Understanding Secondary Data in Research
VerifiedAdded on 2020/02/24
|10
|2595
|95
AI Summary
This assignment delves into the concept of secondary data in research. It explains how this pre-existing information, collected from archives or databases, can be valuable for researchers. The text highlights the advantages of using secondary data, such as cost-effectiveness, time-saving, and high statistical precision when obtained from large samples. It also emphasizes the importance of verifying the accuracy, relevance, and representativeness of secondary data before utilizing it in a study.
Contribute Materials
Your contribution can guide someone’s learning journey. Share your
documents today.
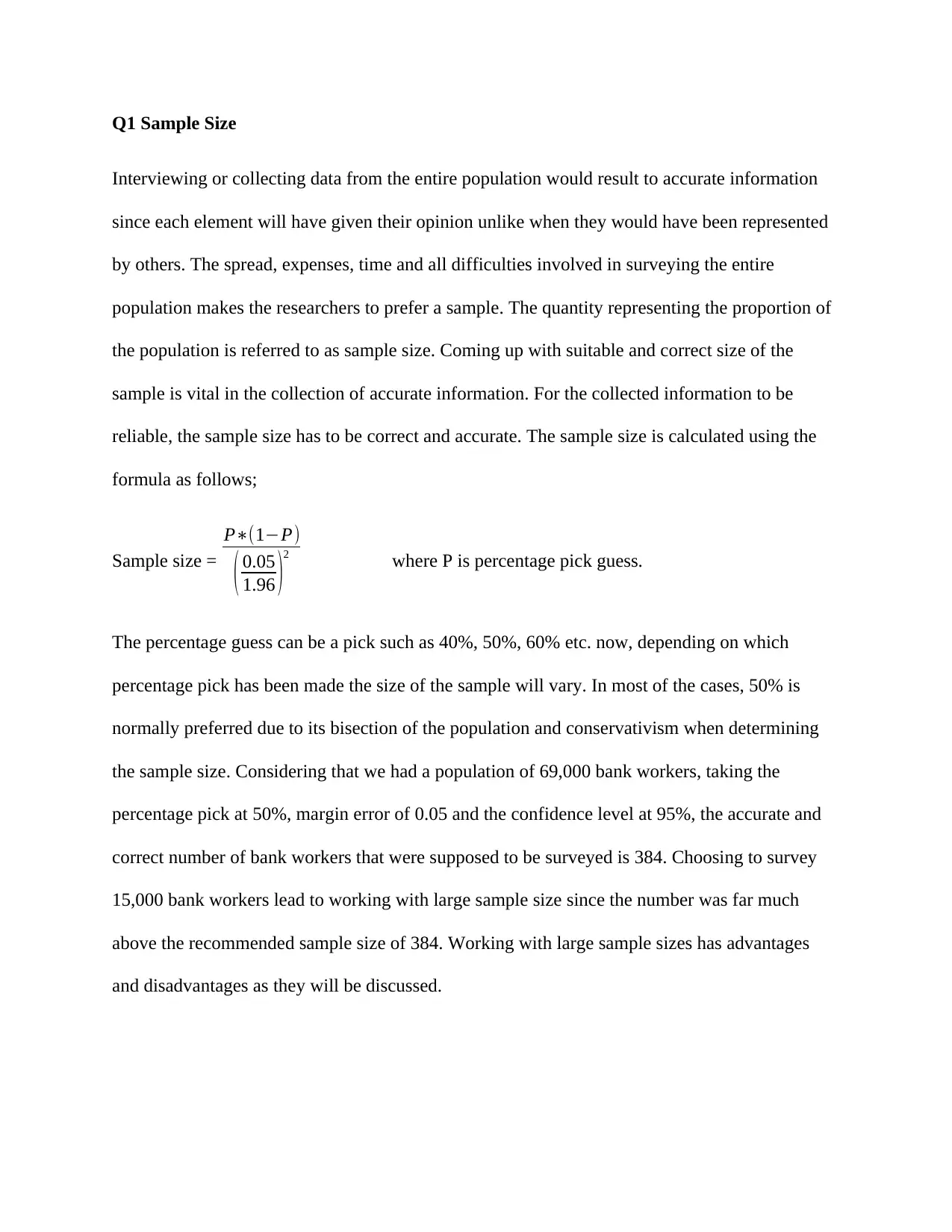
Q1 Sample Size
Interviewing or collecting data from the entire population would result to accurate information
since each element will have given their opinion unlike when they would have been represented
by others. The spread, expenses, time and all difficulties involved in surveying the entire
population makes the researchers to prefer a sample. The quantity representing the proportion of
the population is referred to as sample size. Coming up with suitable and correct size of the
sample is vital in the collection of accurate information. For the collected information to be
reliable, the sample size has to be correct and accurate. The sample size is calculated using the
formula as follows;
Sample size =
P∗(1−P)
( 0.05
1.96 )
2 where P is percentage pick guess.
The percentage guess can be a pick such as 40%, 50%, 60% etc. now, depending on which
percentage pick has been made the size of the sample will vary. In most of the cases, 50% is
normally preferred due to its bisection of the population and conservativism when determining
the sample size. Considering that we had a population of 69,000 bank workers, taking the
percentage pick at 50%, margin error of 0.05 and the confidence level at 95%, the accurate and
correct number of bank workers that were supposed to be surveyed is 384. Choosing to survey
15,000 bank workers lead to working with large sample size since the number was far much
above the recommended sample size of 384. Working with large sample sizes has advantages
and disadvantages as they will be discussed.
Interviewing or collecting data from the entire population would result to accurate information
since each element will have given their opinion unlike when they would have been represented
by others. The spread, expenses, time and all difficulties involved in surveying the entire
population makes the researchers to prefer a sample. The quantity representing the proportion of
the population is referred to as sample size. Coming up with suitable and correct size of the
sample is vital in the collection of accurate information. For the collected information to be
reliable, the sample size has to be correct and accurate. The sample size is calculated using the
formula as follows;
Sample size =
P∗(1−P)
( 0.05
1.96 )
2 where P is percentage pick guess.
The percentage guess can be a pick such as 40%, 50%, 60% etc. now, depending on which
percentage pick has been made the size of the sample will vary. In most of the cases, 50% is
normally preferred due to its bisection of the population and conservativism when determining
the sample size. Considering that we had a population of 69,000 bank workers, taking the
percentage pick at 50%, margin error of 0.05 and the confidence level at 95%, the accurate and
correct number of bank workers that were supposed to be surveyed is 384. Choosing to survey
15,000 bank workers lead to working with large sample size since the number was far much
above the recommended sample size of 384. Working with large sample sizes has advantages
and disadvantages as they will be discussed.
Secure Best Marks with AI Grader
Need help grading? Try our AI Grader for instant feedback on your assignments.
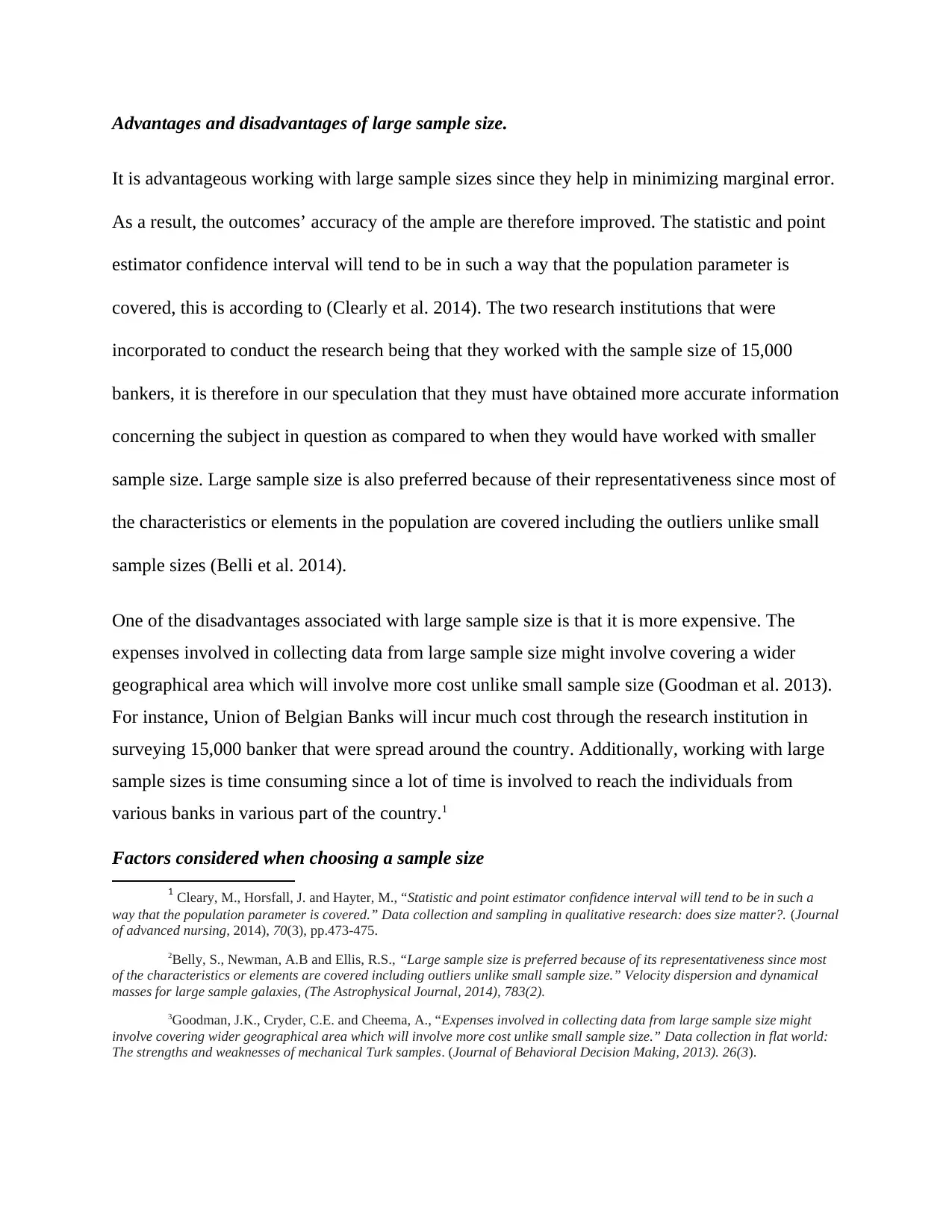
Advantages and disadvantages of large sample size.
It is advantageous working with large sample sizes since they help in minimizing marginal error.
As a result, the outcomes’ accuracy of the ample are therefore improved. The statistic and point
estimator confidence interval will tend to be in such a way that the population parameter is
covered, this is according to (Clearly et al. 2014). The two research institutions that were
incorporated to conduct the research being that they worked with the sample size of 15,000
bankers, it is therefore in our speculation that they must have obtained more accurate information
concerning the subject in question as compared to when they would have worked with smaller
sample size. Large sample size is also preferred because of their representativeness since most of
the characteristics or elements in the population are covered including the outliers unlike small
sample sizes (Belli et al. 2014).
One of the disadvantages associated with large sample size is that it is more expensive. The
expenses involved in collecting data from large sample size might involve covering a wider
geographical area which will involve more cost unlike small sample size (Goodman et al. 2013).
For instance, Union of Belgian Banks will incur much cost through the research institution in
surveying 15,000 banker that were spread around the country. Additionally, working with large
sample sizes is time consuming since a lot of time is involved to reach the individuals from
various banks in various part of the country.1
Factors considered when choosing a sample size
1 Cleary, M., Horsfall, J. and Hayter, M., “Statistic and point estimator confidence interval will tend to be in such a
way that the population parameter is covered.” Data collection and sampling in qualitative research: does size matter?. (Journal
of advanced nursing, 2014), 70(3), pp.473-475.
2Belly, S., Newman, A.B and Ellis, R.S., “Large sample size is preferred because of its representativeness since most
of the characteristics or elements are covered including outliers unlike small sample size.” Velocity dispersion and dynamical
masses for large sample galaxies, (The Astrophysical Journal, 2014), 783(2).
3Goodman, J.K., Cryder, C.E. and Cheema, A., “Expenses involved in collecting data from large sample size might
involve covering wider geographical area which will involve more cost unlike small sample size.” Data collection in flat world:
The strengths and weaknesses of mechanical Turk samples. (Journal of Behavioral Decision Making, 2013). 26(3).
It is advantageous working with large sample sizes since they help in minimizing marginal error.
As a result, the outcomes’ accuracy of the ample are therefore improved. The statistic and point
estimator confidence interval will tend to be in such a way that the population parameter is
covered, this is according to (Clearly et al. 2014). The two research institutions that were
incorporated to conduct the research being that they worked with the sample size of 15,000
bankers, it is therefore in our speculation that they must have obtained more accurate information
concerning the subject in question as compared to when they would have worked with smaller
sample size. Large sample size is also preferred because of their representativeness since most of
the characteristics or elements in the population are covered including the outliers unlike small
sample sizes (Belli et al. 2014).
One of the disadvantages associated with large sample size is that it is more expensive. The
expenses involved in collecting data from large sample size might involve covering a wider
geographical area which will involve more cost unlike small sample size (Goodman et al. 2013).
For instance, Union of Belgian Banks will incur much cost through the research institution in
surveying 15,000 banker that were spread around the country. Additionally, working with large
sample sizes is time consuming since a lot of time is involved to reach the individuals from
various banks in various part of the country.1
Factors considered when choosing a sample size
1 Cleary, M., Horsfall, J. and Hayter, M., “Statistic and point estimator confidence interval will tend to be in such a
way that the population parameter is covered.” Data collection and sampling in qualitative research: does size matter?. (Journal
of advanced nursing, 2014), 70(3), pp.473-475.
2Belly, S., Newman, A.B and Ellis, R.S., “Large sample size is preferred because of its representativeness since most
of the characteristics or elements are covered including outliers unlike small sample size.” Velocity dispersion and dynamical
masses for large sample galaxies, (The Astrophysical Journal, 2014), 783(2).
3Goodman, J.K., Cryder, C.E. and Cheema, A., “Expenses involved in collecting data from large sample size might
involve covering wider geographical area which will involve more cost unlike small sample size.” Data collection in flat world:
The strengths and weaknesses of mechanical Turk samples. (Journal of Behavioral Decision Making, 2013). 26(3).
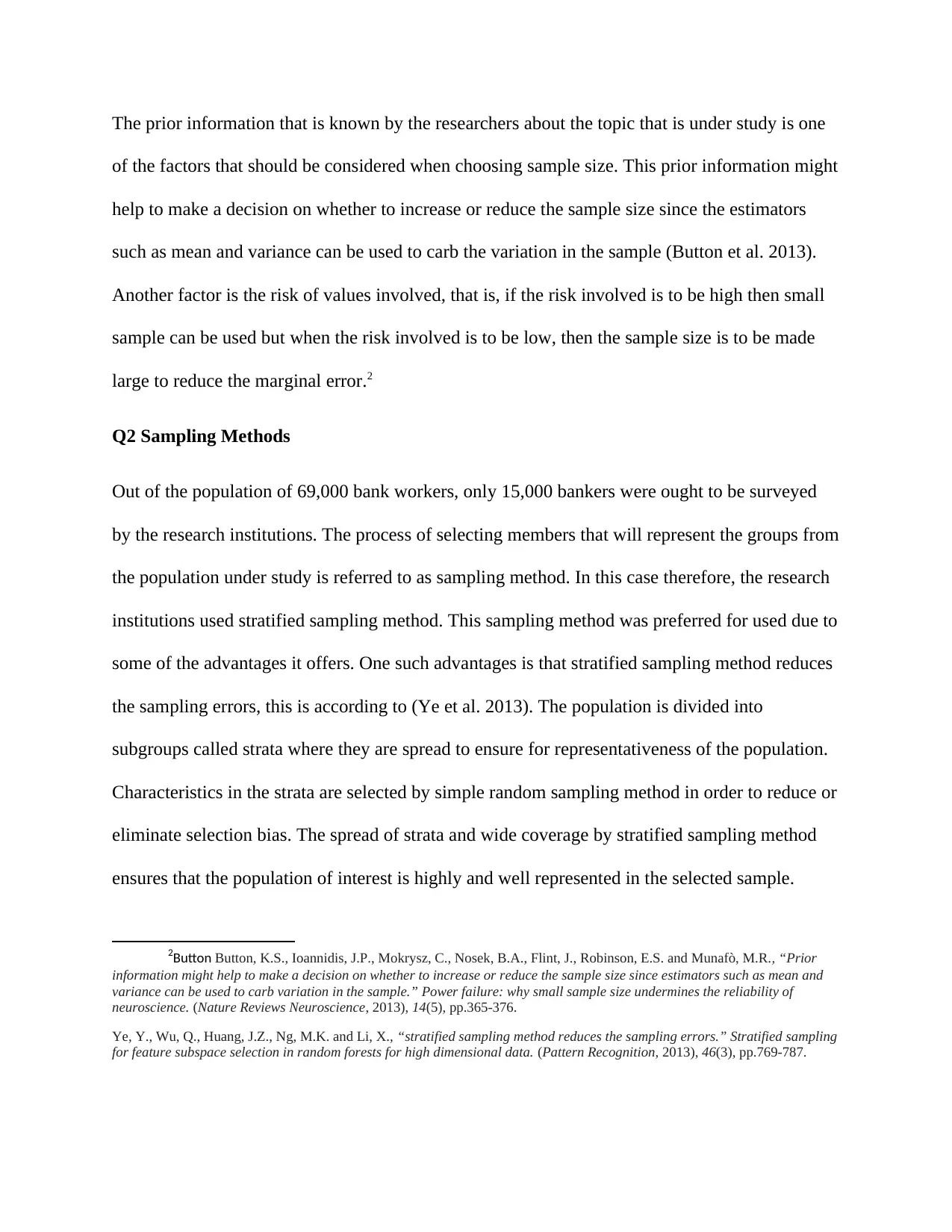
The prior information that is known by the researchers about the topic that is under study is one
of the factors that should be considered when choosing sample size. This prior information might
help to make a decision on whether to increase or reduce the sample size since the estimators
such as mean and variance can be used to carb the variation in the sample (Button et al. 2013).
Another factor is the risk of values involved, that is, if the risk involved is to be high then small
sample can be used but when the risk involved is to be low, then the sample size is to be made
large to reduce the marginal error.2
Q2 Sampling Methods
Out of the population of 69,000 bank workers, only 15,000 bankers were ought to be surveyed
by the research institutions. The process of selecting members that will represent the groups from
the population under study is referred to as sampling method. In this case therefore, the research
institutions used stratified sampling method. This sampling method was preferred for used due to
some of the advantages it offers. One such advantages is that stratified sampling method reduces
the sampling errors, this is according to (Ye et al. 2013). The population is divided into
subgroups called strata where they are spread to ensure for representativeness of the population.
Characteristics in the strata are selected by simple random sampling method in order to reduce or
eliminate selection bias. The spread of strata and wide coverage by stratified sampling method
ensures that the population of interest is highly and well represented in the selected sample.
2Button Button, K.S., Ioannidis, J.P., Mokrysz, C., Nosek, B.A., Flint, J., Robinson, E.S. and Munafò, M.R., “Prior
information might help to make a decision on whether to increase or reduce the sample size since estimators such as mean and
variance can be used to carb variation in the sample.” Power failure: why small sample size undermines the reliability of
neuroscience. (Nature Reviews Neuroscience, 2013), 14(5), pp.365-376.
Ye, Y., Wu, Q., Huang, J.Z., Ng, M.K. and Li, X., “stratified sampling method reduces the sampling errors.” Stratified sampling
for feature subspace selection in random forests for high dimensional data. (Pattern Recognition, 2013), 46(3), pp.769-787.
of the factors that should be considered when choosing sample size. This prior information might
help to make a decision on whether to increase or reduce the sample size since the estimators
such as mean and variance can be used to carb the variation in the sample (Button et al. 2013).
Another factor is the risk of values involved, that is, if the risk involved is to be high then small
sample can be used but when the risk involved is to be low, then the sample size is to be made
large to reduce the marginal error.2
Q2 Sampling Methods
Out of the population of 69,000 bank workers, only 15,000 bankers were ought to be surveyed
by the research institutions. The process of selecting members that will represent the groups from
the population under study is referred to as sampling method. In this case therefore, the research
institutions used stratified sampling method. This sampling method was preferred for used due to
some of the advantages it offers. One such advantages is that stratified sampling method reduces
the sampling errors, this is according to (Ye et al. 2013). The population is divided into
subgroups called strata where they are spread to ensure for representativeness of the population.
Characteristics in the strata are selected by simple random sampling method in order to reduce or
eliminate selection bias. The spread of strata and wide coverage by stratified sampling method
ensures that the population of interest is highly and well represented in the selected sample.
2Button Button, K.S., Ioannidis, J.P., Mokrysz, C., Nosek, B.A., Flint, J., Robinson, E.S. and Munafò, M.R., “Prior
information might help to make a decision on whether to increase or reduce the sample size since estimators such as mean and
variance can be used to carb variation in the sample.” Power failure: why small sample size undermines the reliability of
neuroscience. (Nature Reviews Neuroscience, 2013), 14(5), pp.365-376.
Ye, Y., Wu, Q., Huang, J.Z., Ng, M.K. and Li, X., “stratified sampling method reduces the sampling errors.” Stratified sampling
for feature subspace selection in random forests for high dimensional data. (Pattern Recognition, 2013), 46(3), pp.769-787.
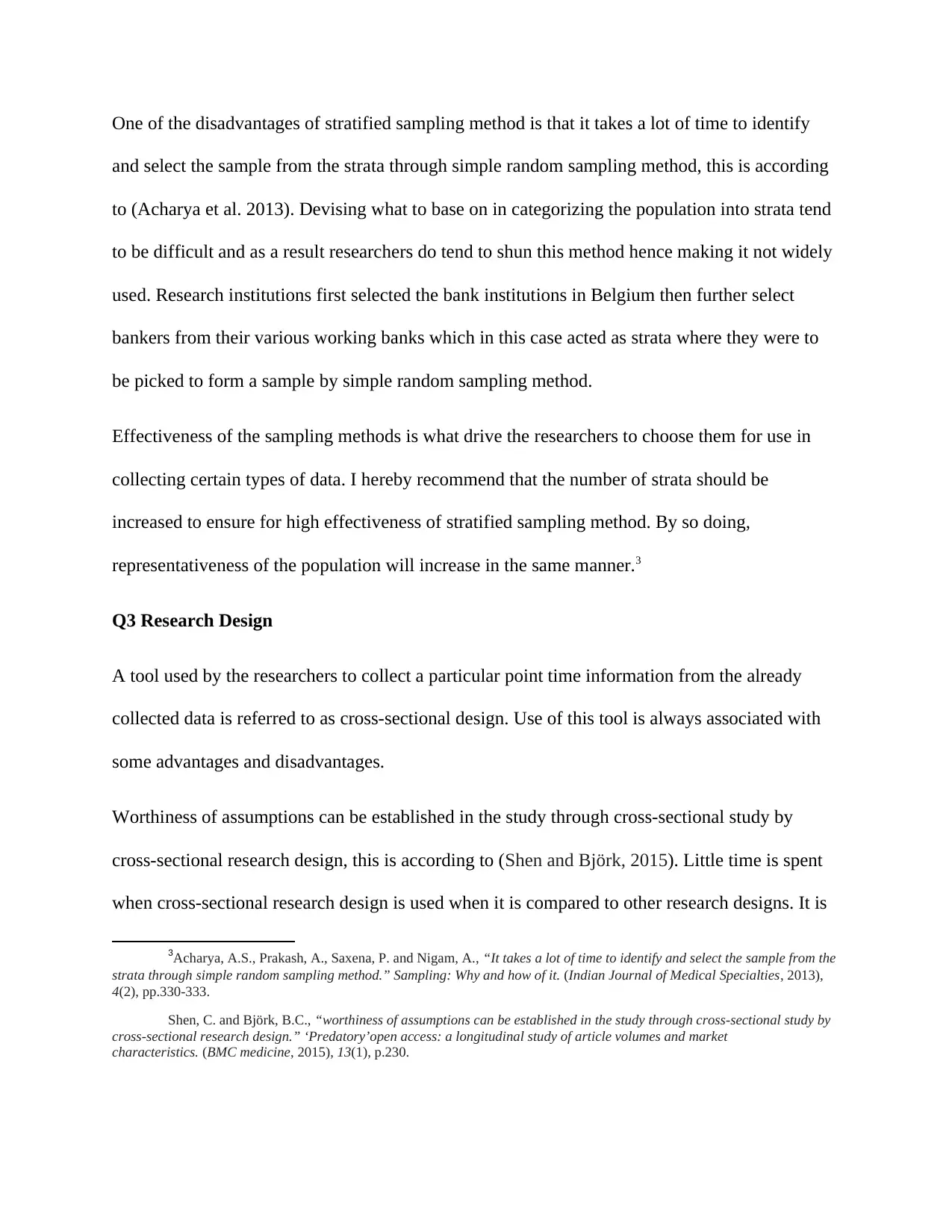
One of the disadvantages of stratified sampling method is that it takes a lot of time to identify
and select the sample from the strata through simple random sampling method, this is according
to (Acharya et al. 2013). Devising what to base on in categorizing the population into strata tend
to be difficult and as a result researchers do tend to shun this method hence making it not widely
used. Research institutions first selected the bank institutions in Belgium then further select
bankers from their various working banks which in this case acted as strata where they were to
be picked to form a sample by simple random sampling method.
Effectiveness of the sampling methods is what drive the researchers to choose them for use in
collecting certain types of data. I hereby recommend that the number of strata should be
increased to ensure for high effectiveness of stratified sampling method. By so doing,
representativeness of the population will increase in the same manner.3
Q3 Research Design
A tool used by the researchers to collect a particular point time information from the already
collected data is referred to as cross-sectional design. Use of this tool is always associated with
some advantages and disadvantages.
Worthiness of assumptions can be established in the study through cross-sectional study by
cross-sectional research design, this is according to (Shen and Björk, 2015). Little time is spent
when cross-sectional research design is used when it is compared to other research designs. It is
3Acharya, A.S., Prakash, A., Saxena, P. and Nigam, A., “It takes a lot of time to identify and select the sample from the
strata through simple random sampling method.” Sampling: Why and how of it. (Indian Journal of Medical Specialties, 2013),
4(2), pp.330-333.
Shen, C. and Björk, B.C., “worthiness of assumptions can be established in the study through cross-sectional study by
cross-sectional research design.” ‘Predatory’open access: a longitudinal study of article volumes and market
characteristics. (BMC medicine, 2015), 13(1), p.230.
and select the sample from the strata through simple random sampling method, this is according
to (Acharya et al. 2013). Devising what to base on in categorizing the population into strata tend
to be difficult and as a result researchers do tend to shun this method hence making it not widely
used. Research institutions first selected the bank institutions in Belgium then further select
bankers from their various working banks which in this case acted as strata where they were to
be picked to form a sample by simple random sampling method.
Effectiveness of the sampling methods is what drive the researchers to choose them for use in
collecting certain types of data. I hereby recommend that the number of strata should be
increased to ensure for high effectiveness of stratified sampling method. By so doing,
representativeness of the population will increase in the same manner.3
Q3 Research Design
A tool used by the researchers to collect a particular point time information from the already
collected data is referred to as cross-sectional design. Use of this tool is always associated with
some advantages and disadvantages.
Worthiness of assumptions can be established in the study through cross-sectional study by
cross-sectional research design, this is according to (Shen and Björk, 2015). Little time is spent
when cross-sectional research design is used when it is compared to other research designs. It is
3Acharya, A.S., Prakash, A., Saxena, P. and Nigam, A., “It takes a lot of time to identify and select the sample from the
strata through simple random sampling method.” Sampling: Why and how of it. (Indian Journal of Medical Specialties, 2013),
4(2), pp.330-333.
Shen, C. and Björk, B.C., “worthiness of assumptions can be established in the study through cross-sectional study by
cross-sectional research design.” ‘Predatory’open access: a longitudinal study of article volumes and market
characteristics. (BMC medicine, 2015), 13(1), p.230.
Secure Best Marks with AI Grader
Need help grading? Try our AI Grader for instant feedback on your assignments.
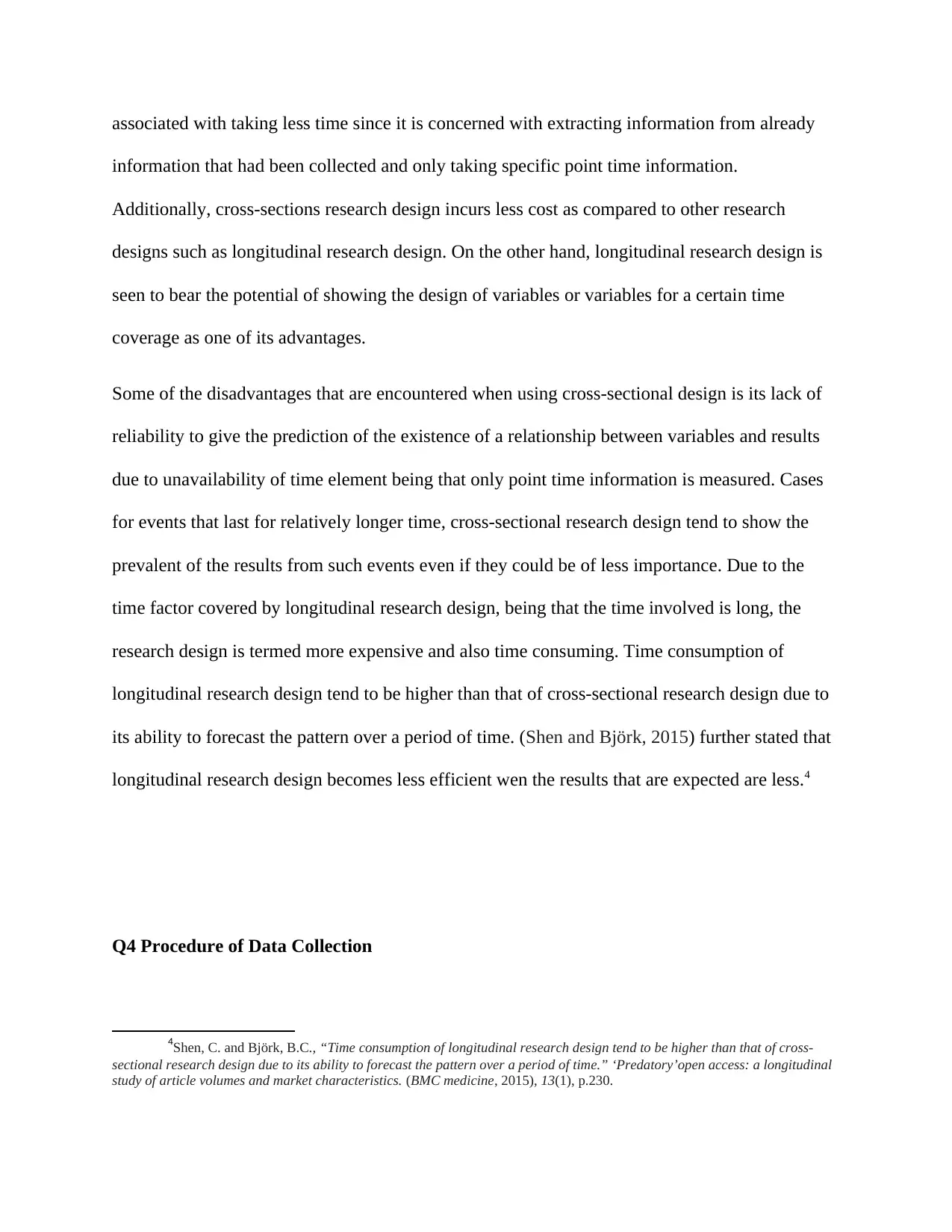
associated with taking less time since it is concerned with extracting information from already
information that had been collected and only taking specific point time information.
Additionally, cross-sections research design incurs less cost as compared to other research
designs such as longitudinal research design. On the other hand, longitudinal research design is
seen to bear the potential of showing the design of variables or variables for a certain time
coverage as one of its advantages.
Some of the disadvantages that are encountered when using cross-sectional design is its lack of
reliability to give the prediction of the existence of a relationship between variables and results
due to unavailability of time element being that only point time information is measured. Cases
for events that last for relatively longer time, cross-sectional research design tend to show the
prevalent of the results from such events even if they could be of less importance. Due to the
time factor covered by longitudinal research design, being that the time involved is long, the
research design is termed more expensive and also time consuming. Time consumption of
longitudinal research design tend to be higher than that of cross-sectional research design due to
its ability to forecast the pattern over a period of time. (Shen and Björk, 2015) further stated that
longitudinal research design becomes less efficient wen the results that are expected are less.4
Q4 Procedure of Data Collection
4Shen, C. and Björk, B.C., “Time consumption of longitudinal research design tend to be higher than that of cross-
sectional research design due to its ability to forecast the pattern over a period of time.” ‘Predatory’open access: a longitudinal
study of article volumes and market characteristics. (BMC medicine, 2015), 13(1), p.230.
information that had been collected and only taking specific point time information.
Additionally, cross-sections research design incurs less cost as compared to other research
designs such as longitudinal research design. On the other hand, longitudinal research design is
seen to bear the potential of showing the design of variables or variables for a certain time
coverage as one of its advantages.
Some of the disadvantages that are encountered when using cross-sectional design is its lack of
reliability to give the prediction of the existence of a relationship between variables and results
due to unavailability of time element being that only point time information is measured. Cases
for events that last for relatively longer time, cross-sectional research design tend to show the
prevalent of the results from such events even if they could be of less importance. Due to the
time factor covered by longitudinal research design, being that the time involved is long, the
research design is termed more expensive and also time consuming. Time consumption of
longitudinal research design tend to be higher than that of cross-sectional research design due to
its ability to forecast the pattern over a period of time. (Shen and Björk, 2015) further stated that
longitudinal research design becomes less efficient wen the results that are expected are less.4
Q4 Procedure of Data Collection
4Shen, C. and Björk, B.C., “Time consumption of longitudinal research design tend to be higher than that of cross-
sectional research design due to its ability to forecast the pattern over a period of time.” ‘Predatory’open access: a longitudinal
study of article volumes and market characteristics. (BMC medicine, 2015), 13(1), p.230.
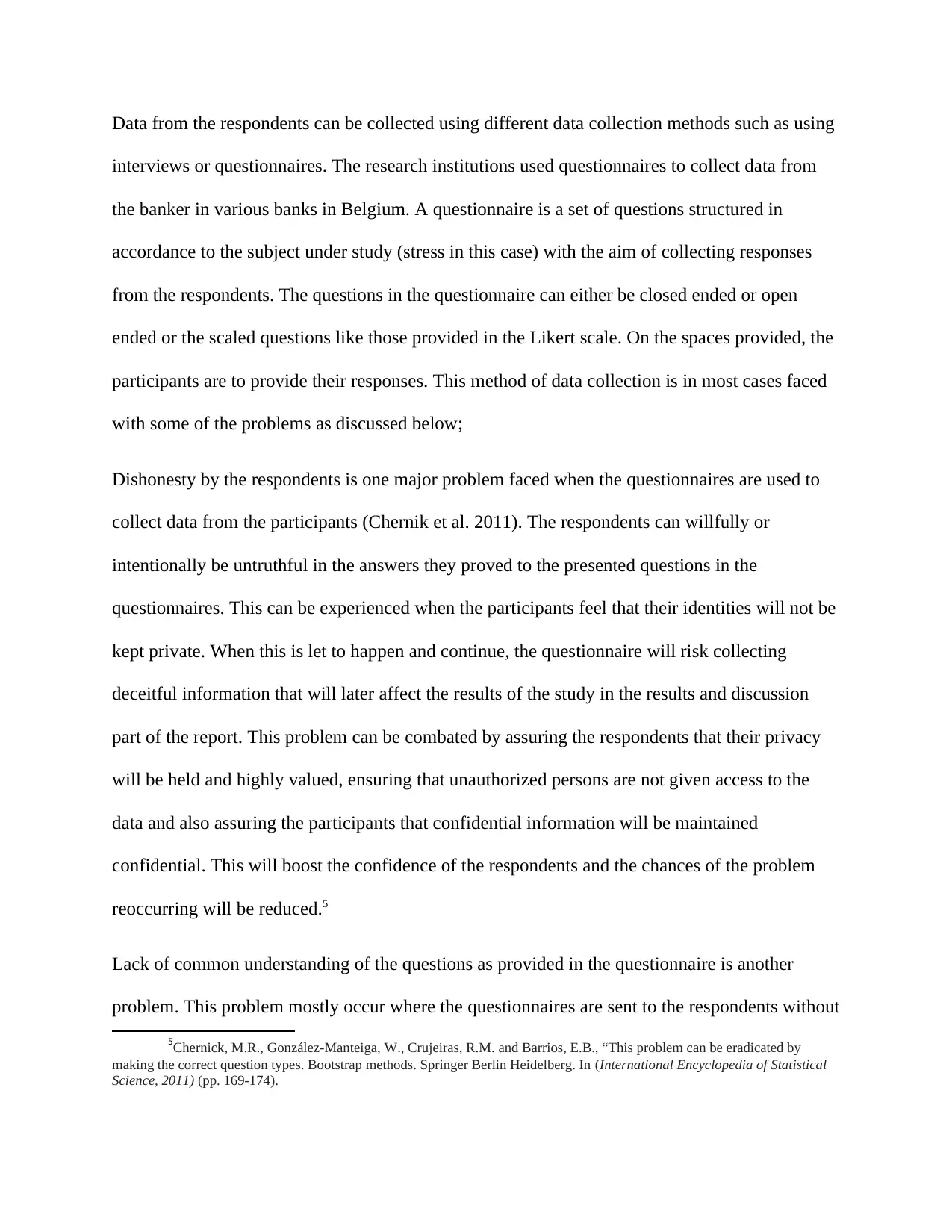
Data from the respondents can be collected using different data collection methods such as using
interviews or questionnaires. The research institutions used questionnaires to collect data from
the banker in various banks in Belgium. A questionnaire is a set of questions structured in
accordance to the subject under study (stress in this case) with the aim of collecting responses
from the respondents. The questions in the questionnaire can either be closed ended or open
ended or the scaled questions like those provided in the Likert scale. On the spaces provided, the
participants are to provide their responses. This method of data collection is in most cases faced
with some of the problems as discussed below;
Dishonesty by the respondents is one major problem faced when the questionnaires are used to
collect data from the participants (Chernik et al. 2011). The respondents can willfully or
intentionally be untruthful in the answers they proved to the presented questions in the
questionnaires. This can be experienced when the participants feel that their identities will not be
kept private. When this is let to happen and continue, the questionnaire will risk collecting
deceitful information that will later affect the results of the study in the results and discussion
part of the report. This problem can be combated by assuring the respondents that their privacy
will be held and highly valued, ensuring that unauthorized persons are not given access to the
data and also assuring the participants that confidential information will be maintained
confidential. This will boost the confidence of the respondents and the chances of the problem
reoccurring will be reduced.5
Lack of common understanding of the questions as provided in the questionnaire is another
problem. This problem mostly occur where the questionnaires are sent to the respondents without
5Chernick, M.R., González-Manteiga, W., Crujeiras, R.M. and Barrios, E.B., “This problem can be eradicated by
making the correct question types. Bootstrap methods. Springer Berlin Heidelberg. In (International Encyclopedia of Statistical
Science, 2011) (pp. 169-174).
interviews or questionnaires. The research institutions used questionnaires to collect data from
the banker in various banks in Belgium. A questionnaire is a set of questions structured in
accordance to the subject under study (stress in this case) with the aim of collecting responses
from the respondents. The questions in the questionnaire can either be closed ended or open
ended or the scaled questions like those provided in the Likert scale. On the spaces provided, the
participants are to provide their responses. This method of data collection is in most cases faced
with some of the problems as discussed below;
Dishonesty by the respondents is one major problem faced when the questionnaires are used to
collect data from the participants (Chernik et al. 2011). The respondents can willfully or
intentionally be untruthful in the answers they proved to the presented questions in the
questionnaires. This can be experienced when the participants feel that their identities will not be
kept private. When this is let to happen and continue, the questionnaire will risk collecting
deceitful information that will later affect the results of the study in the results and discussion
part of the report. This problem can be combated by assuring the respondents that their privacy
will be held and highly valued, ensuring that unauthorized persons are not given access to the
data and also assuring the participants that confidential information will be maintained
confidential. This will boost the confidence of the respondents and the chances of the problem
reoccurring will be reduced.5
Lack of common understanding of the questions as provided in the questionnaire is another
problem. This problem mostly occur where the questionnaires are sent to the respondents without
5Chernick, M.R., González-Manteiga, W., Crujeiras, R.M. and Barrios, E.B., “This problem can be eradicated by
making the correct question types. Bootstrap methods. Springer Berlin Heidelberg. In (International Encyclopedia of Statistical
Science, 2011) (pp. 169-174).
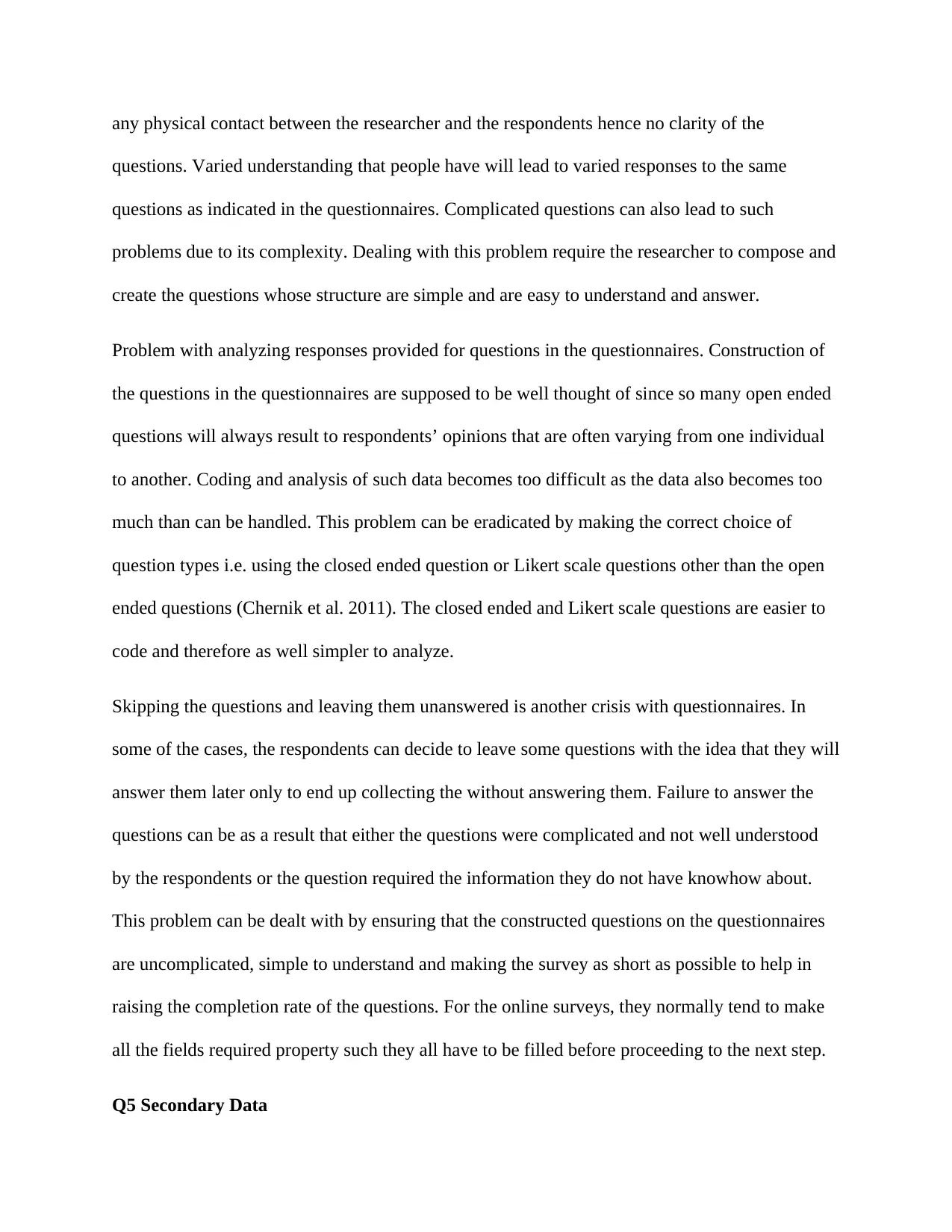
any physical contact between the researcher and the respondents hence no clarity of the
questions. Varied understanding that people have will lead to varied responses to the same
questions as indicated in the questionnaires. Complicated questions can also lead to such
problems due to its complexity. Dealing with this problem require the researcher to compose and
create the questions whose structure are simple and are easy to understand and answer.
Problem with analyzing responses provided for questions in the questionnaires. Construction of
the questions in the questionnaires are supposed to be well thought of since so many open ended
questions will always result to respondents’ opinions that are often varying from one individual
to another. Coding and analysis of such data becomes too difficult as the data also becomes too
much than can be handled. This problem can be eradicated by making the correct choice of
question types i.e. using the closed ended question or Likert scale questions other than the open
ended questions (Chernik et al. 2011). The closed ended and Likert scale questions are easier to
code and therefore as well simpler to analyze.
Skipping the questions and leaving them unanswered is another crisis with questionnaires. In
some of the cases, the respondents can decide to leave some questions with the idea that they will
answer them later only to end up collecting the without answering them. Failure to answer the
questions can be as a result that either the questions were complicated and not well understood
by the respondents or the question required the information they do not have knowhow about.
This problem can be dealt with by ensuring that the constructed questions on the questionnaires
are uncomplicated, simple to understand and making the survey as short as possible to help in
raising the completion rate of the questions. For the online surveys, they normally tend to make
all the fields required property such they all have to be filled before proceeding to the next step.
Q5 Secondary Data
questions. Varied understanding that people have will lead to varied responses to the same
questions as indicated in the questionnaires. Complicated questions can also lead to such
problems due to its complexity. Dealing with this problem require the researcher to compose and
create the questions whose structure are simple and are easy to understand and answer.
Problem with analyzing responses provided for questions in the questionnaires. Construction of
the questions in the questionnaires are supposed to be well thought of since so many open ended
questions will always result to respondents’ opinions that are often varying from one individual
to another. Coding and analysis of such data becomes too difficult as the data also becomes too
much than can be handled. This problem can be eradicated by making the correct choice of
question types i.e. using the closed ended question or Likert scale questions other than the open
ended questions (Chernik et al. 2011). The closed ended and Likert scale questions are easier to
code and therefore as well simpler to analyze.
Skipping the questions and leaving them unanswered is another crisis with questionnaires. In
some of the cases, the respondents can decide to leave some questions with the idea that they will
answer them later only to end up collecting the without answering them. Failure to answer the
questions can be as a result that either the questions were complicated and not well understood
by the respondents or the question required the information they do not have knowhow about.
This problem can be dealt with by ensuring that the constructed questions on the questionnaires
are uncomplicated, simple to understand and making the survey as short as possible to help in
raising the completion rate of the questions. For the online surveys, they normally tend to make
all the fields required property such they all have to be filled before proceeding to the next step.
Q5 Secondary Data
Paraphrase This Document
Need a fresh take? Get an instant paraphrase of this document with our AI Paraphraser
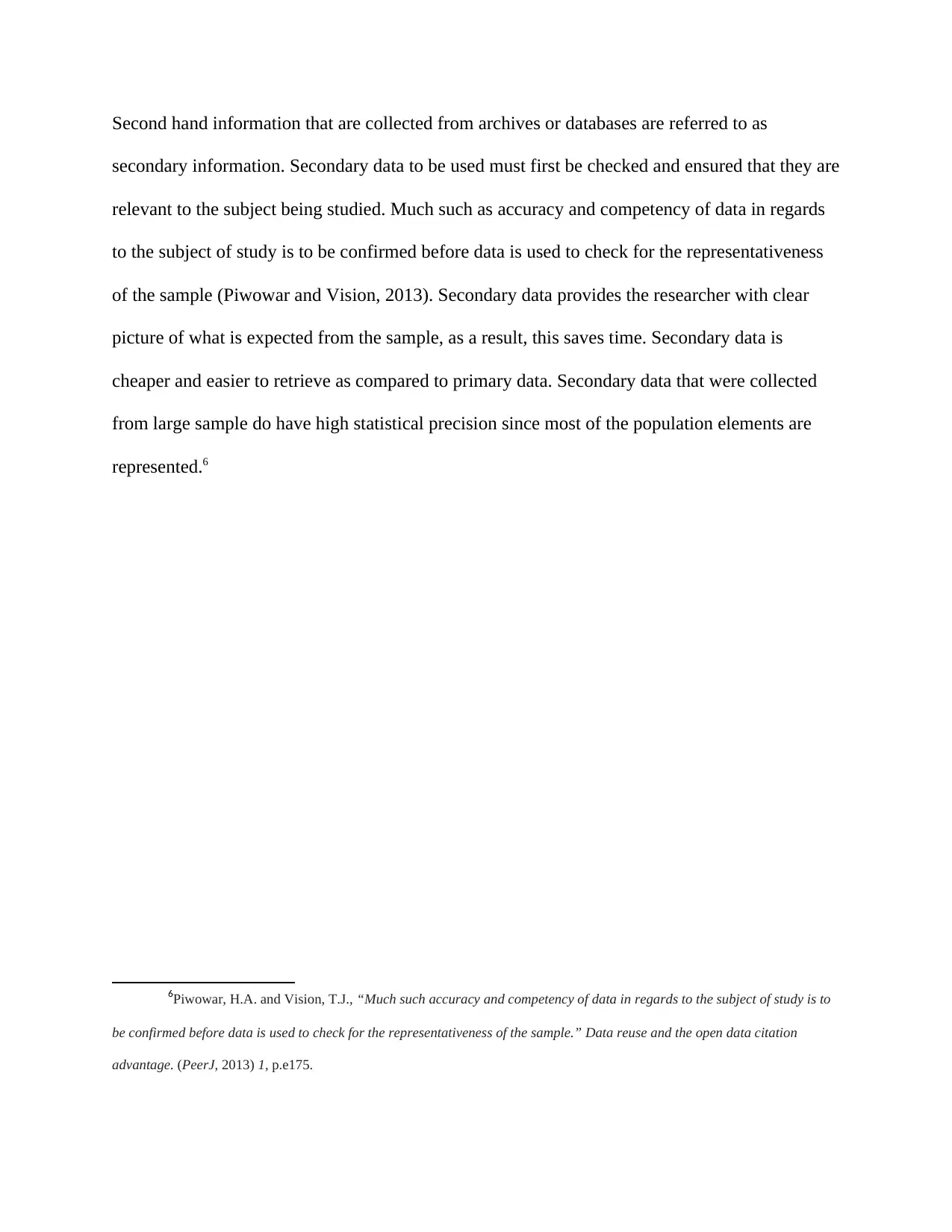
Second hand information that are collected from archives or databases are referred to as
secondary information. Secondary data to be used must first be checked and ensured that they are
relevant to the subject being studied. Much such as accuracy and competency of data in regards
to the subject of study is to be confirmed before data is used to check for the representativeness
of the sample (Piwowar and Vision, 2013). Secondary data provides the researcher with clear
picture of what is expected from the sample, as a result, this saves time. Secondary data is
cheaper and easier to retrieve as compared to primary data. Secondary data that were collected
from large sample do have high statistical precision since most of the population elements are
represented.6
6Piwowar, H.A. and Vision, T.J., “Much such accuracy and competency of data in regards to the subject of study is to
be confirmed before data is used to check for the representativeness of the sample.” Data reuse and the open data citation
advantage. (PeerJ, 2013) 1, p.e175.
secondary information. Secondary data to be used must first be checked and ensured that they are
relevant to the subject being studied. Much such as accuracy and competency of data in regards
to the subject of study is to be confirmed before data is used to check for the representativeness
of the sample (Piwowar and Vision, 2013). Secondary data provides the researcher with clear
picture of what is expected from the sample, as a result, this saves time. Secondary data is
cheaper and easier to retrieve as compared to primary data. Secondary data that were collected
from large sample do have high statistical precision since most of the population elements are
represented.6
6Piwowar, H.A. and Vision, T.J., “Much such accuracy and competency of data in regards to the subject of study is to
be confirmed before data is used to check for the representativeness of the sample.” Data reuse and the open data citation
advantage. (PeerJ, 2013) 1, p.e175.
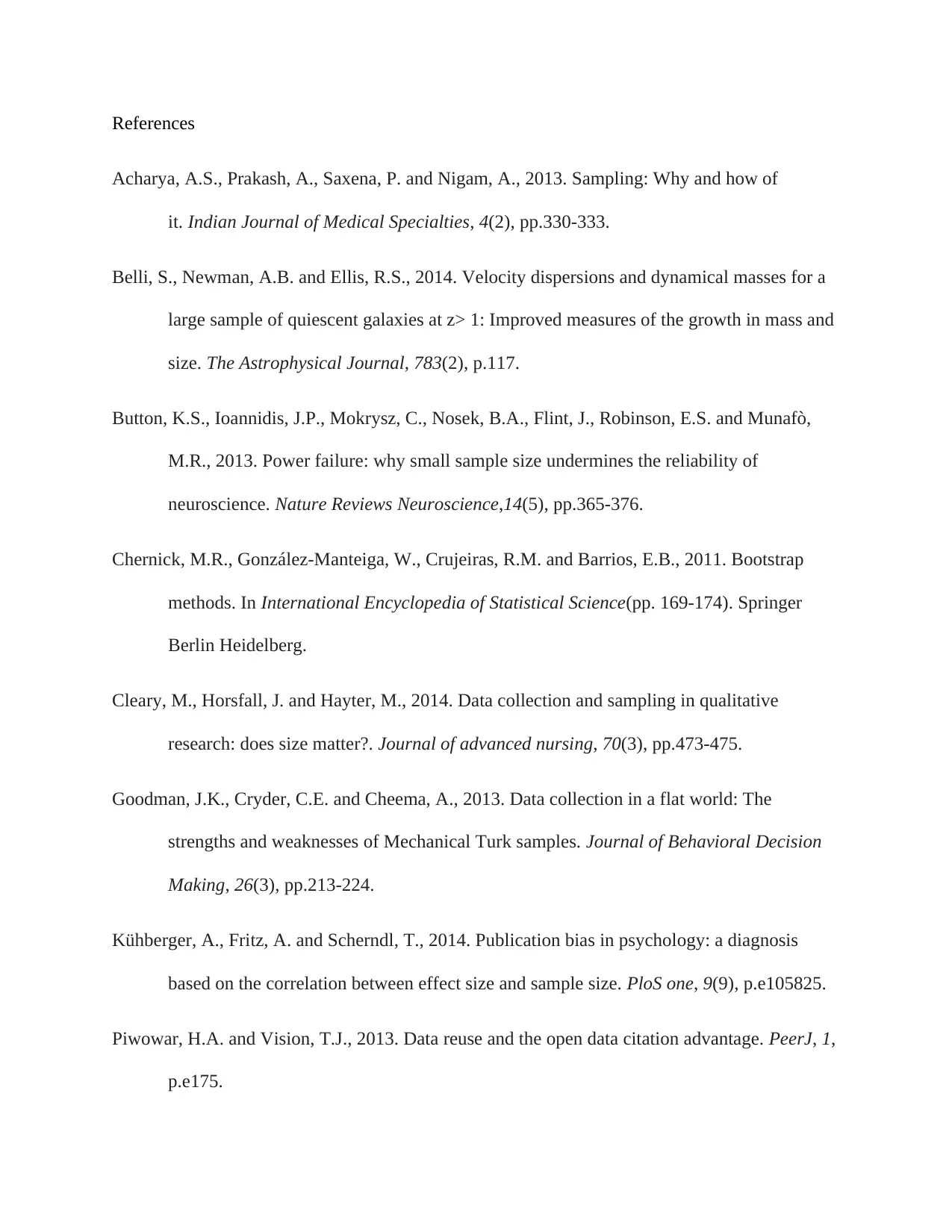
References
Acharya, A.S., Prakash, A., Saxena, P. and Nigam, A., 2013. Sampling: Why and how of
it. Indian Journal of Medical Specialties, 4(2), pp.330-333.
Belli, S., Newman, A.B. and Ellis, R.S., 2014. Velocity dispersions and dynamical masses for a
large sample of quiescent galaxies at z> 1: Improved measures of the growth in mass and
size. The Astrophysical Journal, 783(2), p.117.
Button, K.S., Ioannidis, J.P., Mokrysz, C., Nosek, B.A., Flint, J., Robinson, E.S. and Munafò,
M.R., 2013. Power failure: why small sample size undermines the reliability of
neuroscience. Nature Reviews Neuroscience,14(5), pp.365-376.
Chernick, M.R., González-Manteiga, W., Crujeiras, R.M. and Barrios, E.B., 2011. Bootstrap
methods. In International Encyclopedia of Statistical Science(pp. 169-174). Springer
Berlin Heidelberg.
Cleary, M., Horsfall, J. and Hayter, M., 2014. Data collection and sampling in qualitative
research: does size matter?. Journal of advanced nursing, 70(3), pp.473-475.
Goodman, J.K., Cryder, C.E. and Cheema, A., 2013. Data collection in a flat world: The
strengths and weaknesses of Mechanical Turk samples. Journal of Behavioral Decision
Making, 26(3), pp.213-224.
Kühberger, A., Fritz, A. and Scherndl, T., 2014. Publication bias in psychology: a diagnosis
based on the correlation between effect size and sample size. PloS one, 9(9), p.e105825.
Piwowar, H.A. and Vision, T.J., 2013. Data reuse and the open data citation advantage. PeerJ, 1,
p.e175.
Acharya, A.S., Prakash, A., Saxena, P. and Nigam, A., 2013. Sampling: Why and how of
it. Indian Journal of Medical Specialties, 4(2), pp.330-333.
Belli, S., Newman, A.B. and Ellis, R.S., 2014. Velocity dispersions and dynamical masses for a
large sample of quiescent galaxies at z> 1: Improved measures of the growth in mass and
size. The Astrophysical Journal, 783(2), p.117.
Button, K.S., Ioannidis, J.P., Mokrysz, C., Nosek, B.A., Flint, J., Robinson, E.S. and Munafò,
M.R., 2013. Power failure: why small sample size undermines the reliability of
neuroscience. Nature Reviews Neuroscience,14(5), pp.365-376.
Chernick, M.R., González-Manteiga, W., Crujeiras, R.M. and Barrios, E.B., 2011. Bootstrap
methods. In International Encyclopedia of Statistical Science(pp. 169-174). Springer
Berlin Heidelberg.
Cleary, M., Horsfall, J. and Hayter, M., 2014. Data collection and sampling in qualitative
research: does size matter?. Journal of advanced nursing, 70(3), pp.473-475.
Goodman, J.K., Cryder, C.E. and Cheema, A., 2013. Data collection in a flat world: The
strengths and weaknesses of Mechanical Turk samples. Journal of Behavioral Decision
Making, 26(3), pp.213-224.
Kühberger, A., Fritz, A. and Scherndl, T., 2014. Publication bias in psychology: a diagnosis
based on the correlation between effect size and sample size. PloS one, 9(9), p.e105825.
Piwowar, H.A. and Vision, T.J., 2013. Data reuse and the open data citation advantage. PeerJ, 1,
p.e175.
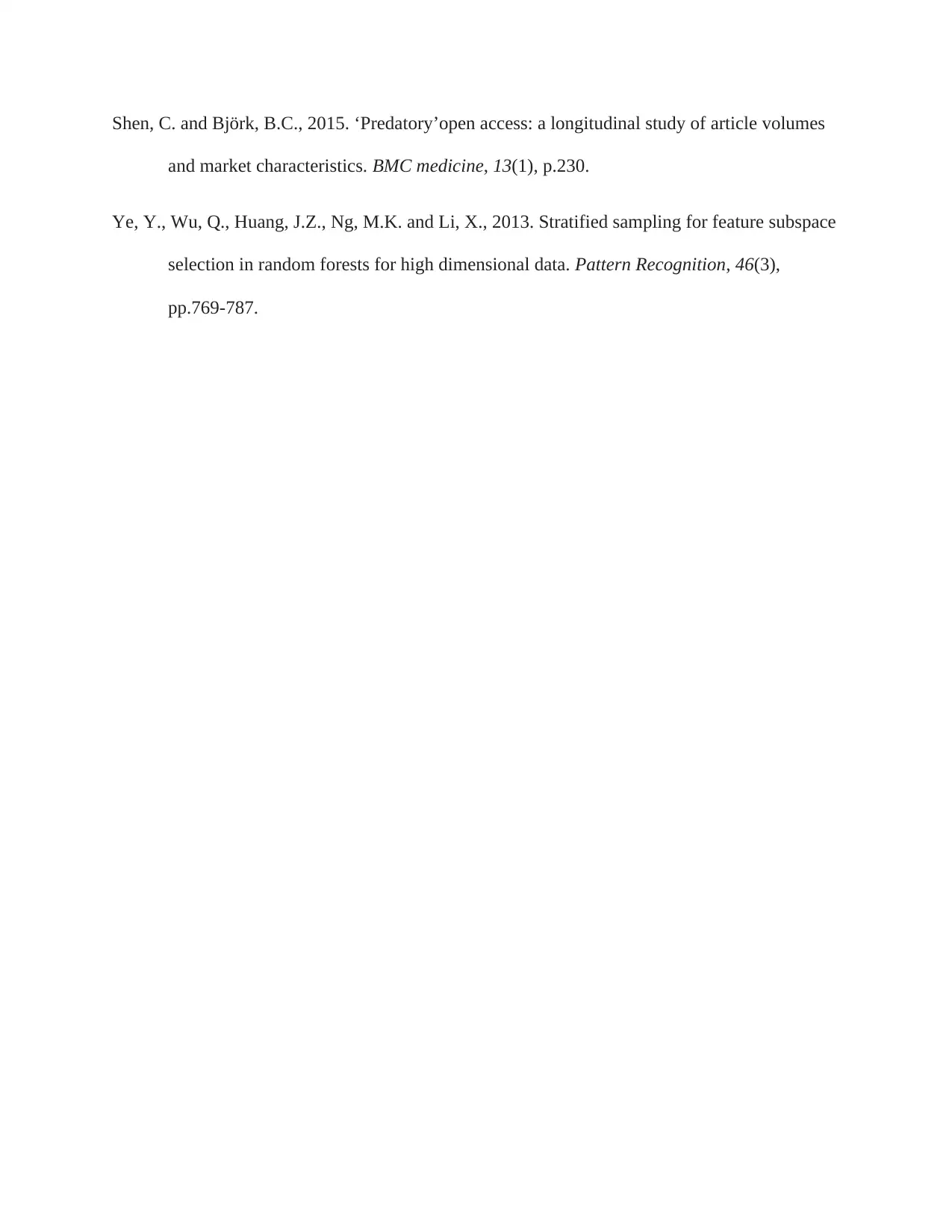
Shen, C. and Björk, B.C., 2015. ‘Predatory’open access: a longitudinal study of article volumes
and market characteristics. BMC medicine, 13(1), p.230.
Ye, Y., Wu, Q., Huang, J.Z., Ng, M.K. and Li, X., 2013. Stratified sampling for feature subspace
selection in random forests for high dimensional data. Pattern Recognition, 46(3),
pp.769-787.
and market characteristics. BMC medicine, 13(1), p.230.
Ye, Y., Wu, Q., Huang, J.Z., Ng, M.K. and Li, X., 2013. Stratified sampling for feature subspace
selection in random forests for high dimensional data. Pattern Recognition, 46(3),
pp.769-787.
1 out of 10
Related Documents
![[object Object]](/_next/image/?url=%2F_next%2Fstatic%2Fmedia%2Flogo.6d15ce61.png&w=640&q=75)
Your All-in-One AI-Powered Toolkit for Academic Success.
+13062052269
info@desklib.com
Available 24*7 on WhatsApp / Email
Unlock your academic potential
© 2024 | Zucol Services PVT LTD | All rights reserved.