Newcastle Player Performance Analysis
VerifiedAdded on 2020/06/04
|18
|2191
|100
AI Summary
This assignment delves into the performance of Newcastle players by analyzing their fees, goals scored, and positions using cluster analysis. The analysis aims to determine if there's a significant relationship between a player's fee and their goal-scoring ability. It also investigates similarities in player performance based on average passes, key passes, and position on the field. The findings will contribute to understanding how player value and performance are correlated within Newcastle United.
Contribute Materials
Your contribution can guide someone’s learning journey. Share your
documents today.
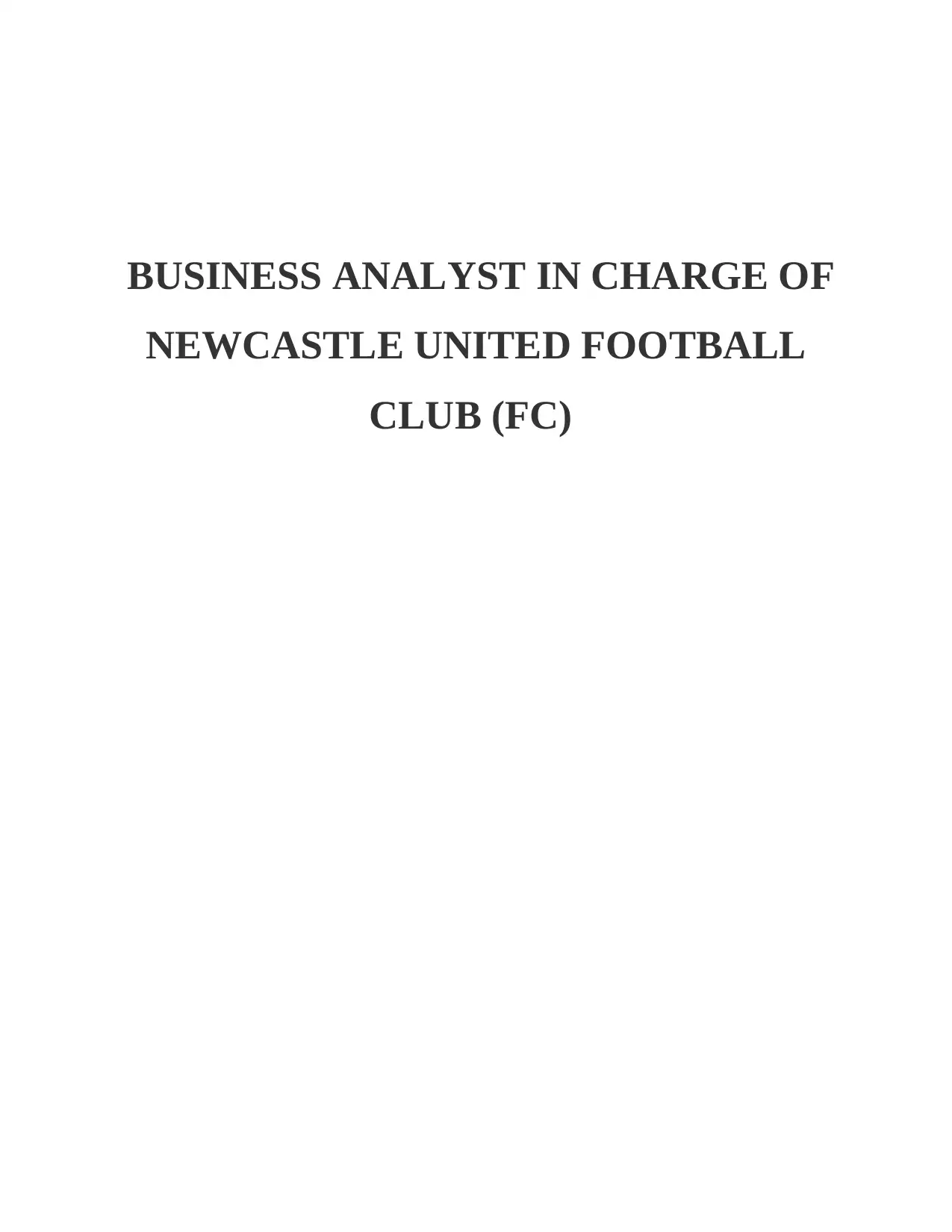
BUSINESS ANALYST IN CHARGE OF
NEWCASTLE UNITED FOOTBALL
CLUB (FC)
NEWCASTLE UNITED FOOTBALL
CLUB (FC)
Secure Best Marks with AI Grader
Need help grading? Try our AI Grader for instant feedback on your assignments.
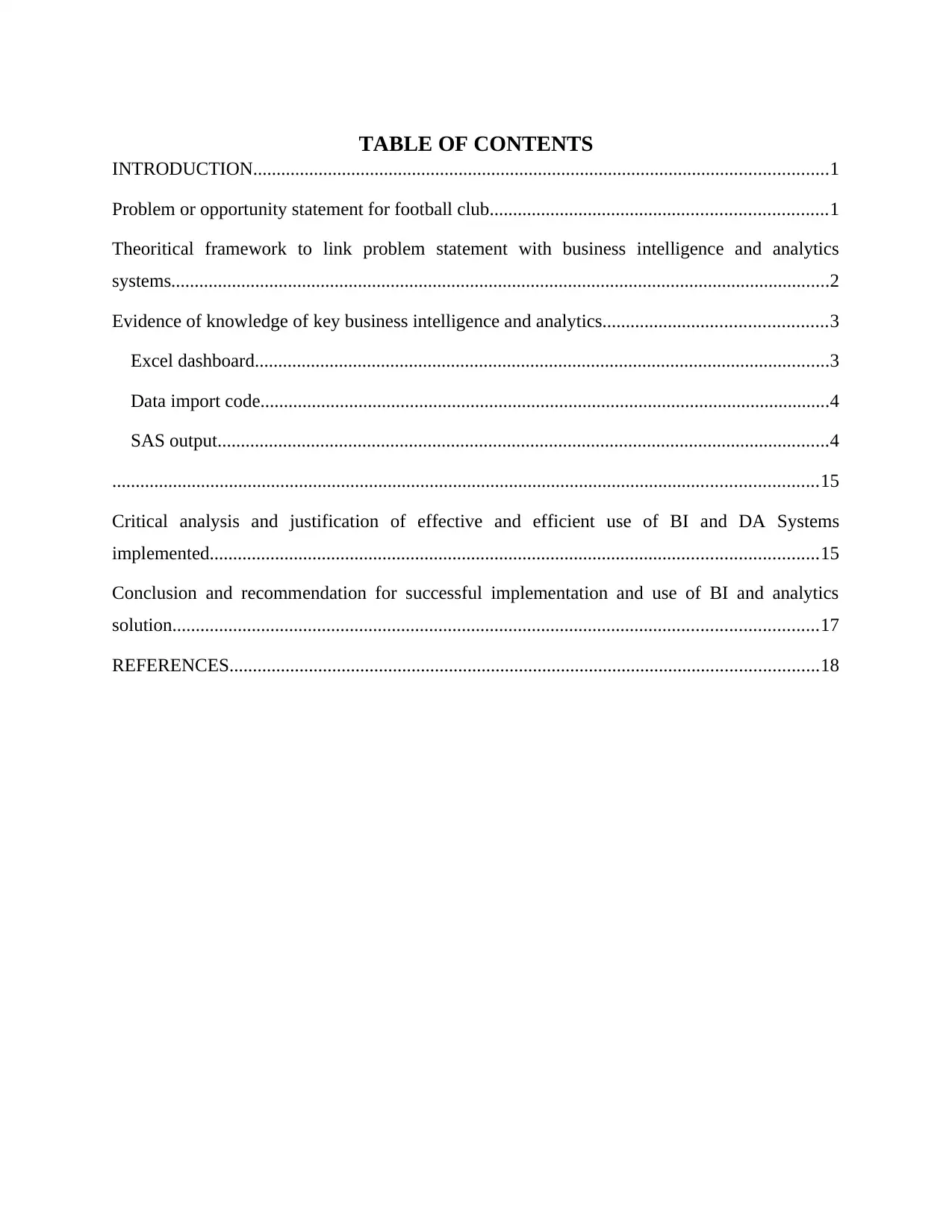
TABLE OF CONTENTS
INTRODUCTION...........................................................................................................................1
Problem or opportunity statement for football club........................................................................1
Theoritical framework to link problem statement with business intelligence and analytics
systems.............................................................................................................................................2
Evidence of knowledge of key business intelligence and analytics................................................3
Excel dashboard...........................................................................................................................3
Data import code..........................................................................................................................4
SAS output...................................................................................................................................4
.......................................................................................................................................................15
Critical analysis and justification of effective and efficient use of BI and DA Systems
implemented..................................................................................................................................15
Conclusion and recommendation for successful implementation and use of BI and analytics
solution..........................................................................................................................................17
REFERENCES..............................................................................................................................18
INTRODUCTION...........................................................................................................................1
Problem or opportunity statement for football club........................................................................1
Theoritical framework to link problem statement with business intelligence and analytics
systems.............................................................................................................................................2
Evidence of knowledge of key business intelligence and analytics................................................3
Excel dashboard...........................................................................................................................3
Data import code..........................................................................................................................4
SAS output...................................................................................................................................4
.......................................................................................................................................................15
Critical analysis and justification of effective and efficient use of BI and DA Systems
implemented..................................................................................................................................15
Conclusion and recommendation for successful implementation and use of BI and analytics
solution..........................................................................................................................................17
REFERENCES..............................................................................................................................18
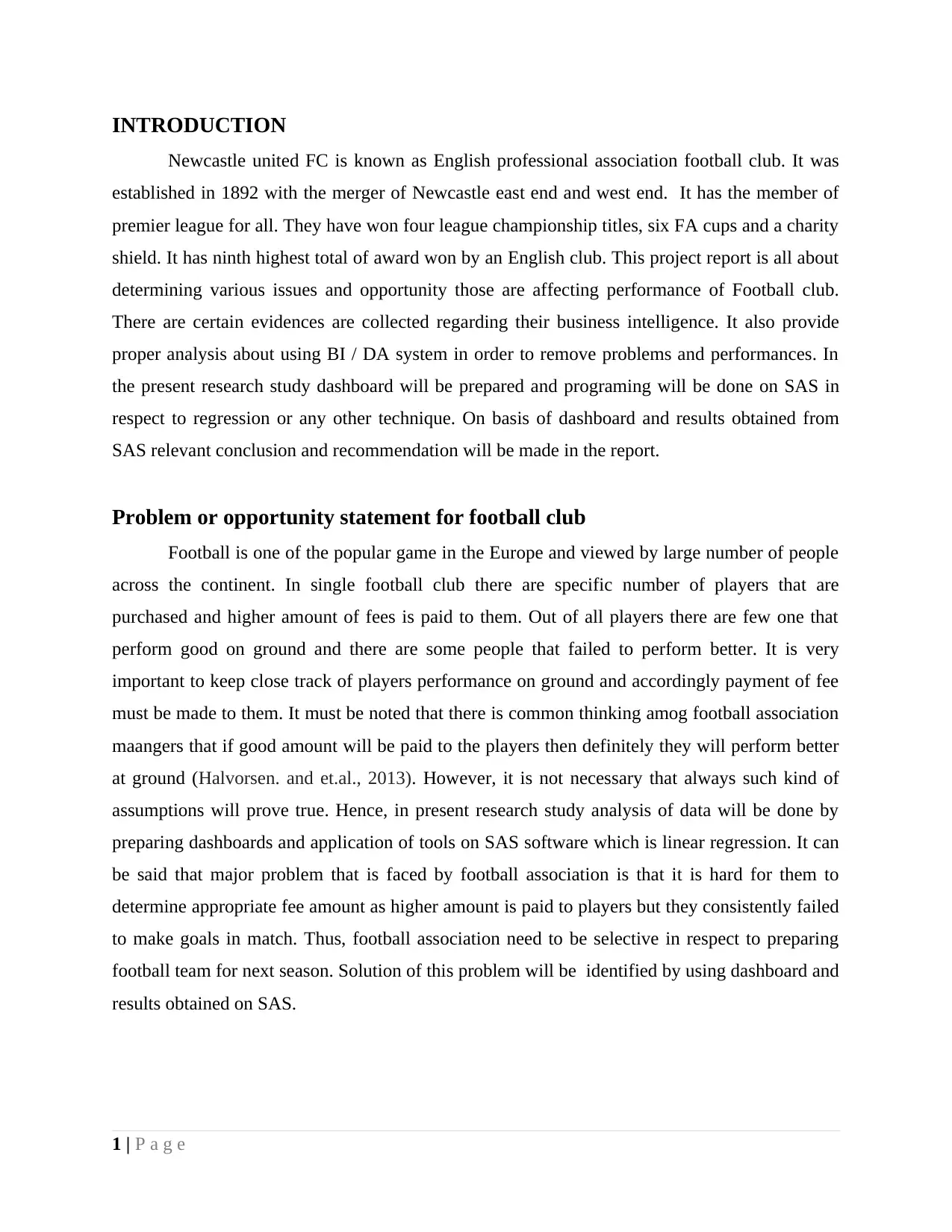
INTRODUCTION
Newcastle united FC is known as English professional association football club. It was
established in 1892 with the merger of Newcastle east end and west end. It has the member of
premier league for all. They have won four league championship titles, six FA cups and a charity
shield. It has ninth highest total of award won by an English club. This project report is all about
determining various issues and opportunity those are affecting performance of Football club.
There are certain evidences are collected regarding their business intelligence. It also provide
proper analysis about using BI / DA system in order to remove problems and performances. In
the present research study dashboard will be prepared and programing will be done on SAS in
respect to regression or any other technique. On basis of dashboard and results obtained from
SAS relevant conclusion and recommendation will be made in the report.
Problem or opportunity statement for football club
Football is one of the popular game in the Europe and viewed by large number of people
across the continent. In single football club there are specific number of players that are
purchased and higher amount of fees is paid to them. Out of all players there are few one that
perform good on ground and there are some people that failed to perform better. It is very
important to keep close track of players performance on ground and accordingly payment of fee
must be made to them. It must be noted that there is common thinking amog football association
maangers that if good amount will be paid to the players then definitely they will perform better
at ground (Halvorsen. and et.al., 2013). However, it is not necessary that always such kind of
assumptions will prove true. Hence, in present research study analysis of data will be done by
preparing dashboards and application of tools on SAS software which is linear regression. It can
be said that major problem that is faced by football association is that it is hard for them to
determine appropriate fee amount as higher amount is paid to players but they consistently failed
to make goals in match. Thus, football association need to be selective in respect to preparing
football team for next season. Solution of this problem will be identified by using dashboard and
results obtained on SAS.
1 | P a g e
Newcastle united FC is known as English professional association football club. It was
established in 1892 with the merger of Newcastle east end and west end. It has the member of
premier league for all. They have won four league championship titles, six FA cups and a charity
shield. It has ninth highest total of award won by an English club. This project report is all about
determining various issues and opportunity those are affecting performance of Football club.
There are certain evidences are collected regarding their business intelligence. It also provide
proper analysis about using BI / DA system in order to remove problems and performances. In
the present research study dashboard will be prepared and programing will be done on SAS in
respect to regression or any other technique. On basis of dashboard and results obtained from
SAS relevant conclusion and recommendation will be made in the report.
Problem or opportunity statement for football club
Football is one of the popular game in the Europe and viewed by large number of people
across the continent. In single football club there are specific number of players that are
purchased and higher amount of fees is paid to them. Out of all players there are few one that
perform good on ground and there are some people that failed to perform better. It is very
important to keep close track of players performance on ground and accordingly payment of fee
must be made to them. It must be noted that there is common thinking amog football association
maangers that if good amount will be paid to the players then definitely they will perform better
at ground (Halvorsen. and et.al., 2013). However, it is not necessary that always such kind of
assumptions will prove true. Hence, in present research study analysis of data will be done by
preparing dashboards and application of tools on SAS software which is linear regression. It can
be said that major problem that is faced by football association is that it is hard for them to
determine appropriate fee amount as higher amount is paid to players but they consistently failed
to make goals in match. Thus, football association need to be selective in respect to preparing
football team for next season. Solution of this problem will be identified by using dashboard and
results obtained on SAS.
1 | P a g e
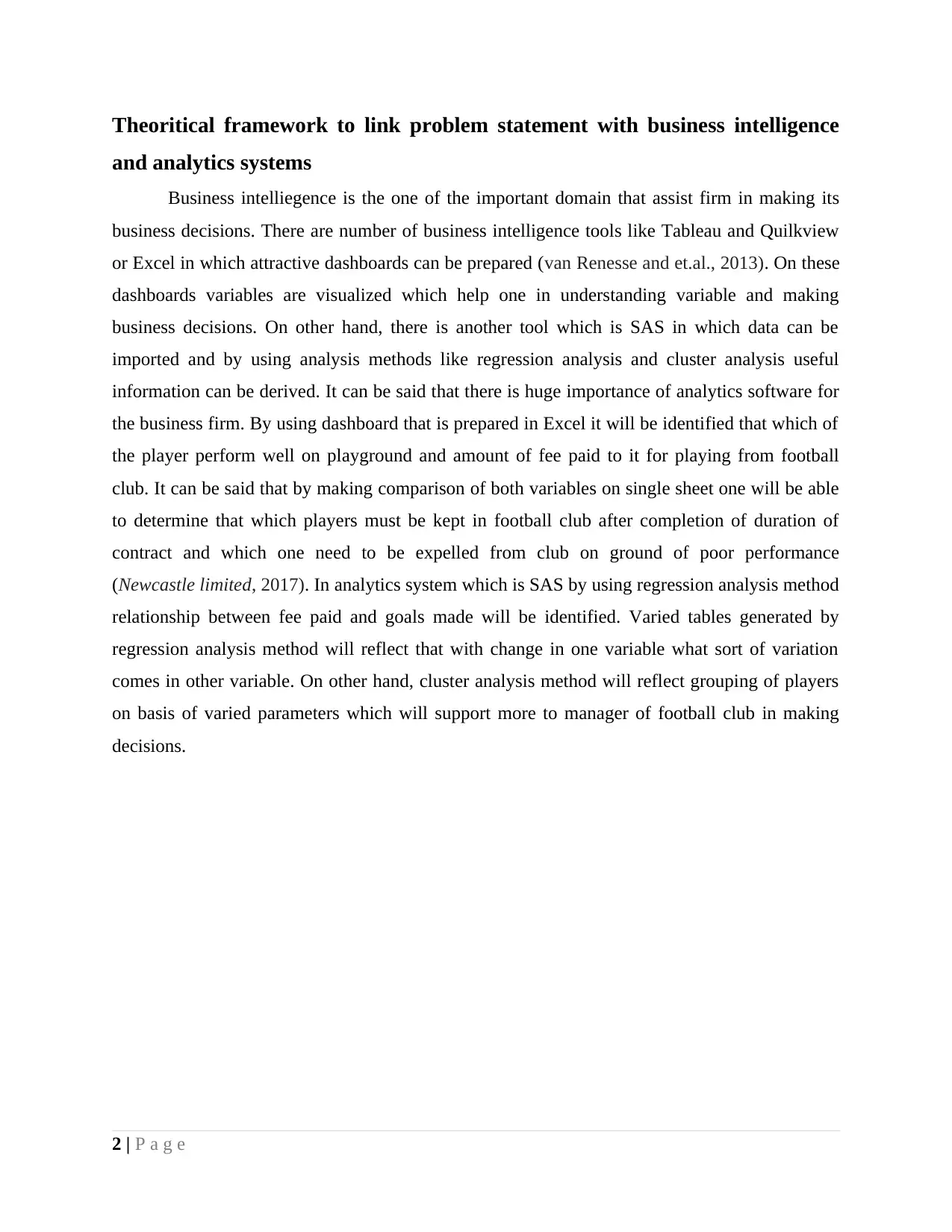
Theoritical framework to link problem statement with business intelligence
and analytics systems
Business intelliegence is the one of the important domain that assist firm in making its
business decisions. There are number of business intelligence tools like Tableau and Quilkview
or Excel in which attractive dashboards can be prepared (van Renesse and et.al., 2013). On these
dashboards variables are visualized which help one in understanding variable and making
business decisions. On other hand, there is another tool which is SAS in which data can be
imported and by using analysis methods like regression analysis and cluster analysis useful
information can be derived. It can be said that there is huge importance of analytics software for
the business firm. By using dashboard that is prepared in Excel it will be identified that which of
the player perform well on playground and amount of fee paid to it for playing from football
club. It can be said that by making comparison of both variables on single sheet one will be able
to determine that which players must be kept in football club after completion of duration of
contract and which one need to be expelled from club on ground of poor performance
(Newcastle limited, 2017). In analytics system which is SAS by using regression analysis method
relationship between fee paid and goals made will be identified. Varied tables generated by
regression analysis method will reflect that with change in one variable what sort of variation
comes in other variable. On other hand, cluster analysis method will reflect grouping of players
on basis of varied parameters which will support more to manager of football club in making
decisions.
2 | P a g e
and analytics systems
Business intelliegence is the one of the important domain that assist firm in making its
business decisions. There are number of business intelligence tools like Tableau and Quilkview
or Excel in which attractive dashboards can be prepared (van Renesse and et.al., 2013). On these
dashboards variables are visualized which help one in understanding variable and making
business decisions. On other hand, there is another tool which is SAS in which data can be
imported and by using analysis methods like regression analysis and cluster analysis useful
information can be derived. It can be said that there is huge importance of analytics software for
the business firm. By using dashboard that is prepared in Excel it will be identified that which of
the player perform well on playground and amount of fee paid to it for playing from football
club. It can be said that by making comparison of both variables on single sheet one will be able
to determine that which players must be kept in football club after completion of duration of
contract and which one need to be expelled from club on ground of poor performance
(Newcastle limited, 2017). In analytics system which is SAS by using regression analysis method
relationship between fee paid and goals made will be identified. Varied tables generated by
regression analysis method will reflect that with change in one variable what sort of variation
comes in other variable. On other hand, cluster analysis method will reflect grouping of players
on basis of varied parameters which will support more to manager of football club in making
decisions.
2 | P a g e
Secure Best Marks with AI Grader
Need help grading? Try our AI Grader for instant feedback on your assignments.
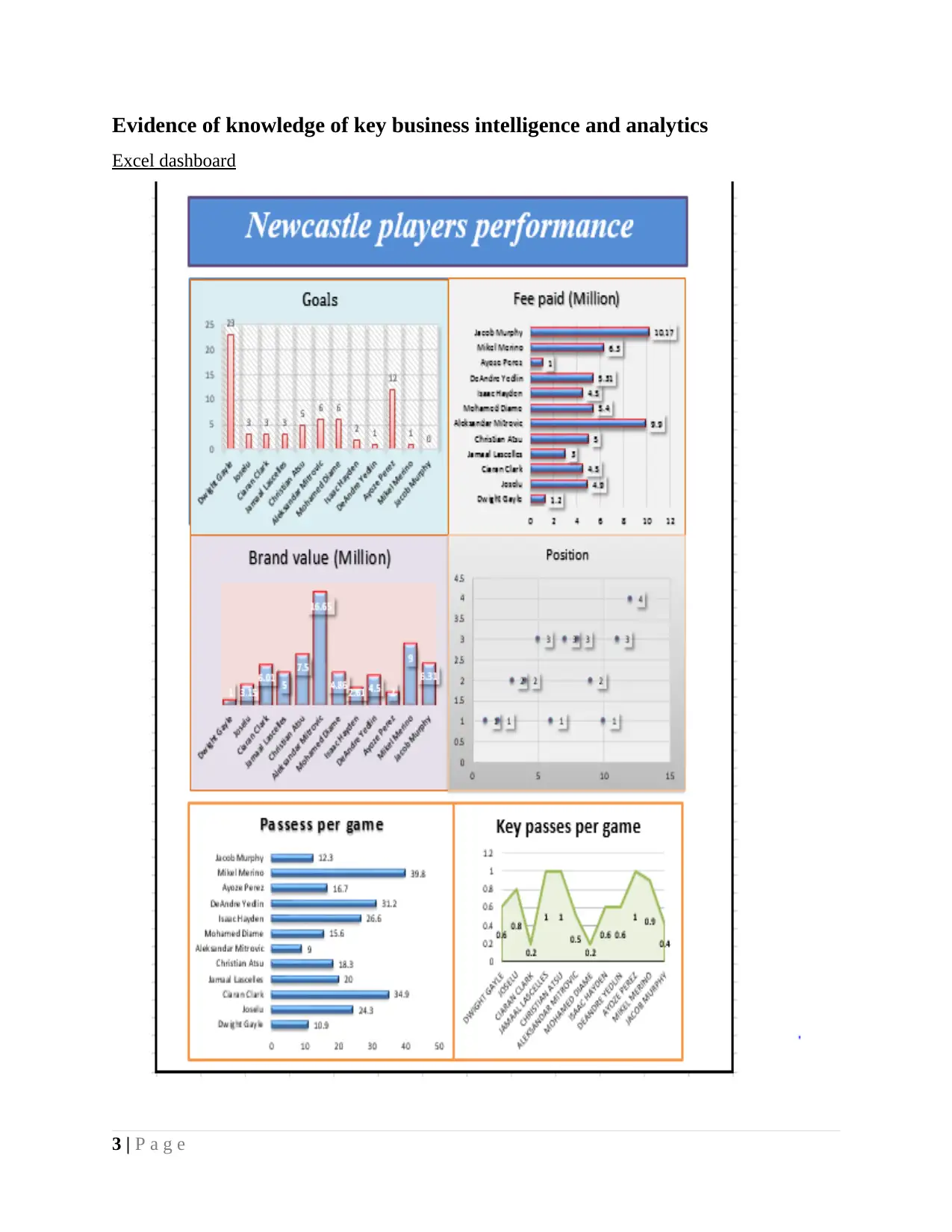
Evidence of knowledge of key business intelligence and analytics
Excel dashboard
3 | P a g e
Excel dashboard
3 | P a g e
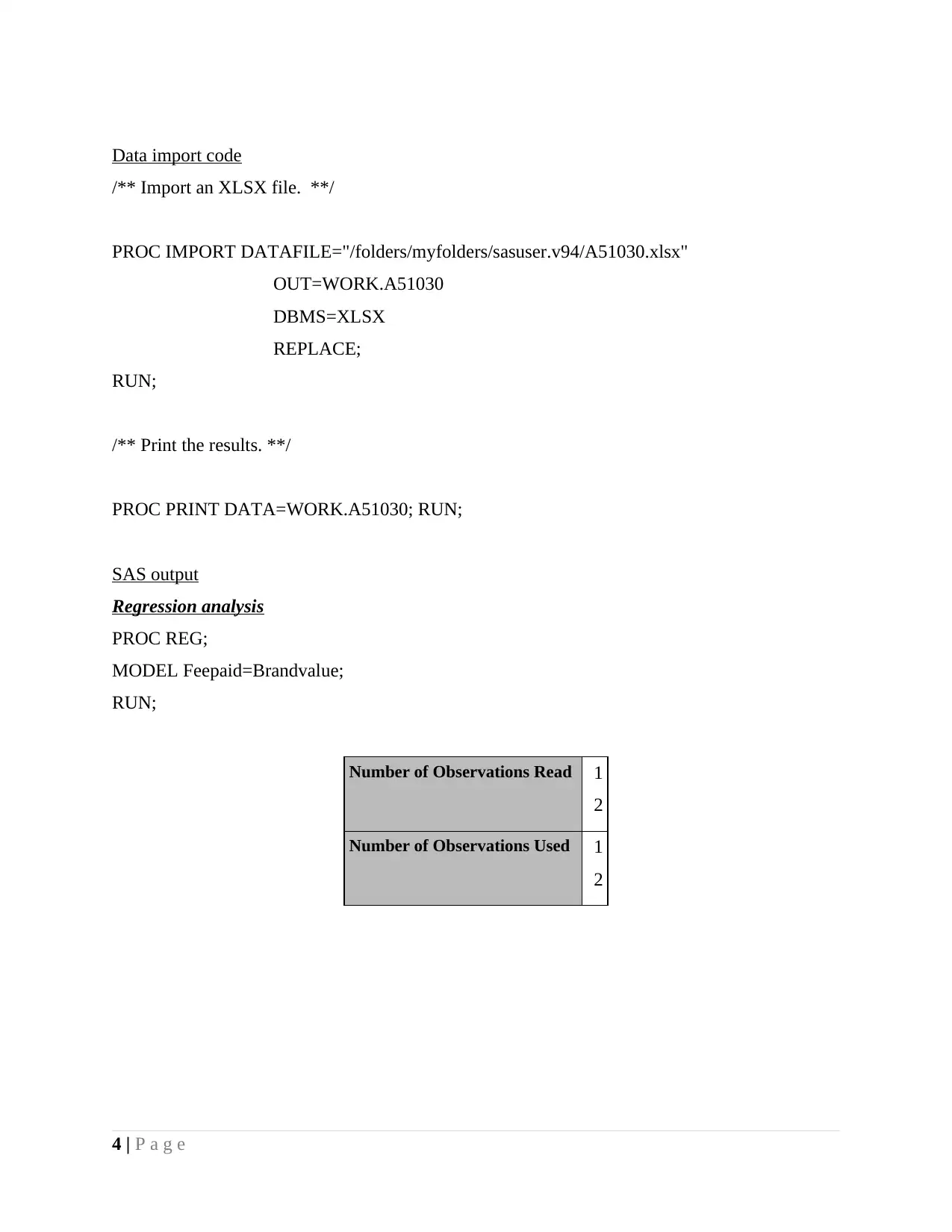
Data import code
/** Import an XLSX file. **/
PROC IMPORT DATAFILE="/folders/myfolders/sasuser.v94/A51030.xlsx"
OUT=WORK.A51030
DBMS=XLSX
REPLACE;
RUN;
/** Print the results. **/
PROC PRINT DATA=WORK.A51030; RUN;
SAS output
Regression analysis
PROC REG;
MODEL Feepaid=Brandvalue;
RUN;
Number of Observations Read 1
2
Number of Observations Used 1
2
4 | P a g e
/** Import an XLSX file. **/
PROC IMPORT DATAFILE="/folders/myfolders/sasuser.v94/A51030.xlsx"
OUT=WORK.A51030
DBMS=XLSX
REPLACE;
RUN;
/** Print the results. **/
PROC PRINT DATA=WORK.A51030; RUN;
SAS output
Regression analysis
PROC REG;
MODEL Feepaid=Brandvalue;
RUN;
Number of Observations Read 1
2
Number of Observations Used 1
2
4 | P a g e
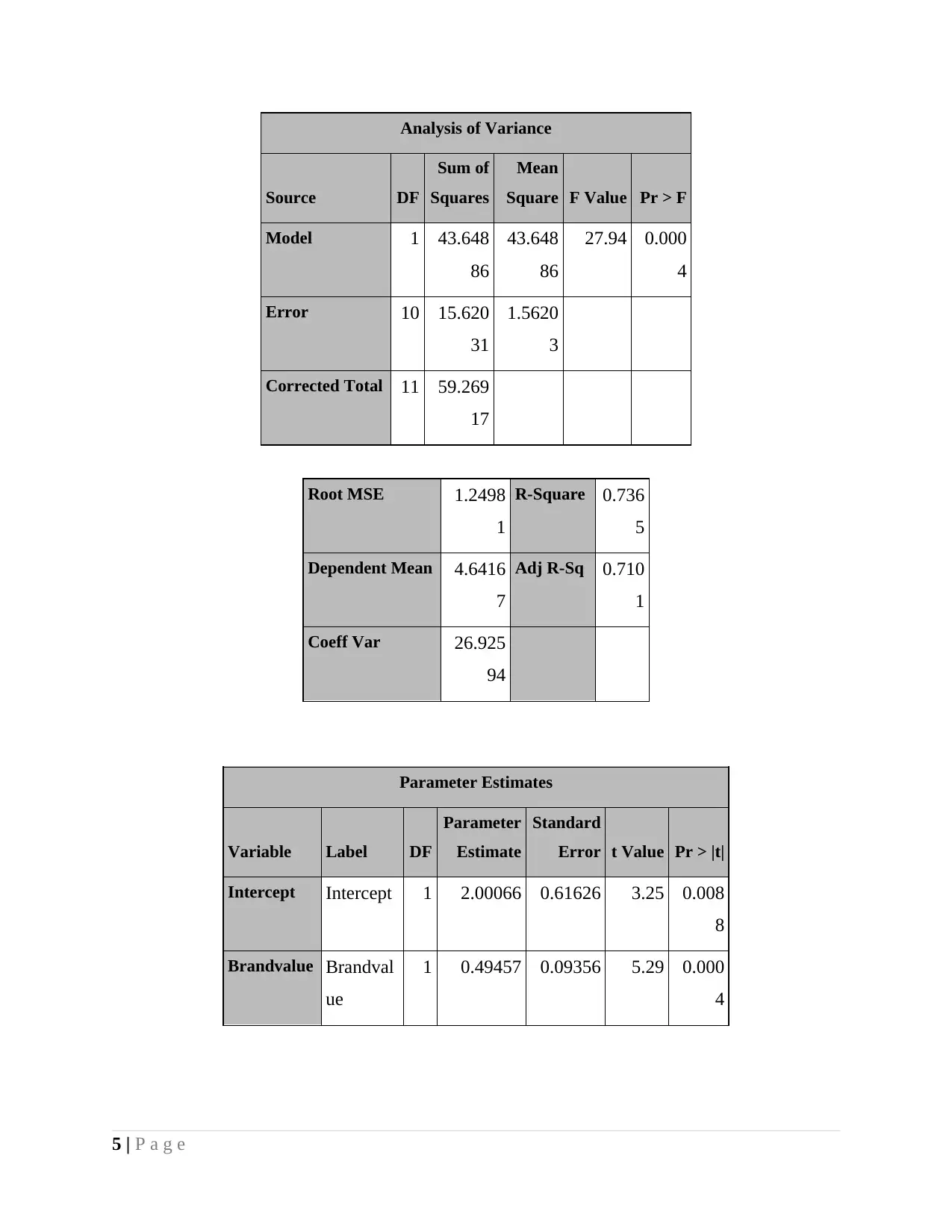
Analysis of Variance
Source DF
Sum of
Squares
Mean
Square F Value Pr > F
Model 1 43.648
86
43.648
86
27.94 0.000
4
Error 10 15.620
31
1.5620
3
Corrected Total 11 59.269
17
Root MSE 1.2498
1
R-Square 0.736
5
Dependent Mean 4.6416
7
Adj R-Sq 0.710
1
Coeff Var 26.925
94
Parameter Estimates
Variable Label DF
Parameter
Estimate
Standard
Error t Value Pr > |t|
Intercept Intercept 1 2.00066 0.61626 3.25 0.008
8
Brandvalue Brandval
ue
1 0.49457 0.09356 5.29 0.000
4
5 | P a g e
Source DF
Sum of
Squares
Mean
Square F Value Pr > F
Model 1 43.648
86
43.648
86
27.94 0.000
4
Error 10 15.620
31
1.5620
3
Corrected Total 11 59.269
17
Root MSE 1.2498
1
R-Square 0.736
5
Dependent Mean 4.6416
7
Adj R-Sq 0.710
1
Coeff Var 26.925
94
Parameter Estimates
Variable Label DF
Parameter
Estimate
Standard
Error t Value Pr > |t|
Intercept Intercept 1 2.00066 0.61626 3.25 0.008
8
Brandvalue Brandval
ue
1 0.49457 0.09356 5.29 0.000
4
5 | P a g e
Paraphrase This Document
Need a fresh take? Get an instant paraphrase of this document with our AI Paraphraser
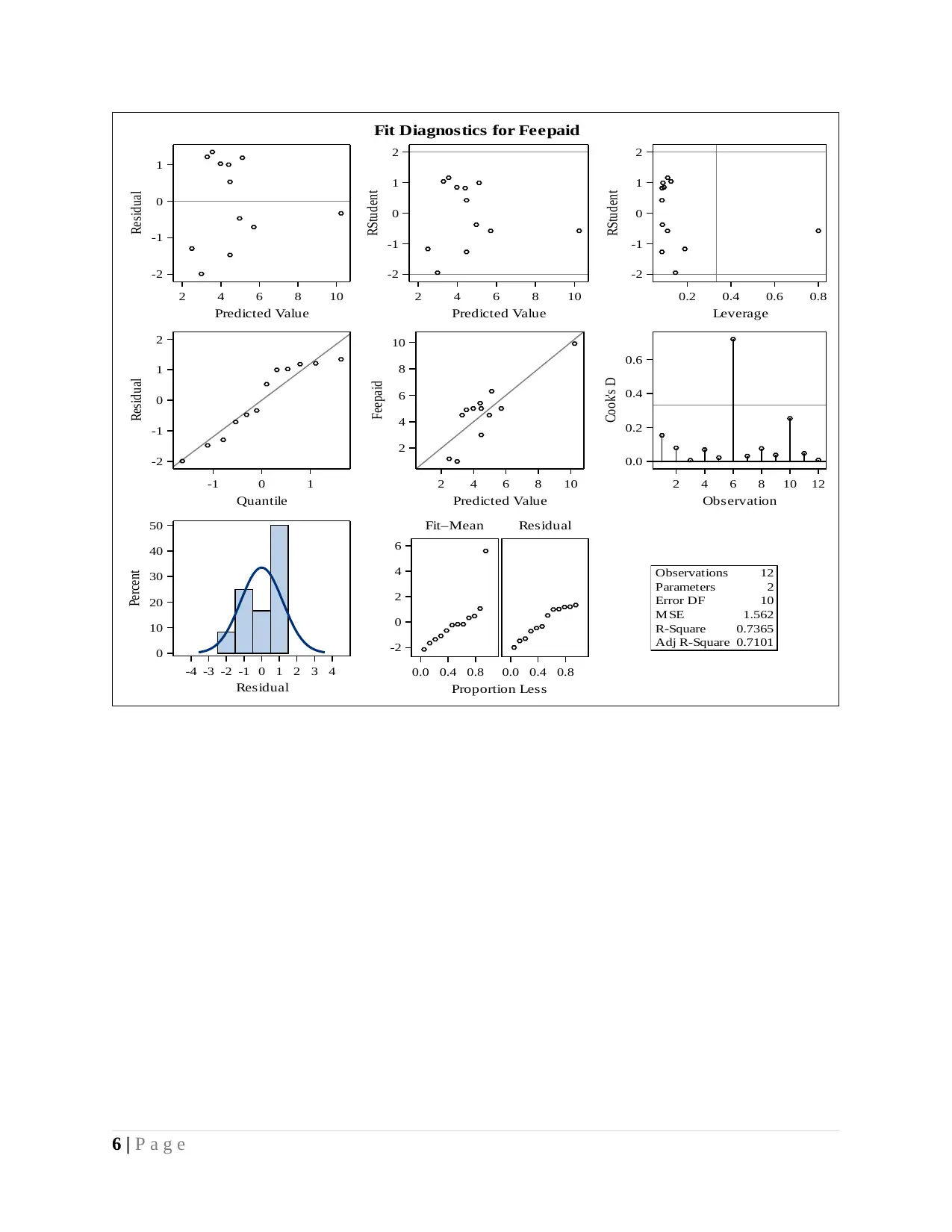
Fit Diagnostics for Feepaid
0.7101Adj R-Square
0.7365R-Square
1.562M SE
10Error DF
2Parameters
12Observations
Proportion Less
0.0 0.4 0.8
Residual
0.0 0.4 0.8
Fit–Mean
-2
0
2
4
6
-4 -3 -2 -1 0 1 2 3 4
Residual
0
10
20
30
40
50
Percent
2 4 6 8 10 12
Observation
0.0
0.2
0.4
0.6
Cook's D
2 4 6 8 10
Predicted Value
2
4
6
8
10
Feepaid
-1 0 1
Quantile
-2
-1
0
1
2
Residual
0.2 0.4 0.6 0.8
Leverage
-2
-1
0
1
2
RStudent
2 4 6 8 10
Predicted Value
-2
-1
0
1
2
RStudent
2 4 6 8 10
Predicted Value
-2
-1
0
1
Residual
6 | P a g e
0.7101Adj R-Square
0.7365R-Square
1.562M SE
10Error DF
2Parameters
12Observations
Proportion Less
0.0 0.4 0.8
Residual
0.0 0.4 0.8
Fit–Mean
-2
0
2
4
6
-4 -3 -2 -1 0 1 2 3 4
Residual
0
10
20
30
40
50
Percent
2 4 6 8 10 12
Observation
0.0
0.2
0.4
0.6
Cook's D
2 4 6 8 10
Predicted Value
2
4
6
8
10
Feepaid
-1 0 1
Quantile
-2
-1
0
1
2
Residual
0.2 0.4 0.6 0.8
Leverage
-2
-1
0
1
2
RStudent
2 4 6 8 10
Predicted Value
-2
-1
0
1
2
RStudent
2 4 6 8 10
Predicted Value
-2
-1
0
1
Residual
6 | P a g e
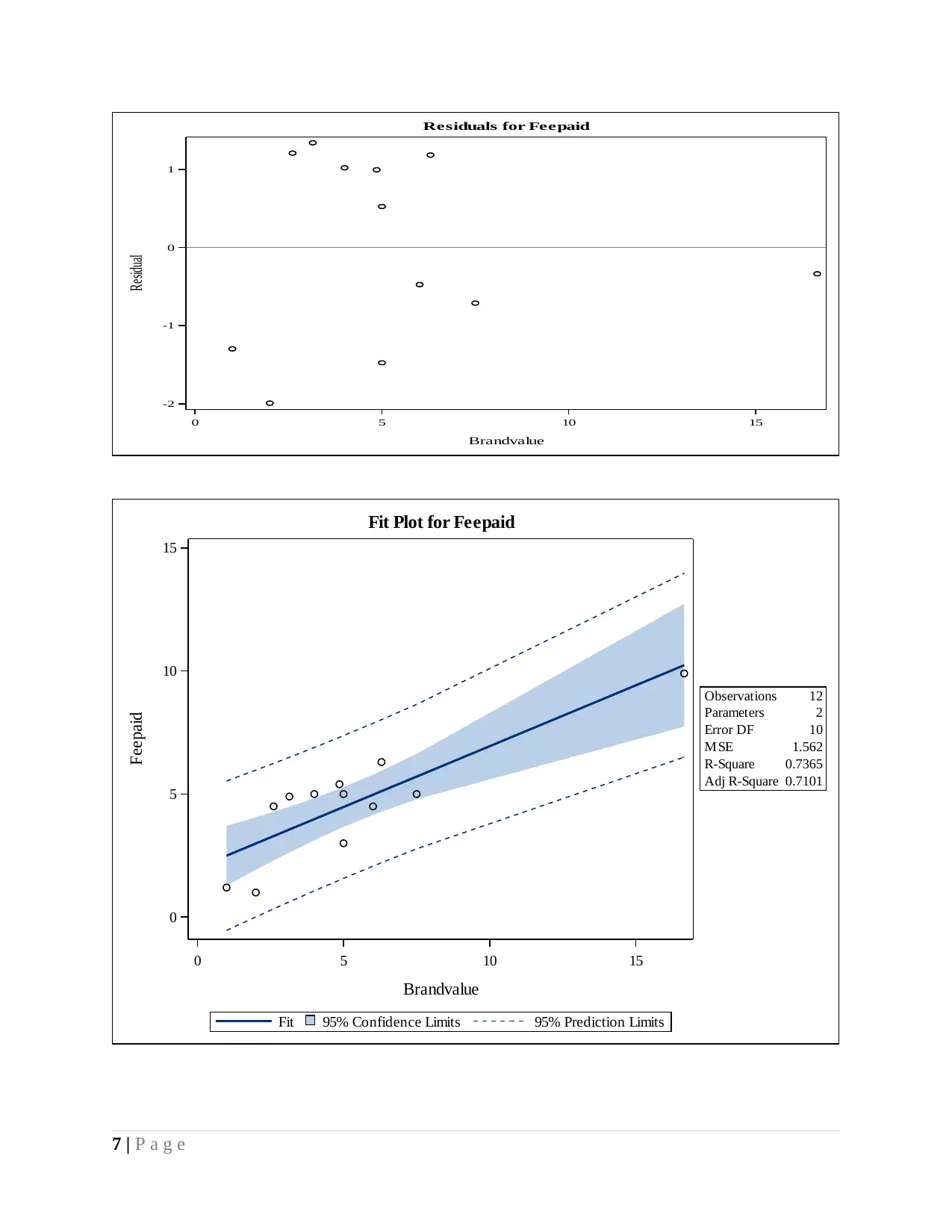
0 5 10 15
Brandvalue
-2
-1
0
1
Residual
Residuals for Feepaid
0 5 10 15
Brandvalue
0
5
10
15
Feepaid
95% Prediction Limits95% Confidence LimitsFit
0.7101Adj R-Square
0.7365R-Square
1.562M SE
10Error DF
2Parameters
12Observations
Fit Plot for Feepaid
7 | P a g e
Brandvalue
-2
-1
0
1
Residual
Residuals for Feepaid
0 5 10 15
Brandvalue
0
5
10
15
Feepaid
95% Prediction Limits95% Confidence LimitsFit
0.7101Adj R-Square
0.7365R-Square
1.562M SE
10Error DF
2Parameters
12Observations
Fit Plot for Feepaid
7 | P a g e
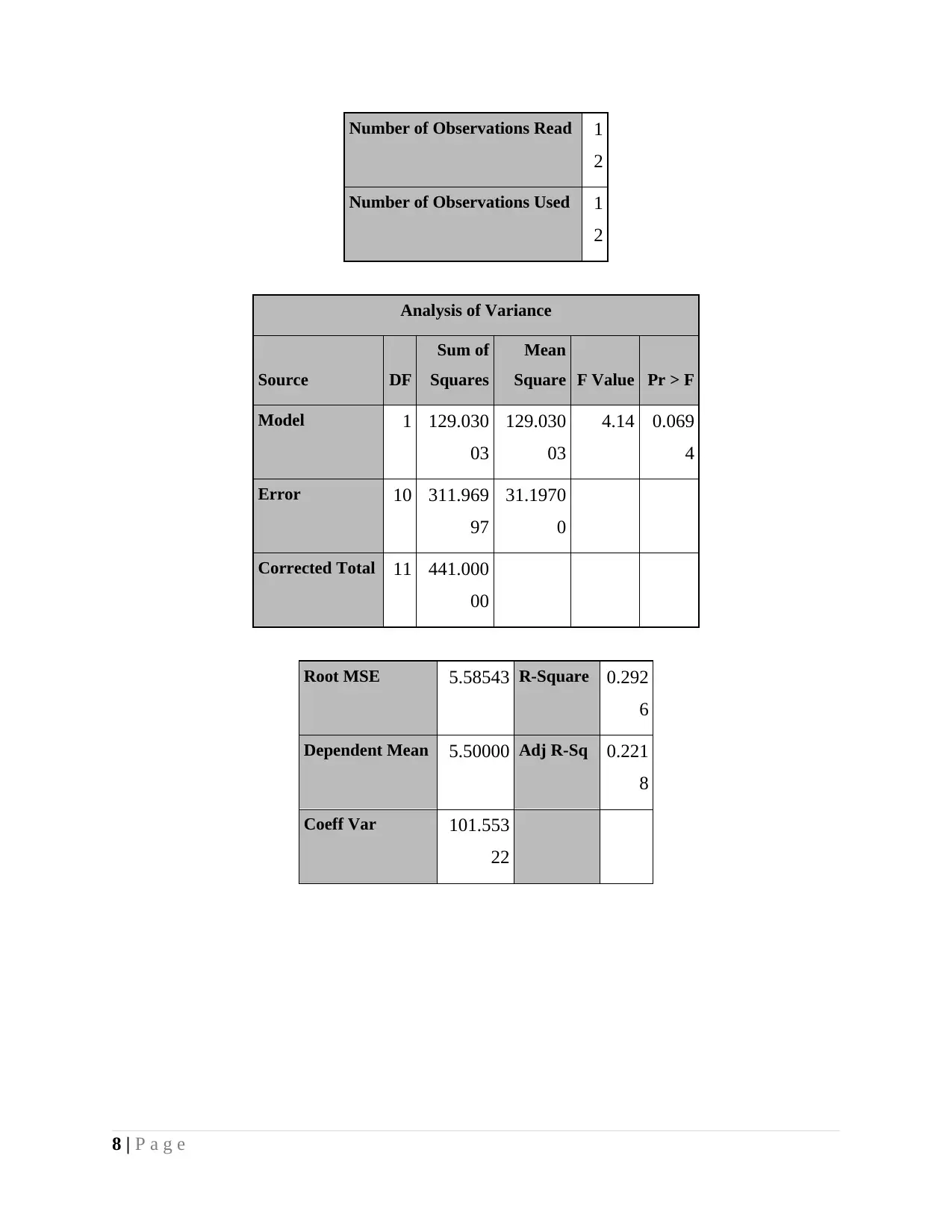
Number of Observations Read 1
2
Number of Observations Used 1
2
Analysis of Variance
Source DF
Sum of
Squares
Mean
Square F Value Pr > F
Model 1 129.030
03
129.030
03
4.14 0.069
4
Error 10 311.969
97
31.1970
0
Corrected Total 11 441.000
00
Root MSE 5.58543 R-Square 0.292
6
Dependent Mean 5.50000 Adj R-Sq 0.221
8
Coeff Var 101.553
22
8 | P a g e
2
Number of Observations Used 1
2
Analysis of Variance
Source DF
Sum of
Squares
Mean
Square F Value Pr > F
Model 1 129.030
03
129.030
03
4.14 0.069
4
Error 10 311.969
97
31.1970
0
Corrected Total 11 441.000
00
Root MSE 5.58543 R-Square 0.292
6
Dependent Mean 5.50000 Adj R-Sq 0.221
8
Coeff Var 101.553
22
8 | P a g e
Secure Best Marks with AI Grader
Need help grading? Try our AI Grader for instant feedback on your assignments.
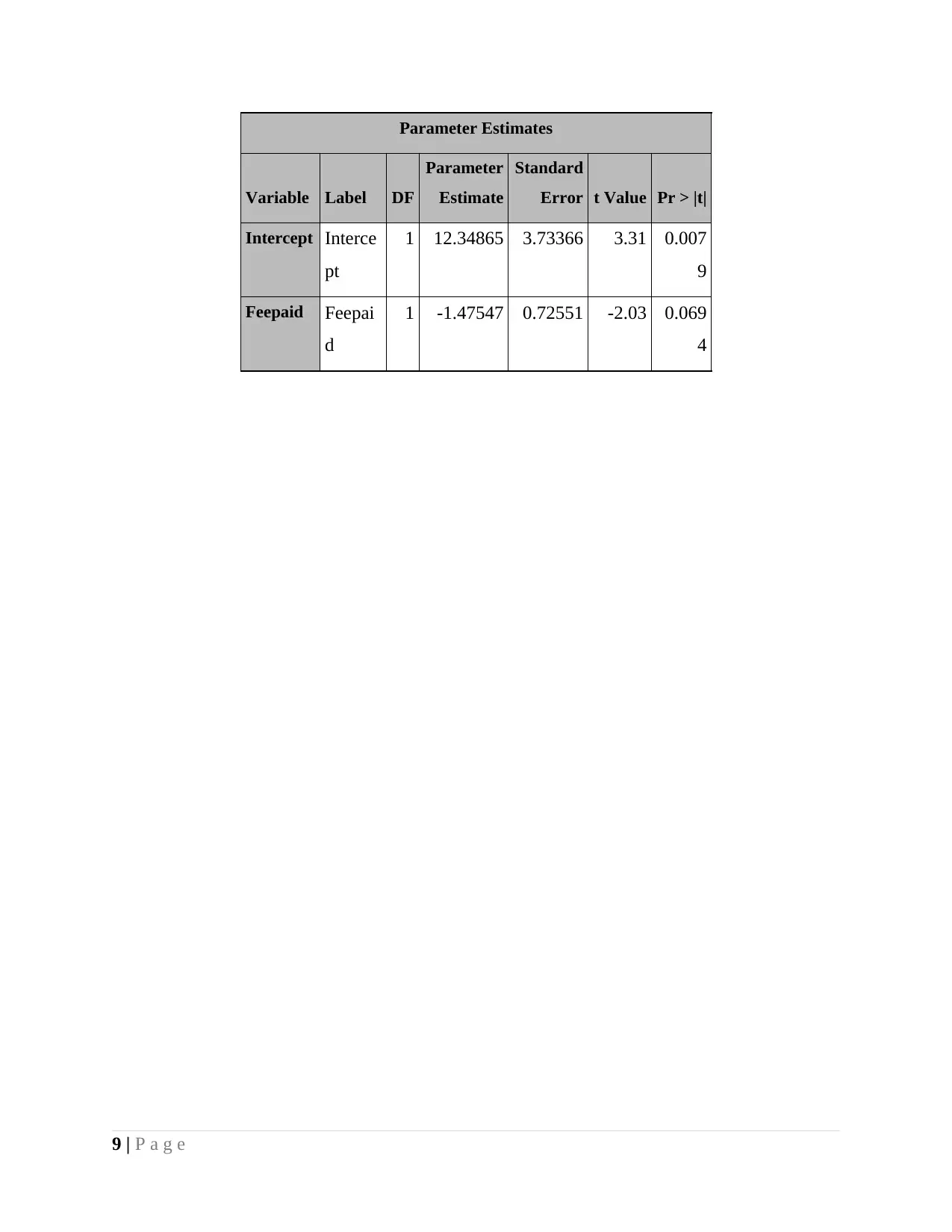
Parameter Estimates
Variable Label DF
Parameter
Estimate
Standard
Error t Value Pr > |t|
Intercept Interce
pt
1 12.34865 3.73366 3.31 0.007
9
Feepaid Feepai
d
1 -1.47547 0.72551 -2.03 0.069
4
9 | P a g e
Variable Label DF
Parameter
Estimate
Standard
Error t Value Pr > |t|
Intercept Interce
pt
1 12.34865 3.73366 3.31 0.007
9
Feepaid Feepai
d
1 -1.47547 0.72551 -2.03 0.069
4
9 | P a g e
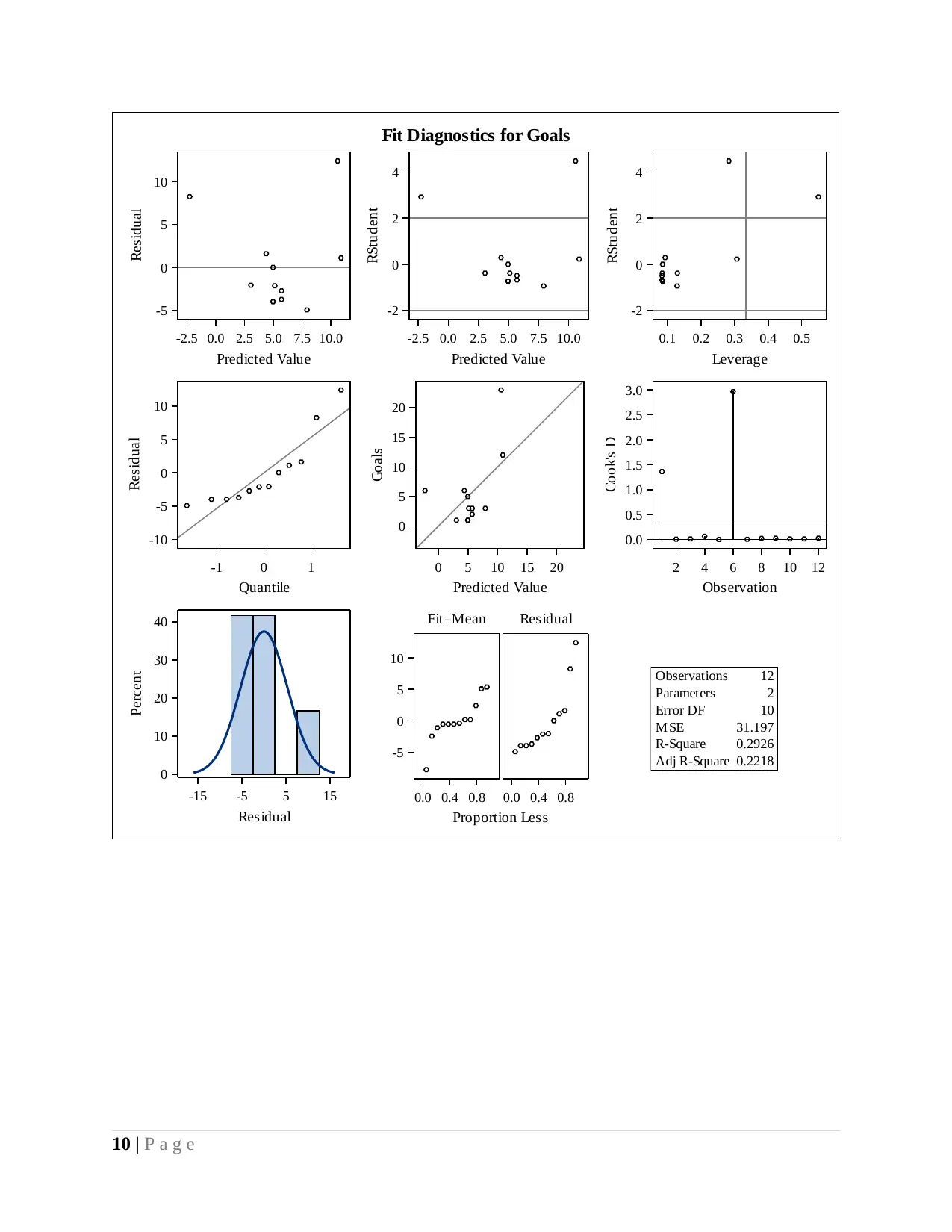
Fit Diagnostics for Goals
0.2218Adj R-Square
0.2926R-Square
31.197M SE
10Error DF
2Parameters
12Observations
Proportion Less
0.0 0.4 0.8
Residual
0.0 0.4 0.8
Fit–Mean
-5
0
5
10
-15 -5 5 15
Residual
0
10
20
30
40
Percent
2 4 6 8 10 12
Observation
0.0
0.5
1.0
1.5
2.0
2.5
3.0
Cook's D
0 5 10 15 20
Predicted Value
0
5
10
15
20
Goals
-1 0 1
Quantile
-10
-5
0
5
10
Residual
0.1 0.2 0.3 0.4 0.5
Leverage
-2
0
2
4
RStudent
-2.5 0.0 2.5 5.0 7.5 10.0
Predicted Value
-2
0
2
4
RStudent
-2.5 0.0 2.5 5.0 7.5 10.0
Predicted Value
-5
0
5
10
Residual
10 | P a g e
0.2218Adj R-Square
0.2926R-Square
31.197M SE
10Error DF
2Parameters
12Observations
Proportion Less
0.0 0.4 0.8
Residual
0.0 0.4 0.8
Fit–Mean
-5
0
5
10
-15 -5 5 15
Residual
0
10
20
30
40
Percent
2 4 6 8 10 12
Observation
0.0
0.5
1.0
1.5
2.0
2.5
3.0
Cook's D
0 5 10 15 20
Predicted Value
0
5
10
15
20
Goals
-1 0 1
Quantile
-10
-5
0
5
10
Residual
0.1 0.2 0.3 0.4 0.5
Leverage
-2
0
2
4
RStudent
-2.5 0.0 2.5 5.0 7.5 10.0
Predicted Value
-2
0
2
4
RStudent
-2.5 0.0 2.5 5.0 7.5 10.0
Predicted Value
-5
0
5
10
Residual
10 | P a g e
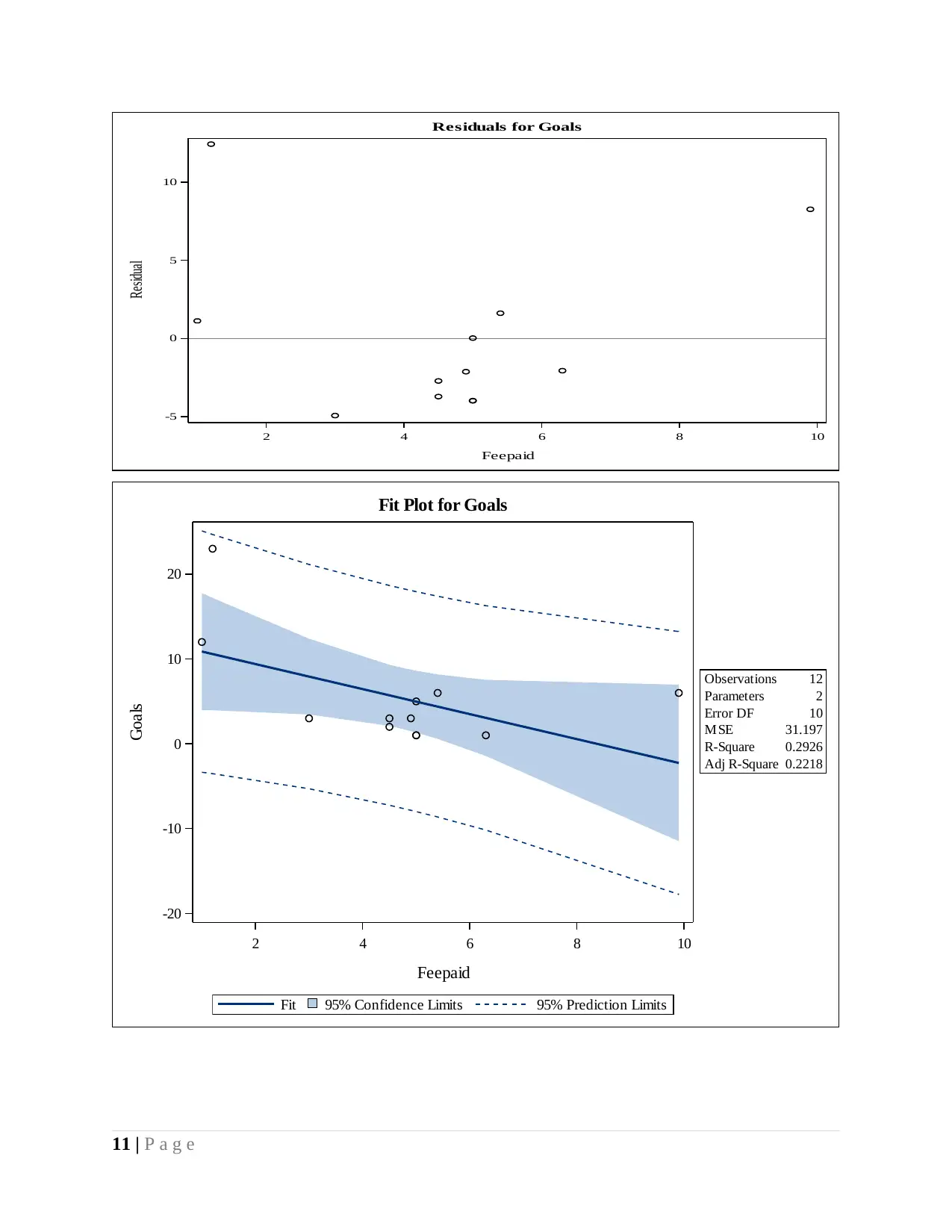
2 4 6 8 10
Feepaid
-5
0
5
10
Residual
Residuals for Goals
2 4 6 8 10
Feepaid
-20
-10
0
10
20
Goals
95% Prediction Limits95% Confidence LimitsFit
0.2218Adj R-Square
0.2926R-Square
31.197M SE
10Error DF
2Parameters
12Observations
Fit Plot for Goals
11 | P a g e
Feepaid
-5
0
5
10
Residual
Residuals for Goals
2 4 6 8 10
Feepaid
-20
-10
0
10
20
Goals
95% Prediction Limits95% Confidence LimitsFit
0.2218Adj R-Square
0.2926R-Square
31.197M SE
10Error DF
2Parameters
12Observations
Fit Plot for Goals
11 | P a g e
Paraphrase This Document
Need a fresh take? Get an instant paraphrase of this document with our AI Paraphraser
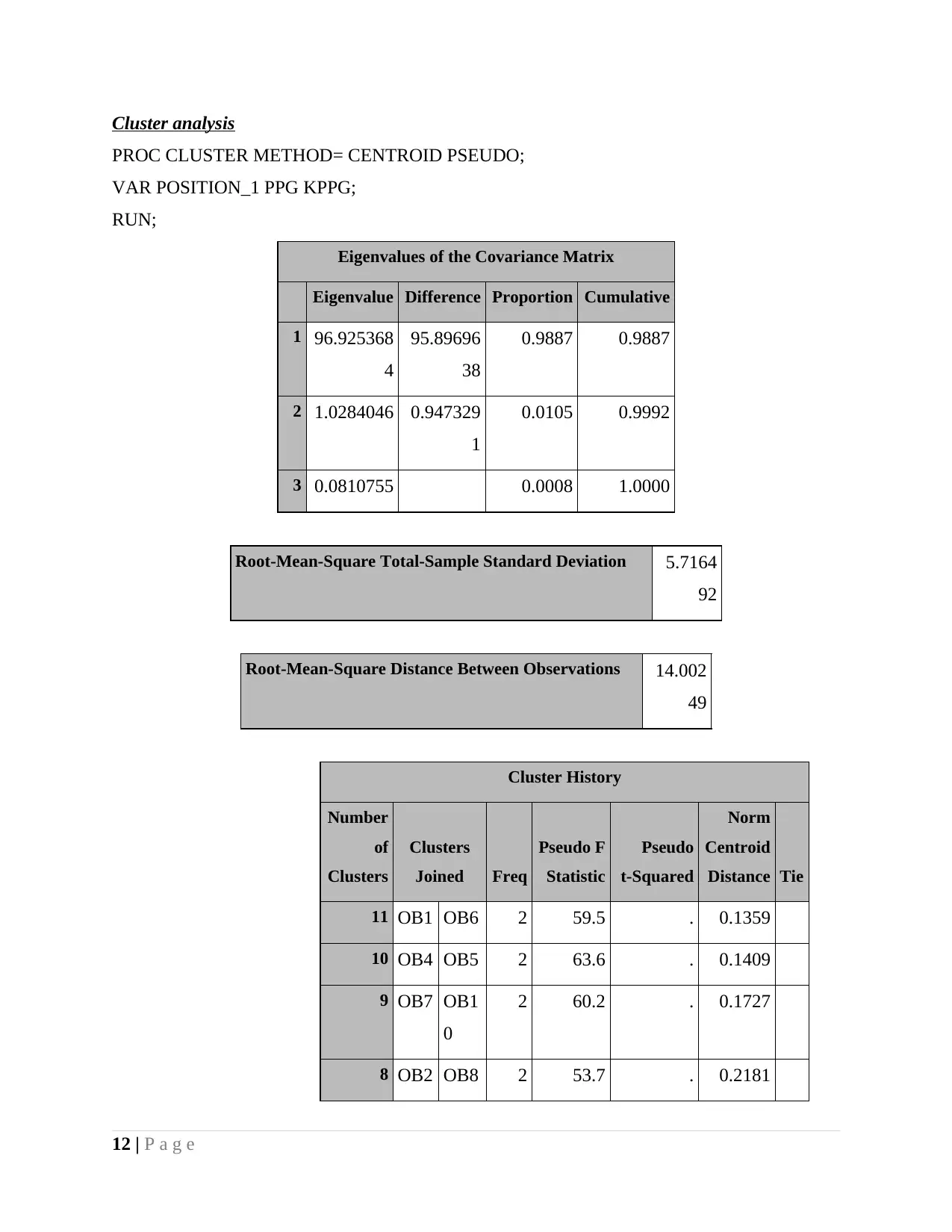
Cluster analysis
PROC CLUSTER METHOD= CENTROID PSEUDO;
VAR POSITION_1 PPG KPPG;
RUN;
Eigenvalues of the Covariance Matrix
Eigenvalue Difference Proportion Cumulative
1 96.925368
4
95.89696
38
0.9887 0.9887
2 1.0284046 0.947329
1
0.0105 0.9992
3 0.0810755 0.0008 1.0000
Root-Mean-Square Total-Sample Standard Deviation 5.7164
92
Root-Mean-Square Distance Between Observations 14.002
49
Cluster History
Number
of
Clusters
Clusters
Joined Freq
Pseudo F
Statistic
Pseudo
t-Squared
Norm
Centroid
Distance Tie
11 OB1 OB6 2 59.5 . 0.1359
10 OB4 OB5 2 63.6 . 0.1409
9 OB7 OB1
0
2 60.2 . 0.1727
8 OB2 OB8 2 53.7 . 0.2181
12 | P a g e
PROC CLUSTER METHOD= CENTROID PSEUDO;
VAR POSITION_1 PPG KPPG;
RUN;
Eigenvalues of the Covariance Matrix
Eigenvalue Difference Proportion Cumulative
1 96.925368
4
95.89696
38
0.9887 0.9887
2 1.0284046 0.947329
1
0.0105 0.9992
3 0.0810755 0.0008 1.0000
Root-Mean-Square Total-Sample Standard Deviation 5.7164
92
Root-Mean-Square Distance Between Observations 14.002
49
Cluster History
Number
of
Clusters
Clusters
Joined Freq
Pseudo F
Statistic
Pseudo
t-Squared
Norm
Centroid
Distance Tie
11 OB1 OB6 2 59.5 . 0.1359
10 OB4 OB5 2 63.6 . 0.1409
9 OB7 OB1
0
2 60.2 . 0.1727
8 OB2 OB8 2 53.7 . 0.2181
12 | P a g e
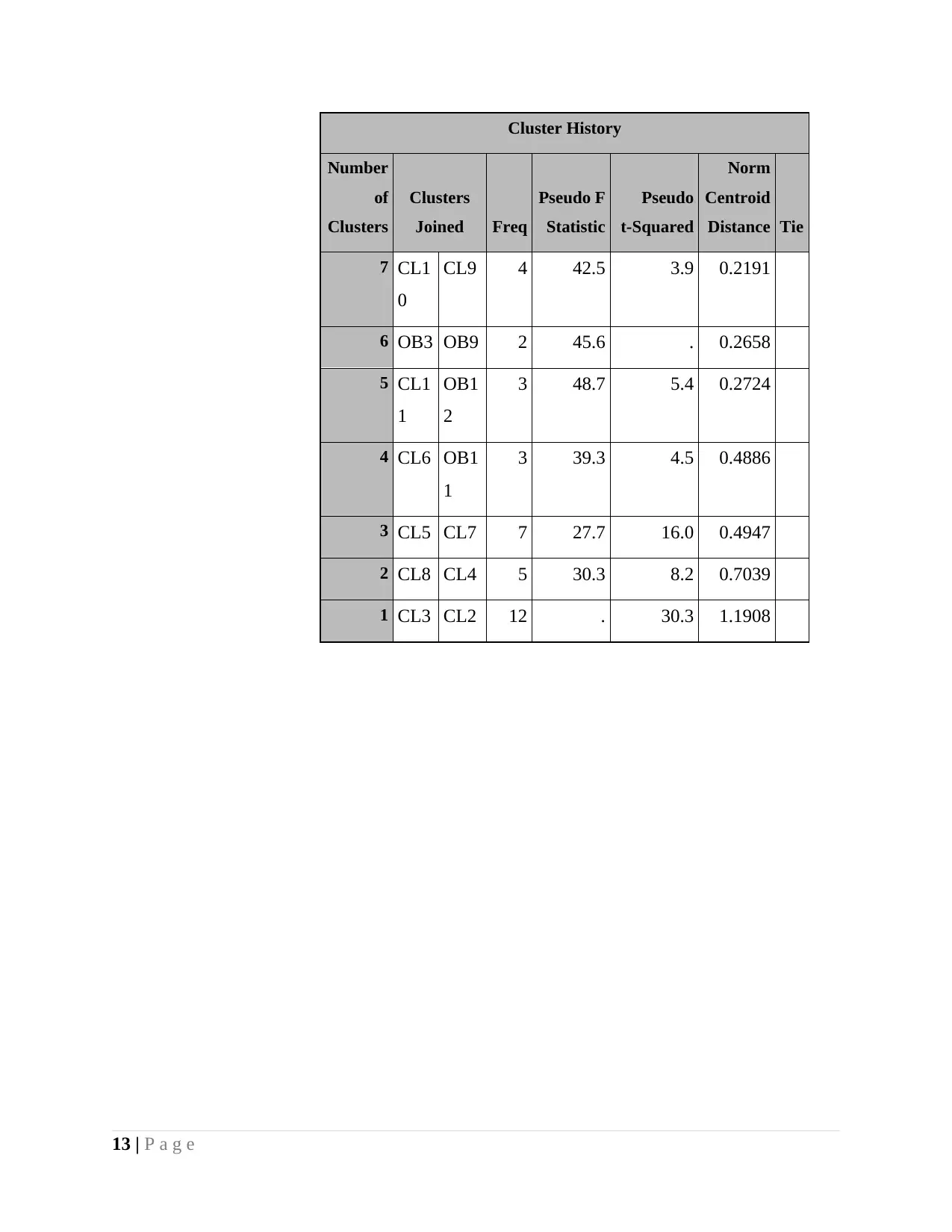
Cluster History
Number
of
Clusters
Clusters
Joined Freq
Pseudo F
Statistic
Pseudo
t-Squared
Norm
Centroid
Distance Tie
7 CL1
0
CL9 4 42.5 3.9 0.2191
6 OB3 OB9 2 45.6 . 0.2658
5 CL1
1
OB1
2
3 48.7 5.4 0.2724
4 CL6 OB1
1
3 39.3 4.5 0.4886
3 CL5 CL7 7 27.7 16.0 0.4947
2 CL8 CL4 5 30.3 8.2 0.7039
1 CL3 CL2 12 . 30.3 1.1908
13 | P a g e
Number
of
Clusters
Clusters
Joined Freq
Pseudo F
Statistic
Pseudo
t-Squared
Norm
Centroid
Distance Tie
7 CL1
0
CL9 4 42.5 3.9 0.2191
6 OB3 OB9 2 45.6 . 0.2658
5 CL1
1
OB1
2
3 48.7 5.4 0.2724
4 CL6 OB1
1
3 39.3 4.5 0.4886
3 CL5 CL7 7 27.7 16.0 0.4947
2 CL8 CL4 5 30.3 8.2 0.7039
1 CL3 CL2 12 . 30.3 1.1908
13 | P a g e
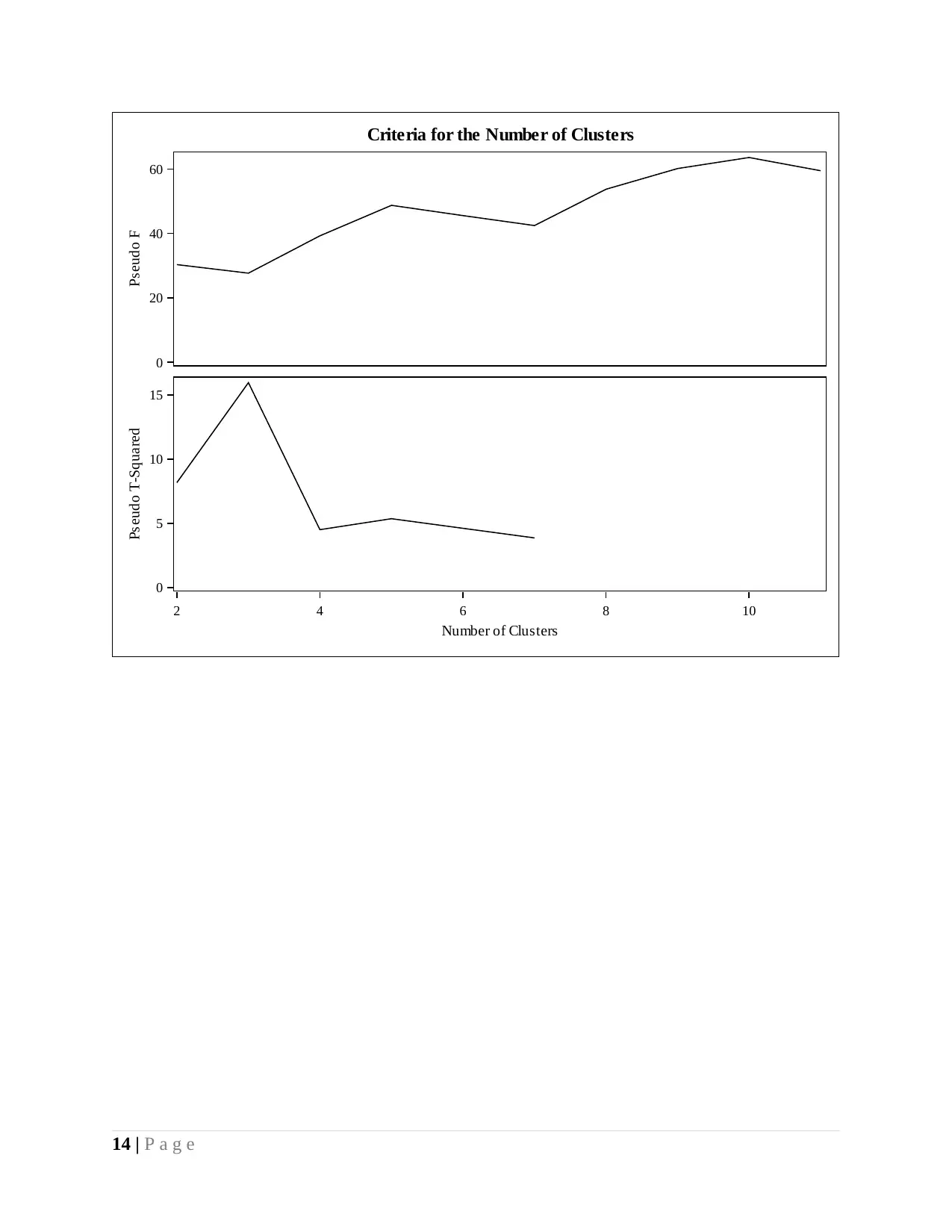
Criteria for the Number of Clusters
0
5
10
15
Pseudo T-Squared
0
20
40
60
Pseudo F
2 4 6 8 10
Number of Clusters
14 | P a g e
0
5
10
15
Pseudo T-Squared
0
20
40
60
Pseudo F
2 4 6 8 10
Number of Clusters
14 | P a g e
Secure Best Marks with AI Grader
Need help grading? Try our AI Grader for instant feedback on your assignments.
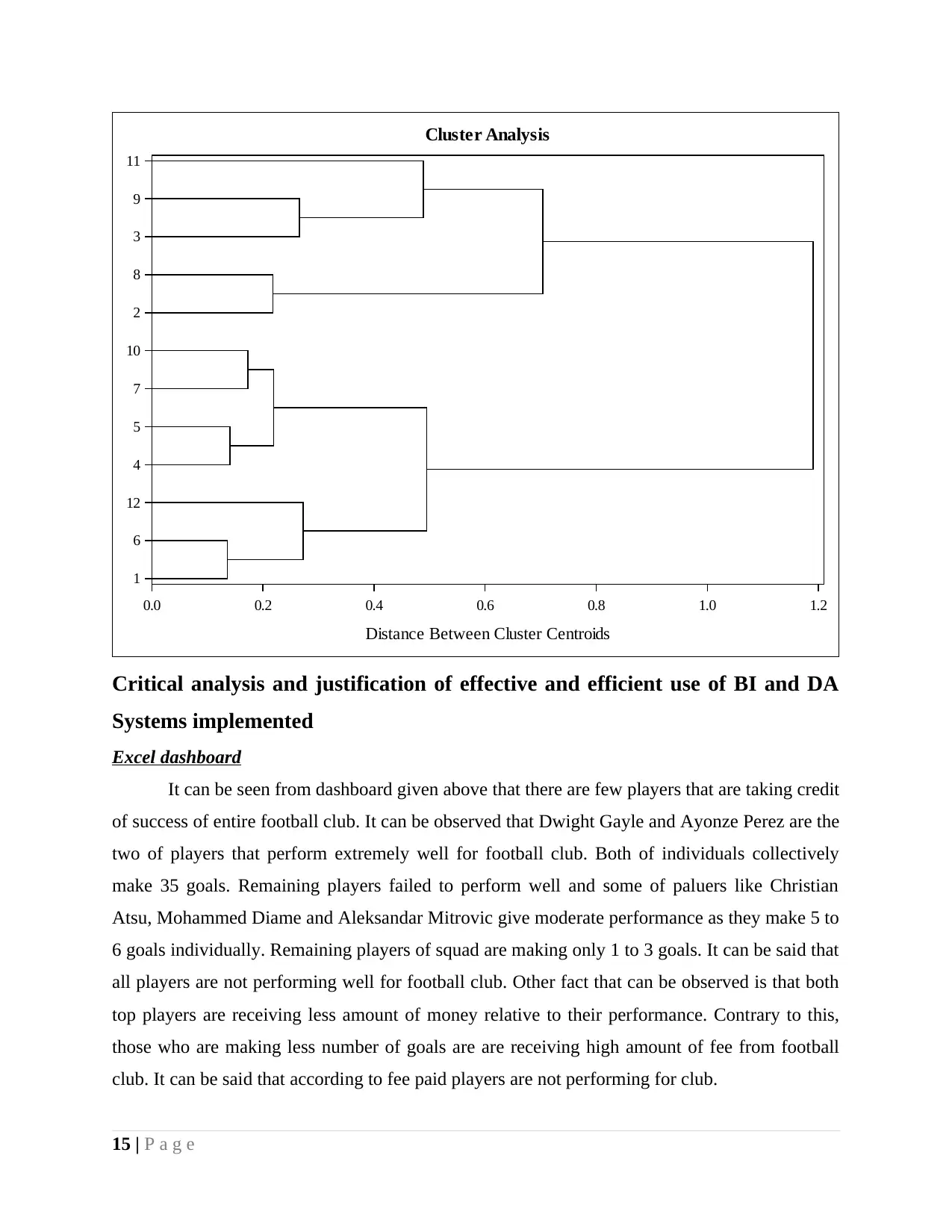
0.0 0.2 0.4 0.6 0.8 1.0 1.2
Distance Between Cluster Centroids
1
6
12
4
5
7
10
2
8
3
9
11
Cluster Analysis
Critical analysis and justification of effective and efficient use of BI and DA
Systems implemented
Excel dashboard
It can be seen from dashboard given above that there are few players that are taking credit
of success of entire football club. It can be observed that Dwight Gayle and Ayonze Perez are the
two of players that perform extremely well for football club. Both of individuals collectively
make 35 goals. Remaining players failed to perform well and some of paluers like Christian
Atsu, Mohammed Diame and Aleksandar Mitrovic give moderate performance as they make 5 to
6 goals individually. Remaining players of squad are making only 1 to 3 goals. It can be said that
all players are not performing well for football club. Other fact that can be observed is that both
top players are receiving less amount of money relative to their performance. Contrary to this,
those who are making less number of goals are are receiving high amount of fee from football
club. It can be said that according to fee paid players are not performing for club.
15 | P a g e
Distance Between Cluster Centroids
1
6
12
4
5
7
10
2
8
3
9
11
Cluster Analysis
Critical analysis and justification of effective and efficient use of BI and DA
Systems implemented
Excel dashboard
It can be seen from dashboard given above that there are few players that are taking credit
of success of entire football club. It can be observed that Dwight Gayle and Ayonze Perez are the
two of players that perform extremely well for football club. Both of individuals collectively
make 35 goals. Remaining players failed to perform well and some of paluers like Christian
Atsu, Mohammed Diame and Aleksandar Mitrovic give moderate performance as they make 5 to
6 goals individually. Remaining players of squad are making only 1 to 3 goals. It can be said that
all players are not performing well for football club. Other fact that can be observed is that both
top players are receiving less amount of money relative to their performance. Contrary to this,
those who are making less number of goals are are receiving high amount of fee from football
club. It can be said that according to fee paid players are not performing for club.
15 | P a g e
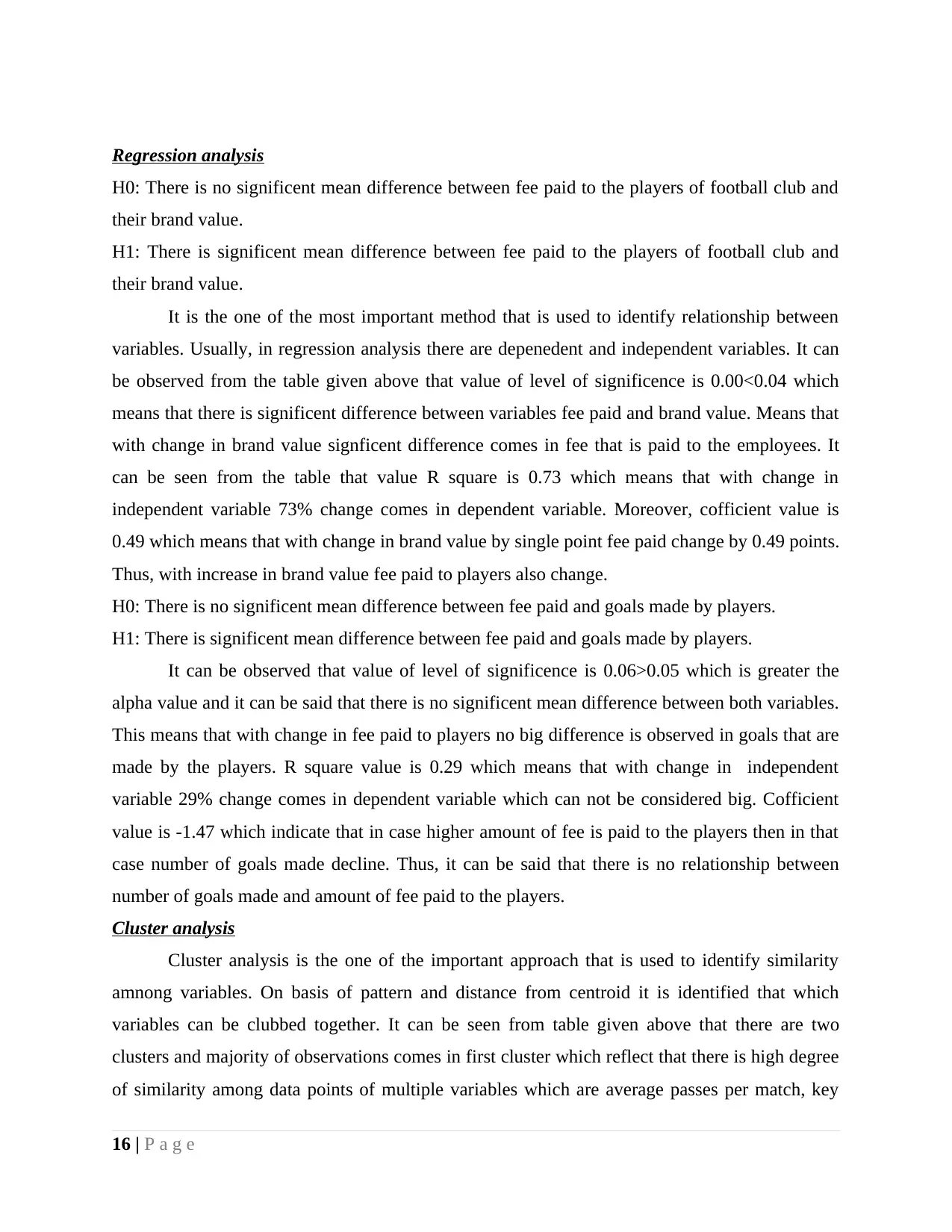
Regression analysis
H0: There is no significent mean difference between fee paid to the players of football club and
their brand value.
H1: There is significent mean difference between fee paid to the players of football club and
their brand value.
It is the one of the most important method that is used to identify relationship between
variables. Usually, in regression analysis there are depenedent and independent variables. It can
be observed from the table given above that value of level of significence is 0.00<0.04 which
means that there is significent difference between variables fee paid and brand value. Means that
with change in brand value signficent difference comes in fee that is paid to the employees. It
can be seen from the table that value R square is 0.73 which means that with change in
independent variable 73% change comes in dependent variable. Moreover, cofficient value is
0.49 which means that with change in brand value by single point fee paid change by 0.49 points.
Thus, with increase in brand value fee paid to players also change.
H0: There is no significent mean difference between fee paid and goals made by players.
H1: There is significent mean difference between fee paid and goals made by players.
It can be observed that value of level of significence is 0.06>0.05 which is greater the
alpha value and it can be said that there is no significent mean difference between both variables.
This means that with change in fee paid to players no big difference is observed in goals that are
made by the players. R square value is 0.29 which means that with change in independent
variable 29% change comes in dependent variable which can not be considered big. Cofficient
value is -1.47 which indicate that in case higher amount of fee is paid to the players then in that
case number of goals made decline. Thus, it can be said that there is no relationship between
number of goals made and amount of fee paid to the players.
Cluster analysis
Cluster analysis is the one of the important approach that is used to identify similarity
amnong variables. On basis of pattern and distance from centroid it is identified that which
variables can be clubbed together. It can be seen from table given above that there are two
clusters and majority of observations comes in first cluster which reflect that there is high degree
of similarity among data points of multiple variables which are average passes per match, key
16 | P a g e
H0: There is no significent mean difference between fee paid to the players of football club and
their brand value.
H1: There is significent mean difference between fee paid to the players of football club and
their brand value.
It is the one of the most important method that is used to identify relationship between
variables. Usually, in regression analysis there are depenedent and independent variables. It can
be observed from the table given above that value of level of significence is 0.00<0.04 which
means that there is significent difference between variables fee paid and brand value. Means that
with change in brand value signficent difference comes in fee that is paid to the employees. It
can be seen from the table that value R square is 0.73 which means that with change in
independent variable 73% change comes in dependent variable. Moreover, cofficient value is
0.49 which means that with change in brand value by single point fee paid change by 0.49 points.
Thus, with increase in brand value fee paid to players also change.
H0: There is no significent mean difference between fee paid and goals made by players.
H1: There is significent mean difference between fee paid and goals made by players.
It can be observed that value of level of significence is 0.06>0.05 which is greater the
alpha value and it can be said that there is no significent mean difference between both variables.
This means that with change in fee paid to players no big difference is observed in goals that are
made by the players. R square value is 0.29 which means that with change in independent
variable 29% change comes in dependent variable which can not be considered big. Cofficient
value is -1.47 which indicate that in case higher amount of fee is paid to the players then in that
case number of goals made decline. Thus, it can be said that there is no relationship between
number of goals made and amount of fee paid to the players.
Cluster analysis
Cluster analysis is the one of the important approach that is used to identify similarity
amnong variables. On basis of pattern and distance from centroid it is identified that which
variables can be clubbed together. It can be seen from table given above that there are two
clusters and majority of observations comes in first cluster which reflect that there is high degree
of similarity among data points of multiple variables which are average passes per match, key
16 | P a g e
1 out of 18
Related Documents
![[object Object]](/_next/image/?url=%2F_next%2Fstatic%2Fmedia%2Flogo.6d15ce61.png&w=640&q=75)
Your All-in-One AI-Powered Toolkit for Academic Success.
+13062052269
info@desklib.com
Available 24*7 on WhatsApp / Email
Unlock your academic potential
© 2024 | Zucol Services PVT LTD | All rights reserved.