Assignment on Artificial Intelligence - Pedestrian Detection for the Self-driving Cars
VerifiedAdded on  2022/08/22
|6
|1648
|12
AI Summary
Contribute Materials
Your contribution can guide someone’s learning journey. Share your
documents today.
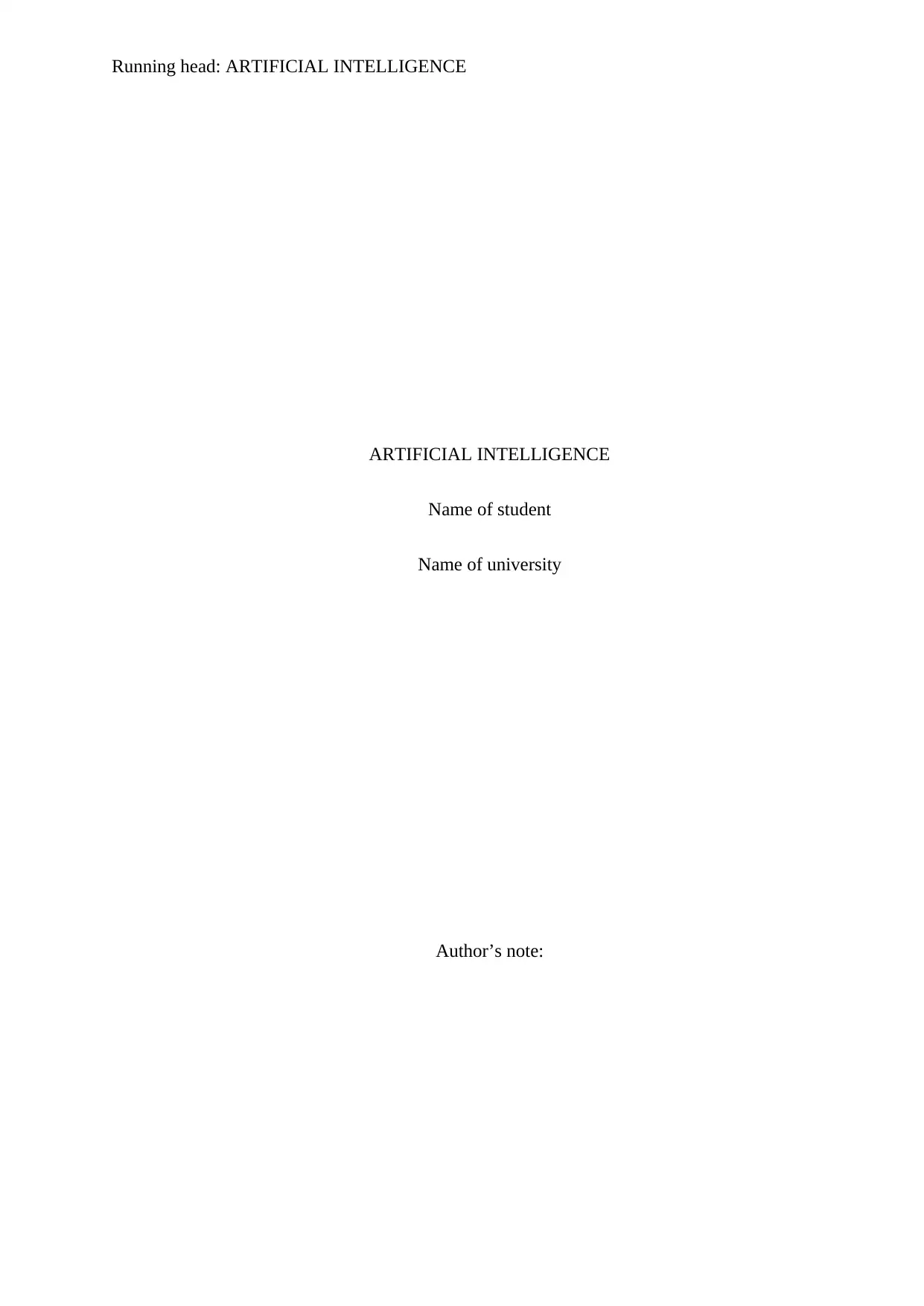
Running head: ARTIFICIAL INTELLIGENCE
ARTIFICIAL INTELLIGENCE
Name of student
Name of university
Author’s note:
ARTIFICIAL INTELLIGENCE
Name of student
Name of university
Author’s note:
Secure Best Marks with AI Grader
Need help grading? Try our AI Grader for instant feedback on your assignments.
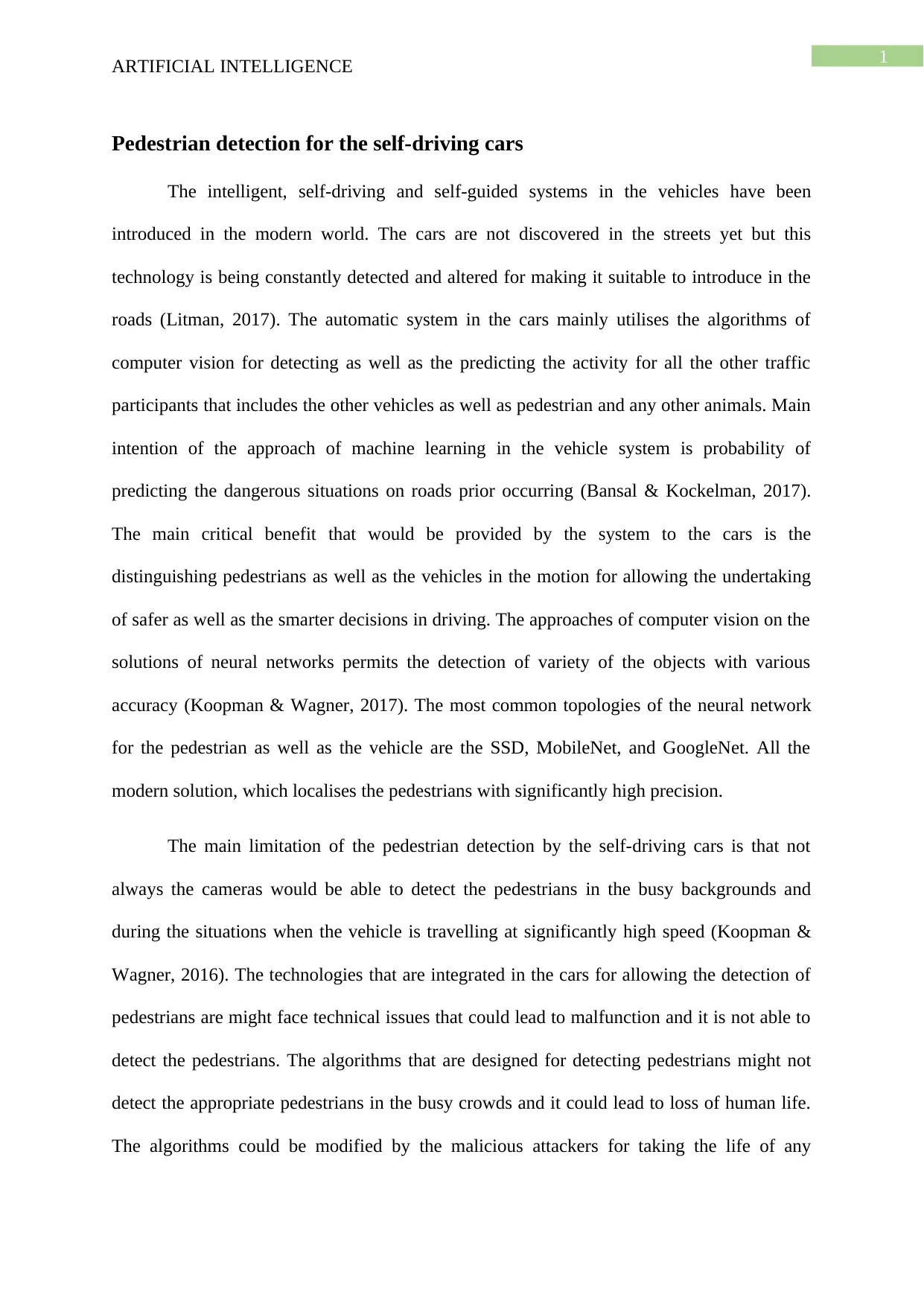
1
ARTIFICIAL INTELLIGENCE
Pedestrian detection for the self-driving cars
The intelligent, self-driving and self-guided systems in the vehicles have been
introduced in the modern world. The cars are not discovered in the streets yet but this
technology is being constantly detected and altered for making it suitable to introduce in the
roads (Litman, 2017). The automatic system in the cars mainly utilises the algorithms of
computer vision for detecting as well as the predicting the activity for all the other traffic
participants that includes the other vehicles as well as pedestrian and any other animals. Main
intention of the approach of machine learning in the vehicle system is probability of
predicting the dangerous situations on roads prior occurring (Bansal & Kockelman, 2017).
The main critical benefit that would be provided by the system to the cars is the
distinguishing pedestrians as well as the vehicles in the motion for allowing the undertaking
of safer as well as the smarter decisions in driving. The approaches of computer vision on the
solutions of neural networks permits the detection of variety of the objects with various
accuracy (Koopman & Wagner, 2017). The most common topologies of the neural network
for the pedestrian as well as the vehicle are the SSD, MobileNet, and GoogleNet. All the
modern solution, which localises the pedestrians with significantly high precision.
The main limitation of the pedestrian detection by the self-driving cars is that not
always the cameras would be able to detect the pedestrians in the busy backgrounds and
during the situations when the vehicle is travelling at significantly high speed (Koopman &
Wagner, 2016). The technologies that are integrated in the cars for allowing the detection of
pedestrians are might face technical issues that could lead to malfunction and it is not able to
detect the pedestrians. The algorithms that are designed for detecting pedestrians might not
detect the appropriate pedestrians in the busy crowds and it could lead to loss of human life.
The algorithms could be modified by the malicious attackers for taking the life of any
ARTIFICIAL INTELLIGENCE
Pedestrian detection for the self-driving cars
The intelligent, self-driving and self-guided systems in the vehicles have been
introduced in the modern world. The cars are not discovered in the streets yet but this
technology is being constantly detected and altered for making it suitable to introduce in the
roads (Litman, 2017). The automatic system in the cars mainly utilises the algorithms of
computer vision for detecting as well as the predicting the activity for all the other traffic
participants that includes the other vehicles as well as pedestrian and any other animals. Main
intention of the approach of machine learning in the vehicle system is probability of
predicting the dangerous situations on roads prior occurring (Bansal & Kockelman, 2017).
The main critical benefit that would be provided by the system to the cars is the
distinguishing pedestrians as well as the vehicles in the motion for allowing the undertaking
of safer as well as the smarter decisions in driving. The approaches of computer vision on the
solutions of neural networks permits the detection of variety of the objects with various
accuracy (Koopman & Wagner, 2017). The most common topologies of the neural network
for the pedestrian as well as the vehicle are the SSD, MobileNet, and GoogleNet. All the
modern solution, which localises the pedestrians with significantly high precision.
The main limitation of the pedestrian detection by the self-driving cars is that not
always the cameras would be able to detect the pedestrians in the busy backgrounds and
during the situations when the vehicle is travelling at significantly high speed (Koopman &
Wagner, 2016). The technologies that are integrated in the cars for allowing the detection of
pedestrians are might face technical issues that could lead to malfunction and it is not able to
detect the pedestrians. The algorithms that are designed for detecting pedestrians might not
detect the appropriate pedestrians in the busy crowds and it could lead to loss of human life.
The algorithms could be modified by the malicious attackers for taking the life of any
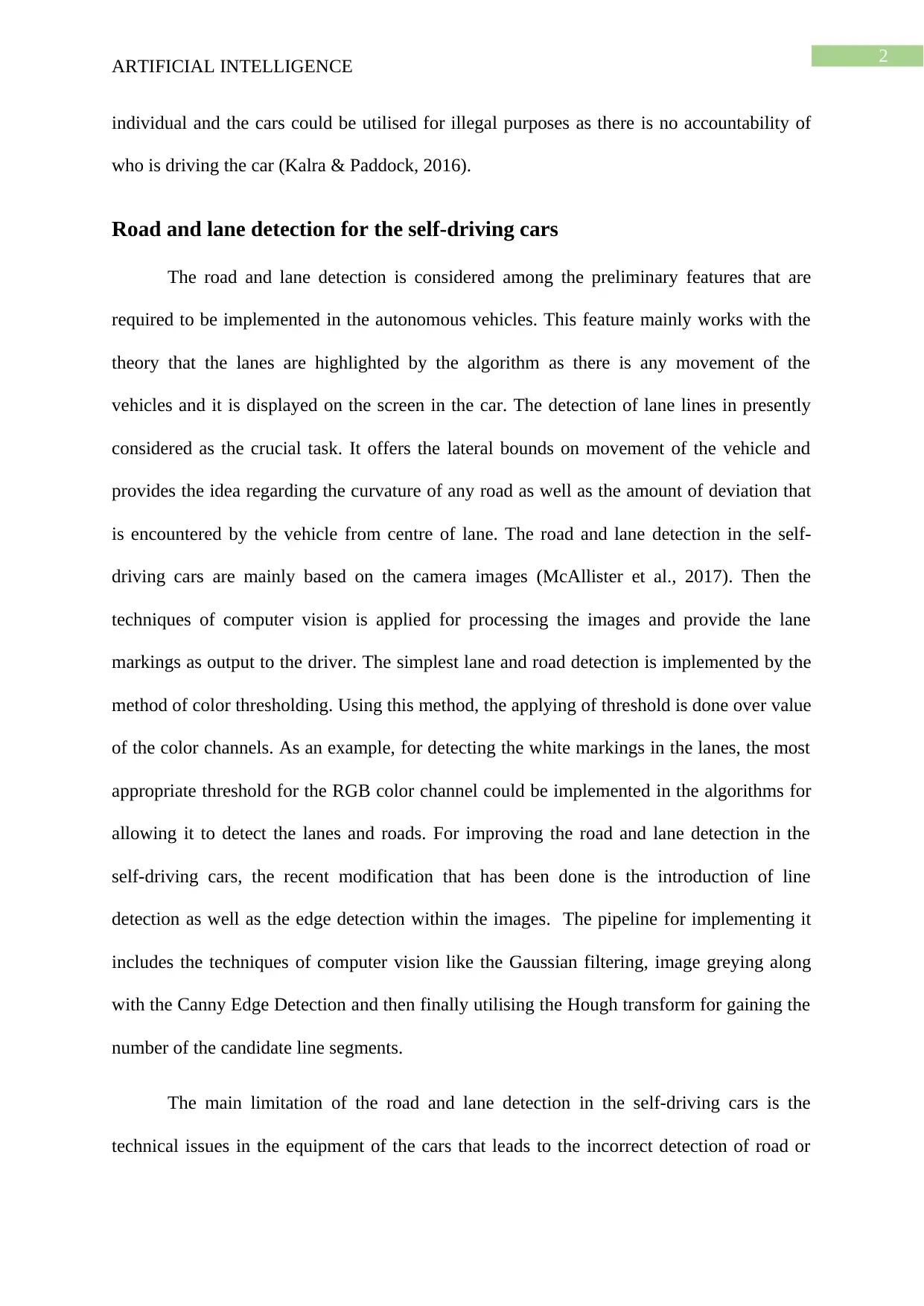
2
ARTIFICIAL INTELLIGENCE
individual and the cars could be utilised for illegal purposes as there is no accountability of
who is driving the car (Kalra & Paddock, 2016).
Road and lane detection for the self-driving cars
The road and lane detection is considered among the preliminary features that are
required to be implemented in the autonomous vehicles. This feature mainly works with the
theory that the lanes are highlighted by the algorithm as there is any movement of the
vehicles and it is displayed on the screen in the car. The detection of lane lines in presently
considered as the crucial task. It offers the lateral bounds on movement of the vehicle and
provides the idea regarding the curvature of any road as well as the amount of deviation that
is encountered by the vehicle from centre of lane. The road and lane detection in the self-
driving cars are mainly based on the camera images (McAllister et al., 2017). Then the
techniques of computer vision is applied for processing the images and provide the lane
markings as output to the driver. The simplest lane and road detection is implemented by the
method of color thresholding. Using this method, the applying of threshold is done over value
of the color channels. As an example, for detecting the white markings in the lanes, the most
appropriate threshold for the RGB color channel could be implemented in the algorithms for
allowing it to detect the lanes and roads. For improving the road and lane detection in the
self-driving cars, the recent modification that has been done is the introduction of line
detection as well as the edge detection within the images. The pipeline for implementing it
includes the techniques of computer vision like the Gaussian filtering, image greying along
with the Canny Edge Detection and then finally utilising the Hough transform for gaining the
number of the candidate line segments.
The main limitation of the road and lane detection in the self-driving cars is the
technical issues in the equipment of the cars that leads to the incorrect detection of road or
ARTIFICIAL INTELLIGENCE
individual and the cars could be utilised for illegal purposes as there is no accountability of
who is driving the car (Kalra & Paddock, 2016).
Road and lane detection for the self-driving cars
The road and lane detection is considered among the preliminary features that are
required to be implemented in the autonomous vehicles. This feature mainly works with the
theory that the lanes are highlighted by the algorithm as there is any movement of the
vehicles and it is displayed on the screen in the car. The detection of lane lines in presently
considered as the crucial task. It offers the lateral bounds on movement of the vehicle and
provides the idea regarding the curvature of any road as well as the amount of deviation that
is encountered by the vehicle from centre of lane. The road and lane detection in the self-
driving cars are mainly based on the camera images (McAllister et al., 2017). Then the
techniques of computer vision is applied for processing the images and provide the lane
markings as output to the driver. The simplest lane and road detection is implemented by the
method of color thresholding. Using this method, the applying of threshold is done over value
of the color channels. As an example, for detecting the white markings in the lanes, the most
appropriate threshold for the RGB color channel could be implemented in the algorithms for
allowing it to detect the lanes and roads. For improving the road and lane detection in the
self-driving cars, the recent modification that has been done is the introduction of line
detection as well as the edge detection within the images. The pipeline for implementing it
includes the techniques of computer vision like the Gaussian filtering, image greying along
with the Canny Edge Detection and then finally utilising the Hough transform for gaining the
number of the candidate line segments.
The main limitation of the road and lane detection in the self-driving cars is the
technical issues in the equipment of the cars that leads to the incorrect detection of road or
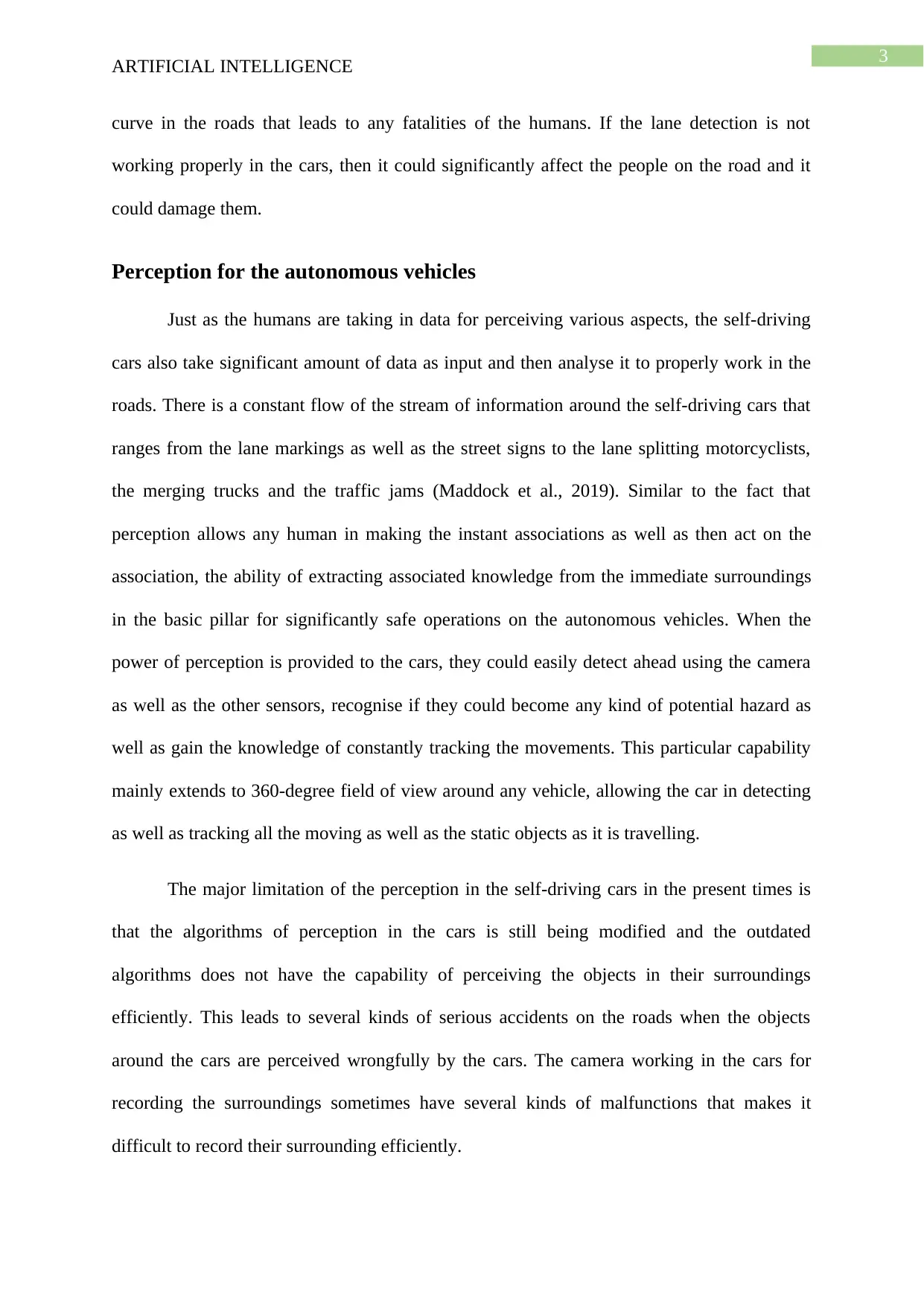
3
ARTIFICIAL INTELLIGENCE
curve in the roads that leads to any fatalities of the humans. If the lane detection is not
working properly in the cars, then it could significantly affect the people on the road and it
could damage them.
Perception for the autonomous vehicles
Just as the humans are taking in data for perceiving various aspects, the self-driving
cars also take significant amount of data as input and then analyse it to properly work in the
roads. There is a constant flow of the stream of information around the self-driving cars that
ranges from the lane markings as well as the street signs to the lane splitting motorcyclists,
the merging trucks and the traffic jams (Maddock et al., 2019). Similar to the fact that
perception allows any human in making the instant associations as well as then act on the
association, the ability of extracting associated knowledge from the immediate surroundings
in the basic pillar for significantly safe operations on the autonomous vehicles. When the
power of perception is provided to the cars, they could easily detect ahead using the camera
as well as the other sensors, recognise if they could become any kind of potential hazard as
well as gain the knowledge of constantly tracking the movements. This particular capability
mainly extends to 360-degree field of view around any vehicle, allowing the car in detecting
as well as tracking all the moving as well as the static objects as it is travelling.
The major limitation of the perception in the self-driving cars in the present times is
that the algorithms of perception in the cars is still being modified and the outdated
algorithms does not have the capability of perceiving the objects in their surroundings
efficiently. This leads to several kinds of serious accidents on the roads when the objects
around the cars are perceived wrongfully by the cars. The camera working in the cars for
recording the surroundings sometimes have several kinds of malfunctions that makes it
difficult to record their surrounding efficiently.
ARTIFICIAL INTELLIGENCE
curve in the roads that leads to any fatalities of the humans. If the lane detection is not
working properly in the cars, then it could significantly affect the people on the road and it
could damage them.
Perception for the autonomous vehicles
Just as the humans are taking in data for perceiving various aspects, the self-driving
cars also take significant amount of data as input and then analyse it to properly work in the
roads. There is a constant flow of the stream of information around the self-driving cars that
ranges from the lane markings as well as the street signs to the lane splitting motorcyclists,
the merging trucks and the traffic jams (Maddock et al., 2019). Similar to the fact that
perception allows any human in making the instant associations as well as then act on the
association, the ability of extracting associated knowledge from the immediate surroundings
in the basic pillar for significantly safe operations on the autonomous vehicles. When the
power of perception is provided to the cars, they could easily detect ahead using the camera
as well as the other sensors, recognise if they could become any kind of potential hazard as
well as gain the knowledge of constantly tracking the movements. This particular capability
mainly extends to 360-degree field of view around any vehicle, allowing the car in detecting
as well as tracking all the moving as well as the static objects as it is travelling.
The major limitation of the perception in the self-driving cars in the present times is
that the algorithms of perception in the cars is still being modified and the outdated
algorithms does not have the capability of perceiving the objects in their surroundings
efficiently. This leads to several kinds of serious accidents on the roads when the objects
around the cars are perceived wrongfully by the cars. The camera working in the cars for
recording the surroundings sometimes have several kinds of malfunctions that makes it
difficult to record their surrounding efficiently.
Secure Best Marks with AI Grader
Need help grading? Try our AI Grader for instant feedback on your assignments.
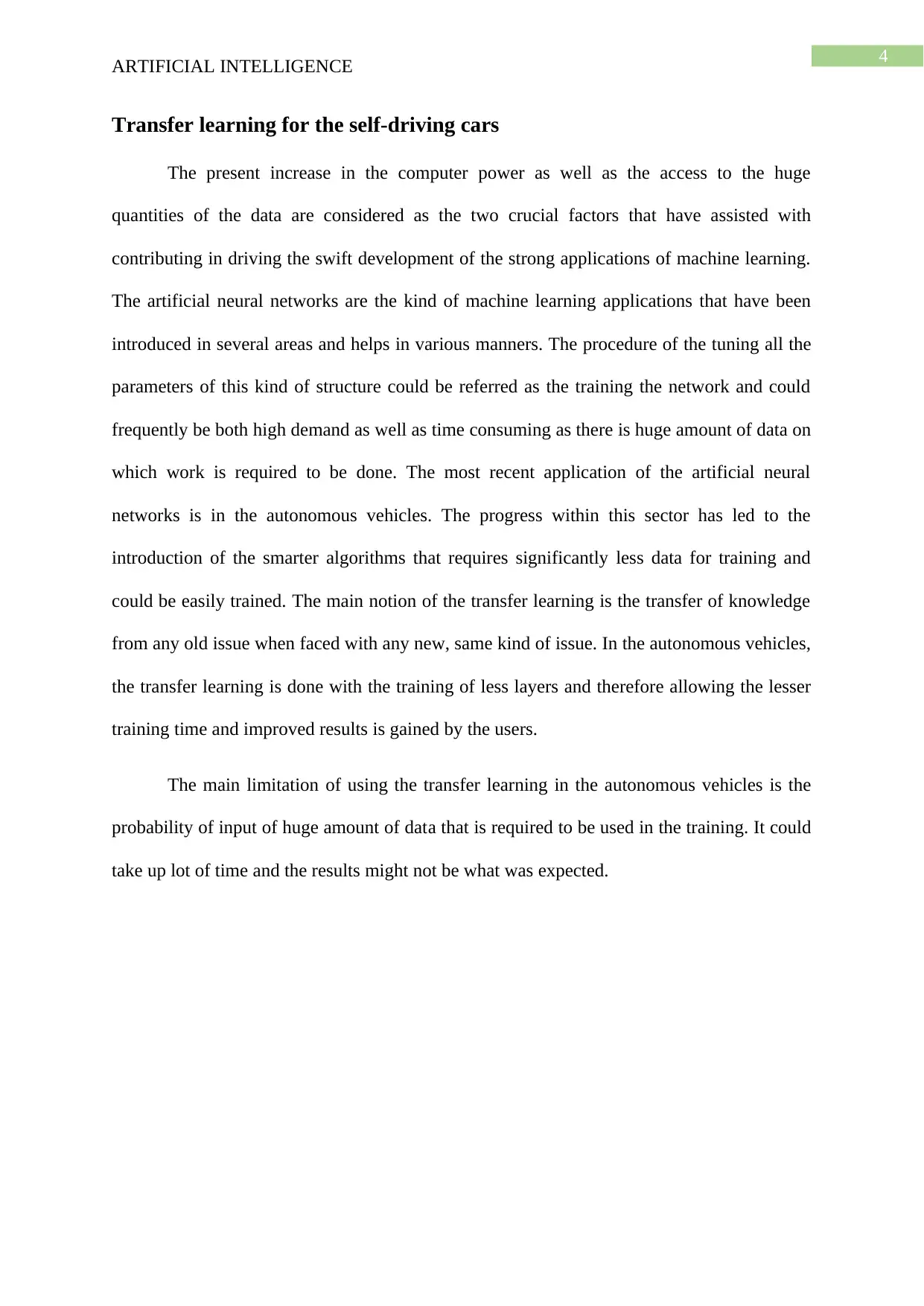
4
ARTIFICIAL INTELLIGENCE
Transfer learning for the self-driving cars
The present increase in the computer power as well as the access to the huge
quantities of the data are considered as the two crucial factors that have assisted with
contributing in driving the swift development of the strong applications of machine learning.
The artificial neural networks are the kind of machine learning applications that have been
introduced in several areas and helps in various manners. The procedure of the tuning all the
parameters of this kind of structure could be referred as the training the network and could
frequently be both high demand as well as time consuming as there is huge amount of data on
which work is required to be done. The most recent application of the artificial neural
networks is in the autonomous vehicles. The progress within this sector has led to the
introduction of the smarter algorithms that requires significantly less data for training and
could be easily trained. The main notion of the transfer learning is the transfer of knowledge
from any old issue when faced with any new, same kind of issue. In the autonomous vehicles,
the transfer learning is done with the training of less layers and therefore allowing the lesser
training time and improved results is gained by the users.
The main limitation of using the transfer learning in the autonomous vehicles is the
probability of input of huge amount of data that is required to be used in the training. It could
take up lot of time and the results might not be what was expected.
ARTIFICIAL INTELLIGENCE
Transfer learning for the self-driving cars
The present increase in the computer power as well as the access to the huge
quantities of the data are considered as the two crucial factors that have assisted with
contributing in driving the swift development of the strong applications of machine learning.
The artificial neural networks are the kind of machine learning applications that have been
introduced in several areas and helps in various manners. The procedure of the tuning all the
parameters of this kind of structure could be referred as the training the network and could
frequently be both high demand as well as time consuming as there is huge amount of data on
which work is required to be done. The most recent application of the artificial neural
networks is in the autonomous vehicles. The progress within this sector has led to the
introduction of the smarter algorithms that requires significantly less data for training and
could be easily trained. The main notion of the transfer learning is the transfer of knowledge
from any old issue when faced with any new, same kind of issue. In the autonomous vehicles,
the transfer learning is done with the training of less layers and therefore allowing the lesser
training time and improved results is gained by the users.
The main limitation of using the transfer learning in the autonomous vehicles is the
probability of input of huge amount of data that is required to be used in the training. It could
take up lot of time and the results might not be what was expected.
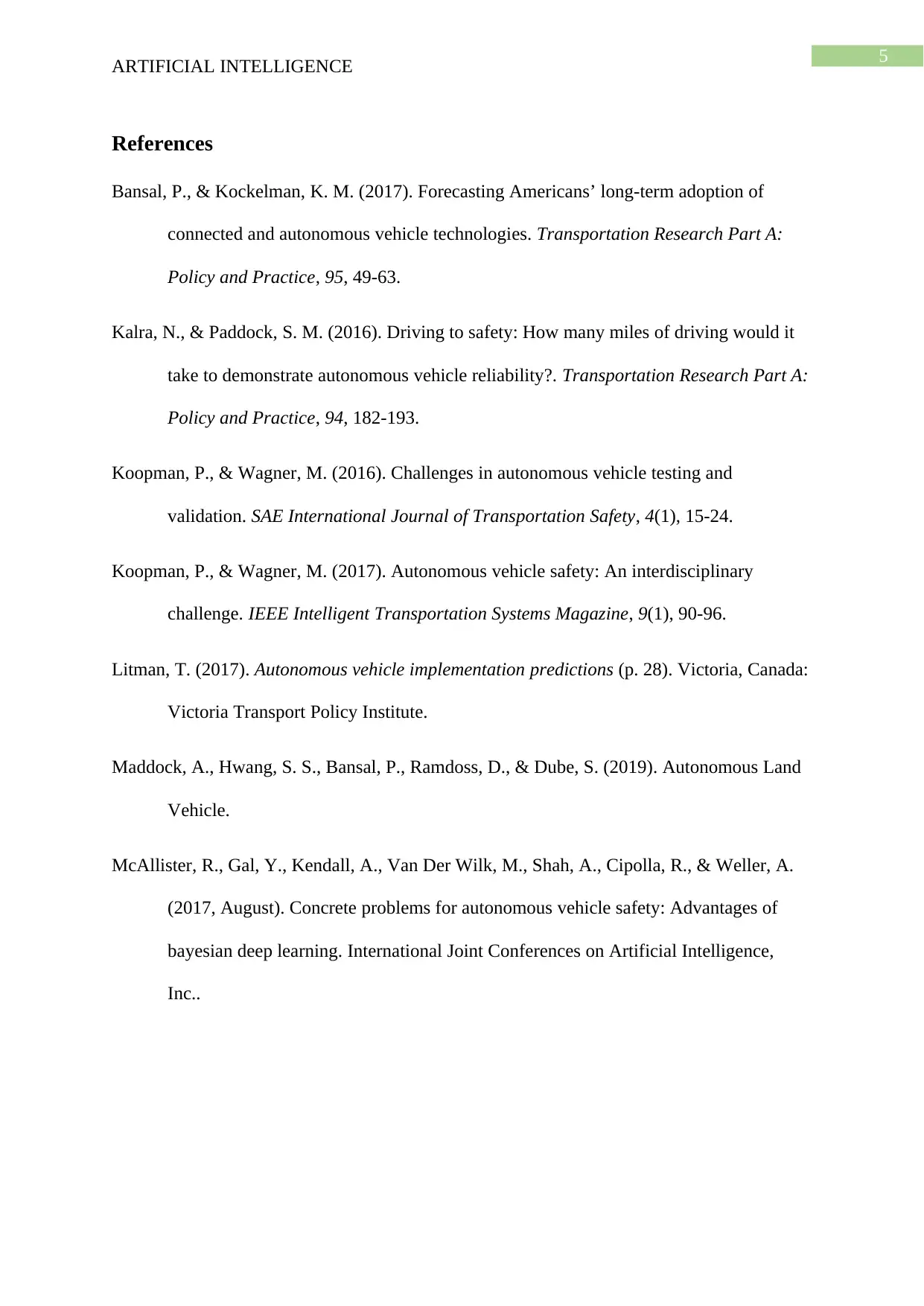
5
ARTIFICIAL INTELLIGENCE
References
Bansal, P., & Kockelman, K. M. (2017). Forecasting Americans’ long-term adoption of
connected and autonomous vehicle technologies. Transportation Research Part A:
Policy and Practice, 95, 49-63.
Kalra, N., & Paddock, S. M. (2016). Driving to safety: How many miles of driving would it
take to demonstrate autonomous vehicle reliability?. Transportation Research Part A:
Policy and Practice, 94, 182-193.
Koopman, P., & Wagner, M. (2016). Challenges in autonomous vehicle testing and
validation. SAE International Journal of Transportation Safety, 4(1), 15-24.
Koopman, P., & Wagner, M. (2017). Autonomous vehicle safety: An interdisciplinary
challenge. IEEE Intelligent Transportation Systems Magazine, 9(1), 90-96.
Litman, T. (2017). Autonomous vehicle implementation predictions (p. 28). Victoria, Canada:
Victoria Transport Policy Institute.
Maddock, A., Hwang, S. S., Bansal, P., Ramdoss, D., & Dube, S. (2019). Autonomous Land
Vehicle.
McAllister, R., Gal, Y., Kendall, A., Van Der Wilk, M., Shah, A., Cipolla, R., & Weller, A.
(2017, August). Concrete problems for autonomous vehicle safety: Advantages of
bayesian deep learning. International Joint Conferences on Artificial Intelligence,
Inc..
ARTIFICIAL INTELLIGENCE
References
Bansal, P., & Kockelman, K. M. (2017). Forecasting Americans’ long-term adoption of
connected and autonomous vehicle technologies. Transportation Research Part A:
Policy and Practice, 95, 49-63.
Kalra, N., & Paddock, S. M. (2016). Driving to safety: How many miles of driving would it
take to demonstrate autonomous vehicle reliability?. Transportation Research Part A:
Policy and Practice, 94, 182-193.
Koopman, P., & Wagner, M. (2016). Challenges in autonomous vehicle testing and
validation. SAE International Journal of Transportation Safety, 4(1), 15-24.
Koopman, P., & Wagner, M. (2017). Autonomous vehicle safety: An interdisciplinary
challenge. IEEE Intelligent Transportation Systems Magazine, 9(1), 90-96.
Litman, T. (2017). Autonomous vehicle implementation predictions (p. 28). Victoria, Canada:
Victoria Transport Policy Institute.
Maddock, A., Hwang, S. S., Bansal, P., Ramdoss, D., & Dube, S. (2019). Autonomous Land
Vehicle.
McAllister, R., Gal, Y., Kendall, A., Van Der Wilk, M., Shah, A., Cipolla, R., & Weller, A.
(2017, August). Concrete problems for autonomous vehicle safety: Advantages of
bayesian deep learning. International Joint Conferences on Artificial Intelligence,
Inc..
1 out of 6
Related Documents
![[object Object]](/_next/image/?url=%2F_next%2Fstatic%2Fmedia%2Flogo.6d15ce61.png&w=640&q=75)
Your All-in-One AI-Powered Toolkit for Academic Success.
 +13062052269
info@desklib.com
Available 24*7 on WhatsApp / Email
Unlock your academic potential
© 2024  |  Zucol Services PVT LTD  |  All rights reserved.