Business Analysis
VerifiedAdded on 2023/03/30
|17
|3107
|302
AI Summary
This document provides an analysis of a business insight scenario, including its overall features, key information derived from the insights report, and suggestions for improvements. It also discusses regression and correlation analysis, effective applications of regression analysis, and the equation of the regression line. Additionally, it explains the difference between classification and prediction, methods of classification, and decision trees.
Contribute Materials
Your contribution can guide someone’s learning journey. Share your
documents today.
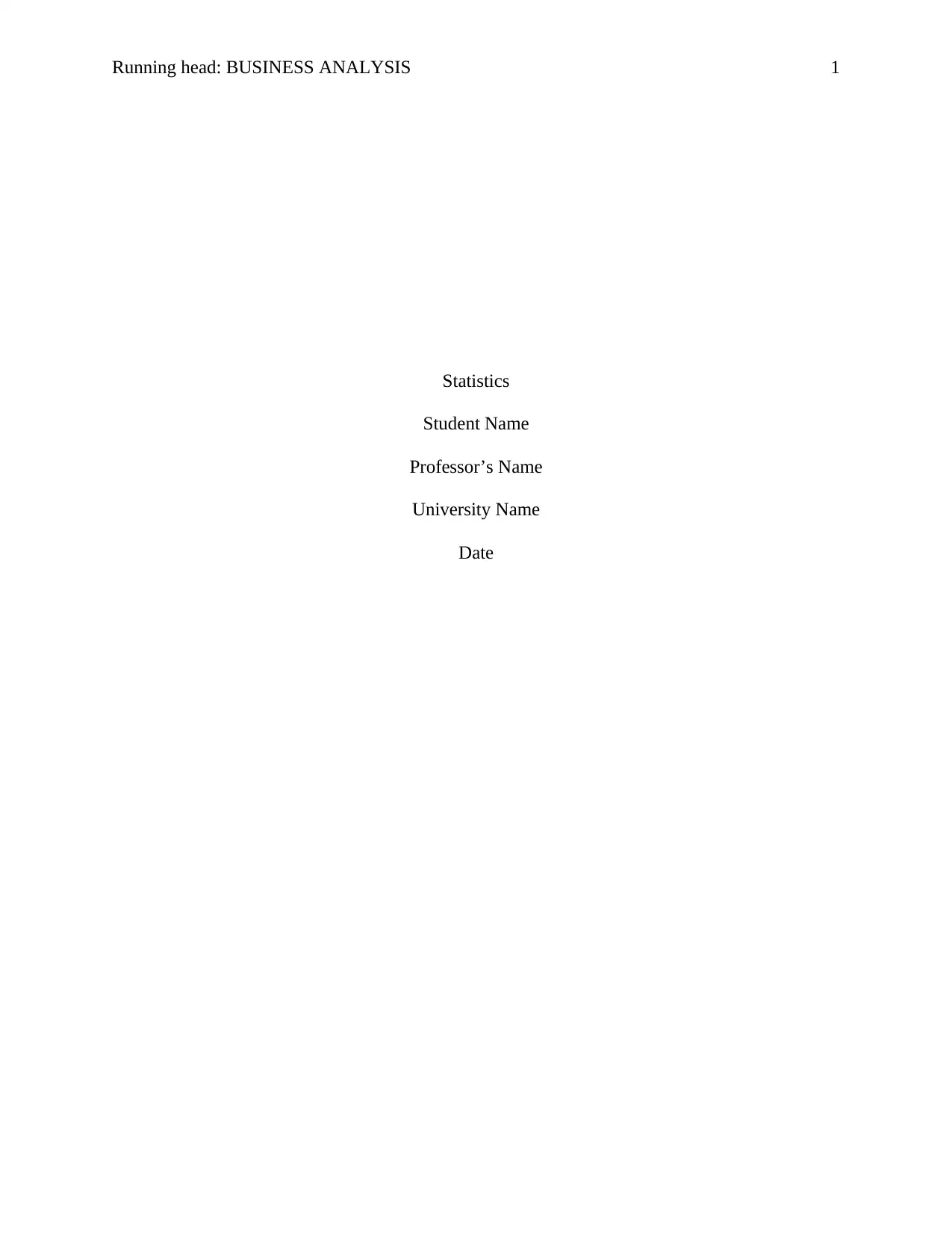
Running head: BUSINESS ANALYSIS 1
Statistics
Student Name
Professor’s Name
University Name
Date
Statistics
Student Name
Professor’s Name
University Name
Date
Secure Best Marks with AI Grader
Need help grading? Try our AI Grader for instant feedback on your assignments.
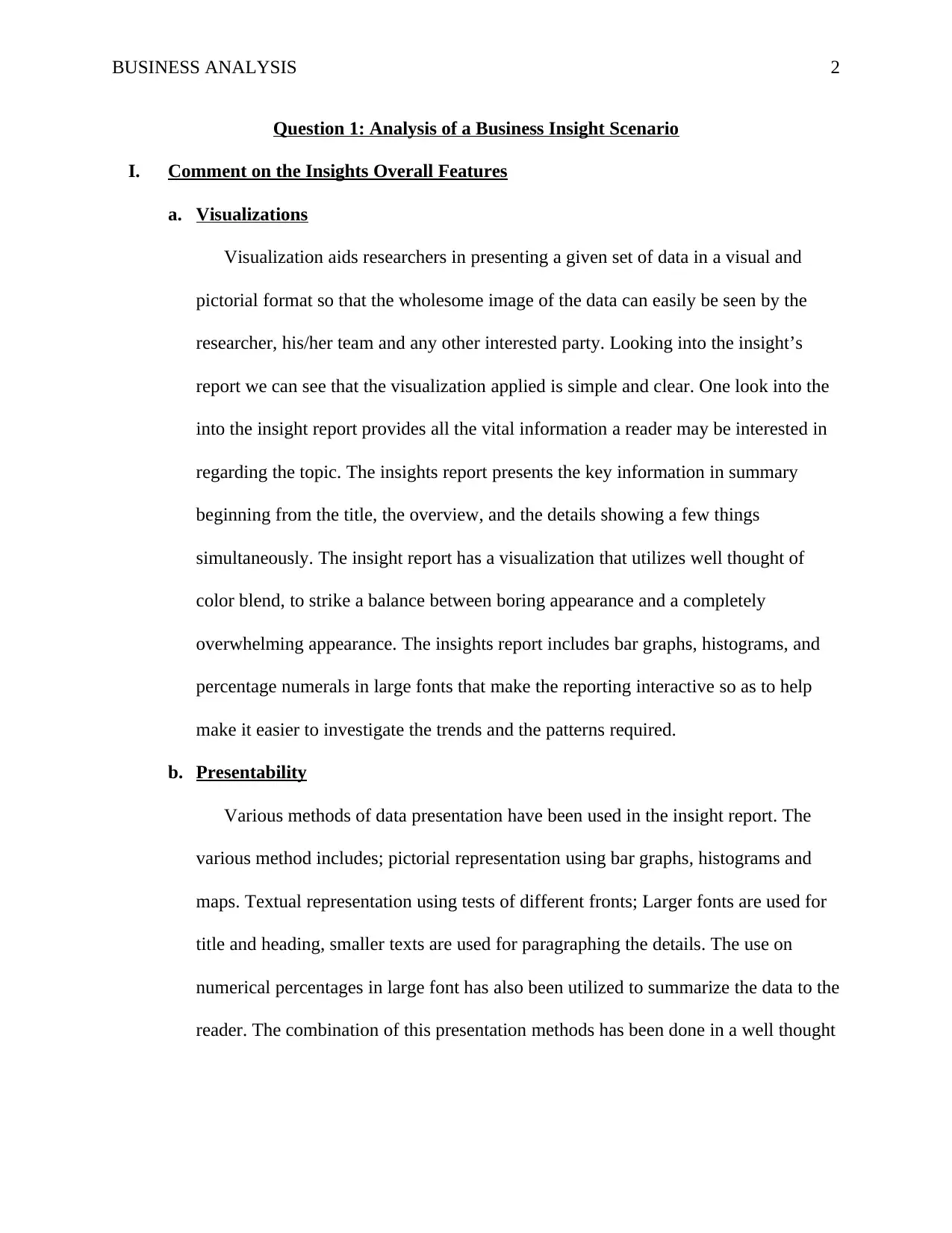
BUSINESS ANALYSIS 2
Question 1: Analysis of a Business Insight Scenario
I. Comment on the Insights Overall Features
a. Visualizations
Visualization aids researchers in presenting a given set of data in a visual and
pictorial format so that the wholesome image of the data can easily be seen by the
researcher, his/her team and any other interested party. Looking into the insight’s
report we can see that the visualization applied is simple and clear. One look into the
into the insight report provides all the vital information a reader may be interested in
regarding the topic. The insights report presents the key information in summary
beginning from the title, the overview, and the details showing a few things
simultaneously. The insight report has a visualization that utilizes well thought of
color blend, to strike a balance between boring appearance and a completely
overwhelming appearance. The insights report includes bar graphs, histograms, and
percentage numerals in large fonts that make the reporting interactive so as to help
make it easier to investigate the trends and the patterns required.
b. Presentability
Various methods of data presentation have been used in the insight report. The
various method includes; pictorial representation using bar graphs, histograms and
maps. Textual representation using tests of different fronts; Larger fonts are used for
title and heading, smaller texts are used for paragraphing the details. The use on
numerical percentages in large font has also been utilized to summarize the data to the
reader. The combination of this presentation methods has been done in a well thought
Question 1: Analysis of a Business Insight Scenario
I. Comment on the Insights Overall Features
a. Visualizations
Visualization aids researchers in presenting a given set of data in a visual and
pictorial format so that the wholesome image of the data can easily be seen by the
researcher, his/her team and any other interested party. Looking into the insight’s
report we can see that the visualization applied is simple and clear. One look into the
into the insight report provides all the vital information a reader may be interested in
regarding the topic. The insights report presents the key information in summary
beginning from the title, the overview, and the details showing a few things
simultaneously. The insight report has a visualization that utilizes well thought of
color blend, to strike a balance between boring appearance and a completely
overwhelming appearance. The insights report includes bar graphs, histograms, and
percentage numerals in large fonts that make the reporting interactive so as to help
make it easier to investigate the trends and the patterns required.
b. Presentability
Various methods of data presentation have been used in the insight report. The
various method includes; pictorial representation using bar graphs, histograms and
maps. Textual representation using tests of different fronts; Larger fonts are used for
title and heading, smaller texts are used for paragraphing the details. The use on
numerical percentages in large font has also been utilized to summarize the data to the
reader. The combination of this presentation methods has been done in a well thought
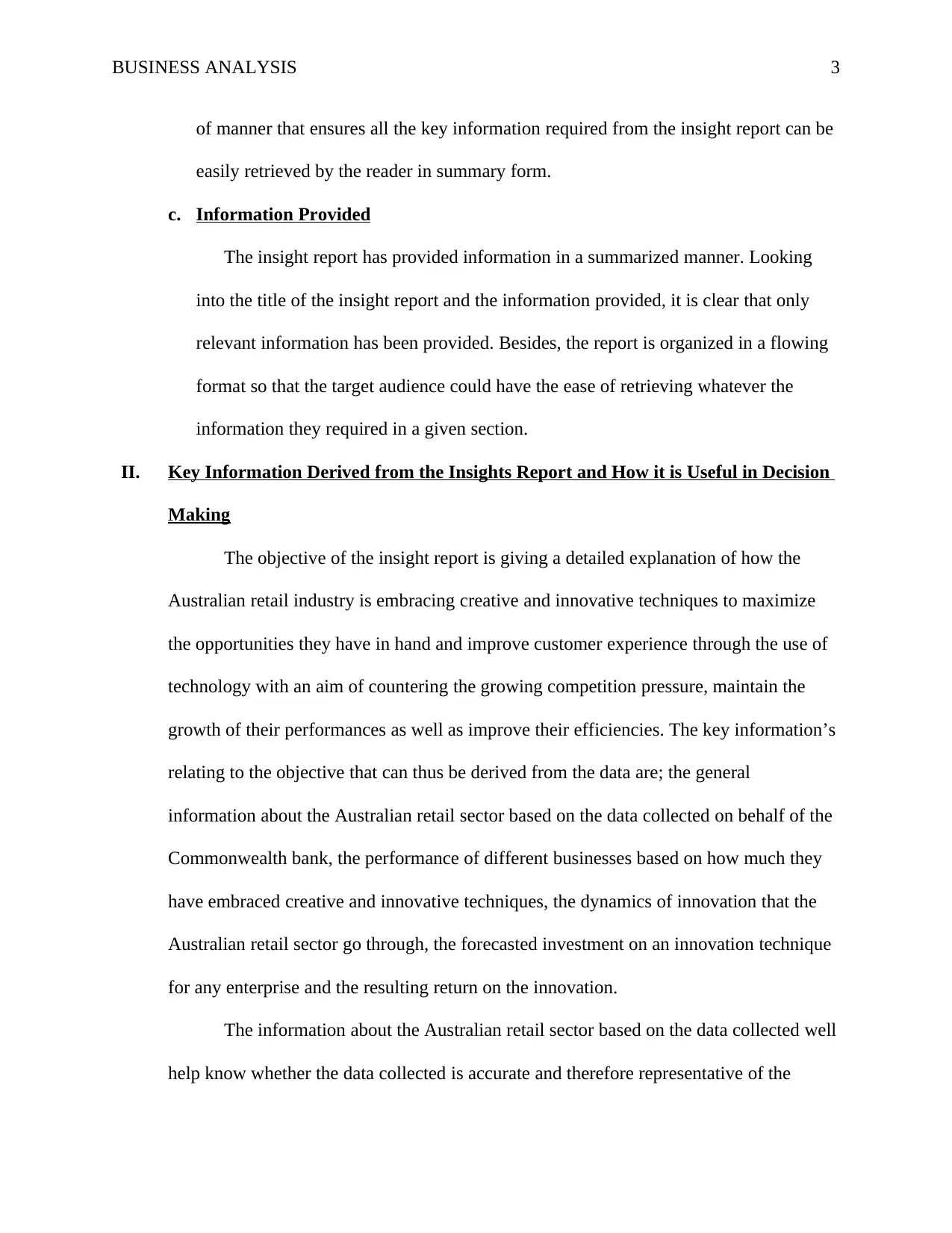
BUSINESS ANALYSIS 3
of manner that ensures all the key information required from the insight report can be
easily retrieved by the reader in summary form.
c. Information Provided
The insight report has provided information in a summarized manner. Looking
into the title of the insight report and the information provided, it is clear that only
relevant information has been provided. Besides, the report is organized in a flowing
format so that the target audience could have the ease of retrieving whatever the
information they required in a given section.
II. Key Information Derived from the Insights Report and How it is Useful in Decision
Making
The objective of the insight report is giving a detailed explanation of how the
Australian retail industry is embracing creative and innovative techniques to maximize
the opportunities they have in hand and improve customer experience through the use of
technology with an aim of countering the growing competition pressure, maintain the
growth of their performances as well as improve their efficiencies. The key information’s
relating to the objective that can thus be derived from the data are; the general
information about the Australian retail sector based on the data collected on behalf of the
Commonwealth bank, the performance of different businesses based on how much they
have embraced creative and innovative techniques, the dynamics of innovation that the
Australian retail sector go through, the forecasted investment on an innovation technique
for any enterprise and the resulting return on the innovation.
The information about the Australian retail sector based on the data collected well
help know whether the data collected is accurate and therefore representative of the
of manner that ensures all the key information required from the insight report can be
easily retrieved by the reader in summary form.
c. Information Provided
The insight report has provided information in a summarized manner. Looking
into the title of the insight report and the information provided, it is clear that only
relevant information has been provided. Besides, the report is organized in a flowing
format so that the target audience could have the ease of retrieving whatever the
information they required in a given section.
II. Key Information Derived from the Insights Report and How it is Useful in Decision
Making
The objective of the insight report is giving a detailed explanation of how the
Australian retail industry is embracing creative and innovative techniques to maximize
the opportunities they have in hand and improve customer experience through the use of
technology with an aim of countering the growing competition pressure, maintain the
growth of their performances as well as improve their efficiencies. The key information’s
relating to the objective that can thus be derived from the data are; the general
information about the Australian retail sector based on the data collected on behalf of the
Commonwealth bank, the performance of different businesses based on how much they
have embraced creative and innovative techniques, the dynamics of innovation that the
Australian retail sector go through, the forecasted investment on an innovation technique
for any enterprise and the resulting return on the innovation.
The information about the Australian retail sector based on the data collected well
help know whether the data collected is accurate and therefore representative of the
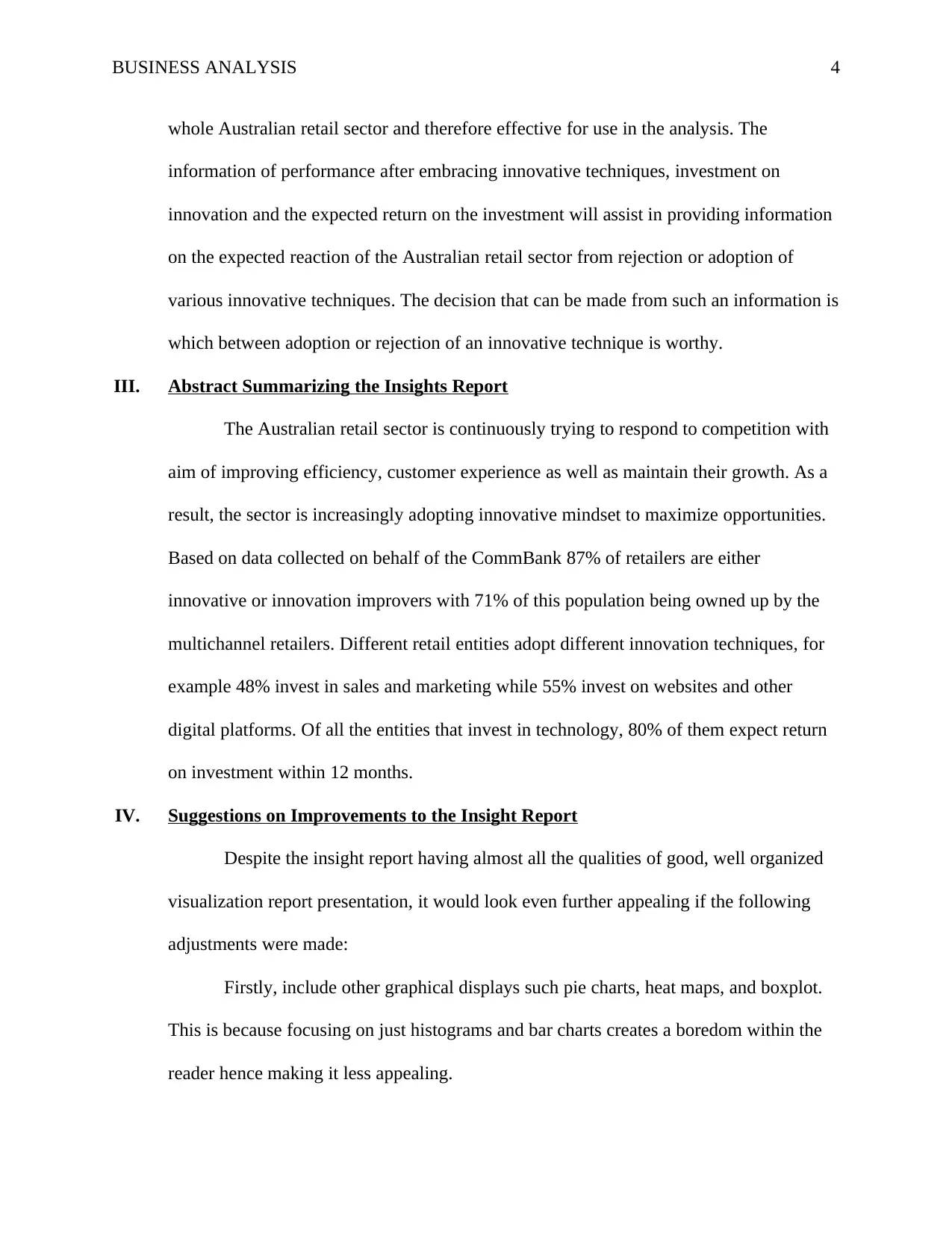
BUSINESS ANALYSIS 4
whole Australian retail sector and therefore effective for use in the analysis. The
information of performance after embracing innovative techniques, investment on
innovation and the expected return on the investment will assist in providing information
on the expected reaction of the Australian retail sector from rejection or adoption of
various innovative techniques. The decision that can be made from such an information is
which between adoption or rejection of an innovative technique is worthy.
III. Abstract Summarizing the Insights Report
The Australian retail sector is continuously trying to respond to competition with
aim of improving efficiency, customer experience as well as maintain their growth. As a
result, the sector is increasingly adopting innovative mindset to maximize opportunities.
Based on data collected on behalf of the CommBank 87% of retailers are either
innovative or innovation improvers with 71% of this population being owned up by the
multichannel retailers. Different retail entities adopt different innovation techniques, for
example 48% invest in sales and marketing while 55% invest on websites and other
digital platforms. Of all the entities that invest in technology, 80% of them expect return
on investment within 12 months.
IV. Suggestions on Improvements to the Insight Report
Despite the insight report having almost all the qualities of good, well organized
visualization report presentation, it would look even further appealing if the following
adjustments were made:
Firstly, include other graphical displays such pie charts, heat maps, and boxplot.
This is because focusing on just histograms and bar charts creates a boredom within the
reader hence making it less appealing.
whole Australian retail sector and therefore effective for use in the analysis. The
information of performance after embracing innovative techniques, investment on
innovation and the expected return on the investment will assist in providing information
on the expected reaction of the Australian retail sector from rejection or adoption of
various innovative techniques. The decision that can be made from such an information is
which between adoption or rejection of an innovative technique is worthy.
III. Abstract Summarizing the Insights Report
The Australian retail sector is continuously trying to respond to competition with
aim of improving efficiency, customer experience as well as maintain their growth. As a
result, the sector is increasingly adopting innovative mindset to maximize opportunities.
Based on data collected on behalf of the CommBank 87% of retailers are either
innovative or innovation improvers with 71% of this population being owned up by the
multichannel retailers. Different retail entities adopt different innovation techniques, for
example 48% invest in sales and marketing while 55% invest on websites and other
digital platforms. Of all the entities that invest in technology, 80% of them expect return
on investment within 12 months.
IV. Suggestions on Improvements to the Insight Report
Despite the insight report having almost all the qualities of good, well organized
visualization report presentation, it would look even further appealing if the following
adjustments were made:
Firstly, include other graphical displays such pie charts, heat maps, and boxplot.
This is because focusing on just histograms and bar charts creates a boredom within the
reader hence making it less appealing.
Secure Best Marks with AI Grader
Need help grading? Try our AI Grader for instant feedback on your assignments.
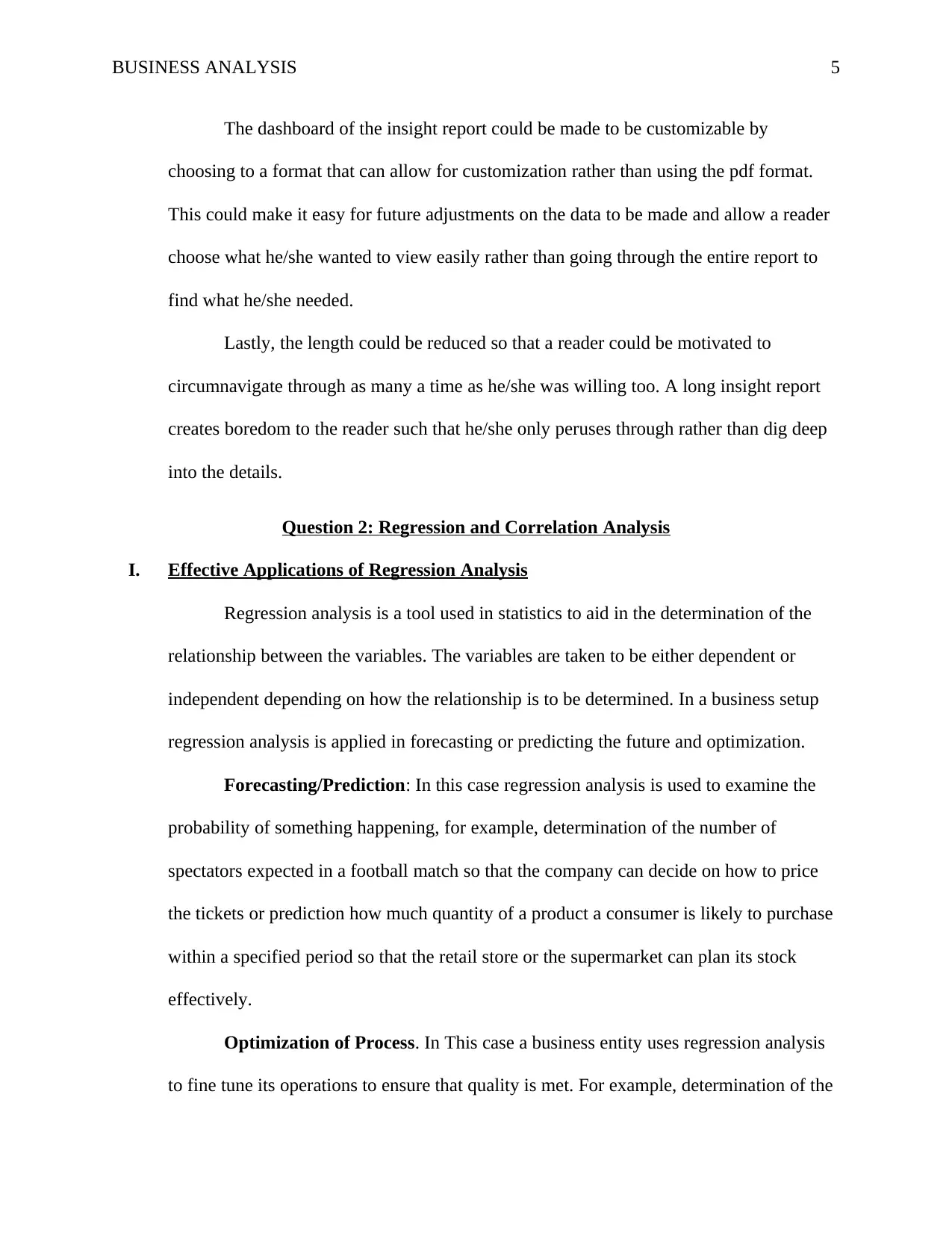
BUSINESS ANALYSIS 5
The dashboard of the insight report could be made to be customizable by
choosing to a format that can allow for customization rather than using the pdf format.
This could make it easy for future adjustments on the data to be made and allow a reader
choose what he/she wanted to view easily rather than going through the entire report to
find what he/she needed.
Lastly, the length could be reduced so that a reader could be motivated to
circumnavigate through as many a time as he/she was willing too. A long insight report
creates boredom to the reader such that he/she only peruses through rather than dig deep
into the details.
Question 2: Regression and Correlation Analysis
I. Effective Applications of Regression Analysis
Regression analysis is a tool used in statistics to aid in the determination of the
relationship between the variables. The variables are taken to be either dependent or
independent depending on how the relationship is to be determined. In a business setup
regression analysis is applied in forecasting or predicting the future and optimization.
Forecasting/Prediction: In this case regression analysis is used to examine the
probability of something happening, for example, determination of the number of
spectators expected in a football match so that the company can decide on how to price
the tickets or prediction how much quantity of a product a consumer is likely to purchase
within a specified period so that the retail store or the supermarket can plan its stock
effectively.
Optimization of Process. In This case a business entity uses regression analysis
to fine tune its operations to ensure that quality is met. For example, determination of the
The dashboard of the insight report could be made to be customizable by
choosing to a format that can allow for customization rather than using the pdf format.
This could make it easy for future adjustments on the data to be made and allow a reader
choose what he/she wanted to view easily rather than going through the entire report to
find what he/she needed.
Lastly, the length could be reduced so that a reader could be motivated to
circumnavigate through as many a time as he/she was willing too. A long insight report
creates boredom to the reader such that he/she only peruses through rather than dig deep
into the details.
Question 2: Regression and Correlation Analysis
I. Effective Applications of Regression Analysis
Regression analysis is a tool used in statistics to aid in the determination of the
relationship between the variables. The variables are taken to be either dependent or
independent depending on how the relationship is to be determined. In a business setup
regression analysis is applied in forecasting or predicting the future and optimization.
Forecasting/Prediction: In this case regression analysis is used to examine the
probability of something happening, for example, determination of the number of
spectators expected in a football match so that the company can decide on how to price
the tickets or prediction how much quantity of a product a consumer is likely to purchase
within a specified period so that the retail store or the supermarket can plan its stock
effectively.
Optimization of Process. In This case a business entity uses regression analysis
to fine tune its operations to ensure that quality is met. For example, determination of the
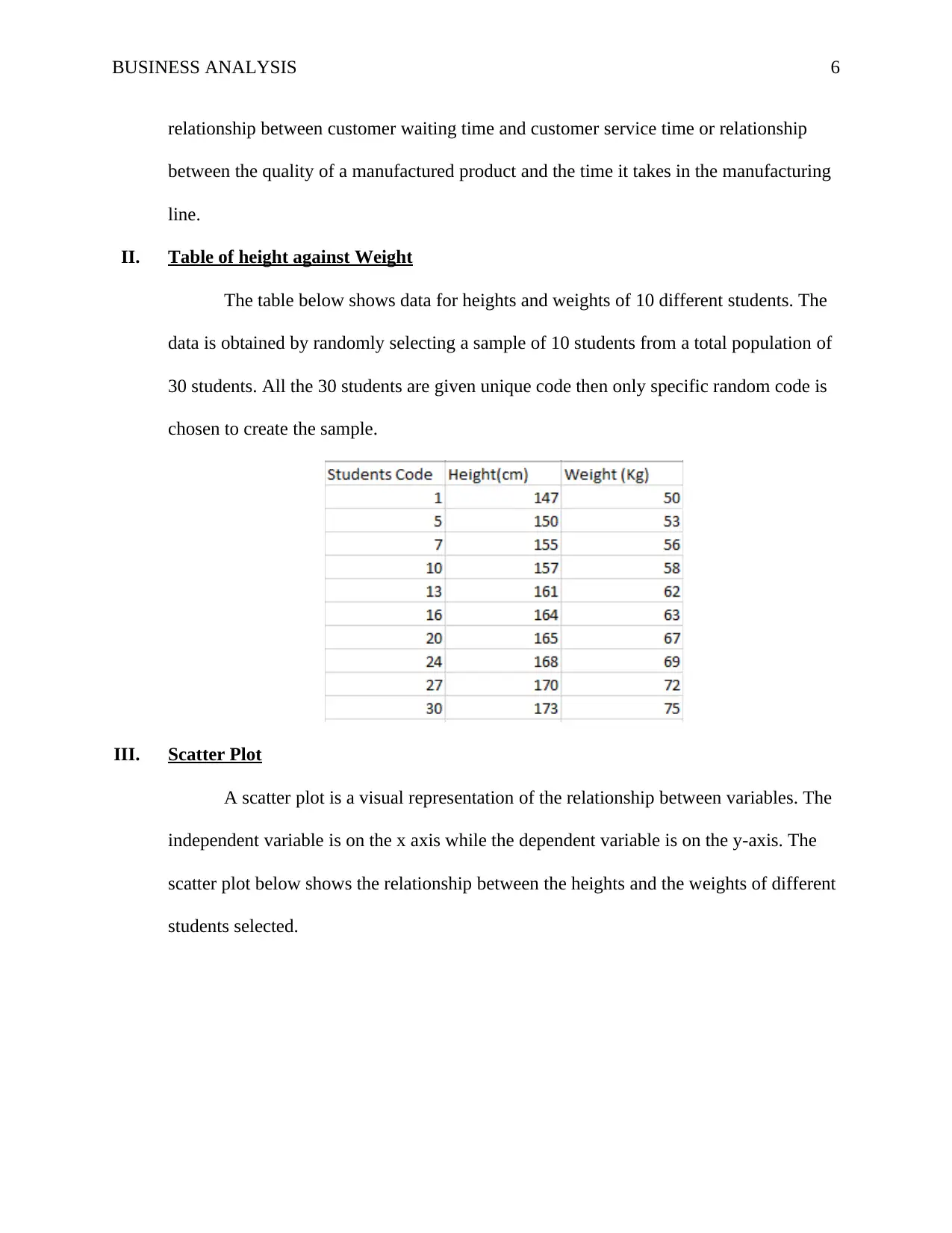
BUSINESS ANALYSIS 6
relationship between customer waiting time and customer service time or relationship
between the quality of a manufactured product and the time it takes in the manufacturing
line.
II. Table of height against Weight
The table below shows data for heights and weights of 10 different students. The
data is obtained by randomly selecting a sample of 10 students from a total population of
30 students. All the 30 students are given unique code then only specific random code is
chosen to create the sample.
III. Scatter Plot
A scatter plot is a visual representation of the relationship between variables. The
independent variable is on the x axis while the dependent variable is on the y-axis. The
scatter plot below shows the relationship between the heights and the weights of different
students selected.
relationship between customer waiting time and customer service time or relationship
between the quality of a manufactured product and the time it takes in the manufacturing
line.
II. Table of height against Weight
The table below shows data for heights and weights of 10 different students. The
data is obtained by randomly selecting a sample of 10 students from a total population of
30 students. All the 30 students are given unique code then only specific random code is
chosen to create the sample.
III. Scatter Plot
A scatter plot is a visual representation of the relationship between variables. The
independent variable is on the x axis while the dependent variable is on the y-axis. The
scatter plot below shows the relationship between the heights and the weights of different
students selected.
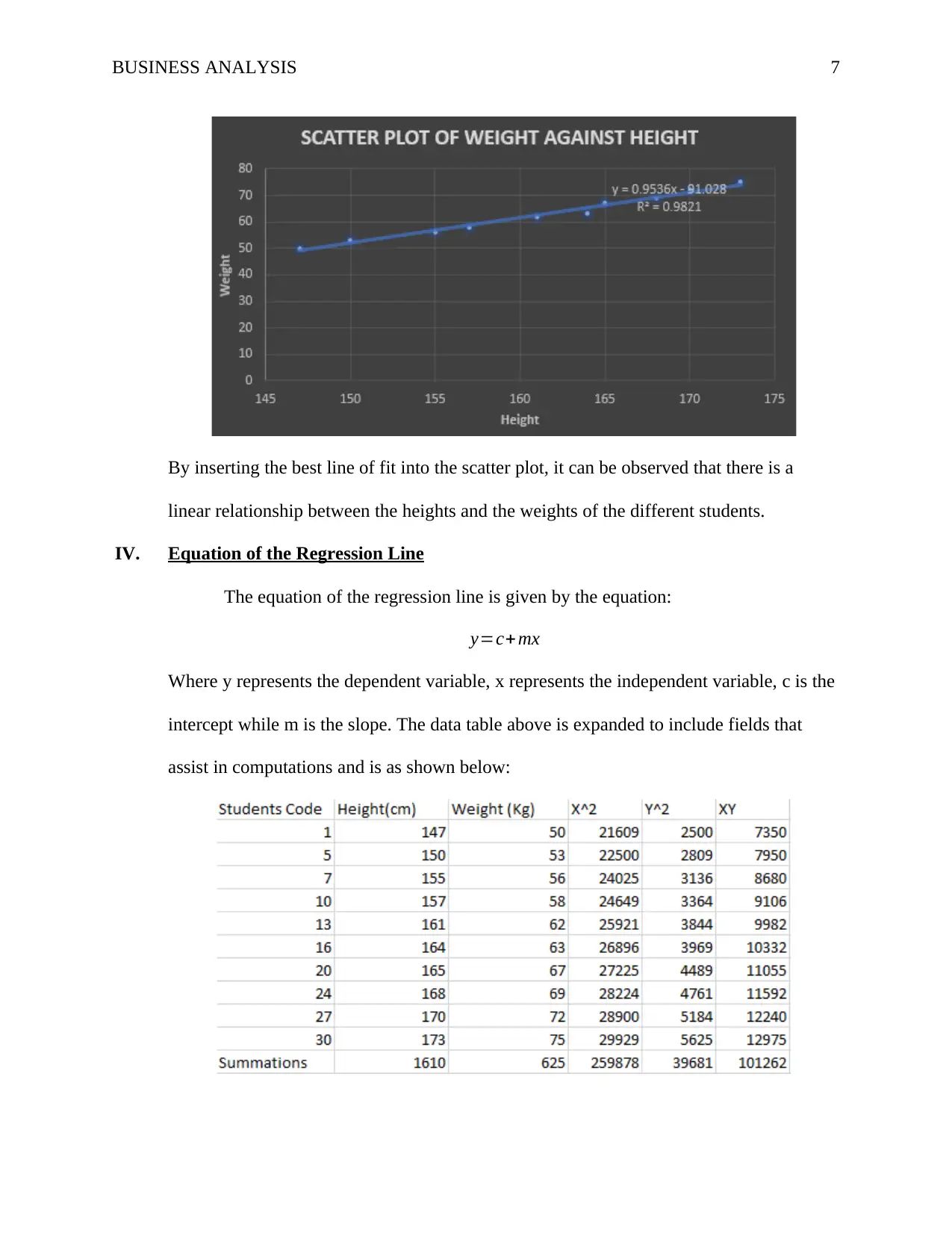
BUSINESS ANALYSIS 7
By inserting the best line of fit into the scatter plot, it can be observed that there is a
linear relationship between the heights and the weights of the different students.
IV. Equation of the Regression Line
The equation of the regression line is given by the equation:
y=c+ mx
Where y represents the dependent variable, x represents the independent variable, c is the
intercept while m is the slope. The data table above is expanded to include fields that
assist in computations and is as shown below:
By inserting the best line of fit into the scatter plot, it can be observed that there is a
linear relationship between the heights and the weights of the different students.
IV. Equation of the Regression Line
The equation of the regression line is given by the equation:
y=c+ mx
Where y represents the dependent variable, x represents the independent variable, c is the
intercept while m is the slope. The data table above is expanded to include fields that
assist in computations and is as shown below:
Paraphrase This Document
Need a fresh take? Get an instant paraphrase of this document with our AI Paraphraser
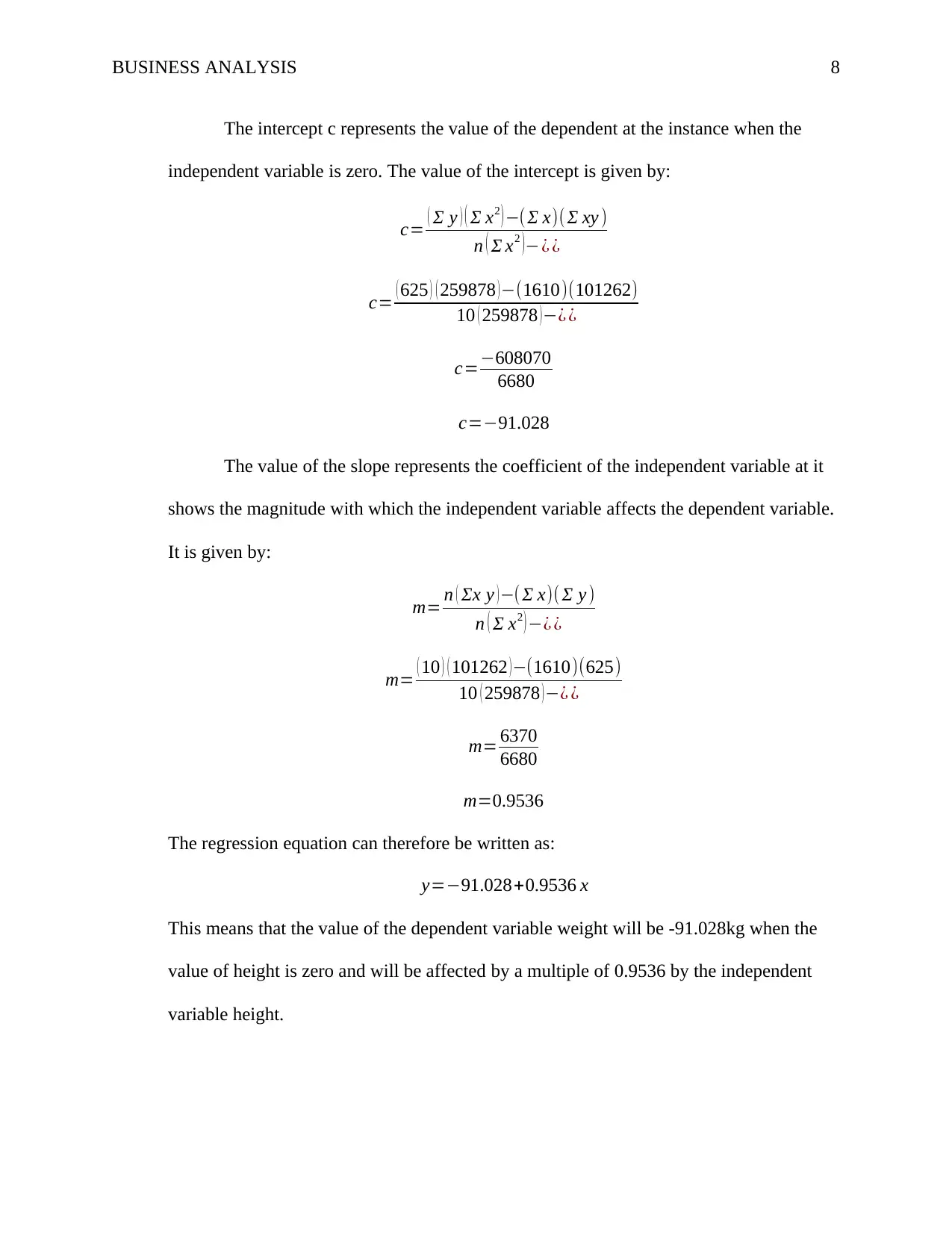
BUSINESS ANALYSIS 8
The intercept c represents the value of the dependent at the instance when the
independent variable is zero. The value of the intercept is given by:
c= ( Σ y ) ( Σ x2 ) −( Σ x)( Σ xy )
n ( Σ x2 )−¿ ¿
c= ( 625 ) ( 259878 ) −(1610)(101262)
10 ( 259878 ) −¿ ¿
c=−608070
6680
c=−91.028
The value of the slope represents the coefficient of the independent variable at it
shows the magnitude with which the independent variable affects the dependent variable.
It is given by:
m= n ( Σx y ) −( Σ x)( Σ y )
n ( Σ x2 ) −¿ ¿
m= ( 10 ) ( 101262 ) −(1610)(625)
10 ( 259878 ) −¿ ¿
m= 6370
6680
m=0.9536
The regression equation can therefore be written as:
y=−91.028+0.9536 x
This means that the value of the dependent variable weight will be -91.028kg when the
value of height is zero and will be affected by a multiple of 0.9536 by the independent
variable height.
The intercept c represents the value of the dependent at the instance when the
independent variable is zero. The value of the intercept is given by:
c= ( Σ y ) ( Σ x2 ) −( Σ x)( Σ xy )
n ( Σ x2 )−¿ ¿
c= ( 625 ) ( 259878 ) −(1610)(101262)
10 ( 259878 ) −¿ ¿
c=−608070
6680
c=−91.028
The value of the slope represents the coefficient of the independent variable at it
shows the magnitude with which the independent variable affects the dependent variable.
It is given by:
m= n ( Σx y ) −( Σ x)( Σ y )
n ( Σ x2 ) −¿ ¿
m= ( 10 ) ( 101262 ) −(1610)(625)
10 ( 259878 ) −¿ ¿
m= 6370
6680
m=0.9536
The regression equation can therefore be written as:
y=−91.028+0.9536 x
This means that the value of the dependent variable weight will be -91.028kg when the
value of height is zero and will be affected by a multiple of 0.9536 by the independent
variable height.
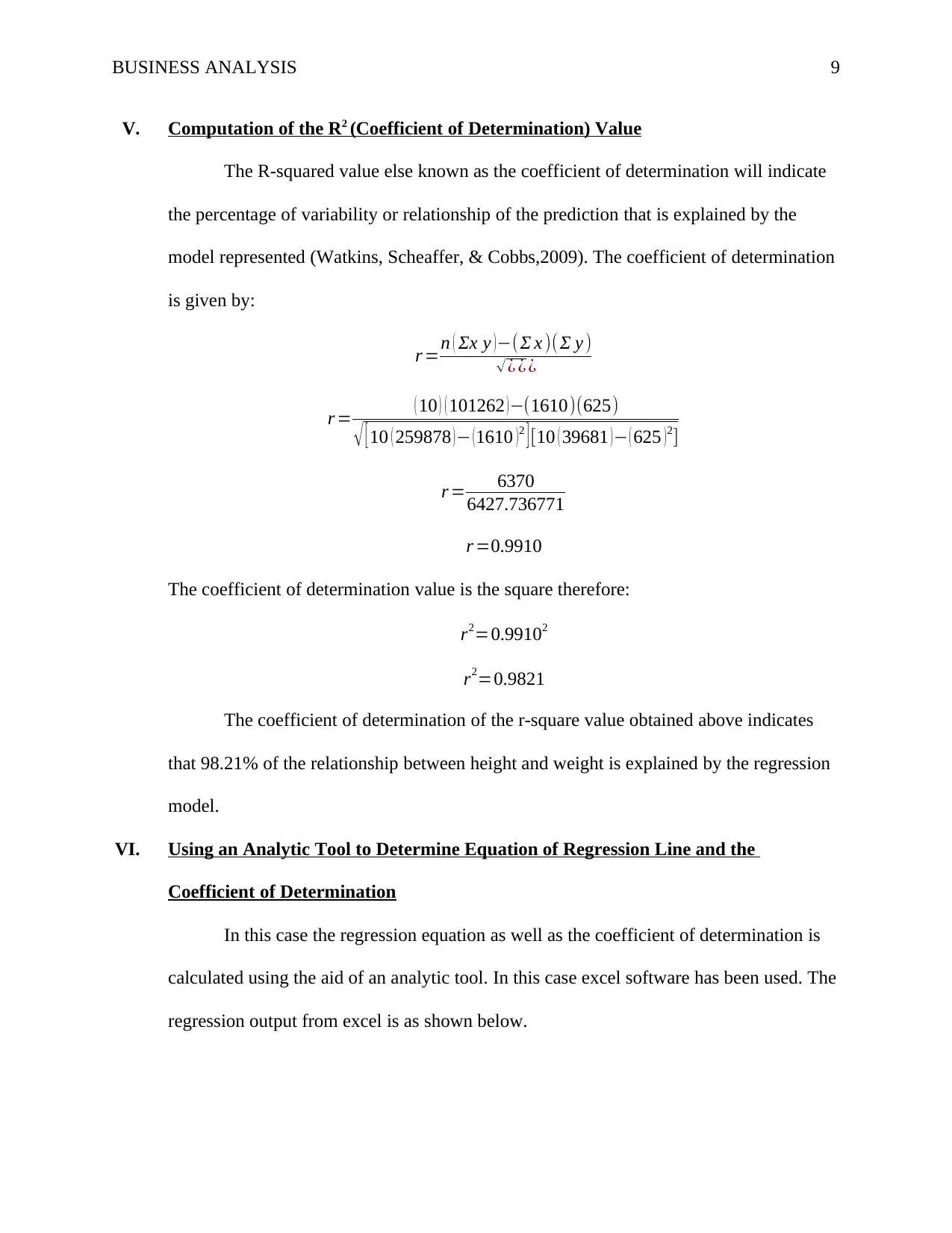
BUSINESS ANALYSIS 9
V. Computation of the R2 (Coefficient of Determination) Value
The R-squared value else known as the coefficient of determination will indicate
the percentage of variability or relationship of the prediction that is explained by the
model represented (Watkins, Scheaffer, & Cobbs,2009). The coefficient of determination
is given by:
r =n ( Σx y )−(Σ x )(Σ y )
√¿ ¿ ¿
r = ( 10 ) ( 101262 )−(1610)(625)
√ [ 10 ( 259878 )− (1610 )2 ] [10 ( 39681 )− ( 625 )2]
r = 6370
6427.736771
r =0.9910
The coefficient of determination value is the square therefore:
r2=0.99102
r2=0.9821
The coefficient of determination of the r-square value obtained above indicates
that 98.21% of the relationship between height and weight is explained by the regression
model.
VI. Using an Analytic Tool to Determine Equation of Regression Line and the
Coefficient of Determination
In this case the regression equation as well as the coefficient of determination is
calculated using the aid of an analytic tool. In this case excel software has been used. The
regression output from excel is as shown below.
V. Computation of the R2 (Coefficient of Determination) Value
The R-squared value else known as the coefficient of determination will indicate
the percentage of variability or relationship of the prediction that is explained by the
model represented (Watkins, Scheaffer, & Cobbs,2009). The coefficient of determination
is given by:
r =n ( Σx y )−(Σ x )(Σ y )
√¿ ¿ ¿
r = ( 10 ) ( 101262 )−(1610)(625)
√ [ 10 ( 259878 )− (1610 )2 ] [10 ( 39681 )− ( 625 )2]
r = 6370
6427.736771
r =0.9910
The coefficient of determination value is the square therefore:
r2=0.99102
r2=0.9821
The coefficient of determination of the r-square value obtained above indicates
that 98.21% of the relationship between height and weight is explained by the regression
model.
VI. Using an Analytic Tool to Determine Equation of Regression Line and the
Coefficient of Determination
In this case the regression equation as well as the coefficient of determination is
calculated using the aid of an analytic tool. In this case excel software has been used. The
regression output from excel is as shown below.

BUSINESS ANALYSIS 10
The regression equation in this case can be written as:
y=−91.028+0.9536 x
The value of the coefficient of determination is 0.9821. It is evident that the values
obtained manually are similar to the values determined using the excel analytic tool.
Question 3: Classification and Clustering
I. Difference Between Classification and Prediction
Both classification and prediction are techniques used in statistics for data
analysis. The utilized in scenarios that involve retrieving are extracting models that can
be used to determine and explain various important classes of data. Additionally,
prediction and classification are used in statistic to predict the forthcomings and forecast
the expected patterns from a given set of data (Shao,2010). However, classification and
prediction are distinct in the following ways:
The regression equation in this case can be written as:
y=−91.028+0.9536 x
The value of the coefficient of determination is 0.9821. It is evident that the values
obtained manually are similar to the values determined using the excel analytic tool.
Question 3: Classification and Clustering
I. Difference Between Classification and Prediction
Both classification and prediction are techniques used in statistics for data
analysis. The utilized in scenarios that involve retrieving are extracting models that can
be used to determine and explain various important classes of data. Additionally,
prediction and classification are used in statistic to predict the forthcomings and forecast
the expected patterns from a given set of data (Shao,2010). However, classification and
prediction are distinct in the following ways:
Secure Best Marks with AI Grader
Need help grading? Try our AI Grader for instant feedback on your assignments.
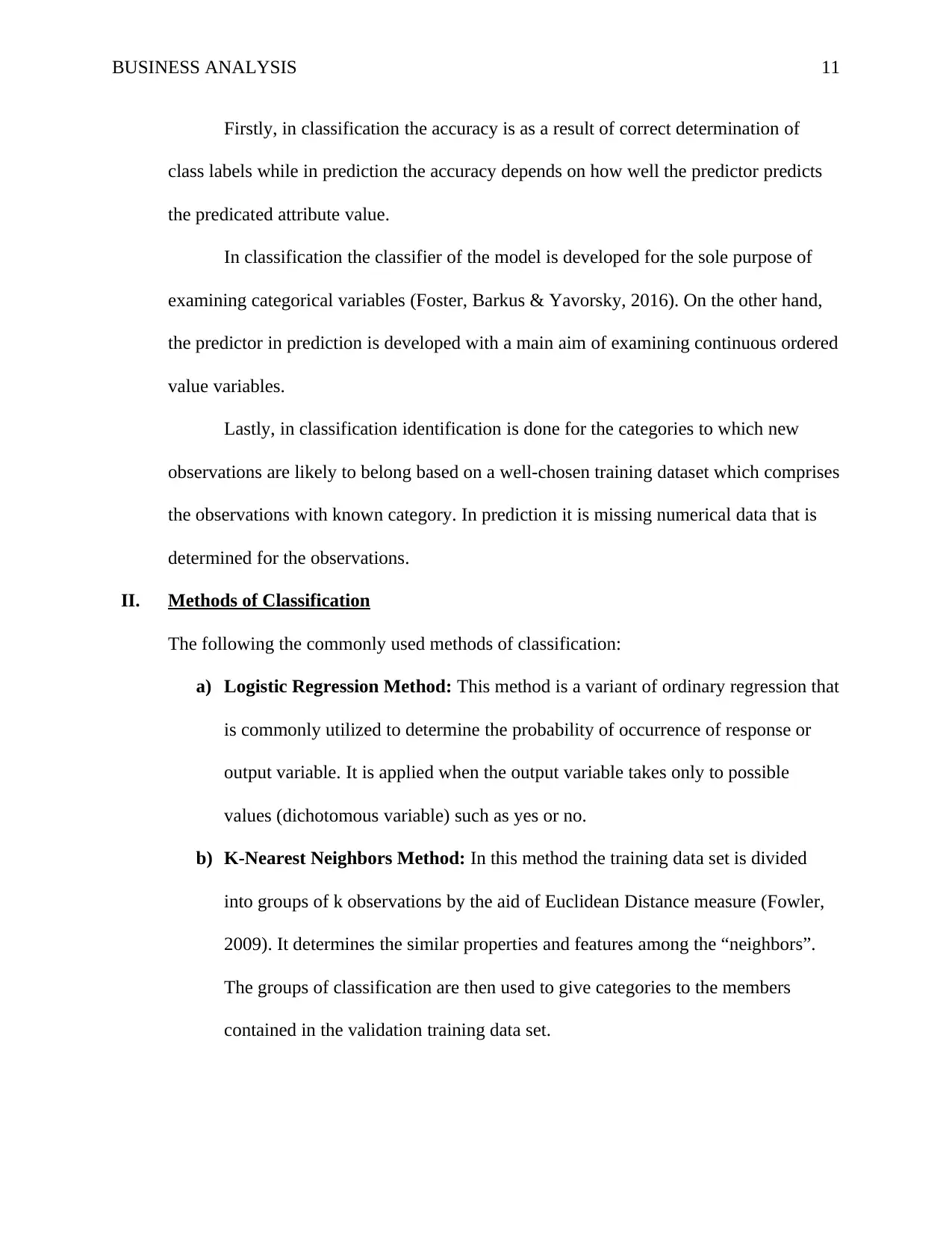
BUSINESS ANALYSIS 11
Firstly, in classification the accuracy is as a result of correct determination of
class labels while in prediction the accuracy depends on how well the predictor predicts
the predicated attribute value.
In classification the classifier of the model is developed for the sole purpose of
examining categorical variables (Foster, Barkus & Yavorsky, 2016). On the other hand,
the predictor in prediction is developed with a main aim of examining continuous ordered
value variables.
Lastly, in classification identification is done for the categories to which new
observations are likely to belong based on a well-chosen training dataset which comprises
the observations with known category. In prediction it is missing numerical data that is
determined for the observations.
II. Methods of Classification
The following the commonly used methods of classification:
a) Logistic Regression Method: This method is a variant of ordinary regression that
is commonly utilized to determine the probability of occurrence of response or
output variable. It is applied when the output variable takes only to possible
values (dichotomous variable) such as yes or no.
b) K-Nearest Neighbors Method: In this method the training data set is divided
into groups of k observations by the aid of Euclidean Distance measure (Fowler,
2009). It determines the similar properties and features among the “neighbors”.
The groups of classification are then used to give categories to the members
contained in the validation training data set.
Firstly, in classification the accuracy is as a result of correct determination of
class labels while in prediction the accuracy depends on how well the predictor predicts
the predicated attribute value.
In classification the classifier of the model is developed for the sole purpose of
examining categorical variables (Foster, Barkus & Yavorsky, 2016). On the other hand,
the predictor in prediction is developed with a main aim of examining continuous ordered
value variables.
Lastly, in classification identification is done for the categories to which new
observations are likely to belong based on a well-chosen training dataset which comprises
the observations with known category. In prediction it is missing numerical data that is
determined for the observations.
II. Methods of Classification
The following the commonly used methods of classification:
a) Logistic Regression Method: This method is a variant of ordinary regression that
is commonly utilized to determine the probability of occurrence of response or
output variable. It is applied when the output variable takes only to possible
values (dichotomous variable) such as yes or no.
b) K-Nearest Neighbors Method: In this method the training data set is divided
into groups of k observations by the aid of Euclidean Distance measure (Fowler,
2009). It determines the similar properties and features among the “neighbors”.
The groups of classification are then used to give categories to the members
contained in the validation training data set.
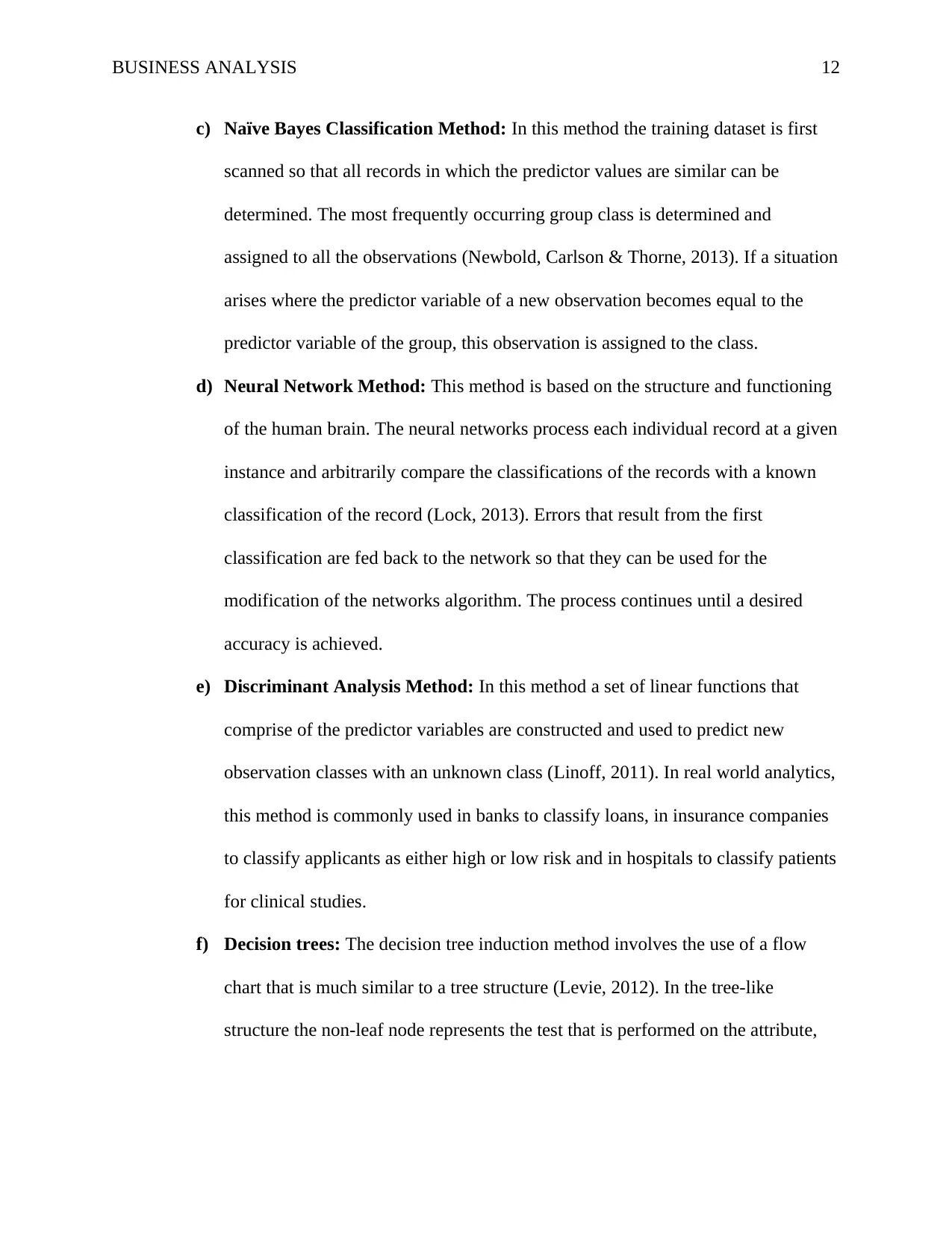
BUSINESS ANALYSIS 12
c) Naïve Bayes Classification Method: In this method the training dataset is first
scanned so that all records in which the predictor values are similar can be
determined. The most frequently occurring group class is determined and
assigned to all the observations (Newbold, Carlson & Thorne, 2013). If a situation
arises where the predictor variable of a new observation becomes equal to the
predictor variable of the group, this observation is assigned to the class.
d) Neural Network Method: This method is based on the structure and functioning
of the human brain. The neural networks process each individual record at a given
instance and arbitrarily compare the classifications of the records with a known
classification of the record (Lock, 2013). Errors that result from the first
classification are fed back to the network so that they can be used for the
modification of the networks algorithm. The process continues until a desired
accuracy is achieved.
e) Discriminant Analysis Method: In this method a set of linear functions that
comprise of the predictor variables are constructed and used to predict new
observation classes with an unknown class (Linoff, 2011). In real world analytics,
this method is commonly used in banks to classify loans, in insurance companies
to classify applicants as either high or low risk and in hospitals to classify patients
for clinical studies.
f) Decision trees: The decision tree induction method involves the use of a flow
chart that is much similar to a tree structure (Levie, 2012). In the tree-like
structure the non-leaf node represents the test that is performed on the attribute,
c) Naïve Bayes Classification Method: In this method the training dataset is first
scanned so that all records in which the predictor values are similar can be
determined. The most frequently occurring group class is determined and
assigned to all the observations (Newbold, Carlson & Thorne, 2013). If a situation
arises where the predictor variable of a new observation becomes equal to the
predictor variable of the group, this observation is assigned to the class.
d) Neural Network Method: This method is based on the structure and functioning
of the human brain. The neural networks process each individual record at a given
instance and arbitrarily compare the classifications of the records with a known
classification of the record (Lock, 2013). Errors that result from the first
classification are fed back to the network so that they can be used for the
modification of the networks algorithm. The process continues until a desired
accuracy is achieved.
e) Discriminant Analysis Method: In this method a set of linear functions that
comprise of the predictor variables are constructed and used to predict new
observation classes with an unknown class (Linoff, 2011). In real world analytics,
this method is commonly used in banks to classify loans, in insurance companies
to classify applicants as either high or low risk and in hospitals to classify patients
for clinical studies.
f) Decision trees: The decision tree induction method involves the use of a flow
chart that is much similar to a tree structure (Levie, 2012). In the tree-like
structure the non-leaf node represents the test that is performed on the attribute,
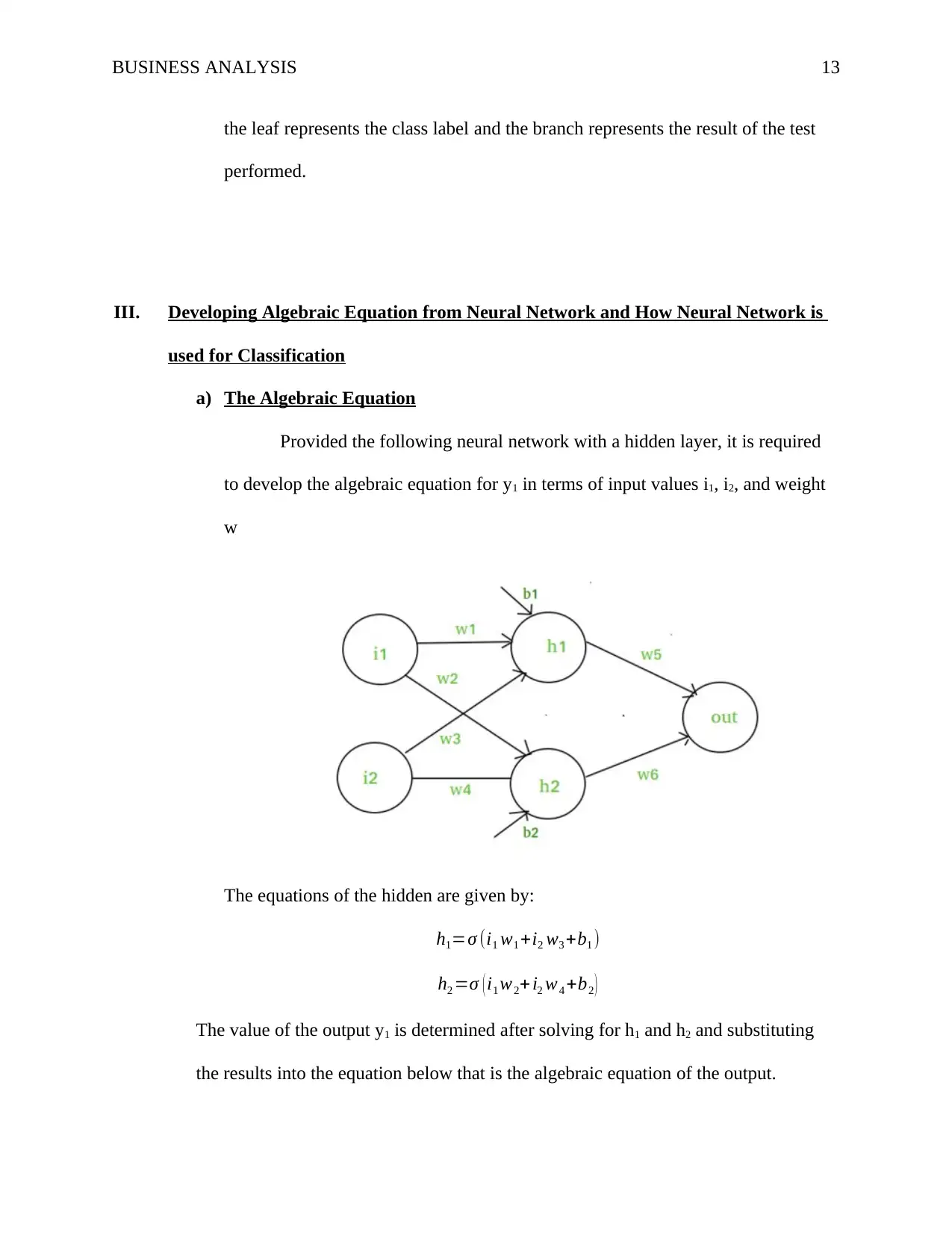
BUSINESS ANALYSIS 13
the leaf represents the class label and the branch represents the result of the test
performed.
III. Developing Algebraic Equation from Neural Network and How Neural Network is
used for Classification
a) The Algebraic Equation
Provided the following neural network with a hidden layer, it is required
to develop the algebraic equation for y1 in terms of input values i1, i2, and weight
w
The equations of the hidden are given by:
h1=σ (i1 w1 +i2 w3 +b1 )
h2 =σ ( i1 w2+ i2 w4 +b2 )
The value of the output y1 is determined after solving for h1 and h2 and substituting
the results into the equation below that is the algebraic equation of the output.
the leaf represents the class label and the branch represents the result of the test
performed.
III. Developing Algebraic Equation from Neural Network and How Neural Network is
used for Classification
a) The Algebraic Equation
Provided the following neural network with a hidden layer, it is required
to develop the algebraic equation for y1 in terms of input values i1, i2, and weight
w
The equations of the hidden are given by:
h1=σ (i1 w1 +i2 w3 +b1 )
h2 =σ ( i1 w2+ i2 w4 +b2 )
The value of the output y1 is determined after solving for h1 and h2 and substituting
the results into the equation below that is the algebraic equation of the output.
Paraphrase This Document
Need a fresh take? Get an instant paraphrase of this document with our AI Paraphraser
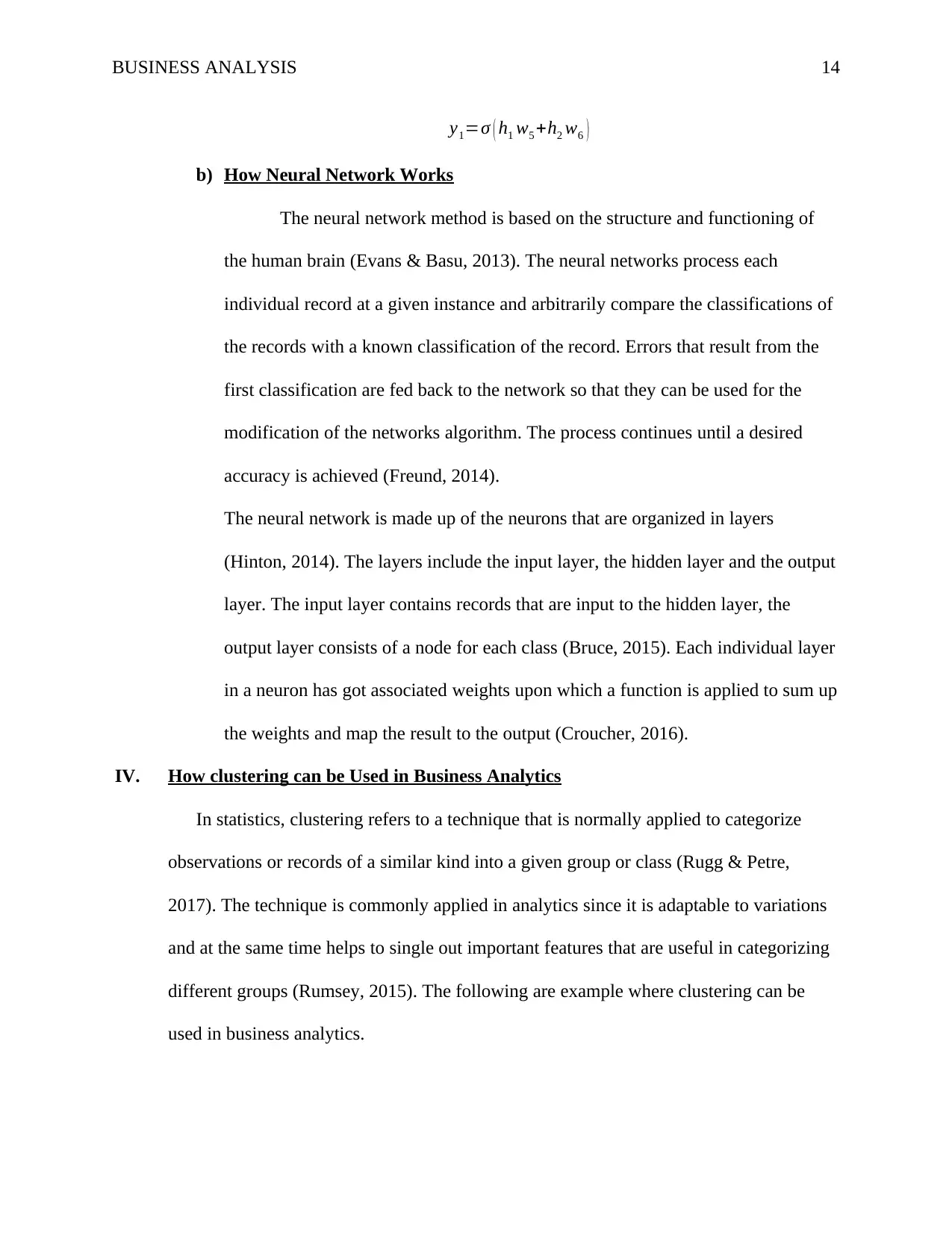
BUSINESS ANALYSIS 14
y1=σ ( h1 w5 +h2 w6 )
b) How Neural Network Works
The neural network method is based on the structure and functioning of
the human brain (Evans & Basu, 2013). The neural networks process each
individual record at a given instance and arbitrarily compare the classifications of
the records with a known classification of the record. Errors that result from the
first classification are fed back to the network so that they can be used for the
modification of the networks algorithm. The process continues until a desired
accuracy is achieved (Freund, 2014).
The neural network is made up of the neurons that are organized in layers
(Hinton, 2014). The layers include the input layer, the hidden layer and the output
layer. The input layer contains records that are input to the hidden layer, the
output layer consists of a node for each class (Bruce, 2015). Each individual layer
in a neuron has got associated weights upon which a function is applied to sum up
the weights and map the result to the output (Croucher, 2016).
IV. How clustering can be Used in Business Analytics
In statistics, clustering refers to a technique that is normally applied to categorize
observations or records of a similar kind into a given group or class (Rugg & Petre,
2017). The technique is commonly applied in analytics since it is adaptable to variations
and at the same time helps to single out important features that are useful in categorizing
different groups (Rumsey, 2015). The following are example where clustering can be
used in business analytics.
y1=σ ( h1 w5 +h2 w6 )
b) How Neural Network Works
The neural network method is based on the structure and functioning of
the human brain (Evans & Basu, 2013). The neural networks process each
individual record at a given instance and arbitrarily compare the classifications of
the records with a known classification of the record. Errors that result from the
first classification are fed back to the network so that they can be used for the
modification of the networks algorithm. The process continues until a desired
accuracy is achieved (Freund, 2014).
The neural network is made up of the neurons that are organized in layers
(Hinton, 2014). The layers include the input layer, the hidden layer and the output
layer. The input layer contains records that are input to the hidden layer, the
output layer consists of a node for each class (Bruce, 2015). Each individual layer
in a neuron has got associated weights upon which a function is applied to sum up
the weights and map the result to the output (Croucher, 2016).
IV. How clustering can be Used in Business Analytics
In statistics, clustering refers to a technique that is normally applied to categorize
observations or records of a similar kind into a given group or class (Rugg & Petre,
2017). The technique is commonly applied in analytics since it is adaptable to variations
and at the same time helps to single out important features that are useful in categorizing
different groups (Rumsey, 2015). The following are example where clustering can be
used in business analytics.
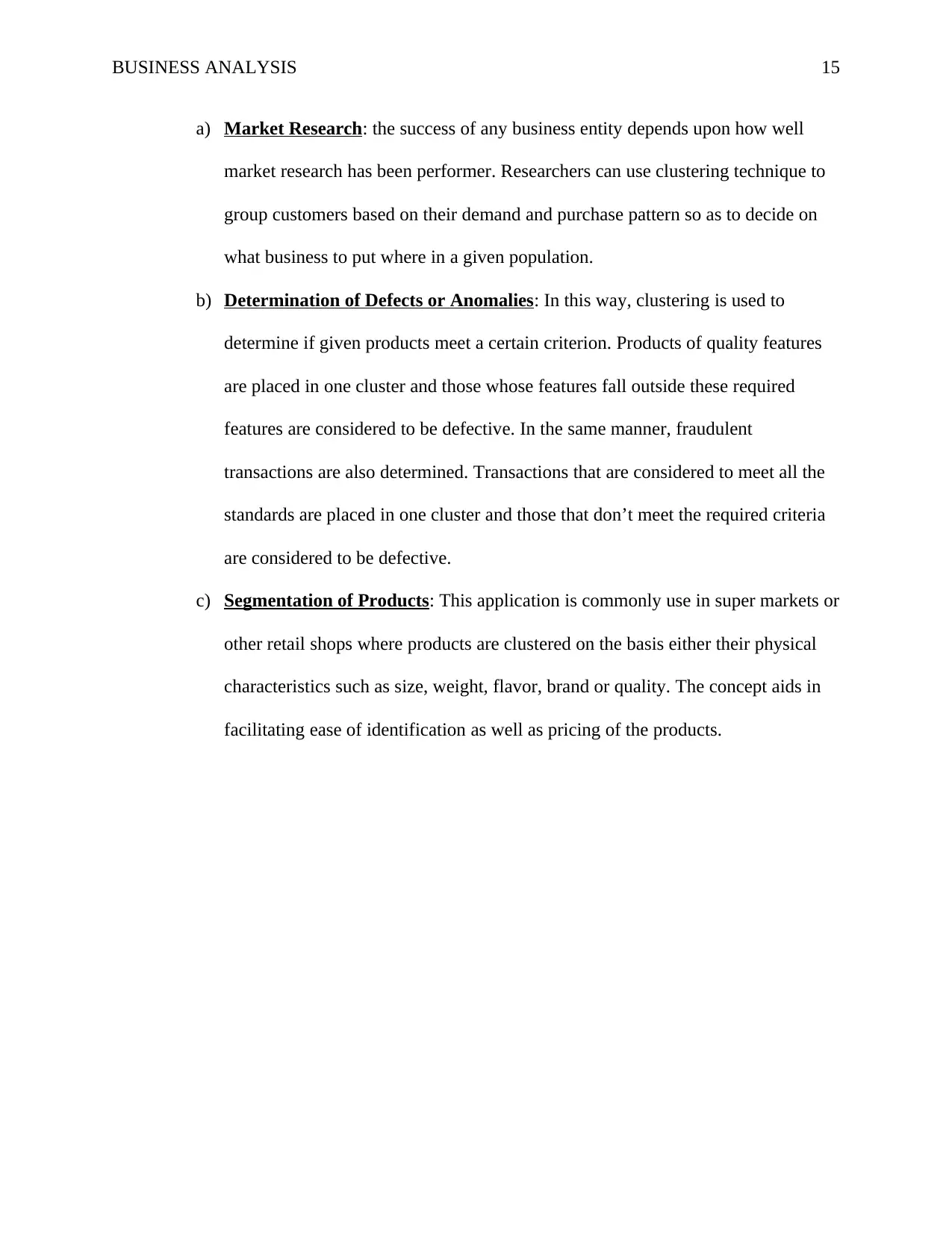
BUSINESS ANALYSIS 15
a) Market Research: the success of any business entity depends upon how well
market research has been performer. Researchers can use clustering technique to
group customers based on their demand and purchase pattern so as to decide on
what business to put where in a given population.
b) Determination of Defects or Anomalies: In this way, clustering is used to
determine if given products meet a certain criterion. Products of quality features
are placed in one cluster and those whose features fall outside these required
features are considered to be defective. In the same manner, fraudulent
transactions are also determined. Transactions that are considered to meet all the
standards are placed in one cluster and those that don’t meet the required criteria
are considered to be defective.
c) Segmentation of Products: This application is commonly use in super markets or
other retail shops where products are clustered on the basis either their physical
characteristics such as size, weight, flavor, brand or quality. The concept aids in
facilitating ease of identification as well as pricing of the products.
a) Market Research: the success of any business entity depends upon how well
market research has been performer. Researchers can use clustering technique to
group customers based on their demand and purchase pattern so as to decide on
what business to put where in a given population.
b) Determination of Defects or Anomalies: In this way, clustering is used to
determine if given products meet a certain criterion. Products of quality features
are placed in one cluster and those whose features fall outside these required
features are considered to be defective. In the same manner, fraudulent
transactions are also determined. Transactions that are considered to meet all the
standards are placed in one cluster and those that don’t meet the required criteria
are considered to be defective.
c) Segmentation of Products: This application is commonly use in super markets or
other retail shops where products are clustered on the basis either their physical
characteristics such as size, weight, flavor, brand or quality. The concept aids in
facilitating ease of identification as well as pricing of the products.
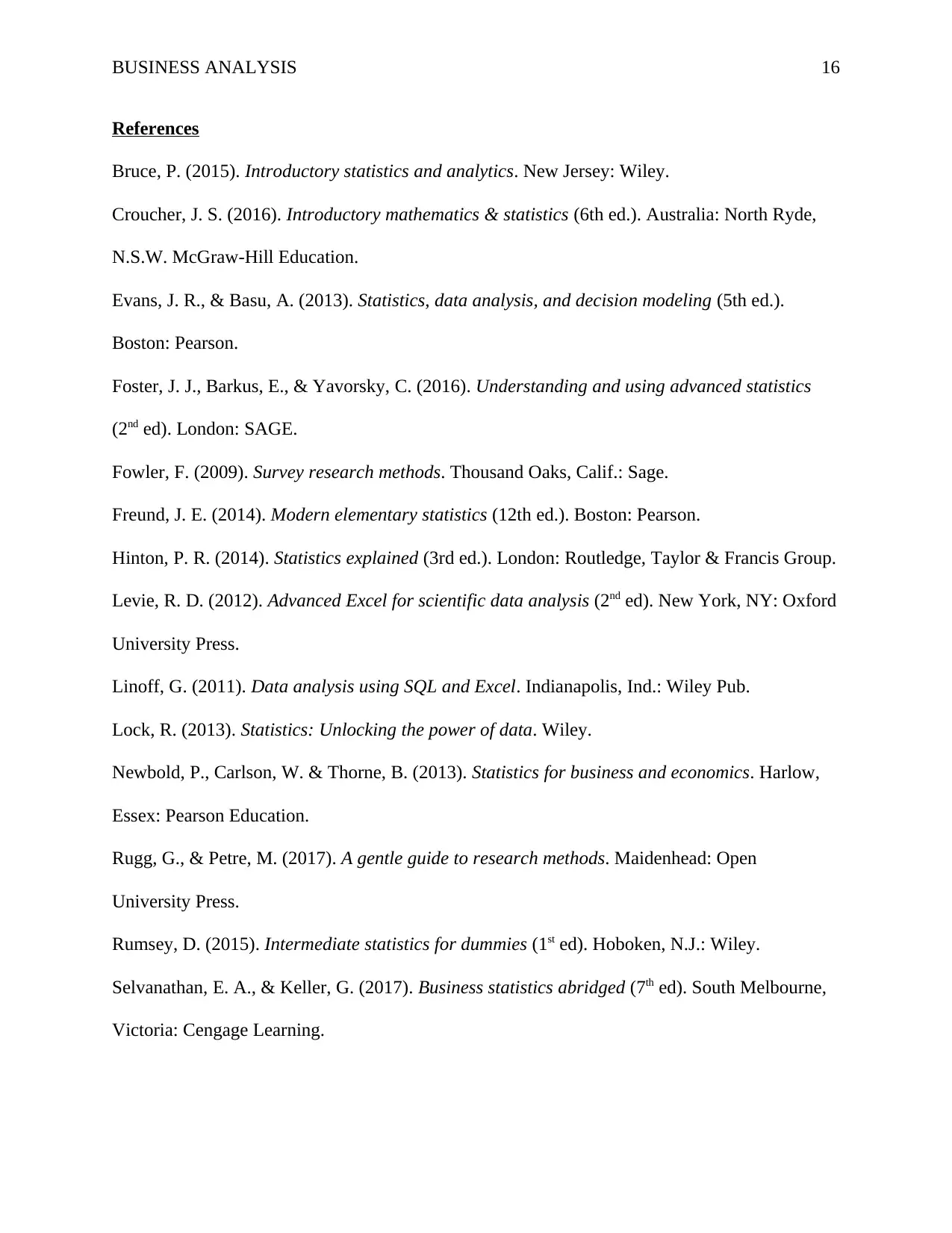
BUSINESS ANALYSIS 16
References
Bruce, P. (2015). Introductory statistics and analytics. New Jersey: Wiley.
Croucher, J. S. (2016). Introductory mathematics & statistics (6th ed.). Australia: North Ryde,
N.S.W. McGraw-Hill Education.
Evans, J. R., & Basu, A. (2013). Statistics, data analysis, and decision modeling (5th ed.).
Boston: Pearson.
Foster, J. J., Barkus, E., & Yavorsky, C. (2016). Understanding and using advanced statistics
(2nd ed). London: SAGE.
Fowler, F. (2009). Survey research methods. Thousand Oaks, Calif.: Sage.
Freund, J. E. (2014). Modern elementary statistics (12th ed.). Boston: Pearson.
Hinton, P. R. (2014). Statistics explained (3rd ed.). London: Routledge, Taylor & Francis Group.
Levie, R. D. (2012). Advanced Excel for scientific data analysis (2nd ed). New York, NY: Oxford
University Press.
Linoff, G. (2011). Data analysis using SQL and Excel. Indianapolis, Ind.: Wiley Pub.
Lock, R. (2013). Statistics: Unlocking the power of data. Wiley.
Newbold, P., Carlson, W. & Thorne, B. (2013). Statistics for business and economics. Harlow,
Essex: Pearson Education.
Rugg, G., & Petre, M. (2017). A gentle guide to research methods. Maidenhead: Open
University Press.
Rumsey, D. (2015). Intermediate statistics for dummies (1st ed). Hoboken, N.J.: Wiley.
Selvanathan, E. A., & Keller, G. (2017). Business statistics abridged (7th ed). South Melbourne,
Victoria: Cengage Learning.
References
Bruce, P. (2015). Introductory statistics and analytics. New Jersey: Wiley.
Croucher, J. S. (2016). Introductory mathematics & statistics (6th ed.). Australia: North Ryde,
N.S.W. McGraw-Hill Education.
Evans, J. R., & Basu, A. (2013). Statistics, data analysis, and decision modeling (5th ed.).
Boston: Pearson.
Foster, J. J., Barkus, E., & Yavorsky, C. (2016). Understanding and using advanced statistics
(2nd ed). London: SAGE.
Fowler, F. (2009). Survey research methods. Thousand Oaks, Calif.: Sage.
Freund, J. E. (2014). Modern elementary statistics (12th ed.). Boston: Pearson.
Hinton, P. R. (2014). Statistics explained (3rd ed.). London: Routledge, Taylor & Francis Group.
Levie, R. D. (2012). Advanced Excel for scientific data analysis (2nd ed). New York, NY: Oxford
University Press.
Linoff, G. (2011). Data analysis using SQL and Excel. Indianapolis, Ind.: Wiley Pub.
Lock, R. (2013). Statistics: Unlocking the power of data. Wiley.
Newbold, P., Carlson, W. & Thorne, B. (2013). Statistics for business and economics. Harlow,
Essex: Pearson Education.
Rugg, G., & Petre, M. (2017). A gentle guide to research methods. Maidenhead: Open
University Press.
Rumsey, D. (2015). Intermediate statistics for dummies (1st ed). Hoboken, N.J.: Wiley.
Selvanathan, E. A., & Keller, G. (2017). Business statistics abridged (7th ed). South Melbourne,
Victoria: Cengage Learning.
Secure Best Marks with AI Grader
Need help grading? Try our AI Grader for instant feedback on your assignments.
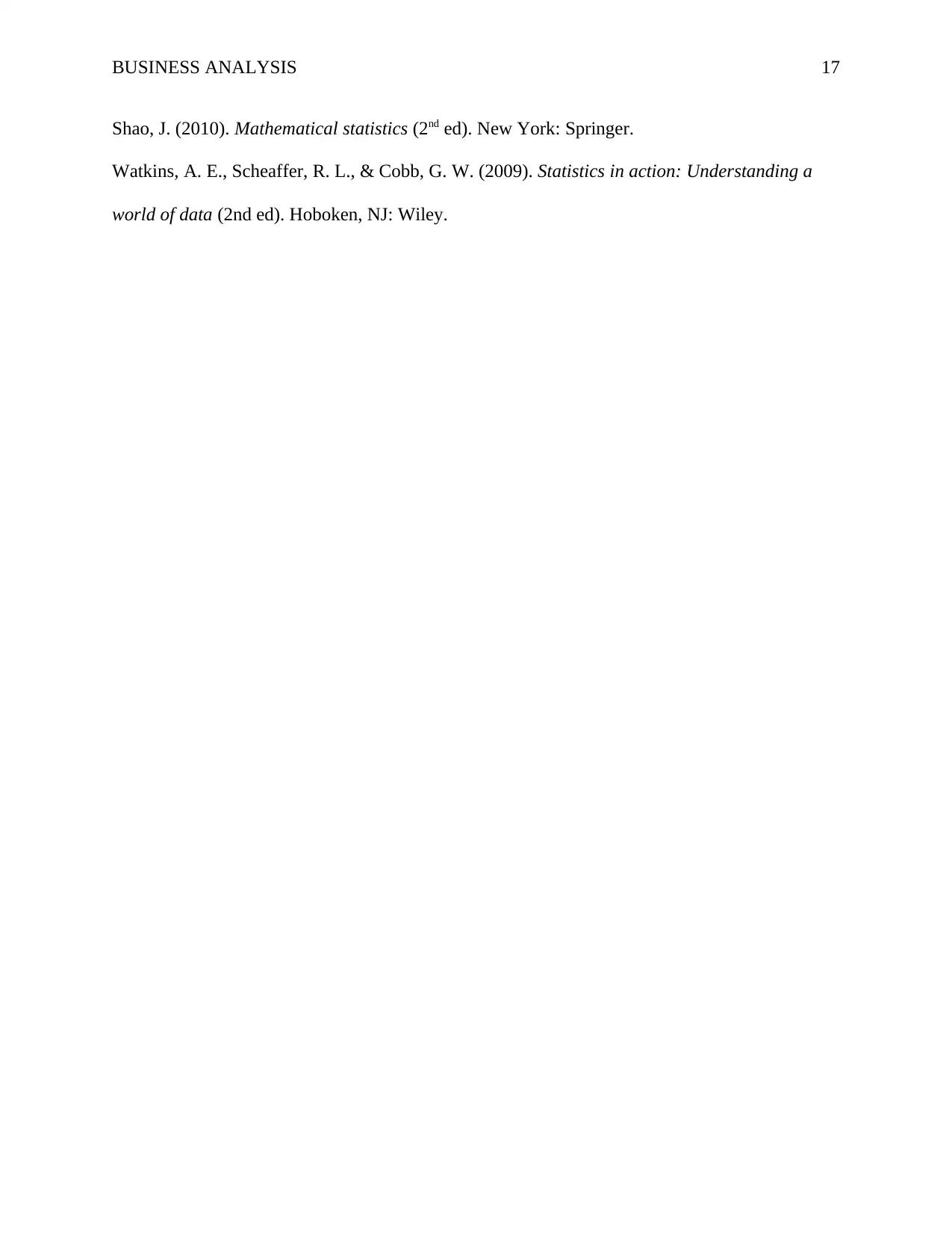
BUSINESS ANALYSIS 17
Shao, J. (2010). Mathematical statistics (2nd ed). New York: Springer.
Watkins, A. E., Scheaffer, R. L., & Cobb, G. W. (2009). Statistics in action: Understanding a
world of data (2nd ed). Hoboken, NJ: Wiley.
Shao, J. (2010). Mathematical statistics (2nd ed). New York: Springer.
Watkins, A. E., Scheaffer, R. L., & Cobb, G. W. (2009). Statistics in action: Understanding a
world of data (2nd ed). Hoboken, NJ: Wiley.
1 out of 17
Related Documents
![[object Object]](/_next/image/?url=%2F_next%2Fstatic%2Fmedia%2Flogo.6d15ce61.png&w=640&q=75)
Your All-in-One AI-Powered Toolkit for Academic Success.
+13062052269
info@desklib.com
Available 24*7 on WhatsApp / Email
Unlock your academic potential
© 2024 | Zucol Services PVT LTD | All rights reserved.