Data Sampling Techniques in Business Analytics
VerifiedAdded on 2023/03/17
|14
|1452
|66
AI Summary
This article discusses the application of data sampling techniques in business analytics and their importance in analyzing populations and making predictions. It explores probability sampling methods like simple random sampling and quota sampling, as well as simulation techniques like Monte Carlo and bootstrap sampling. The article highlights the benefits of these techniques in enhancing company performance and decision-making.
Contribute Materials
Your contribution can guide someone’s learning journey. Share your
documents today.
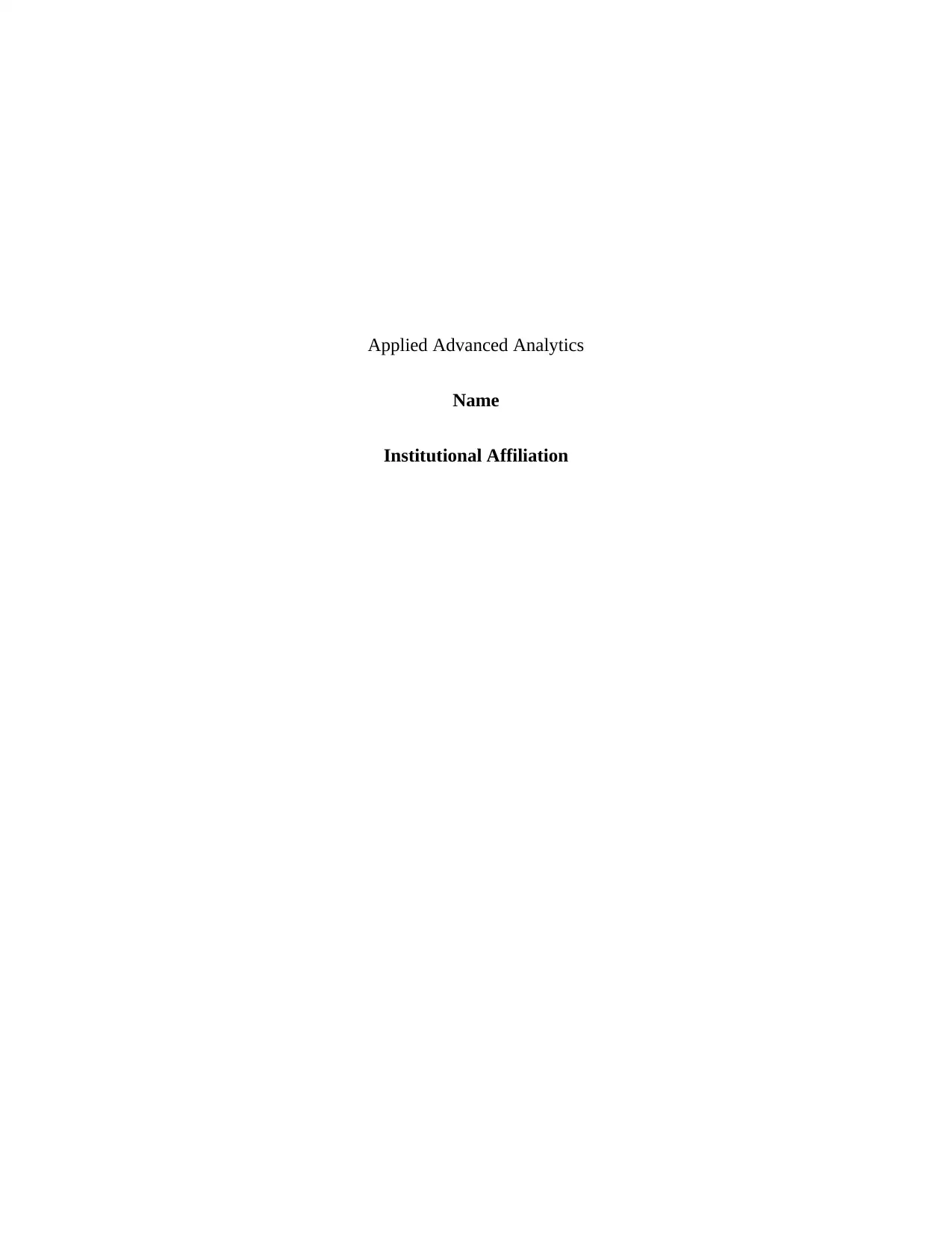
Applied Advanced Analytics
Name
Institutional Affiliation
Name
Institutional Affiliation
Secure Best Marks with AI Grader
Need help grading? Try our AI Grader for instant feedback on your assignments.
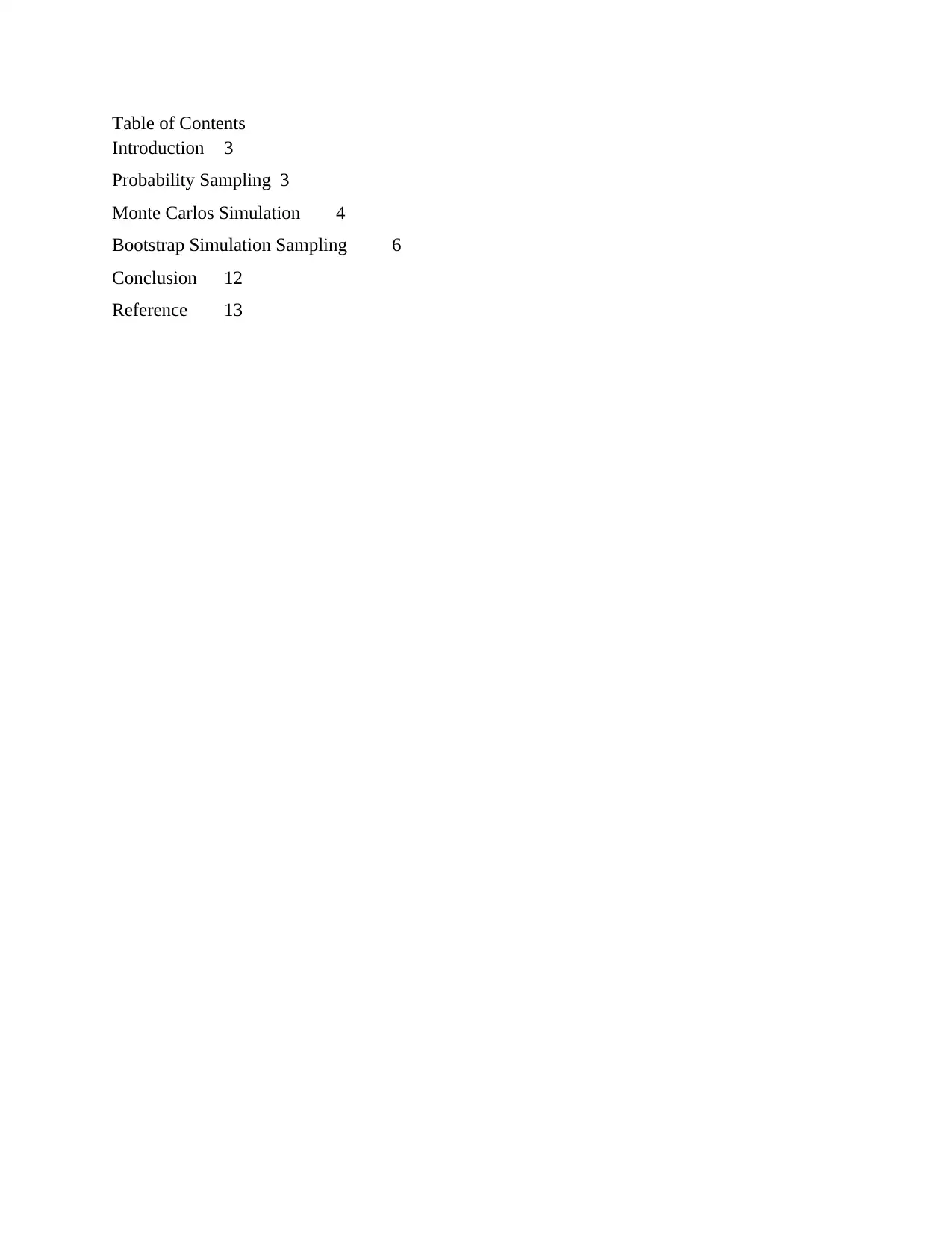
Table of Contents
Introduction 3
Probability Sampling 3
Monte Carlos Simulation 4
Bootstrap Simulation Sampling 6
Conclusion 12
Reference 13
Introduction 3
Probability Sampling 3
Monte Carlos Simulation 4
Bootstrap Simulation Sampling 6
Conclusion 12
Reference 13
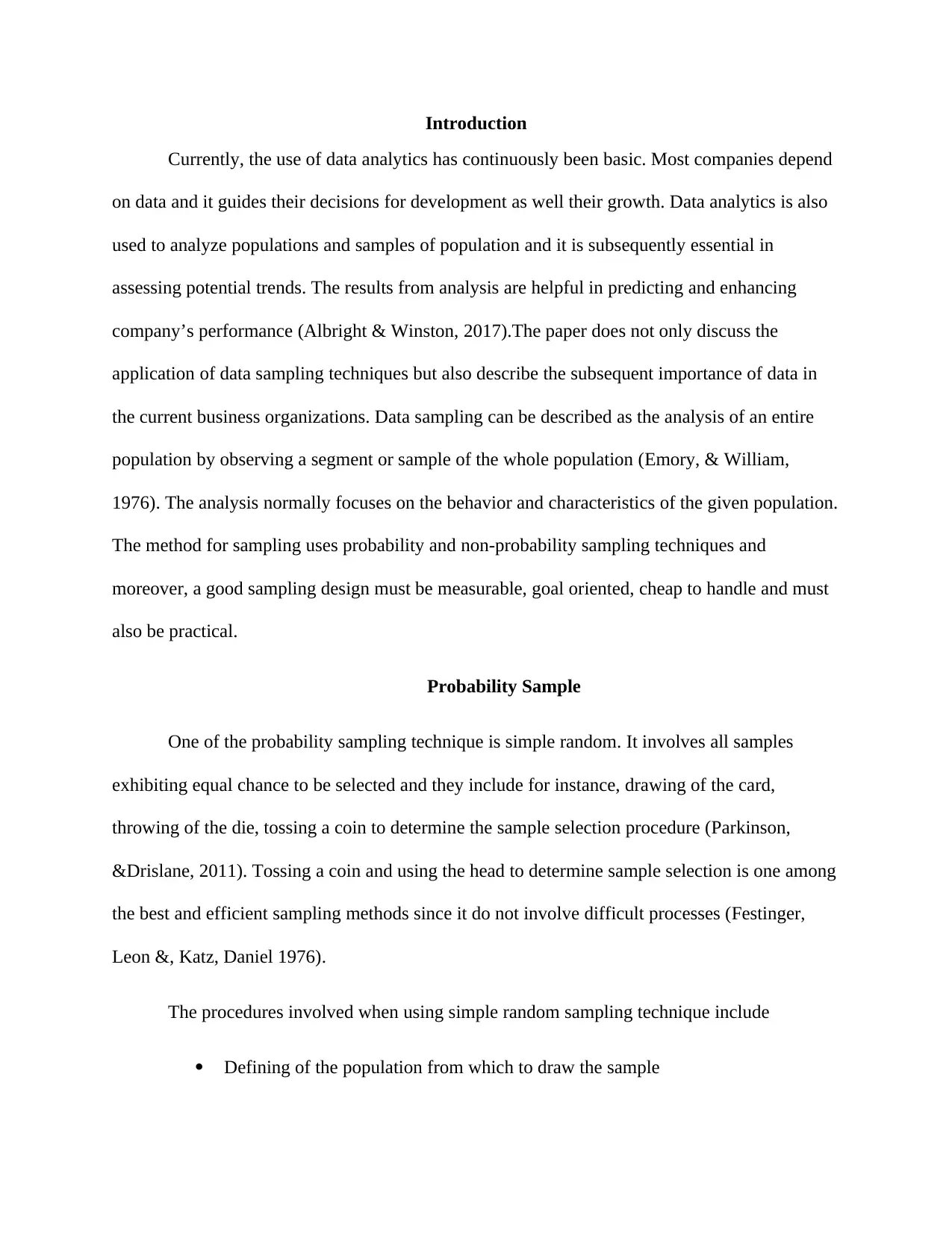
Introduction
Currently, the use of data analytics has continuously been basic. Most companies depend
on data and it guides their decisions for development as well their growth. Data analytics is also
used to analyze populations and samples of population and it is subsequently essential in
assessing potential trends. The results from analysis are helpful in predicting and enhancing
company’s performance (Albright & Winston, 2017).The paper does not only discuss the
application of data sampling techniques but also describe the subsequent importance of data in
the current business organizations. Data sampling can be described as the analysis of an entire
population by observing a segment or sample of the whole population (Emory, & William,
1976). The analysis normally focuses on the behavior and characteristics of the given population.
The method for sampling uses probability and non-probability sampling techniques and
moreover, a good sampling design must be measurable, goal oriented, cheap to handle and must
also be practical.
Probability Sample
One of the probability sampling technique is simple random. It involves all samples
exhibiting equal chance to be selected and they include for instance, drawing of the card,
throwing of the die, tossing a coin to determine the sample selection procedure (Parkinson,
&Drislane, 2011). Tossing a coin and using the head to determine sample selection is one among
the best and efficient sampling methods since it do not involve difficult processes (Festinger,
Leon &, Katz, Daniel 1976).
The procedures involved when using simple random sampling technique include
Defining of the population from which to draw the sample
Currently, the use of data analytics has continuously been basic. Most companies depend
on data and it guides their decisions for development as well their growth. Data analytics is also
used to analyze populations and samples of population and it is subsequently essential in
assessing potential trends. The results from analysis are helpful in predicting and enhancing
company’s performance (Albright & Winston, 2017).The paper does not only discuss the
application of data sampling techniques but also describe the subsequent importance of data in
the current business organizations. Data sampling can be described as the analysis of an entire
population by observing a segment or sample of the whole population (Emory, & William,
1976). The analysis normally focuses on the behavior and characteristics of the given population.
The method for sampling uses probability and non-probability sampling techniques and
moreover, a good sampling design must be measurable, goal oriented, cheap to handle and must
also be practical.
Probability Sample
One of the probability sampling technique is simple random. It involves all samples
exhibiting equal chance to be selected and they include for instance, drawing of the card,
throwing of the die, tossing a coin to determine the sample selection procedure (Parkinson,
&Drislane, 2011). Tossing a coin and using the head to determine sample selection is one among
the best and efficient sampling methods since it do not involve difficult processes (Festinger,
Leon &, Katz, Daniel 1976).
The procedures involved when using simple random sampling technique include
Defining of the population from which to draw the sample
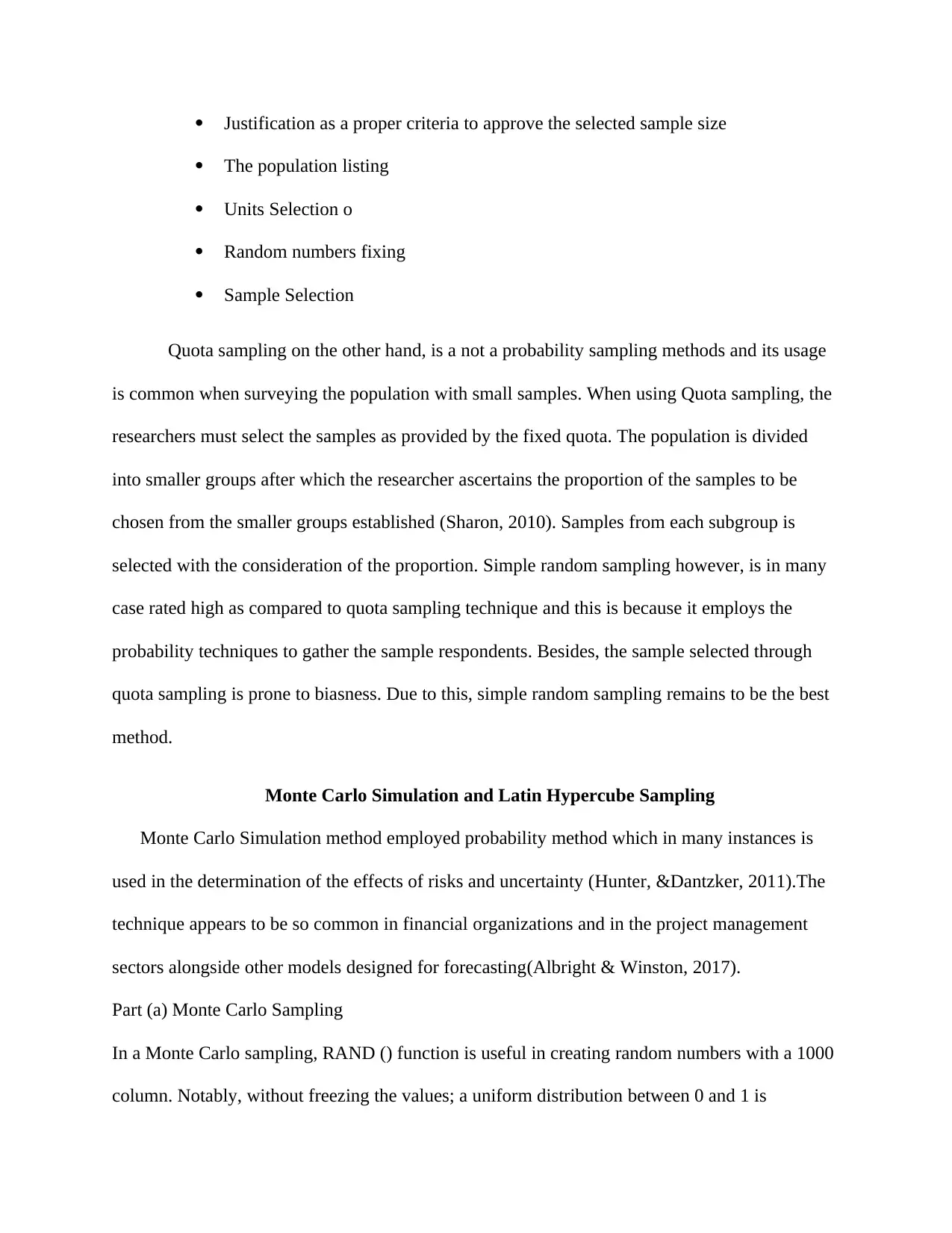
Justification as a proper criteria to approve the selected sample size
The population listing
Units Selection o
Random numbers fixing
Sample Selection
Quota sampling on the other hand, is a not a probability sampling methods and its usage
is common when surveying the population with small samples. When using Quota sampling, the
researchers must select the samples as provided by the fixed quota. The population is divided
into smaller groups after which the researcher ascertains the proportion of the samples to be
chosen from the smaller groups established (Sharon, 2010). Samples from each subgroup is
selected with the consideration of the proportion. Simple random sampling however, is in many
case rated high as compared to quota sampling technique and this is because it employs the
probability techniques to gather the sample respondents. Besides, the sample selected through
quota sampling is prone to biasness. Due to this, simple random sampling remains to be the best
method.
Monte Carlo Simulation and Latin Hypercube Sampling
Monte Carlo Simulation method employed probability method which in many instances is
used in the determination of the effects of risks and uncertainty (Hunter, &Dantzker, 2011).The
technique appears to be so common in financial organizations and in the project management
sectors alongside other models designed for forecasting(Albright & Winston, 2017).
Part (a) Monte Carlo Sampling
In a Monte Carlo sampling, RAND () function is useful in creating random numbers with a 1000
column. Notably, without freezing the values; a uniform distribution between 0 and 1 is
The population listing
Units Selection o
Random numbers fixing
Sample Selection
Quota sampling on the other hand, is a not a probability sampling methods and its usage
is common when surveying the population with small samples. When using Quota sampling, the
researchers must select the samples as provided by the fixed quota. The population is divided
into smaller groups after which the researcher ascertains the proportion of the samples to be
chosen from the smaller groups established (Sharon, 2010). Samples from each subgroup is
selected with the consideration of the proportion. Simple random sampling however, is in many
case rated high as compared to quota sampling technique and this is because it employs the
probability techniques to gather the sample respondents. Besides, the sample selected through
quota sampling is prone to biasness. Due to this, simple random sampling remains to be the best
method.
Monte Carlo Simulation and Latin Hypercube Sampling
Monte Carlo Simulation method employed probability method which in many instances is
used in the determination of the effects of risks and uncertainty (Hunter, &Dantzker, 2011).The
technique appears to be so common in financial organizations and in the project management
sectors alongside other models designed for forecasting(Albright & Winston, 2017).
Part (a) Monte Carlo Sampling
In a Monte Carlo sampling, RAND () function is useful in creating random numbers with a 1000
column. Notably, without freezing the values; a uniform distribution between 0 and 1 is
Secure Best Marks with AI Grader
Need help grading? Try our AI Grader for instant feedback on your assignments.
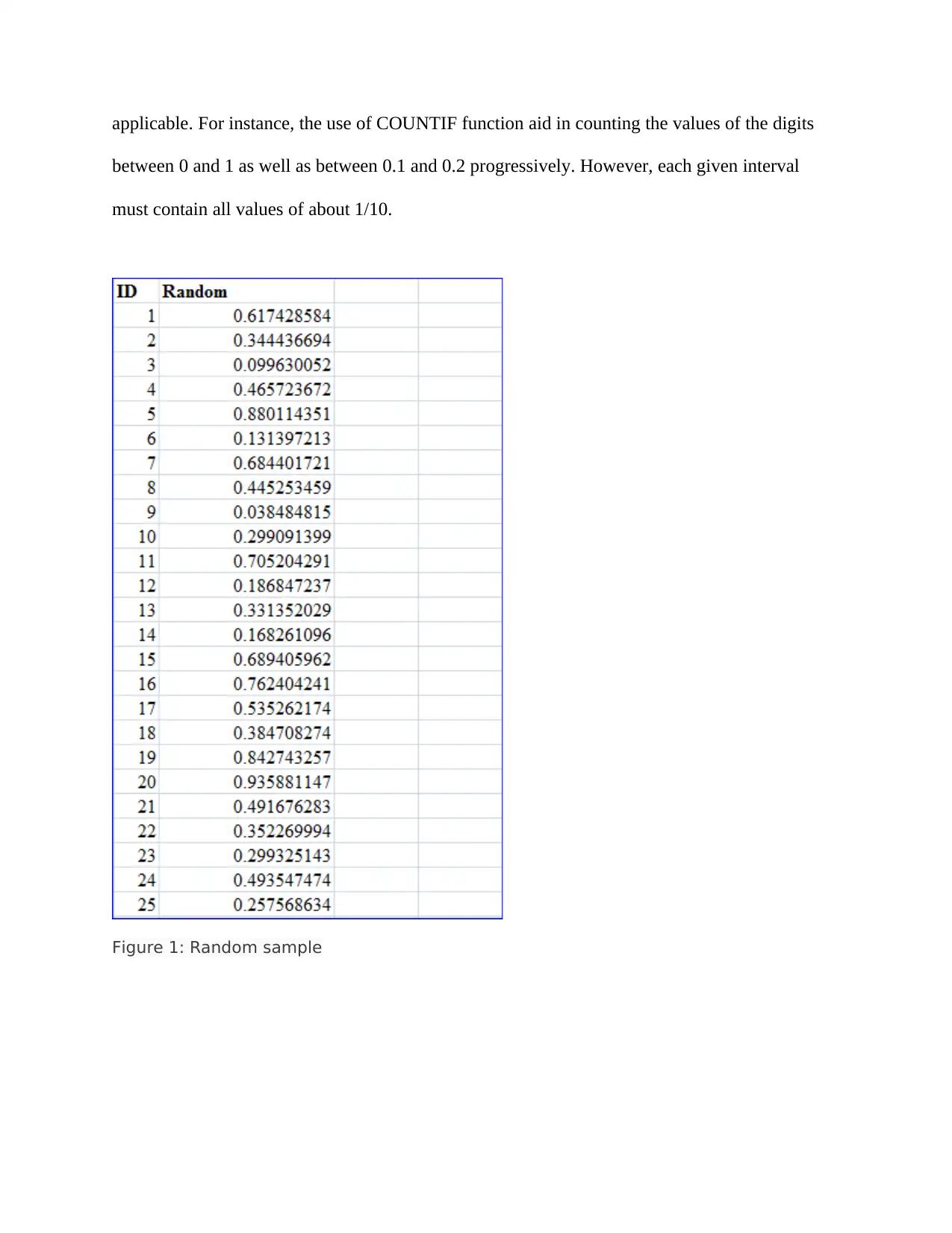
applicable. For instance, the use of COUNTIF function aid in counting the values of the digits
between 0 and 1 as well as between 0.1 and 0.2 progressively. However, each given interval
must contain all values of about 1/10.
Figure 1: Random sample
between 0 and 1 as well as between 0.1 and 0.2 progressively. However, each given interval
must contain all values of about 1/10.
Figure 1: Random sample
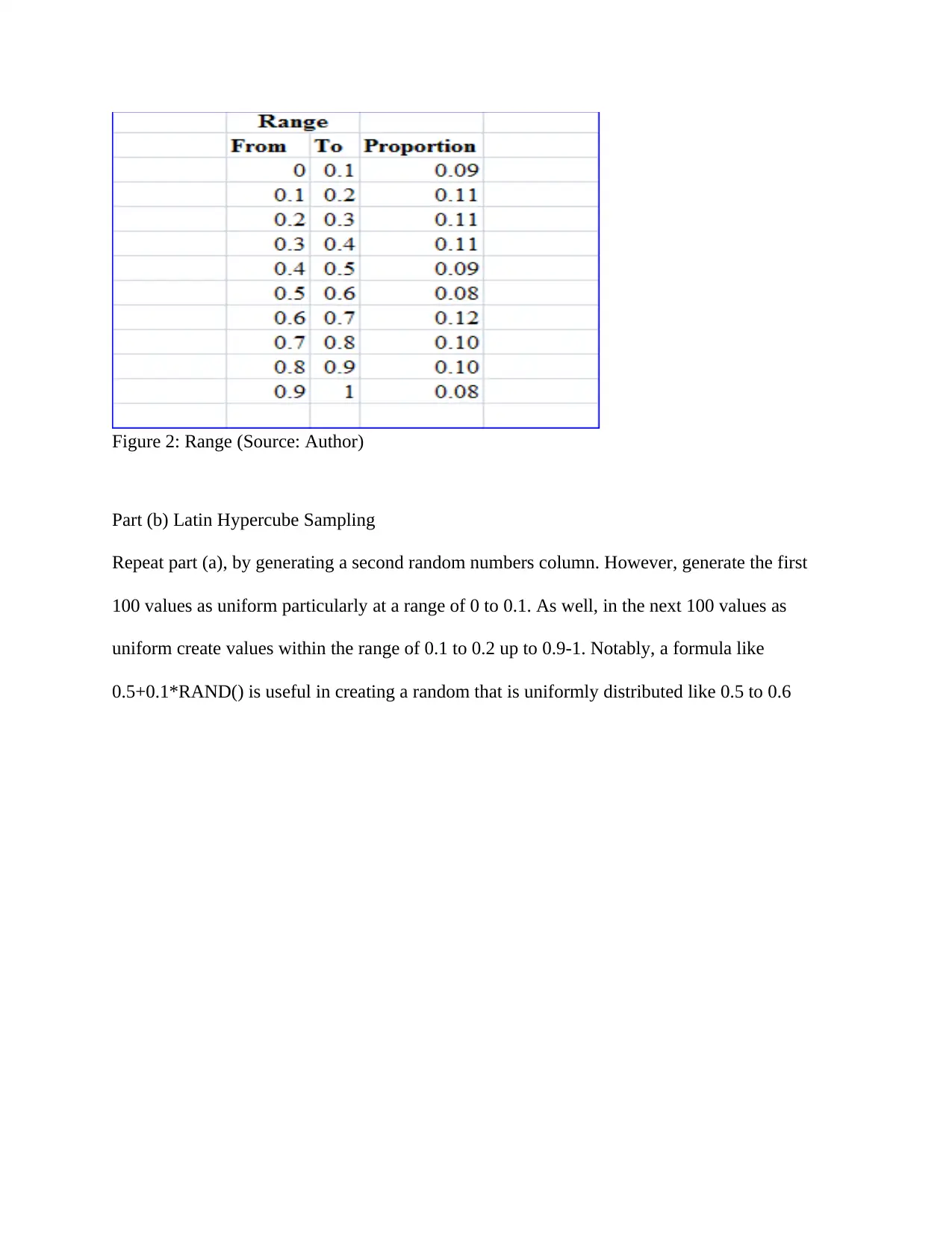
Figure 2: Range (Source: Author)
Part (b) Latin Hypercube Sampling
Repeat part (a), by generating a second random numbers column. However, generate the first
100 values as uniform particularly at a range of 0 to 0.1. As well, in the next 100 values as
uniform create values within the range of 0.1 to 0.2 up to 0.9-1. Notably, a formula like
0.5+0.1*RAND() is useful in creating a random that is uniformly distributed like 0.5 to 0.6
Part (b) Latin Hypercube Sampling
Repeat part (a), by generating a second random numbers column. However, generate the first
100 values as uniform particularly at a range of 0 to 0.1. As well, in the next 100 values as
uniform create values within the range of 0.1 to 0.2 up to 0.9-1. Notably, a formula like
0.5+0.1*RAND() is useful in creating a random that is uniformly distributed like 0.5 to 0.6
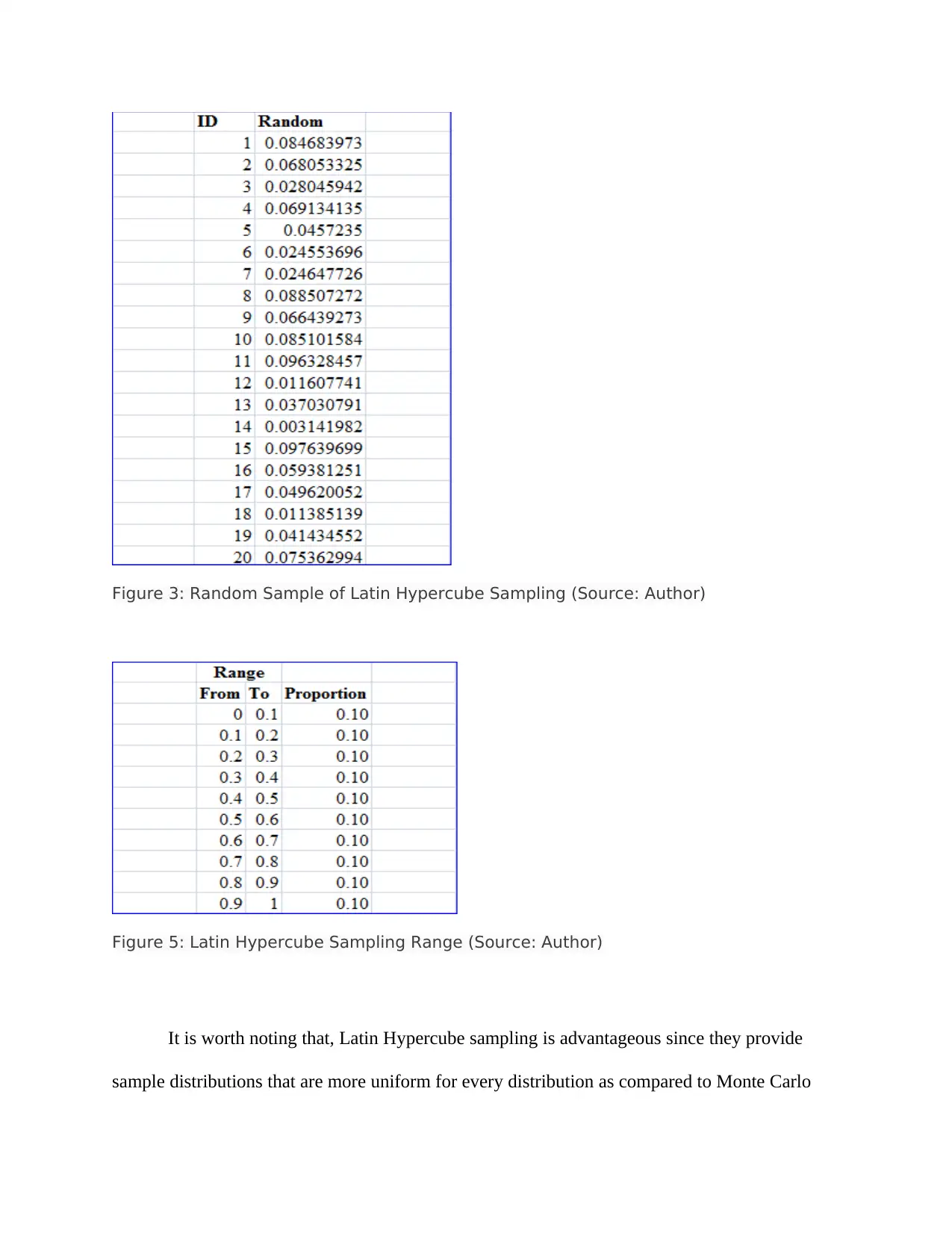
Figure 3: Random Sample of Latin Hypercube Sampling (Source: Author)
Figure 5: Latin Hypercube Sampling Range (Source: Author)
It is worth noting that, Latin Hypercube sampling is advantageous since they provide
sample distributions that are more uniform for every distribution as compared to Monte Carlo
Figure 5: Latin Hypercube Sampling Range (Source: Author)
It is worth noting that, Latin Hypercube sampling is advantageous since they provide
sample distributions that are more uniform for every distribution as compared to Monte Carlo
Paraphrase This Document
Need a fresh take? Get an instant paraphrase of this document with our AI Paraphraser
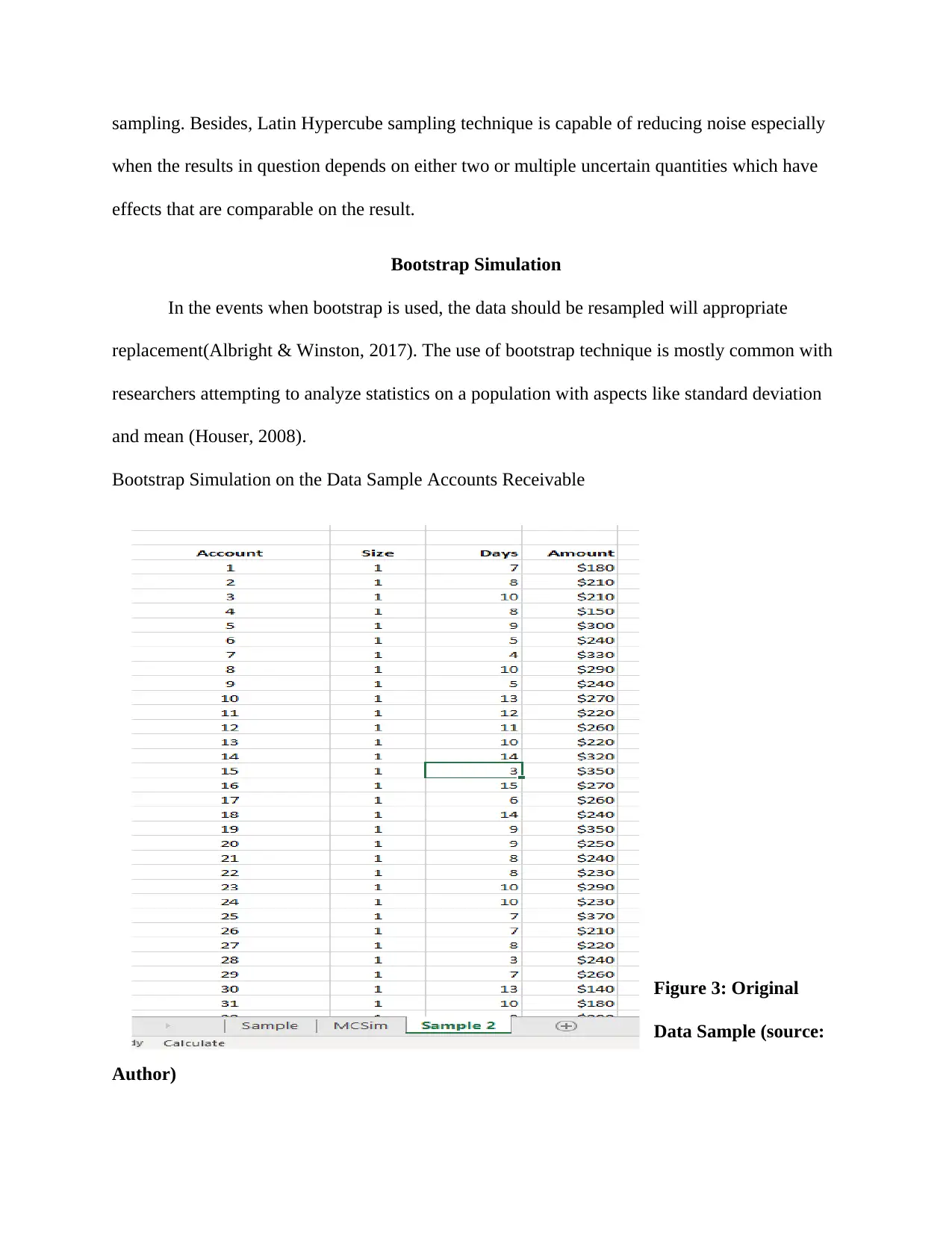
sampling. Besides, Latin Hypercube sampling technique is capable of reducing noise especially
when the results in question depends on either two or multiple uncertain quantities which have
effects that are comparable on the result.
Bootstrap Simulation
In the events when bootstrap is used, the data should be resampled will appropriate
replacement(Albright & Winston, 2017). The use of bootstrap technique is mostly common with
researchers attempting to analyze statistics on a population with aspects like standard deviation
and mean (Houser, 2008).
Bootstrap Simulation on the Data Sample Accounts Receivable
Figure 3: Original
Data Sample (source:
Author)
when the results in question depends on either two or multiple uncertain quantities which have
effects that are comparable on the result.
Bootstrap Simulation
In the events when bootstrap is used, the data should be resampled will appropriate
replacement(Albright & Winston, 2017). The use of bootstrap technique is mostly common with
researchers attempting to analyze statistics on a population with aspects like standard deviation
and mean (Houser, 2008).
Bootstrap Simulation on the Data Sample Accounts Receivable
Figure 3: Original
Data Sample (source:
Author)
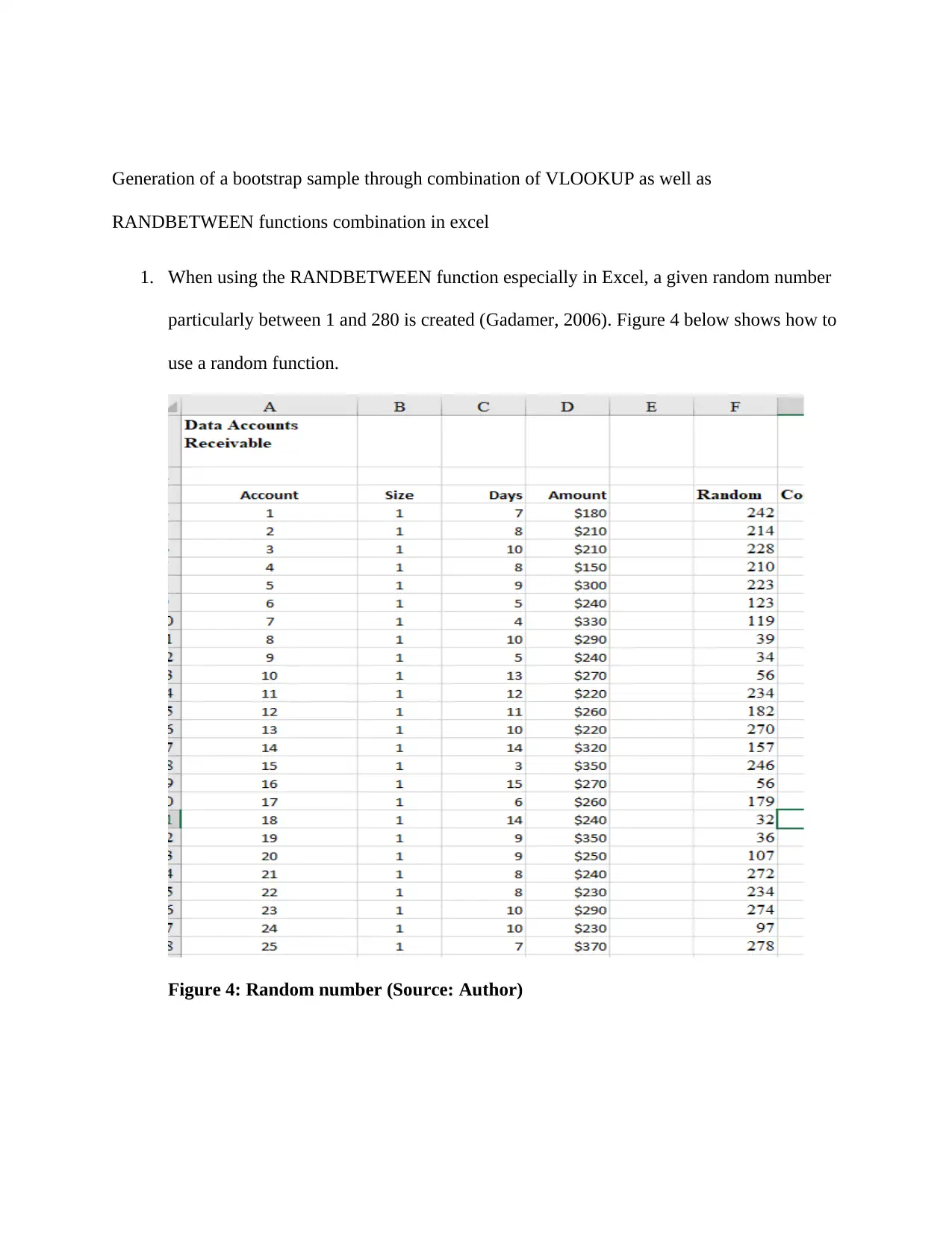
Generation of a bootstrap sample through combination of VLOOKUP as well as
RANDBETWEEN functions combination in excel
1. When using the RANDBETWEEN function especially in Excel, a given random number
particularly between 1 and 280 is created (Gadamer, 2006). Figure 4 below shows how to
use a random function.
Figure 4: Random number (Source: Author)
RANDBETWEEN functions combination in excel
1. When using the RANDBETWEEN function especially in Excel, a given random number
particularly between 1 and 280 is created (Gadamer, 2006). Figure 4 below shows how to
use a random function.
Figure 4: Random number (Source: Author)
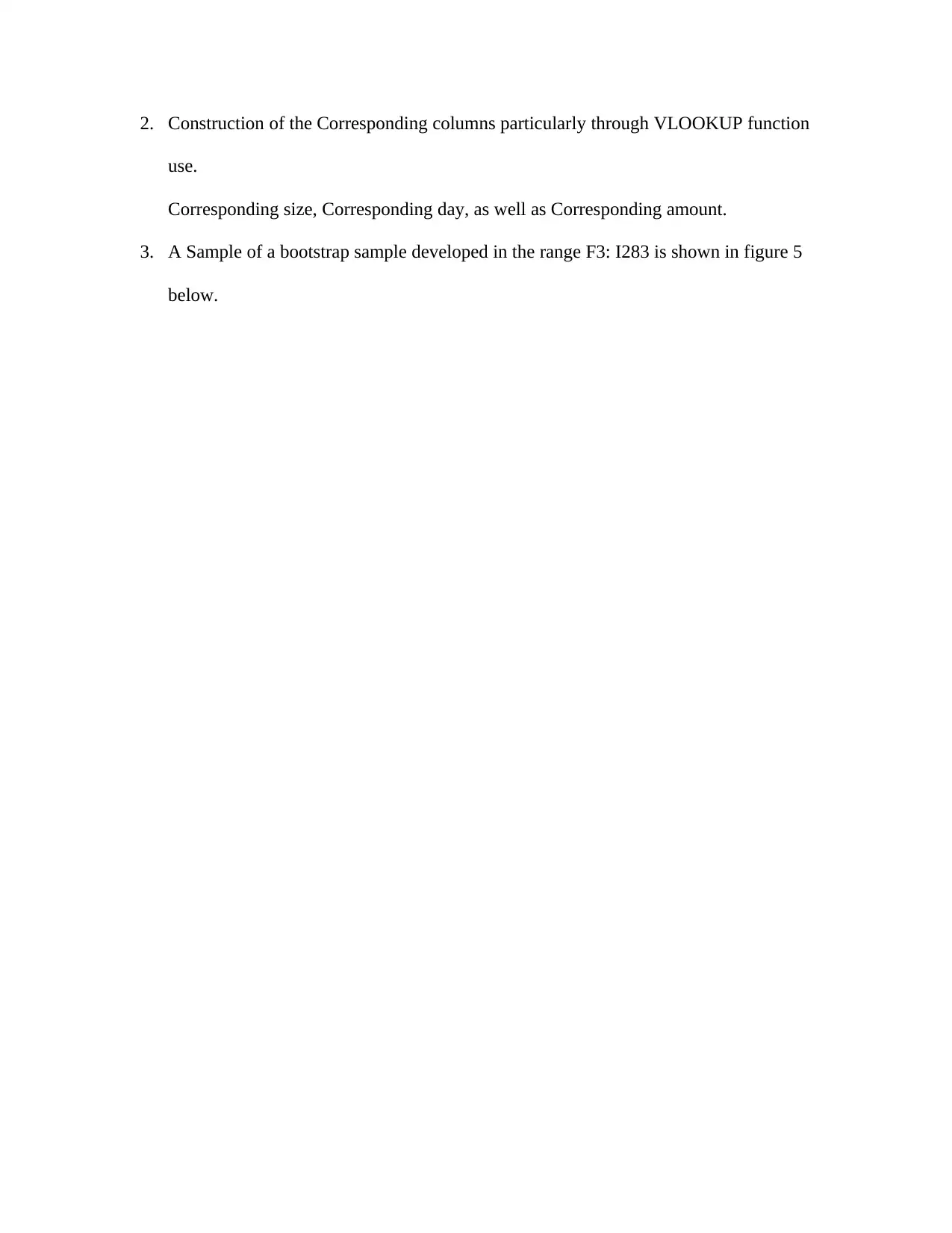
2. Construction of the Corresponding columns particularly through VLOOKUP function
use.
Corresponding size, Corresponding day, as well as Corresponding amount.
3. A Sample of a bootstrap sample developed in the range F3: I283 is shown in figure 5
below.
use.
Corresponding size, Corresponding day, as well as Corresponding amount.
3. A Sample of a bootstrap sample developed in the range F3: I283 is shown in figure 5
below.
Secure Best Marks with AI Grader
Need help grading? Try our AI Grader for instant feedback on your assignments.
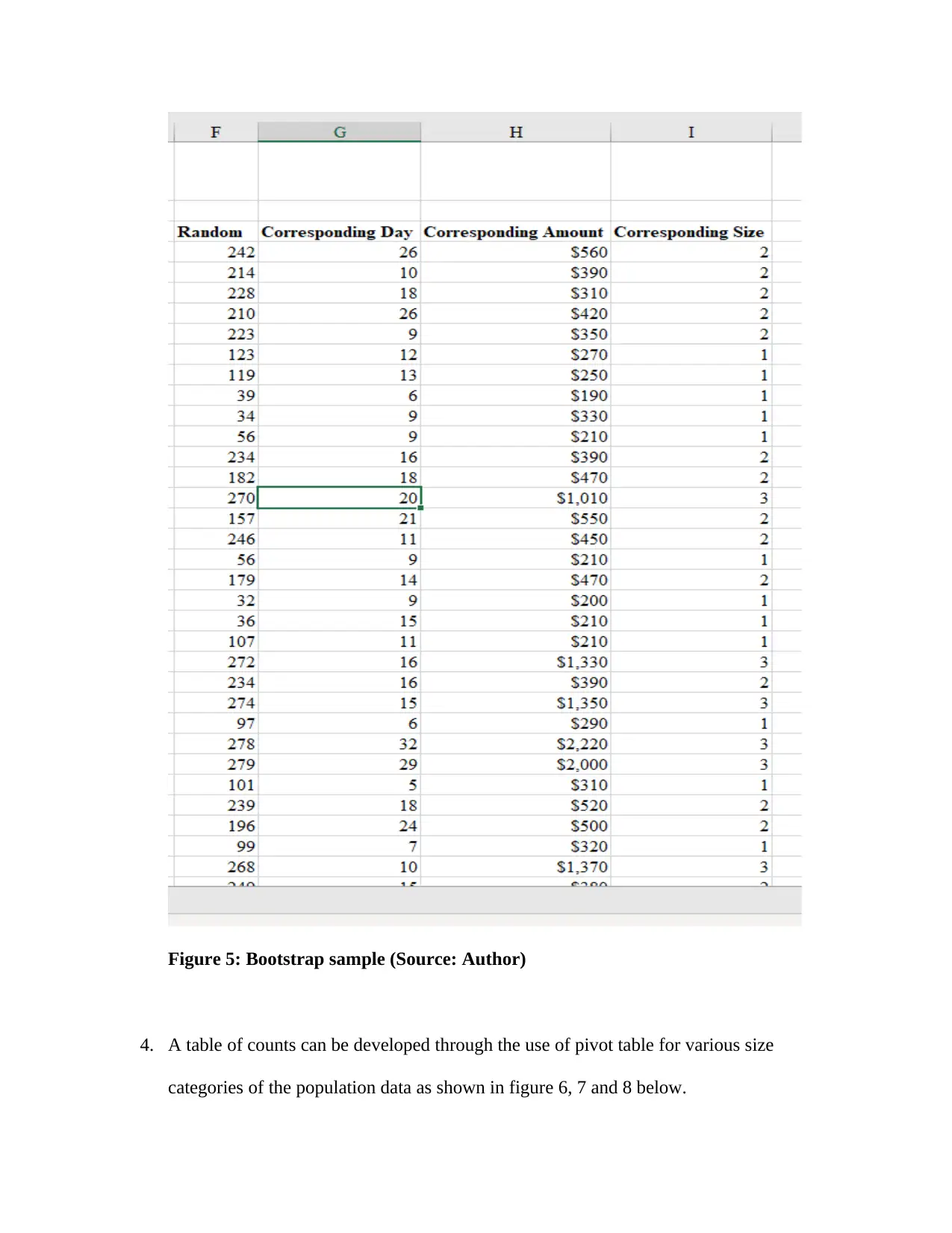
Figure 5: Bootstrap sample (Source: Author)
4. A table of counts can be developed through the use of pivot table for various size
categories of the population data as shown in figure 6, 7 and 8 below.
4. A table of counts can be developed through the use of pivot table for various size
categories of the population data as shown in figure 6, 7 and 8 below.
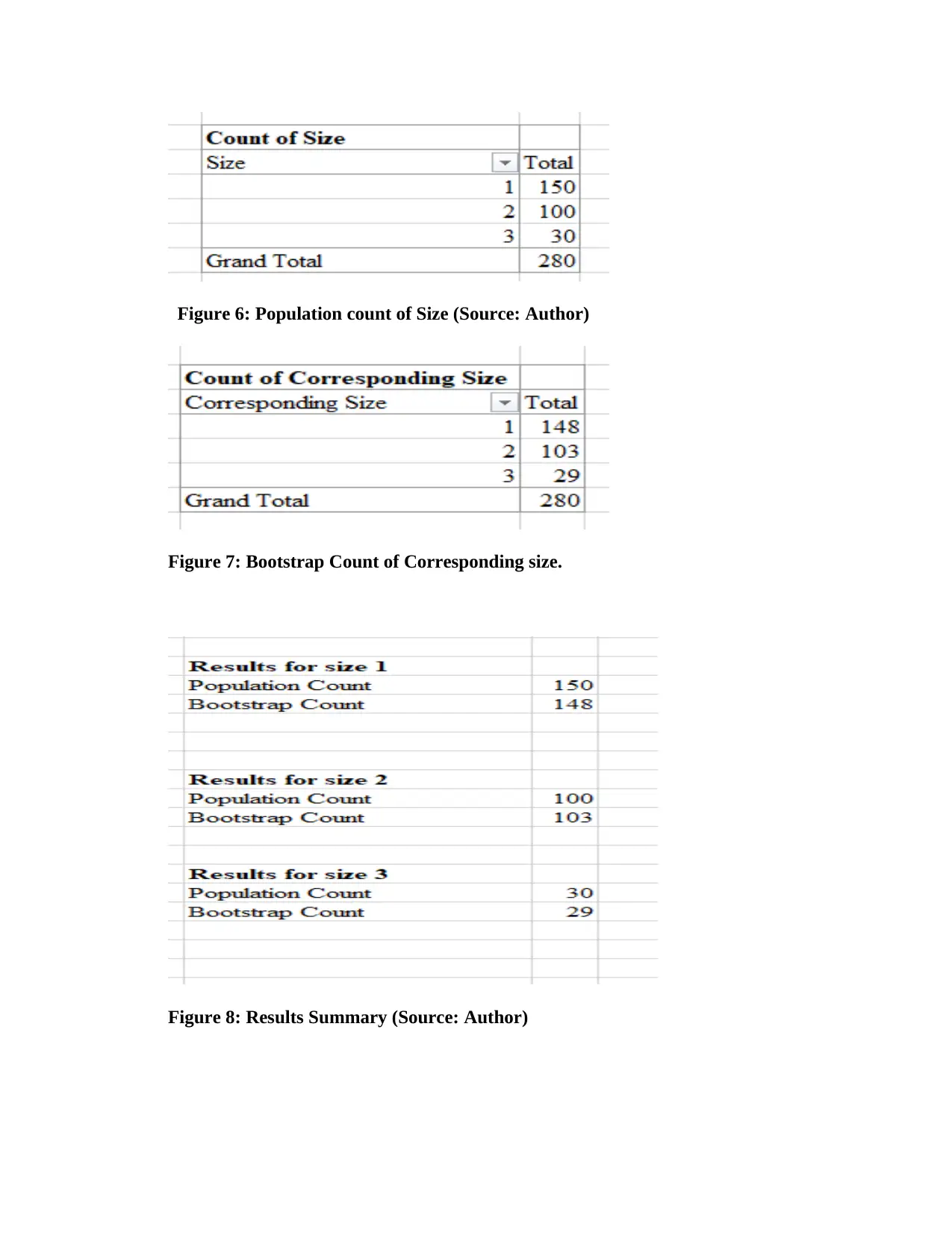
Figure 6: Population count of Size (Source: Author)
Figure 7: Bootstrap Count of Corresponding size.
Figure 8: Results Summary (Source: Author)
Figure 7: Bootstrap Count of Corresponding size.
Figure 8: Results Summary (Source: Author)
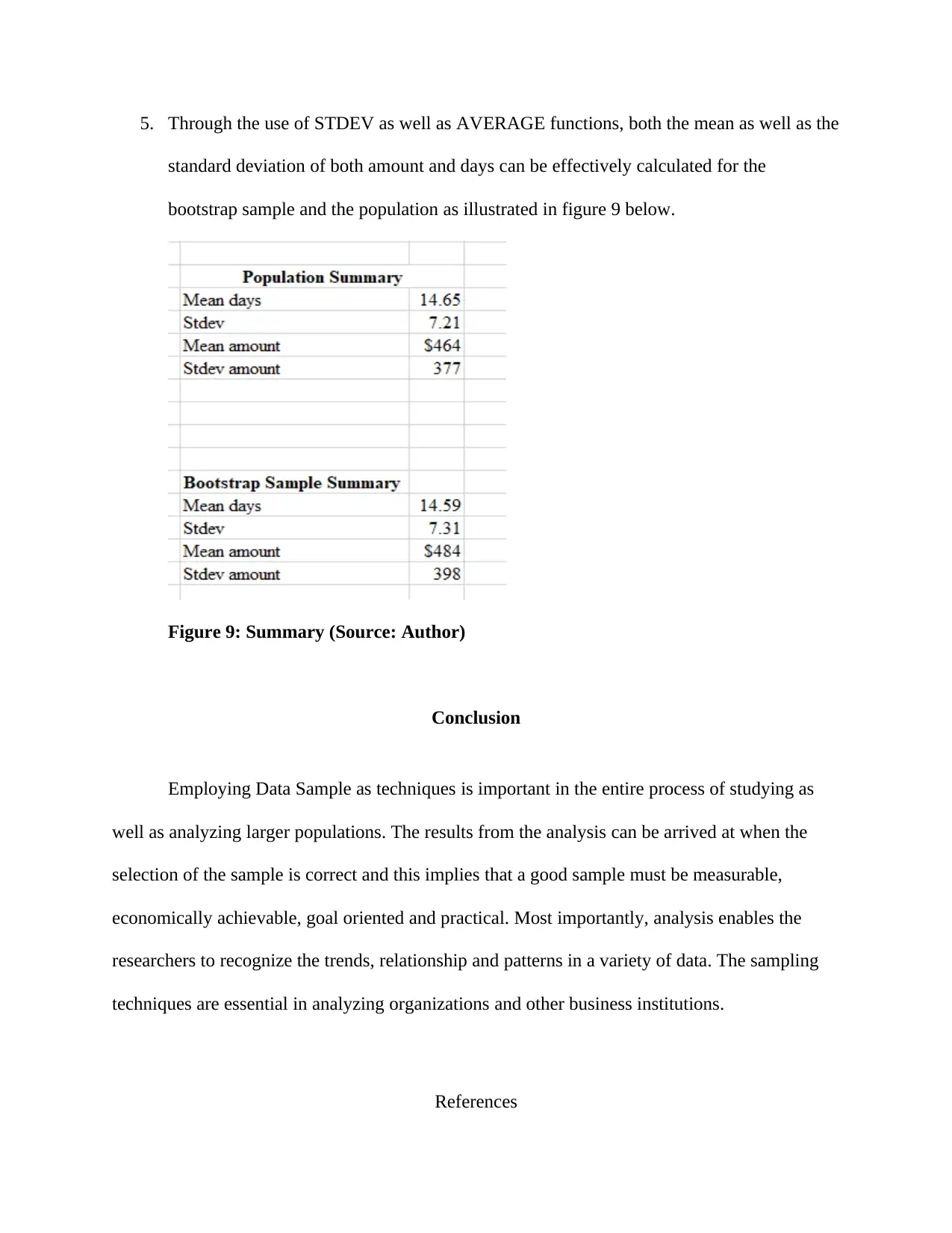
5. Through the use of STDEV as well as AVERAGE functions, both the mean as well as the
standard deviation of both amount and days can be effectively calculated for the
bootstrap sample and the population as illustrated in figure 9 below.
Figure 9: Summary (Source: Author)
Conclusion
Employing Data Sample as techniques is important in the entire process of studying as
well as analyzing larger populations. The results from the analysis can be arrived at when the
selection of the sample is correct and this implies that a good sample must be measurable,
economically achievable, goal oriented and practical. Most importantly, analysis enables the
researchers to recognize the trends, relationship and patterns in a variety of data. The sampling
techniques are essential in analyzing organizations and other business institutions.
References
standard deviation of both amount and days can be effectively calculated for the
bootstrap sample and the population as illustrated in figure 9 below.
Figure 9: Summary (Source: Author)
Conclusion
Employing Data Sample as techniques is important in the entire process of studying as
well as analyzing larger populations. The results from the analysis can be arrived at when the
selection of the sample is correct and this implies that a good sample must be measurable,
economically achievable, goal oriented and practical. Most importantly, analysis enables the
researchers to recognize the trends, relationship and patterns in a variety of data. The sampling
techniques are essential in analyzing organizations and other business institutions.
References
Paraphrase This Document
Need a fresh take? Get an instant paraphrase of this document with our AI Paraphraser
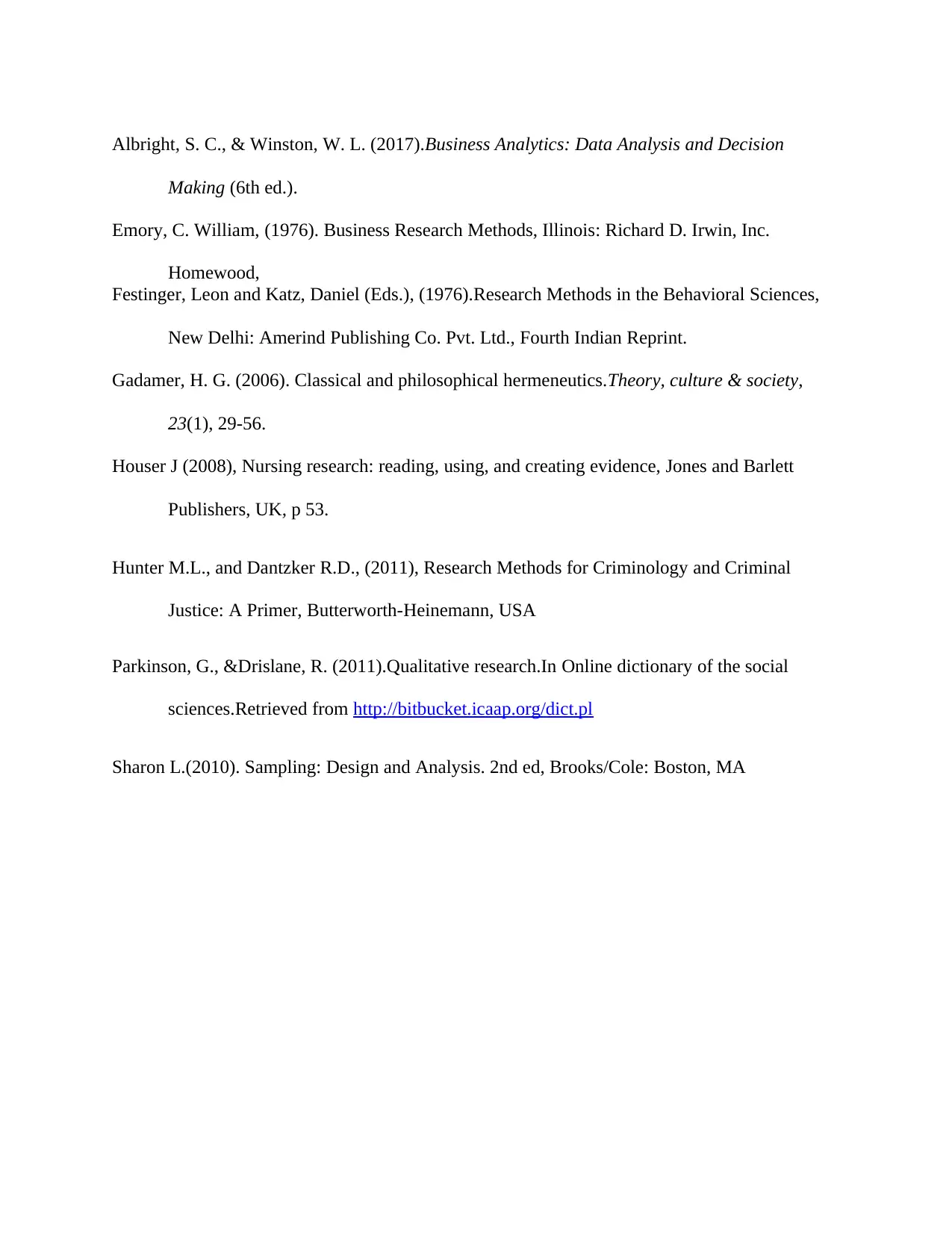
Albright, S. C., & Winston, W. L. (2017).Business Analytics: Data Analysis and Decision
Making (6th ed.).
Emory, C. William, (1976). Business Research Methods, Illinois: Richard D. Irwin, Inc.
Homewood,
Festinger, Leon and Katz, Daniel (Eds.), (1976).Research Methods in the Behavioral Sciences,
New Delhi: Amerind Publishing Co. Pvt. Ltd., Fourth Indian Reprint.
Gadamer, H. G. (2006). Classical and philosophical hermeneutics.Theory, culture & society,
23(1), 29-56.
Houser J (2008), Nursing research: reading, using, and creating evidence, Jones and Barlett
Publishers, UK, p 53.
Hunter M.L., and Dantzker R.D., (2011), Research Methods for Criminology and Criminal
Justice: A Primer, Butterworth-Heinemann, USA
Parkinson, G., &Drislane, R. (2011).Qualitative research.In Online dictionary of the social
sciences.Retrieved from http://bitbucket.icaap.org/dict.pl
Sharon L.(2010). Sampling: Design and Analysis. 2nd ed, Brooks/Cole: Boston, MA
Making (6th ed.).
Emory, C. William, (1976). Business Research Methods, Illinois: Richard D. Irwin, Inc.
Homewood,
Festinger, Leon and Katz, Daniel (Eds.), (1976).Research Methods in the Behavioral Sciences,
New Delhi: Amerind Publishing Co. Pvt. Ltd., Fourth Indian Reprint.
Gadamer, H. G. (2006). Classical and philosophical hermeneutics.Theory, culture & society,
23(1), 29-56.
Houser J (2008), Nursing research: reading, using, and creating evidence, Jones and Barlett
Publishers, UK, p 53.
Hunter M.L., and Dantzker R.D., (2011), Research Methods for Criminology and Criminal
Justice: A Primer, Butterworth-Heinemann, USA
Parkinson, G., &Drislane, R. (2011).Qualitative research.In Online dictionary of the social
sciences.Retrieved from http://bitbucket.icaap.org/dict.pl
Sharon L.(2010). Sampling: Design and Analysis. 2nd ed, Brooks/Cole: Boston, MA
1 out of 14
Related Documents
![[object Object]](/_next/image/?url=%2F_next%2Fstatic%2Fmedia%2Flogo.6d15ce61.png&w=640&q=75)
Your All-in-One AI-Powered Toolkit for Academic Success.
+13062052269
info@desklib.com
Available 24*7 on WhatsApp / Email
Unlock your academic potential
© 2024 | Zucol Services PVT LTD | All rights reserved.