Big Data and Industrial Future: Industry 4.0 - Preparing for the Future
VerifiedAdded on 2023/06/09
|14
|4266
|64
AI Summary
This study examines the industrial importance of ‘Big Data’ and the responses from all kinds of businesses. It covers the latest trends in ‘Big Data’ and establishes a linkage with current practices of ‘Big Data', ‘IoT' and ‘Industry 4.0'.
Contribute Materials
Your contribution can guide someone’s learning journey. Share your
documents today.
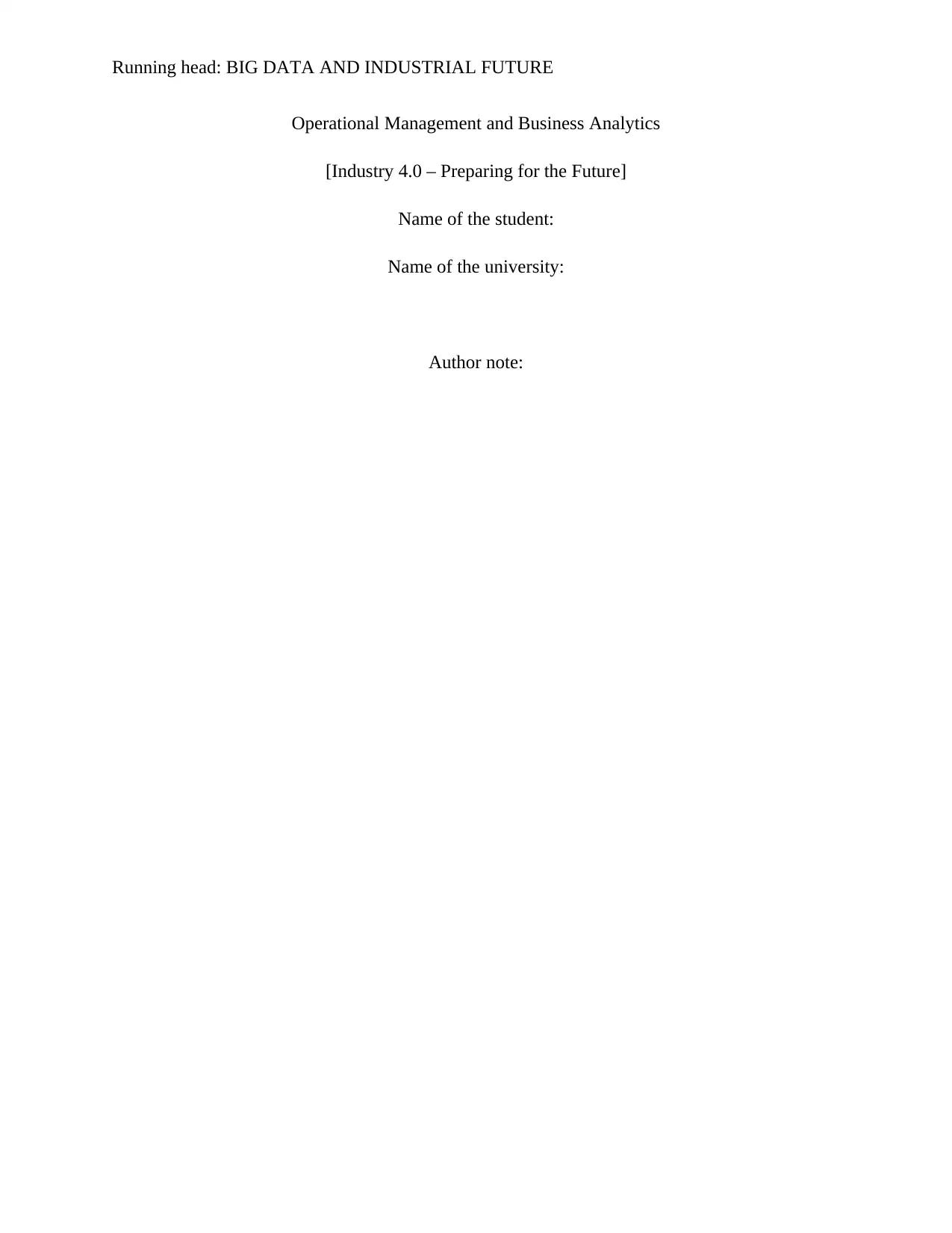
Running head: BIG DATA AND INDUSTRIAL FUTURE
Operational Management and Business Analytics
[Industry 4.0 – Preparing for the Future]
Name of the student:
Name of the university:
Author note:
Operational Management and Business Analytics
[Industry 4.0 – Preparing for the Future]
Name of the student:
Name of the university:
Author note:
Secure Best Marks with AI Grader
Need help grading? Try our AI Grader for instant feedback on your assignments.
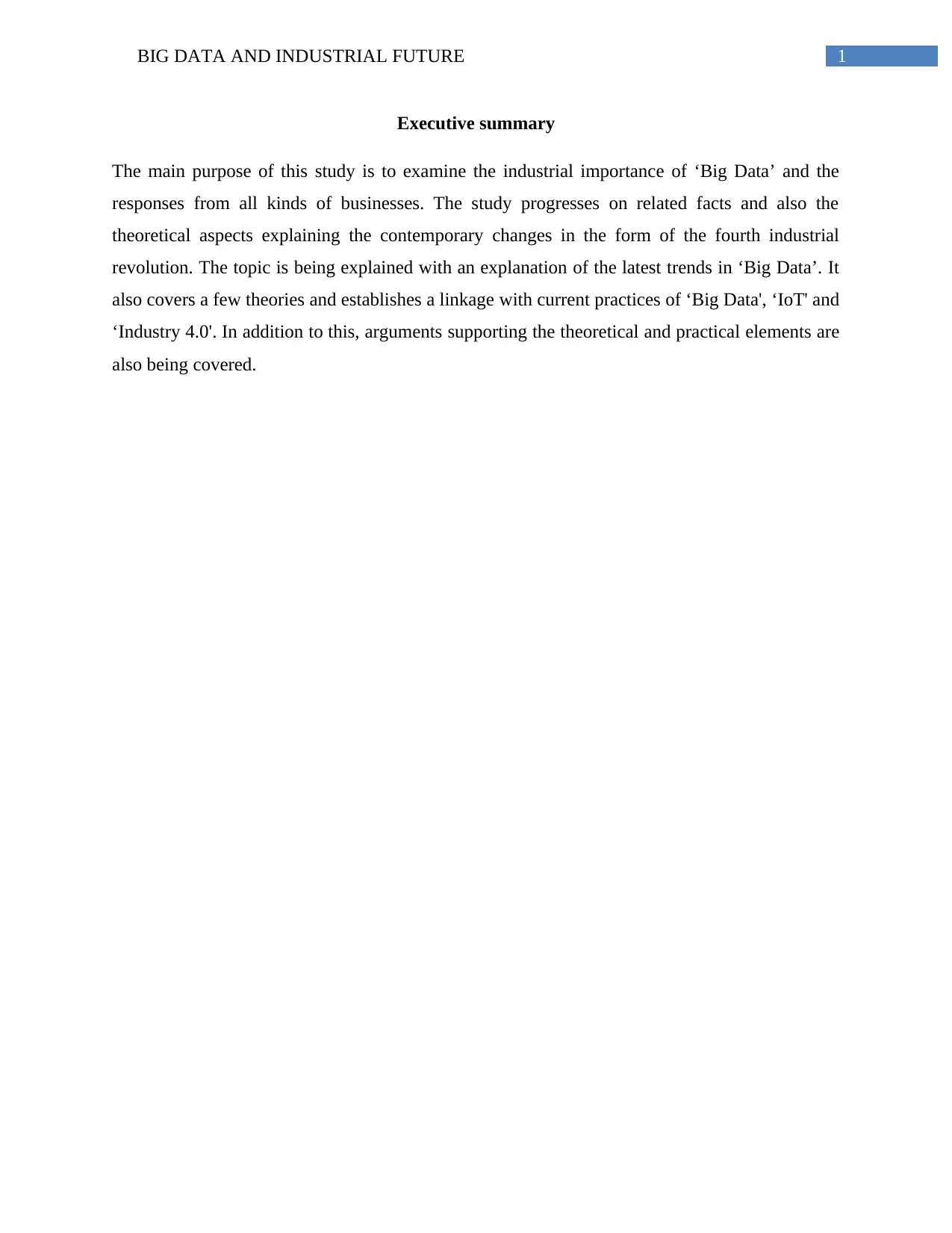
1BIG DATA AND INDUSTRIAL FUTURE
Executive summary
The main purpose of this study is to examine the industrial importance of ‘Big Data’ and the
responses from all kinds of businesses. The study progresses on related facts and also the
theoretical aspects explaining the contemporary changes in the form of the fourth industrial
revolution. The topic is being explained with an explanation of the latest trends in ‘Big Data’. It
also covers a few theories and establishes a linkage with current practices of ‘Big Data', ‘IoT' and
‘Industry 4.0'. In addition to this, arguments supporting the theoretical and practical elements are
also being covered.
Executive summary
The main purpose of this study is to examine the industrial importance of ‘Big Data’ and the
responses from all kinds of businesses. The study progresses on related facts and also the
theoretical aspects explaining the contemporary changes in the form of the fourth industrial
revolution. The topic is being explained with an explanation of the latest trends in ‘Big Data’. It
also covers a few theories and establishes a linkage with current practices of ‘Big Data', ‘IoT' and
‘Industry 4.0'. In addition to this, arguments supporting the theoretical and practical elements are
also being covered.
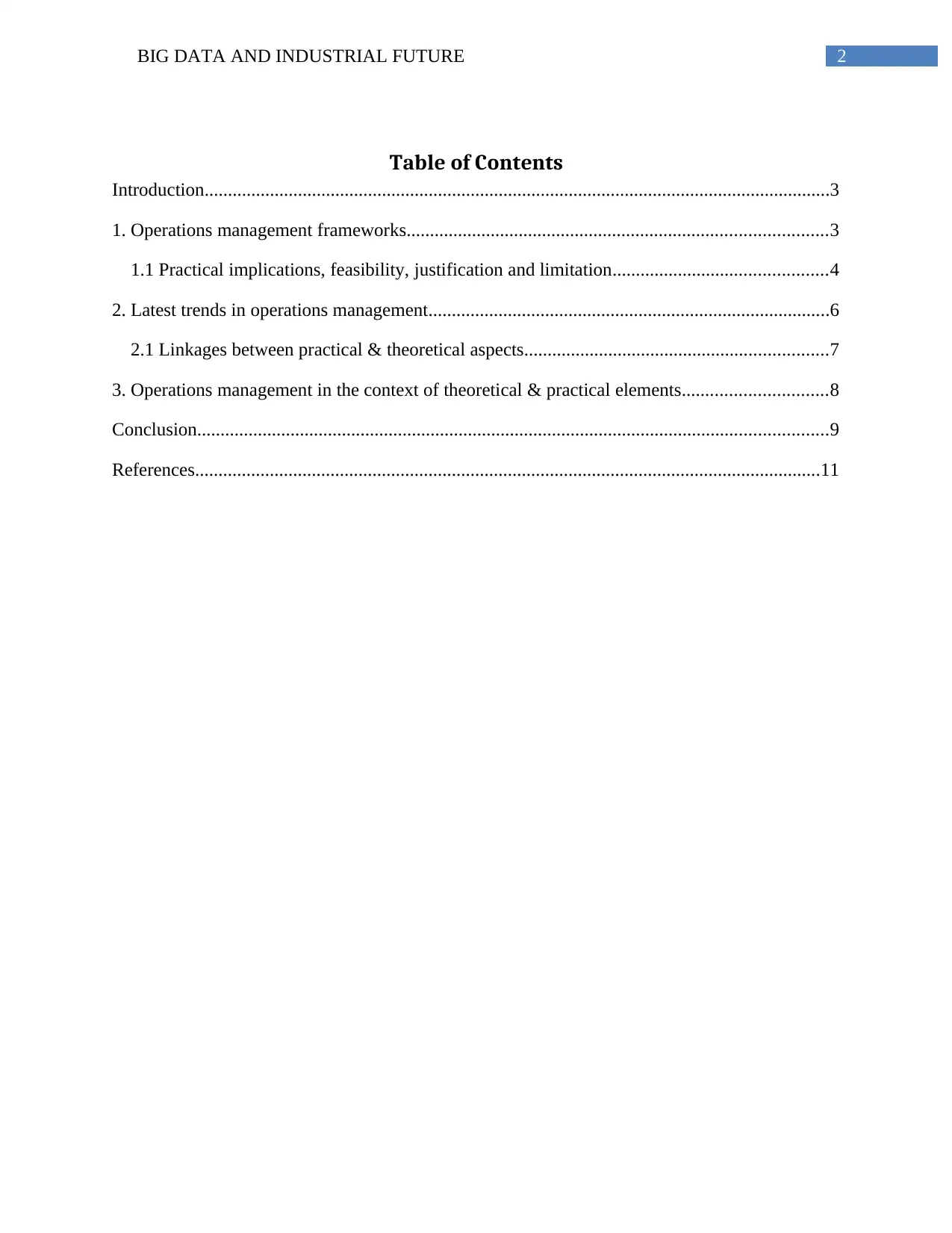
2BIG DATA AND INDUSTRIAL FUTURE
Table of Contents
Introduction......................................................................................................................................3
1. Operations management frameworks..........................................................................................3
1.1 Practical implications, feasibility, justification and limitation..............................................4
2. Latest trends in operations management......................................................................................6
2.1 Linkages between practical & theoretical aspects.................................................................7
3. Operations management in the context of theoretical & practical elements...............................8
Conclusion.......................................................................................................................................9
References......................................................................................................................................11
Table of Contents
Introduction......................................................................................................................................3
1. Operations management frameworks..........................................................................................3
1.1 Practical implications, feasibility, justification and limitation..............................................4
2. Latest trends in operations management......................................................................................6
2.1 Linkages between practical & theoretical aspects.................................................................7
3. Operations management in the context of theoretical & practical elements...............................8
Conclusion.......................................................................................................................................9
References......................................................................................................................................11
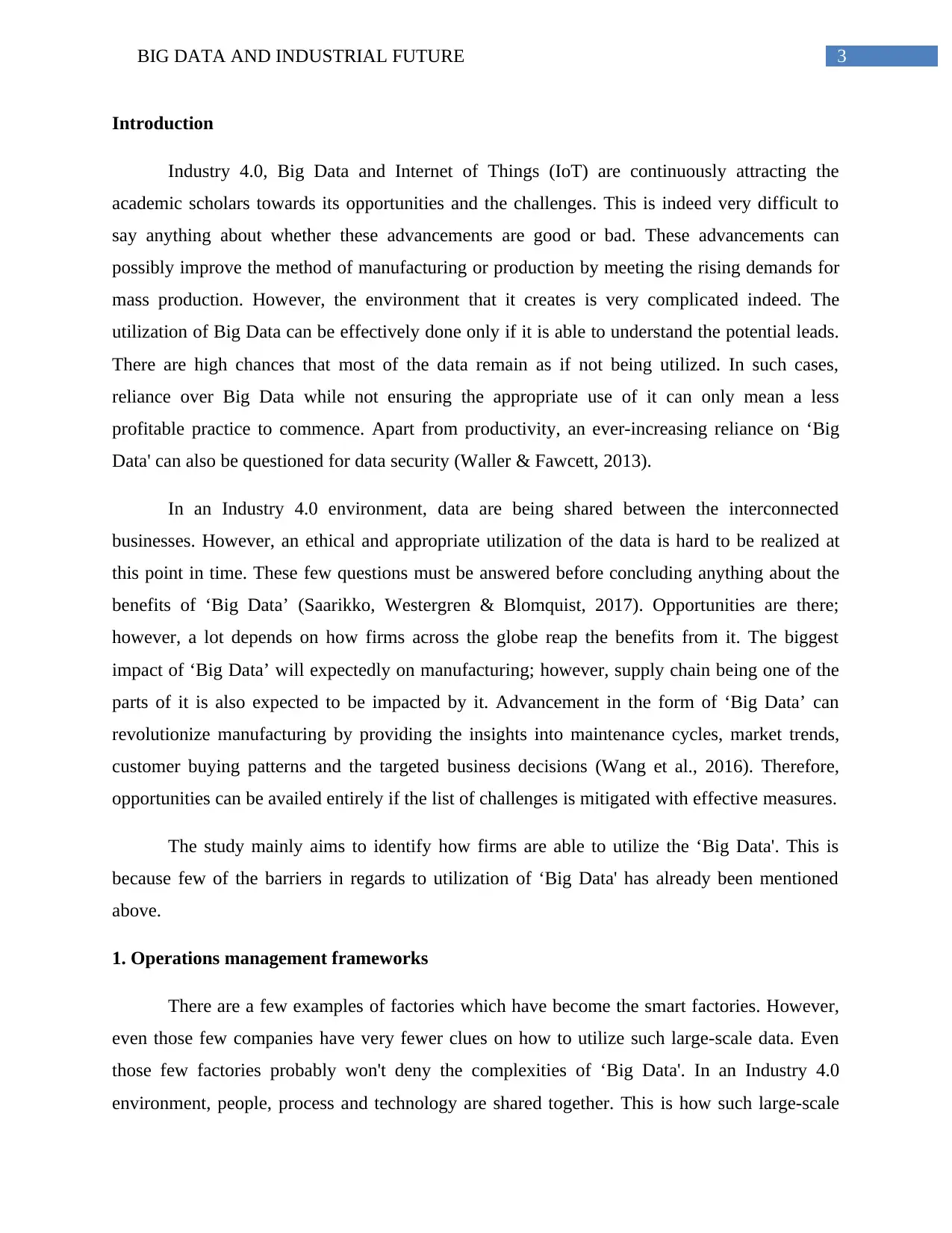
3BIG DATA AND INDUSTRIAL FUTURE
Introduction
Industry 4.0, Big Data and Internet of Things (IoT) are continuously attracting the
academic scholars towards its opportunities and the challenges. This is indeed very difficult to
say anything about whether these advancements are good or bad. These advancements can
possibly improve the method of manufacturing or production by meeting the rising demands for
mass production. However, the environment that it creates is very complicated indeed. The
utilization of Big Data can be effectively done only if it is able to understand the potential leads.
There are high chances that most of the data remain as if not being utilized. In such cases,
reliance over Big Data while not ensuring the appropriate use of it can only mean a less
profitable practice to commence. Apart from productivity, an ever-increasing reliance on ‘Big
Data' can also be questioned for data security (Waller & Fawcett, 2013).
In an Industry 4.0 environment, data are being shared between the interconnected
businesses. However, an ethical and appropriate utilization of the data is hard to be realized at
this point in time. These few questions must be answered before concluding anything about the
benefits of ‘Big Data’ (Saarikko, Westergren & Blomquist, 2017). Opportunities are there;
however, a lot depends on how firms across the globe reap the benefits from it. The biggest
impact of ‘Big Data’ will expectedly on manufacturing; however, supply chain being one of the
parts of it is also expected to be impacted by it. Advancement in the form of ‘Big Data’ can
revolutionize manufacturing by providing the insights into maintenance cycles, market trends,
customer buying patterns and the targeted business decisions (Wang et al., 2016). Therefore,
opportunities can be availed entirely if the list of challenges is mitigated with effective measures.
The study mainly aims to identify how firms are able to utilize the ‘Big Data'. This is
because few of the barriers in regards to utilization of ‘Big Data' has already been mentioned
above.
1. Operations management frameworks
There are a few examples of factories which have become the smart factories. However,
even those few companies have very fewer clues on how to utilize such large-scale data. Even
those few factories probably won't deny the complexities of ‘Big Data'. In an Industry 4.0
environment, people, process and technology are shared together. This is how such large-scale
Introduction
Industry 4.0, Big Data and Internet of Things (IoT) are continuously attracting the
academic scholars towards its opportunities and the challenges. This is indeed very difficult to
say anything about whether these advancements are good or bad. These advancements can
possibly improve the method of manufacturing or production by meeting the rising demands for
mass production. However, the environment that it creates is very complicated indeed. The
utilization of Big Data can be effectively done only if it is able to understand the potential leads.
There are high chances that most of the data remain as if not being utilized. In such cases,
reliance over Big Data while not ensuring the appropriate use of it can only mean a less
profitable practice to commence. Apart from productivity, an ever-increasing reliance on ‘Big
Data' can also be questioned for data security (Waller & Fawcett, 2013).
In an Industry 4.0 environment, data are being shared between the interconnected
businesses. However, an ethical and appropriate utilization of the data is hard to be realized at
this point in time. These few questions must be answered before concluding anything about the
benefits of ‘Big Data’ (Saarikko, Westergren & Blomquist, 2017). Opportunities are there;
however, a lot depends on how firms across the globe reap the benefits from it. The biggest
impact of ‘Big Data’ will expectedly on manufacturing; however, supply chain being one of the
parts of it is also expected to be impacted by it. Advancement in the form of ‘Big Data’ can
revolutionize manufacturing by providing the insights into maintenance cycles, market trends,
customer buying patterns and the targeted business decisions (Wang et al., 2016). Therefore,
opportunities can be availed entirely if the list of challenges is mitigated with effective measures.
The study mainly aims to identify how firms are able to utilize the ‘Big Data'. This is
because few of the barriers in regards to utilization of ‘Big Data' has already been mentioned
above.
1. Operations management frameworks
There are a few examples of factories which have become the smart factories. However,
even those few companies have very fewer clues on how to utilize such large-scale data. Even
those few factories probably won't deny the complexities of ‘Big Data'. In an Industry 4.0
environment, people, process and technology are shared together. This is how such large-scale
Secure Best Marks with AI Grader
Need help grading? Try our AI Grader for instant feedback on your assignments.
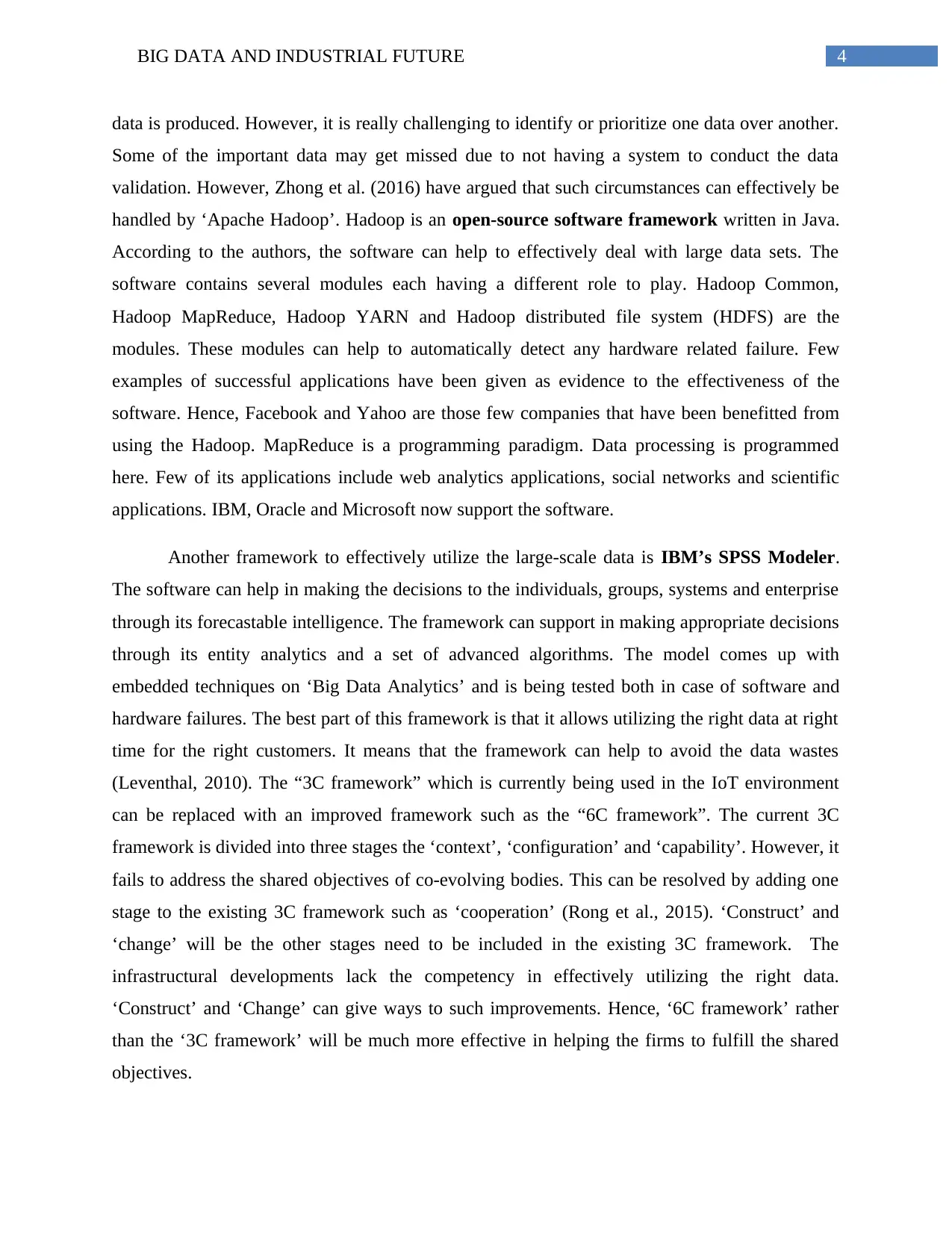
4BIG DATA AND INDUSTRIAL FUTURE
data is produced. However, it is really challenging to identify or prioritize one data over another.
Some of the important data may get missed due to not having a system to conduct the data
validation. However, Zhong et al. (2016) have argued that such circumstances can effectively be
handled by ‘Apache Hadoop’. Hadoop is an open-source software framework written in Java.
According to the authors, the software can help to effectively deal with large data sets. The
software contains several modules each having a different role to play. Hadoop Common,
Hadoop MapReduce, Hadoop YARN and Hadoop distributed file system (HDFS) are the
modules. These modules can help to automatically detect any hardware related failure. Few
examples of successful applications have been given as evidence to the effectiveness of the
software. Hence, Facebook and Yahoo are those few companies that have been benefitted from
using the Hadoop. MapReduce is a programming paradigm. Data processing is programmed
here. Few of its applications include web analytics applications, social networks and scientific
applications. IBM, Oracle and Microsoft now support the software.
Another framework to effectively utilize the large-scale data is IBM’s SPSS Modeler.
The software can help in making the decisions to the individuals, groups, systems and enterprise
through its forecastable intelligence. The framework can support in making appropriate decisions
through its entity analytics and a set of advanced algorithms. The model comes up with
embedded techniques on ‘Big Data Analytics’ and is being tested both in case of software and
hardware failures. The best part of this framework is that it allows utilizing the right data at right
time for the right customers. It means that the framework can help to avoid the data wastes
(Leventhal, 2010). The “3C framework” which is currently being used in the IoT environment
can be replaced with an improved framework such as the “6C framework”. The current 3C
framework is divided into three stages the ‘context’, ‘configuration’ and ‘capability’. However, it
fails to address the shared objectives of co-evolving bodies. This can be resolved by adding one
stage to the existing 3C framework such as ‘cooperation’ (Rong et al., 2015). ‘Construct’ and
‘change’ will be the other stages need to be included in the existing 3C framework. The
infrastructural developments lack the competency in effectively utilizing the right data.
‘Construct’ and ‘Change’ can give ways to such improvements. Hence, ‘6C framework’ rather
than the ‘3C framework’ will be much more effective in helping the firms to fulfill the shared
objectives.
data is produced. However, it is really challenging to identify or prioritize one data over another.
Some of the important data may get missed due to not having a system to conduct the data
validation. However, Zhong et al. (2016) have argued that such circumstances can effectively be
handled by ‘Apache Hadoop’. Hadoop is an open-source software framework written in Java.
According to the authors, the software can help to effectively deal with large data sets. The
software contains several modules each having a different role to play. Hadoop Common,
Hadoop MapReduce, Hadoop YARN and Hadoop distributed file system (HDFS) are the
modules. These modules can help to automatically detect any hardware related failure. Few
examples of successful applications have been given as evidence to the effectiveness of the
software. Hence, Facebook and Yahoo are those few companies that have been benefitted from
using the Hadoop. MapReduce is a programming paradigm. Data processing is programmed
here. Few of its applications include web analytics applications, social networks and scientific
applications. IBM, Oracle and Microsoft now support the software.
Another framework to effectively utilize the large-scale data is IBM’s SPSS Modeler.
The software can help in making the decisions to the individuals, groups, systems and enterprise
through its forecastable intelligence. The framework can support in making appropriate decisions
through its entity analytics and a set of advanced algorithms. The model comes up with
embedded techniques on ‘Big Data Analytics’ and is being tested both in case of software and
hardware failures. The best part of this framework is that it allows utilizing the right data at right
time for the right customers. It means that the framework can help to avoid the data wastes
(Leventhal, 2010). The “3C framework” which is currently being used in the IoT environment
can be replaced with an improved framework such as the “6C framework”. The current 3C
framework is divided into three stages the ‘context’, ‘configuration’ and ‘capability’. However, it
fails to address the shared objectives of co-evolving bodies. This can be resolved by adding one
stage to the existing 3C framework such as ‘cooperation’ (Rong et al., 2015). ‘Construct’ and
‘change’ will be the other stages need to be included in the existing 3C framework. The
infrastructural developments lack the competency in effectively utilizing the right data.
‘Construct’ and ‘Change’ can give ways to such improvements. Hence, ‘6C framework’ rather
than the ‘3C framework’ will be much more effective in helping the firms to fulfill the shared
objectives.
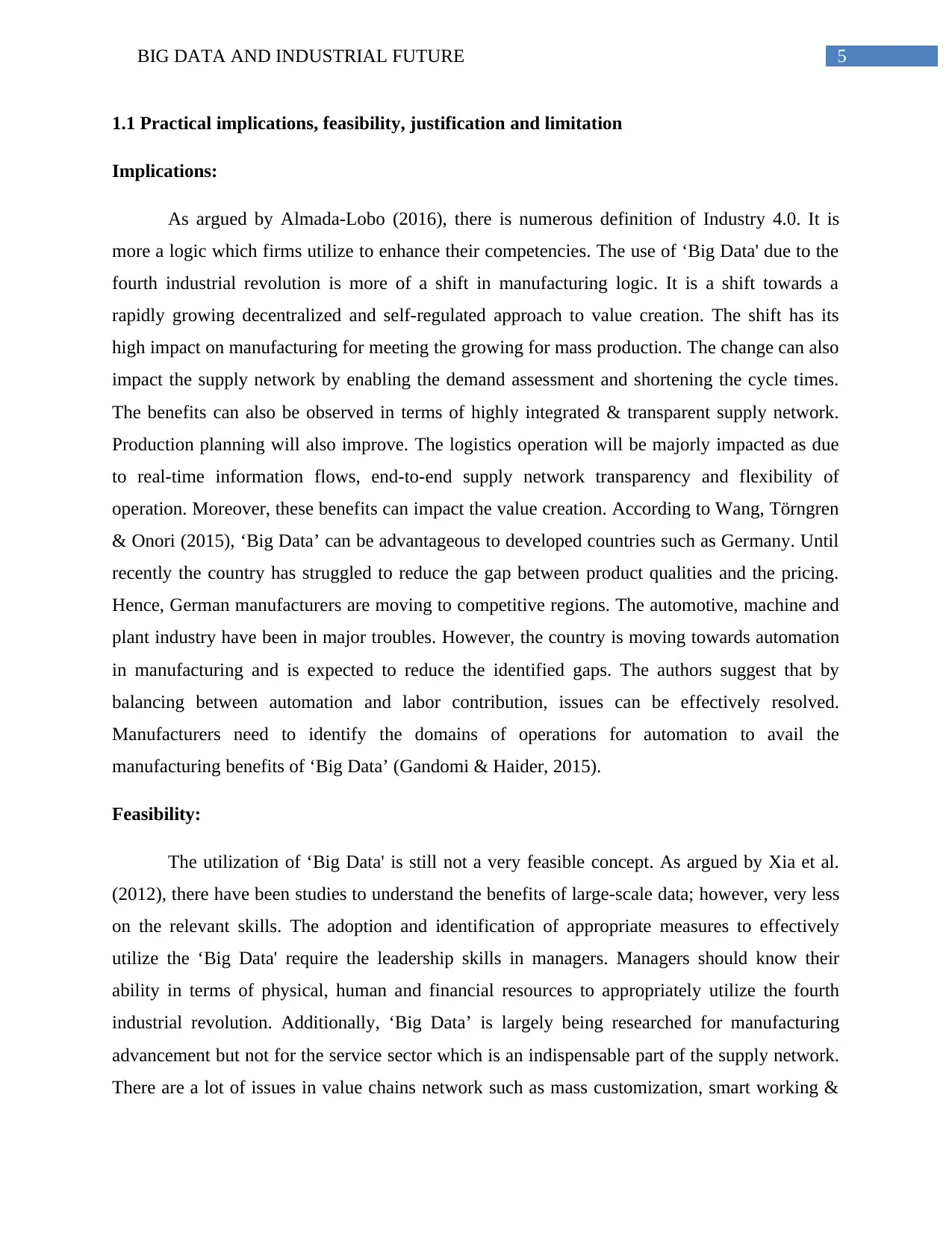
5BIG DATA AND INDUSTRIAL FUTURE
1.1 Practical implications, feasibility, justification and limitation
Implications:
As argued by Almada-Lobo (2016), there is numerous definition of Industry 4.0. It is
more a logic which firms utilize to enhance their competencies. The use of ‘Big Data' due to the
fourth industrial revolution is more of a shift in manufacturing logic. It is a shift towards a
rapidly growing decentralized and self-regulated approach to value creation. The shift has its
high impact on manufacturing for meeting the growing for mass production. The change can also
impact the supply network by enabling the demand assessment and shortening the cycle times.
The benefits can also be observed in terms of highly integrated & transparent supply network.
Production planning will also improve. The logistics operation will be majorly impacted as due
to real-time information flows, end-to-end supply network transparency and flexibility of
operation. Moreover, these benefits can impact the value creation. According to Wang, Törngren
& Onori (2015), ‘Big Data’ can be advantageous to developed countries such as Germany. Until
recently the country has struggled to reduce the gap between product qualities and the pricing.
Hence, German manufacturers are moving to competitive regions. The automotive, machine and
plant industry have been in major troubles. However, the country is moving towards automation
in manufacturing and is expected to reduce the identified gaps. The authors suggest that by
balancing between automation and labor contribution, issues can be effectively resolved.
Manufacturers need to identify the domains of operations for automation to avail the
manufacturing benefits of ‘Big Data’ (Gandomi & Haider, 2015).
Feasibility:
The utilization of ‘Big Data' is still not a very feasible concept. As argued by Xia et al.
(2012), there have been studies to understand the benefits of large-scale data; however, very less
on the relevant skills. The adoption and identification of appropriate measures to effectively
utilize the ‘Big Data' require the leadership skills in managers. Managers should know their
ability in terms of physical, human and financial resources to appropriately utilize the fourth
industrial revolution. Additionally, ‘Big Data’ is largely being researched for manufacturing
advancement but not for the service sector which is an indispensable part of the supply network.
There are a lot of issues in value chains network such as mass customization, smart working &
1.1 Practical implications, feasibility, justification and limitation
Implications:
As argued by Almada-Lobo (2016), there is numerous definition of Industry 4.0. It is
more a logic which firms utilize to enhance their competencies. The use of ‘Big Data' due to the
fourth industrial revolution is more of a shift in manufacturing logic. It is a shift towards a
rapidly growing decentralized and self-regulated approach to value creation. The shift has its
high impact on manufacturing for meeting the growing for mass production. The change can also
impact the supply network by enabling the demand assessment and shortening the cycle times.
The benefits can also be observed in terms of highly integrated & transparent supply network.
Production planning will also improve. The logistics operation will be majorly impacted as due
to real-time information flows, end-to-end supply network transparency and flexibility of
operation. Moreover, these benefits can impact the value creation. According to Wang, Törngren
& Onori (2015), ‘Big Data’ can be advantageous to developed countries such as Germany. Until
recently the country has struggled to reduce the gap between product qualities and the pricing.
Hence, German manufacturers are moving to competitive regions. The automotive, machine and
plant industry have been in major troubles. However, the country is moving towards automation
in manufacturing and is expected to reduce the identified gaps. The authors suggest that by
balancing between automation and labor contribution, issues can be effectively resolved.
Manufacturers need to identify the domains of operations for automation to avail the
manufacturing benefits of ‘Big Data’ (Gandomi & Haider, 2015).
Feasibility:
The utilization of ‘Big Data' is still not a very feasible concept. As argued by Xia et al.
(2012), there have been studies to understand the benefits of large-scale data; however, very less
on the relevant skills. The adoption and identification of appropriate measures to effectively
utilize the ‘Big Data' require the leadership skills in managers. Managers should know their
ability in terms of physical, human and financial resources to appropriately utilize the fourth
industrial revolution. Additionally, ‘Big Data’ is largely being researched for manufacturing
advancement but not for the service sector which is an indispensable part of the supply network.
There are a lot of issues in value chains network such as mass customization, smart working &
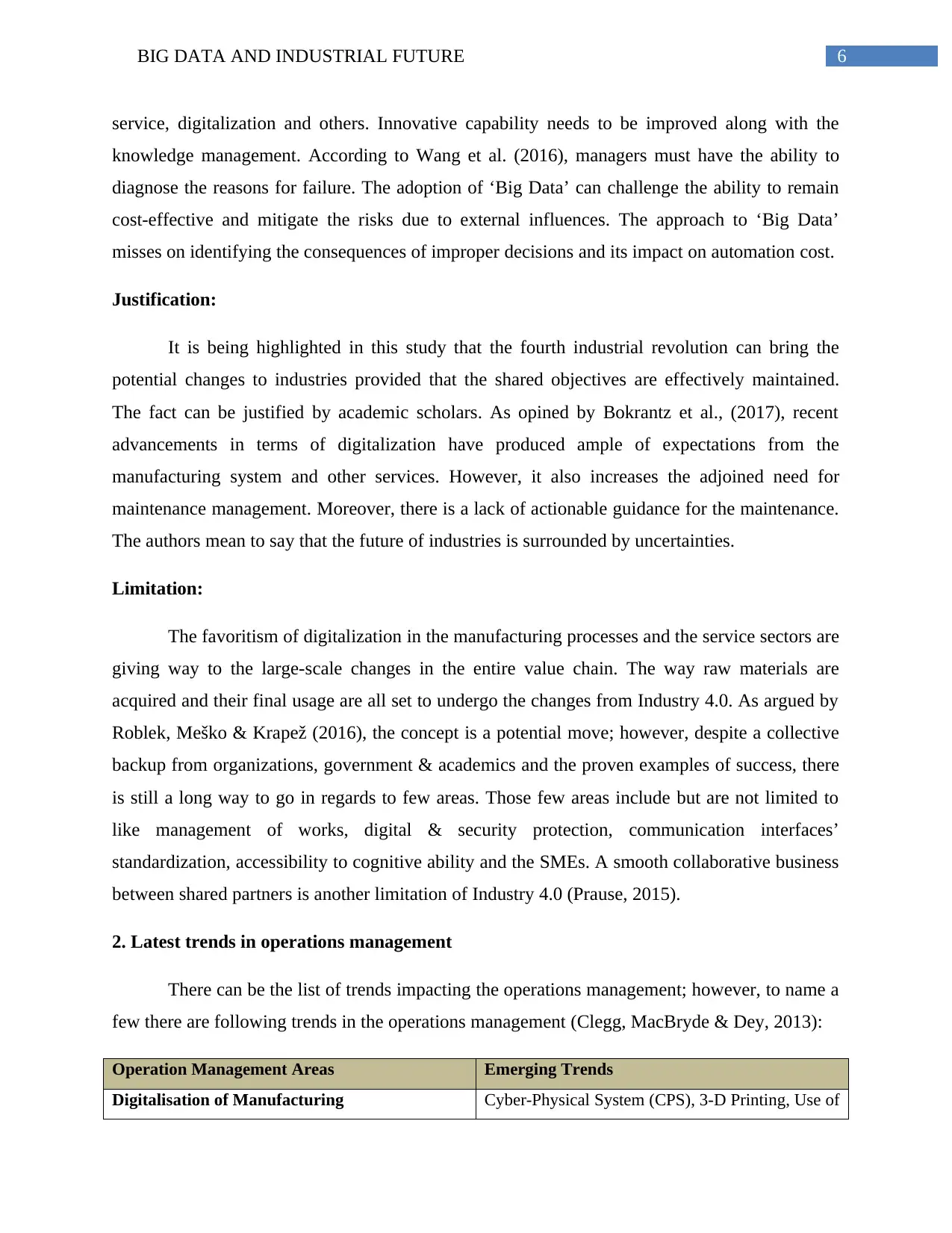
6BIG DATA AND INDUSTRIAL FUTURE
service, digitalization and others. Innovative capability needs to be improved along with the
knowledge management. According to Wang et al. (2016), managers must have the ability to
diagnose the reasons for failure. The adoption of ‘Big Data’ can challenge the ability to remain
cost-effective and mitigate the risks due to external influences. The approach to ‘Big Data’
misses on identifying the consequences of improper decisions and its impact on automation cost.
Justification:
It is being highlighted in this study that the fourth industrial revolution can bring the
potential changes to industries provided that the shared objectives are effectively maintained.
The fact can be justified by academic scholars. As opined by Bokrantz et al., (2017), recent
advancements in terms of digitalization have produced ample of expectations from the
manufacturing system and other services. However, it also increases the adjoined need for
maintenance management. Moreover, there is a lack of actionable guidance for the maintenance.
The authors mean to say that the future of industries is surrounded by uncertainties.
Limitation:
The favoritism of digitalization in the manufacturing processes and the service sectors are
giving way to the large-scale changes in the entire value chain. The way raw materials are
acquired and their final usage are all set to undergo the changes from Industry 4.0. As argued by
Roblek, Meško & Krapež (2016), the concept is a potential move; however, despite a collective
backup from organizations, government & academics and the proven examples of success, there
is still a long way to go in regards to few areas. Those few areas include but are not limited to
like management of works, digital & security protection, communication interfaces’
standardization, accessibility to cognitive ability and the SMEs. A smooth collaborative business
between shared partners is another limitation of Industry 4.0 (Prause, 2015).
2. Latest trends in operations management
There can be the list of trends impacting the operations management; however, to name a
few there are following trends in the operations management (Clegg, MacBryde & Dey, 2013):
Operation Management Areas Emerging Trends
Digitalisation of Manufacturing Cyber-Physical System (CPS), 3-D Printing, Use of
service, digitalization and others. Innovative capability needs to be improved along with the
knowledge management. According to Wang et al. (2016), managers must have the ability to
diagnose the reasons for failure. The adoption of ‘Big Data’ can challenge the ability to remain
cost-effective and mitigate the risks due to external influences. The approach to ‘Big Data’
misses on identifying the consequences of improper decisions and its impact on automation cost.
Justification:
It is being highlighted in this study that the fourth industrial revolution can bring the
potential changes to industries provided that the shared objectives are effectively maintained.
The fact can be justified by academic scholars. As opined by Bokrantz et al., (2017), recent
advancements in terms of digitalization have produced ample of expectations from the
manufacturing system and other services. However, it also increases the adjoined need for
maintenance management. Moreover, there is a lack of actionable guidance for the maintenance.
The authors mean to say that the future of industries is surrounded by uncertainties.
Limitation:
The favoritism of digitalization in the manufacturing processes and the service sectors are
giving way to the large-scale changes in the entire value chain. The way raw materials are
acquired and their final usage are all set to undergo the changes from Industry 4.0. As argued by
Roblek, Meško & Krapež (2016), the concept is a potential move; however, despite a collective
backup from organizations, government & academics and the proven examples of success, there
is still a long way to go in regards to few areas. Those few areas include but are not limited to
like management of works, digital & security protection, communication interfaces’
standardization, accessibility to cognitive ability and the SMEs. A smooth collaborative business
between shared partners is another limitation of Industry 4.0 (Prause, 2015).
2. Latest trends in operations management
There can be the list of trends impacting the operations management; however, to name a
few there are following trends in the operations management (Clegg, MacBryde & Dey, 2013):
Operation Management Areas Emerging Trends
Digitalisation of Manufacturing Cyber-Physical System (CPS), 3-D Printing, Use of
Paraphrase This Document
Need a fresh take? Get an instant paraphrase of this document with our AI Paraphraser
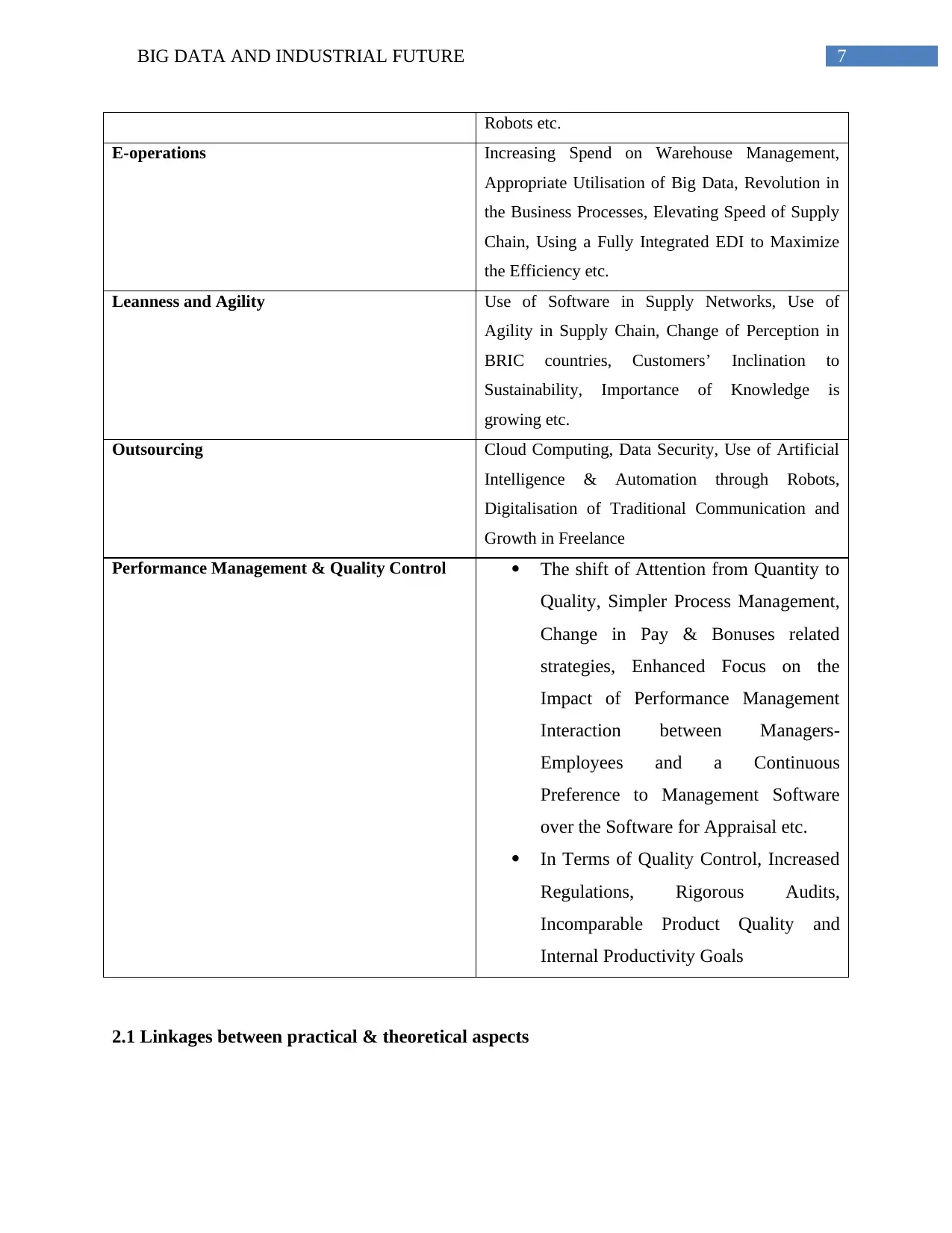
7BIG DATA AND INDUSTRIAL FUTURE
Robots etc.
E-operations Increasing Spend on Warehouse Management,
Appropriate Utilisation of Big Data, Revolution in
the Business Processes, Elevating Speed of Supply
Chain, Using a Fully Integrated EDI to Maximize
the Efficiency etc.
Leanness and Agility Use of Software in Supply Networks, Use of
Agility in Supply Chain, Change of Perception in
BRIC countries, Customers’ Inclination to
Sustainability, Importance of Knowledge is
growing etc.
Outsourcing Cloud Computing, Data Security, Use of Artificial
Intelligence & Automation through Robots,
Digitalisation of Traditional Communication and
Growth in Freelance
Performance Management & Quality Control The shift of Attention from Quantity to
Quality, Simpler Process Management,
Change in Pay & Bonuses related
strategies, Enhanced Focus on the
Impact of Performance Management
Interaction between Managers-
Employees and a Continuous
Preference to Management Software
over the Software for Appraisal etc.
In Terms of Quality Control, Increased
Regulations, Rigorous Audits,
Incomparable Product Quality and
Internal Productivity Goals
2.1 Linkages between practical & theoretical aspects
Robots etc.
E-operations Increasing Spend on Warehouse Management,
Appropriate Utilisation of Big Data, Revolution in
the Business Processes, Elevating Speed of Supply
Chain, Using a Fully Integrated EDI to Maximize
the Efficiency etc.
Leanness and Agility Use of Software in Supply Networks, Use of
Agility in Supply Chain, Change of Perception in
BRIC countries, Customers’ Inclination to
Sustainability, Importance of Knowledge is
growing etc.
Outsourcing Cloud Computing, Data Security, Use of Artificial
Intelligence & Automation through Robots,
Digitalisation of Traditional Communication and
Growth in Freelance
Performance Management & Quality Control The shift of Attention from Quantity to
Quality, Simpler Process Management,
Change in Pay & Bonuses related
strategies, Enhanced Focus on the
Impact of Performance Management
Interaction between Managers-
Employees and a Continuous
Preference to Management Software
over the Software for Appraisal etc.
In Terms of Quality Control, Increased
Regulations, Rigorous Audits,
Incomparable Product Quality and
Internal Productivity Goals
2.1 Linkages between practical & theoretical aspects
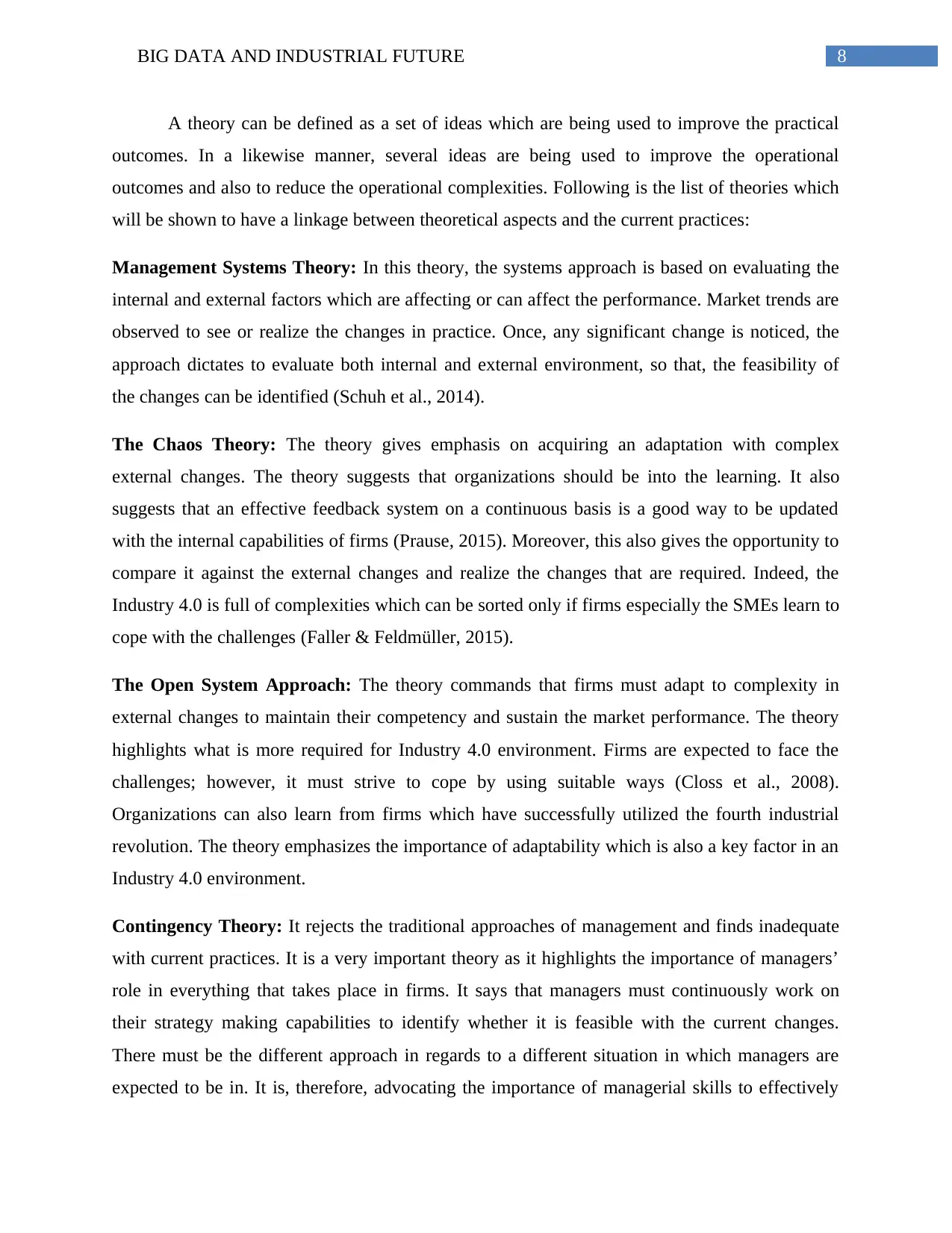
8BIG DATA AND INDUSTRIAL FUTURE
A theory can be defined as a set of ideas which are being used to improve the practical
outcomes. In a likewise manner, several ideas are being used to improve the operational
outcomes and also to reduce the operational complexities. Following is the list of theories which
will be shown to have a linkage between theoretical aspects and the current practices:
Management Systems Theory: In this theory, the systems approach is based on evaluating the
internal and external factors which are affecting or can affect the performance. Market trends are
observed to see or realize the changes in practice. Once, any significant change is noticed, the
approach dictates to evaluate both internal and external environment, so that, the feasibility of
the changes can be identified (Schuh et al., 2014).
The Chaos Theory: The theory gives emphasis on acquiring an adaptation with complex
external changes. The theory suggests that organizations should be into the learning. It also
suggests that an effective feedback system on a continuous basis is a good way to be updated
with the internal capabilities of firms (Prause, 2015). Moreover, this also gives the opportunity to
compare it against the external changes and realize the changes that are required. Indeed, the
Industry 4.0 is full of complexities which can be sorted only if firms especially the SMEs learn to
cope with the challenges (Faller & Feldmüller, 2015).
The Open System Approach: The theory commands that firms must adapt to complexity in
external changes to maintain their competency and sustain the market performance. The theory
highlights what is more required for Industry 4.0 environment. Firms are expected to face the
challenges; however, it must strive to cope by using suitable ways (Closs et al., 2008).
Organizations can also learn from firms which have successfully utilized the fourth industrial
revolution. The theory emphasizes the importance of adaptability which is also a key factor in an
Industry 4.0 environment.
Contingency Theory: It rejects the traditional approaches of management and finds inadequate
with current practices. It is a very important theory as it highlights the importance of managers’
role in everything that takes place in firms. It says that managers must continuously work on
their strategy making capabilities to identify whether it is feasible with the current changes.
There must be the different approach in regards to a different situation in which managers are
expected to be in. It is, therefore, advocating the importance of managerial skills to effectively
A theory can be defined as a set of ideas which are being used to improve the practical
outcomes. In a likewise manner, several ideas are being used to improve the operational
outcomes and also to reduce the operational complexities. Following is the list of theories which
will be shown to have a linkage between theoretical aspects and the current practices:
Management Systems Theory: In this theory, the systems approach is based on evaluating the
internal and external factors which are affecting or can affect the performance. Market trends are
observed to see or realize the changes in practice. Once, any significant change is noticed, the
approach dictates to evaluate both internal and external environment, so that, the feasibility of
the changes can be identified (Schuh et al., 2014).
The Chaos Theory: The theory gives emphasis on acquiring an adaptation with complex
external changes. The theory suggests that organizations should be into the learning. It also
suggests that an effective feedback system on a continuous basis is a good way to be updated
with the internal capabilities of firms (Prause, 2015). Moreover, this also gives the opportunity to
compare it against the external changes and realize the changes that are required. Indeed, the
Industry 4.0 is full of complexities which can be sorted only if firms especially the SMEs learn to
cope with the challenges (Faller & Feldmüller, 2015).
The Open System Approach: The theory commands that firms must adapt to complexity in
external changes to maintain their competency and sustain the market performance. The theory
highlights what is more required for Industry 4.0 environment. Firms are expected to face the
challenges; however, it must strive to cope by using suitable ways (Closs et al., 2008).
Organizations can also learn from firms which have successfully utilized the fourth industrial
revolution. The theory emphasizes the importance of adaptability which is also a key factor in an
Industry 4.0 environment.
Contingency Theory: It rejects the traditional approaches of management and finds inadequate
with current practices. It is a very important theory as it highlights the importance of managers’
role in everything that takes place in firms. It says that managers must continuously work on
their strategy making capabilities to identify whether it is feasible with the current changes.
There must be the different approach in regards to a different situation in which managers are
expected to be in. It is, therefore, advocating the importance of managerial skills to effectively
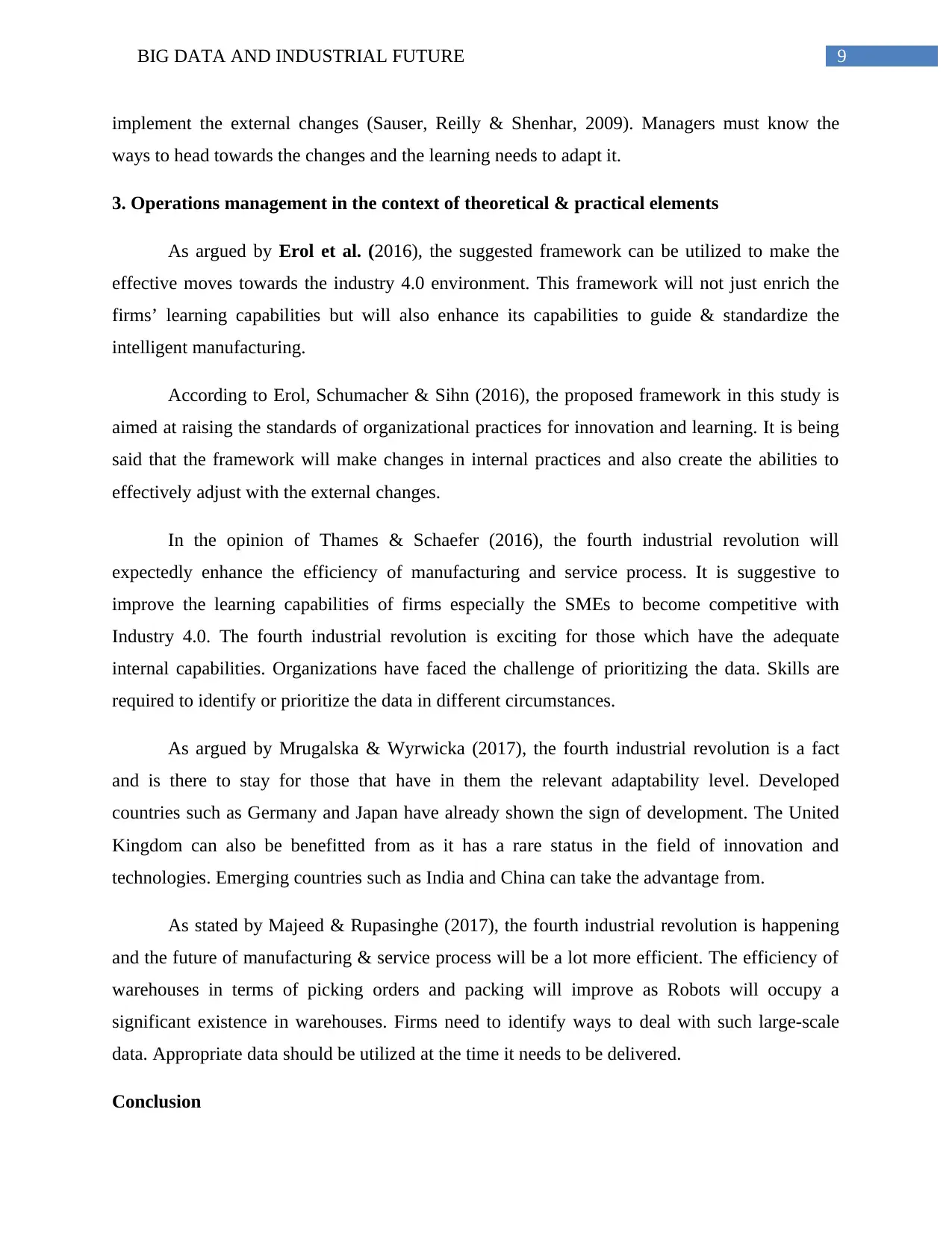
9BIG DATA AND INDUSTRIAL FUTURE
implement the external changes (Sauser, Reilly & Shenhar, 2009). Managers must know the
ways to head towards the changes and the learning needs to adapt it.
3. Operations management in the context of theoretical & practical elements
As argued by Erol et al. (2016), the suggested framework can be utilized to make the
effective moves towards the industry 4.0 environment. This framework will not just enrich the
firms’ learning capabilities but will also enhance its capabilities to guide & standardize the
intelligent manufacturing.
According to Erol, Schumacher & Sihn (2016), the proposed framework in this study is
aimed at raising the standards of organizational practices for innovation and learning. It is being
said that the framework will make changes in internal practices and also create the abilities to
effectively adjust with the external changes.
In the opinion of Thames & Schaefer (2016), the fourth industrial revolution will
expectedly enhance the efficiency of manufacturing and service process. It is suggestive to
improve the learning capabilities of firms especially the SMEs to become competitive with
Industry 4.0. The fourth industrial revolution is exciting for those which have the adequate
internal capabilities. Organizations have faced the challenge of prioritizing the data. Skills are
required to identify or prioritize the data in different circumstances.
As argued by Mrugalska & Wyrwicka (2017), the fourth industrial revolution is a fact
and is there to stay for those that have in them the relevant adaptability level. Developed
countries such as Germany and Japan have already shown the sign of development. The United
Kingdom can also be benefitted from as it has a rare status in the field of innovation and
technologies. Emerging countries such as India and China can take the advantage from.
As stated by Majeed & Rupasinghe (2017), the fourth industrial revolution is happening
and the future of manufacturing & service process will be a lot more efficient. The efficiency of
warehouses in terms of picking orders and packing will improve as Robots will occupy a
significant existence in warehouses. Firms need to identify ways to deal with such large-scale
data. Appropriate data should be utilized at the time it needs to be delivered.
Conclusion
implement the external changes (Sauser, Reilly & Shenhar, 2009). Managers must know the
ways to head towards the changes and the learning needs to adapt it.
3. Operations management in the context of theoretical & practical elements
As argued by Erol et al. (2016), the suggested framework can be utilized to make the
effective moves towards the industry 4.0 environment. This framework will not just enrich the
firms’ learning capabilities but will also enhance its capabilities to guide & standardize the
intelligent manufacturing.
According to Erol, Schumacher & Sihn (2016), the proposed framework in this study is
aimed at raising the standards of organizational practices for innovation and learning. It is being
said that the framework will make changes in internal practices and also create the abilities to
effectively adjust with the external changes.
In the opinion of Thames & Schaefer (2016), the fourth industrial revolution will
expectedly enhance the efficiency of manufacturing and service process. It is suggestive to
improve the learning capabilities of firms especially the SMEs to become competitive with
Industry 4.0. The fourth industrial revolution is exciting for those which have the adequate
internal capabilities. Organizations have faced the challenge of prioritizing the data. Skills are
required to identify or prioritize the data in different circumstances.
As argued by Mrugalska & Wyrwicka (2017), the fourth industrial revolution is a fact
and is there to stay for those that have in them the relevant adaptability level. Developed
countries such as Germany and Japan have already shown the sign of development. The United
Kingdom can also be benefitted from as it has a rare status in the field of innovation and
technologies. Emerging countries such as India and China can take the advantage from.
As stated by Majeed & Rupasinghe (2017), the fourth industrial revolution is happening
and the future of manufacturing & service process will be a lot more efficient. The efficiency of
warehouses in terms of picking orders and packing will improve as Robots will occupy a
significant existence in warehouses. Firms need to identify ways to deal with such large-scale
data. Appropriate data should be utilized at the time it needs to be delivered.
Conclusion
Secure Best Marks with AI Grader
Need help grading? Try our AI Grader for instant feedback on your assignments.
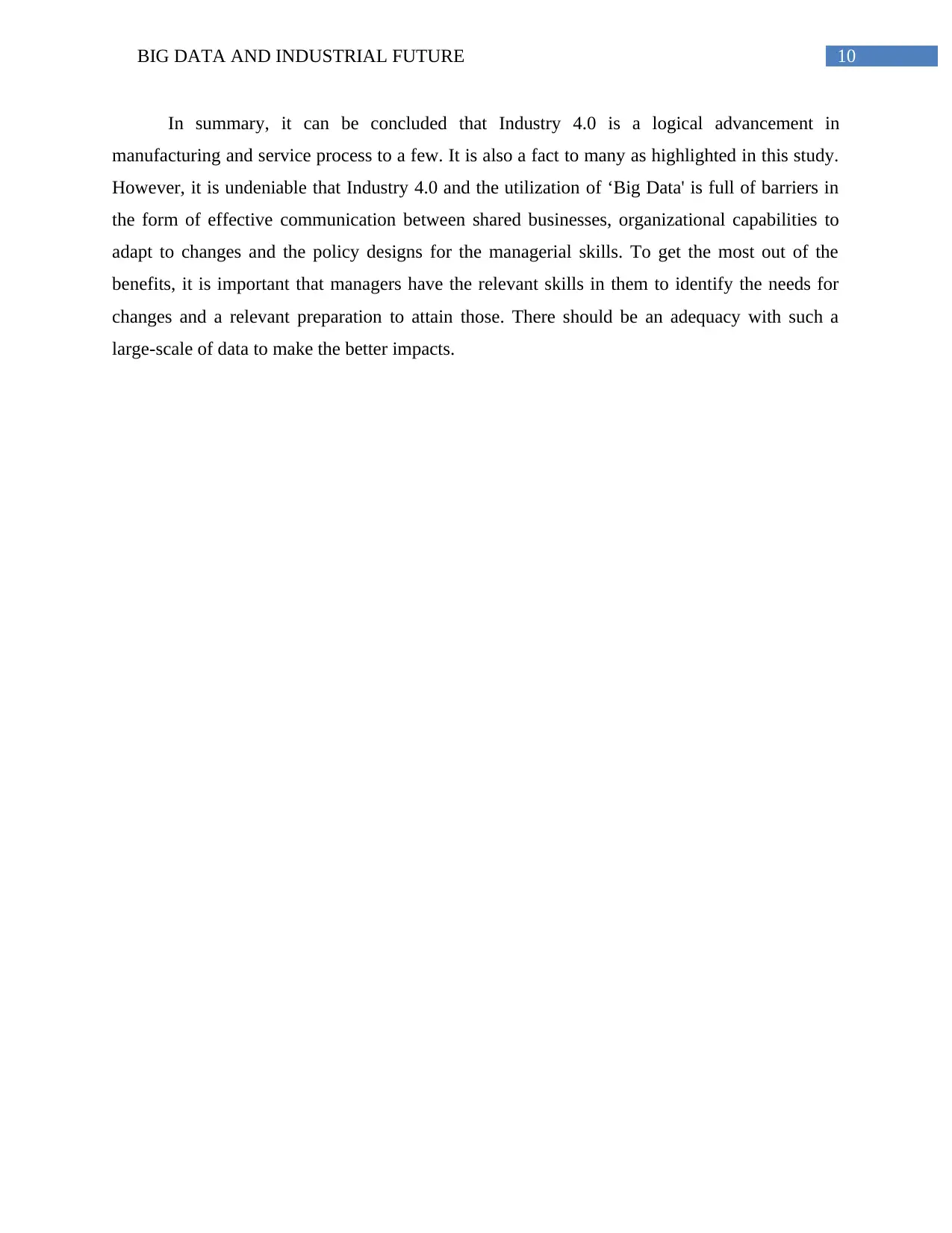
10BIG DATA AND INDUSTRIAL FUTURE
In summary, it can be concluded that Industry 4.0 is a logical advancement in
manufacturing and service process to a few. It is also a fact to many as highlighted in this study.
However, it is undeniable that Industry 4.0 and the utilization of ‘Big Data' is full of barriers in
the form of effective communication between shared businesses, organizational capabilities to
adapt to changes and the policy designs for the managerial skills. To get the most out of the
benefits, it is important that managers have the relevant skills in them to identify the needs for
changes and a relevant preparation to attain those. There should be an adequacy with such a
large-scale of data to make the better impacts.
In summary, it can be concluded that Industry 4.0 is a logical advancement in
manufacturing and service process to a few. It is also a fact to many as highlighted in this study.
However, it is undeniable that Industry 4.0 and the utilization of ‘Big Data' is full of barriers in
the form of effective communication between shared businesses, organizational capabilities to
adapt to changes and the policy designs for the managerial skills. To get the most out of the
benefits, it is important that managers have the relevant skills in them to identify the needs for
changes and a relevant preparation to attain those. There should be an adequacy with such a
large-scale of data to make the better impacts.
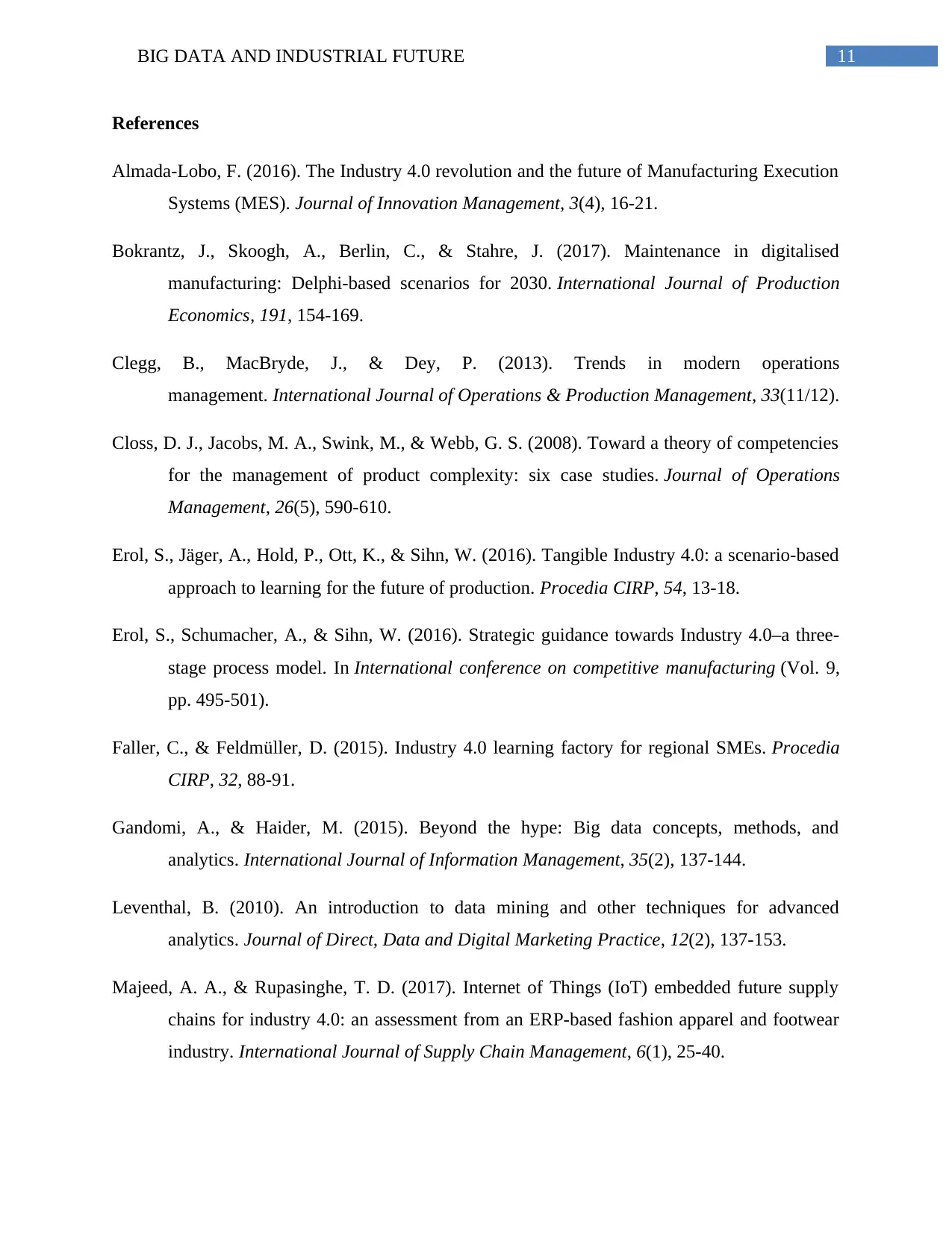
11BIG DATA AND INDUSTRIAL FUTURE
References
Almada-Lobo, F. (2016). The Industry 4.0 revolution and the future of Manufacturing Execution
Systems (MES). Journal of Innovation Management, 3(4), 16-21.
Bokrantz, J., Skoogh, A., Berlin, C., & Stahre, J. (2017). Maintenance in digitalised
manufacturing: Delphi-based scenarios for 2030. International Journal of Production
Economics, 191, 154-169.
Clegg, B., MacBryde, J., & Dey, P. (2013). Trends in modern operations
management. International Journal of Operations & Production Management, 33(11/12).
Closs, D. J., Jacobs, M. A., Swink, M., & Webb, G. S. (2008). Toward a theory of competencies
for the management of product complexity: six case studies. Journal of Operations
Management, 26(5), 590-610.
Erol, S., Jäger, A., Hold, P., Ott, K., & Sihn, W. (2016). Tangible Industry 4.0: a scenario-based
approach to learning for the future of production. Procedia CIRP, 54, 13-18.
Erol, S., Schumacher, A., & Sihn, W. (2016). Strategic guidance towards Industry 4.0–a three-
stage process model. In International conference on competitive manufacturing (Vol. 9,
pp. 495-501).
Faller, C., & Feldmüller, D. (2015). Industry 4.0 learning factory for regional SMEs. Procedia
CIRP, 32, 88-91.
Gandomi, A., & Haider, M. (2015). Beyond the hype: Big data concepts, methods, and
analytics. International Journal of Information Management, 35(2), 137-144.
Leventhal, B. (2010). An introduction to data mining and other techniques for advanced
analytics. Journal of Direct, Data and Digital Marketing Practice, 12(2), 137-153.
Majeed, A. A., & Rupasinghe, T. D. (2017). Internet of Things (IoT) embedded future supply
chains for industry 4.0: an assessment from an ERP-based fashion apparel and footwear
industry. International Journal of Supply Chain Management, 6(1), 25-40.
References
Almada-Lobo, F. (2016). The Industry 4.0 revolution and the future of Manufacturing Execution
Systems (MES). Journal of Innovation Management, 3(4), 16-21.
Bokrantz, J., Skoogh, A., Berlin, C., & Stahre, J. (2017). Maintenance in digitalised
manufacturing: Delphi-based scenarios for 2030. International Journal of Production
Economics, 191, 154-169.
Clegg, B., MacBryde, J., & Dey, P. (2013). Trends in modern operations
management. International Journal of Operations & Production Management, 33(11/12).
Closs, D. J., Jacobs, M. A., Swink, M., & Webb, G. S. (2008). Toward a theory of competencies
for the management of product complexity: six case studies. Journal of Operations
Management, 26(5), 590-610.
Erol, S., Jäger, A., Hold, P., Ott, K., & Sihn, W. (2016). Tangible Industry 4.0: a scenario-based
approach to learning for the future of production. Procedia CIRP, 54, 13-18.
Erol, S., Schumacher, A., & Sihn, W. (2016). Strategic guidance towards Industry 4.0–a three-
stage process model. In International conference on competitive manufacturing (Vol. 9,
pp. 495-501).
Faller, C., & Feldmüller, D. (2015). Industry 4.0 learning factory for regional SMEs. Procedia
CIRP, 32, 88-91.
Gandomi, A., & Haider, M. (2015). Beyond the hype: Big data concepts, methods, and
analytics. International Journal of Information Management, 35(2), 137-144.
Leventhal, B. (2010). An introduction to data mining and other techniques for advanced
analytics. Journal of Direct, Data and Digital Marketing Practice, 12(2), 137-153.
Majeed, A. A., & Rupasinghe, T. D. (2017). Internet of Things (IoT) embedded future supply
chains for industry 4.0: an assessment from an ERP-based fashion apparel and footwear
industry. International Journal of Supply Chain Management, 6(1), 25-40.
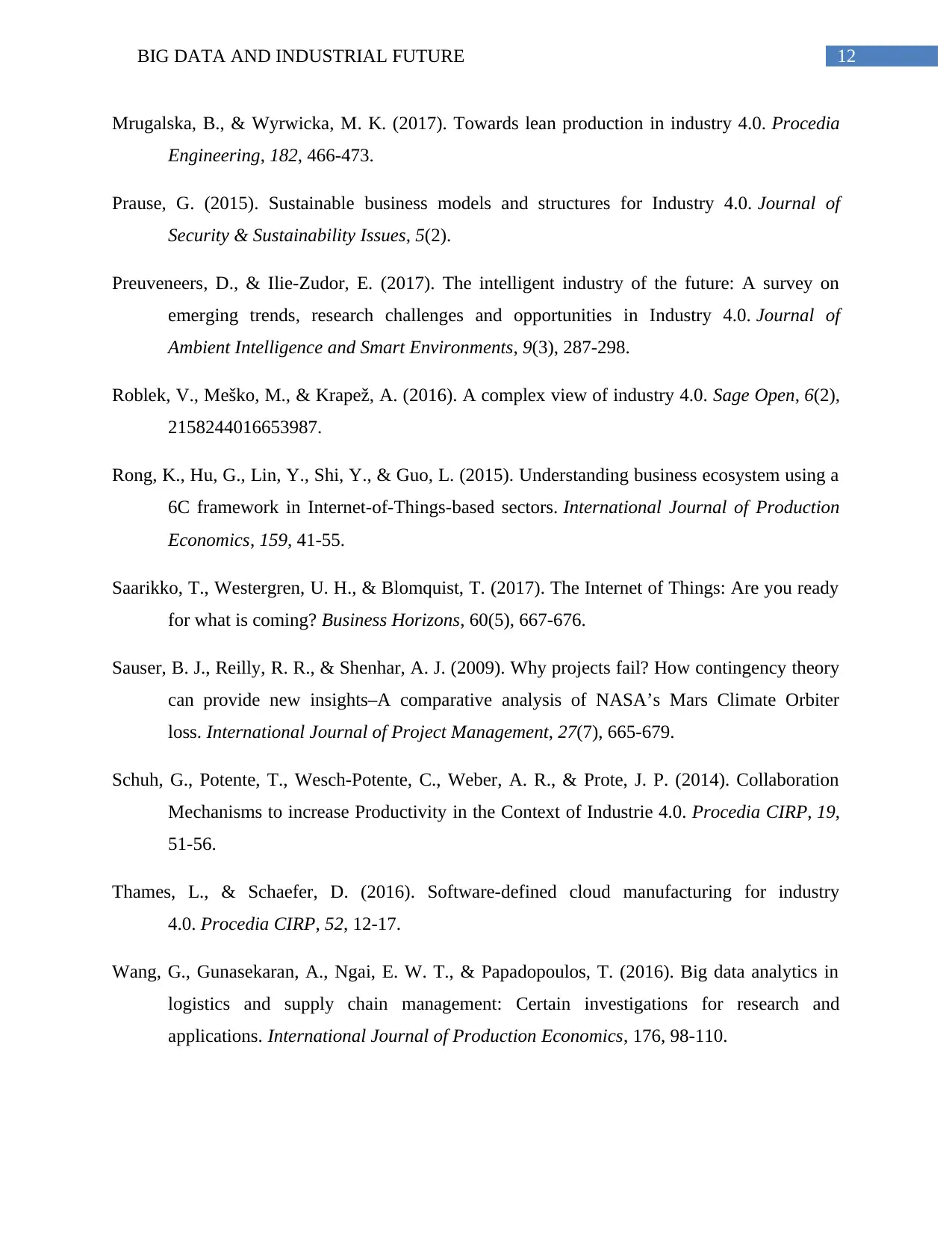
12BIG DATA AND INDUSTRIAL FUTURE
Mrugalska, B., & Wyrwicka, M. K. (2017). Towards lean production in industry 4.0. Procedia
Engineering, 182, 466-473.
Prause, G. (2015). Sustainable business models and structures for Industry 4.0. Journal of
Security & Sustainability Issues, 5(2).
Preuveneers, D., & Ilie-Zudor, E. (2017). The intelligent industry of the future: A survey on
emerging trends, research challenges and opportunities in Industry 4.0. Journal of
Ambient Intelligence and Smart Environments, 9(3), 287-298.
Roblek, V., Meško, M., & Krapež, A. (2016). A complex view of industry 4.0. Sage Open, 6(2),
2158244016653987.
Rong, K., Hu, G., Lin, Y., Shi, Y., & Guo, L. (2015). Understanding business ecosystem using a
6C framework in Internet-of-Things-based sectors. International Journal of Production
Economics, 159, 41-55.
Saarikko, T., Westergren, U. H., & Blomquist, T. (2017). The Internet of Things: Are you ready
for what is coming? Business Horizons, 60(5), 667-676.
Sauser, B. J., Reilly, R. R., & Shenhar, A. J. (2009). Why projects fail? How contingency theory
can provide new insights–A comparative analysis of NASA’s Mars Climate Orbiter
loss. International Journal of Project Management, 27(7), 665-679.
Schuh, G., Potente, T., Wesch-Potente, C., Weber, A. R., & Prote, J. P. (2014). Collaboration
Mechanisms to increase Productivity in the Context of Industrie 4.0. Procedia CIRP, 19,
51-56.
Thames, L., & Schaefer, D. (2016). Software-defined cloud manufacturing for industry
4.0. Procedia CIRP, 52, 12-17.
Wang, G., Gunasekaran, A., Ngai, E. W. T., & Papadopoulos, T. (2016). Big data analytics in
logistics and supply chain management: Certain investigations for research and
applications. International Journal of Production Economics, 176, 98-110.
Mrugalska, B., & Wyrwicka, M. K. (2017). Towards lean production in industry 4.0. Procedia
Engineering, 182, 466-473.
Prause, G. (2015). Sustainable business models and structures for Industry 4.0. Journal of
Security & Sustainability Issues, 5(2).
Preuveneers, D., & Ilie-Zudor, E. (2017). The intelligent industry of the future: A survey on
emerging trends, research challenges and opportunities in Industry 4.0. Journal of
Ambient Intelligence and Smart Environments, 9(3), 287-298.
Roblek, V., Meško, M., & Krapež, A. (2016). A complex view of industry 4.0. Sage Open, 6(2),
2158244016653987.
Rong, K., Hu, G., Lin, Y., Shi, Y., & Guo, L. (2015). Understanding business ecosystem using a
6C framework in Internet-of-Things-based sectors. International Journal of Production
Economics, 159, 41-55.
Saarikko, T., Westergren, U. H., & Blomquist, T. (2017). The Internet of Things: Are you ready
for what is coming? Business Horizons, 60(5), 667-676.
Sauser, B. J., Reilly, R. R., & Shenhar, A. J. (2009). Why projects fail? How contingency theory
can provide new insights–A comparative analysis of NASA’s Mars Climate Orbiter
loss. International Journal of Project Management, 27(7), 665-679.
Schuh, G., Potente, T., Wesch-Potente, C., Weber, A. R., & Prote, J. P. (2014). Collaboration
Mechanisms to increase Productivity in the Context of Industrie 4.0. Procedia CIRP, 19,
51-56.
Thames, L., & Schaefer, D. (2016). Software-defined cloud manufacturing for industry
4.0. Procedia CIRP, 52, 12-17.
Wang, G., Gunasekaran, A., Ngai, E. W. T., & Papadopoulos, T. (2016). Big data analytics in
logistics and supply chain management: Certain investigations for research and
applications. International Journal of Production Economics, 176, 98-110.
Paraphrase This Document
Need a fresh take? Get an instant paraphrase of this document with our AI Paraphraser
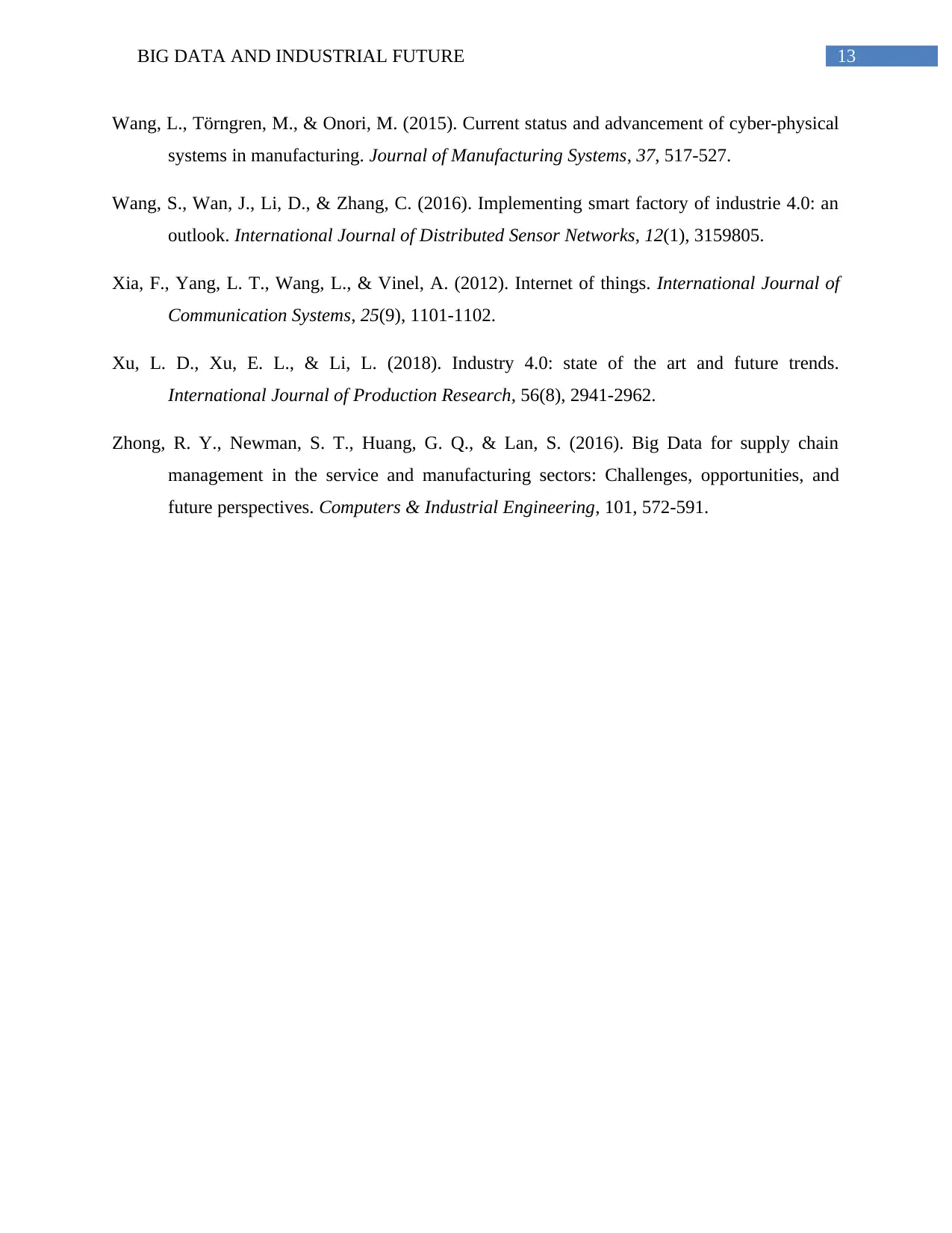
13BIG DATA AND INDUSTRIAL FUTURE
Wang, L., Törngren, M., & Onori, M. (2015). Current status and advancement of cyber-physical
systems in manufacturing. Journal of Manufacturing Systems, 37, 517-527.
Wang, S., Wan, J., Li, D., & Zhang, C. (2016). Implementing smart factory of industrie 4.0: an
outlook. International Journal of Distributed Sensor Networks, 12(1), 3159805.
Xia, F., Yang, L. T., Wang, L., & Vinel, A. (2012). Internet of things. International Journal of
Communication Systems, 25(9), 1101-1102.
Xu, L. D., Xu, E. L., & Li, L. (2018). Industry 4.0: state of the art and future trends.
International Journal of Production Research, 56(8), 2941-2962.
Zhong, R. Y., Newman, S. T., Huang, G. Q., & Lan, S. (2016). Big Data for supply chain
management in the service and manufacturing sectors: Challenges, opportunities, and
future perspectives. Computers & Industrial Engineering, 101, 572-591.
Wang, L., Törngren, M., & Onori, M. (2015). Current status and advancement of cyber-physical
systems in manufacturing. Journal of Manufacturing Systems, 37, 517-527.
Wang, S., Wan, J., Li, D., & Zhang, C. (2016). Implementing smart factory of industrie 4.0: an
outlook. International Journal of Distributed Sensor Networks, 12(1), 3159805.
Xia, F., Yang, L. T., Wang, L., & Vinel, A. (2012). Internet of things. International Journal of
Communication Systems, 25(9), 1101-1102.
Xu, L. D., Xu, E. L., & Li, L. (2018). Industry 4.0: state of the art and future trends.
International Journal of Production Research, 56(8), 2941-2962.
Zhong, R. Y., Newman, S. T., Huang, G. Q., & Lan, S. (2016). Big Data for supply chain
management in the service and manufacturing sectors: Challenges, opportunities, and
future perspectives. Computers & Industrial Engineering, 101, 572-591.
1 out of 14
Related Documents
![[object Object]](/_next/image/?url=%2F_next%2Fstatic%2Fmedia%2Flogo.6d15ce61.png&w=640&q=75)
Your All-in-One AI-Powered Toolkit for Academic Success.
+13062052269
info@desklib.com
Available 24*7 on WhatsApp / Email
Unlock your academic potential
© 2024 | Zucol Services PVT LTD | All rights reserved.