Challenges of Big Data in Business Marketing
VerifiedAdded on Ā 2023/06/15
|11
|3125
|367
AI Summary
This article discusses the challenges of big data in business marketing, including storage, adoption, quality, security, and organizational resistance. It also explores how big data impacts economic development and provides real-world examples of successful big data implementation.
Contribute Materials
Your contribution can guide someoneās learning journey. Share your
documents today.
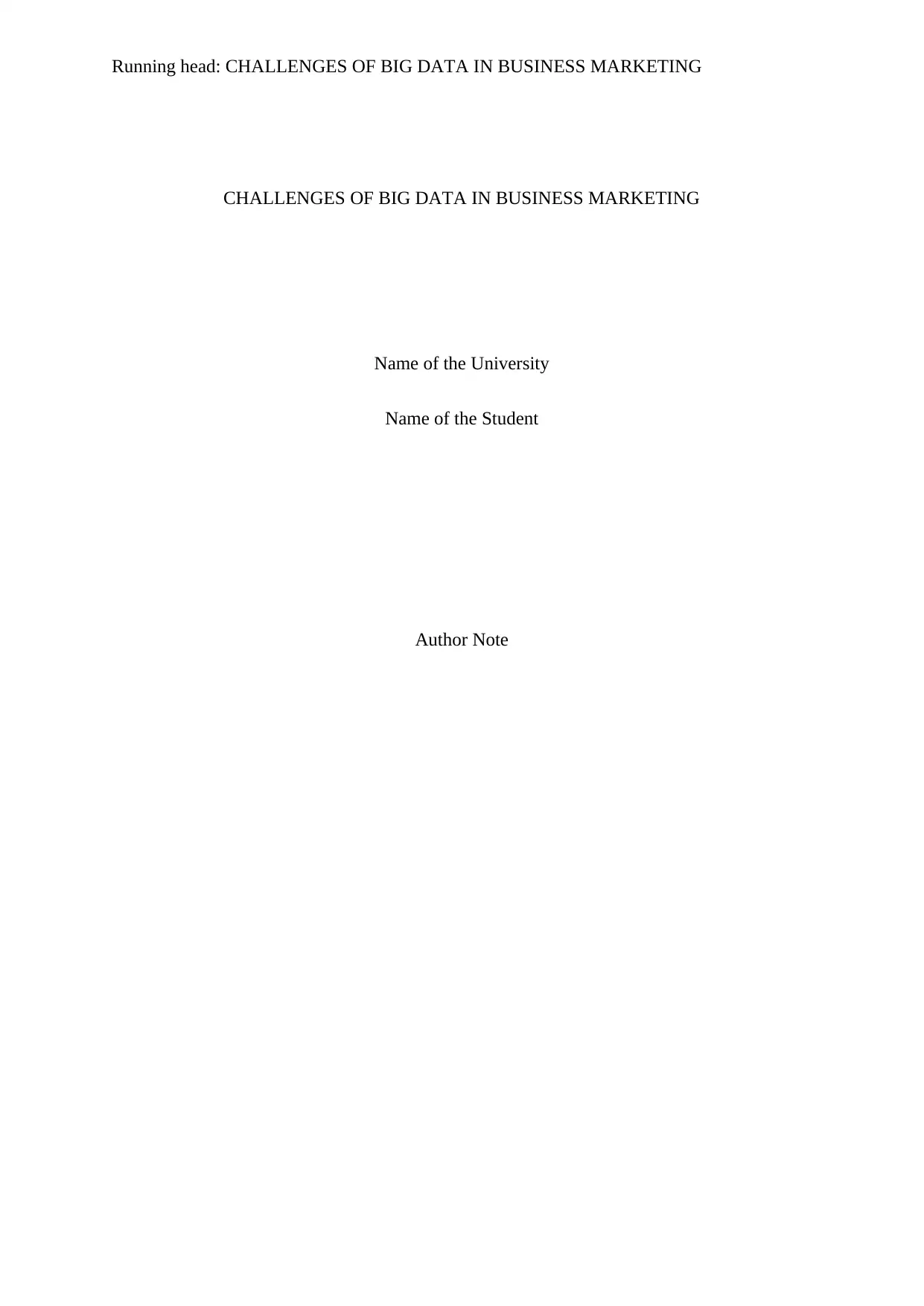
Running head: CHALLENGES OF BIG DATA IN BUSINESS MARKETING
CHALLENGES OF BIG DATA IN BUSINESS MARKETING
Name of the University
Name of the Student
Author Note
CHALLENGES OF BIG DATA IN BUSINESS MARKETING
Name of the University
Name of the Student
Author Note
Secure Best Marks with AI Grader
Need help grading? Try our AI Grader for instant feedback on your assignments.
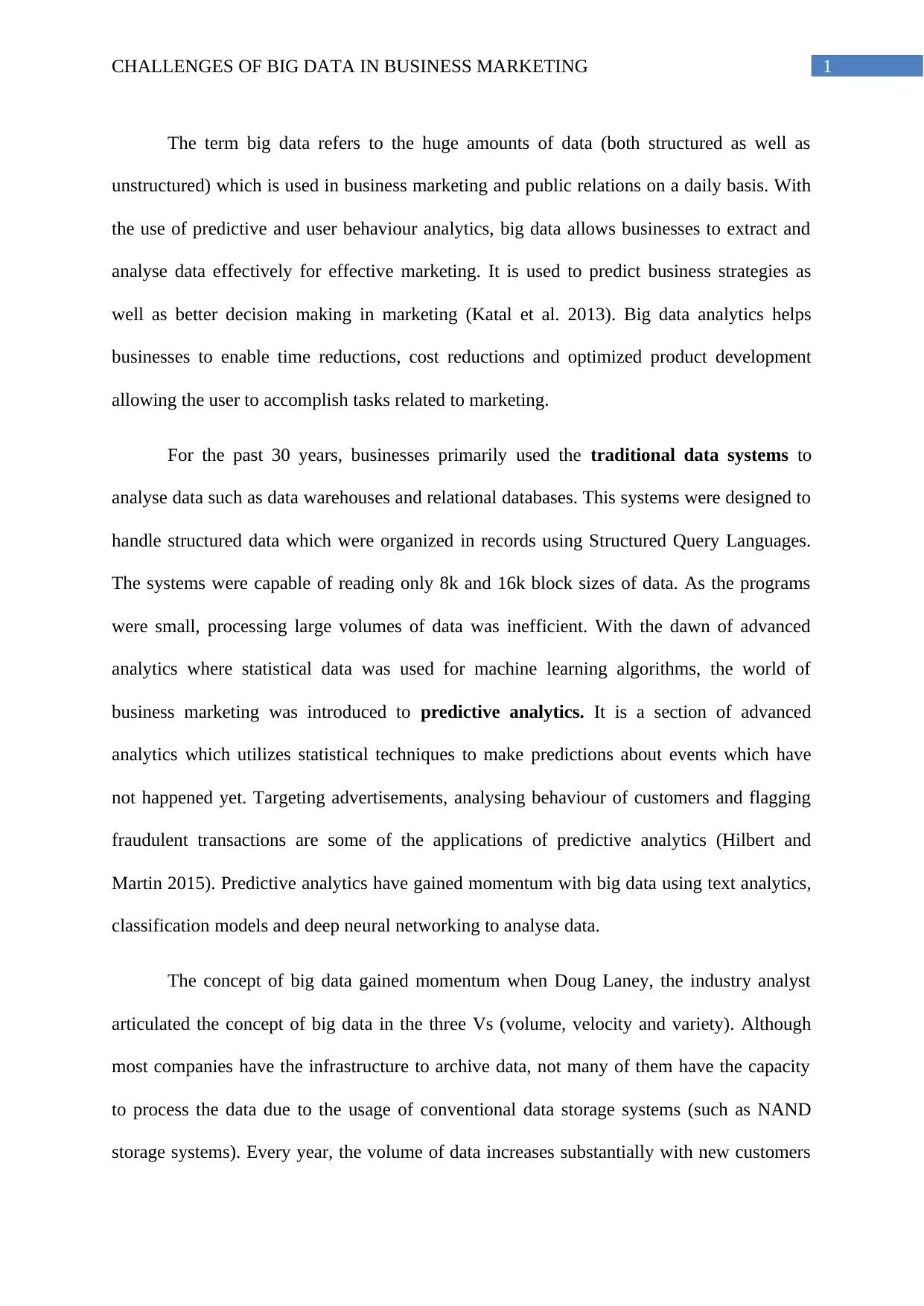
1CHALLENGES OF BIG DATA IN BUSINESS MARKETING
The term big data refers to the huge amounts of data (both structured as well as
unstructured) which is used in business marketing and public relations on a daily basis. With
the use of predictive and user behaviour analytics, big data allows businesses to extract and
analyse data effectively for effective marketing. It is used to predict business strategies as
well as better decision making in marketing (Katal et al. 2013). Big data analytics helps
businesses to enable time reductions, cost reductions and optimized product development
allowing the user to accomplish tasks related to marketing.
For the past 30 years, businesses primarily used the traditional data systems to
analyse data such as data warehouses and relational databases. This systems were designed to
handle structured data which were organized in records using Structured Query Languages.
The systems were capable of reading only 8k and 16k block sizes of data. As the programs
were small, processing large volumes of data was inefficient. With the dawn of advanced
analytics where statistical data was used for machine learning algorithms, the world of
business marketing was introduced to predictive analytics. It is a section of advanced
analytics which utilizes statistical techniques to make predictions about events which have
not happened yet. Targeting advertisements, analysing behaviour of customers and flagging
fraudulent transactions are some of the applications of predictive analytics (Hilbert and
Martin 2015). Predictive analytics have gained momentum with big data using text analytics,
classification models and deep neural networking to analyse data.
The concept of big data gained momentum when Doug Laney, the industry analyst
articulated the concept of big data in the three Vs (volume, velocity and variety). Although
most companies have the infrastructure to archive data, not many of them have the capacity
to process the data due to the usage of conventional data storage systems (such as NAND
storage systems). Every year, the volume of data increases substantially with new customers
The term big data refers to the huge amounts of data (both structured as well as
unstructured) which is used in business marketing and public relations on a daily basis. With
the use of predictive and user behaviour analytics, big data allows businesses to extract and
analyse data effectively for effective marketing. It is used to predict business strategies as
well as better decision making in marketing (Katal et al. 2013). Big data analytics helps
businesses to enable time reductions, cost reductions and optimized product development
allowing the user to accomplish tasks related to marketing.
For the past 30 years, businesses primarily used the traditional data systems to
analyse data such as data warehouses and relational databases. This systems were designed to
handle structured data which were organized in records using Structured Query Languages.
The systems were capable of reading only 8k and 16k block sizes of data. As the programs
were small, processing large volumes of data was inefficient. With the dawn of advanced
analytics where statistical data was used for machine learning algorithms, the world of
business marketing was introduced to predictive analytics. It is a section of advanced
analytics which utilizes statistical techniques to make predictions about events which have
not happened yet. Targeting advertisements, analysing behaviour of customers and flagging
fraudulent transactions are some of the applications of predictive analytics (Hilbert and
Martin 2015). Predictive analytics have gained momentum with big data using text analytics,
classification models and deep neural networking to analyse data.
The concept of big data gained momentum when Doug Laney, the industry analyst
articulated the concept of big data in the three Vs (volume, velocity and variety). Although
most companies have the infrastructure to archive data, not many of them have the capacity
to process the data due to the usage of conventional data storage systems (such as NAND
storage systems). Every year, the volume of data increases substantially with new customers
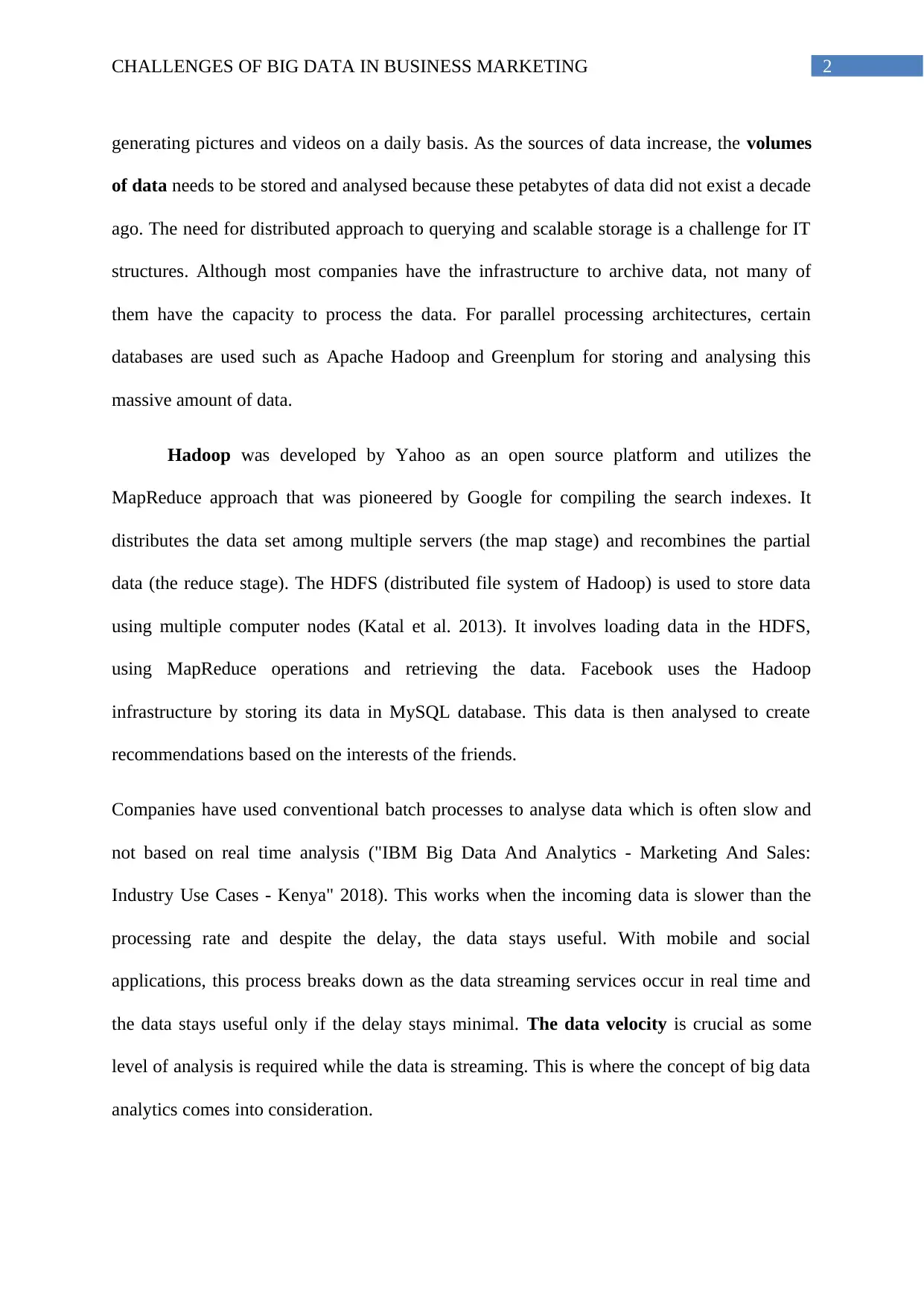
2CHALLENGES OF BIG DATA IN BUSINESS MARKETING
generating pictures and videos on a daily basis. As the sources of data increase, the volumes
of data needs to be stored and analysed because these petabytes of data did not exist a decade
ago. The need for distributed approach to querying and scalable storage is a challenge for IT
structures. Although most companies have the infrastructure to archive data, not many of
them have the capacity to process the data. For parallel processing architectures, certain
databases are used such as Apache Hadoop and Greenplum for storing and analysing this
massive amount of data.
Hadoop was developed by Yahoo as an open source platform and utilizes the
MapReduce approach that was pioneered by Google for compiling the search indexes. It
distributes the data set among multiple servers (the map stage) and recombines the partial
data (the reduce stage). The HDFS (distributed file system of Hadoop) is used to store data
using multiple computer nodes (Katal et al. 2013). It involves loading data in the HDFS,
using MapReduce operations and retrieving the data. Facebook uses the Hadoop
infrastructure by storing its data in MySQL database. This data is then analysed to create
recommendations based on the interests of the friends.
Companies have used conventional batch processes to analyse data which is often slow and
not based on real time analysis ("IBM Big Data And Analytics - Marketing And Sales:
Industry Use Cases - Kenya" 2018). This works when the incoming data is slower than the
processing rate and despite the delay, the data stays useful. With mobile and social
applications, this process breaks down as the data streaming services occur in real time and
the data stays useful only if the delay stays minimal. The data velocity is crucial as some
level of analysis is required while the data is streaming. This is where the concept of big data
analytics comes into consideration.
generating pictures and videos on a daily basis. As the sources of data increase, the volumes
of data needs to be stored and analysed because these petabytes of data did not exist a decade
ago. The need for distributed approach to querying and scalable storage is a challenge for IT
structures. Although most companies have the infrastructure to archive data, not many of
them have the capacity to process the data. For parallel processing architectures, certain
databases are used such as Apache Hadoop and Greenplum for storing and analysing this
massive amount of data.
Hadoop was developed by Yahoo as an open source platform and utilizes the
MapReduce approach that was pioneered by Google for compiling the search indexes. It
distributes the data set among multiple servers (the map stage) and recombines the partial
data (the reduce stage). The HDFS (distributed file system of Hadoop) is used to store data
using multiple computer nodes (Katal et al. 2013). It involves loading data in the HDFS,
using MapReduce operations and retrieving the data. Facebook uses the Hadoop
infrastructure by storing its data in MySQL database. This data is then analysed to create
recommendations based on the interests of the friends.
Companies have used conventional batch processes to analyse data which is often slow and
not based on real time analysis ("IBM Big Data And Analytics - Marketing And Sales:
Industry Use Cases - Kenya" 2018). This works when the incoming data is slower than the
processing rate and despite the delay, the data stays useful. With mobile and social
applications, this process breaks down as the data streaming services occur in real time and
the data stays useful only if the delay stays minimal. The data velocity is crucial as some
level of analysis is required while the data is streaming. This is where the concept of big data
analytics comes into consideration.
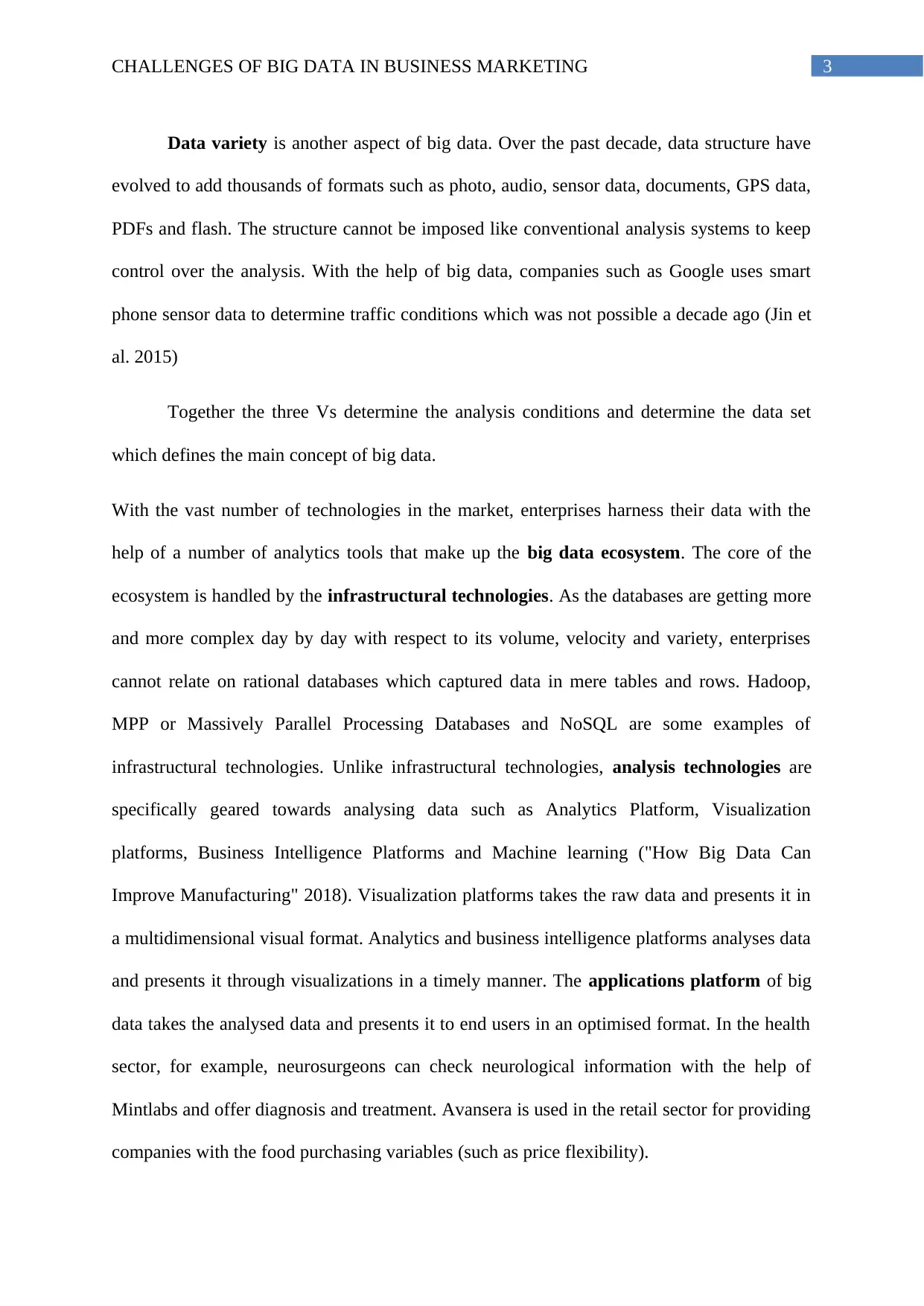
3CHALLENGES OF BIG DATA IN BUSINESS MARKETING
Data variety is another aspect of big data. Over the past decade, data structure have
evolved to add thousands of formats such as photo, audio, sensor data, documents, GPS data,
PDFs and flash. The structure cannot be imposed like conventional analysis systems to keep
control over the analysis. With the help of big data, companies such as Google uses smart
phone sensor data to determine traffic conditions which was not possible a decade ago (Jin et
al. 2015)
Together the three Vs determine the analysis conditions and determine the data set
which defines the main concept of big data.
With the vast number of technologies in the market, enterprises harness their data with the
help of a number of analytics tools that make up the big data ecosystem. The core of the
ecosystem is handled by the infrastructural technologies. As the databases are getting more
and more complex day by day with respect to its volume, velocity and variety, enterprises
cannot relate on rational databases which captured data in mere tables and rows. Hadoop,
MPP or Massively Parallel Processing Databases and NoSQL are some examples of
infrastructural technologies. Unlike infrastructural technologies, analysis technologies are
specifically geared towards analysing data such as Analytics Platform, Visualization
platforms, Business Intelligence Platforms and Machine learning ("How Big Data Can
Improve Manufacturing" 2018). Visualization platforms takes the raw data and presents it in
a multidimensional visual format. Analytics and business intelligence platforms analyses data
and presents it through visualizations in a timely manner. The applications platform of big
data takes the analysed data and presents it to end users in an optimised format. In the health
sector, for example, neurosurgeons can check neurological information with the help of
Mintlabs and offer diagnosis and treatment. Avansera is used in the retail sector for providing
companies with the food purchasing variables (such as price flexibility).
Data variety is another aspect of big data. Over the past decade, data structure have
evolved to add thousands of formats such as photo, audio, sensor data, documents, GPS data,
PDFs and flash. The structure cannot be imposed like conventional analysis systems to keep
control over the analysis. With the help of big data, companies such as Google uses smart
phone sensor data to determine traffic conditions which was not possible a decade ago (Jin et
al. 2015)
Together the three Vs determine the analysis conditions and determine the data set
which defines the main concept of big data.
With the vast number of technologies in the market, enterprises harness their data with the
help of a number of analytics tools that make up the big data ecosystem. The core of the
ecosystem is handled by the infrastructural technologies. As the databases are getting more
and more complex day by day with respect to its volume, velocity and variety, enterprises
cannot relate on rational databases which captured data in mere tables and rows. Hadoop,
MPP or Massively Parallel Processing Databases and NoSQL are some examples of
infrastructural technologies. Unlike infrastructural technologies, analysis technologies are
specifically geared towards analysing data such as Analytics Platform, Visualization
platforms, Business Intelligence Platforms and Machine learning ("How Big Data Can
Improve Manufacturing" 2018). Visualization platforms takes the raw data and presents it in
a multidimensional visual format. Analytics and business intelligence platforms analyses data
and presents it through visualizations in a timely manner. The applications platform of big
data takes the analysed data and presents it to end users in an optimised format. In the health
sector, for example, neurosurgeons can check neurological information with the help of
Mintlabs and offer diagnosis and treatment. Avansera is used in the retail sector for providing
companies with the food purchasing variables (such as price flexibility).
Secure Best Marks with AI Grader
Need help grading? Try our AI Grader for instant feedback on your assignments.
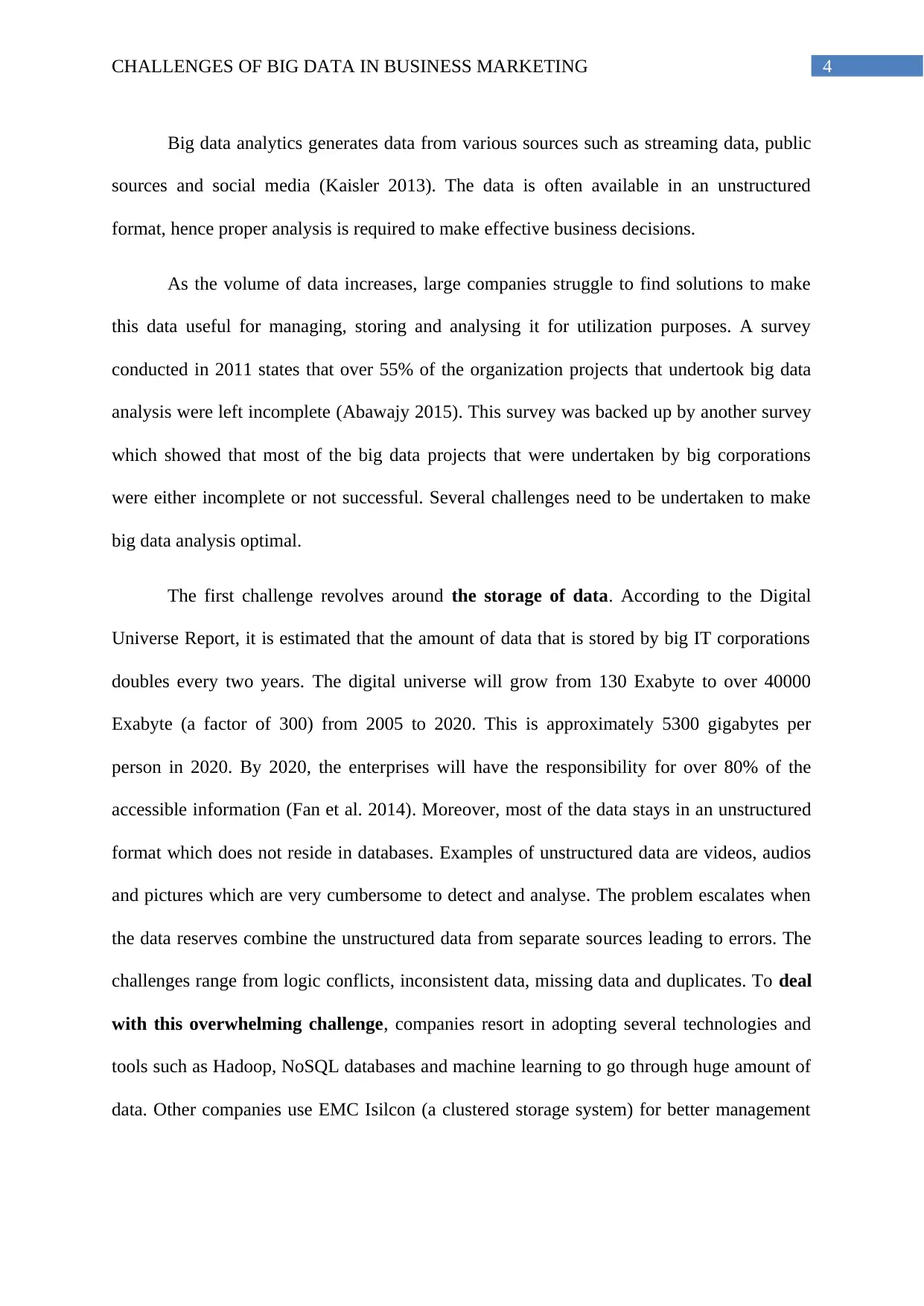
4CHALLENGES OF BIG DATA IN BUSINESS MARKETING
Big data analytics generates data from various sources such as streaming data, public
sources and social media (Kaisler 2013). The data is often available in an unstructured
format, hence proper analysis is required to make effective business decisions.
As the volume of data increases, large companies struggle to find solutions to make
this data useful for managing, storing and analysing it for utilization purposes. A survey
conducted in 2011 states that over 55% of the organization projects that undertook big data
analysis were left incomplete (Abawajy 2015). This survey was backed up by another survey
which showed that most of the big data projects that were undertaken by big corporations
were either incomplete or not successful. Several challenges need to be undertaken to make
big data analysis optimal.
The first challenge revolves around the storage of data. According to the Digital
Universe Report, it is estimated that the amount of data that is stored by big IT corporations
doubles every two years. The digital universe will grow from 130 Exabyte to over 40000
Exabyte (a factor of 300) from 2005 to 2020. This is approximately 5300 gigabytes per
person in 2020. By 2020, the enterprises will have the responsibility for over 80% of the
accessible information (Fan et al. 2014). Moreover, most of the data stays in an unstructured
format which does not reside in databases. Examples of unstructured data are videos, audios
and pictures which are very cumbersome to detect and analyse. The problem escalates when
the data reserves combine the unstructured data from separate sources leading to errors. The
challenges range from logic conflicts, inconsistent data, missing data and duplicates. To deal
with this overwhelming challenge, companies resort in adopting several technologies and
tools such as Hadoop, NoSQL databases and machine learning to go through huge amount of
data. Other companies use EMC Isilcon (a clustered storage system) for better management
Big data analytics generates data from various sources such as streaming data, public
sources and social media (Kaisler 2013). The data is often available in an unstructured
format, hence proper analysis is required to make effective business decisions.
As the volume of data increases, large companies struggle to find solutions to make
this data useful for managing, storing and analysing it for utilization purposes. A survey
conducted in 2011 states that over 55% of the organization projects that undertook big data
analysis were left incomplete (Abawajy 2015). This survey was backed up by another survey
which showed that most of the big data projects that were undertaken by big corporations
were either incomplete or not successful. Several challenges need to be undertaken to make
big data analysis optimal.
The first challenge revolves around the storage of data. According to the Digital
Universe Report, it is estimated that the amount of data that is stored by big IT corporations
doubles every two years. The digital universe will grow from 130 Exabyte to over 40000
Exabyte (a factor of 300) from 2005 to 2020. This is approximately 5300 gigabytes per
person in 2020. By 2020, the enterprises will have the responsibility for over 80% of the
accessible information (Fan et al. 2014). Moreover, most of the data stays in an unstructured
format which does not reside in databases. Examples of unstructured data are videos, audios
and pictures which are very cumbersome to detect and analyse. The problem escalates when
the data reserves combine the unstructured data from separate sources leading to errors. The
challenges range from logic conflicts, inconsistent data, missing data and duplicates. To deal
with this overwhelming challenge, companies resort in adopting several technologies and
tools such as Hadoop, NoSQL databases and machine learning to go through huge amount of
data. Other companies use EMC Isilcon (a clustered storage system) for better management
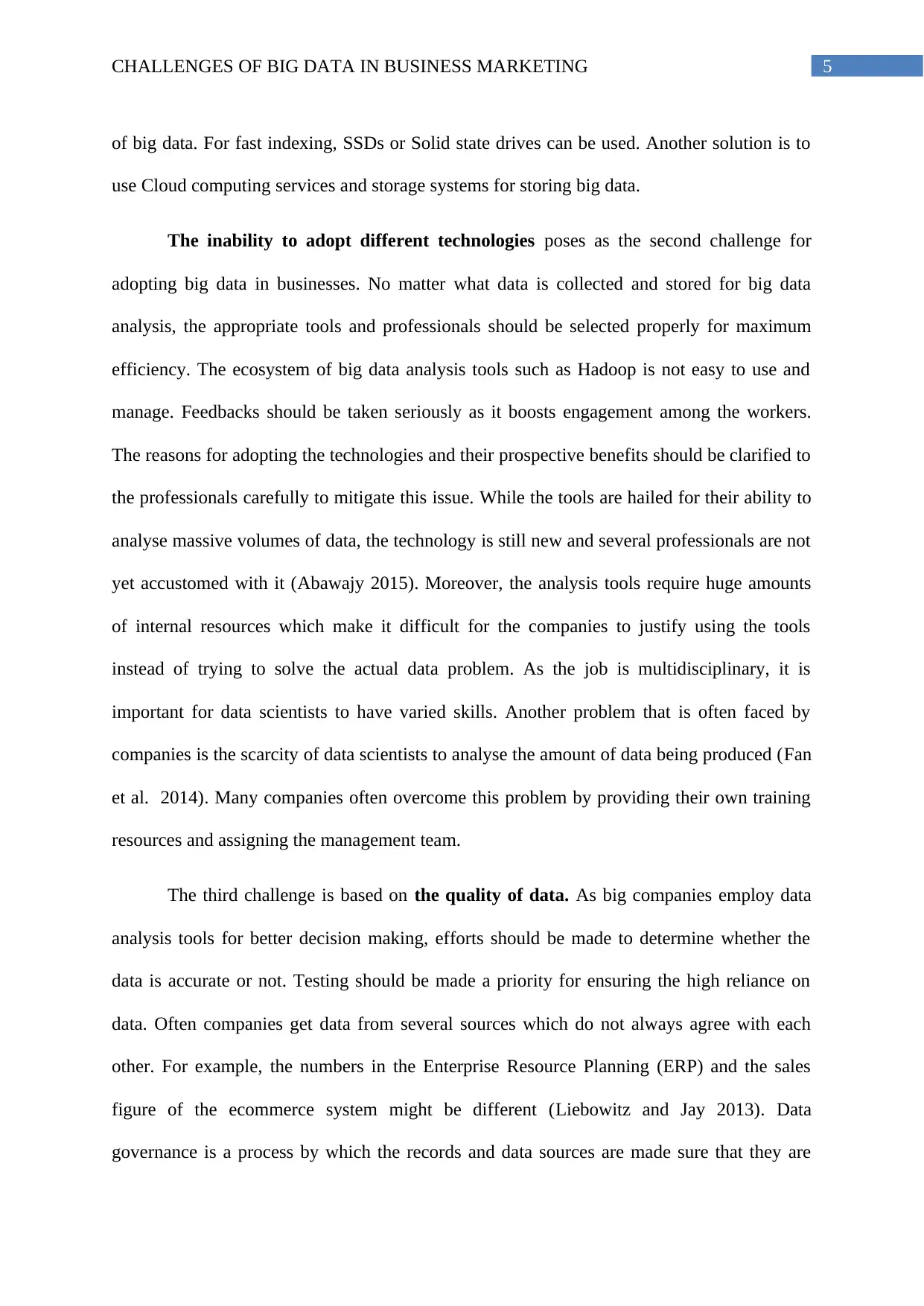
5CHALLENGES OF BIG DATA IN BUSINESS MARKETING
of big data. For fast indexing, SSDs or Solid state drives can be used. Another solution is to
use Cloud computing services and storage systems for storing big data.
The inability to adopt different technologies poses as the second challenge for
adopting big data in businesses. No matter what data is collected and stored for big data
analysis, the appropriate tools and professionals should be selected properly for maximum
efficiency. The ecosystem of big data analysis tools such as Hadoop is not easy to use and
manage. Feedbacks should be taken seriously as it boosts engagement among the workers.
The reasons for adopting the technologies and their prospective benefits should be clarified to
the professionals carefully to mitigate this issue. While the tools are hailed for their ability to
analyse massive volumes of data, the technology is still new and several professionals are not
yet accustomed with it (Abawajy 2015). Moreover, the analysis tools require huge amounts
of internal resources which make it difficult for the companies to justify using the tools
instead of trying to solve the actual data problem. As the job is multidisciplinary, it is
important for data scientists to have varied skills. Another problem that is often faced by
companies is the scarcity of data scientists to analyse the amount of data being produced (Fan
et al. 2014). Many companies often overcome this problem by providing their own training
resources and assigning the management team.
The third challenge is based on the quality of data. As big companies employ data
analysis tools for better decision making, efforts should be made to determine whether the
data is accurate or not. Testing should be made a priority for ensuring the high reliance on
data. Often companies get data from several sources which do not always agree with each
other. For example, the numbers in the Enterprise Resource Planning (ERP) and the sales
figure of the ecommerce system might be different (Liebowitz and Jay 2013). Data
governance is a process by which the records and data sources are made sure that they are
of big data. For fast indexing, SSDs or Solid state drives can be used. Another solution is to
use Cloud computing services and storage systems for storing big data.
The inability to adopt different technologies poses as the second challenge for
adopting big data in businesses. No matter what data is collected and stored for big data
analysis, the appropriate tools and professionals should be selected properly for maximum
efficiency. The ecosystem of big data analysis tools such as Hadoop is not easy to use and
manage. Feedbacks should be taken seriously as it boosts engagement among the workers.
The reasons for adopting the technologies and their prospective benefits should be clarified to
the professionals carefully to mitigate this issue. While the tools are hailed for their ability to
analyse massive volumes of data, the technology is still new and several professionals are not
yet accustomed with it (Abawajy 2015). Moreover, the analysis tools require huge amounts
of internal resources which make it difficult for the companies to justify using the tools
instead of trying to solve the actual data problem. As the job is multidisciplinary, it is
important for data scientists to have varied skills. Another problem that is often faced by
companies is the scarcity of data scientists to analyse the amount of data being produced (Fan
et al. 2014). Many companies often overcome this problem by providing their own training
resources and assigning the management team.
The third challenge is based on the quality of data. As big companies employ data
analysis tools for better decision making, efforts should be made to determine whether the
data is accurate or not. Testing should be made a priority for ensuring the high reliance on
data. Often companies get data from several sources which do not always agree with each
other. For example, the numbers in the Enterprise Resource Planning (ERP) and the sales
figure of the ecommerce system might be different (Liebowitz and Jay 2013). Data
governance is a process by which the records and data sources are made sure that they are
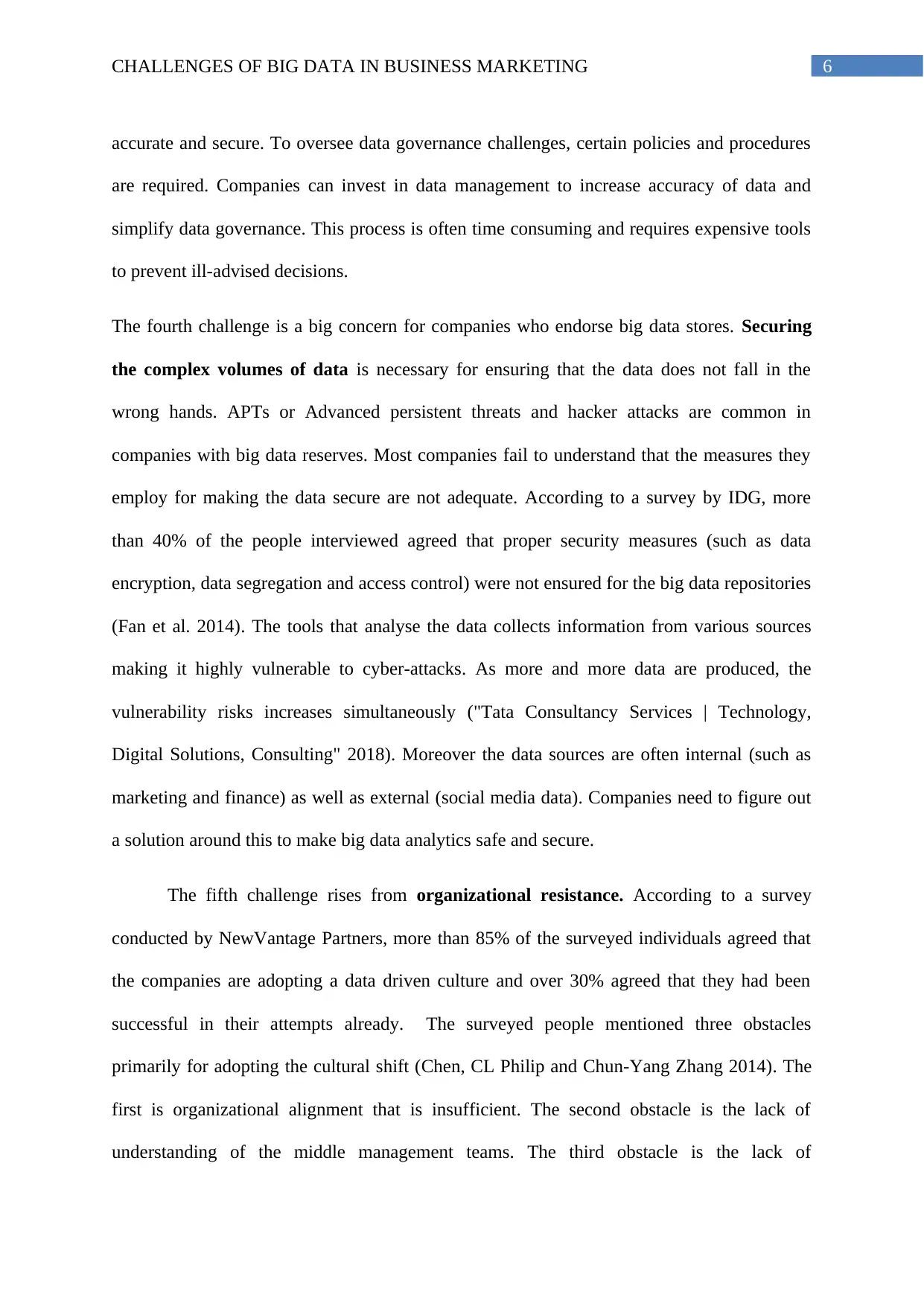
6CHALLENGES OF BIG DATA IN BUSINESS MARKETING
accurate and secure. To oversee data governance challenges, certain policies and procedures
are required. Companies can invest in data management to increase accuracy of data and
simplify data governance. This process is often time consuming and requires expensive tools
to prevent ill-advised decisions.
The fourth challenge is a big concern for companies who endorse big data stores. Securing
the complex volumes of data is necessary for ensuring that the data does not fall in the
wrong hands. APTs or Advanced persistent threats and hacker attacks are common in
companies with big data reserves. Most companies fail to understand that the measures they
employ for making the data secure are not adequate. According to a survey by IDG, more
than 40% of the people interviewed agreed that proper security measures (such as data
encryption, data segregation and access control) were not ensured for the big data repositories
(Fan et al. 2014). The tools that analyse the data collects information from various sources
making it highly vulnerable to cyber-attacks. As more and more data are produced, the
vulnerability risks increases simultaneously ("Tata Consultancy Services | Technology,
Digital Solutions, Consulting" 2018). Moreover the data sources are often internal (such as
marketing and finance) as well as external (social media data). Companies need to figure out
a solution around this to make big data analytics safe and secure.
The fifth challenge rises from organizational resistance. According to a survey
conducted by NewVantage Partners, more than 85% of the surveyed individuals agreed that
the companies are adopting a data driven culture and over 30% agreed that they had been
successful in their attempts already. The surveyed people mentioned three obstacles
primarily for adopting the cultural shift (Chen, CL Philip and Chun-Yang Zhang 2014). The
first is organizational alignment that is insufficient. The second obstacle is the lack of
understanding of the middle management teams. The third obstacle is the lack of
accurate and secure. To oversee data governance challenges, certain policies and procedures
are required. Companies can invest in data management to increase accuracy of data and
simplify data governance. This process is often time consuming and requires expensive tools
to prevent ill-advised decisions.
The fourth challenge is a big concern for companies who endorse big data stores. Securing
the complex volumes of data is necessary for ensuring that the data does not fall in the
wrong hands. APTs or Advanced persistent threats and hacker attacks are common in
companies with big data reserves. Most companies fail to understand that the measures they
employ for making the data secure are not adequate. According to a survey by IDG, more
than 40% of the people interviewed agreed that proper security measures (such as data
encryption, data segregation and access control) were not ensured for the big data repositories
(Fan et al. 2014). The tools that analyse the data collects information from various sources
making it highly vulnerable to cyber-attacks. As more and more data are produced, the
vulnerability risks increases simultaneously ("Tata Consultancy Services | Technology,
Digital Solutions, Consulting" 2018). Moreover the data sources are often internal (such as
marketing and finance) as well as external (social media data). Companies need to figure out
a solution around this to make big data analytics safe and secure.
The fifth challenge rises from organizational resistance. According to a survey
conducted by NewVantage Partners, more than 85% of the surveyed individuals agreed that
the companies are adopting a data driven culture and over 30% agreed that they had been
successful in their attempts already. The surveyed people mentioned three obstacles
primarily for adopting the cultural shift (Chen, CL Philip and Chun-Yang Zhang 2014). The
first is organizational alignment that is insufficient. The second obstacle is the lack of
understanding of the middle management teams. The third obstacle is the lack of
Paraphrase This Document
Need a fresh take? Get an instant paraphrase of this document with our AI Paraphraser
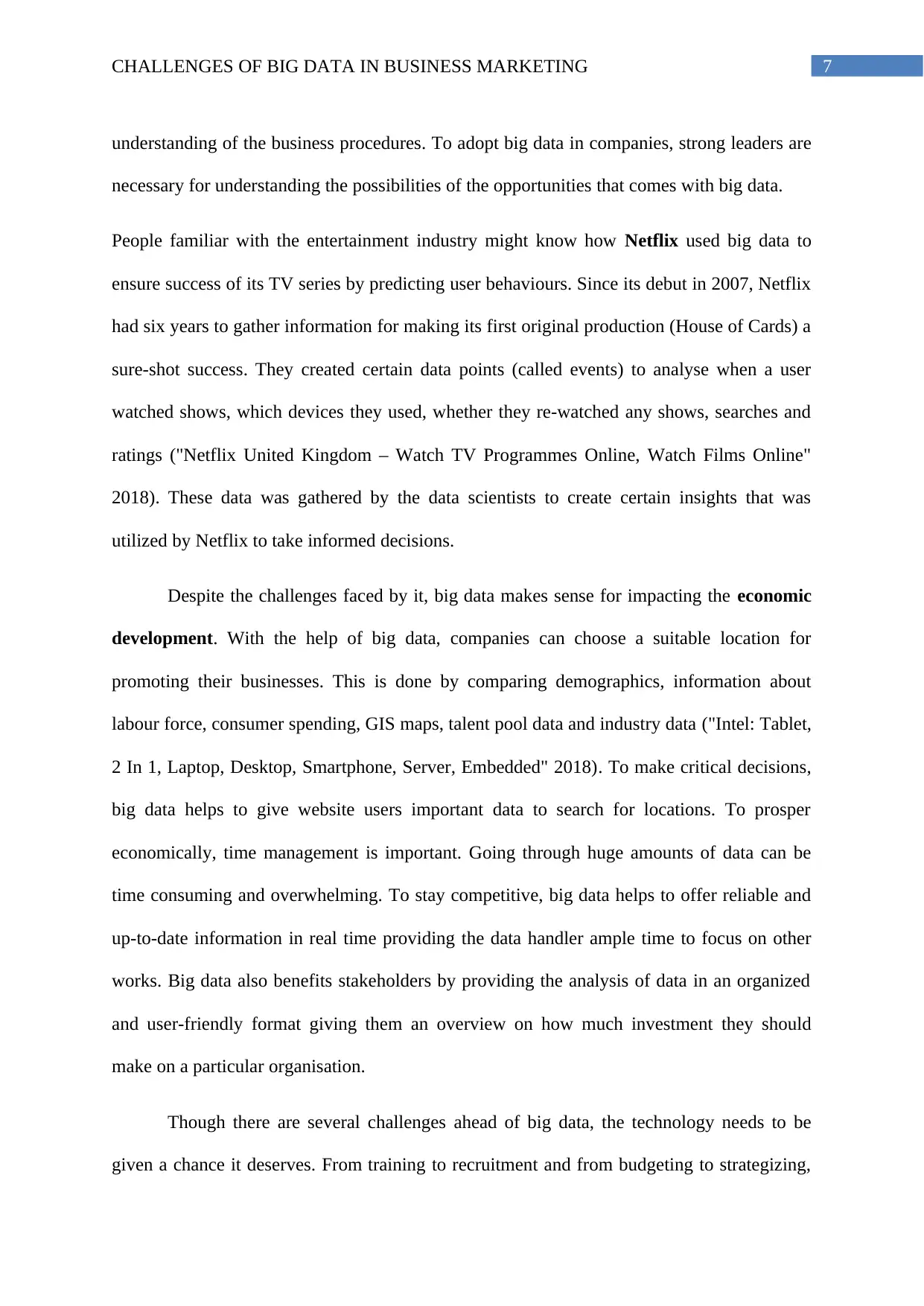
7CHALLENGES OF BIG DATA IN BUSINESS MARKETING
understanding of the business procedures. To adopt big data in companies, strong leaders are
necessary for understanding the possibilities of the opportunities that comes with big data.
People familiar with the entertainment industry might know how Netflix used big data to
ensure success of its TV series by predicting user behaviours. Since its debut in 2007, Netflix
had six years to gather information for making its first original production (House of Cards) a
sure-shot success. They created certain data points (called events) to analyse when a user
watched shows, which devices they used, whether they re-watched any shows, searches and
ratings ("Netflix United Kingdom ā Watch TV Programmes Online, Watch Films Online"
2018). These data was gathered by the data scientists to create certain insights that was
utilized by Netflix to take informed decisions.
Despite the challenges faced by it, big data makes sense for impacting the economic
development. With the help of big data, companies can choose a suitable location for
promoting their businesses. This is done by comparing demographics, information about
labour force, consumer spending, GIS maps, talent pool data and industry data ("Intel: Tablet,
2 In 1, Laptop, Desktop, Smartphone, Server, Embedded" 2018). To make critical decisions,
big data helps to give website users important data to search for locations. To prosper
economically, time management is important. Going through huge amounts of data can be
time consuming and overwhelming. To stay competitive, big data helps to offer reliable and
up-to-date information in real time providing the data handler ample time to focus on other
works. Big data also benefits stakeholders by providing the analysis of data in an organized
and user-friendly format giving them an overview on how much investment they should
make on a particular organisation.
Though there are several challenges ahead of big data, the technology needs to be
given a chance it deserves. From training to recruitment and from budgeting to strategizing,
understanding of the business procedures. To adopt big data in companies, strong leaders are
necessary for understanding the possibilities of the opportunities that comes with big data.
People familiar with the entertainment industry might know how Netflix used big data to
ensure success of its TV series by predicting user behaviours. Since its debut in 2007, Netflix
had six years to gather information for making its first original production (House of Cards) a
sure-shot success. They created certain data points (called events) to analyse when a user
watched shows, which devices they used, whether they re-watched any shows, searches and
ratings ("Netflix United Kingdom ā Watch TV Programmes Online, Watch Films Online"
2018). These data was gathered by the data scientists to create certain insights that was
utilized by Netflix to take informed decisions.
Despite the challenges faced by it, big data makes sense for impacting the economic
development. With the help of big data, companies can choose a suitable location for
promoting their businesses. This is done by comparing demographics, information about
labour force, consumer spending, GIS maps, talent pool data and industry data ("Intel: Tablet,
2 In 1, Laptop, Desktop, Smartphone, Server, Embedded" 2018). To make critical decisions,
big data helps to give website users important data to search for locations. To prosper
economically, time management is important. Going through huge amounts of data can be
time consuming and overwhelming. To stay competitive, big data helps to offer reliable and
up-to-date information in real time providing the data handler ample time to focus on other
works. Big data also benefits stakeholders by providing the analysis of data in an organized
and user-friendly format giving them an overview on how much investment they should
make on a particular organisation.
Though there are several challenges ahead of big data, the technology needs to be
given a chance it deserves. From training to recruitment and from budgeting to strategizing,
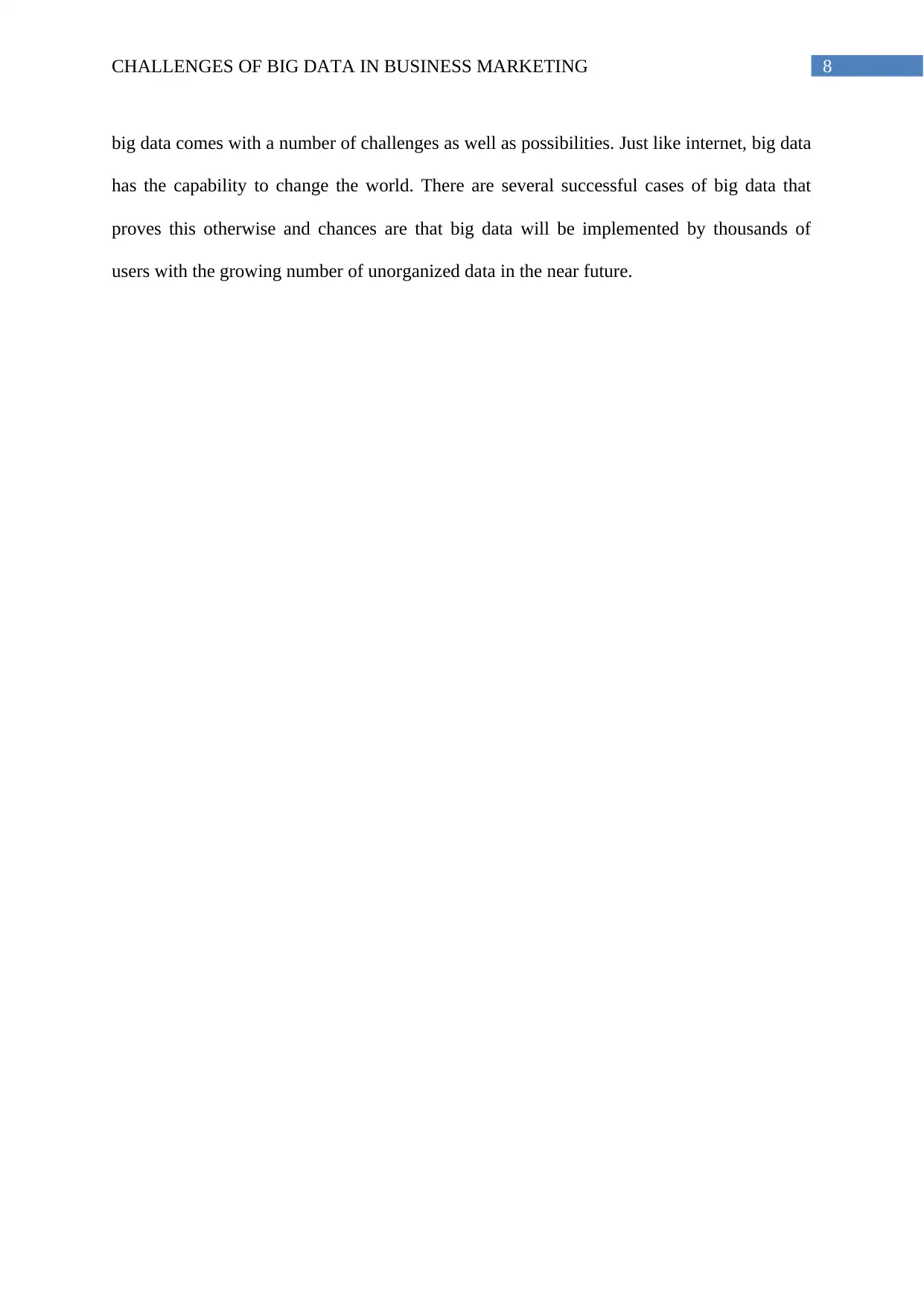
8CHALLENGES OF BIG DATA IN BUSINESS MARKETING
big data comes with a number of challenges as well as possibilities. Just like internet, big data
has the capability to change the world. There are several successful cases of big data that
proves this otherwise and chances are that big data will be implemented by thousands of
users with the growing number of unorganized data in the near future.
big data comes with a number of challenges as well as possibilities. Just like internet, big data
has the capability to change the world. There are several successful cases of big data that
proves this otherwise and chances are that big data will be implemented by thousands of
users with the growing number of unorganized data in the near future.
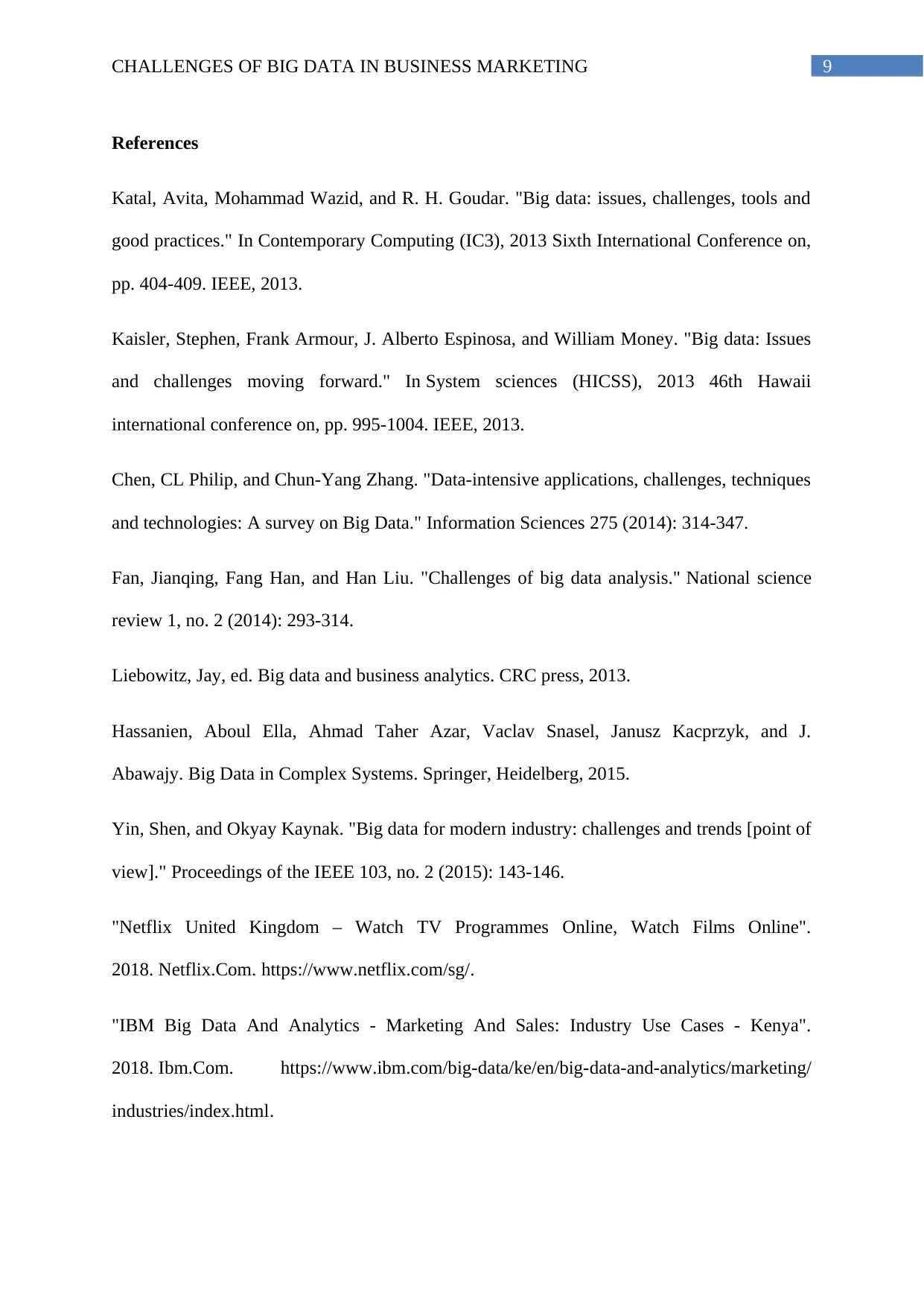
9CHALLENGES OF BIG DATA IN BUSINESS MARKETING
References
Katal, Avita, Mohammad Wazid, and R. H. Goudar. "Big data: issues, challenges, tools and
good practices." In Contemporary Computing (IC3), 2013 Sixth International Conference on,
pp. 404-409. IEEE, 2013.
Kaisler, Stephen, Frank Armour, J. Alberto Espinosa, and William Money. "Big data: Issues
and challenges moving forward." In System sciences (HICSS), 2013 46th Hawaii
international conference on, pp. 995-1004. IEEE, 2013.
Chen, CL Philip, and Chun-Yang Zhang. "Data-intensive applications, challenges, techniques
and technologies: A survey on Big Data." Information Sciences 275 (2014): 314-347.
Fan, Jianqing, Fang Han, and Han Liu. "Challenges of big data analysis." National science
review 1, no. 2 (2014): 293-314.
Liebowitz, Jay, ed. Big data and business analytics. CRC press, 2013.
Hassanien, Aboul Ella, Ahmad Taher Azar, Vaclav Snasel, Janusz Kacprzyk, and J.
Abawajy. Big Data in Complex Systems. Springer, Heidelberg, 2015.
Yin, Shen, and Okyay Kaynak. "Big data for modern industry: challenges and trends [point of
view]." Proceedings of the IEEE 103, no. 2 (2015): 143-146.
"Netflix United Kingdom ā Watch TV Programmes Online, Watch Films Online".
2018. Netflix.Com. https://www.netflix.com/sg/.
"IBM Big Data And Analytics - Marketing And Sales: Industry Use Cases - Kenya".
2018. Ibm.Com. https://www.ibm.com/big-data/ke/en/big-data-and-analytics/marketing/
industries/index.html.
References
Katal, Avita, Mohammad Wazid, and R. H. Goudar. "Big data: issues, challenges, tools and
good practices." In Contemporary Computing (IC3), 2013 Sixth International Conference on,
pp. 404-409. IEEE, 2013.
Kaisler, Stephen, Frank Armour, J. Alberto Espinosa, and William Money. "Big data: Issues
and challenges moving forward." In System sciences (HICSS), 2013 46th Hawaii
international conference on, pp. 995-1004. IEEE, 2013.
Chen, CL Philip, and Chun-Yang Zhang. "Data-intensive applications, challenges, techniques
and technologies: A survey on Big Data." Information Sciences 275 (2014): 314-347.
Fan, Jianqing, Fang Han, and Han Liu. "Challenges of big data analysis." National science
review 1, no. 2 (2014): 293-314.
Liebowitz, Jay, ed. Big data and business analytics. CRC press, 2013.
Hassanien, Aboul Ella, Ahmad Taher Azar, Vaclav Snasel, Janusz Kacprzyk, and J.
Abawajy. Big Data in Complex Systems. Springer, Heidelberg, 2015.
Yin, Shen, and Okyay Kaynak. "Big data for modern industry: challenges and trends [point of
view]." Proceedings of the IEEE 103, no. 2 (2015): 143-146.
"Netflix United Kingdom ā Watch TV Programmes Online, Watch Films Online".
2018. Netflix.Com. https://www.netflix.com/sg/.
"IBM Big Data And Analytics - Marketing And Sales: Industry Use Cases - Kenya".
2018. Ibm.Com. https://www.ibm.com/big-data/ke/en/big-data-and-analytics/marketing/
industries/index.html.
Secure Best Marks with AI Grader
Need help grading? Try our AI Grader for instant feedback on your assignments.
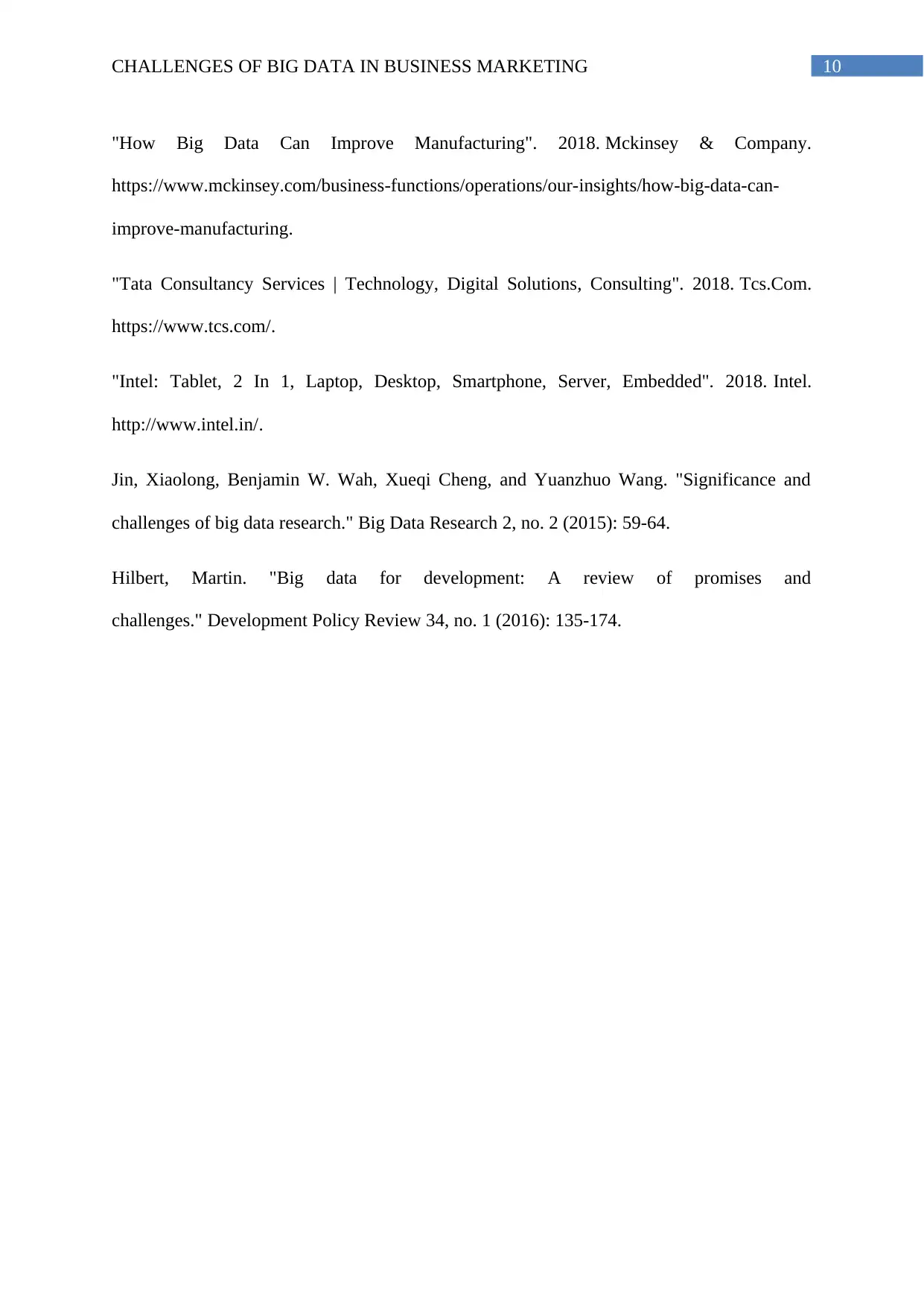
10CHALLENGES OF BIG DATA IN BUSINESS MARKETING
"How Big Data Can Improve Manufacturing". 2018. Mckinsey & Company.
https://www.mckinsey.com/business-functions/operations/our-insights/how-big-data-can-
improve-manufacturing.
"Tata Consultancy Services | Technology, Digital Solutions, Consulting". 2018. Tcs.Com.
https://www.tcs.com/.
"Intel: Tablet, 2 In 1, Laptop, Desktop, Smartphone, Server, Embedded". 2018. Intel.
http://www.intel.in/.
Jin, Xiaolong, Benjamin W. Wah, Xueqi Cheng, and Yuanzhuo Wang. "Significance and
challenges of big data research." Big Data Research 2, no. 2 (2015): 59-64.
Hilbert, Martin. "Big data for development: A review of promises and
challenges." Development Policy Review 34, no. 1 (2016): 135-174.
"How Big Data Can Improve Manufacturing". 2018. Mckinsey & Company.
https://www.mckinsey.com/business-functions/operations/our-insights/how-big-data-can-
improve-manufacturing.
"Tata Consultancy Services | Technology, Digital Solutions, Consulting". 2018. Tcs.Com.
https://www.tcs.com/.
"Intel: Tablet, 2 In 1, Laptop, Desktop, Smartphone, Server, Embedded". 2018. Intel.
http://www.intel.in/.
Jin, Xiaolong, Benjamin W. Wah, Xueqi Cheng, and Yuanzhuo Wang. "Significance and
challenges of big data research." Big Data Research 2, no. 2 (2015): 59-64.
Hilbert, Martin. "Big data for development: A review of promises and
challenges." Development Policy Review 34, no. 1 (2016): 135-174.
1 out of 11
Related Documents
![[object Object]](/_next/image/?url=%2F_next%2Fstatic%2Fmedia%2Flogo.6d15ce61.png&w=640&q=75)
Your All-in-One AI-Powered Toolkit for Academic Success.
Ā +13062052269
info@desklib.com
Available 24*7 on WhatsApp / Email
Unlock your academic potential
Ā© 2024 Ā | Ā Zucol Services PVT LTD Ā | Ā All rights reserved.