Analysis of Popular Names in Australia
VerifiedAdded on  2023/03/17
|11
|1789
|77
AI Summary
This article analyzes the popularity of names in Australia over the years and provides recommendations for buying stock based on popular names. It discusses the stages of the data life cycle, the use of software for handling big data, and presents charts showing the popularity of different names. The article also suggests collecting additional data from sources such as successful persons' names and stage names of characters to predict future trends in popular names.
Contribute Materials
Your contribution can guide someone’s learning journey. Share your
documents today.
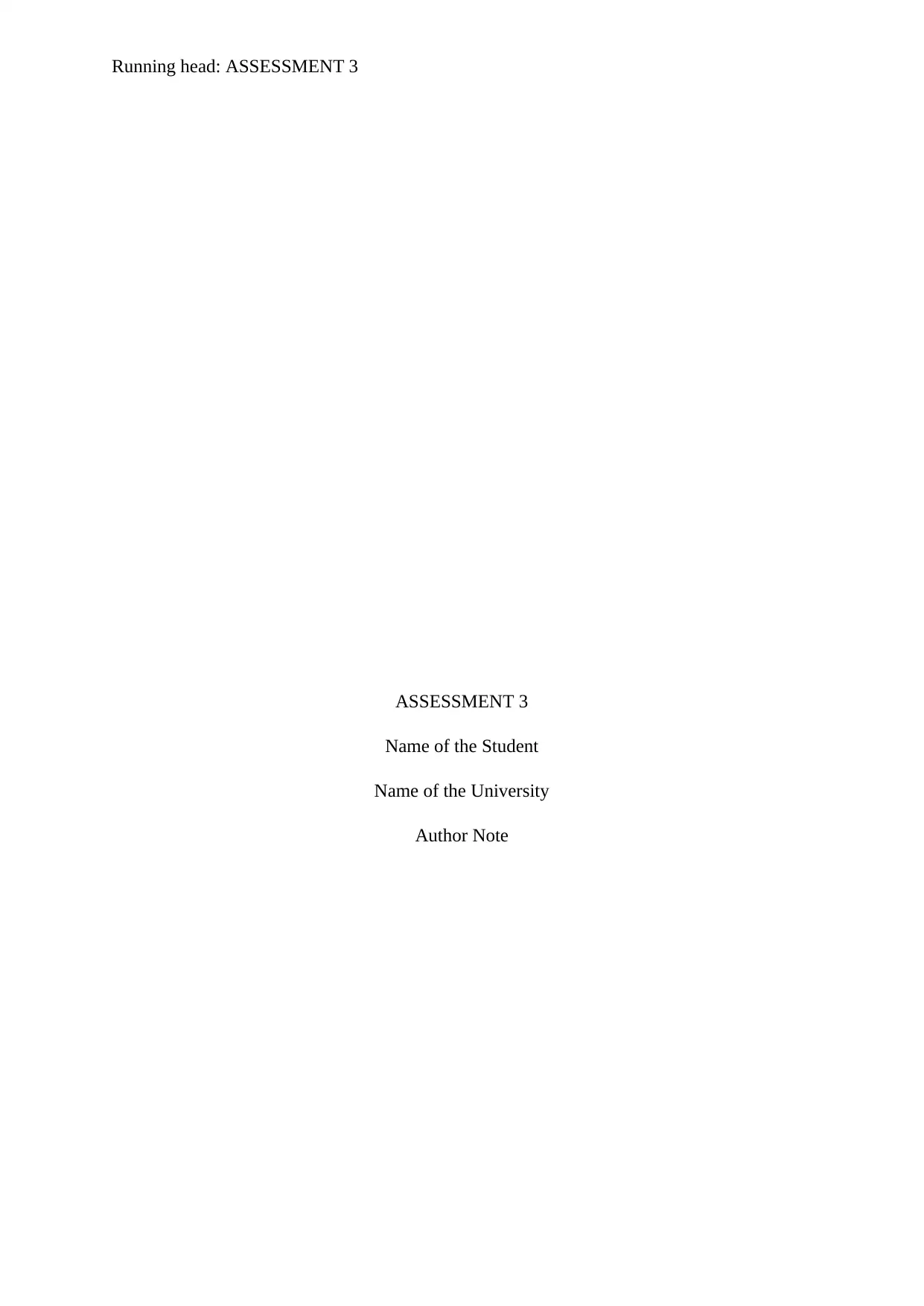
Running head: ASSESSMENT 3
ASSESSMENT 3
Name of the Student
Name of the University
Author Note
ASSESSMENT 3
Name of the Student
Name of the University
Author Note
Secure Best Marks with AI Grader
Need help grading? Try our AI Grader for instant feedback on your assignments.
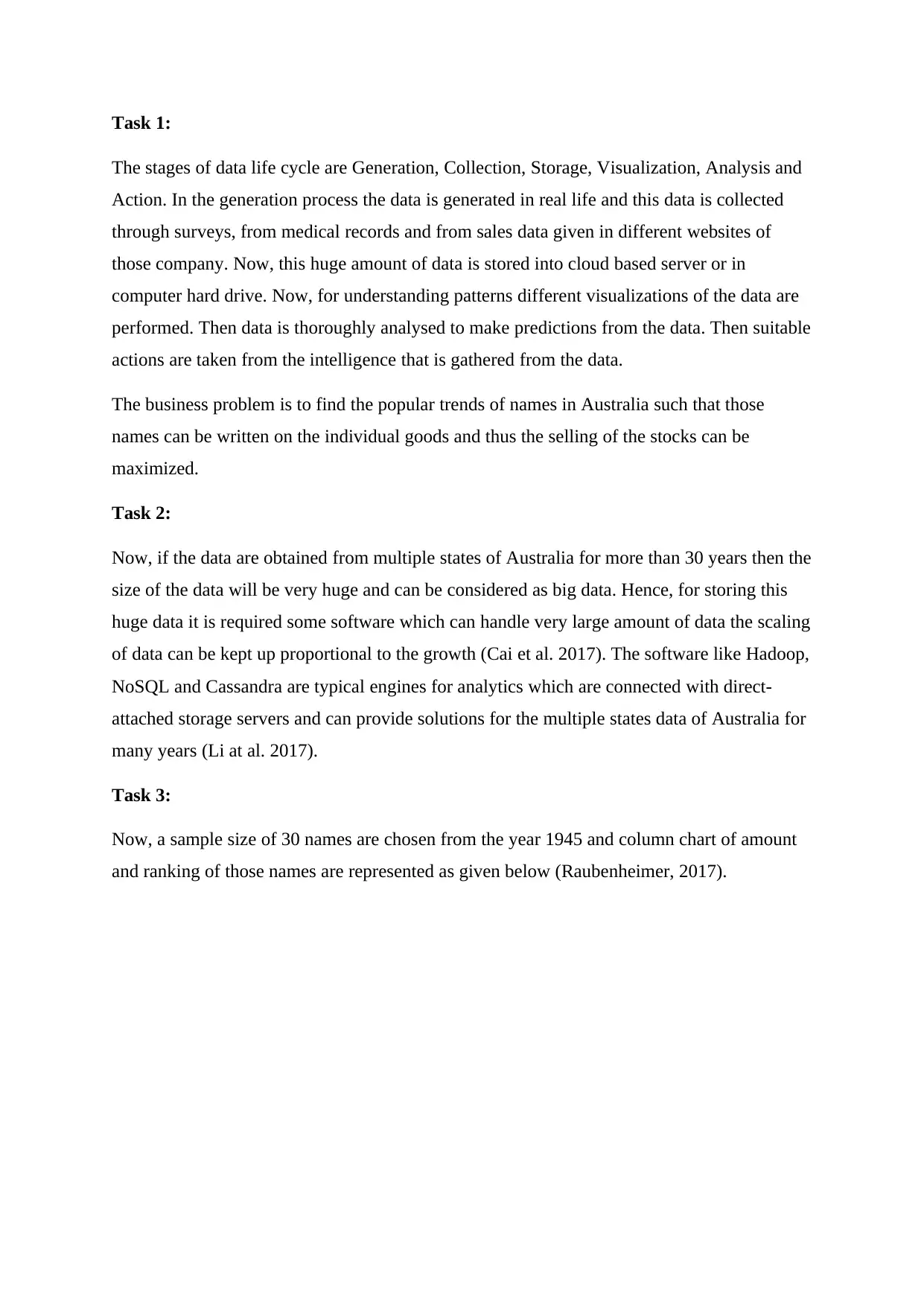
Task 1:
The stages of data life cycle are Generation, Collection, Storage, Visualization, Analysis and
Action. In the generation process the data is generated in real life and this data is collected
through surveys, from medical records and from sales data given in different websites of
those company. Now, this huge amount of data is stored into cloud based server or in
computer hard drive. Now, for understanding patterns different visualizations of the data are
performed. Then data is thoroughly analysed to make predictions from the data. Then suitable
actions are taken from the intelligence that is gathered from the data.
The business problem is to find the popular trends of names in Australia such that those
names can be written on the individual goods and thus the selling of the stocks can be
maximized.
Task 2:
Now, if the data are obtained from multiple states of Australia for more than 30 years then the
size of the data will be very huge and can be considered as big data. Hence, for storing this
huge data it is required some software which can handle very large amount of data the scaling
of data can be kept up proportional to the growth (Cai et al. 2017). The software like Hadoop,
NoSQL and Cassandra are typical engines for analytics which are connected with direct-
attached storage servers and can provide solutions for the multiple states data of Australia for
many years (Li at al. 2017).
Task 3:
Now, a sample size of 30 names are chosen from the year 1945 and column chart of amount
and ranking of those names are represented as given below (Raubenheimer, 2017).
The stages of data life cycle are Generation, Collection, Storage, Visualization, Analysis and
Action. In the generation process the data is generated in real life and this data is collected
through surveys, from medical records and from sales data given in different websites of
those company. Now, this huge amount of data is stored into cloud based server or in
computer hard drive. Now, for understanding patterns different visualizations of the data are
performed. Then data is thoroughly analysed to make predictions from the data. Then suitable
actions are taken from the intelligence that is gathered from the data.
The business problem is to find the popular trends of names in Australia such that those
names can be written on the individual goods and thus the selling of the stocks can be
maximized.
Task 2:
Now, if the data are obtained from multiple states of Australia for more than 30 years then the
size of the data will be very huge and can be considered as big data. Hence, for storing this
huge data it is required some software which can handle very large amount of data the scaling
of data can be kept up proportional to the growth (Cai et al. 2017). The software like Hadoop,
NoSQL and Cassandra are typical engines for analytics which are connected with direct-
attached storage servers and can provide solutions for the multiple states data of Australia for
many years (Li at al. 2017).
Task 3:
Now, a sample size of 30 names are chosen from the year 1945 and column chart of amount
and ranking of those names are represented as given below (Raubenheimer, 2017).
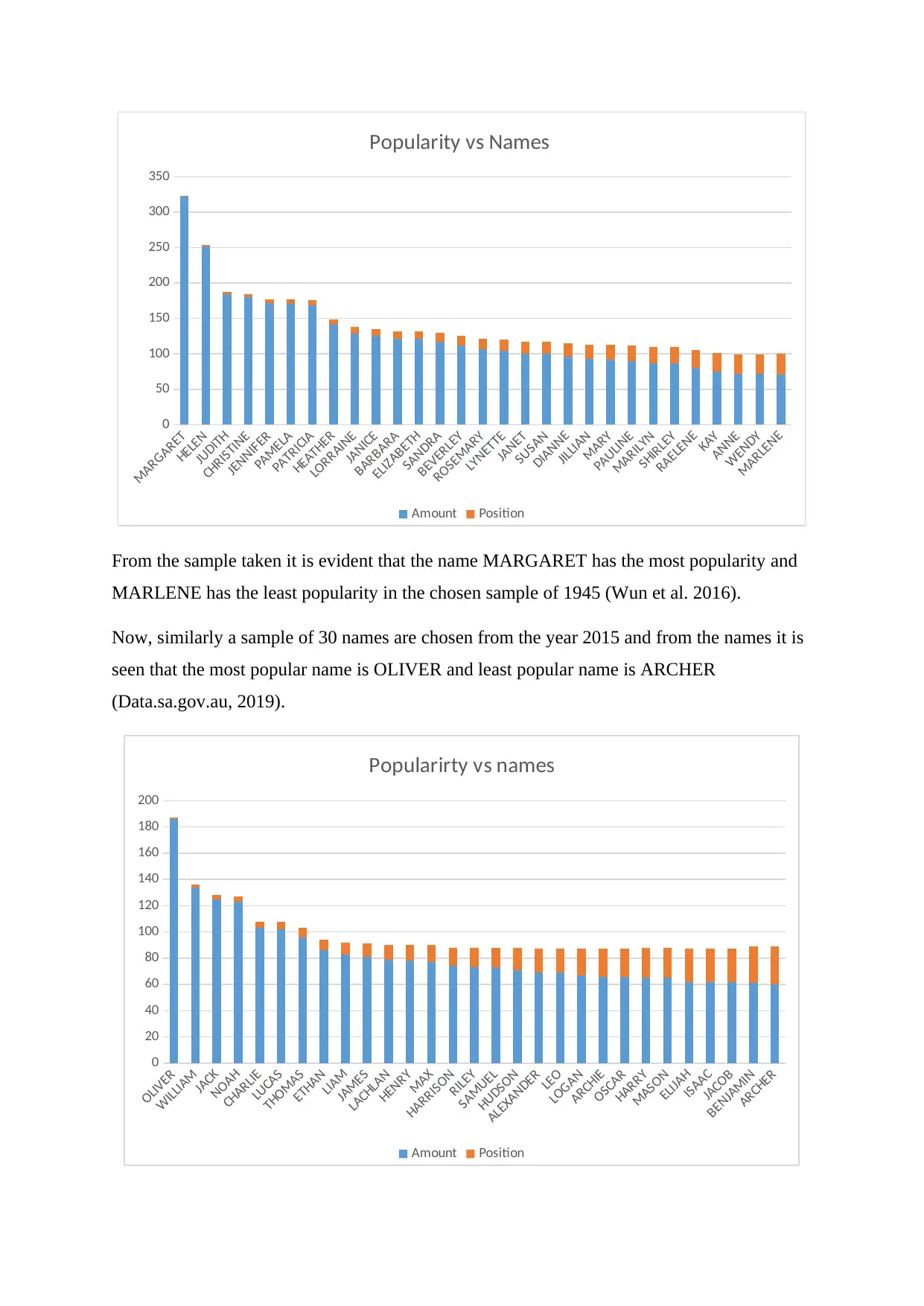
MARGARET
HELEN
JUDITH
CHRISTINE
JENNIFER
PAMELA
PATRICIA
HEATHER
LORRAINE
JANICE
BARBARA
ELIZABETH
SANDRA
BEVERLEY
ROSEMARY
LYNETTE
JANET
SUSAN
DIANNE
JILLIAN
MARY
PAULINE
MARILYN
SHIRLEY
RAELENE
KAY
ANNE
WENDY
MARLENE
0
50
100
150
200
250
300
350
Popularity vs Names
Amount Position
From the sample taken it is evident that the name MARGARET has the most popularity and
MARLENE has the least popularity in the chosen sample of 1945 (Wun et al. 2016).
Now, similarly a sample of 30 names are chosen from the year 2015 and from the names it is
seen that the most popular name is OLIVER and least popular name is ARCHER
(Data.sa.gov.au, 2019).
OLIVER
WILLIAM
JACK
NOAH
CHARLIE
LUCAS
THOMAS
ETHAN
LIAM
JAMES
LACHLAN
HENRY
MAX
HARRISON
RILEY
SAMUEL
HUDSON
ALEXANDER
LEO
LOGAN
ARCHIE
OSCAR
HARRY
MASON
ELIJAH
ISAAC
JACOB
BENJAMIN
ARCHER
0
20
40
60
80
100
120
140
160
180
200
Popularirty vs names
Amount Position
HELEN
JUDITH
CHRISTINE
JENNIFER
PAMELA
PATRICIA
HEATHER
LORRAINE
JANICE
BARBARA
ELIZABETH
SANDRA
BEVERLEY
ROSEMARY
LYNETTE
JANET
SUSAN
DIANNE
JILLIAN
MARY
PAULINE
MARILYN
SHIRLEY
RAELENE
KAY
ANNE
WENDY
MARLENE
0
50
100
150
200
250
300
350
Popularity vs Names
Amount Position
From the sample taken it is evident that the name MARGARET has the most popularity and
MARLENE has the least popularity in the chosen sample of 1945 (Wun et al. 2016).
Now, similarly a sample of 30 names are chosen from the year 2015 and from the names it is
seen that the most popular name is OLIVER and least popular name is ARCHER
(Data.sa.gov.au, 2019).
OLIVER
WILLIAM
JACK
NOAH
CHARLIE
LUCAS
THOMAS
ETHAN
LIAM
JAMES
LACHLAN
HENRY
MAX
HARRISON
RILEY
SAMUEL
HUDSON
ALEXANDER
LEO
LOGAN
ARCHIE
OSCAR
HARRY
MASON
ELIJAH
ISAAC
JACOB
BENJAMIN
ARCHER
0
20
40
60
80
100
120
140
160
180
200
Popularirty vs names
Amount Position
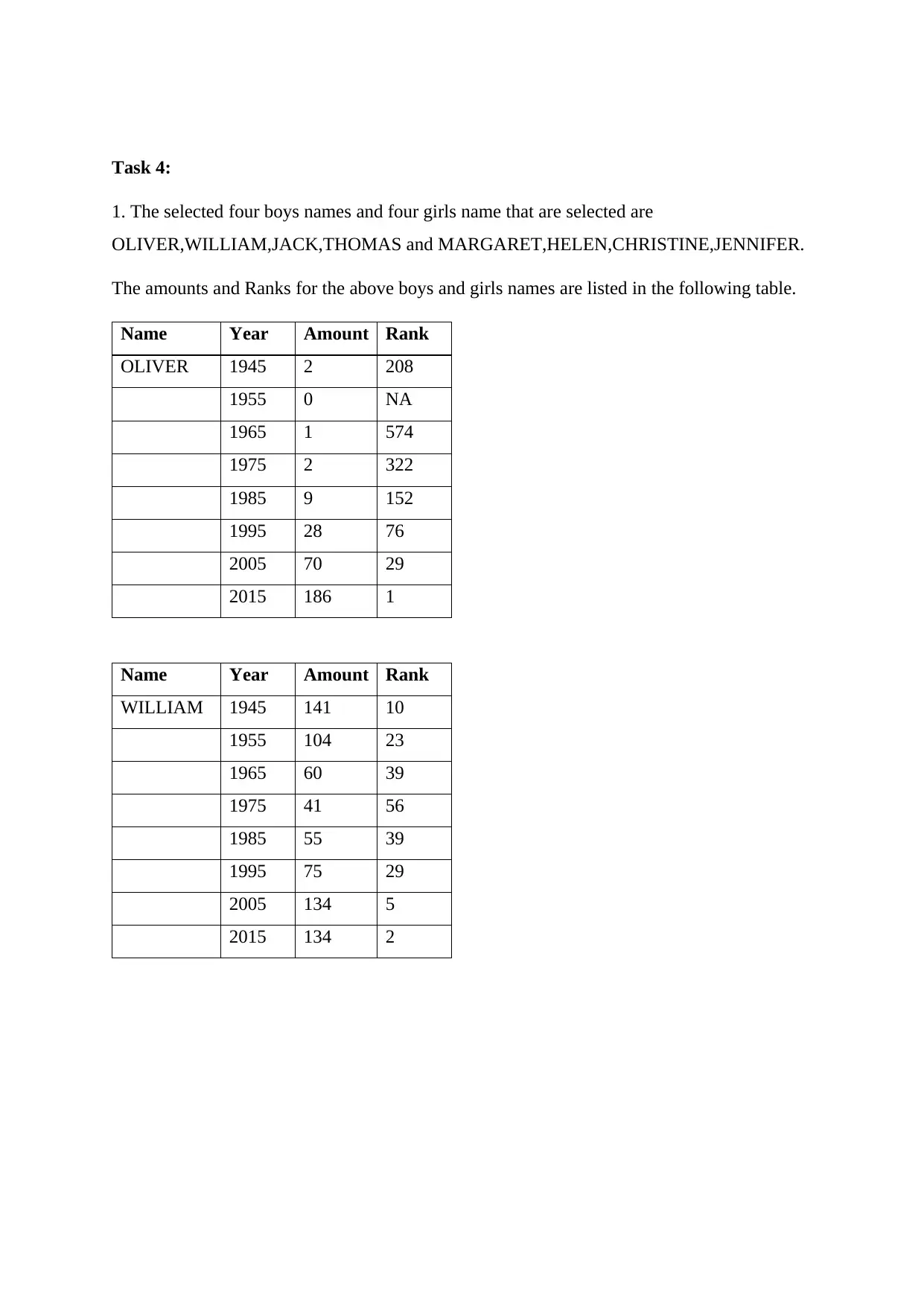
Task 4:
1. The selected four boys names and four girls name that are selected are
OLIVER,WILLIAM,JACK,THOMAS and MARGARET,HELEN,CHRISTINE,JENNIFER.
The amounts and Ranks for the above boys and girls names are listed in the following table.
Name Year Amount Rank
OLIVER 1945 2 208
1955 0 NA
1965 1 574
1975 2 322
1985 9 152
1995 28 76
2005 70 29
2015 186 1
Name Year Amount Rank
WILLIAM 1945 141 10
1955 104 23
1965 60 39
1975 41 56
1985 55 39
1995 75 29
2005 134 5
2015 134 2
1. The selected four boys names and four girls name that are selected are
OLIVER,WILLIAM,JACK,THOMAS and MARGARET,HELEN,CHRISTINE,JENNIFER.
The amounts and Ranks for the above boys and girls names are listed in the following table.
Name Year Amount Rank
OLIVER 1945 2 208
1955 0 NA
1965 1 574
1975 2 322
1985 9 152
1995 28 76
2005 70 29
2015 186 1
Name Year Amount Rank
WILLIAM 1945 141 10
1955 104 23
1965 60 39
1975 41 56
1985 55 39
1995 75 29
2005 134 5
2015 134 2
Secure Best Marks with AI Grader
Need help grading? Try our AI Grader for instant feedback on your assignments.
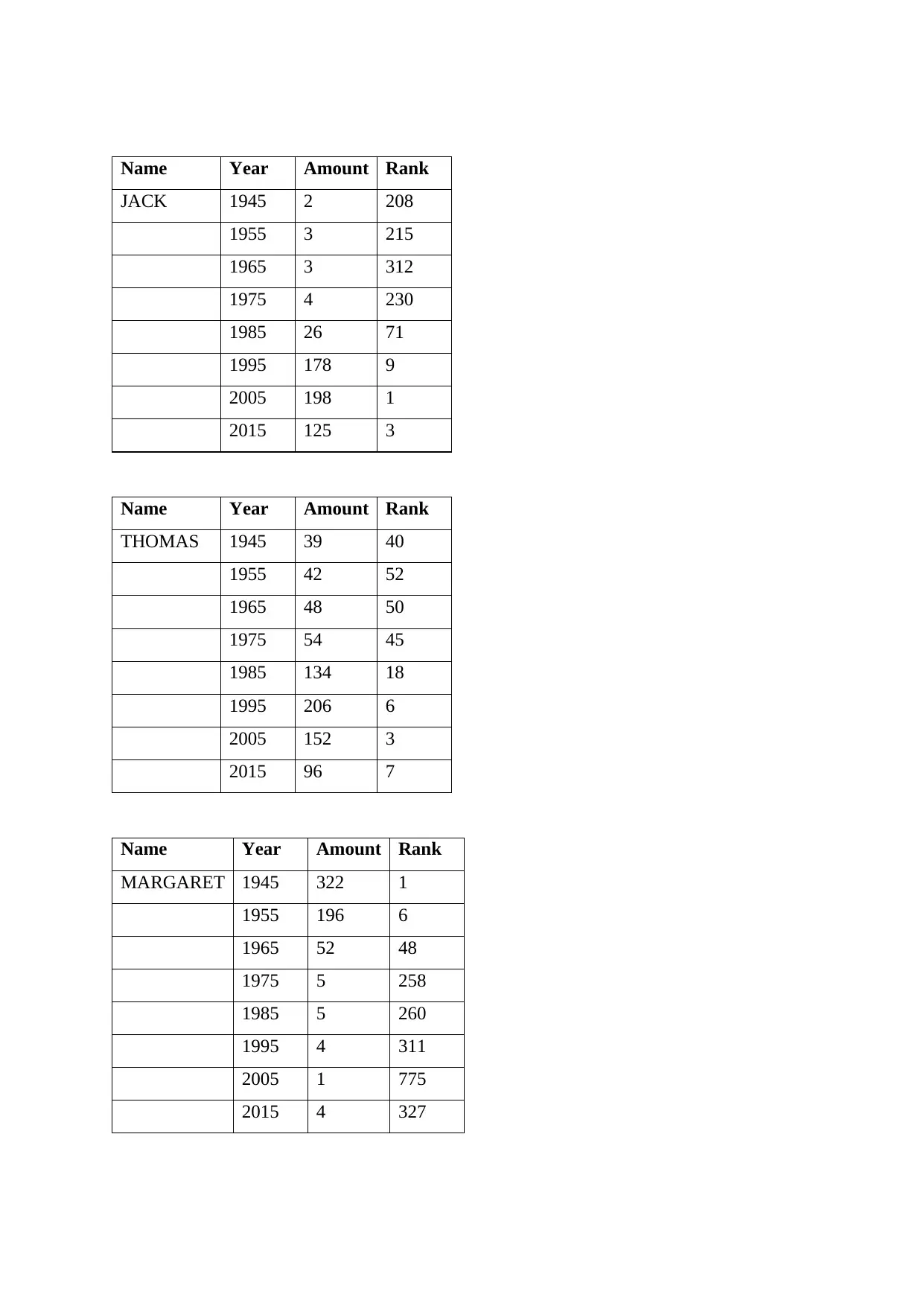
Name Year Amount Rank
JACK 1945 2 208
1955 3 215
1965 3 312
1975 4 230
1985 26 71
1995 178 9
2005 198 1
2015 125 3
Name Year Amount Rank
THOMAS 1945 39 40
1955 42 52
1965 48 50
1975 54 45
1985 134 18
1995 206 6
2005 152 3
2015 96 7
Name Year Amount Rank
MARGARET 1945 322 1
1955 196 6
1965 52 48
1975 5 258
1985 5 260
1995 4 311
2005 1 775
2015 4 327
JACK 1945 2 208
1955 3 215
1965 3 312
1975 4 230
1985 26 71
1995 178 9
2005 198 1
2015 125 3
Name Year Amount Rank
THOMAS 1945 39 40
1955 42 52
1965 48 50
1975 54 45
1985 134 18
1995 206 6
2005 152 3
2015 96 7
Name Year Amount Rank
MARGARET 1945 322 1
1955 196 6
1965 52 48
1975 5 258
1985 5 260
1995 4 311
2005 1 775
2015 4 327
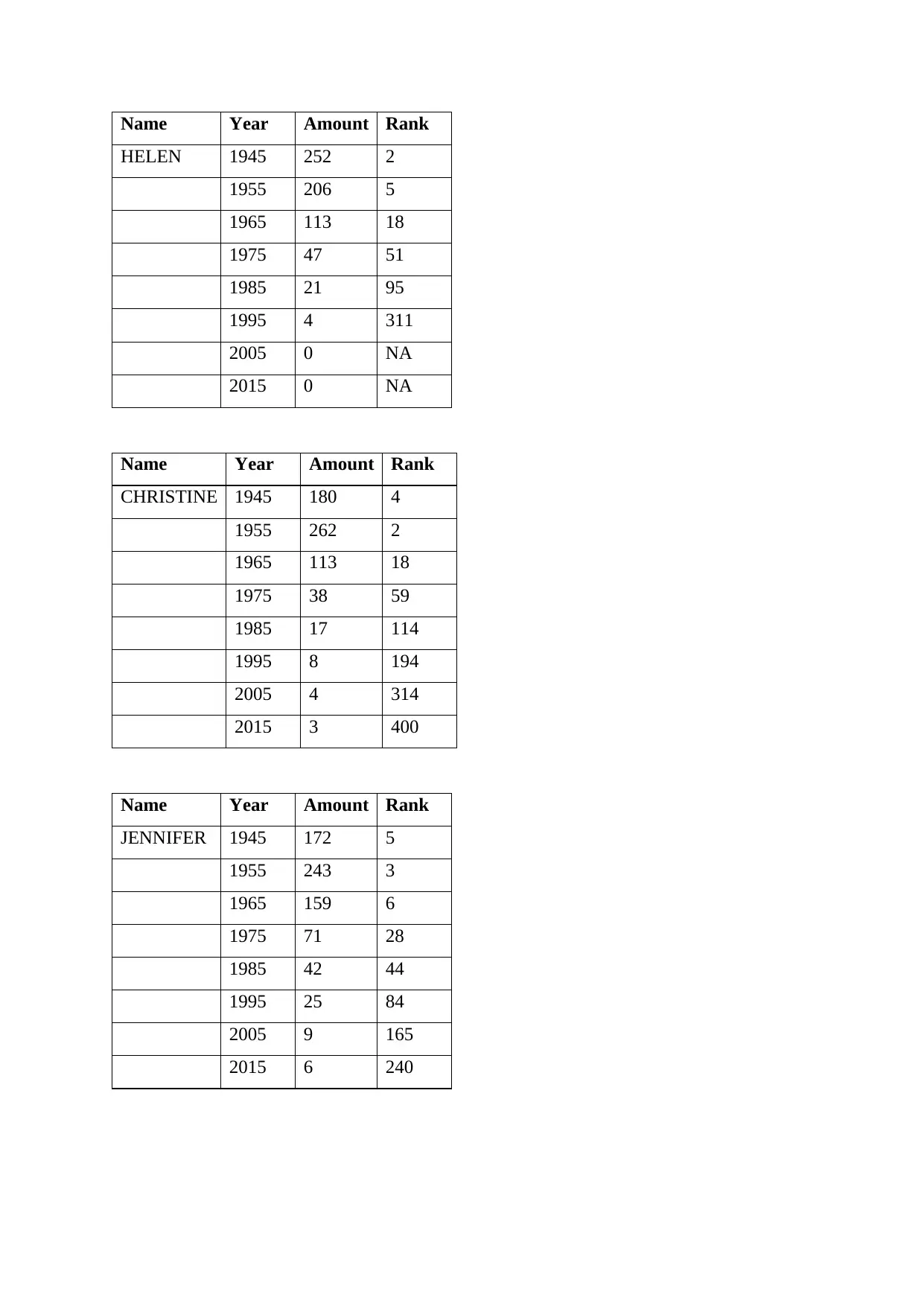
Name Year Amount Rank
HELEN 1945 252 2
1955 206 5
1965 113 18
1975 47 51
1985 21 95
1995 4 311
2005 0 NA
2015 0 NA
Name Year Amount Rank
CHRISTINE 1945 180 4
1955 262 2
1965 113 18
1975 38 59
1985 17 114
1995 8 194
2005 4 314
2015 3 400
Name Year Amount Rank
JENNIFER 1945 172 5
1955 243 3
1965 159 6
1975 71 28
1985 42 44
1995 25 84
2005 9 165
2015 6 240
HELEN 1945 252 2
1955 206 5
1965 113 18
1975 47 51
1985 21 95
1995 4 311
2005 0 NA
2015 0 NA
Name Year Amount Rank
CHRISTINE 1945 180 4
1955 262 2
1965 113 18
1975 38 59
1985 17 114
1995 8 194
2005 4 314
2015 3 400
Name Year Amount Rank
JENNIFER 1945 172 5
1955 243 3
1965 159 6
1975 71 28
1985 42 44
1995 25 84
2005 9 165
2015 6 240
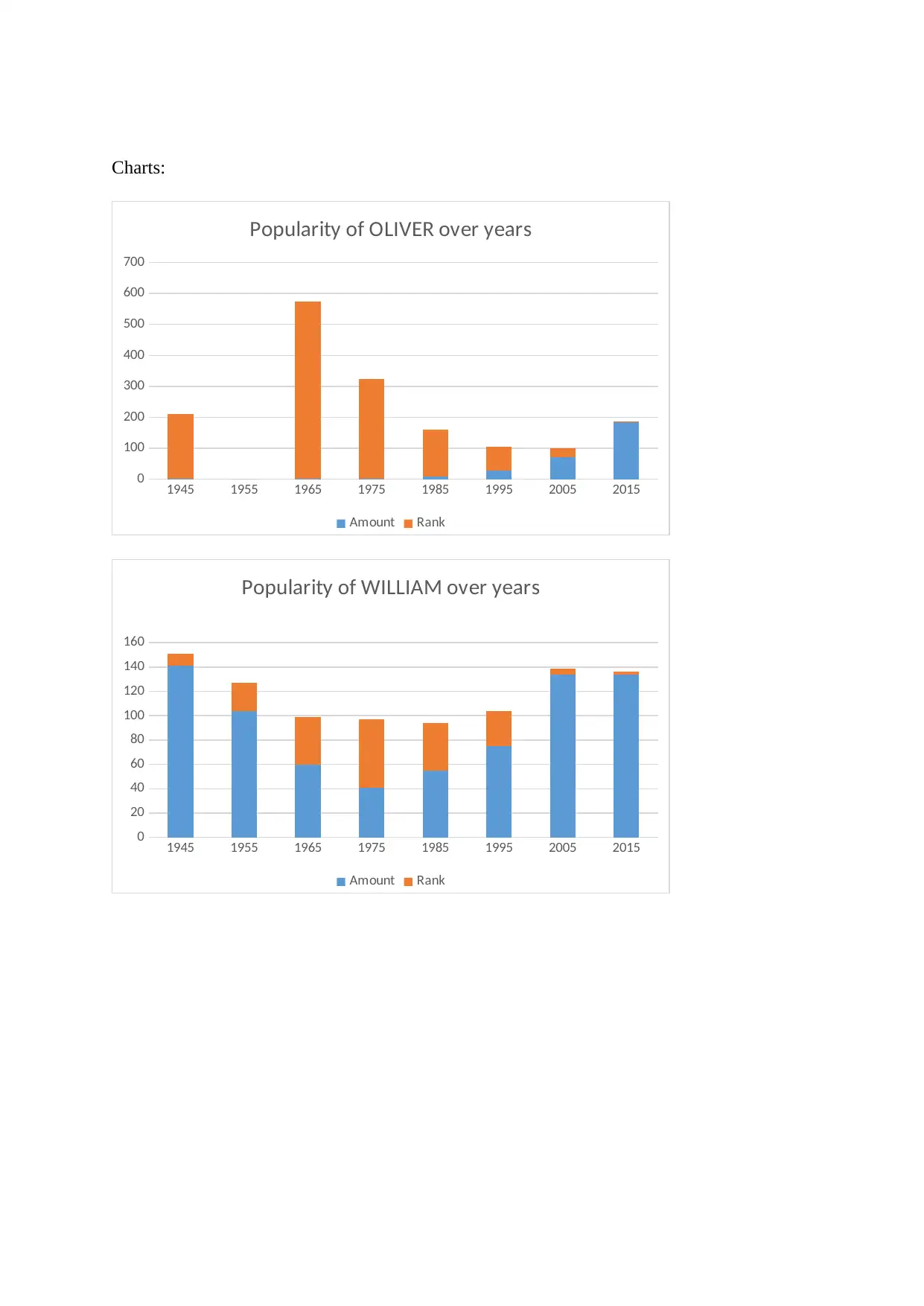
Charts:
1945 1955 1965 1975 1985 1995 2005 2015
0
100
200
300
400
500
600
700
Popularity of OLIVER over years
Amount Rank
1945 1955 1965 1975 1985 1995 2005 2015
0
20
40
60
80
100
120
140
160
Popularity of WILLIAM over years
Amount Rank
1945 1955 1965 1975 1985 1995 2005 2015
0
100
200
300
400
500
600
700
Popularity of OLIVER over years
Amount Rank
1945 1955 1965 1975 1985 1995 2005 2015
0
20
40
60
80
100
120
140
160
Popularity of WILLIAM over years
Amount Rank
Paraphrase This Document
Need a fresh take? Get an instant paraphrase of this document with our AI Paraphraser
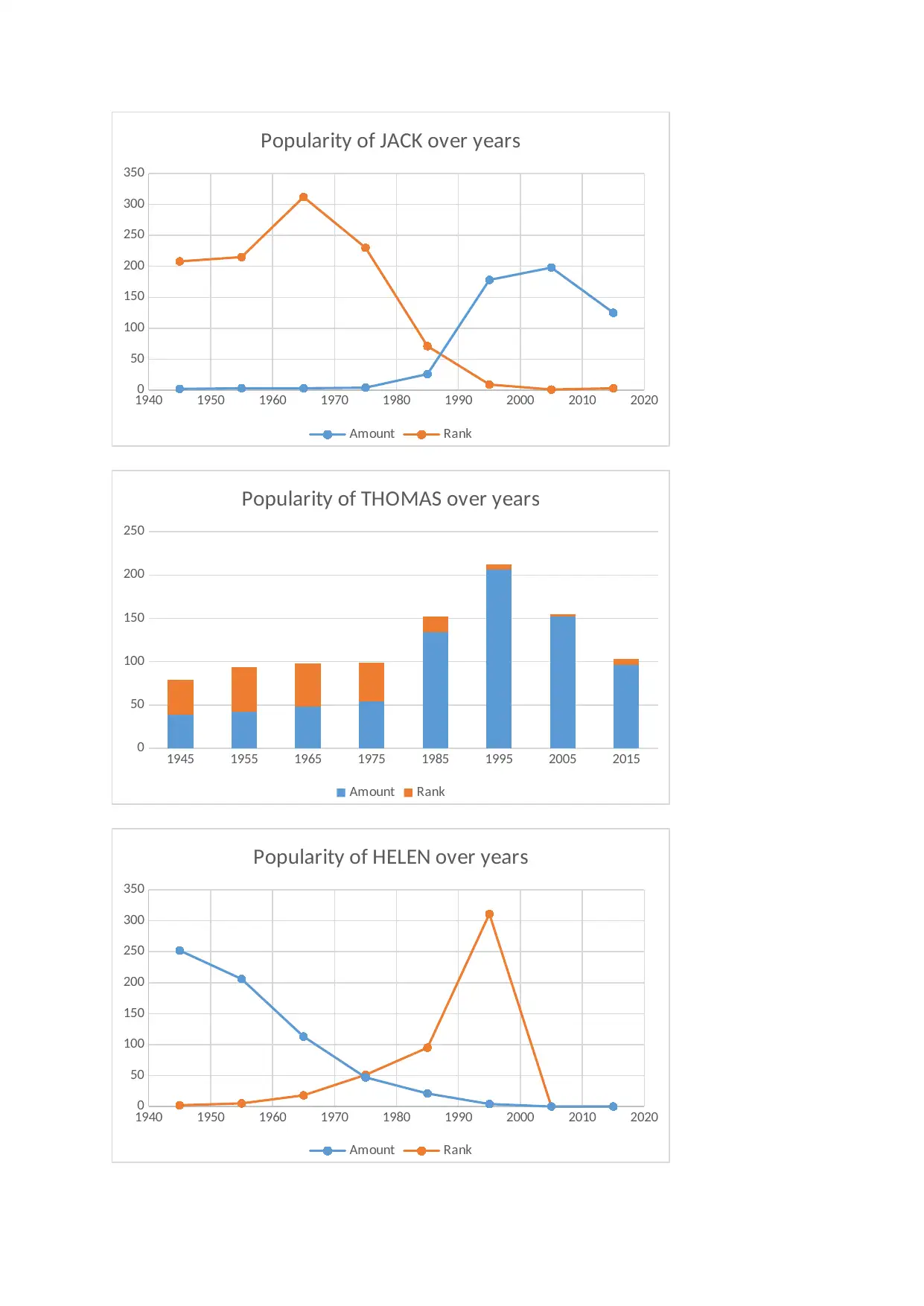
1940 1950 1960 1970 1980 1990 2000 2010 2020
0
50
100
150
200
250
300
350
Popularity of JACK over years
Amount Rank
1945 1955 1965 1975 1985 1995 2005 2015
0
50
100
150
200
250
Popularity of THOMAS over years
Amount Rank
1940 1950 1960 1970 1980 1990 2000 2010 2020
0
50
100
150
200
250
300
350
Popularity of HELEN over years
Amount Rank
0
50
100
150
200
250
300
350
Popularity of JACK over years
Amount Rank
1945 1955 1965 1975 1985 1995 2005 2015
0
50
100
150
200
250
Popularity of THOMAS over years
Amount Rank
1940 1950 1960 1970 1980 1990 2000 2010 2020
0
50
100
150
200
250
300
350
Popularity of HELEN over years
Amount Rank
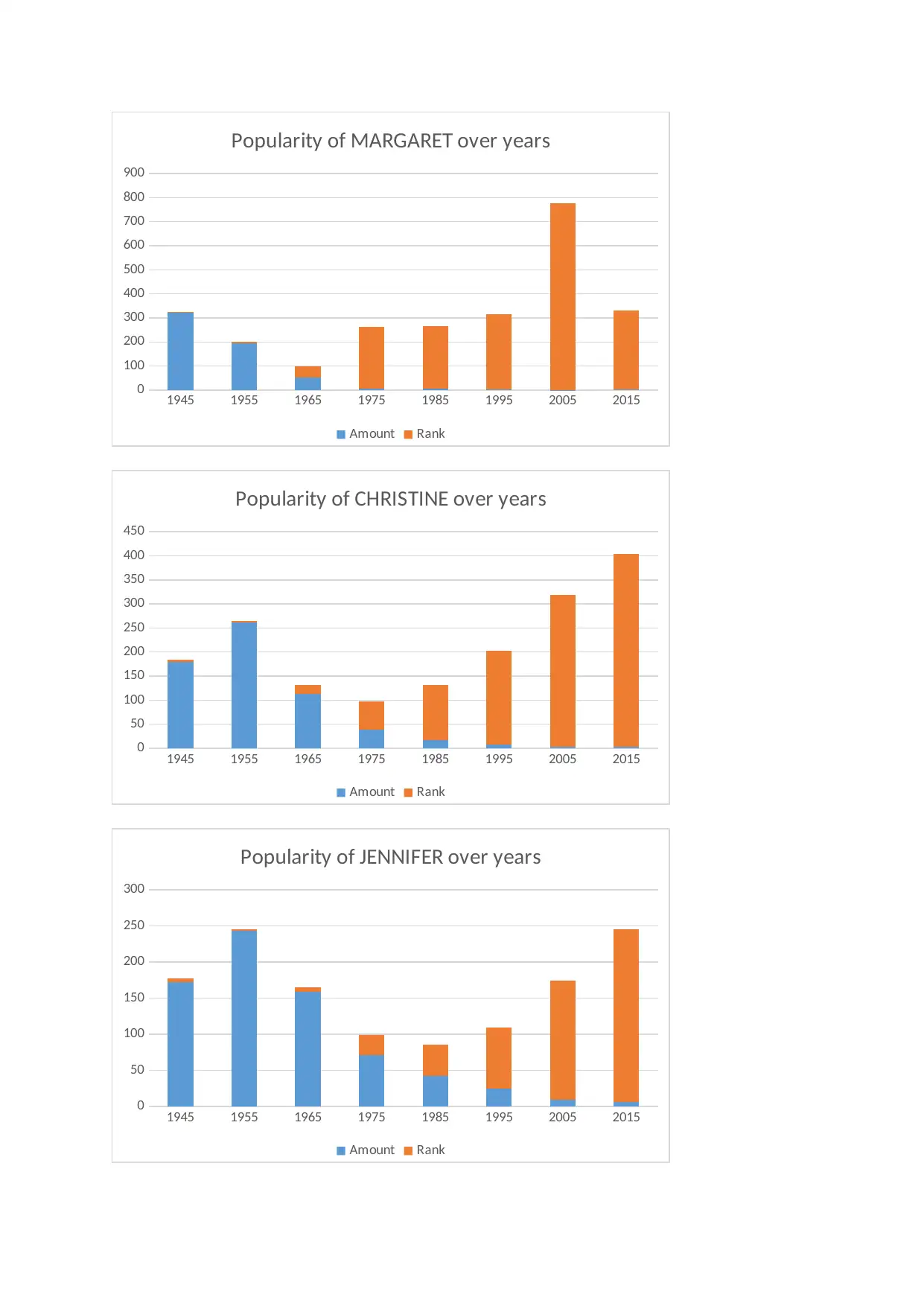
1945 1955 1965 1975 1985 1995 2005 2015
0
100
200
300
400
500
600
700
800
900
Popularity of MARGARET over years
Amount Rank
1945 1955 1965 1975 1985 1995 2005 2015
0
50
100
150
200
250
300
350
400
450
Popularity of CHRISTINE over years
Amount Rank
1945 1955 1965 1975 1985 1995 2005 2015
0
50
100
150
200
250
300
Popularity of JENNIFER over years
Amount Rank
0
100
200
300
400
500
600
700
800
900
Popularity of MARGARET over years
Amount Rank
1945 1955 1965 1975 1985 1995 2005 2015
0
50
100
150
200
250
300
350
400
450
Popularity of CHRISTINE over years
Amount Rank
1945 1955 1965 1975 1985 1995 2005 2015
0
50
100
150
200
250
300
Popularity of JENNIFER over years
Amount Rank
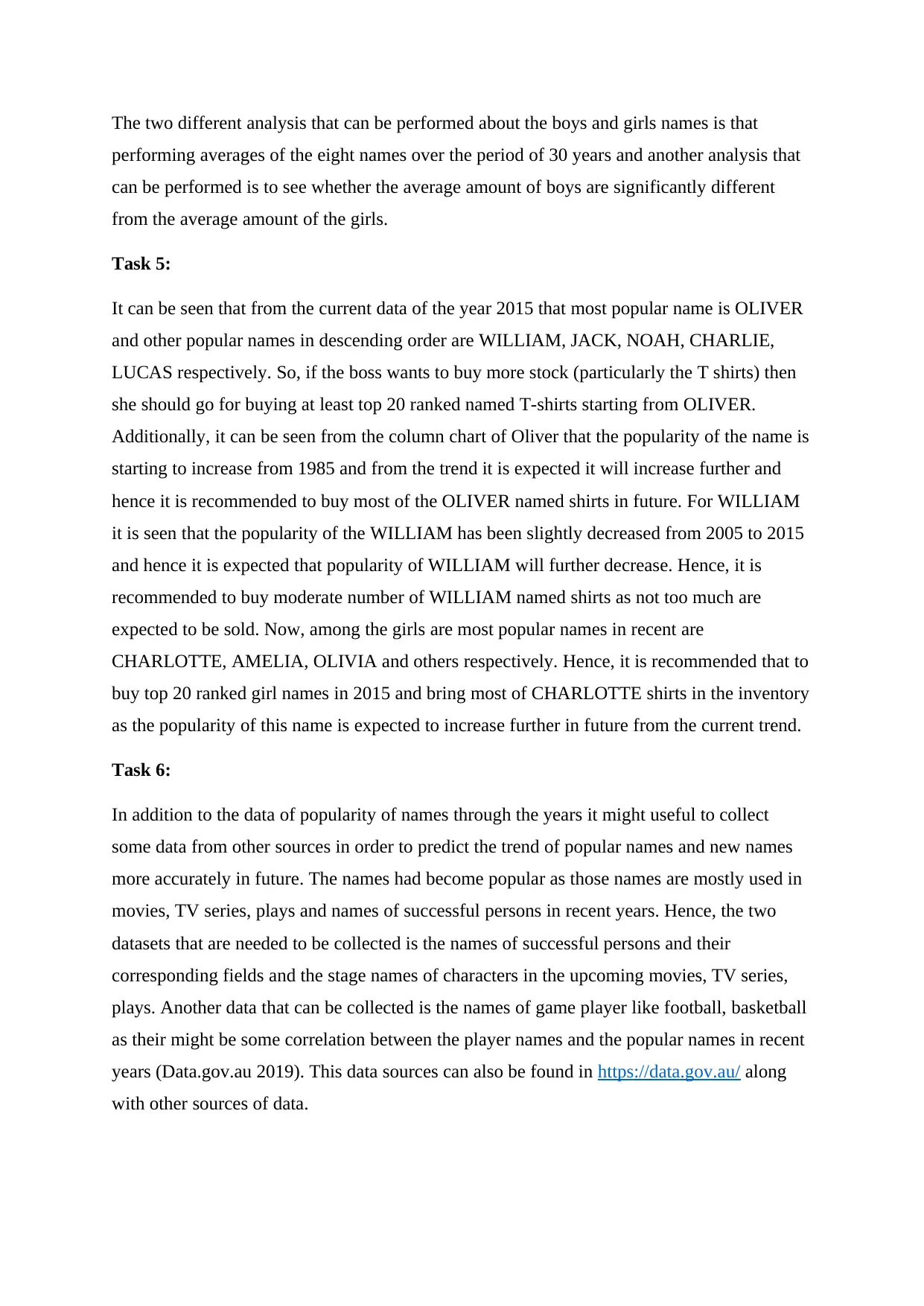
The two different analysis that can be performed about the boys and girls names is that
performing averages of the eight names over the period of 30 years and another analysis that
can be performed is to see whether the average amount of boys are significantly different
from the average amount of the girls.
Task 5:
It can be seen that from the current data of the year 2015 that most popular name is OLIVER
and other popular names in descending order are WILLIAM, JACK, NOAH, CHARLIE,
LUCAS respectively. So, if the boss wants to buy more stock (particularly the T shirts) then
she should go for buying at least top 20 ranked named T-shirts starting from OLIVER.
Additionally, it can be seen from the column chart of Oliver that the popularity of the name is
starting to increase from 1985 and from the trend it is expected it will increase further and
hence it is recommended to buy most of the OLIVER named shirts in future. For WILLIAM
it is seen that the popularity of the WILLIAM has been slightly decreased from 2005 to 2015
and hence it is expected that popularity of WILLIAM will further decrease. Hence, it is
recommended to buy moderate number of WILLIAM named shirts as not too much are
expected to be sold. Now, among the girls are most popular names in recent are
CHARLOTTE, AMELIA, OLIVIA and others respectively. Hence, it is recommended that to
buy top 20 ranked girl names in 2015 and bring most of CHARLOTTE shirts in the inventory
as the popularity of this name is expected to increase further in future from the current trend.
Task 6:
In addition to the data of popularity of names through the years it might useful to collect
some data from other sources in order to predict the trend of popular names and new names
more accurately in future. The names had become popular as those names are mostly used in
movies, TV series, plays and names of successful persons in recent years. Hence, the two
datasets that are needed to be collected is the names of successful persons and their
corresponding fields and the stage names of characters in the upcoming movies, TV series,
plays. Another data that can be collected is the names of game player like football, basketball
as their might be some correlation between the player names and the popular names in recent
years (Data.gov.au 2019). This data sources can also be found in https://data.gov.au/ along
with other sources of data.
performing averages of the eight names over the period of 30 years and another analysis that
can be performed is to see whether the average amount of boys are significantly different
from the average amount of the girls.
Task 5:
It can be seen that from the current data of the year 2015 that most popular name is OLIVER
and other popular names in descending order are WILLIAM, JACK, NOAH, CHARLIE,
LUCAS respectively. So, if the boss wants to buy more stock (particularly the T shirts) then
she should go for buying at least top 20 ranked named T-shirts starting from OLIVER.
Additionally, it can be seen from the column chart of Oliver that the popularity of the name is
starting to increase from 1985 and from the trend it is expected it will increase further and
hence it is recommended to buy most of the OLIVER named shirts in future. For WILLIAM
it is seen that the popularity of the WILLIAM has been slightly decreased from 2005 to 2015
and hence it is expected that popularity of WILLIAM will further decrease. Hence, it is
recommended to buy moderate number of WILLIAM named shirts as not too much are
expected to be sold. Now, among the girls are most popular names in recent are
CHARLOTTE, AMELIA, OLIVIA and others respectively. Hence, it is recommended that to
buy top 20 ranked girl names in 2015 and bring most of CHARLOTTE shirts in the inventory
as the popularity of this name is expected to increase further in future from the current trend.
Task 6:
In addition to the data of popularity of names through the years it might useful to collect
some data from other sources in order to predict the trend of popular names and new names
more accurately in future. The names had become popular as those names are mostly used in
movies, TV series, plays and names of successful persons in recent years. Hence, the two
datasets that are needed to be collected is the names of successful persons and their
corresponding fields and the stage names of characters in the upcoming movies, TV series,
plays. Another data that can be collected is the names of game player like football, basketball
as their might be some correlation between the player names and the popular names in recent
years (Data.gov.au 2019). This data sources can also be found in https://data.gov.au/ along
with other sources of data.
Secure Best Marks with AI Grader
Need help grading? Try our AI Grader for instant feedback on your assignments.
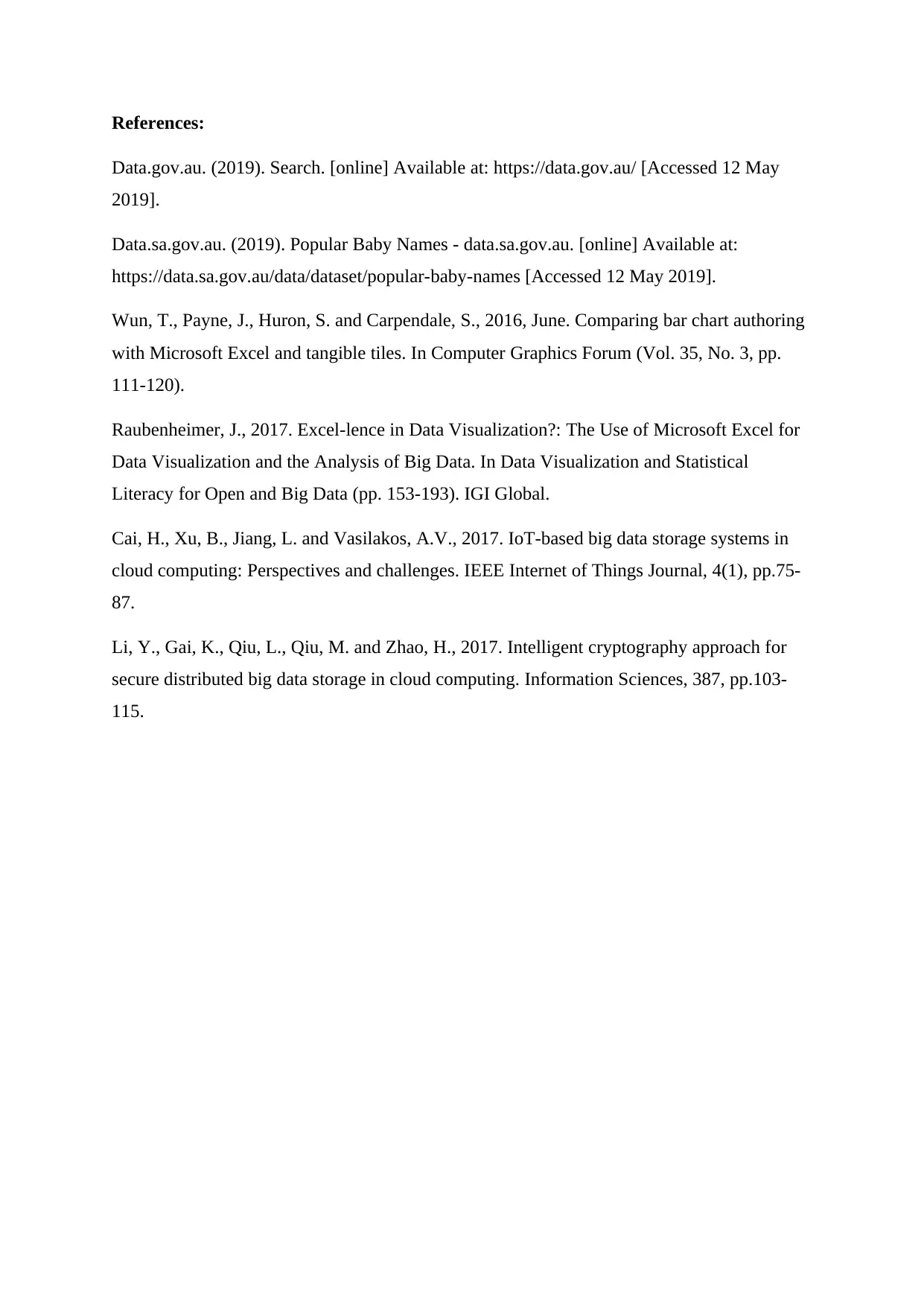
References:
Data.gov.au. (2019). Search. [online] Available at: https://data.gov.au/ [Accessed 12 May
2019].
Data.sa.gov.au. (2019). Popular Baby Names - data.sa.gov.au. [online] Available at:
https://data.sa.gov.au/data/dataset/popular-baby-names [Accessed 12 May 2019].
Wun, T., Payne, J., Huron, S. and Carpendale, S., 2016, June. Comparing bar chart authoring
with Microsoft Excel and tangible tiles. In Computer Graphics Forum (Vol. 35, No. 3, pp.
111-120).
Raubenheimer, J., 2017. Excel-lence in Data Visualization?: The Use of Microsoft Excel for
Data Visualization and the Analysis of Big Data. In Data Visualization and Statistical
Literacy for Open and Big Data (pp. 153-193). IGI Global.
Cai, H., Xu, B., Jiang, L. and Vasilakos, A.V., 2017. IoT-based big data storage systems in
cloud computing: Perspectives and challenges. IEEE Internet of Things Journal, 4(1), pp.75-
87.
Li, Y., Gai, K., Qiu, L., Qiu, M. and Zhao, H., 2017. Intelligent cryptography approach for
secure distributed big data storage in cloud computing. Information Sciences, 387, pp.103-
115.
Data.gov.au. (2019). Search. [online] Available at: https://data.gov.au/ [Accessed 12 May
2019].
Data.sa.gov.au. (2019). Popular Baby Names - data.sa.gov.au. [online] Available at:
https://data.sa.gov.au/data/dataset/popular-baby-names [Accessed 12 May 2019].
Wun, T., Payne, J., Huron, S. and Carpendale, S., 2016, June. Comparing bar chart authoring
with Microsoft Excel and tangible tiles. In Computer Graphics Forum (Vol. 35, No. 3, pp.
111-120).
Raubenheimer, J., 2017. Excel-lence in Data Visualization?: The Use of Microsoft Excel for
Data Visualization and the Analysis of Big Data. In Data Visualization and Statistical
Literacy for Open and Big Data (pp. 153-193). IGI Global.
Cai, H., Xu, B., Jiang, L. and Vasilakos, A.V., 2017. IoT-based big data storage systems in
cloud computing: Perspectives and challenges. IEEE Internet of Things Journal, 4(1), pp.75-
87.
Li, Y., Gai, K., Qiu, L., Qiu, M. and Zhao, H., 2017. Intelligent cryptography approach for
secure distributed big data storage in cloud computing. Information Sciences, 387, pp.103-
115.
1 out of 11
![[object Object]](/_next/image/?url=%2F_next%2Fstatic%2Fmedia%2Flogo.6d15ce61.png&w=640&q=75)
Your All-in-One AI-Powered Toolkit for Academic Success.
 +13062052269
info@desklib.com
Available 24*7 on WhatsApp / Email
Unlock your academic potential
© 2024  |  Zucol Services PVT LTD  |  All rights reserved.