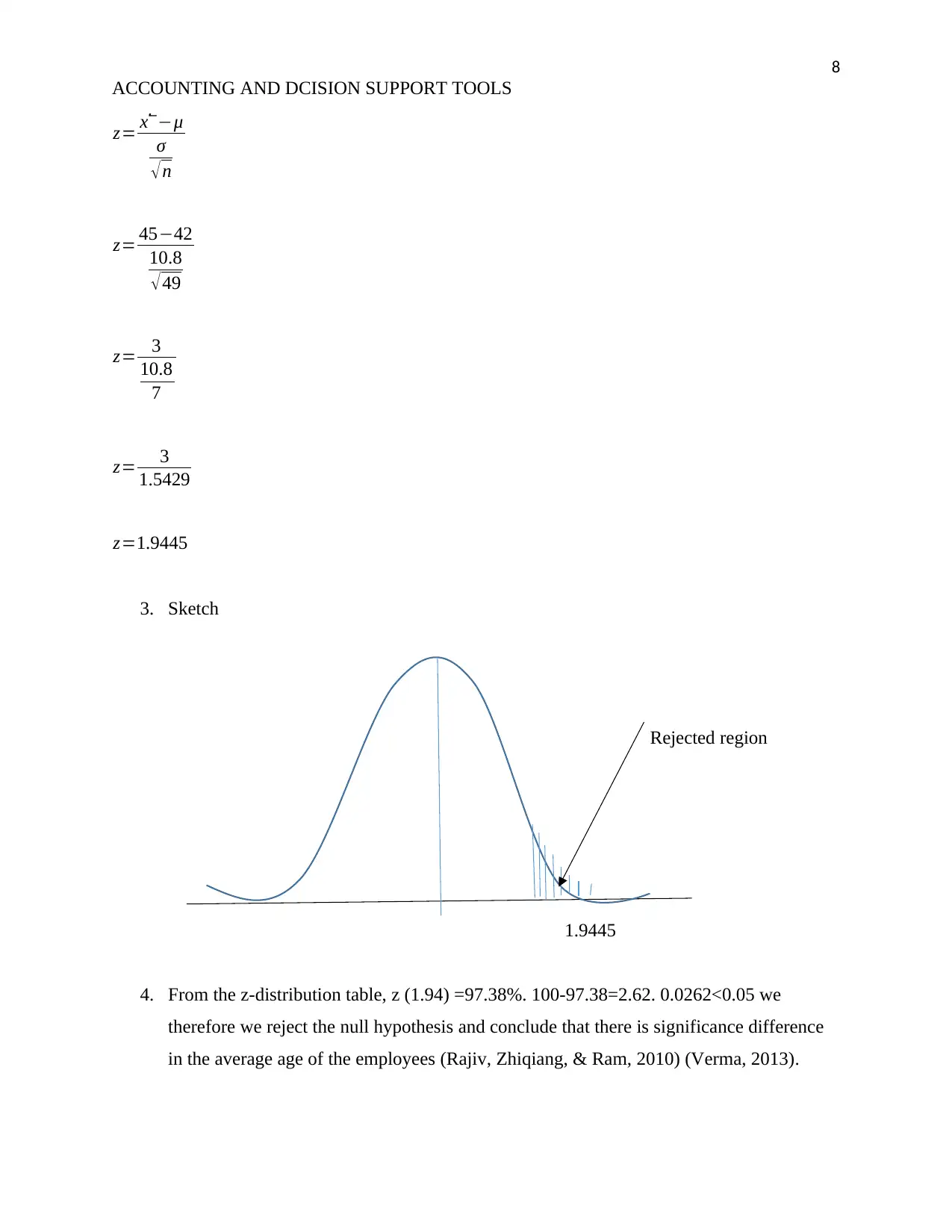
8
ACCOUNTING AND DCISION SUPPORT TOOLS
z= x՟−μ
σ
√ n
z= 45−42
10.8
√ 49
z= 3
10.8
7
z= 3
1.5429
z=1.9445
3. Sketch
Rejected region
1.9445
4. From the z-distribution table, z (1.94) =97.38%. 100-97.38=2.62. 0.0262<0.05 we
therefore we reject the null hypothesis and conclude that there is significance difference
in the average age of the employees (Rajiv, Zhiqiang, & Ram, 2010) (Verma, 2013).
ACCOUNTING AND DCISION SUPPORT TOOLS
z= x՟−μ
σ
√ n
z= 45−42
10.8
√ 49
z= 3
10.8
7
z= 3
1.5429
z=1.9445
3. Sketch
Rejected region
1.9445
4. From the z-distribution table, z (1.94) =97.38%. 100-97.38=2.62. 0.0262<0.05 we
therefore we reject the null hypothesis and conclude that there is significance difference
in the average age of the employees (Rajiv, Zhiqiang, & Ram, 2010) (Verma, 2013).
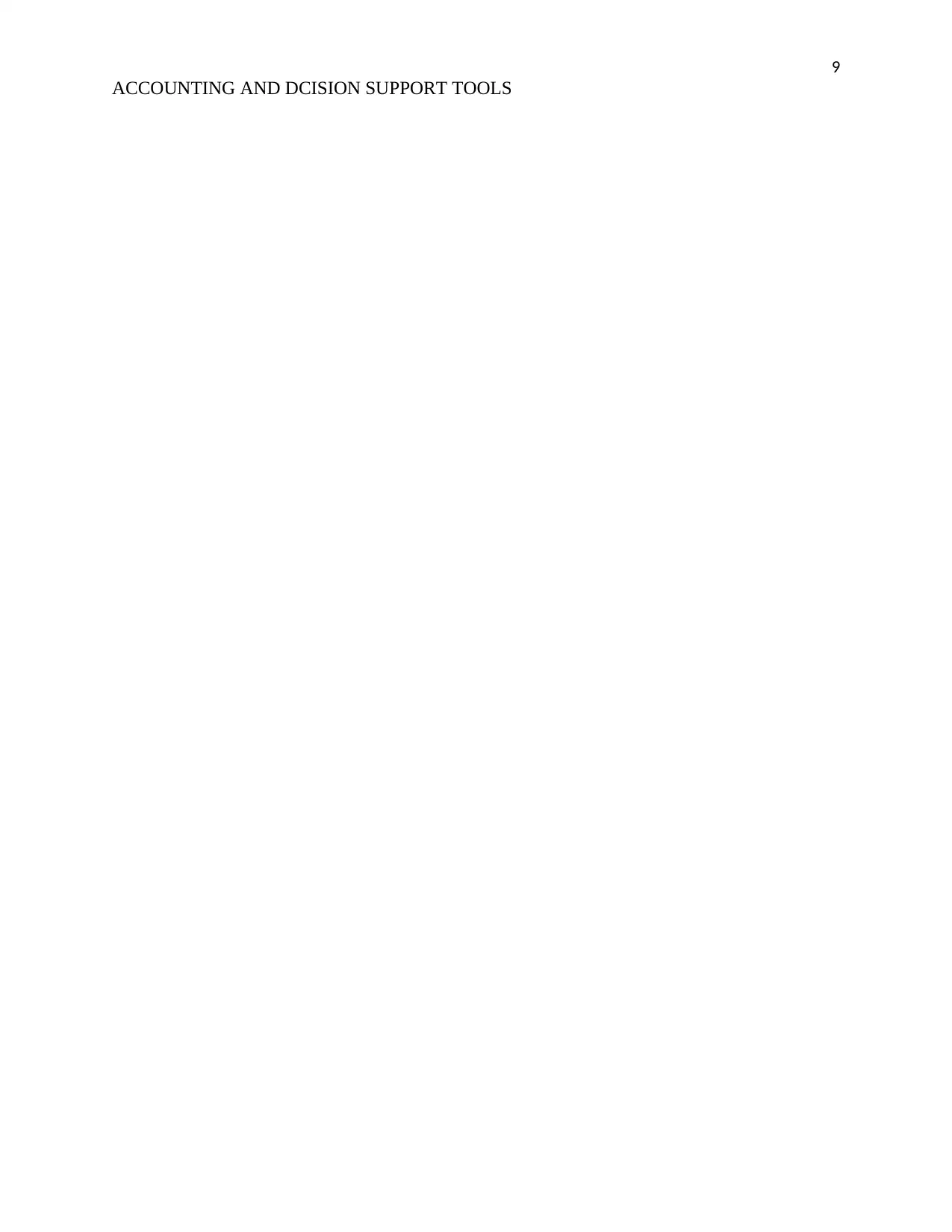
9
ACCOUNTING AND DCISION SUPPORT TOOLS
ACCOUNTING AND DCISION SUPPORT TOOLS
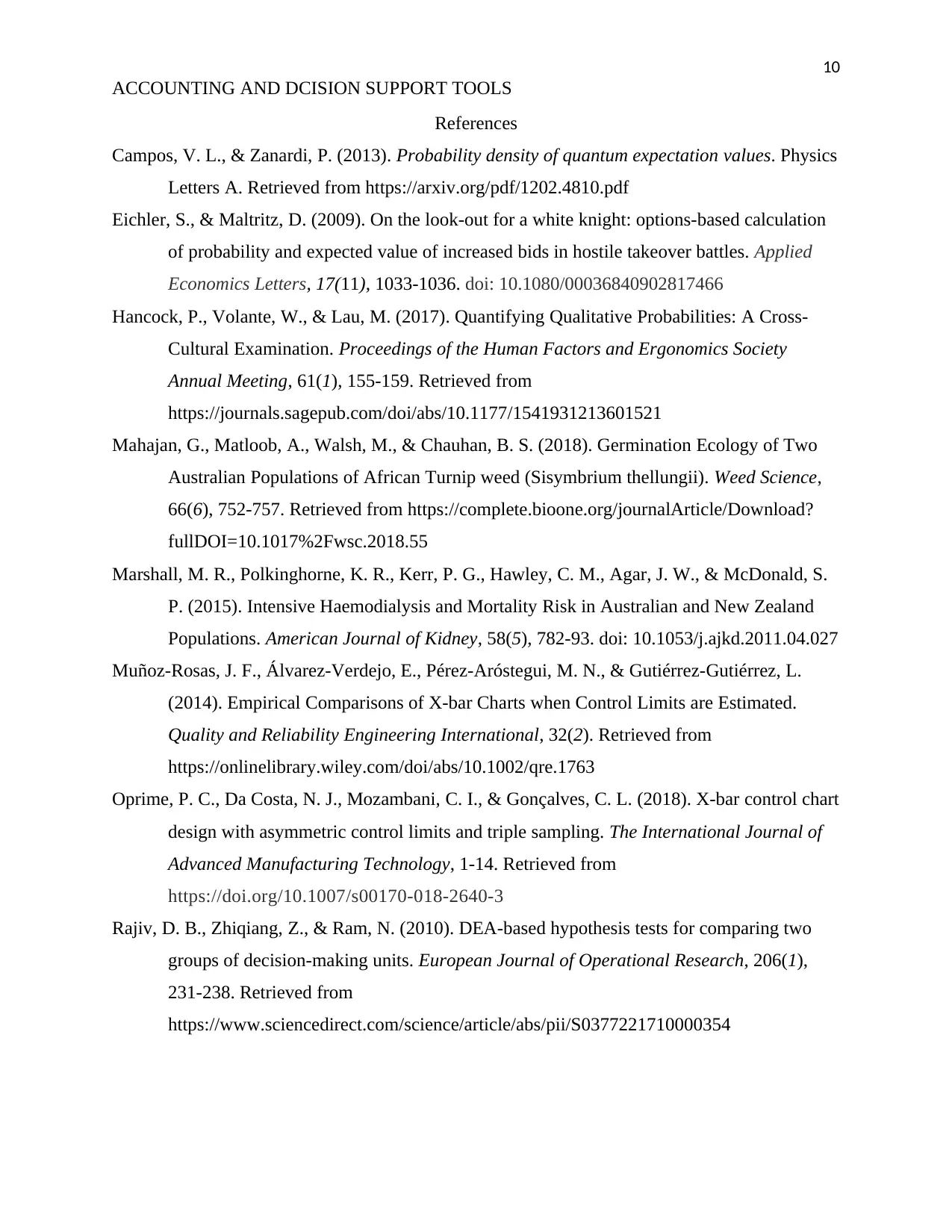
10
ACCOUNTING AND DCISION SUPPORT TOOLS
References
Campos, V. L., & Zanardi, P. (2013). Probability density of quantum expectation values. Physics
Letters A. Retrieved from https://arxiv.org/pdf/1202.4810.pdf
Eichler, S., & Maltritz, D. (2009). On the look-out for a white knight: options-based calculation
of probability and expected value of increased bids in hostile takeover battles. Applied
Economics Letters, 17(11), 1033-1036. doi: 10.1080/00036840902817466
Hancock, P., Volante, W., & Lau, M. (2017). Quantifying Qualitative Probabilities: A Cross-
Cultural Examination. Proceedings of the Human Factors and Ergonomics Society
Annual Meeting, 61(1), 155-159. Retrieved from
https://journals.sagepub.com/doi/abs/10.1177/1541931213601521
Mahajan, G., Matloob, A., Walsh, M., & Chauhan, B. S. (2018). Germination Ecology of Two
Australian Populations of African Turnip weed (Sisymbrium thellungii). Weed Science,
66(6), 752-757. Retrieved from https://complete.bioone.org/journalArticle/Download?
fullDOI=10.1017%2Fwsc.2018.55
Marshall, M. R., Polkinghorne, K. R., Kerr, P. G., Hawley, C. M., Agar, J. W., & McDonald, S.
P. (2015). Intensive Haemodialysis and Mortality Risk in Australian and New Zealand
Populations. American Journal of Kidney, 58(5), 782-93. doi: 10.1053/j.ajkd.2011.04.027
Muñoz-Rosas, J. F., Álvarez-Verdejo, E., Pérez-Aróstegui, M. N., & Gutiérrez-Gutiérrez, L.
(2014). Empirical Comparisons of X-bar Charts when Control Limits are Estimated.
Quality and Reliability Engineering International, 32(2). Retrieved from
https://onlinelibrary.wiley.com/doi/abs/10.1002/qre.1763
Oprime, P. C., Da Costa, N. J., Mozambani, C. I., & Gonçalves, C. L. (2018). X-bar control chart
design with asymmetric control limits and triple sampling. The International Journal of
Advanced Manufacturing Technology, 1-14. Retrieved from
https://doi.org/10.1007/s00170-018-2640-3
Rajiv, D. B., Zhiqiang, Z., & Ram, N. (2010). DEA-based hypothesis tests for comparing two
groups of decision-making units. European Journal of Operational Research, 206(1),
231-238. Retrieved from
https://www.sciencedirect.com/science/article/abs/pii/S0377221710000354
ACCOUNTING AND DCISION SUPPORT TOOLS
References
Campos, V. L., & Zanardi, P. (2013). Probability density of quantum expectation values. Physics
Letters A. Retrieved from https://arxiv.org/pdf/1202.4810.pdf
Eichler, S., & Maltritz, D. (2009). On the look-out for a white knight: options-based calculation
of probability and expected value of increased bids in hostile takeover battles. Applied
Economics Letters, 17(11), 1033-1036. doi: 10.1080/00036840902817466
Hancock, P., Volante, W., & Lau, M. (2017). Quantifying Qualitative Probabilities: A Cross-
Cultural Examination. Proceedings of the Human Factors and Ergonomics Society
Annual Meeting, 61(1), 155-159. Retrieved from
https://journals.sagepub.com/doi/abs/10.1177/1541931213601521
Mahajan, G., Matloob, A., Walsh, M., & Chauhan, B. S. (2018). Germination Ecology of Two
Australian Populations of African Turnip weed (Sisymbrium thellungii). Weed Science,
66(6), 752-757. Retrieved from https://complete.bioone.org/journalArticle/Download?
fullDOI=10.1017%2Fwsc.2018.55
Marshall, M. R., Polkinghorne, K. R., Kerr, P. G., Hawley, C. M., Agar, J. W., & McDonald, S.
P. (2015). Intensive Haemodialysis and Mortality Risk in Australian and New Zealand
Populations. American Journal of Kidney, 58(5), 782-93. doi: 10.1053/j.ajkd.2011.04.027
Muñoz-Rosas, J. F., Álvarez-Verdejo, E., Pérez-Aróstegui, M. N., & Gutiérrez-Gutiérrez, L.
(2014). Empirical Comparisons of X-bar Charts when Control Limits are Estimated.
Quality and Reliability Engineering International, 32(2). Retrieved from
https://onlinelibrary.wiley.com/doi/abs/10.1002/qre.1763
Oprime, P. C., Da Costa, N. J., Mozambani, C. I., & Gonçalves, C. L. (2018). X-bar control chart
design with asymmetric control limits and triple sampling. The International Journal of
Advanced Manufacturing Technology, 1-14. Retrieved from
https://doi.org/10.1007/s00170-018-2640-3
Rajiv, D. B., Zhiqiang, Z., & Ram, N. (2010). DEA-based hypothesis tests for comparing two
groups of decision-making units. European Journal of Operational Research, 206(1),
231-238. Retrieved from
https://www.sciencedirect.com/science/article/abs/pii/S0377221710000354
Secure Best Marks with AI Grader
Need help grading? Try our AI Grader for instant feedback on your assignments.
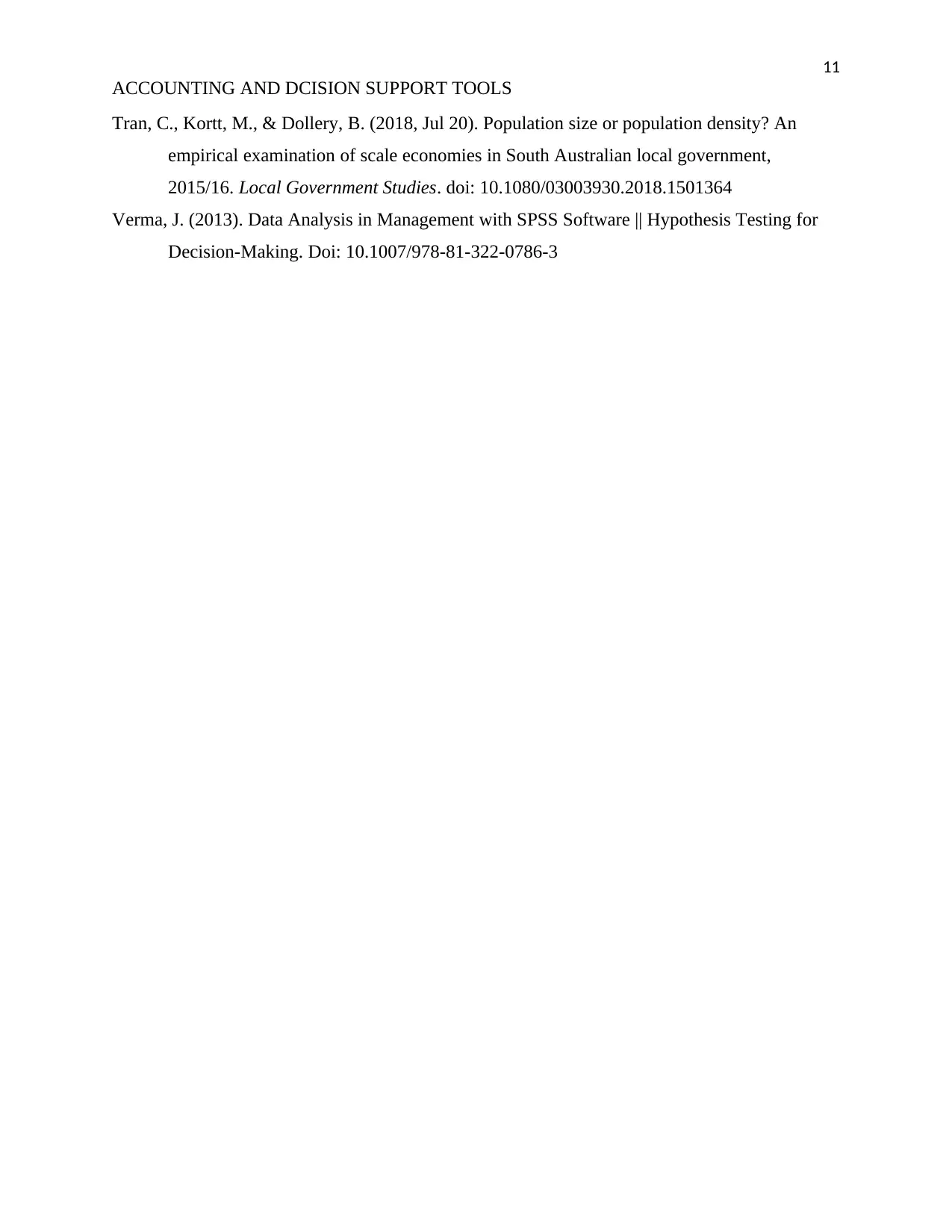
11
ACCOUNTING AND DCISION SUPPORT TOOLS
Tran, C., Kortt, M., & Dollery, B. (2018, Jul 20). Population size or population density? An
empirical examination of scale economies in South Australian local government,
2015/16. Local Government Studies. doi: 10.1080/03003930.2018.1501364
Verma, J. (2013). Data Analysis in Management with SPSS Software || Hypothesis Testing for
Decision-Making. Doi: 10.1007/978-81-322-0786-3
ACCOUNTING AND DCISION SUPPORT TOOLS
Tran, C., Kortt, M., & Dollery, B. (2018, Jul 20). Population size or population density? An
empirical examination of scale economies in South Australian local government,
2015/16. Local Government Studies. doi: 10.1080/03003930.2018.1501364
Verma, J. (2013). Data Analysis in Management with SPSS Software || Hypothesis Testing for
Decision-Making. Doi: 10.1007/978-81-322-0786-3
1 out of 11
Related Documents
![[object Object]](/_next/image/?url=%2F_next%2Fstatic%2Fmedia%2Flogo.6d15ce61.png&w=640&q=75)
Your All-in-One AI-Powered Toolkit for Academic Success.
+13062052269
info@desklib.com
Available 24*7 on WhatsApp / Email
Unlock your academic potential
© 2024 | Zucol Services PVT LTD | All rights reserved.