Analysis of Secondary Data
VerifiedAdded on 2023/04/19
|6
|1480
|90
AI Summary
This article discusses the importance of weighting in secondary data analysis. It explains how weighting can overcome limitations and provide accurate results. Examples are provided to illustrate how and why weighting is used. The current literature on weighting in healthcare studies is also reviewed.
Contribute Materials
Your contribution can guide someone’s learning journey. Share your
documents today.
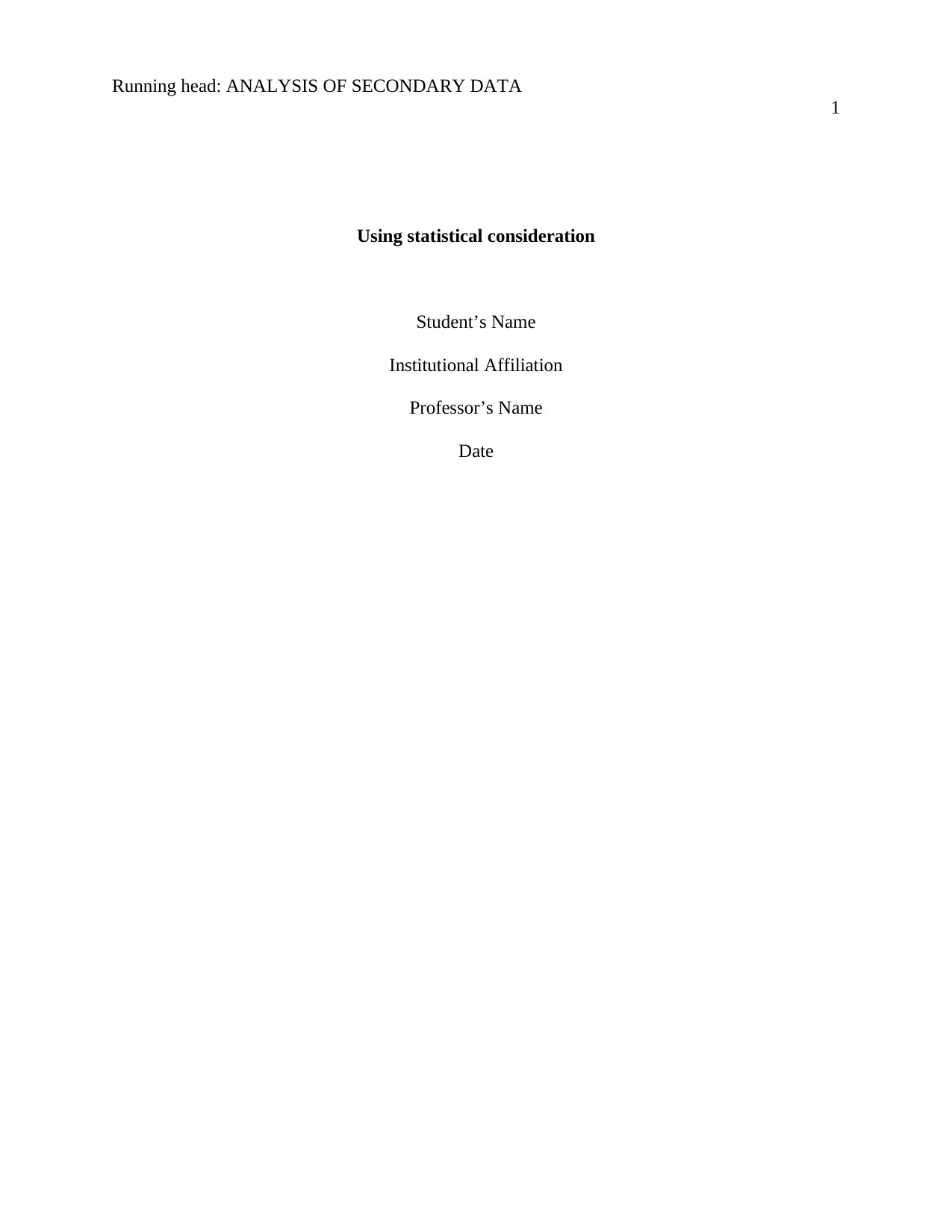
Running head: ANALYSIS OF SECONDARY DATA
1
Using statistical consideration
Student’s Name
Institutional Affiliation
Professor’s Name
Date
1
Using statistical consideration
Student’s Name
Institutional Affiliation
Professor’s Name
Date
Secure Best Marks with AI Grader
Need help grading? Try our AI Grader for instant feedback on your assignments.
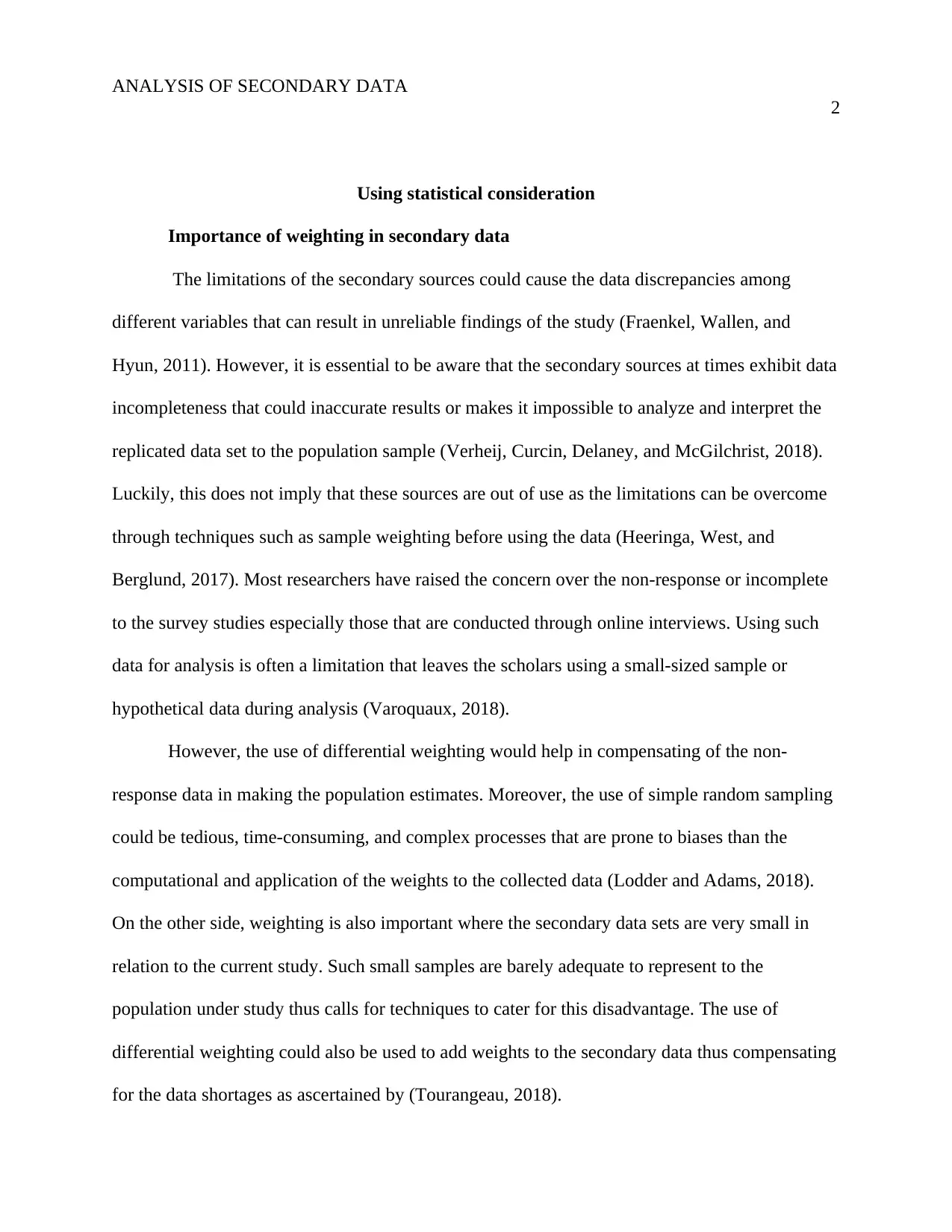
ANALYSIS OF SECONDARY DATA
2
Using statistical consideration
Importance of weighting in secondary data
The limitations of the secondary sources could cause the data discrepancies among
different variables that can result in unreliable findings of the study (Fraenkel, Wallen, and
Hyun, 2011). However, it is essential to be aware that the secondary sources at times exhibit data
incompleteness that could inaccurate results or makes it impossible to analyze and interpret the
replicated data set to the population sample (Verheij, Curcin, Delaney, and McGilchrist, 2018).
Luckily, this does not imply that these sources are out of use as the limitations can be overcome
through techniques such as sample weighting before using the data (Heeringa, West, and
Berglund, 2017). Most researchers have raised the concern over the non-response or incomplete
to the survey studies especially those that are conducted through online interviews. Using such
data for analysis is often a limitation that leaves the scholars using a small-sized sample or
hypothetical data during analysis (Varoquaux, 2018).
However, the use of differential weighting would help in compensating of the non-
response data in making the population estimates. Moreover, the use of simple random sampling
could be tedious, time-consuming, and complex processes that are prone to biases than the
computational and application of the weights to the collected data (Lodder and Adams, 2018).
On the other side, weighting is also important where the secondary data sets are very small in
relation to the current study. Such small samples are barely adequate to represent to the
population under study thus calls for techniques to cater for this disadvantage. The use of
differential weighting could also be used to add weights to the secondary data thus compensating
for the data shortages as ascertained by (Tourangeau, 2018).
2
Using statistical consideration
Importance of weighting in secondary data
The limitations of the secondary sources could cause the data discrepancies among
different variables that can result in unreliable findings of the study (Fraenkel, Wallen, and
Hyun, 2011). However, it is essential to be aware that the secondary sources at times exhibit data
incompleteness that could inaccurate results or makes it impossible to analyze and interpret the
replicated data set to the population sample (Verheij, Curcin, Delaney, and McGilchrist, 2018).
Luckily, this does not imply that these sources are out of use as the limitations can be overcome
through techniques such as sample weighting before using the data (Heeringa, West, and
Berglund, 2017). Most researchers have raised the concern over the non-response or incomplete
to the survey studies especially those that are conducted through online interviews. Using such
data for analysis is often a limitation that leaves the scholars using a small-sized sample or
hypothetical data during analysis (Varoquaux, 2018).
However, the use of differential weighting would help in compensating of the non-
response data in making the population estimates. Moreover, the use of simple random sampling
could be tedious, time-consuming, and complex processes that are prone to biases than the
computational and application of the weights to the collected data (Lodder and Adams, 2018).
On the other side, weighting is also important where the secondary data sets are very small in
relation to the current study. Such small samples are barely adequate to represent to the
population under study thus calls for techniques to cater for this disadvantage. The use of
differential weighting could also be used to add weights to the secondary data thus compensating
for the data shortages as ascertained by (Tourangeau, 2018).
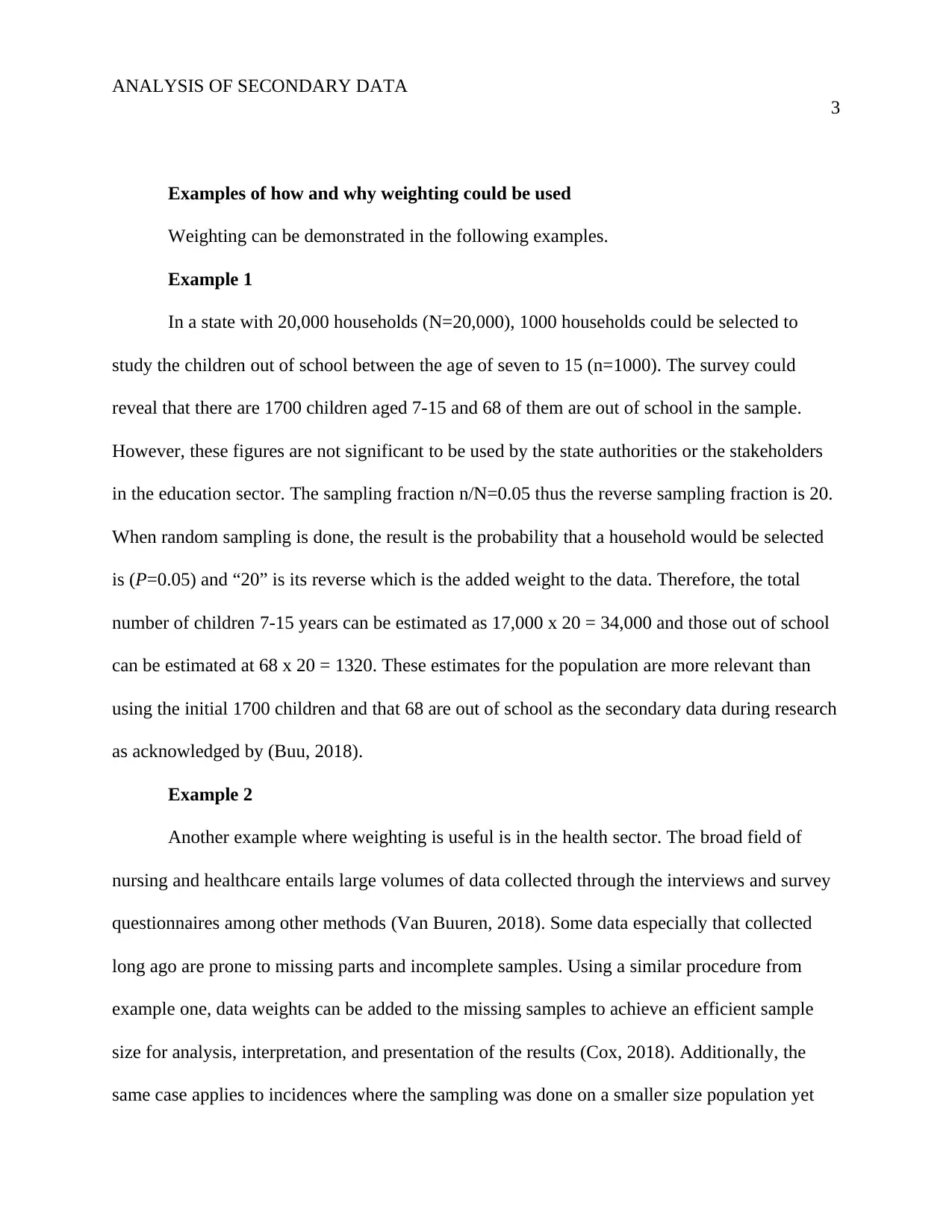
ANALYSIS OF SECONDARY DATA
3
Examples of how and why weighting could be used
Weighting can be demonstrated in the following examples.
Example 1
In a state with 20,000 households (N=20,000), 1000 households could be selected to
study the children out of school between the age of seven to 15 (n=1000). The survey could
reveal that there are 1700 children aged 7-15 and 68 of them are out of school in the sample.
However, these figures are not significant to be used by the state authorities or the stakeholders
in the education sector. The sampling fraction n/N=0.05 thus the reverse sampling fraction is 20.
When random sampling is done, the result is the probability that a household would be selected
is (P=0.05) and “20” is its reverse which is the added weight to the data. Therefore, the total
number of children 7-15 years can be estimated as 17,000 x 20 = 34,000 and those out of school
can be estimated at 68 x 20 = 1320. These estimates for the population are more relevant than
using the initial 1700 children and that 68 are out of school as the secondary data during research
as acknowledged by (Buu, 2018).
Example 2
Another example where weighting is useful is in the health sector. The broad field of
nursing and healthcare entails large volumes of data collected through the interviews and survey
questionnaires among other methods (Van Buuren, 2018). Some data especially that collected
long ago are prone to missing parts and incomplete samples. Using a similar procedure from
example one, data weights can be added to the missing samples to achieve an efficient sample
size for analysis, interpretation, and presentation of the results (Cox, 2018). Additionally, the
same case applies to incidences where the sampling was done on a smaller size population yet
3
Examples of how and why weighting could be used
Weighting can be demonstrated in the following examples.
Example 1
In a state with 20,000 households (N=20,000), 1000 households could be selected to
study the children out of school between the age of seven to 15 (n=1000). The survey could
reveal that there are 1700 children aged 7-15 and 68 of them are out of school in the sample.
However, these figures are not significant to be used by the state authorities or the stakeholders
in the education sector. The sampling fraction n/N=0.05 thus the reverse sampling fraction is 20.
When random sampling is done, the result is the probability that a household would be selected
is (P=0.05) and “20” is its reverse which is the added weight to the data. Therefore, the total
number of children 7-15 years can be estimated as 17,000 x 20 = 34,000 and those out of school
can be estimated at 68 x 20 = 1320. These estimates for the population are more relevant than
using the initial 1700 children and that 68 are out of school as the secondary data during research
as acknowledged by (Buu, 2018).
Example 2
Another example where weighting is useful is in the health sector. The broad field of
nursing and healthcare entails large volumes of data collected through the interviews and survey
questionnaires among other methods (Van Buuren, 2018). Some data especially that collected
long ago are prone to missing parts and incomplete samples. Using a similar procedure from
example one, data weights can be added to the missing samples to achieve an efficient sample
size for analysis, interpretation, and presentation of the results (Cox, 2018). Additionally, the
same case applies to incidences where the sampling was done on a smaller size population yet
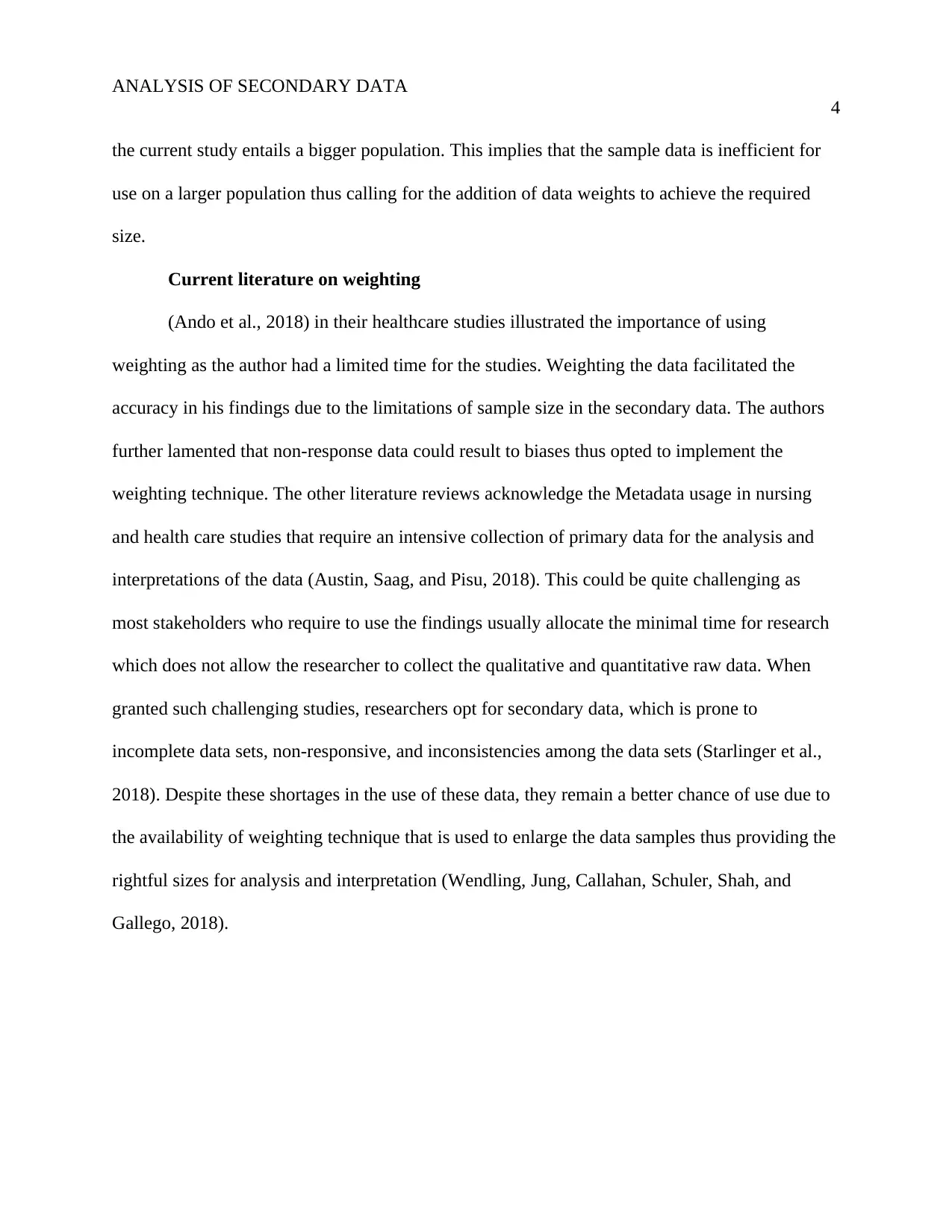
ANALYSIS OF SECONDARY DATA
4
the current study entails a bigger population. This implies that the sample data is inefficient for
use on a larger population thus calling for the addition of data weights to achieve the required
size.
Current literature on weighting
(Ando et al., 2018) in their healthcare studies illustrated the importance of using
weighting as the author had a limited time for the studies. Weighting the data facilitated the
accuracy in his findings due to the limitations of sample size in the secondary data. The authors
further lamented that non-response data could result to biases thus opted to implement the
weighting technique. The other literature reviews acknowledge the Metadata usage in nursing
and health care studies that require an intensive collection of primary data for the analysis and
interpretations of the data (Austin, Saag, and Pisu, 2018). This could be quite challenging as
most stakeholders who require to use the findings usually allocate the minimal time for research
which does not allow the researcher to collect the qualitative and quantitative raw data. When
granted such challenging studies, researchers opt for secondary data, which is prone to
incomplete data sets, non-responsive, and inconsistencies among the data sets (Starlinger et al.,
2018). Despite these shortages in the use of these data, they remain a better chance of use due to
the availability of weighting technique that is used to enlarge the data samples thus providing the
rightful sizes for analysis and interpretation (Wendling, Jung, Callahan, Schuler, Shah, and
Gallego, 2018).
4
the current study entails a bigger population. This implies that the sample data is inefficient for
use on a larger population thus calling for the addition of data weights to achieve the required
size.
Current literature on weighting
(Ando et al., 2018) in their healthcare studies illustrated the importance of using
weighting as the author had a limited time for the studies. Weighting the data facilitated the
accuracy in his findings due to the limitations of sample size in the secondary data. The authors
further lamented that non-response data could result to biases thus opted to implement the
weighting technique. The other literature reviews acknowledge the Metadata usage in nursing
and health care studies that require an intensive collection of primary data for the analysis and
interpretations of the data (Austin, Saag, and Pisu, 2018). This could be quite challenging as
most stakeholders who require to use the findings usually allocate the minimal time for research
which does not allow the researcher to collect the qualitative and quantitative raw data. When
granted such challenging studies, researchers opt for secondary data, which is prone to
incomplete data sets, non-responsive, and inconsistencies among the data sets (Starlinger et al.,
2018). Despite these shortages in the use of these data, they remain a better chance of use due to
the availability of weighting technique that is used to enlarge the data samples thus providing the
rightful sizes for analysis and interpretation (Wendling, Jung, Callahan, Schuler, Shah, and
Gallego, 2018).
Secure Best Marks with AI Grader
Need help grading? Try our AI Grader for instant feedback on your assignments.
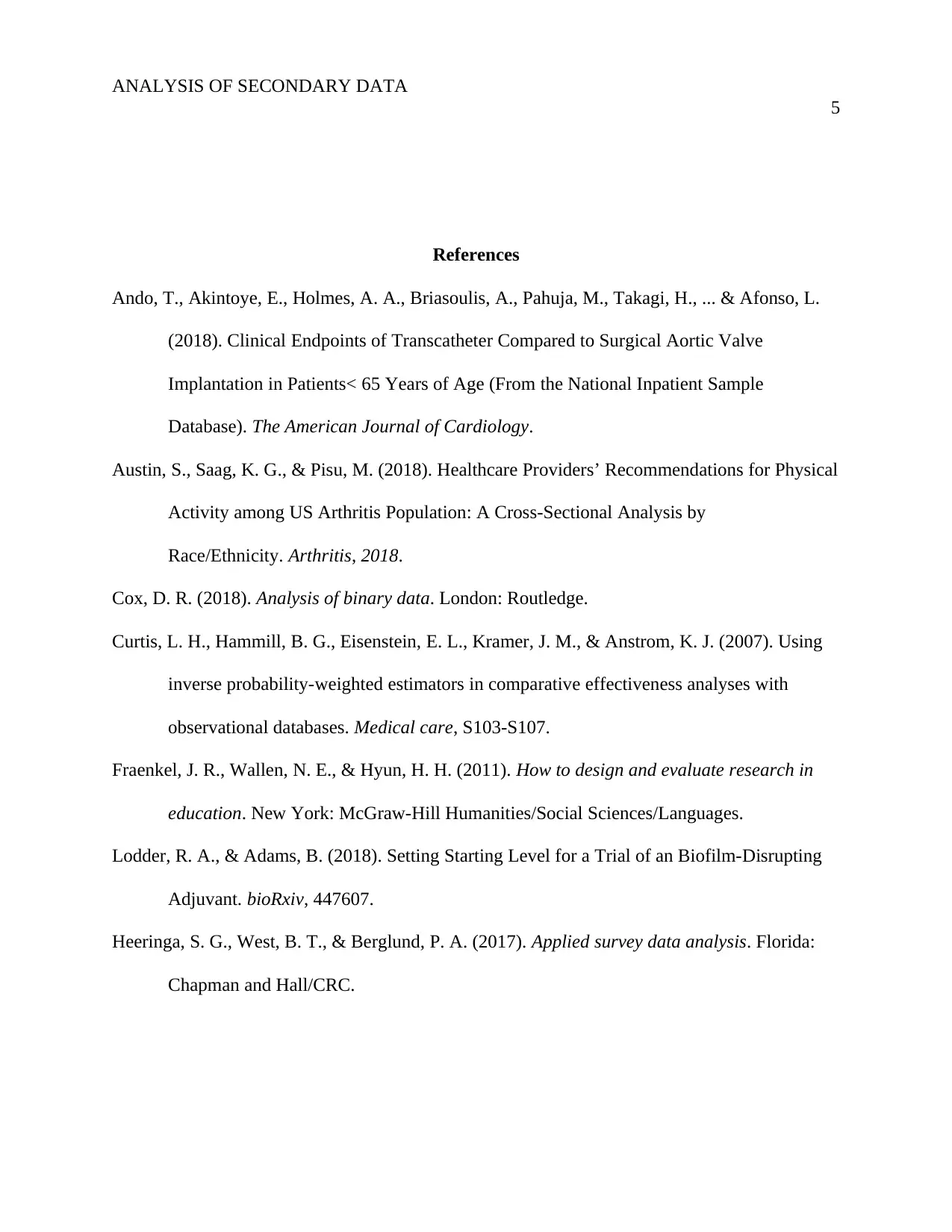
ANALYSIS OF SECONDARY DATA
5
References
Ando, T., Akintoye, E., Holmes, A. A., Briasoulis, A., Pahuja, M., Takagi, H., ... & Afonso, L.
(2018). Clinical Endpoints of Transcatheter Compared to Surgical Aortic Valve
Implantation in Patients< 65 Years of Age (From the National Inpatient Sample
Database). The American Journal of Cardiology.
Austin, S., Saag, K. G., & Pisu, M. (2018). Healthcare Providers’ Recommendations for Physical
Activity among US Arthritis Population: A Cross-Sectional Analysis by
Race/Ethnicity. Arthritis, 2018.
Cox, D. R. (2018). Analysis of binary data. London: Routledge.
Curtis, L. H., Hammill, B. G., Eisenstein, E. L., Kramer, J. M., & Anstrom, K. J. (2007). Using
inverse probability-weighted estimators in comparative effectiveness analyses with
observational databases. Medical care, S103-S107.
Fraenkel, J. R., Wallen, N. E., & Hyun, H. H. (2011). How to design and evaluate research in
education. New York: McGraw-Hill Humanities/Social Sciences/Languages.
Lodder, R. A., & Adams, B. (2018). Setting Starting Level for a Trial of an Biofilm-Disrupting
Adjuvant. bioRxiv, 447607.
Heeringa, S. G., West, B. T., & Berglund, P. A. (2017). Applied survey data analysis. Florida:
Chapman and Hall/CRC.
5
References
Ando, T., Akintoye, E., Holmes, A. A., Briasoulis, A., Pahuja, M., Takagi, H., ... & Afonso, L.
(2018). Clinical Endpoints of Transcatheter Compared to Surgical Aortic Valve
Implantation in Patients< 65 Years of Age (From the National Inpatient Sample
Database). The American Journal of Cardiology.
Austin, S., Saag, K. G., & Pisu, M. (2018). Healthcare Providers’ Recommendations for Physical
Activity among US Arthritis Population: A Cross-Sectional Analysis by
Race/Ethnicity. Arthritis, 2018.
Cox, D. R. (2018). Analysis of binary data. London: Routledge.
Curtis, L. H., Hammill, B. G., Eisenstein, E. L., Kramer, J. M., & Anstrom, K. J. (2007). Using
inverse probability-weighted estimators in comparative effectiveness analyses with
observational databases. Medical care, S103-S107.
Fraenkel, J. R., Wallen, N. E., & Hyun, H. H. (2011). How to design and evaluate research in
education. New York: McGraw-Hill Humanities/Social Sciences/Languages.
Lodder, R. A., & Adams, B. (2018). Setting Starting Level for a Trial of an Biofilm-Disrupting
Adjuvant. bioRxiv, 447607.
Heeringa, S. G., West, B. T., & Berglund, P. A. (2017). Applied survey data analysis. Florida:
Chapman and Hall/CRC.
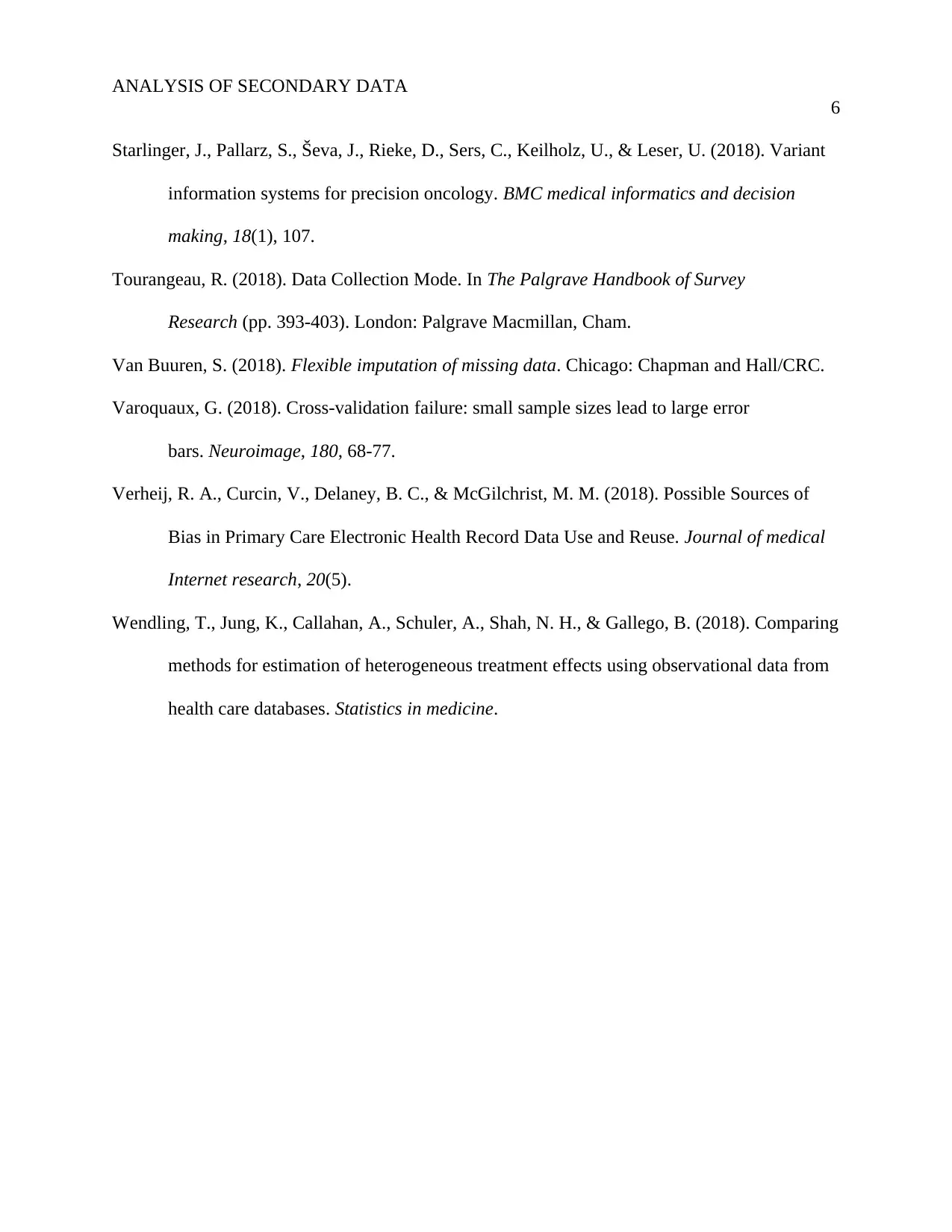
ANALYSIS OF SECONDARY DATA
6
Starlinger, J., Pallarz, S., Ševa, J., Rieke, D., Sers, C., Keilholz, U., & Leser, U. (2018). Variant
information systems for precision oncology. BMC medical informatics and decision
making, 18(1), 107.
Tourangeau, R. (2018). Data Collection Mode. In The Palgrave Handbook of Survey
Research (pp. 393-403). London: Palgrave Macmillan, Cham.
Van Buuren, S. (2018). Flexible imputation of missing data. Chicago: Chapman and Hall/CRC.
Varoquaux, G. (2018). Cross-validation failure: small sample sizes lead to large error
bars. Neuroimage, 180, 68-77.
Verheij, R. A., Curcin, V., Delaney, B. C., & McGilchrist, M. M. (2018). Possible Sources of
Bias in Primary Care Electronic Health Record Data Use and Reuse. Journal of medical
Internet research, 20(5).
Wendling, T., Jung, K., Callahan, A., Schuler, A., Shah, N. H., & Gallego, B. (2018). Comparing
methods for estimation of heterogeneous treatment effects using observational data from
health care databases. Statistics in medicine.
6
Starlinger, J., Pallarz, S., Ševa, J., Rieke, D., Sers, C., Keilholz, U., & Leser, U. (2018). Variant
information systems for precision oncology. BMC medical informatics and decision
making, 18(1), 107.
Tourangeau, R. (2018). Data Collection Mode. In The Palgrave Handbook of Survey
Research (pp. 393-403). London: Palgrave Macmillan, Cham.
Van Buuren, S. (2018). Flexible imputation of missing data. Chicago: Chapman and Hall/CRC.
Varoquaux, G. (2018). Cross-validation failure: small sample sizes lead to large error
bars. Neuroimage, 180, 68-77.
Verheij, R. A., Curcin, V., Delaney, B. C., & McGilchrist, M. M. (2018). Possible Sources of
Bias in Primary Care Electronic Health Record Data Use and Reuse. Journal of medical
Internet research, 20(5).
Wendling, T., Jung, K., Callahan, A., Schuler, A., Shah, N. H., & Gallego, B. (2018). Comparing
methods for estimation of heterogeneous treatment effects using observational data from
health care databases. Statistics in medicine.
1 out of 6
Related Documents
![[object Object]](/_next/image/?url=%2F_next%2Fstatic%2Fmedia%2Flogo.6d15ce61.png&w=640&q=75)
Your All-in-One AI-Powered Toolkit for Academic Success.
+13062052269
info@desklib.com
Available 24*7 on WhatsApp / Email
Unlock your academic potential
© 2024 | Zucol Services PVT LTD | All rights reserved.