GDP and Life Satisfaction in Portugal
VerifiedAdded on 2020/01/23
|11
|1676
|133
Report
AI Summary
This assignment examines the correlation between GDP per capita and life satisfaction in Portugal. It analyzes a dataset, employing linear regression to determine the strength of the association between these variables. The analysis reveals a significant positive relationship, suggesting that higher GDP per capita correlates with increased life satisfaction. Based on the findings, policy recommendations are provided to policymakers, focusing on strategies to maximize GDP per capita and enhance overall well-being in Portugal.
Contribute Materials
Your contribution can guide someone’s learning journey. Share your
documents today.
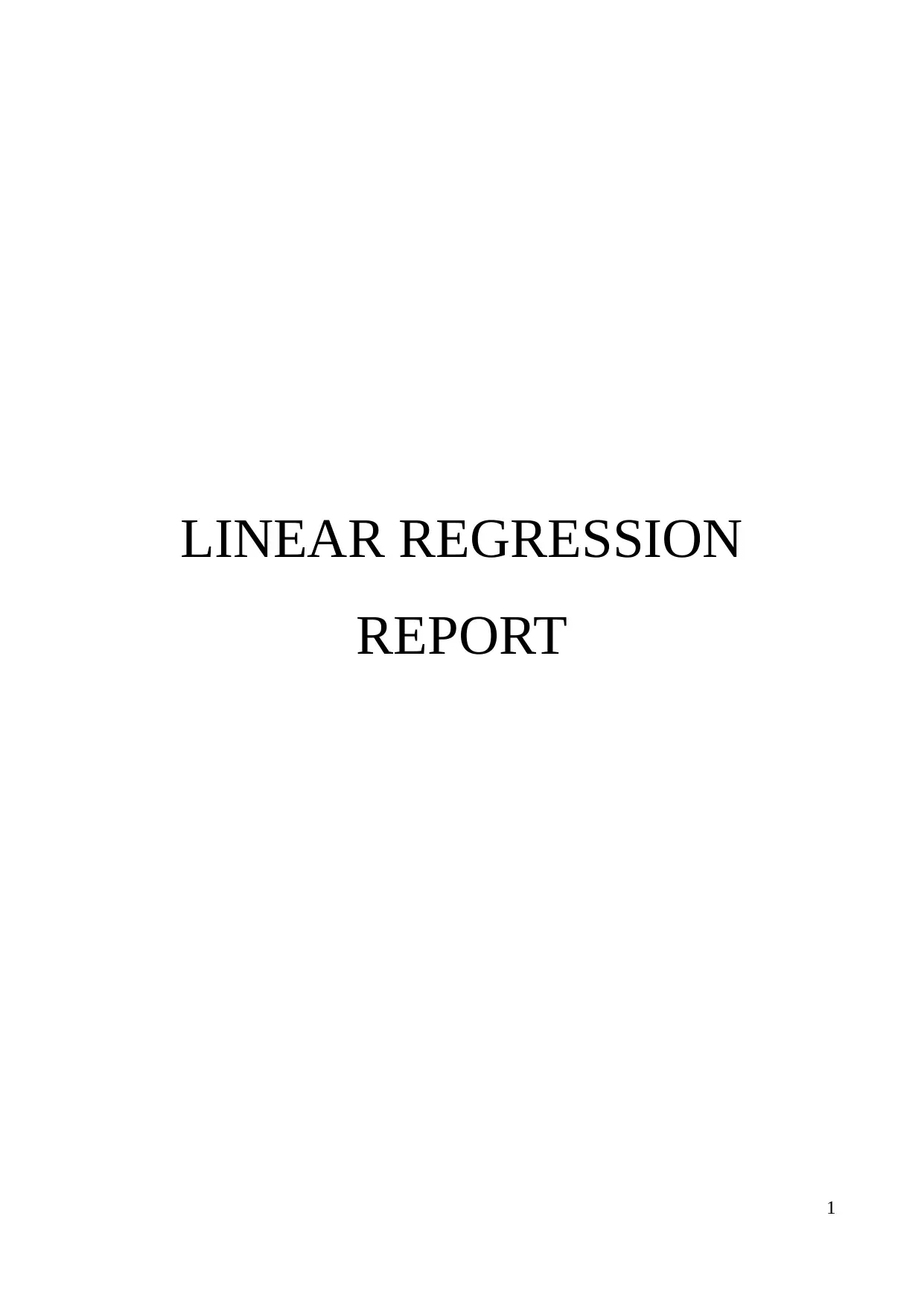
LINEAR REGRESSION
REPORT
1
REPORT
1
Secure Best Marks with AI Grader
Need help grading? Try our AI Grader for instant feedback on your assignments.
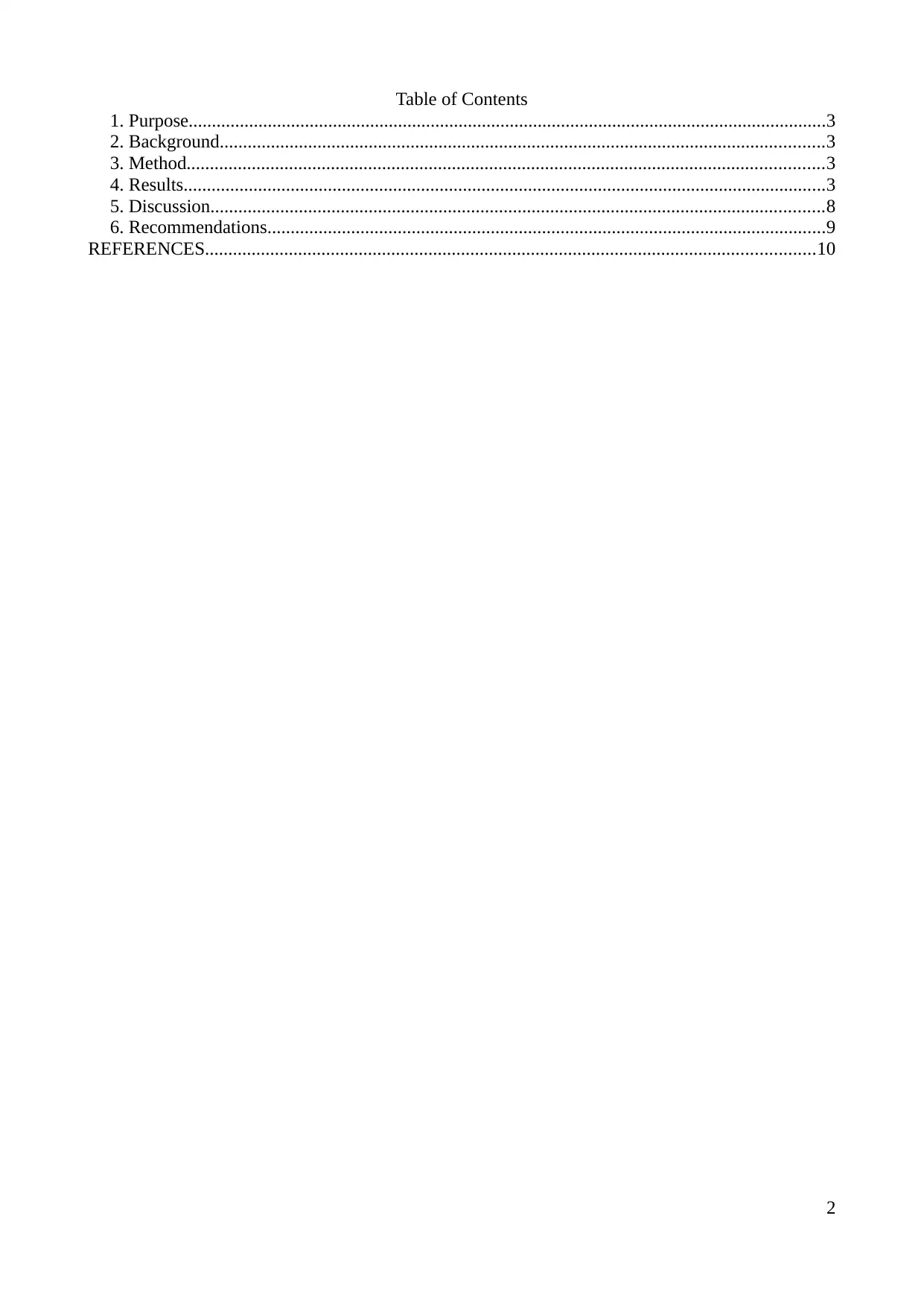
Table of Contents
1. Purpose.........................................................................................................................................3
2. Background..................................................................................................................................3
3. Method.........................................................................................................................................3
4. Results..........................................................................................................................................3
5. Discussion....................................................................................................................................8
6. Recommendations........................................................................................................................9
REFERENCES...................................................................................................................................10
2
1. Purpose.........................................................................................................................................3
2. Background..................................................................................................................................3
3. Method.........................................................................................................................................3
4. Results..........................................................................................................................................3
5. Discussion....................................................................................................................................8
6. Recommendations........................................................................................................................9
REFERENCES...................................................................................................................................10
2
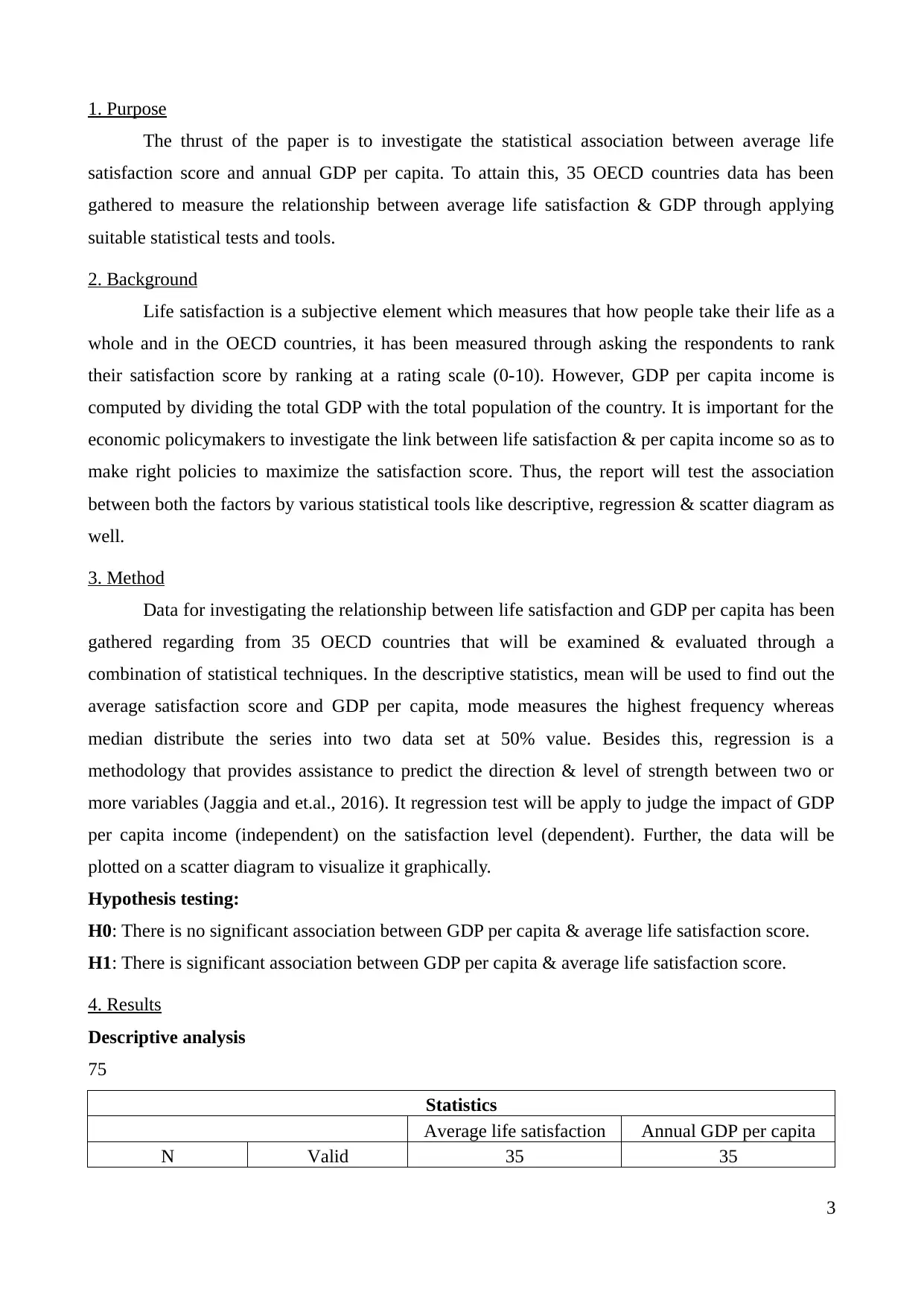
1. Purpose
The thrust of the paper is to investigate the statistical association between average life
satisfaction score and annual GDP per capita. To attain this, 35 OECD countries data has been
gathered to measure the relationship between average life satisfaction & GDP through applying
suitable statistical tests and tools.
2. Background
Life satisfaction is a subjective element which measures that how people take their life as a
whole and in the OECD countries, it has been measured through asking the respondents to rank
their satisfaction score by ranking at a rating scale (0-10). However, GDP per capita income is
computed by dividing the total GDP with the total population of the country. It is important for the
economic policymakers to investigate the link between life satisfaction & per capita income so as to
make right policies to maximize the satisfaction score. Thus, the report will test the association
between both the factors by various statistical tools like descriptive, regression & scatter diagram as
well.
3. Method
Data for investigating the relationship between life satisfaction and GDP per capita has been
gathered regarding from 35 OECD countries that will be examined & evaluated through a
combination of statistical techniques. In the descriptive statistics, mean will be used to find out the
average satisfaction score and GDP per capita, mode measures the highest frequency whereas
median distribute the series into two data set at 50% value. Besides this, regression is a
methodology that provides assistance to predict the direction & level of strength between two or
more variables (Jaggia and et.al., 2016). It regression test will be apply to judge the impact of GDP
per capita income (independent) on the satisfaction level (dependent). Further, the data will be
plotted on a scatter diagram to visualize it graphically.
Hypothesis testing:
H0: There is no significant association between GDP per capita & average life satisfaction score.
H1: There is significant association between GDP per capita & average life satisfaction score.
4. Results
Descriptive analysis
75
Statistics
Average life satisfaction Annual GDP per capita
N Valid 35 35
3
The thrust of the paper is to investigate the statistical association between average life
satisfaction score and annual GDP per capita. To attain this, 35 OECD countries data has been
gathered to measure the relationship between average life satisfaction & GDP through applying
suitable statistical tests and tools.
2. Background
Life satisfaction is a subjective element which measures that how people take their life as a
whole and in the OECD countries, it has been measured through asking the respondents to rank
their satisfaction score by ranking at a rating scale (0-10). However, GDP per capita income is
computed by dividing the total GDP with the total population of the country. It is important for the
economic policymakers to investigate the link between life satisfaction & per capita income so as to
make right policies to maximize the satisfaction score. Thus, the report will test the association
between both the factors by various statistical tools like descriptive, regression & scatter diagram as
well.
3. Method
Data for investigating the relationship between life satisfaction and GDP per capita has been
gathered regarding from 35 OECD countries that will be examined & evaluated through a
combination of statistical techniques. In the descriptive statistics, mean will be used to find out the
average satisfaction score and GDP per capita, mode measures the highest frequency whereas
median distribute the series into two data set at 50% value. Besides this, regression is a
methodology that provides assistance to predict the direction & level of strength between two or
more variables (Jaggia and et.al., 2016). It regression test will be apply to judge the impact of GDP
per capita income (independent) on the satisfaction level (dependent). Further, the data will be
plotted on a scatter diagram to visualize it graphically.
Hypothesis testing:
H0: There is no significant association between GDP per capita & average life satisfaction score.
H1: There is significant association between GDP per capita & average life satisfaction score.
4. Results
Descriptive analysis
75
Statistics
Average life satisfaction Annual GDP per capita
N Valid 35 35
3
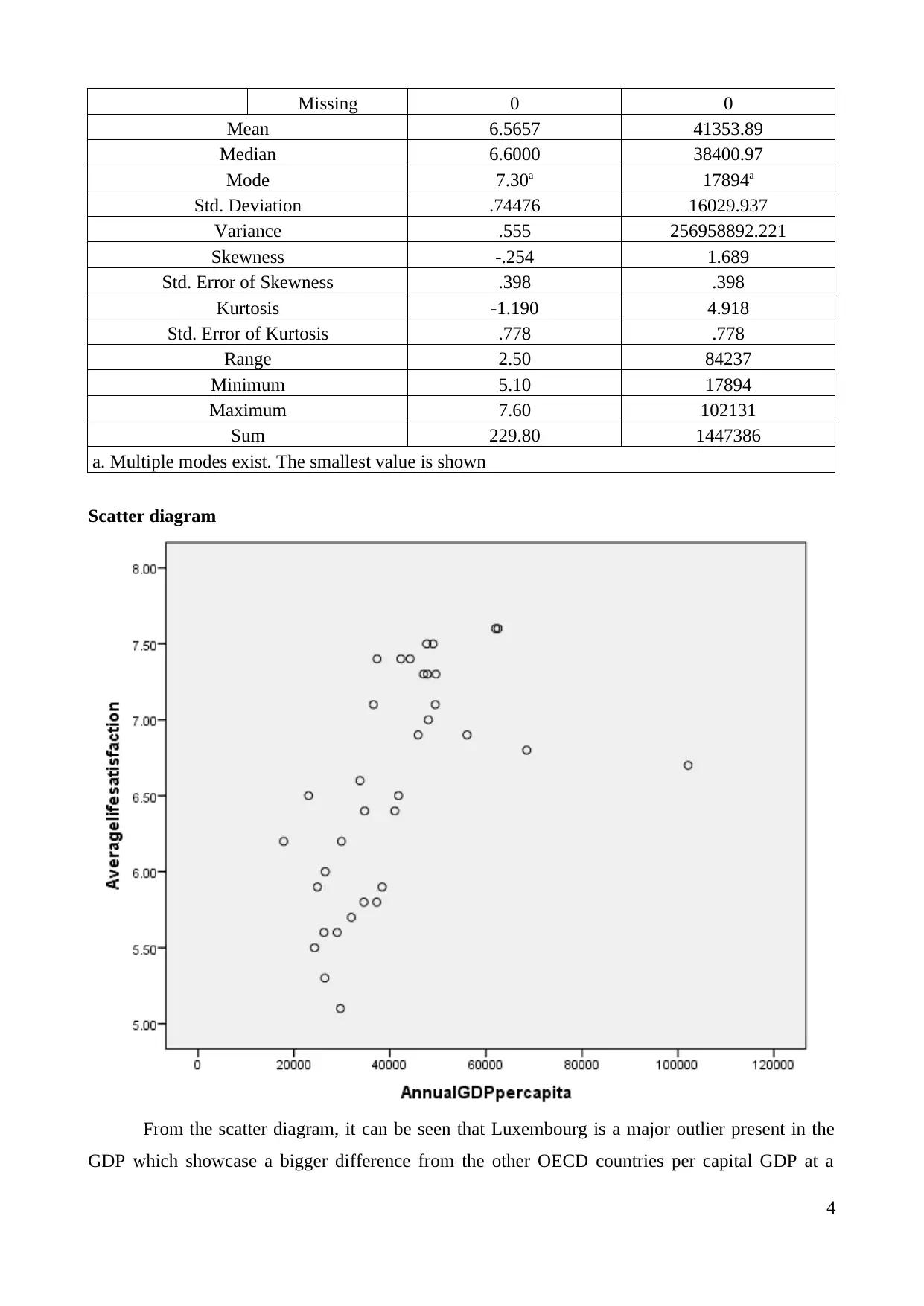
Missing 0 0
Mean 6.5657 41353.89
Median 6.6000 38400.97
Mode 7.30a 17894a
Std. Deviation .74476 16029.937
Variance .555 256958892.221
Skewness -.254 1.689
Std. Error of Skewness .398 .398
Kurtosis -1.190 4.918
Std. Error of Kurtosis .778 .778
Range 2.50 84237
Minimum 5.10 17894
Maximum 7.60 102131
Sum 229.80 1447386
a. Multiple modes exist. The smallest value is shown
Scatter diagram
From the scatter diagram, it can be seen that Luxembourg is a major outlier present in the
GDP which showcase a bigger difference from the other OECD countries per capital GDP at a
4
Mean 6.5657 41353.89
Median 6.6000 38400.97
Mode 7.30a 17894a
Std. Deviation .74476 16029.937
Variance .555 256958892.221
Skewness -.254 1.689
Std. Error of Skewness .398 .398
Kurtosis -1.190 4.918
Std. Error of Kurtosis .778 .778
Range 2.50 84237
Minimum 5.10 17894
Maximum 7.60 102131
Sum 229.80 1447386
a. Multiple modes exist. The smallest value is shown
Scatter diagram
From the scatter diagram, it can be seen that Luxembourg is a major outlier present in the
GDP which showcase a bigger difference from the other OECD countries per capital GDP at a
4
Paraphrase This Document
Need a fresh take? Get an instant paraphrase of this document with our AI Paraphraser
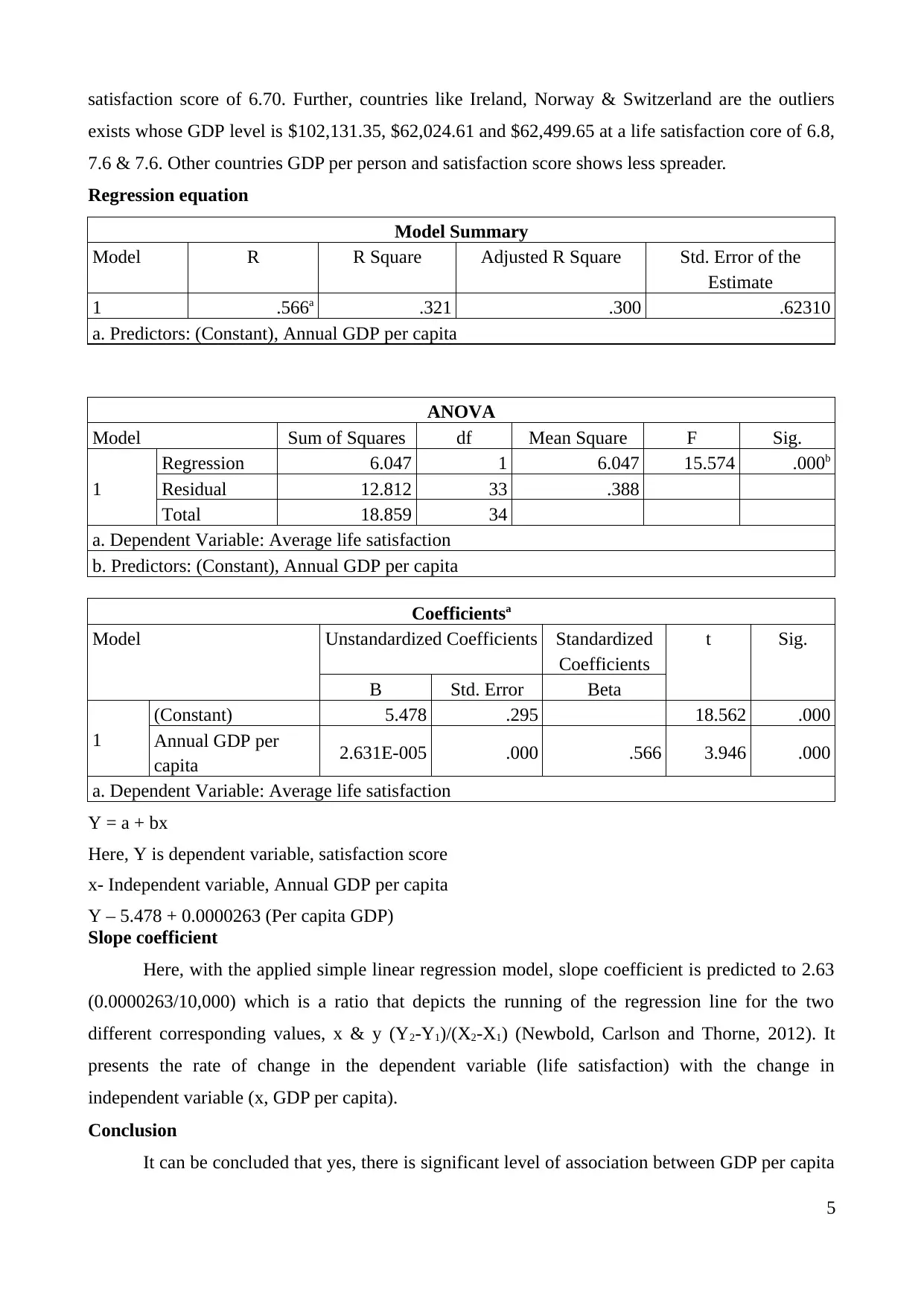
satisfaction score of 6.70. Further, countries like Ireland, Norway & Switzerland are the outliers
exists whose GDP level is $102,131.35, $62,024.61 and $62,499.65 at a life satisfaction core of 6.8,
7.6 & 7.6. Other countries GDP per person and satisfaction score shows less spreader.
Regression equation
Model Summary
Model R R Square Adjusted R Square Std. Error of the
Estimate
1 .566a .321 .300 .62310
a. Predictors: (Constant), Annual GDP per capita
ANOVA
Model Sum of Squares df Mean Square F Sig.
1
Regression 6.047 1 6.047 15.574 .000b
Residual 12.812 33 .388
Total 18.859 34
a. Dependent Variable: Average life satisfaction
b. Predictors: (Constant), Annual GDP per capita
Coefficientsa
Model Unstandardized Coefficients Standardized
Coefficients
t Sig.
B Std. Error Beta
1
(Constant) 5.478 .295 18.562 .000
Annual GDP per
capita 2.631E-005 .000 .566 3.946 .000
a. Dependent Variable: Average life satisfaction
Y = a + bx
Here, Y is dependent variable, satisfaction score
x- Independent variable, Annual GDP per capita
Y – 5.478 + 0.0000263 (Per capita GDP)
Slope coefficient
Here, with the applied simple linear regression model, slope coefficient is predicted to 2.63
(0.0000263/10,000) which is a ratio that depicts the running of the regression line for the two
different corresponding values, x & y (Y2-Y1)/(X2-X1) (Newbold, Carlson and Thorne, 2012). It
presents the rate of change in the dependent variable (life satisfaction) with the change in
independent variable (x, GDP per capita).
Conclusion
It can be concluded that yes, there is significant level of association between GDP per capita
5
exists whose GDP level is $102,131.35, $62,024.61 and $62,499.65 at a life satisfaction core of 6.8,
7.6 & 7.6. Other countries GDP per person and satisfaction score shows less spreader.
Regression equation
Model Summary
Model R R Square Adjusted R Square Std. Error of the
Estimate
1 .566a .321 .300 .62310
a. Predictors: (Constant), Annual GDP per capita
ANOVA
Model Sum of Squares df Mean Square F Sig.
1
Regression 6.047 1 6.047 15.574 .000b
Residual 12.812 33 .388
Total 18.859 34
a. Dependent Variable: Average life satisfaction
b. Predictors: (Constant), Annual GDP per capita
Coefficientsa
Model Unstandardized Coefficients Standardized
Coefficients
t Sig.
B Std. Error Beta
1
(Constant) 5.478 .295 18.562 .000
Annual GDP per
capita 2.631E-005 .000 .566 3.946 .000
a. Dependent Variable: Average life satisfaction
Y = a + bx
Here, Y is dependent variable, satisfaction score
x- Independent variable, Annual GDP per capita
Y – 5.478 + 0.0000263 (Per capita GDP)
Slope coefficient
Here, with the applied simple linear regression model, slope coefficient is predicted to 2.63
(0.0000263/10,000) which is a ratio that depicts the running of the regression line for the two
different corresponding values, x & y (Y2-Y1)/(X2-X1) (Newbold, Carlson and Thorne, 2012). It
presents the rate of change in the dependent variable (life satisfaction) with the change in
independent variable (x, GDP per capita).
Conclusion
It can be concluded that yes, there is significant level of association between GDP per capita
5
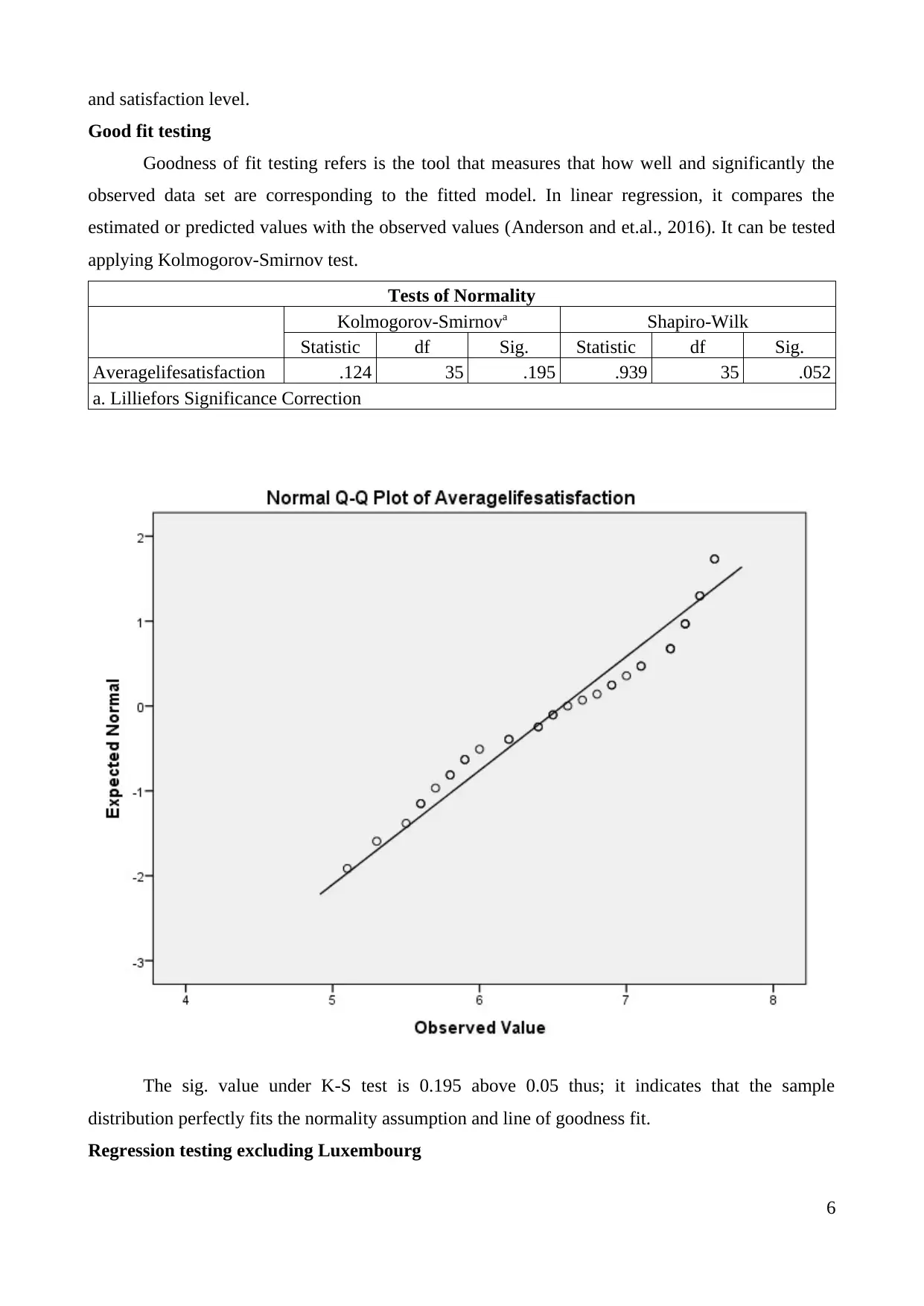
and satisfaction level.
Good fit testing
Goodness of fit testing refers is the tool that measures that how well and significantly the
observed data set are corresponding to the fitted model. In linear regression, it compares the
estimated or predicted values with the observed values (Anderson and et.al., 2016). It can be tested
applying Kolmogorov-Smirnov test.
Tests of Normality
Kolmogorov-Smirnova Shapiro-Wilk
Statistic df Sig. Statistic df Sig.
Averagelifesatisfaction .124 35 .195 .939 35 .052
a. Lilliefors Significance Correction
The sig. value under K-S test is 0.195 above 0.05 thus; it indicates that the sample
distribution perfectly fits the normality assumption and line of goodness fit.
Regression testing excluding Luxembourg
6
Good fit testing
Goodness of fit testing refers is the tool that measures that how well and significantly the
observed data set are corresponding to the fitted model. In linear regression, it compares the
estimated or predicted values with the observed values (Anderson and et.al., 2016). It can be tested
applying Kolmogorov-Smirnov test.
Tests of Normality
Kolmogorov-Smirnova Shapiro-Wilk
Statistic df Sig. Statistic df Sig.
Averagelifesatisfaction .124 35 .195 .939 35 .052
a. Lilliefors Significance Correction
The sig. value under K-S test is 0.195 above 0.05 thus; it indicates that the sample
distribution perfectly fits the normality assumption and line of goodness fit.
Regression testing excluding Luxembourg
6
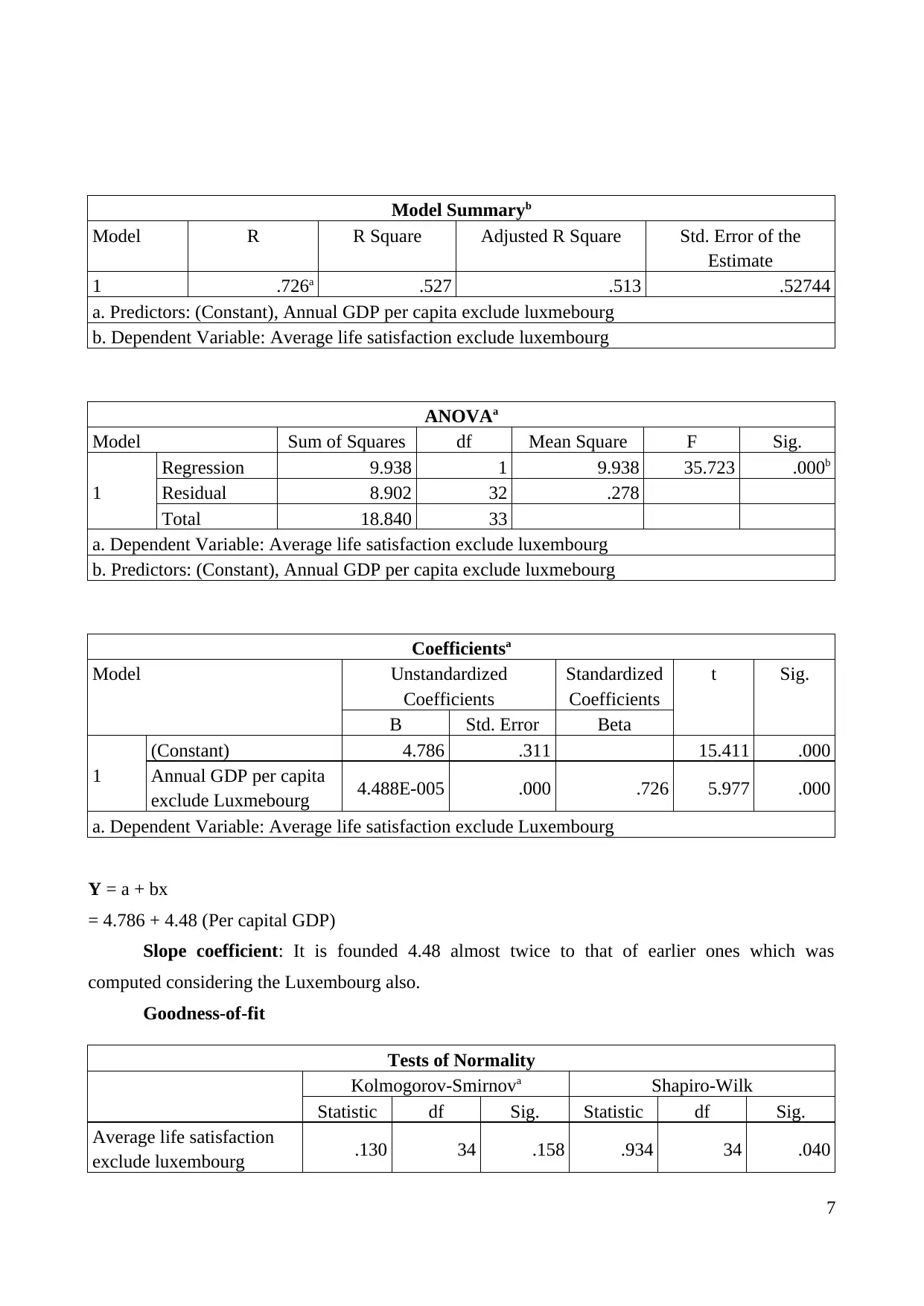
Model Summaryb
Model R R Square Adjusted R Square Std. Error of the
Estimate
1 .726a .527 .513 .52744
a. Predictors: (Constant), Annual GDP per capita exclude luxmebourg
b. Dependent Variable: Average life satisfaction exclude luxembourg
ANOVAa
Model Sum of Squares df Mean Square F Sig.
1
Regression 9.938 1 9.938 35.723 .000b
Residual 8.902 32 .278
Total 18.840 33
a. Dependent Variable: Average life satisfaction exclude luxembourg
b. Predictors: (Constant), Annual GDP per capita exclude luxmebourg
Coefficientsa
Model Unstandardized
Coefficients
Standardized
Coefficients
t Sig.
B Std. Error Beta
1
(Constant) 4.786 .311 15.411 .000
Annual GDP per capita
exclude Luxmebourg 4.488E-005 .000 .726 5.977 .000
a. Dependent Variable: Average life satisfaction exclude Luxembourg
Y = a + bx
= 4.786 + 4.48 (Per capital GDP)
Slope coefficient: It is founded 4.48 almost twice to that of earlier ones which was
computed considering the Luxembourg also.
Goodness-of-fit
Tests of Normality
Kolmogorov-Smirnova Shapiro-Wilk
Statistic df Sig. Statistic df Sig.
Average life satisfaction
exclude luxembourg .130 34 .158 .934 34 .040
7
Model R R Square Adjusted R Square Std. Error of the
Estimate
1 .726a .527 .513 .52744
a. Predictors: (Constant), Annual GDP per capita exclude luxmebourg
b. Dependent Variable: Average life satisfaction exclude luxembourg
ANOVAa
Model Sum of Squares df Mean Square F Sig.
1
Regression 9.938 1 9.938 35.723 .000b
Residual 8.902 32 .278
Total 18.840 33
a. Dependent Variable: Average life satisfaction exclude luxembourg
b. Predictors: (Constant), Annual GDP per capita exclude luxmebourg
Coefficientsa
Model Unstandardized
Coefficients
Standardized
Coefficients
t Sig.
B Std. Error Beta
1
(Constant) 4.786 .311 15.411 .000
Annual GDP per capita
exclude Luxmebourg 4.488E-005 .000 .726 5.977 .000
a. Dependent Variable: Average life satisfaction exclude Luxembourg
Y = a + bx
= 4.786 + 4.48 (Per capital GDP)
Slope coefficient: It is founded 4.48 almost twice to that of earlier ones which was
computed considering the Luxembourg also.
Goodness-of-fit
Tests of Normality
Kolmogorov-Smirnova Shapiro-Wilk
Statistic df Sig. Statistic df Sig.
Average life satisfaction
exclude luxembourg .130 34 .158 .934 34 .040
7
Secure Best Marks with AI Grader
Need help grading? Try our AI Grader for instant feedback on your assignments.
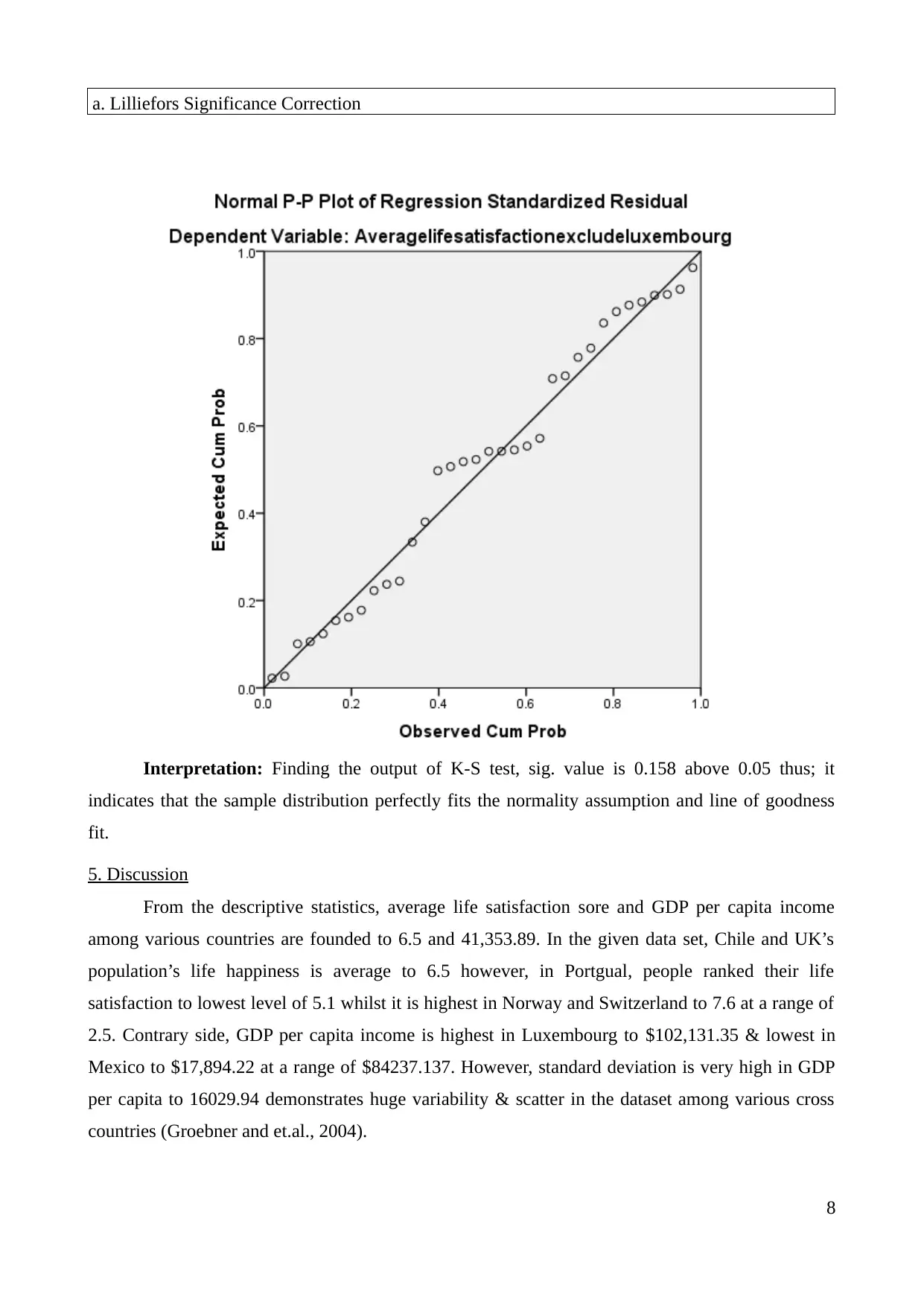
a. Lilliefors Significance Correction
Interpretation: Finding the output of K-S test, sig. value is 0.158 above 0.05 thus; it
indicates that the sample distribution perfectly fits the normality assumption and line of goodness
fit.
5. Discussion
From the descriptive statistics, average life satisfaction sore and GDP per capita income
among various countries are founded to 6.5 and 41,353.89. In the given data set, Chile and UK’s
population’s life happiness is average to 6.5 however, in Portgual, people ranked their life
satisfaction to lowest level of 5.1 whilst it is highest in Norway and Switzerland to 7.6 at a range of
2.5. Contrary side, GDP per capita income is highest in Luxembourg to $102,131.35 & lowest in
Mexico to $17,894.22 at a range of $84237.137. However, standard deviation is very high in GDP
per capita to 16029.94 demonstrates huge variability & scatter in the dataset among various cross
countries (Groebner and et.al., 2004).
8
Interpretation: Finding the output of K-S test, sig. value is 0.158 above 0.05 thus; it
indicates that the sample distribution perfectly fits the normality assumption and line of goodness
fit.
5. Discussion
From the descriptive statistics, average life satisfaction sore and GDP per capita income
among various countries are founded to 6.5 and 41,353.89. In the given data set, Chile and UK’s
population’s life happiness is average to 6.5 however, in Portgual, people ranked their life
satisfaction to lowest level of 5.1 whilst it is highest in Norway and Switzerland to 7.6 at a range of
2.5. Contrary side, GDP per capita income is highest in Luxembourg to $102,131.35 & lowest in
Mexico to $17,894.22 at a range of $84237.137. However, standard deviation is very high in GDP
per capita to 16029.94 demonstrates huge variability & scatter in the dataset among various cross
countries (Groebner and et.al., 2004).
8
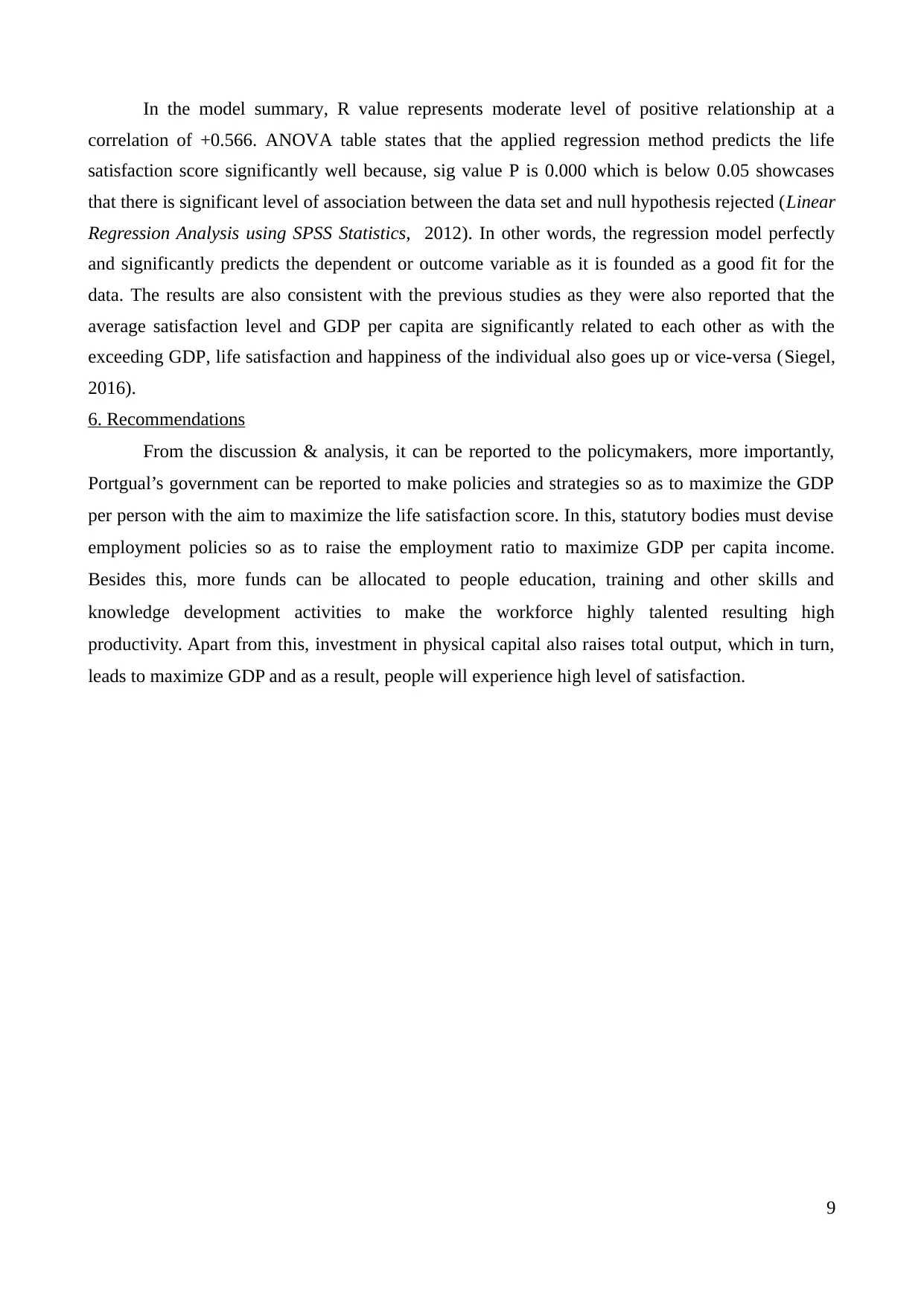
In the model summary, R value represents moderate level of positive relationship at a
correlation of +0.566. ANOVA table states that the applied regression method predicts the life
satisfaction score significantly well because, sig value P is 0.000 which is below 0.05 showcases
that there is significant level of association between the data set and null hypothesis rejected (Linear
Regression Analysis using SPSS Statistics, 2012). In other words, the regression model perfectly
and significantly predicts the dependent or outcome variable as it is founded as a good fit for the
data. The results are also consistent with the previous studies as they were also reported that the
average satisfaction level and GDP per capita are significantly related to each other as with the
exceeding GDP, life satisfaction and happiness of the individual also goes up or vice-versa (Siegel,
2016).
6. Recommendations
From the discussion & analysis, it can be reported to the policymakers, more importantly,
Portgual’s government can be reported to make policies and strategies so as to maximize the GDP
per person with the aim to maximize the life satisfaction score. In this, statutory bodies must devise
employment policies so as to raise the employment ratio to maximize GDP per capita income.
Besides this, more funds can be allocated to people education, training and other skills and
knowledge development activities to make the workforce highly talented resulting high
productivity. Apart from this, investment in physical capital also raises total output, which in turn,
leads to maximize GDP and as a result, people will experience high level of satisfaction.
9
correlation of +0.566. ANOVA table states that the applied regression method predicts the life
satisfaction score significantly well because, sig value P is 0.000 which is below 0.05 showcases
that there is significant level of association between the data set and null hypothesis rejected (Linear
Regression Analysis using SPSS Statistics, 2012). In other words, the regression model perfectly
and significantly predicts the dependent or outcome variable as it is founded as a good fit for the
data. The results are also consistent with the previous studies as they were also reported that the
average satisfaction level and GDP per capita are significantly related to each other as with the
exceeding GDP, life satisfaction and happiness of the individual also goes up or vice-versa (Siegel,
2016).
6. Recommendations
From the discussion & analysis, it can be reported to the policymakers, more importantly,
Portgual’s government can be reported to make policies and strategies so as to maximize the GDP
per person with the aim to maximize the life satisfaction score. In this, statutory bodies must devise
employment policies so as to raise the employment ratio to maximize GDP per capita income.
Besides this, more funds can be allocated to people education, training and other skills and
knowledge development activities to make the workforce highly talented resulting high
productivity. Apart from this, investment in physical capital also raises total output, which in turn,
leads to maximize GDP and as a result, people will experience high level of satisfaction.
9
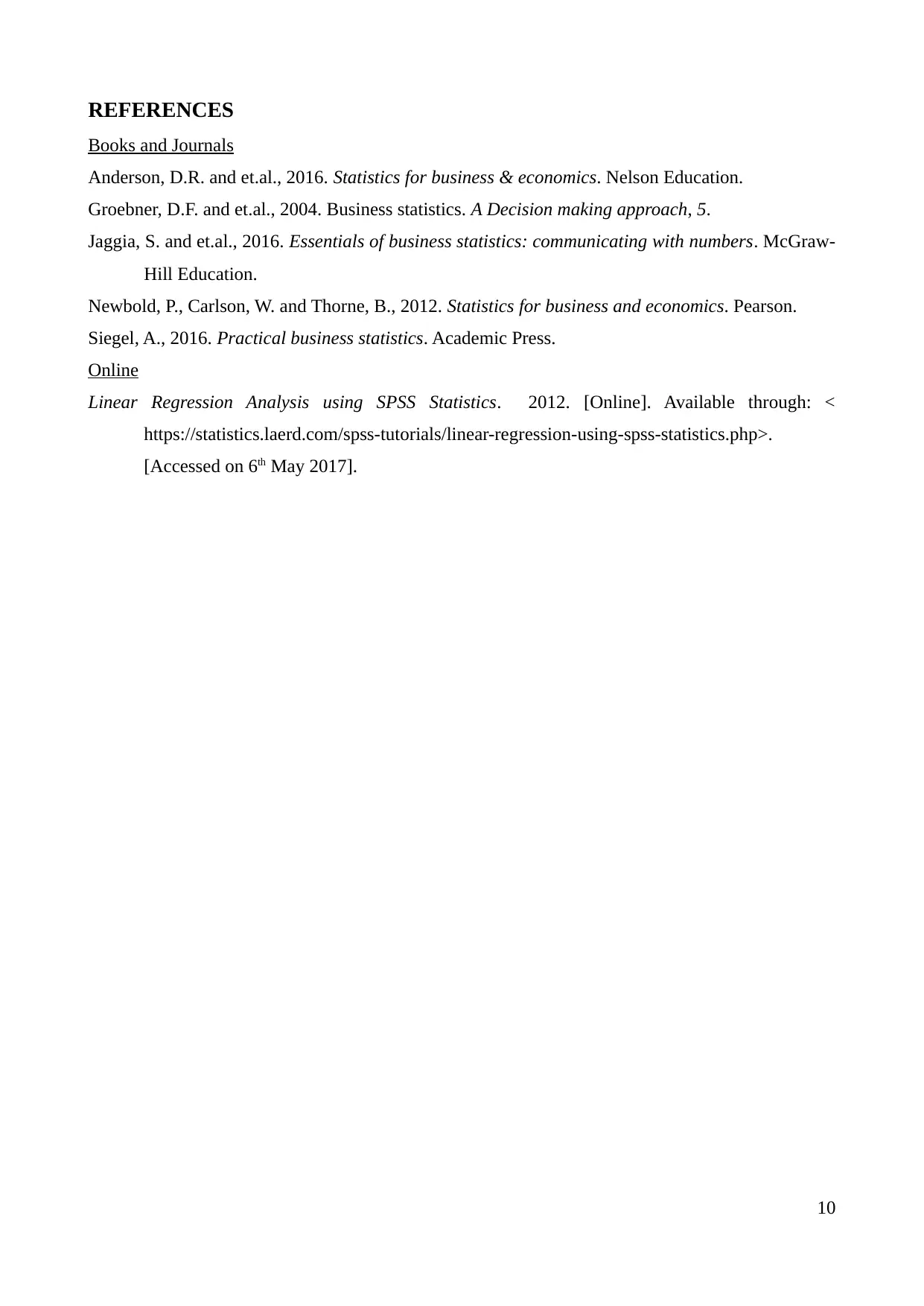
REFERENCES
Books and Journals
Anderson, D.R. and et.al., 2016. Statistics for business & economics. Nelson Education.
Groebner, D.F. and et.al., 2004. Business statistics. A Decision making approach, 5.
Jaggia, S. and et.al., 2016. Essentials of business statistics: communicating with numbers. McGraw-
Hill Education.
Newbold, P., Carlson, W. and Thorne, B., 2012. Statistics for business and economics. Pearson.
Siegel, A., 2016. Practical business statistics. Academic Press.
Online
Linear Regression Analysis using SPSS Statistics. 2012. [Online]. Available through: <
https://statistics.laerd.com/spss-tutorials/linear-regression-using-spss-statistics.php>.
[Accessed on 6th May 2017].
10
Books and Journals
Anderson, D.R. and et.al., 2016. Statistics for business & economics. Nelson Education.
Groebner, D.F. and et.al., 2004. Business statistics. A Decision making approach, 5.
Jaggia, S. and et.al., 2016. Essentials of business statistics: communicating with numbers. McGraw-
Hill Education.
Newbold, P., Carlson, W. and Thorne, B., 2012. Statistics for business and economics. Pearson.
Siegel, A., 2016. Practical business statistics. Academic Press.
Online
Linear Regression Analysis using SPSS Statistics. 2012. [Online]. Available through: <
https://statistics.laerd.com/spss-tutorials/linear-regression-using-spss-statistics.php>.
[Accessed on 6th May 2017].
10
Paraphrase This Document
Need a fresh take? Get an instant paraphrase of this document with our AI Paraphraser
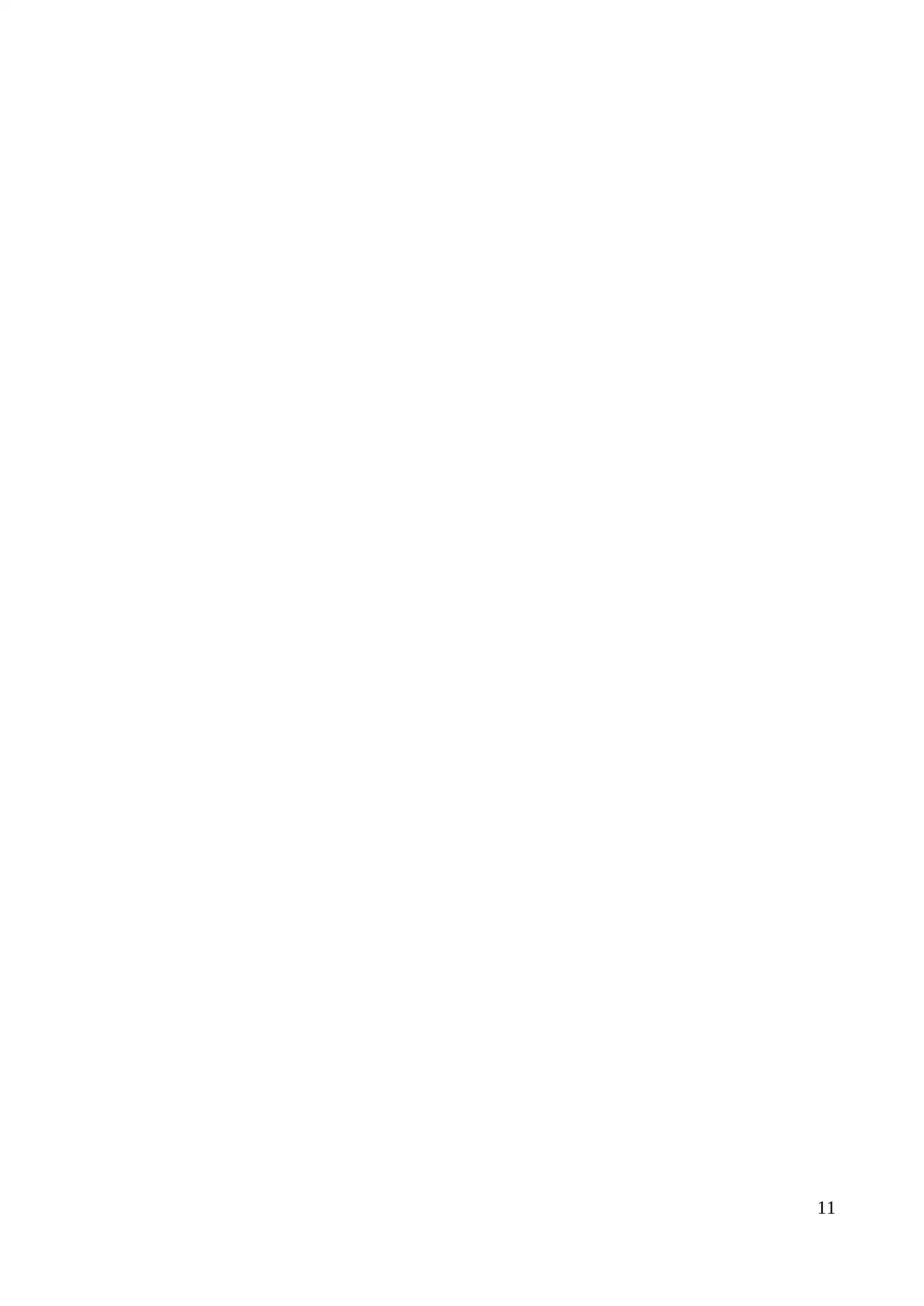
11
1 out of 11
Related Documents
![[object Object]](/_next/image/?url=%2F_next%2Fstatic%2Fmedia%2Flogo.6d15ce61.png&w=640&q=75)
Your All-in-One AI-Powered Toolkit for Academic Success.
+13062052269
info@desklib.com
Available 24*7 on WhatsApp / Email
Unlock your academic potential
© 2024 | Zucol Services PVT LTD | All rights reserved.