Big Data Management: Concepts and Challenges
VerifiedAdded on 2021/04/19
|9
|8129
|35
AI Summary
This assignment provides a detailed overview of big data management, covering its related technologies, challenges, and future prospects. It includes a literature review of big data analytics for IoT and smart city applications, as well as a roadmap for smart environments. The assignment also discusses energy-efficient dynamic traffic offloading and reconfiguration of networked data centers for big data stream mobile computing.
Contribute Materials
Your contribution can guide someone’s learning journey. Share your
documents today.
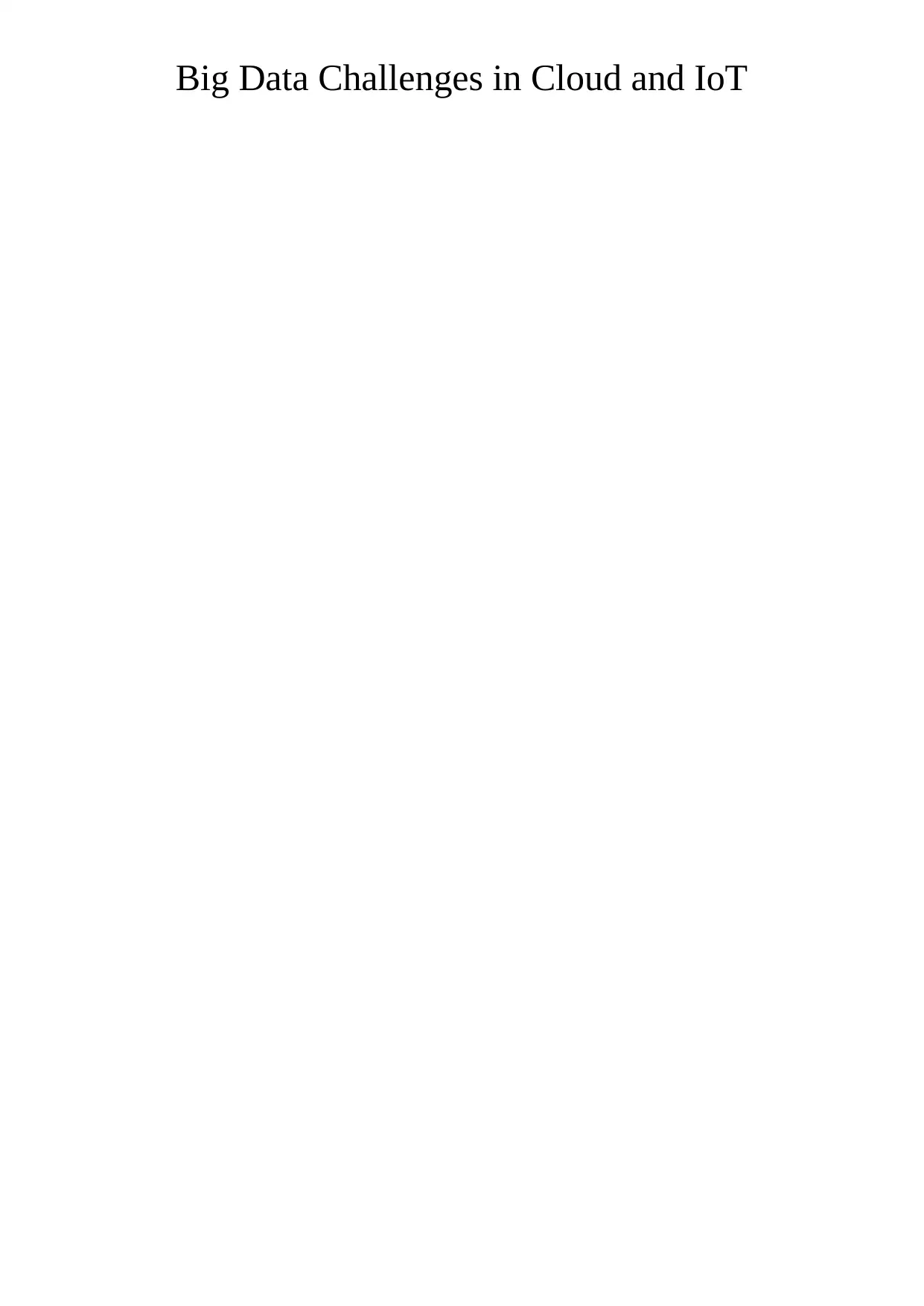
Big Data Challenges in Cloud and IoT
Secure Best Marks with AI Grader
Need help grading? Try our AI Grader for instant feedback on your assignments.
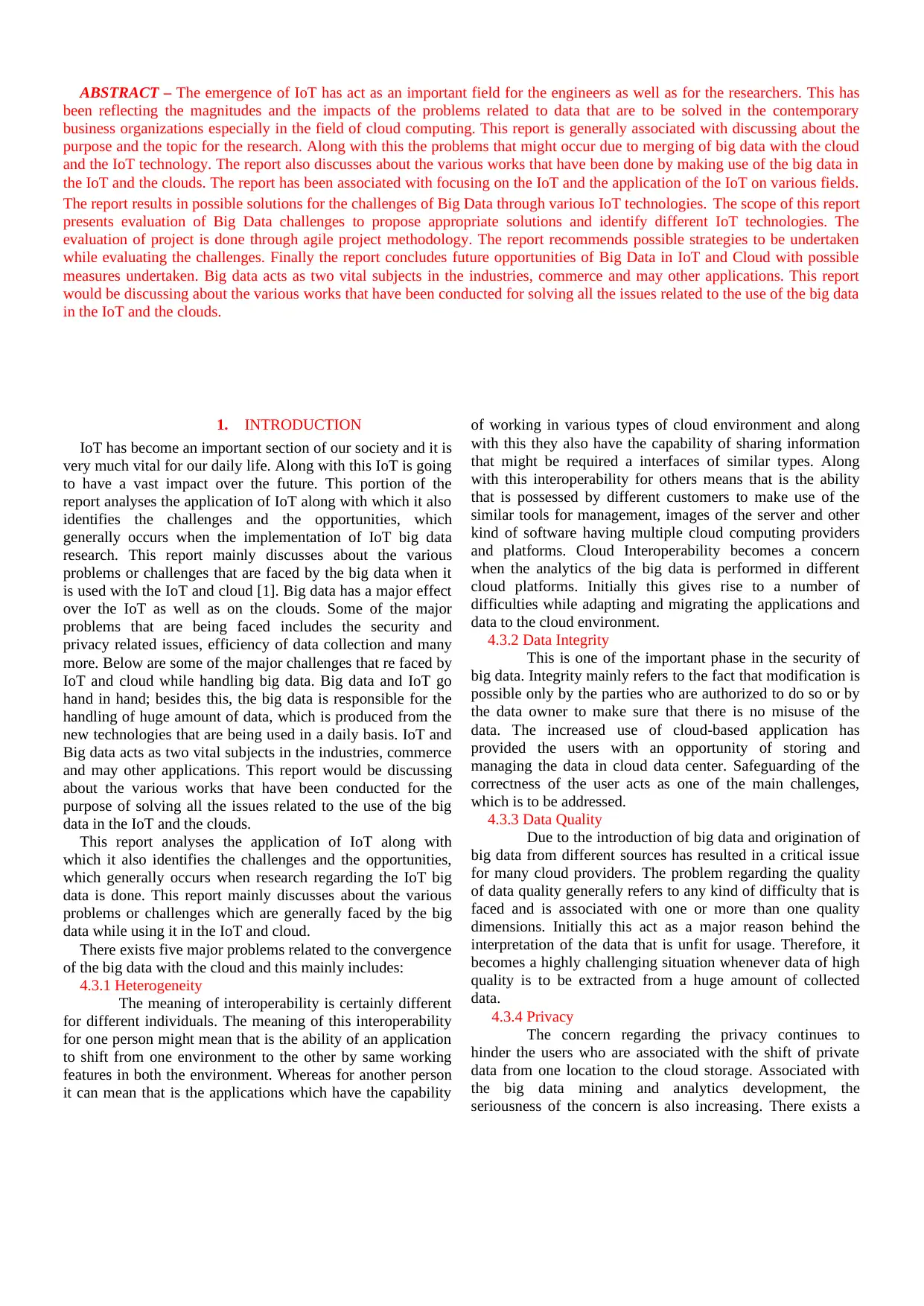
ABSTRACT – The emergence of IoT has act as an important field for the engineers as well as for the researchers. This has
been reflecting the magnitudes and the impacts of the problems related to data that are to be solved in the contemporary
business organizations especially in the field of cloud computing. This report is generally associated with discussing about the
purpose and the topic for the research. Along with this the problems that might occur due to merging of big data with the cloud
and the IoT technology. The report also discusses about the various works that have been done by making use of the big data in
the IoT and the clouds. The report has been associated with focusing on the IoT and the application of the IoT on various fields.
The report results in possible solutions for the challenges of Big Data through various IoT technologies. The scope of this report
presents evaluation of Big Data challenges to propose appropriate solutions and identify different IoT technologies. The
evaluation of project is done through agile project methodology. The report recommends possible strategies to be undertaken
while evaluating the challenges. Finally the report concludes future opportunities of Big Data in IoT and Cloud with possible
measures undertaken. Big data acts as two vital subjects in the industries, commerce and may other applications. This report
would be discussing about the various works that have been conducted for solving all the issues related to the use of the big data
in the IoT and the clouds.
1. INTRODUCTION
IoT has become an important section of our society and it is
very much vital for our daily life. Along with this IoT is going
to have a vast impact over the future. This portion of the
report analyses the application of IoT along with which it also
identifies the challenges and the opportunities, which
generally occurs when the implementation of IoT big data
research. This report mainly discusses about the various
problems or challenges that are faced by the big data when it
is used with the IoT and cloud [1]. Big data has a major effect
over the IoT as well as on the clouds. Some of the major
problems that are being faced includes the security and
privacy related issues, efficiency of data collection and many
more. Below are some of the major challenges that re faced by
IoT and cloud while handling big data. Big data and IoT go
hand in hand; besides this, the big data is responsible for the
handling of huge amount of data, which is produced from the
new technologies that are being used in a daily basis. IoT and
Big data acts as two vital subjects in the industries, commerce
and may other applications. This report would be discussing
about the various works that have been conducted for the
purpose of solving all the issues related to the use of the big
data in the IoT and the clouds.
This report analyses the application of IoT along with
which it also identifies the challenges and the opportunities,
which generally occurs when research regarding the IoT big
data is done. This report mainly discusses about the various
problems or challenges which are generally faced by the big
data while using it in the IoT and cloud.
There exists five major problems related to the convergence
of the big data with the cloud and this mainly includes:
4.3.1 Heterogeneity
The meaning of interoperability is certainly different
for different individuals. The meaning of this interoperability
for one person might mean that is the ability of an application
to shift from one environment to the other by same working
features in both the environment. Whereas for another person
it can mean that is the applications which have the capability
of working in various types of cloud environment and along
with this they also have the capability of sharing information
that might be required a interfaces of similar types. Along
with this interoperability for others means that is the ability
that is possessed by different customers to make use of the
similar tools for management, images of the server and other
kind of software having multiple cloud computing providers
and platforms. Cloud Interoperability becomes a concern
when the analytics of the big data is performed in different
cloud platforms. Initially this gives rise to a number of
difficulties while adapting and migrating the applications and
data to the cloud environment.
4.3.2 Data Integrity
This is one of the important phase in the security of
big data. Integrity mainly refers to the fact that modification is
possible only by the parties who are authorized to do so or by
the data owner to make sure that there is no misuse of the
data. The increased use of cloud-based application has
provided the users with an opportunity of storing and
managing the data in cloud data center. Safeguarding of the
correctness of the user acts as one of the main challenges,
which is to be addressed.
4.3.3 Data Quality
Due to the introduction of big data and origination of
big data from different sources has resulted in a critical issue
for many cloud providers. The problem regarding the quality
of data quality generally refers to any kind of difficulty that is
faced and is associated with one or more than one quality
dimensions. Initially this act as a major reason behind the
interpretation of the data that is unfit for usage. Therefore, it
becomes a highly challenging situation whenever data of high
quality is to be extracted from a huge amount of collected
data.
4.3.4 Privacy
The concern regarding the privacy continues to
hinder the users who are associated with the shift of private
data from one location to the cloud storage. Associated with
the big data mining and analytics development, the
seriousness of the concern is also increasing. There exists a
been reflecting the magnitudes and the impacts of the problems related to data that are to be solved in the contemporary
business organizations especially in the field of cloud computing. This report is generally associated with discussing about the
purpose and the topic for the research. Along with this the problems that might occur due to merging of big data with the cloud
and the IoT technology. The report also discusses about the various works that have been done by making use of the big data in
the IoT and the clouds. The report has been associated with focusing on the IoT and the application of the IoT on various fields.
The report results in possible solutions for the challenges of Big Data through various IoT technologies. The scope of this report
presents evaluation of Big Data challenges to propose appropriate solutions and identify different IoT technologies. The
evaluation of project is done through agile project methodology. The report recommends possible strategies to be undertaken
while evaluating the challenges. Finally the report concludes future opportunities of Big Data in IoT and Cloud with possible
measures undertaken. Big data acts as two vital subjects in the industries, commerce and may other applications. This report
would be discussing about the various works that have been conducted for solving all the issues related to the use of the big data
in the IoT and the clouds.
1. INTRODUCTION
IoT has become an important section of our society and it is
very much vital for our daily life. Along with this IoT is going
to have a vast impact over the future. This portion of the
report analyses the application of IoT along with which it also
identifies the challenges and the opportunities, which
generally occurs when the implementation of IoT big data
research. This report mainly discusses about the various
problems or challenges that are faced by the big data when it
is used with the IoT and cloud [1]. Big data has a major effect
over the IoT as well as on the clouds. Some of the major
problems that are being faced includes the security and
privacy related issues, efficiency of data collection and many
more. Below are some of the major challenges that re faced by
IoT and cloud while handling big data. Big data and IoT go
hand in hand; besides this, the big data is responsible for the
handling of huge amount of data, which is produced from the
new technologies that are being used in a daily basis. IoT and
Big data acts as two vital subjects in the industries, commerce
and may other applications. This report would be discussing
about the various works that have been conducted for the
purpose of solving all the issues related to the use of the big
data in the IoT and the clouds.
This report analyses the application of IoT along with
which it also identifies the challenges and the opportunities,
which generally occurs when research regarding the IoT big
data is done. This report mainly discusses about the various
problems or challenges which are generally faced by the big
data while using it in the IoT and cloud.
There exists five major problems related to the convergence
of the big data with the cloud and this mainly includes:
4.3.1 Heterogeneity
The meaning of interoperability is certainly different
for different individuals. The meaning of this interoperability
for one person might mean that is the ability of an application
to shift from one environment to the other by same working
features in both the environment. Whereas for another person
it can mean that is the applications which have the capability
of working in various types of cloud environment and along
with this they also have the capability of sharing information
that might be required a interfaces of similar types. Along
with this interoperability for others means that is the ability
that is possessed by different customers to make use of the
similar tools for management, images of the server and other
kind of software having multiple cloud computing providers
and platforms. Cloud Interoperability becomes a concern
when the analytics of the big data is performed in different
cloud platforms. Initially this gives rise to a number of
difficulties while adapting and migrating the applications and
data to the cloud environment.
4.3.2 Data Integrity
This is one of the important phase in the security of
big data. Integrity mainly refers to the fact that modification is
possible only by the parties who are authorized to do so or by
the data owner to make sure that there is no misuse of the
data. The increased use of cloud-based application has
provided the users with an opportunity of storing and
managing the data in cloud data center. Safeguarding of the
correctness of the user acts as one of the main challenges,
which is to be addressed.
4.3.3 Data Quality
Due to the introduction of big data and origination of
big data from different sources has resulted in a critical issue
for many cloud providers. The problem regarding the quality
of data quality generally refers to any kind of difficulty that is
faced and is associated with one or more than one quality
dimensions. Initially this act as a major reason behind the
interpretation of the data that is unfit for usage. Therefore, it
becomes a highly challenging situation whenever data of high
quality is to be extracted from a huge amount of collected
data.
4.3.4 Privacy
The concern regarding the privacy continues to
hinder the users who are associated with the shift of private
data from one location to the cloud storage. Associated with
the big data mining and analytics development, the
seriousness of the concern is also increasing. There exists a
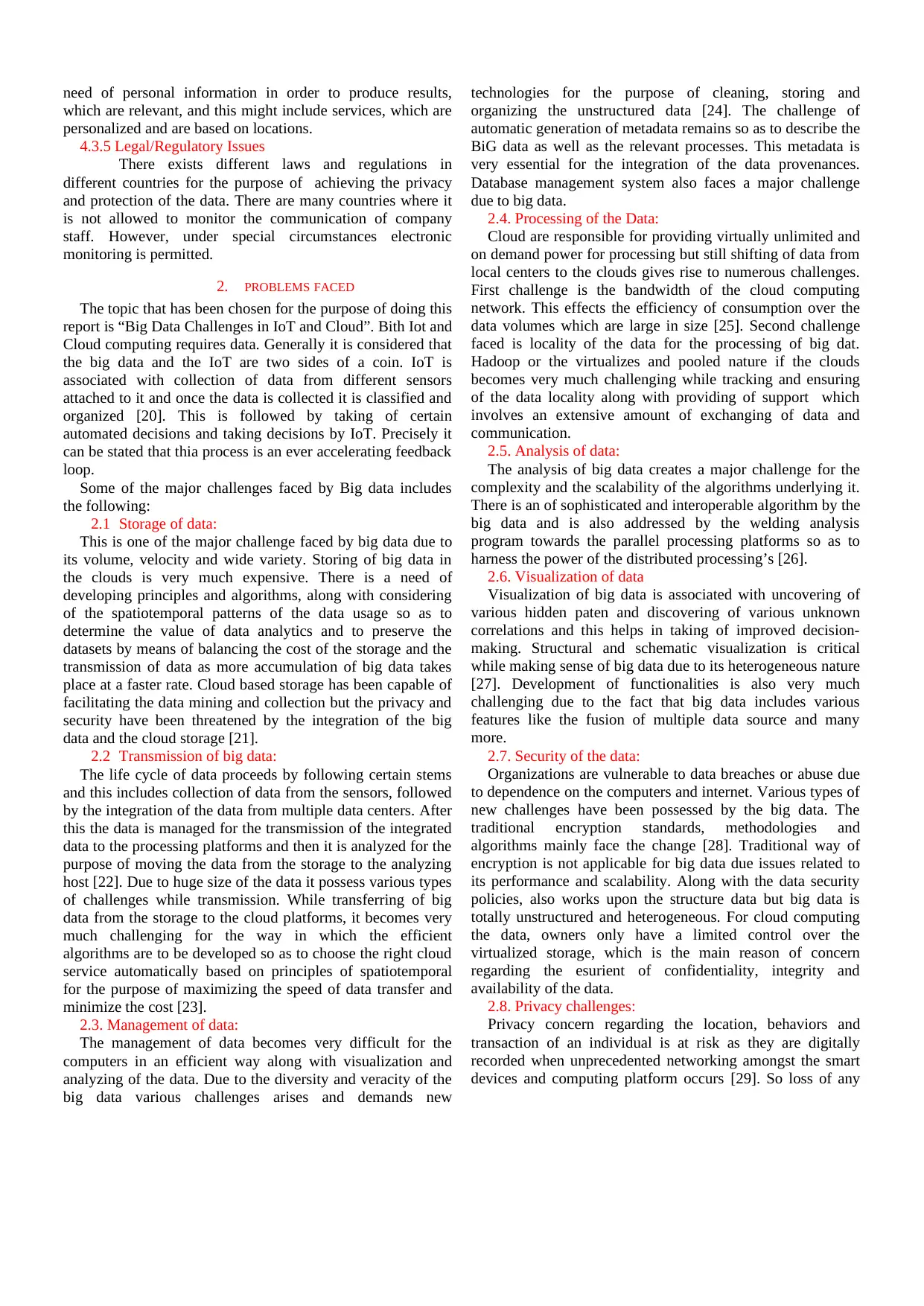
need of personal information in order to produce results,
which are relevant, and this might include services, which are
personalized and are based on locations.
4.3.5 Legal/Regulatory Issues
There exists different laws and regulations in
different countries for the purpose of achieving the privacy
and protection of the data. There are many countries where it
is not allowed to monitor the communication of company
staff. However, under special circumstances electronic
monitoring is permitted.
2. PROBLEMS FACED
The topic that has been chosen for the purpose of doing this
report is “Big Data Challenges in IoT and Cloud”. Bith Iot and
Cloud computing requires data. Generally it is considered that
the big data and the IoT are two sides of a coin. IoT is
associated with collection of data from different sensors
attached to it and once the data is collected it is classified and
organized [20]. This is followed by taking of certain
automated decisions and taking decisions by IoT. Precisely it
can be stated that thia process is an ever accelerating feedback
loop.
Some of the major challenges faced by Big data includes
the following:
2.1 Storage of data:
This is one of the major challenge faced by big data due to
its volume, velocity and wide variety. Storing of big data in
the clouds is very much expensive. There is a need of
developing principles and algorithms, along with considering
of the spatiotemporal patterns of the data usage so as to
determine the value of data analytics and to preserve the
datasets by means of balancing the cost of the storage and the
transmission of data as more accumulation of big data takes
place at a faster rate. Cloud based storage has been capable of
facilitating the data mining and collection but the privacy and
security have been threatened by the integration of the big
data and the cloud storage [21].
2.2 Transmission of big data:
The life cycle of data proceeds by following certain stems
and this includes collection of data from the sensors, followed
by the integration of the data from multiple data centers. After
this the data is managed for the transmission of the integrated
data to the processing platforms and then it is analyzed for the
purpose of moving the data from the storage to the analyzing
host [22]. Due to huge size of the data it possess various types
of challenges while transmission. While transferring of big
data from the storage to the cloud platforms, it becomes very
much challenging for the way in which the efficient
algorithms are to be developed so as to choose the right cloud
service automatically based on principles of spatiotemporal
for the purpose of maximizing the speed of data transfer and
minimize the cost [23].
2.3. Management of data:
The management of data becomes very difficult for the
computers in an efficient way along with visualization and
analyzing of the data. Due to the diversity and veracity of the
big data various challenges arises and demands new
technologies for the purpose of cleaning, storing and
organizing the unstructured data [24]. The challenge of
automatic generation of metadata remains so as to describe the
BiG data as well as the relevant processes. This metadata is
very essential for the integration of the data provenances.
Database management system also faces a major challenge
due to big data.
2.4. Processing of the Data:
Cloud are responsible for providing virtually unlimited and
on demand power for processing but still shifting of data from
local centers to the clouds gives rise to numerous challenges.
First challenge is the bandwidth of the cloud computing
network. This effects the efficiency of consumption over the
data volumes which are large in size [25]. Second challenge
faced is locality of the data for the processing of big dat.
Hadoop or the virtualizes and pooled nature if the clouds
becomes very much challenging while tracking and ensuring
of the data locality along with providing of support which
involves an extensive amount of exchanging of data and
communication.
2.5. Analysis of data:
The analysis of big data creates a major challenge for the
complexity and the scalability of the algorithms underlying it.
There is an of sophisticated and interoperable algorithm by the
big data and is also addressed by the welding analysis
program towards the parallel processing platforms so as to
harness the power of the distributed processing’s [26].
2.6. Visualization of data
Visualization of big data is associated with uncovering of
various hidden paten and discovering of various unknown
correlations and this helps in taking of improved decision-
making. Structural and schematic visualization is critical
while making sense of big data due to its heterogeneous nature
[27]. Development of functionalities is also very much
challenging due to the fact that big data includes various
features like the fusion of multiple data source and many
more.
2.7. Security of the data:
Organizations are vulnerable to data breaches or abuse due
to dependence on the computers and internet. Various types of
new challenges have been possessed by the big data. The
traditional encryption standards, methodologies and
algorithms mainly face the change [28]. Traditional way of
encryption is not applicable for big data due issues related to
its performance and scalability. Along with the data security
policies, also works upon the structure data but big data is
totally unstructured and heterogeneous. For cloud computing
the data, owners only have a limited control over the
virtualized storage, which is the main reason of concern
regarding the esurient of confidentiality, integrity and
availability of the data.
2.8. Privacy challenges:
Privacy concern regarding the location, behaviors and
transaction of an individual is at risk as they are digitally
recorded when unprecedented networking amongst the smart
devices and computing platform occurs [29]. So loss of any
which are relevant, and this might include services, which are
personalized and are based on locations.
4.3.5 Legal/Regulatory Issues
There exists different laws and regulations in
different countries for the purpose of achieving the privacy
and protection of the data. There are many countries where it
is not allowed to monitor the communication of company
staff. However, under special circumstances electronic
monitoring is permitted.
2. PROBLEMS FACED
The topic that has been chosen for the purpose of doing this
report is “Big Data Challenges in IoT and Cloud”. Bith Iot and
Cloud computing requires data. Generally it is considered that
the big data and the IoT are two sides of a coin. IoT is
associated with collection of data from different sensors
attached to it and once the data is collected it is classified and
organized [20]. This is followed by taking of certain
automated decisions and taking decisions by IoT. Precisely it
can be stated that thia process is an ever accelerating feedback
loop.
Some of the major challenges faced by Big data includes
the following:
2.1 Storage of data:
This is one of the major challenge faced by big data due to
its volume, velocity and wide variety. Storing of big data in
the clouds is very much expensive. There is a need of
developing principles and algorithms, along with considering
of the spatiotemporal patterns of the data usage so as to
determine the value of data analytics and to preserve the
datasets by means of balancing the cost of the storage and the
transmission of data as more accumulation of big data takes
place at a faster rate. Cloud based storage has been capable of
facilitating the data mining and collection but the privacy and
security have been threatened by the integration of the big
data and the cloud storage [21].
2.2 Transmission of big data:
The life cycle of data proceeds by following certain stems
and this includes collection of data from the sensors, followed
by the integration of the data from multiple data centers. After
this the data is managed for the transmission of the integrated
data to the processing platforms and then it is analyzed for the
purpose of moving the data from the storage to the analyzing
host [22]. Due to huge size of the data it possess various types
of challenges while transmission. While transferring of big
data from the storage to the cloud platforms, it becomes very
much challenging for the way in which the efficient
algorithms are to be developed so as to choose the right cloud
service automatically based on principles of spatiotemporal
for the purpose of maximizing the speed of data transfer and
minimize the cost [23].
2.3. Management of data:
The management of data becomes very difficult for the
computers in an efficient way along with visualization and
analyzing of the data. Due to the diversity and veracity of the
big data various challenges arises and demands new
technologies for the purpose of cleaning, storing and
organizing the unstructured data [24]. The challenge of
automatic generation of metadata remains so as to describe the
BiG data as well as the relevant processes. This metadata is
very essential for the integration of the data provenances.
Database management system also faces a major challenge
due to big data.
2.4. Processing of the Data:
Cloud are responsible for providing virtually unlimited and
on demand power for processing but still shifting of data from
local centers to the clouds gives rise to numerous challenges.
First challenge is the bandwidth of the cloud computing
network. This effects the efficiency of consumption over the
data volumes which are large in size [25]. Second challenge
faced is locality of the data for the processing of big dat.
Hadoop or the virtualizes and pooled nature if the clouds
becomes very much challenging while tracking and ensuring
of the data locality along with providing of support which
involves an extensive amount of exchanging of data and
communication.
2.5. Analysis of data:
The analysis of big data creates a major challenge for the
complexity and the scalability of the algorithms underlying it.
There is an of sophisticated and interoperable algorithm by the
big data and is also addressed by the welding analysis
program towards the parallel processing platforms so as to
harness the power of the distributed processing’s [26].
2.6. Visualization of data
Visualization of big data is associated with uncovering of
various hidden paten and discovering of various unknown
correlations and this helps in taking of improved decision-
making. Structural and schematic visualization is critical
while making sense of big data due to its heterogeneous nature
[27]. Development of functionalities is also very much
challenging due to the fact that big data includes various
features like the fusion of multiple data source and many
more.
2.7. Security of the data:
Organizations are vulnerable to data breaches or abuse due
to dependence on the computers and internet. Various types of
new challenges have been possessed by the big data. The
traditional encryption standards, methodologies and
algorithms mainly face the change [28]. Traditional way of
encryption is not applicable for big data due issues related to
its performance and scalability. Along with the data security
policies, also works upon the structure data but big data is
totally unstructured and heterogeneous. For cloud computing
the data, owners only have a limited control over the
virtualized storage, which is the main reason of concern
regarding the esurient of confidentiality, integrity and
availability of the data.
2.8. Privacy challenges:
Privacy concern regarding the location, behaviors and
transaction of an individual is at risk as they are digitally
recorded when unprecedented networking amongst the smart
devices and computing platform occurs [29]. So loss of any
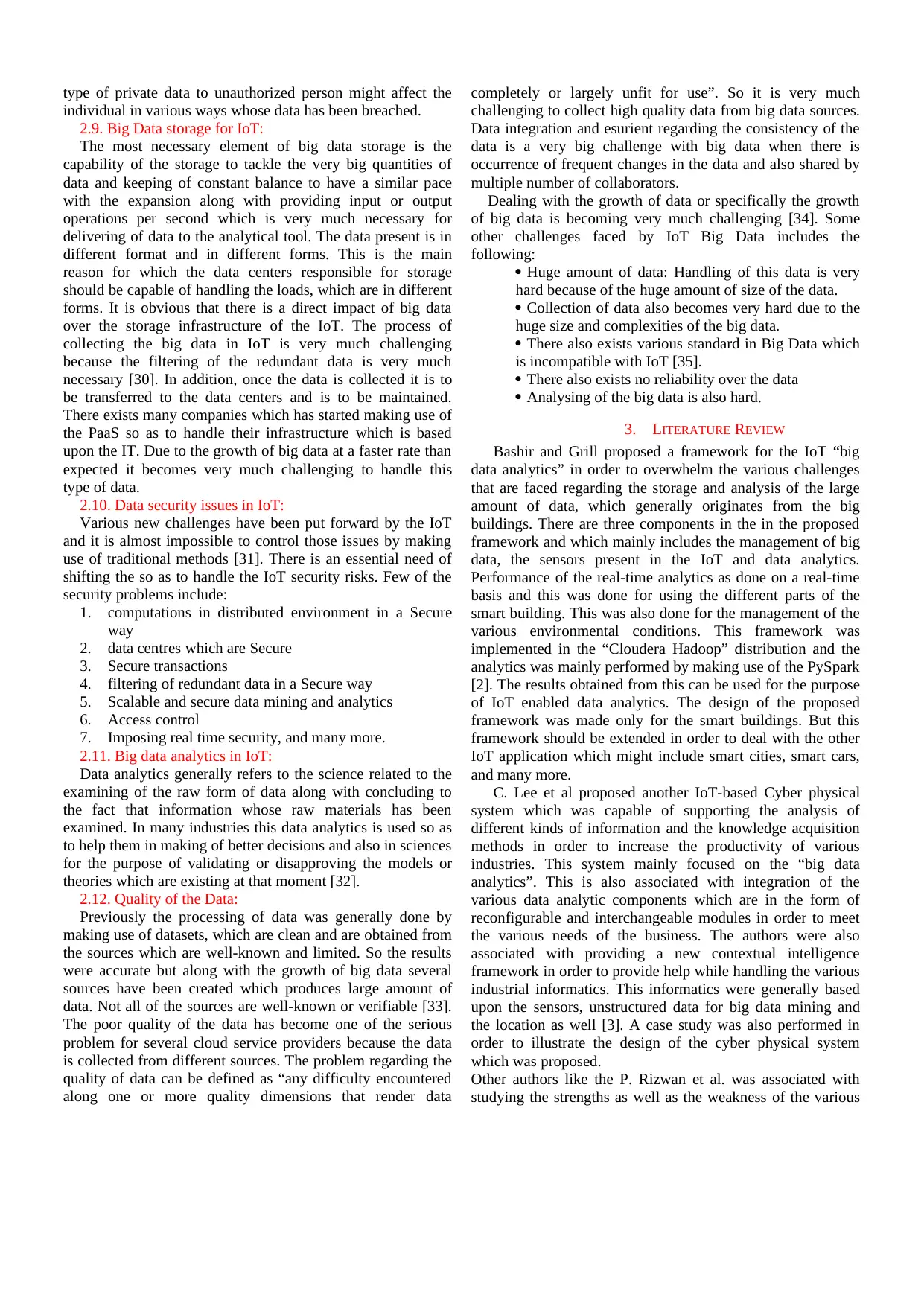
type of private data to unauthorized person might affect the
individual in various ways whose data has been breached.
2.9. Big Data storage for IoT:
The most necessary element of big data storage is the
capability of the storage to tackle the very big quantities of
data and keeping of constant balance to have a similar pace
with the expansion along with providing input or output
operations per second which is very much necessary for
delivering of data to the analytical tool. The data present is in
different format and in different forms. This is the main
reason for which the data centers responsible for storage
should be capable of handling the loads, which are in different
forms. It is obvious that there is a direct impact of big data
over the storage infrastructure of the IoT. The process of
collecting the big data in IoT is very much challenging
because the filtering of the redundant data is very much
necessary [30]. In addition, once the data is collected it is to
be transferred to the data centers and is to be maintained.
There exists many companies which has started making use of
the PaaS so as to handle their infrastructure which is based
upon the IT. Due to the growth of big data at a faster rate than
expected it becomes very much challenging to handle this
type of data.
2.10. Data security issues in IoT:
Various new challenges have been put forward by the IoT
and it is almost impossible to control those issues by making
use of traditional methods [31]. There is an essential need of
shifting the so as to handle the IoT security risks. Few of the
security problems include:
1. computations in distributed environment in a Secure
way
2. data centres which are Secure
3. Secure transactions
4. filtering of redundant data in a Secure way
5. Scalable and secure data mining and analytics
6. Access control
7. Imposing real time security, and many more.
2.11. Big data analytics in IoT:
Data analytics generally refers to the science related to the
examining of the raw form of data along with concluding to
the fact that information whose raw materials has been
examined. In many industries this data analytics is used so as
to help them in making of better decisions and also in sciences
for the purpose of validating or disapproving the models or
theories which are existing at that moment [32].
2.12. Quality of the Data:
Previously the processing of data was generally done by
making use of datasets, which are clean and are obtained from
the sources which are well-known and limited. So the results
were accurate but along with the growth of big data several
sources have been created which produces large amount of
data. Not all of the sources are well-known or verifiable [33].
The poor quality of the data has become one of the serious
problem for several cloud service providers because the data
is collected from different sources. The problem regarding the
quality of data can be defined as “any difficulty encountered
along one or more quality dimensions that render data
completely or largely unfit for use”. So it is very much
challenging to collect high quality data from big data sources.
Data integration and esurient regarding the consistency of the
data is a very big challenge with big data when there is
occurrence of frequent changes in the data and also shared by
multiple number of collaborators.
Dealing with the growth of data or specifically the growth
of big data is becoming very much challenging [34]. Some
other challenges faced by IoT Big Data includes the
following:
Huge amount of data: Handling of this data is very
hard because of the huge amount of size of the data.
Collection of data also becomes very hard due to the
huge size and complexities of the big data.
There also exists various standard in Big Data which
is incompatible with IoT [35].
There also exists no reliability over the data
Analysing of the big data is also hard.
3. LITERATURE REVIEW
Bashir and Grill proposed a framework for the IoT “big
data analytics” in order to overwhelm the various challenges
that are faced regarding the storage and analysis of the large
amount of data, which generally originates from the big
buildings. There are three components in the in the proposed
framework and which mainly includes the management of big
data, the sensors present in the IoT and data analytics.
Performance of the real-time analytics as done on a real-time
basis and this was done for using the different parts of the
smart building. This was also done for the management of the
various environmental conditions. This framework was
implemented in the “Cloudera Hadoop” distribution and the
analytics was mainly performed by making use of the PySpark
[2]. The results obtained from this can be used for the purpose
of IoT enabled data analytics. The design of the proposed
framework was made only for the smart buildings. But this
framework should be extended in order to deal with the other
IoT application which might include smart cities, smart cars,
and many more.
C. Lee et al proposed another IoT-based Cyber physical
system which was capable of supporting the analysis of
different kinds of information and the knowledge acquisition
methods in order to increase the productivity of various
industries. This system mainly focused on the “big data
analytics”. This is also associated with integration of the
various data analytic components which are in the form of
reconfigurable and interchangeable modules in order to meet
the various needs of the business. The authors were also
associated with providing a new contextual intelligence
framework in order to provide help while handling the various
industrial informatics. This informatics were generally based
upon the sensors, unstructured data for big data mining and
the location as well [3]. A case study was also performed in
order to illustrate the design of the cyber physical system
which was proposed.
Other authors like the P. Rizwan et al. was associated with
studying the strengths as well as the weakness of the various
individual in various ways whose data has been breached.
2.9. Big Data storage for IoT:
The most necessary element of big data storage is the
capability of the storage to tackle the very big quantities of
data and keeping of constant balance to have a similar pace
with the expansion along with providing input or output
operations per second which is very much necessary for
delivering of data to the analytical tool. The data present is in
different format and in different forms. This is the main
reason for which the data centers responsible for storage
should be capable of handling the loads, which are in different
forms. It is obvious that there is a direct impact of big data
over the storage infrastructure of the IoT. The process of
collecting the big data in IoT is very much challenging
because the filtering of the redundant data is very much
necessary [30]. In addition, once the data is collected it is to
be transferred to the data centers and is to be maintained.
There exists many companies which has started making use of
the PaaS so as to handle their infrastructure which is based
upon the IT. Due to the growth of big data at a faster rate than
expected it becomes very much challenging to handle this
type of data.
2.10. Data security issues in IoT:
Various new challenges have been put forward by the IoT
and it is almost impossible to control those issues by making
use of traditional methods [31]. There is an essential need of
shifting the so as to handle the IoT security risks. Few of the
security problems include:
1. computations in distributed environment in a Secure
way
2. data centres which are Secure
3. Secure transactions
4. filtering of redundant data in a Secure way
5. Scalable and secure data mining and analytics
6. Access control
7. Imposing real time security, and many more.
2.11. Big data analytics in IoT:
Data analytics generally refers to the science related to the
examining of the raw form of data along with concluding to
the fact that information whose raw materials has been
examined. In many industries this data analytics is used so as
to help them in making of better decisions and also in sciences
for the purpose of validating or disapproving the models or
theories which are existing at that moment [32].
2.12. Quality of the Data:
Previously the processing of data was generally done by
making use of datasets, which are clean and are obtained from
the sources which are well-known and limited. So the results
were accurate but along with the growth of big data several
sources have been created which produces large amount of
data. Not all of the sources are well-known or verifiable [33].
The poor quality of the data has become one of the serious
problem for several cloud service providers because the data
is collected from different sources. The problem regarding the
quality of data can be defined as “any difficulty encountered
along one or more quality dimensions that render data
completely or largely unfit for use”. So it is very much
challenging to collect high quality data from big data sources.
Data integration and esurient regarding the consistency of the
data is a very big challenge with big data when there is
occurrence of frequent changes in the data and also shared by
multiple number of collaborators.
Dealing with the growth of data or specifically the growth
of big data is becoming very much challenging [34]. Some
other challenges faced by IoT Big Data includes the
following:
Huge amount of data: Handling of this data is very
hard because of the huge amount of size of the data.
Collection of data also becomes very hard due to the
huge size and complexities of the big data.
There also exists various standard in Big Data which
is incompatible with IoT [35].
There also exists no reliability over the data
Analysing of the big data is also hard.
3. LITERATURE REVIEW
Bashir and Grill proposed a framework for the IoT “big
data analytics” in order to overwhelm the various challenges
that are faced regarding the storage and analysis of the large
amount of data, which generally originates from the big
buildings. There are three components in the in the proposed
framework and which mainly includes the management of big
data, the sensors present in the IoT and data analytics.
Performance of the real-time analytics as done on a real-time
basis and this was done for using the different parts of the
smart building. This was also done for the management of the
various environmental conditions. This framework was
implemented in the “Cloudera Hadoop” distribution and the
analytics was mainly performed by making use of the PySpark
[2]. The results obtained from this can be used for the purpose
of IoT enabled data analytics. The design of the proposed
framework was made only for the smart buildings. But this
framework should be extended in order to deal with the other
IoT application which might include smart cities, smart cars,
and many more.
C. Lee et al proposed another IoT-based Cyber physical
system which was capable of supporting the analysis of
different kinds of information and the knowledge acquisition
methods in order to increase the productivity of various
industries. This system mainly focused on the “big data
analytics”. This is also associated with integration of the
various data analytic components which are in the form of
reconfigurable and interchangeable modules in order to meet
the various needs of the business. The authors were also
associated with providing a new contextual intelligence
framework in order to provide help while handling the various
industrial informatics. This informatics were generally based
upon the sensors, unstructured data for big data mining and
the location as well [3]. A case study was also performed in
order to illustrate the design of the cyber physical system
which was proposed.
Other authors like the P. Rizwan et al. was associated with
studying the strengths as well as the weakness of the various
Secure Best Marks with AI Grader
Need help grading? Try our AI Grader for instant feedback on your assignments.
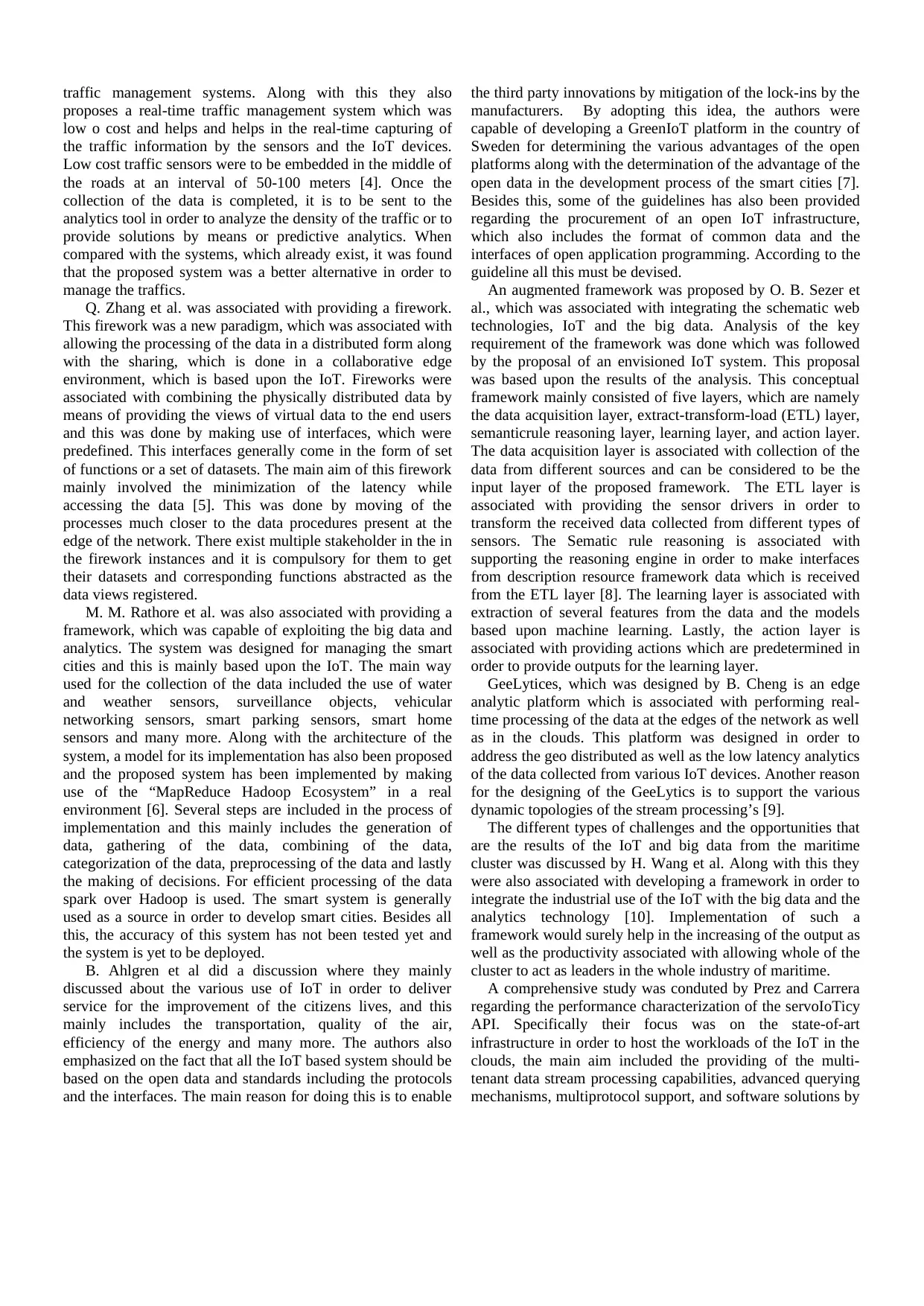
traffic management systems. Along with this they also
proposes a real-time traffic management system which was
low o cost and helps and helps in the real-time capturing of
the traffic information by the sensors and the IoT devices.
Low cost traffic sensors were to be embedded in the middle of
the roads at an interval of 50-100 meters [4]. Once the
collection of the data is completed, it is to be sent to the
analytics tool in order to analyze the density of the traffic or to
provide solutions by means or predictive analytics. When
compared with the systems, which already exist, it was found
that the proposed system was a better alternative in order to
manage the traffics.
Q. Zhang et al. was associated with providing a firework.
This firework was a new paradigm, which was associated with
allowing the processing of the data in a distributed form along
with the sharing, which is done in a collaborative edge
environment, which is based upon the IoT. Fireworks were
associated with combining the physically distributed data by
means of providing the views of virtual data to the end users
and this was done by making use of interfaces, which were
predefined. This interfaces generally come in the form of set
of functions or a set of datasets. The main aim of this firework
mainly involved the minimization of the latency while
accessing the data [5]. This was done by moving of the
processes much closer to the data procedures present at the
edge of the network. There exist multiple stakeholder in the in
the firework instances and it is compulsory for them to get
their datasets and corresponding functions abstracted as the
data views registered.
M. M. Rathore et al. was also associated with providing a
framework, which was capable of exploiting the big data and
analytics. The system was designed for managing the smart
cities and this is mainly based upon the IoT. The main way
used for the collection of the data included the use of water
and weather sensors, surveillance objects, vehicular
networking sensors, smart parking sensors, smart home
sensors and many more. Along with the architecture of the
system, a model for its implementation has also been proposed
and the proposed system has been implemented by making
use of the “MapReduce Hadoop Ecosystem” in a real
environment [6]. Several steps are included in the process of
implementation and this mainly includes the generation of
data, gathering of the data, combining of the data,
categorization of the data, preprocessing of the data and lastly
the making of decisions. For efficient processing of the data
spark over Hadoop is used. The smart system is generally
used as a source in order to develop smart cities. Besides all
this, the accuracy of this system has not been tested yet and
the system is yet to be deployed.
B. Ahlgren et al did a discussion where they mainly
discussed about the various use of IoT in order to deliver
service for the improvement of the citizens lives, and this
mainly includes the transportation, quality of the air,
efficiency of the energy and many more. The authors also
emphasized on the fact that all the IoT based system should be
based on the open data and standards including the protocols
and the interfaces. The main reason for doing this is to enable
the third party innovations by mitigation of the lock-ins by the
manufacturers. By adopting this idea, the authors were
capable of developing a GreenIoT platform in the country of
Sweden for determining the various advantages of the open
platforms along with the determination of the advantage of the
open data in the development process of the smart cities [7].
Besides this, some of the guidelines has also been provided
regarding the procurement of an open IoT infrastructure,
which also includes the format of common data and the
interfaces of open application programming. According to the
guideline all this must be devised.
An augmented framework was proposed by O. B. Sezer et
al., which was associated with integrating the schematic web
technologies, IoT and the big data. Analysis of the key
requirement of the framework was done which was followed
by the proposal of an envisioned IoT system. This proposal
was based upon the results of the analysis. This conceptual
framework mainly consisted of five layers, which are namely
the data acquisition layer, extract-transform-load (ETL) layer,
semanticrule reasoning layer, learning layer, and action layer.
The data acquisition layer is associated with collection of the
data from different sources and can be considered to be the
input layer of the proposed framework. The ETL layer is
associated with providing the sensor drivers in order to
transform the received data collected from different types of
sensors. The Sematic rule reasoning is associated with
supporting the reasoning engine in order to make interfaces
from description resource framework data which is received
from the ETL layer [8]. The learning layer is associated with
extraction of several features from the data and the models
based upon machine learning. Lastly, the action layer is
associated with providing actions which are predetermined in
order to provide outputs for the learning layer.
GeeLytices, which was designed by B. Cheng is an edge
analytic platform which is associated with performing real-
time processing of the data at the edges of the network as well
as in the clouds. This platform was designed in order to
address the geo distributed as well as the low latency analytics
of the data collected from various IoT devices. Another reason
for the designing of the GeeLytics is to support the various
dynamic topologies of the stream processing’s [9].
The different types of challenges and the opportunities that
are the results of the IoT and big data from the maritime
cluster was discussed by H. Wang et al. Along with this they
were also associated with developing a framework in order to
integrate the industrial use of the IoT with the big data and the
analytics technology [10]. Implementation of such a
framework would surely help in the increasing of the output as
well as the productivity associated with allowing whole of the
cluster to act as leaders in the whole industry of maritime.
A comprehensive study was conduted by Prez and Carrera
regarding the performance characterization of the servoIoTicy
API. Specifically their focus was on the state-of-art
infrastructure in order to host the workloads of the IoT in the
clouds, the main aim included the providing of the multi-
tenant data stream processing capabilities, advanced querying
mechanisms, multiprotocol support, and software solutions by
proposes a real-time traffic management system which was
low o cost and helps and helps in the real-time capturing of
the traffic information by the sensors and the IoT devices.
Low cost traffic sensors were to be embedded in the middle of
the roads at an interval of 50-100 meters [4]. Once the
collection of the data is completed, it is to be sent to the
analytics tool in order to analyze the density of the traffic or to
provide solutions by means or predictive analytics. When
compared with the systems, which already exist, it was found
that the proposed system was a better alternative in order to
manage the traffics.
Q. Zhang et al. was associated with providing a firework.
This firework was a new paradigm, which was associated with
allowing the processing of the data in a distributed form along
with the sharing, which is done in a collaborative edge
environment, which is based upon the IoT. Fireworks were
associated with combining the physically distributed data by
means of providing the views of virtual data to the end users
and this was done by making use of interfaces, which were
predefined. This interfaces generally come in the form of set
of functions or a set of datasets. The main aim of this firework
mainly involved the minimization of the latency while
accessing the data [5]. This was done by moving of the
processes much closer to the data procedures present at the
edge of the network. There exist multiple stakeholder in the in
the firework instances and it is compulsory for them to get
their datasets and corresponding functions abstracted as the
data views registered.
M. M. Rathore et al. was also associated with providing a
framework, which was capable of exploiting the big data and
analytics. The system was designed for managing the smart
cities and this is mainly based upon the IoT. The main way
used for the collection of the data included the use of water
and weather sensors, surveillance objects, vehicular
networking sensors, smart parking sensors, smart home
sensors and many more. Along with the architecture of the
system, a model for its implementation has also been proposed
and the proposed system has been implemented by making
use of the “MapReduce Hadoop Ecosystem” in a real
environment [6]. Several steps are included in the process of
implementation and this mainly includes the generation of
data, gathering of the data, combining of the data,
categorization of the data, preprocessing of the data and lastly
the making of decisions. For efficient processing of the data
spark over Hadoop is used. The smart system is generally
used as a source in order to develop smart cities. Besides all
this, the accuracy of this system has not been tested yet and
the system is yet to be deployed.
B. Ahlgren et al did a discussion where they mainly
discussed about the various use of IoT in order to deliver
service for the improvement of the citizens lives, and this
mainly includes the transportation, quality of the air,
efficiency of the energy and many more. The authors also
emphasized on the fact that all the IoT based system should be
based on the open data and standards including the protocols
and the interfaces. The main reason for doing this is to enable
the third party innovations by mitigation of the lock-ins by the
manufacturers. By adopting this idea, the authors were
capable of developing a GreenIoT platform in the country of
Sweden for determining the various advantages of the open
platforms along with the determination of the advantage of the
open data in the development process of the smart cities [7].
Besides this, some of the guidelines has also been provided
regarding the procurement of an open IoT infrastructure,
which also includes the format of common data and the
interfaces of open application programming. According to the
guideline all this must be devised.
An augmented framework was proposed by O. B. Sezer et
al., which was associated with integrating the schematic web
technologies, IoT and the big data. Analysis of the key
requirement of the framework was done which was followed
by the proposal of an envisioned IoT system. This proposal
was based upon the results of the analysis. This conceptual
framework mainly consisted of five layers, which are namely
the data acquisition layer, extract-transform-load (ETL) layer,
semanticrule reasoning layer, learning layer, and action layer.
The data acquisition layer is associated with collection of the
data from different sources and can be considered to be the
input layer of the proposed framework. The ETL layer is
associated with providing the sensor drivers in order to
transform the received data collected from different types of
sensors. The Sematic rule reasoning is associated with
supporting the reasoning engine in order to make interfaces
from description resource framework data which is received
from the ETL layer [8]. The learning layer is associated with
extraction of several features from the data and the models
based upon machine learning. Lastly, the action layer is
associated with providing actions which are predetermined in
order to provide outputs for the learning layer.
GeeLytices, which was designed by B. Cheng is an edge
analytic platform which is associated with performing real-
time processing of the data at the edges of the network as well
as in the clouds. This platform was designed in order to
address the geo distributed as well as the low latency analytics
of the data collected from various IoT devices. Another reason
for the designing of the GeeLytics is to support the various
dynamic topologies of the stream processing’s [9].
The different types of challenges and the opportunities that
are the results of the IoT and big data from the maritime
cluster was discussed by H. Wang et al. Along with this they
were also associated with developing a framework in order to
integrate the industrial use of the IoT with the big data and the
analytics technology [10]. Implementation of such a
framework would surely help in the increasing of the output as
well as the productivity associated with allowing whole of the
cluster to act as leaders in the whole industry of maritime.
A comprehensive study was conduted by Prez and Carrera
regarding the performance characterization of the servoIoTicy
API. Specifically their focus was on the state-of-art
infrastructure in order to host the workloads of the IoT in the
clouds, the main aim included the providing of the multi-
tenant data stream processing capabilities, advanced querying
mechanisms, multiprotocol support, and software solutions by
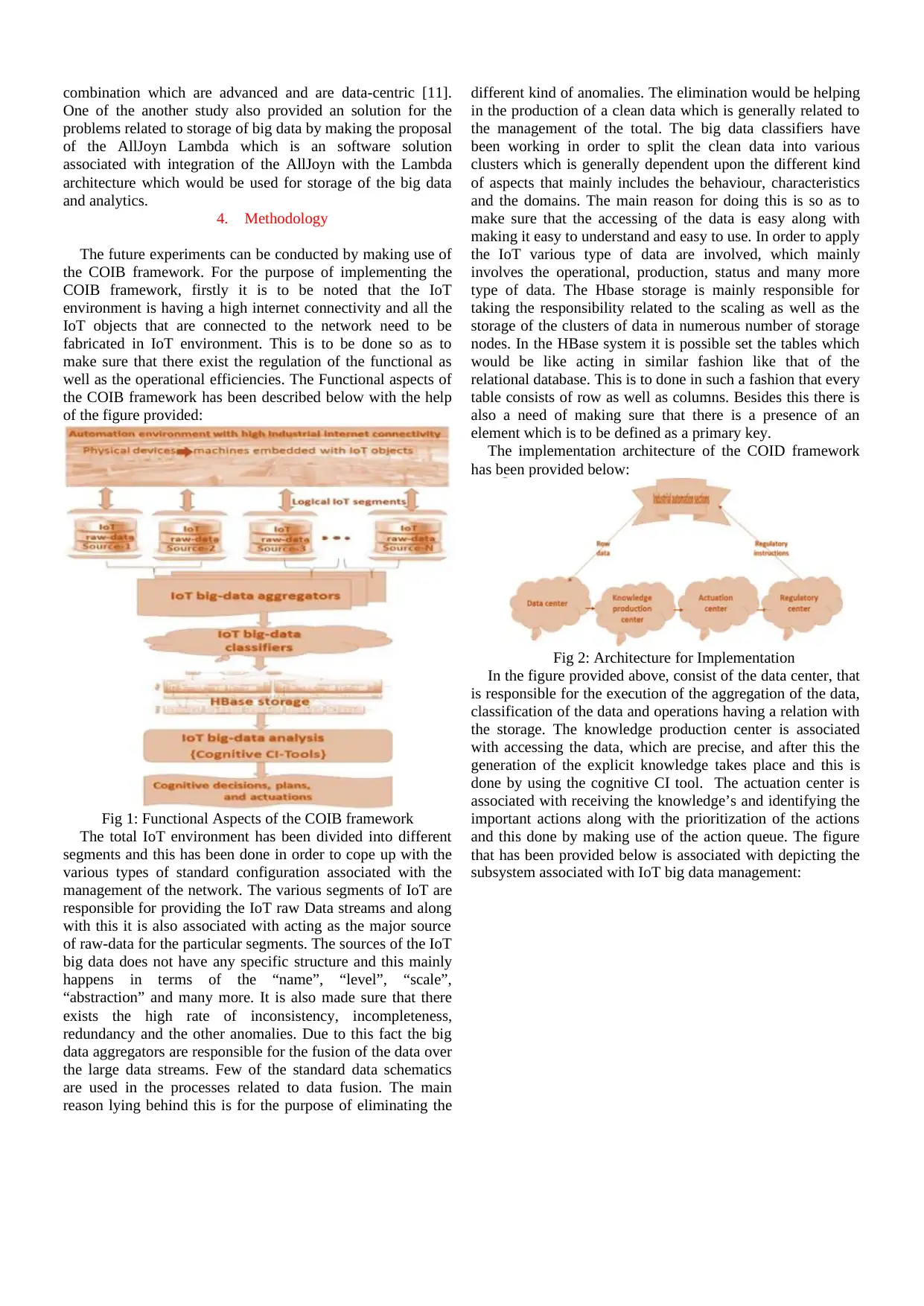
combination which are advanced and are data-centric [11].
One of the another study also provided an solution for the
problems related to storage of big data by making the proposal
of the AllJoyn Lambda which is an software solution
associated with integration of the AllJoyn with the Lambda
architecture which would be used for storage of the big data
and analytics.
4. Methodology
The future experiments can be conducted by making use of
the COIB framework. For the purpose of implementing the
COIB framework, firstly it is to be noted that the IoT
environment is having a high internet connectivity and all the
IoT objects that are connected to the network need to be
fabricated in IoT environment. This is to be done so as to
make sure that there exist the regulation of the functional as
well as the operational efficiencies. The Functional aspects of
the COIB framework has been described below with the help
of the figure provided:
Fig 1: Functional Aspects of the COIB framework
The total IoT environment has been divided into different
segments and this has been done in order to cope up with the
various types of standard configuration associated with the
management of the network. The various segments of IoT are
responsible for providing the IoT raw Data streams and along
with this it is also associated with acting as the major source
of raw-data for the particular segments. The sources of the IoT
big data does not have any specific structure and this mainly
happens in terms of the “name”, “level”, “scale”,
“abstraction” and many more. It is also made sure that there
exists the high rate of inconsistency, incompleteness,
redundancy and the other anomalies. Due to this fact the big
data aggregators are responsible for the fusion of the data over
the large data streams. Few of the standard data schematics
are used in the processes related to data fusion. The main
reason lying behind this is for the purpose of eliminating the
different kind of anomalies. The elimination would be helping
in the production of a clean data which is generally related to
the management of the total. The big data classifiers have
been working in order to split the clean data into various
clusters which is generally dependent upon the different kind
of aspects that mainly includes the behaviour, characteristics
and the domains. The main reason for doing this is so as to
make sure that the accessing of the data is easy along with
making it easy to understand and easy to use. In order to apply
the IoT various type of data are involved, which mainly
involves the operational, production, status and many more
type of data. The Hbase storage is mainly responsible for
taking the responsibility related to the scaling as well as the
storage of the clusters of data in numerous number of storage
nodes. In the HBase system it is possible set the tables which
would be like acting in similar fashion like that of the
relational database. This is to done in such a fashion that every
table consists of row as well as columns. Besides this there is
also a need of making sure that there is a presence of an
element which is to be defined as a primary key.
The implementation architecture of the COID framework
has been provided below:
Fig 2: Architecture for Implementation
In the figure provided above, consist of the data center, that
is responsible for the execution of the aggregation of the data,
classification of the data and operations having a relation with
the storage. The knowledge production center is associated
with accessing the data, which are precise, and after this the
generation of the explicit knowledge takes place and this is
done by using the cognitive CI tool. The actuation center is
associated with receiving the knowledge’s and identifying the
important actions along with the prioritization of the actions
and this done by making use of the action queue. The figure
that has been provided below is associated with depicting the
subsystem associated with IoT big data management:
One of the another study also provided an solution for the
problems related to storage of big data by making the proposal
of the AllJoyn Lambda which is an software solution
associated with integration of the AllJoyn with the Lambda
architecture which would be used for storage of the big data
and analytics.
4. Methodology
The future experiments can be conducted by making use of
the COIB framework. For the purpose of implementing the
COIB framework, firstly it is to be noted that the IoT
environment is having a high internet connectivity and all the
IoT objects that are connected to the network need to be
fabricated in IoT environment. This is to be done so as to
make sure that there exist the regulation of the functional as
well as the operational efficiencies. The Functional aspects of
the COIB framework has been described below with the help
of the figure provided:
Fig 1: Functional Aspects of the COIB framework
The total IoT environment has been divided into different
segments and this has been done in order to cope up with the
various types of standard configuration associated with the
management of the network. The various segments of IoT are
responsible for providing the IoT raw Data streams and along
with this it is also associated with acting as the major source
of raw-data for the particular segments. The sources of the IoT
big data does not have any specific structure and this mainly
happens in terms of the “name”, “level”, “scale”,
“abstraction” and many more. It is also made sure that there
exists the high rate of inconsistency, incompleteness,
redundancy and the other anomalies. Due to this fact the big
data aggregators are responsible for the fusion of the data over
the large data streams. Few of the standard data schematics
are used in the processes related to data fusion. The main
reason lying behind this is for the purpose of eliminating the
different kind of anomalies. The elimination would be helping
in the production of a clean data which is generally related to
the management of the total. The big data classifiers have
been working in order to split the clean data into various
clusters which is generally dependent upon the different kind
of aspects that mainly includes the behaviour, characteristics
and the domains. The main reason for doing this is so as to
make sure that the accessing of the data is easy along with
making it easy to understand and easy to use. In order to apply
the IoT various type of data are involved, which mainly
involves the operational, production, status and many more
type of data. The Hbase storage is mainly responsible for
taking the responsibility related to the scaling as well as the
storage of the clusters of data in numerous number of storage
nodes. In the HBase system it is possible set the tables which
would be like acting in similar fashion like that of the
relational database. This is to done in such a fashion that every
table consists of row as well as columns. Besides this there is
also a need of making sure that there is a presence of an
element which is to be defined as a primary key.
The implementation architecture of the COID framework
has been provided below:
Fig 2: Architecture for Implementation
In the figure provided above, consist of the data center, that
is responsible for the execution of the aggregation of the data,
classification of the data and operations having a relation with
the storage. The knowledge production center is associated
with accessing the data, which are precise, and after this the
generation of the explicit knowledge takes place and this is
done by using the cognitive CI tool. The actuation center is
associated with receiving the knowledge’s and identifying the
important actions along with the prioritization of the actions
and this done by making use of the action queue. The figure
that has been provided below is associated with depicting the
subsystem associated with IoT big data management:
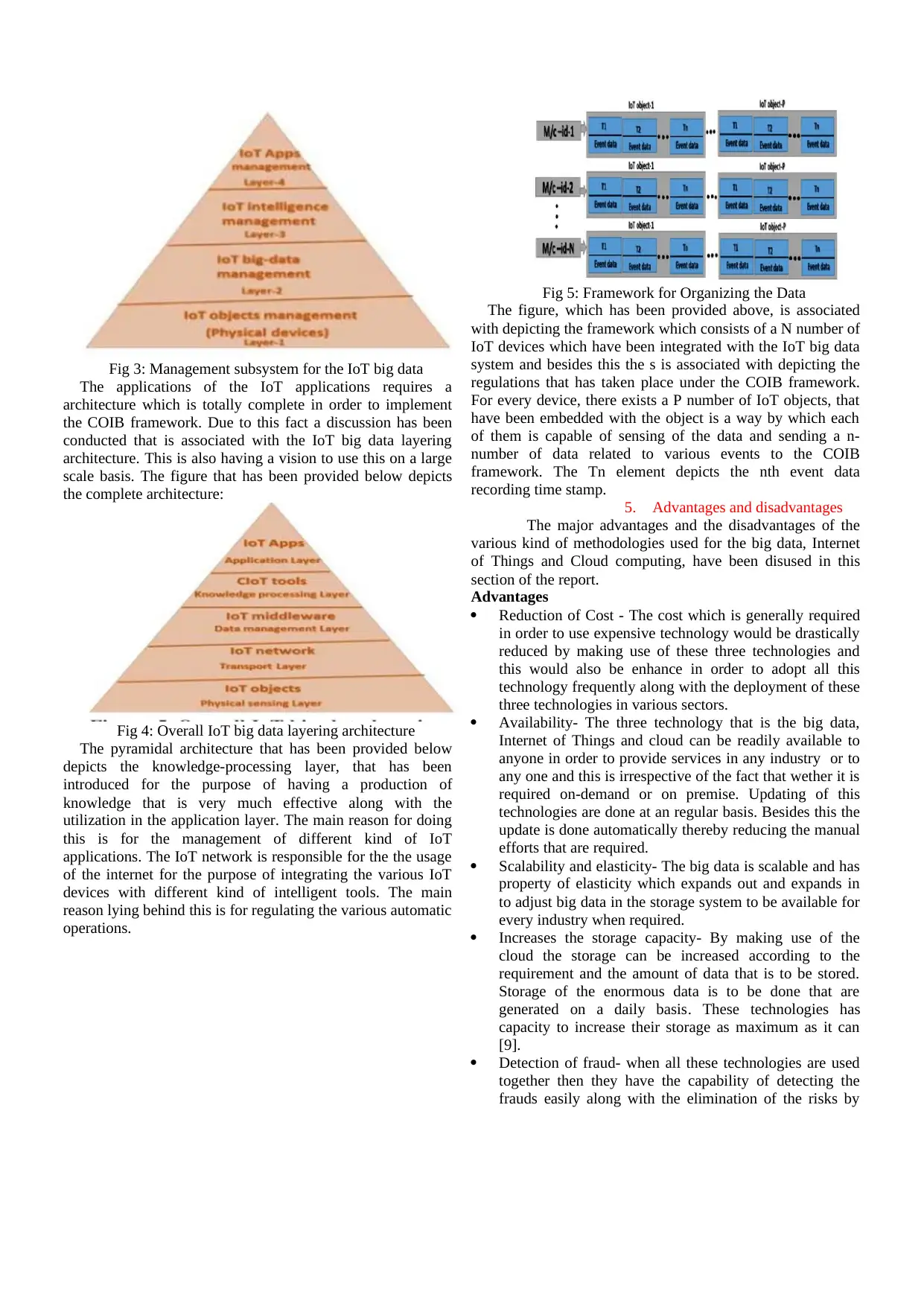
Fig 3: Management subsystem for the IoT big data
The applications of the IoT applications requires a
architecture which is totally complete in order to implement
the COIB framework. Due to this fact a discussion has been
conducted that is associated with the IoT big data layering
architecture. This is also having a vision to use this on a large
scale basis. The figure that has been provided below depicts
the complete architecture:
Fig 4: Overall IoT big data layering architecture
The pyramidal architecture that has been provided below
depicts the knowledge-processing layer, that has been
introduced for the purpose of having a production of
knowledge that is very much effective along with the
utilization in the application layer. The main reason for doing
this is for the management of different kind of IoT
applications. The IoT network is responsible for the the usage
of the internet for the purpose of integrating the various IoT
devices with different kind of intelligent tools. The main
reason lying behind this is for regulating the various automatic
operations.
Fig 5: Framework for Organizing the Data
The figure, which has been provided above, is associated
with depicting the framework which consists of a N number of
IoT devices which have been integrated with the IoT big data
system and besides this the s is associated with depicting the
regulations that has taken place under the COIB framework.
For every device, there exists a P number of IoT objects, that
have been embedded with the object is a way by which each
of them is capable of sensing of the data and sending a n-
number of data related to various events to the COIB
framework. The Tn element depicts the nth event data
recording time stamp.
5. Advantages and disadvantages
The major advantages and the disadvantages of the
various kind of methodologies used for the big data, Internet
of Things and Cloud computing, have been disused in this
section of the report.
Advantages
Reduction of Cost - The cost which is generally required
in order to use expensive technology would be drastically
reduced by making use of these three technologies and
this would also be enhance in order to adopt all this
technology frequently along with the deployment of these
three technologies in various sectors.
Availability- The three technology that is the big data,
Internet of Things and cloud can be readily available to
anyone in order to provide services in any industry or to
any one and this is irrespective of the fact that wether it is
required on-demand or on premise. Updating of this
technologies are done at an regular basis. Besides this the
update is done automatically thereby reducing the manual
efforts that are required.
Scalability and elasticity- The big data is scalable and has
property of elasticity which expands out and expands in
to adjust big data in the storage system to be available for
every industry when required.
Increases the storage capacity- By making use of the
cloud the storage can be increased according to the
requirement and the amount of data that is to be stored.
Storage of the enormous data is to be done that are
generated on a daily basis. These technologies has
capacity to increase their storage as maximum as it can
[9].
Detection of fraud- when all these technologies are used
together then they have the capability of detecting the
frauds easily along with the elimination of the risks by
The applications of the IoT applications requires a
architecture which is totally complete in order to implement
the COIB framework. Due to this fact a discussion has been
conducted that is associated with the IoT big data layering
architecture. This is also having a vision to use this on a large
scale basis. The figure that has been provided below depicts
the complete architecture:
Fig 4: Overall IoT big data layering architecture
The pyramidal architecture that has been provided below
depicts the knowledge-processing layer, that has been
introduced for the purpose of having a production of
knowledge that is very much effective along with the
utilization in the application layer. The main reason for doing
this is for the management of different kind of IoT
applications. The IoT network is responsible for the the usage
of the internet for the purpose of integrating the various IoT
devices with different kind of intelligent tools. The main
reason lying behind this is for regulating the various automatic
operations.
Fig 5: Framework for Organizing the Data
The figure, which has been provided above, is associated
with depicting the framework which consists of a N number of
IoT devices which have been integrated with the IoT big data
system and besides this the s is associated with depicting the
regulations that has taken place under the COIB framework.
For every device, there exists a P number of IoT objects, that
have been embedded with the object is a way by which each
of them is capable of sensing of the data and sending a n-
number of data related to various events to the COIB
framework. The Tn element depicts the nth event data
recording time stamp.
5. Advantages and disadvantages
The major advantages and the disadvantages of the
various kind of methodologies used for the big data, Internet
of Things and Cloud computing, have been disused in this
section of the report.
Advantages
Reduction of Cost - The cost which is generally required
in order to use expensive technology would be drastically
reduced by making use of these three technologies and
this would also be enhance in order to adopt all this
technology frequently along with the deployment of these
three technologies in various sectors.
Availability- The three technology that is the big data,
Internet of Things and cloud can be readily available to
anyone in order to provide services in any industry or to
any one and this is irrespective of the fact that wether it is
required on-demand or on premise. Updating of this
technologies are done at an regular basis. Besides this the
update is done automatically thereby reducing the manual
efforts that are required.
Scalability and elasticity- The big data is scalable and has
property of elasticity which expands out and expands in
to adjust big data in the storage system to be available for
every industry when required.
Increases the storage capacity- By making use of the
cloud the storage can be increased according to the
requirement and the amount of data that is to be stored.
Storage of the enormous data is to be done that are
generated on a daily basis. These technologies has
capacity to increase their storage as maximum as it can
[9].
Detection of fraud- when all these technologies are used
together then they have the capability of detecting the
frauds easily along with the elimination of the risks by
Paraphrase This Document
Need a fresh take? Get an instant paraphrase of this document with our AI Paraphraser
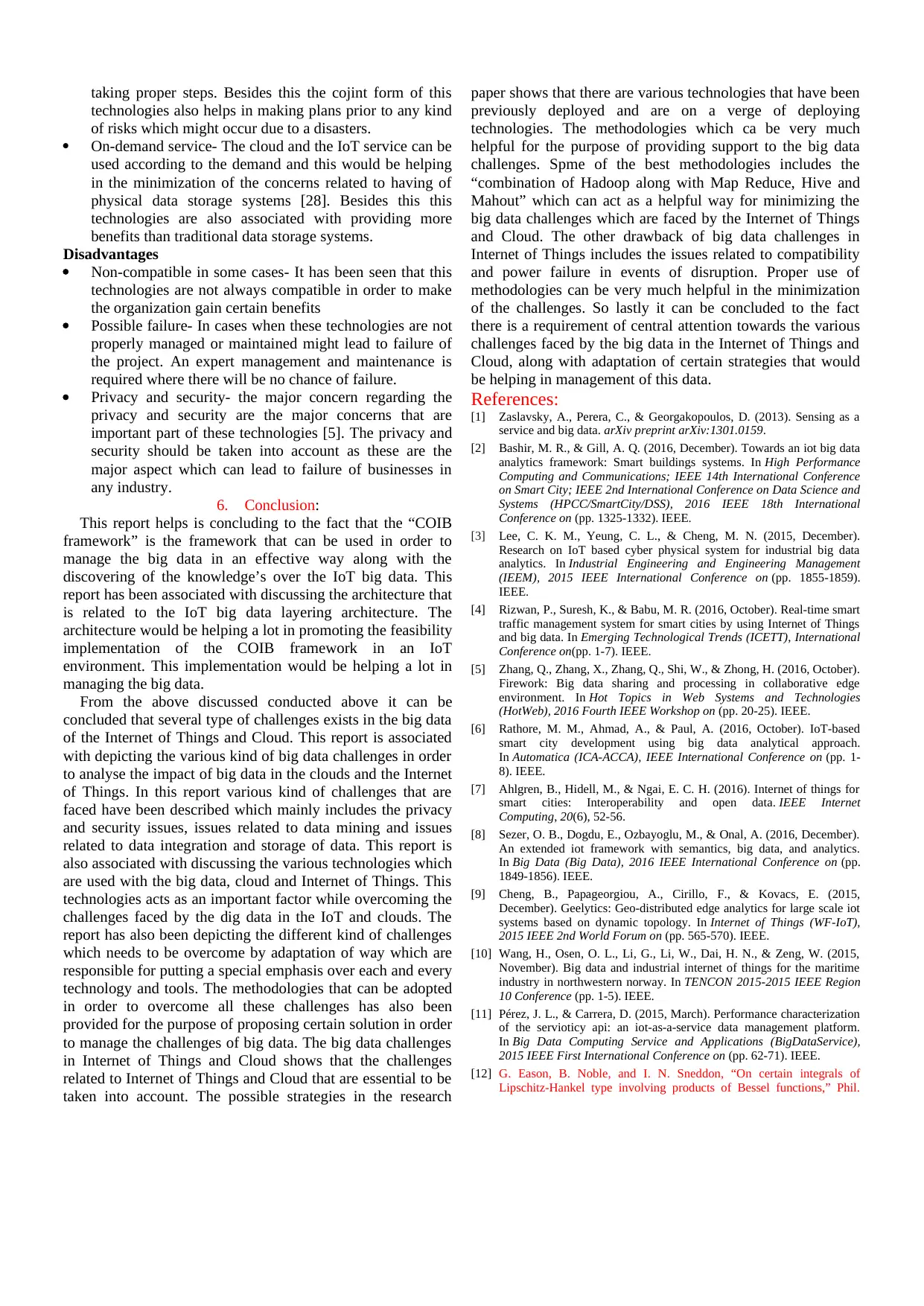
taking proper steps. Besides this the cojint form of this
technologies also helps in making plans prior to any kind
of risks which might occur due to a disasters.
On-demand service- The cloud and the IoT service can be
used according to the demand and this would be helping
in the minimization of the concerns related to having of
physical data storage systems [28]. Besides this this
technologies are also associated with providing more
benefits than traditional data storage systems.
Disadvantages
Non-compatible in some cases- It has been seen that this
technologies are not always compatible in order to make
the organization gain certain benefits
Possible failure- In cases when these technologies are not
properly managed or maintained might lead to failure of
the project. An expert management and maintenance is
required where there will be no chance of failure.
Privacy and security- the major concern regarding the
privacy and security are the major concerns that are
important part of these technologies [5]. The privacy and
security should be taken into account as these are the
major aspect which can lead to failure of businesses in
any industry.
6. Conclusion:
This report helps is concluding to the fact that the “COIB
framework” is the framework that can be used in order to
manage the big data in an effective way along with the
discovering of the knowledge’s over the IoT big data. This
report has been associated with discussing the architecture that
is related to the IoT big data layering architecture. The
architecture would be helping a lot in promoting the feasibility
implementation of the COIB framework in an IoT
environment. This implementation would be helping a lot in
managing the big data.
From the above discussed conducted above it can be
concluded that several type of challenges exists in the big data
of the Internet of Things and Cloud. This report is associated
with depicting the various kind of big data challenges in order
to analyse the impact of big data in the clouds and the Internet
of Things. In this report various kind of challenges that are
faced have been described which mainly includes the privacy
and security issues, issues related to data mining and issues
related to data integration and storage of data. This report is
also associated with discussing the various technologies which
are used with the big data, cloud and Internet of Things. This
technologies acts as an important factor while overcoming the
challenges faced by the dig data in the IoT and clouds. The
report has also been depicting the different kind of challenges
which needs to be overcome by adaptation of way which are
responsible for putting a special emphasis over each and every
technology and tools. The methodologies that can be adopted
in order to overcome all these challenges has also been
provided for the purpose of proposing certain solution in order
to manage the challenges of big data. The big data challenges
in Internet of Things and Cloud shows that the challenges
related to Internet of Things and Cloud that are essential to be
taken into account. The possible strategies in the research
paper shows that there are various technologies that have been
previously deployed and are on a verge of deploying
technologies. The methodologies which ca be very much
helpful for the purpose of providing support to the big data
challenges. Spme of the best methodologies includes the
“combination of Hadoop along with Map Reduce, Hive and
Mahout” which can act as a helpful way for minimizing the
big data challenges which are faced by the Internet of Things
and Cloud. The other drawback of big data challenges in
Internet of Things includes the issues related to compatibility
and power failure in events of disruption. Proper use of
methodologies can be very much helpful in the minimization
of the challenges. So lastly it can be concluded to the fact
there is a requirement of central attention towards the various
challenges faced by the big data in the Internet of Things and
Cloud, along with adaptation of certain strategies that would
be helping in management of this data.
References:
[1] Zaslavsky, A., Perera, C., & Georgakopoulos, D. (2013). Sensing as a
service and big data. arXiv preprint arXiv:1301.0159.
[2] Bashir, M. R., & Gill, A. Q. (2016, December). Towards an iot big data
analytics framework: Smart buildings systems. In High Performance
Computing and Communications; IEEE 14th International Conference
on Smart City; IEEE 2nd International Conference on Data Science and
Systems (HPCC/SmartCity/DSS), 2016 IEEE 18th International
Conference on (pp. 1325-1332). IEEE.
[3] Lee, C. K. M., Yeung, C. L., & Cheng, M. N. (2015, December).
Research on IoT based cyber physical system for industrial big data
analytics. In Industrial Engineering and Engineering Management
(IEEM), 2015 IEEE International Conference on (pp. 1855-1859).
IEEE.
[4] Rizwan, P., Suresh, K., & Babu, M. R. (2016, October). Real-time smart
traffic management system for smart cities by using Internet of Things
and big data. In Emerging Technological Trends (ICETT), International
Conference on(pp. 1-7). IEEE.
[5] Zhang, Q., Zhang, X., Zhang, Q., Shi, W., & Zhong, H. (2016, October).
Firework: Big data sharing and processing in collaborative edge
environment. In Hot Topics in Web Systems and Technologies
(HotWeb), 2016 Fourth IEEE Workshop on (pp. 20-25). IEEE.
[6] Rathore, M. M., Ahmad, A., & Paul, A. (2016, October). IoT-based
smart city development using big data analytical approach.
In Automatica (ICA-ACCA), IEEE International Conference on (pp. 1-
8). IEEE.
[7] Ahlgren, B., Hidell, M., & Ngai, E. C. H. (2016). Internet of things for
smart cities: Interoperability and open data. IEEE Internet
Computing, 20(6), 52-56.
[8] Sezer, O. B., Dogdu, E., Ozbayoglu, M., & Onal, A. (2016, December).
An extended iot framework with semantics, big data, and analytics.
In Big Data (Big Data), 2016 IEEE International Conference on (pp.
1849-1856). IEEE.
[9] Cheng, B., Papageorgiou, A., Cirillo, F., & Kovacs, E. (2015,
December). Geelytics: Geo-distributed edge analytics for large scale iot
systems based on dynamic topology. In Internet of Things (WF-IoT),
2015 IEEE 2nd World Forum on (pp. 565-570). IEEE.
[10] Wang, H., Osen, O. L., Li, G., Li, W., Dai, H. N., & Zeng, W. (2015,
November). Big data and industrial internet of things for the maritime
industry in northwestern norway. In TENCON 2015-2015 IEEE Region
10 Conference (pp. 1-5). IEEE.
[11] Pérez, J. L., & Carrera, D. (2015, March). Performance characterization
of the servioticy api: an iot-as-a-service data management platform.
In Big Data Computing Service and Applications (BigDataService),
2015 IEEE First International Conference on (pp. 62-71). IEEE.
[12] G. Eason, B. Noble, and I. N. Sneddon, “On certain integrals of
Lipschitz-Hankel type involving products of Bessel functions,” Phil.
technologies also helps in making plans prior to any kind
of risks which might occur due to a disasters.
On-demand service- The cloud and the IoT service can be
used according to the demand and this would be helping
in the minimization of the concerns related to having of
physical data storage systems [28]. Besides this this
technologies are also associated with providing more
benefits than traditional data storage systems.
Disadvantages
Non-compatible in some cases- It has been seen that this
technologies are not always compatible in order to make
the organization gain certain benefits
Possible failure- In cases when these technologies are not
properly managed or maintained might lead to failure of
the project. An expert management and maintenance is
required where there will be no chance of failure.
Privacy and security- the major concern regarding the
privacy and security are the major concerns that are
important part of these technologies [5]. The privacy and
security should be taken into account as these are the
major aspect which can lead to failure of businesses in
any industry.
6. Conclusion:
This report helps is concluding to the fact that the “COIB
framework” is the framework that can be used in order to
manage the big data in an effective way along with the
discovering of the knowledge’s over the IoT big data. This
report has been associated with discussing the architecture that
is related to the IoT big data layering architecture. The
architecture would be helping a lot in promoting the feasibility
implementation of the COIB framework in an IoT
environment. This implementation would be helping a lot in
managing the big data.
From the above discussed conducted above it can be
concluded that several type of challenges exists in the big data
of the Internet of Things and Cloud. This report is associated
with depicting the various kind of big data challenges in order
to analyse the impact of big data in the clouds and the Internet
of Things. In this report various kind of challenges that are
faced have been described which mainly includes the privacy
and security issues, issues related to data mining and issues
related to data integration and storage of data. This report is
also associated with discussing the various technologies which
are used with the big data, cloud and Internet of Things. This
technologies acts as an important factor while overcoming the
challenges faced by the dig data in the IoT and clouds. The
report has also been depicting the different kind of challenges
which needs to be overcome by adaptation of way which are
responsible for putting a special emphasis over each and every
technology and tools. The methodologies that can be adopted
in order to overcome all these challenges has also been
provided for the purpose of proposing certain solution in order
to manage the challenges of big data. The big data challenges
in Internet of Things and Cloud shows that the challenges
related to Internet of Things and Cloud that are essential to be
taken into account. The possible strategies in the research
paper shows that there are various technologies that have been
previously deployed and are on a verge of deploying
technologies. The methodologies which ca be very much
helpful for the purpose of providing support to the big data
challenges. Spme of the best methodologies includes the
“combination of Hadoop along with Map Reduce, Hive and
Mahout” which can act as a helpful way for minimizing the
big data challenges which are faced by the Internet of Things
and Cloud. The other drawback of big data challenges in
Internet of Things includes the issues related to compatibility
and power failure in events of disruption. Proper use of
methodologies can be very much helpful in the minimization
of the challenges. So lastly it can be concluded to the fact
there is a requirement of central attention towards the various
challenges faced by the big data in the Internet of Things and
Cloud, along with adaptation of certain strategies that would
be helping in management of this data.
References:
[1] Zaslavsky, A., Perera, C., & Georgakopoulos, D. (2013). Sensing as a
service and big data. arXiv preprint arXiv:1301.0159.
[2] Bashir, M. R., & Gill, A. Q. (2016, December). Towards an iot big data
analytics framework: Smart buildings systems. In High Performance
Computing and Communications; IEEE 14th International Conference
on Smart City; IEEE 2nd International Conference on Data Science and
Systems (HPCC/SmartCity/DSS), 2016 IEEE 18th International
Conference on (pp. 1325-1332). IEEE.
[3] Lee, C. K. M., Yeung, C. L., & Cheng, M. N. (2015, December).
Research on IoT based cyber physical system for industrial big data
analytics. In Industrial Engineering and Engineering Management
(IEEM), 2015 IEEE International Conference on (pp. 1855-1859).
IEEE.
[4] Rizwan, P., Suresh, K., & Babu, M. R. (2016, October). Real-time smart
traffic management system for smart cities by using Internet of Things
and big data. In Emerging Technological Trends (ICETT), International
Conference on(pp. 1-7). IEEE.
[5] Zhang, Q., Zhang, X., Zhang, Q., Shi, W., & Zhong, H. (2016, October).
Firework: Big data sharing and processing in collaborative edge
environment. In Hot Topics in Web Systems and Technologies
(HotWeb), 2016 Fourth IEEE Workshop on (pp. 20-25). IEEE.
[6] Rathore, M. M., Ahmad, A., & Paul, A. (2016, October). IoT-based
smart city development using big data analytical approach.
In Automatica (ICA-ACCA), IEEE International Conference on (pp. 1-
8). IEEE.
[7] Ahlgren, B., Hidell, M., & Ngai, E. C. H. (2016). Internet of things for
smart cities: Interoperability and open data. IEEE Internet
Computing, 20(6), 52-56.
[8] Sezer, O. B., Dogdu, E., Ozbayoglu, M., & Onal, A. (2016, December).
An extended iot framework with semantics, big data, and analytics.
In Big Data (Big Data), 2016 IEEE International Conference on (pp.
1849-1856). IEEE.
[9] Cheng, B., Papageorgiou, A., Cirillo, F., & Kovacs, E. (2015,
December). Geelytics: Geo-distributed edge analytics for large scale iot
systems based on dynamic topology. In Internet of Things (WF-IoT),
2015 IEEE 2nd World Forum on (pp. 565-570). IEEE.
[10] Wang, H., Osen, O. L., Li, G., Li, W., Dai, H. N., & Zeng, W. (2015,
November). Big data and industrial internet of things for the maritime
industry in northwestern norway. In TENCON 2015-2015 IEEE Region
10 Conference (pp. 1-5). IEEE.
[11] Pérez, J. L., & Carrera, D. (2015, March). Performance characterization
of the servioticy api: an iot-as-a-service data management platform.
In Big Data Computing Service and Applications (BigDataService),
2015 IEEE First International Conference on (pp. 62-71). IEEE.
[12] G. Eason, B. Noble, and I. N. Sneddon, “On certain integrals of
Lipschitz-Hankel type involving products of Bessel functions,” Phil.
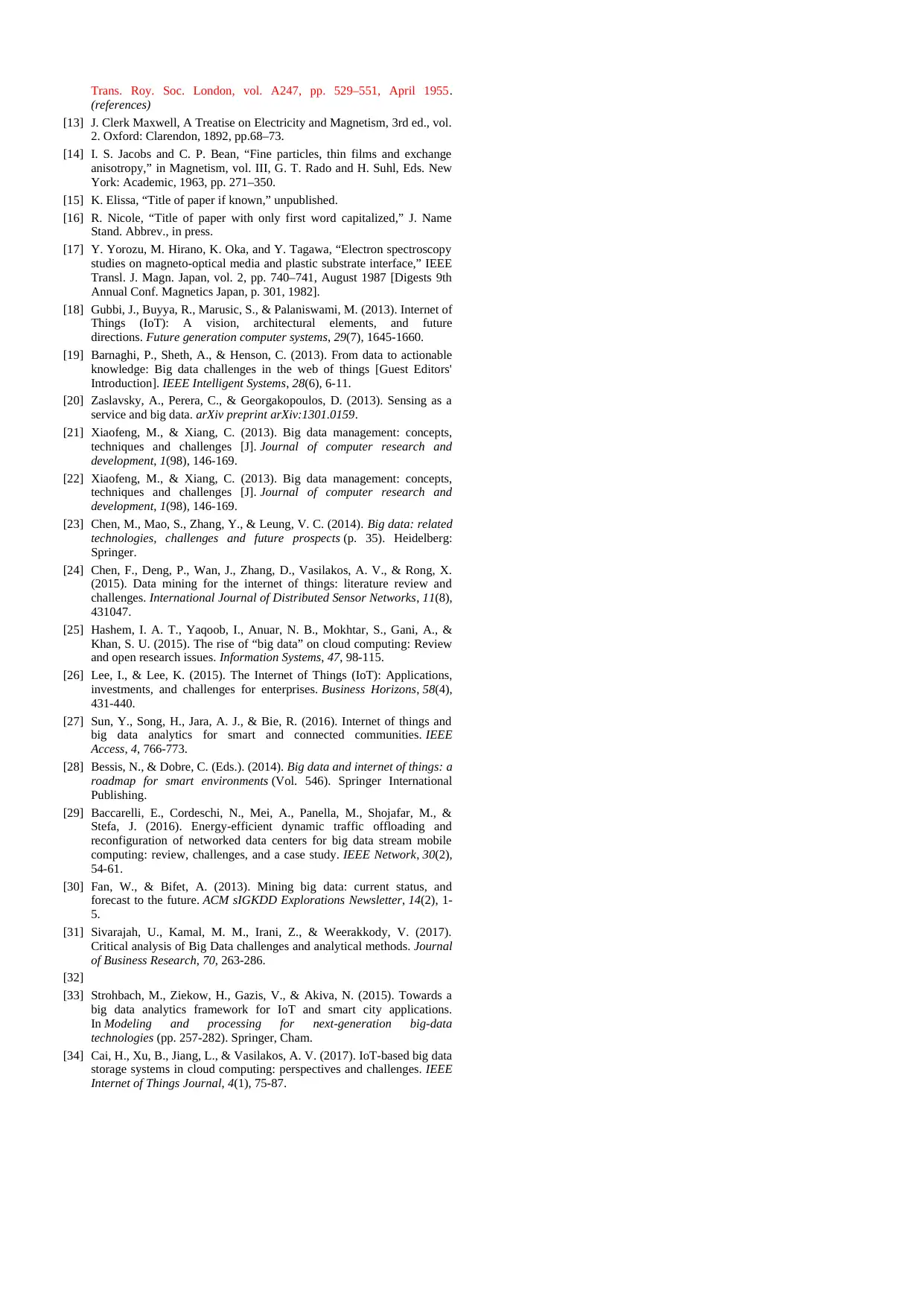
Trans. Roy. Soc. London, vol. A247, pp. 529–551, April 1955.
(references)
[13] J. Clerk Maxwell, A Treatise on Electricity and Magnetism, 3rd ed., vol.
2. Oxford: Clarendon, 1892, pp.68–73.
[14] I. S. Jacobs and C. P. Bean, “Fine particles, thin films and exchange
anisotropy,” in Magnetism, vol. III, G. T. Rado and H. Suhl, Eds. New
York: Academic, 1963, pp. 271–350.
[15] K. Elissa, “Title of paper if known,” unpublished.
[16] R. Nicole, “Title of paper with only first word capitalized,” J. Name
Stand. Abbrev., in press.
[17] Y. Yorozu, M. Hirano, K. Oka, and Y. Tagawa, “Electron spectroscopy
studies on magneto-optical media and plastic substrate interface,” IEEE
Transl. J. Magn. Japan, vol. 2, pp. 740–741, August 1987 [Digests 9th
Annual Conf. Magnetics Japan, p. 301, 1982].
[18] Gubbi, J., Buyya, R., Marusic, S., & Palaniswami, M. (2013). Internet of
Things (IoT): A vision, architectural elements, and future
directions. Future generation computer systems, 29(7), 1645-1660.
[19] Barnaghi, P., Sheth, A., & Henson, C. (2013). From data to actionable
knowledge: Big data challenges in the web of things [Guest Editors'
Introduction]. IEEE Intelligent Systems, 28(6), 6-11.
[20] Zaslavsky, A., Perera, C., & Georgakopoulos, D. (2013). Sensing as a
service and big data. arXiv preprint arXiv:1301.0159.
[21] Xiaofeng, M., & Xiang, C. (2013). Big data management: concepts,
techniques and challenges [J]. Journal of computer research and
development, 1(98), 146-169.
[22] Xiaofeng, M., & Xiang, C. (2013). Big data management: concepts,
techniques and challenges [J]. Journal of computer research and
development, 1(98), 146-169.
[23] Chen, M., Mao, S., Zhang, Y., & Leung, V. C. (2014). Big data: related
technologies, challenges and future prospects (p. 35). Heidelberg:
Springer.
[24] Chen, F., Deng, P., Wan, J., Zhang, D., Vasilakos, A. V., & Rong, X.
(2015). Data mining for the internet of things: literature review and
challenges. International Journal of Distributed Sensor Networks, 11(8),
431047.
[25] Hashem, I. A. T., Yaqoob, I., Anuar, N. B., Mokhtar, S., Gani, A., &
Khan, S. U. (2015). The rise of “big data” on cloud computing: Review
and open research issues. Information Systems, 47, 98-115.
[26] Lee, I., & Lee, K. (2015). The Internet of Things (IoT): Applications,
investments, and challenges for enterprises. Business Horizons, 58(4),
431-440.
[27] Sun, Y., Song, H., Jara, A. J., & Bie, R. (2016). Internet of things and
big data analytics for smart and connected communities. IEEE
Access, 4, 766-773.
[28] Bessis, N., & Dobre, C. (Eds.). (2014). Big data and internet of things: a
roadmap for smart environments (Vol. 546). Springer International
Publishing.
[29] Baccarelli, E., Cordeschi, N., Mei, A., Panella, M., Shojafar, M., &
Stefa, J. (2016). Energy-efficient dynamic traffic offloading and
reconfiguration of networked data centers for big data stream mobile
computing: review, challenges, and a case study. IEEE Network, 30(2),
54-61.
[30] Fan, W., & Bifet, A. (2013). Mining big data: current status, and
forecast to the future. ACM sIGKDD Explorations Newsletter, 14(2), 1-
5.
[31] Sivarajah, U., Kamal, M. M., Irani, Z., & Weerakkody, V. (2017).
Critical analysis of Big Data challenges and analytical methods. Journal
of Business Research, 70, 263-286.
[32]
[33] Strohbach, M., Ziekow, H., Gazis, V., & Akiva, N. (2015). Towards a
big data analytics framework for IoT and smart city applications.
In Modeling and processing for next-generation big-data
technologies (pp. 257-282). Springer, Cham.
[34] Cai, H., Xu, B., Jiang, L., & Vasilakos, A. V. (2017). IoT-based big data
storage systems in cloud computing: perspectives and challenges. IEEE
Internet of Things Journal, 4(1), 75-87.
(references)
[13] J. Clerk Maxwell, A Treatise on Electricity and Magnetism, 3rd ed., vol.
2. Oxford: Clarendon, 1892, pp.68–73.
[14] I. S. Jacobs and C. P. Bean, “Fine particles, thin films and exchange
anisotropy,” in Magnetism, vol. III, G. T. Rado and H. Suhl, Eds. New
York: Academic, 1963, pp. 271–350.
[15] K. Elissa, “Title of paper if known,” unpublished.
[16] R. Nicole, “Title of paper with only first word capitalized,” J. Name
Stand. Abbrev., in press.
[17] Y. Yorozu, M. Hirano, K. Oka, and Y. Tagawa, “Electron spectroscopy
studies on magneto-optical media and plastic substrate interface,” IEEE
Transl. J. Magn. Japan, vol. 2, pp. 740–741, August 1987 [Digests 9th
Annual Conf. Magnetics Japan, p. 301, 1982].
[18] Gubbi, J., Buyya, R., Marusic, S., & Palaniswami, M. (2013). Internet of
Things (IoT): A vision, architectural elements, and future
directions. Future generation computer systems, 29(7), 1645-1660.
[19] Barnaghi, P., Sheth, A., & Henson, C. (2013). From data to actionable
knowledge: Big data challenges in the web of things [Guest Editors'
Introduction]. IEEE Intelligent Systems, 28(6), 6-11.
[20] Zaslavsky, A., Perera, C., & Georgakopoulos, D. (2013). Sensing as a
service and big data. arXiv preprint arXiv:1301.0159.
[21] Xiaofeng, M., & Xiang, C. (2013). Big data management: concepts,
techniques and challenges [J]. Journal of computer research and
development, 1(98), 146-169.
[22] Xiaofeng, M., & Xiang, C. (2013). Big data management: concepts,
techniques and challenges [J]. Journal of computer research and
development, 1(98), 146-169.
[23] Chen, M., Mao, S., Zhang, Y., & Leung, V. C. (2014). Big data: related
technologies, challenges and future prospects (p. 35). Heidelberg:
Springer.
[24] Chen, F., Deng, P., Wan, J., Zhang, D., Vasilakos, A. V., & Rong, X.
(2015). Data mining for the internet of things: literature review and
challenges. International Journal of Distributed Sensor Networks, 11(8),
431047.
[25] Hashem, I. A. T., Yaqoob, I., Anuar, N. B., Mokhtar, S., Gani, A., &
Khan, S. U. (2015). The rise of “big data” on cloud computing: Review
and open research issues. Information Systems, 47, 98-115.
[26] Lee, I., & Lee, K. (2015). The Internet of Things (IoT): Applications,
investments, and challenges for enterprises. Business Horizons, 58(4),
431-440.
[27] Sun, Y., Song, H., Jara, A. J., & Bie, R. (2016). Internet of things and
big data analytics for smart and connected communities. IEEE
Access, 4, 766-773.
[28] Bessis, N., & Dobre, C. (Eds.). (2014). Big data and internet of things: a
roadmap for smart environments (Vol. 546). Springer International
Publishing.
[29] Baccarelli, E., Cordeschi, N., Mei, A., Panella, M., Shojafar, M., &
Stefa, J. (2016). Energy-efficient dynamic traffic offloading and
reconfiguration of networked data centers for big data stream mobile
computing: review, challenges, and a case study. IEEE Network, 30(2),
54-61.
[30] Fan, W., & Bifet, A. (2013). Mining big data: current status, and
forecast to the future. ACM sIGKDD Explorations Newsletter, 14(2), 1-
5.
[31] Sivarajah, U., Kamal, M. M., Irani, Z., & Weerakkody, V. (2017).
Critical analysis of Big Data challenges and analytical methods. Journal
of Business Research, 70, 263-286.
[32]
[33] Strohbach, M., Ziekow, H., Gazis, V., & Akiva, N. (2015). Towards a
big data analytics framework for IoT and smart city applications.
In Modeling and processing for next-generation big-data
technologies (pp. 257-282). Springer, Cham.
[34] Cai, H., Xu, B., Jiang, L., & Vasilakos, A. V. (2017). IoT-based big data
storage systems in cloud computing: perspectives and challenges. IEEE
Internet of Things Journal, 4(1), 75-87.
1 out of 9
Related Documents
![[object Object]](/_next/image/?url=%2F_next%2Fstatic%2Fmedia%2Flogo.6d15ce61.png&w=640&q=75)
Your All-in-One AI-Powered Toolkit for Academic Success.
+13062052269
info@desklib.com
Available 24*7 on WhatsApp / Email
Unlock your academic potential
© 2024 | Zucol Services PVT LTD | All rights reserved.