MIS775: Investment Portfolio Optimisation with Decision Models
VerifiedAdded on 2022/09/22
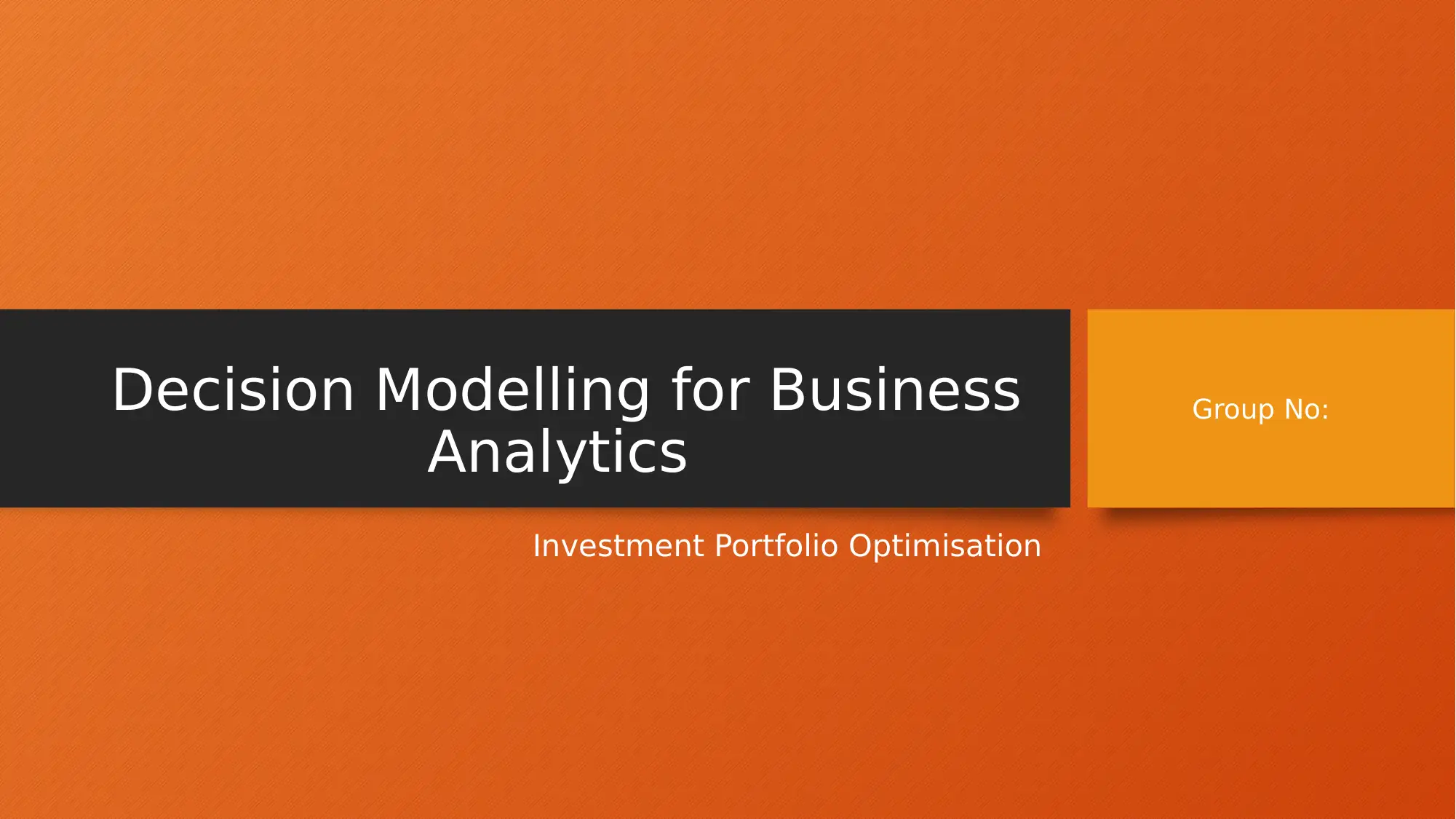
Analytics
Investment Portfolio Optimisation
Group No:
Paraphrase This Document
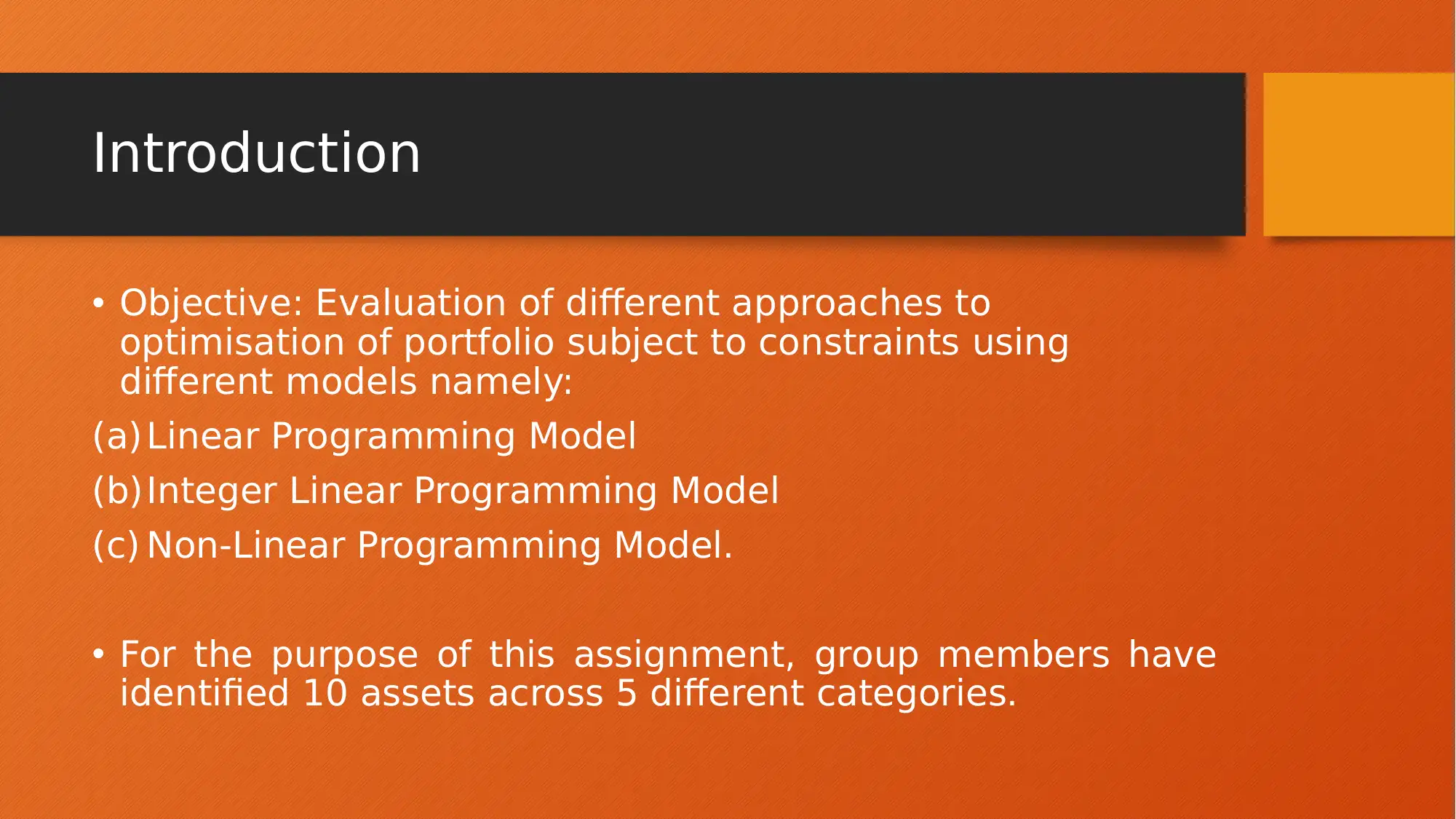
• Objective: Evaluation of different approaches to
optimisation of portfolio subject to constraints using
different models namely:
(a) Linear Programming Model
(b)Integer Linear Programming Model
(c) Non-Linear Programming Model.
• For the purpose of this assignment, group members have
identified 10 assets across 5 different categories.
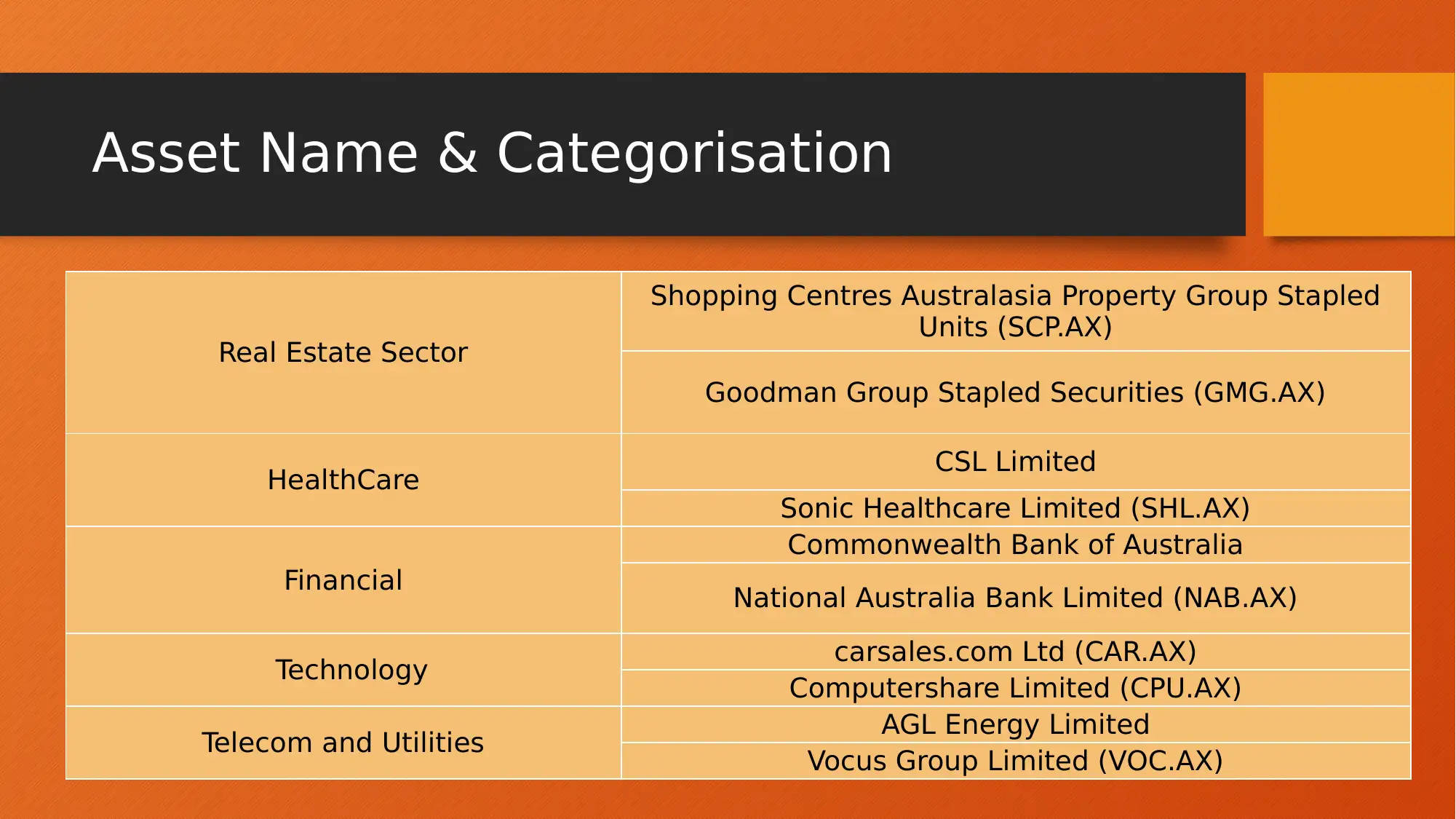
Real Estate Sector
Shopping Centres Australasia Property Group Stapled
Units (SCP.AX)
Goodman Group Stapled Securities (GMG.AX)
HealthCare CSL Limited
Sonic Healthcare Limited (SHL.AX)
Financial
Commonwealth Bank of Australia
National Australia Bank Limited (NAB.AX)
Technology carsales.com Ltd (CAR.AX)
Computershare Limited (CPU.AX)
Telecom and Utilities AGL Energy Limited
Vocus Group Limited (VOC.AX)
⊘ This is a preview!⊘
Do you want full access?
Subscribe today to unlock all pages.

Trusted by 1+ million students worldwide
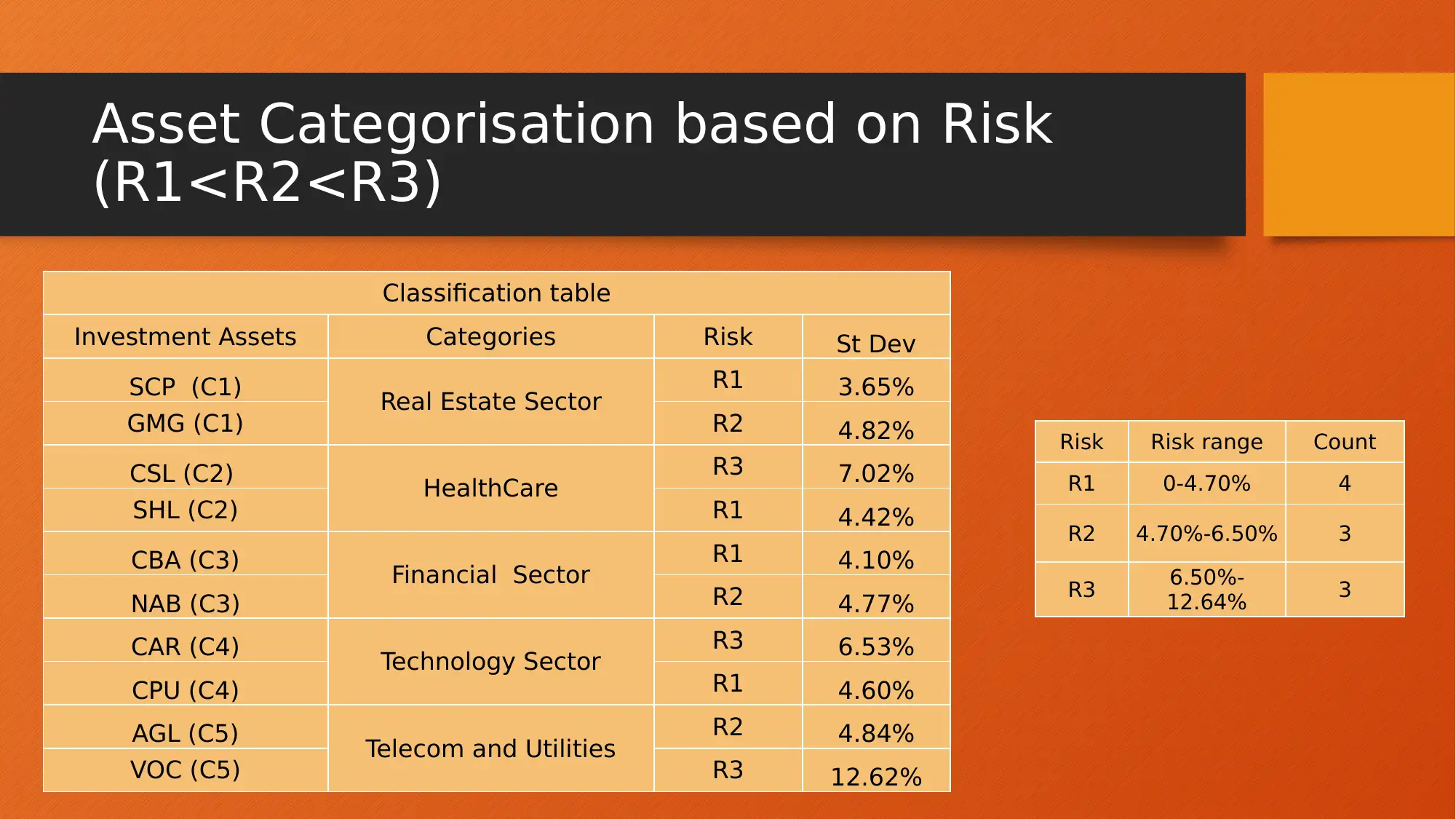
(R1<R2<R3)
Classification table
Investment Assets Categories Risk St Dev
SCP (C1) Real Estate Sector R1 3.65%
GMG (C1) R2 4.82%
CSL (C2) HealthCare R3 7.02%
SHL (C2) R1 4.42%
CBA (C3) Financial Sector R1 4.10%
NAB (C3) R2 4.77%
CAR (C4) Technology Sector R3 6.53%
CPU (C4) R1 4.60%
AGL (C5) Telecom and Utilities R2 4.84%
VOC (C5) R3 12.62%
Risk Risk range Count
R1 0-4.70% 4
R2 4.70%-6.50% 3
R3 6.50%-
12.64% 3
Paraphrase This Document

• The financial stock values for each of these 10 assets were collected over a period of 37
months
• Using this data we calculated the following:
(a) Average and monthly returns for each stock
(b) Average and monthly risk associated with each stock
(c) Average annual return for each stock
• In addition to this, all assets chosen satisfy the following general constraints:
(a) 37 months of data should be collected for each of these assets up to and including
Feb 2020.
(b) The assets should be selected from 5 different categories and there should 2 assets
in each category.
(c)These assets must span a reasonable range of risks and must be classified into 3 risk
groups (R1, R2, R3), with R1 being the least volatile and R4 being the most volatile.
(d) Each risk group must contains at least 3 assets.

Objective & Constraint
Constraints
Total Weight = 100%
Technology <= 20%
Real Estate <= 25%
Health = 10%
Financial <= 30%
Telcom ≤ 30%
R1 <= 20%
R2 <= 15%
R3 >= 10%
Objective: Maximisation of return of portfolio subject to constraint stated
here-in-below
⊘ This is a preview!⊘
Do you want full access?
Subscribe today to unlock all pages.

Trusted by 1+ million students worldwide
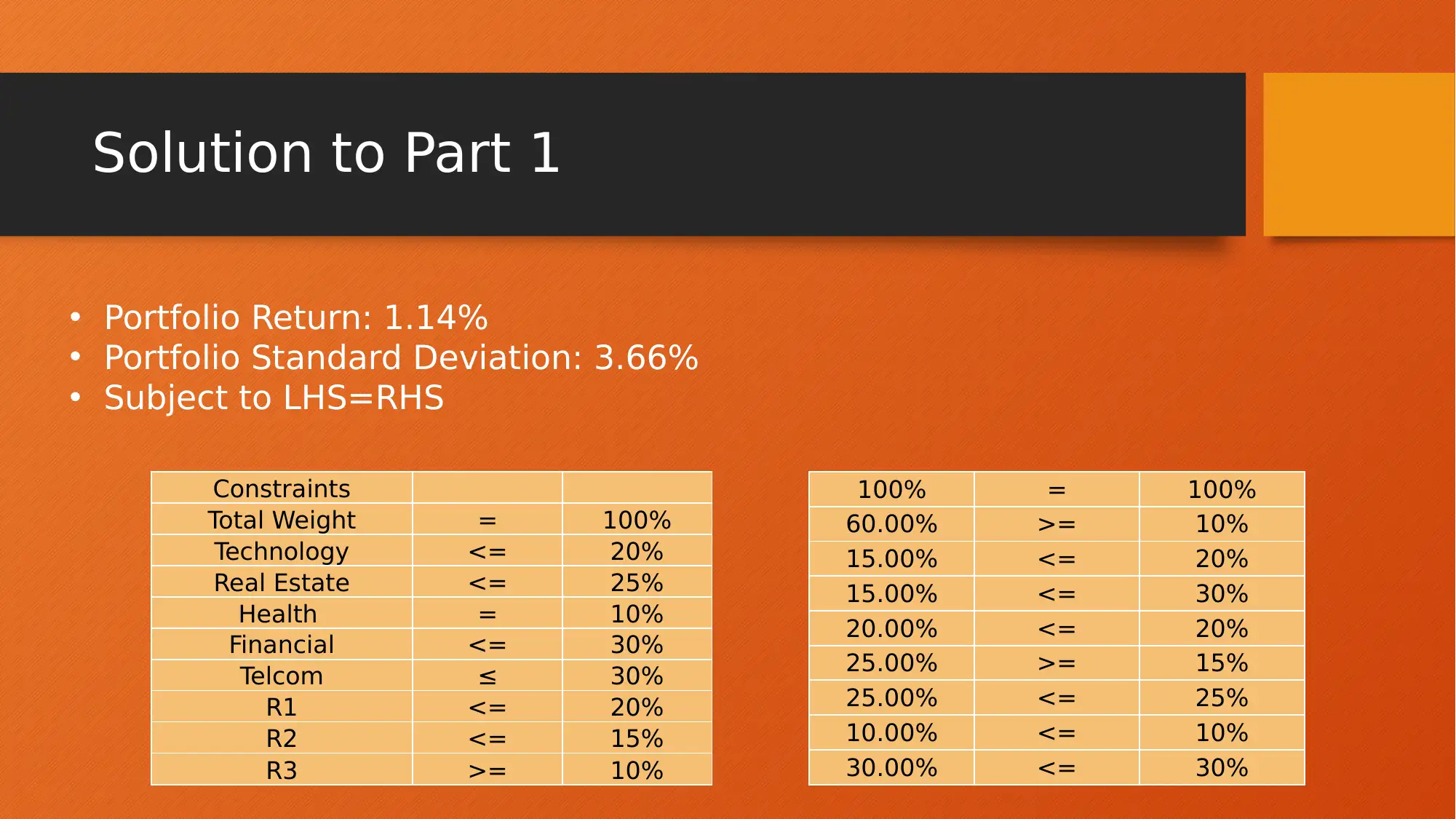
• Portfolio Return: 1.14%
• Portfolio Standard Deviation: 3.66%
• Subject to LHS=RHS
100% = 100%
60.00% >= 10%
15.00% <= 20%
15.00% <= 30%
20.00% <= 20%
25.00% >= 15%
25.00% <= 25%
10.00% <= 10%
30.00% <= 30%
Constraints
Total Weight = 100%
Technology <= 20%
Real Estate <= 25%
Health = 10%
Financial <= 30%
Telcom ≤ 30%
R1 <= 20%
R2 <= 15%
R3 >= 10%
Paraphrase This Document
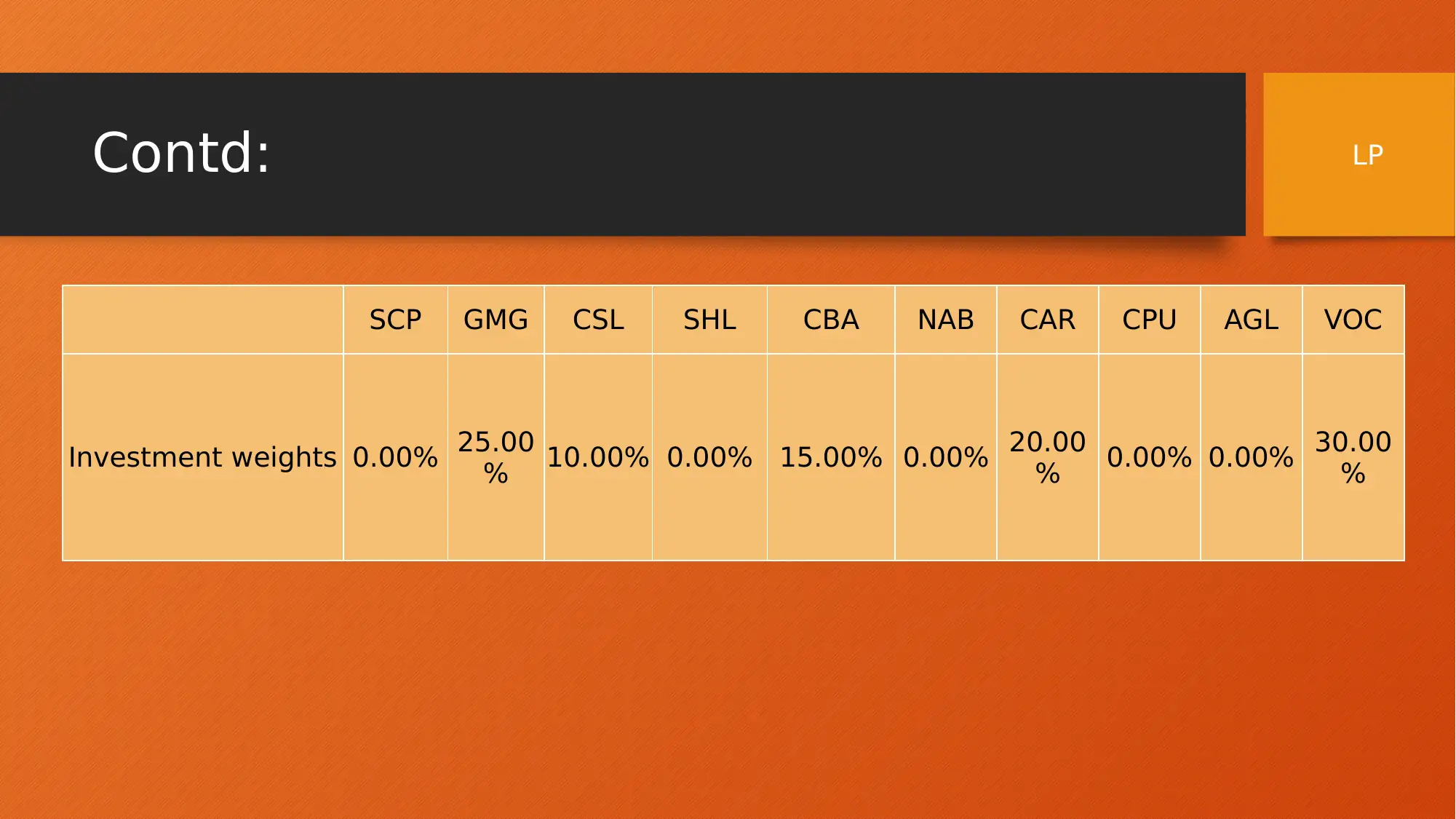
SCP GMG CSL SHL CBA NAB CAR CPU AGL VOC
Investment weights 0.00% 25.00
% 10.00% 0.00% 15.00% 0.00% 20.00
% 0.00% 0.00% 30.00
%
LP
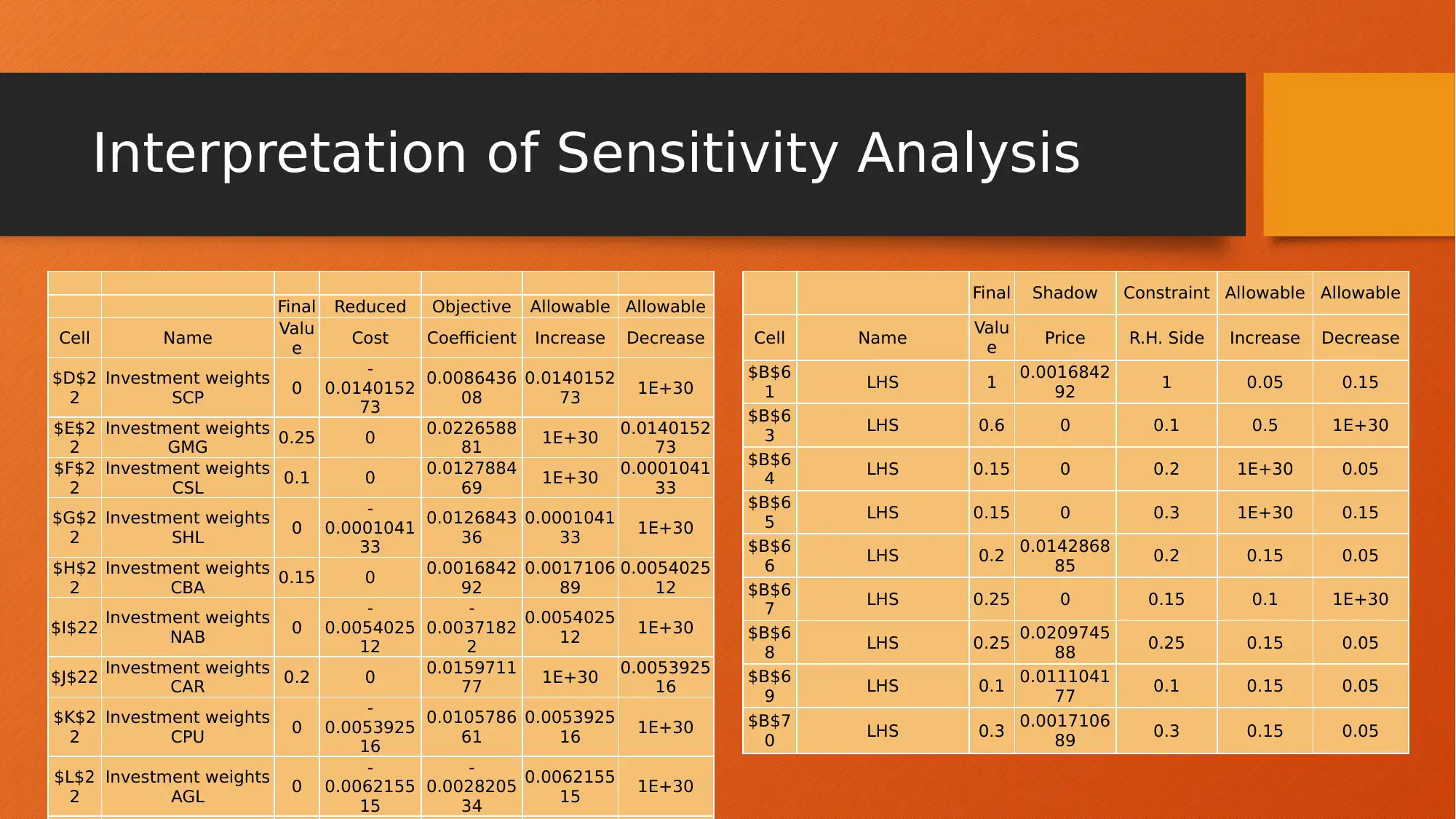
Final Reduced Objective Allowable Allowable
Cell Name Valu
e Cost Coefficient Increase Decrease
$D$2
2
Investment weights
SCP 0
-
0.0140152
73
0.0086436
08
0.0140152
73 1E+30
$E$2
2
Investment weights
GMG 0.25 0 0.0226588
81 1E+30 0.0140152
73
$F$2
2
Investment weights
CSL 0.1 0 0.0127884
69 1E+30 0.0001041
33
$G$2
2
Investment weights
SHL 0
-
0.0001041
33
0.0126843
36
0.0001041
33 1E+30
$H$2
2
Investment weights
CBA 0.15 0 0.0016842
92
0.0017106
89
0.0054025
12
$I$22 Investment weights
NAB 0
-
0.0054025
12
-
0.0037182
2
0.0054025
12 1E+30
$J$22 Investment weights
CAR 0.2 0 0.0159711
77 1E+30 0.0053925
16
$K$2
2
Investment weights
CPU 0
-
0.0053925
16
0.0105786
61
0.0053925
16 1E+30
$L$2
2
Investment weights
AGL 0
-
0.0062155
15
-
0.0028205
34
0.0062155
15 1E+30
Final Shadow Constraint Allowable Allowable
Cell Name Valu
e Price R.H. Side Increase Decrease
$B$6
1 LHS 1 0.0016842
92 1 0.05 0.15
$B$6
3 LHS 0.6 0 0.1 0.5 1E+30
$B$6
4 LHS 0.15 0 0.2 1E+30 0.05
$B$6
5 LHS 0.15 0 0.3 1E+30 0.15
$B$6
6 LHS 0.2 0.0142868
85 0.2 0.15 0.05
$B$6
7 LHS 0.25 0 0.15 0.1 1E+30
$B$6
8 LHS 0.25 0.0209745
88 0.25 0.15 0.05
$B$6
9 LHS 0.1 0.0111041
77 0.1 0.15 0.05
$B$7
0 LHS 0.3 0.0017106
89 0.3 0.15 0.05
⊘ This is a preview!⊘
Do you want full access?
Subscribe today to unlock all pages.

Trusted by 1+ million students worldwide

• On perusal of the above tables, it may be inferred that the there is allowable increase
for five assets in the portfolio and allowable decrease of five asset in the portfolio.
• Further, the reduction in each of the asset has been duly shown to achieve the desired
portfolio.
• Probable increase or decrease in constraint which can impact the return of the portfolio
like allowable increase for weights is 0.05 (increase) to 0.15 (decrease)
• Similarly, Investment in R3 cannot be exceeded further.
• For R2 it has been stated that allowable increase of 0.5 is permitted and so on.
Paraphrase This Document
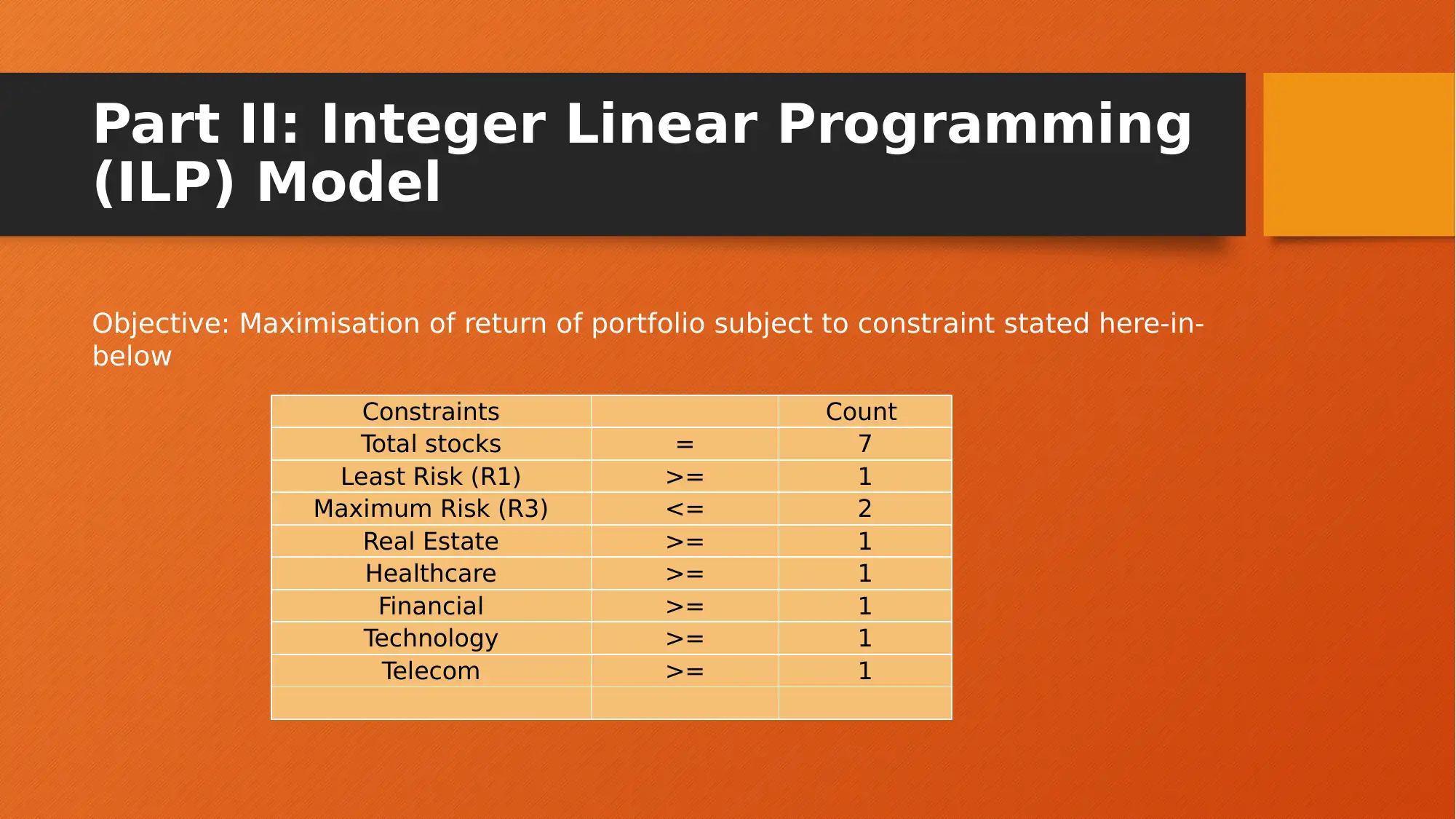
(ILP) Model
Objective: Maximisation of return of portfolio subject to constraint stated here-in-
below
Constraints Count
Total stocks = 7
Least Risk (R1) >= 1
Maximum Risk (R3) <= 2
Real Estate >= 1
Healthcare >= 1
Financial >= 1
Technology >= 1
Telecom >= 1
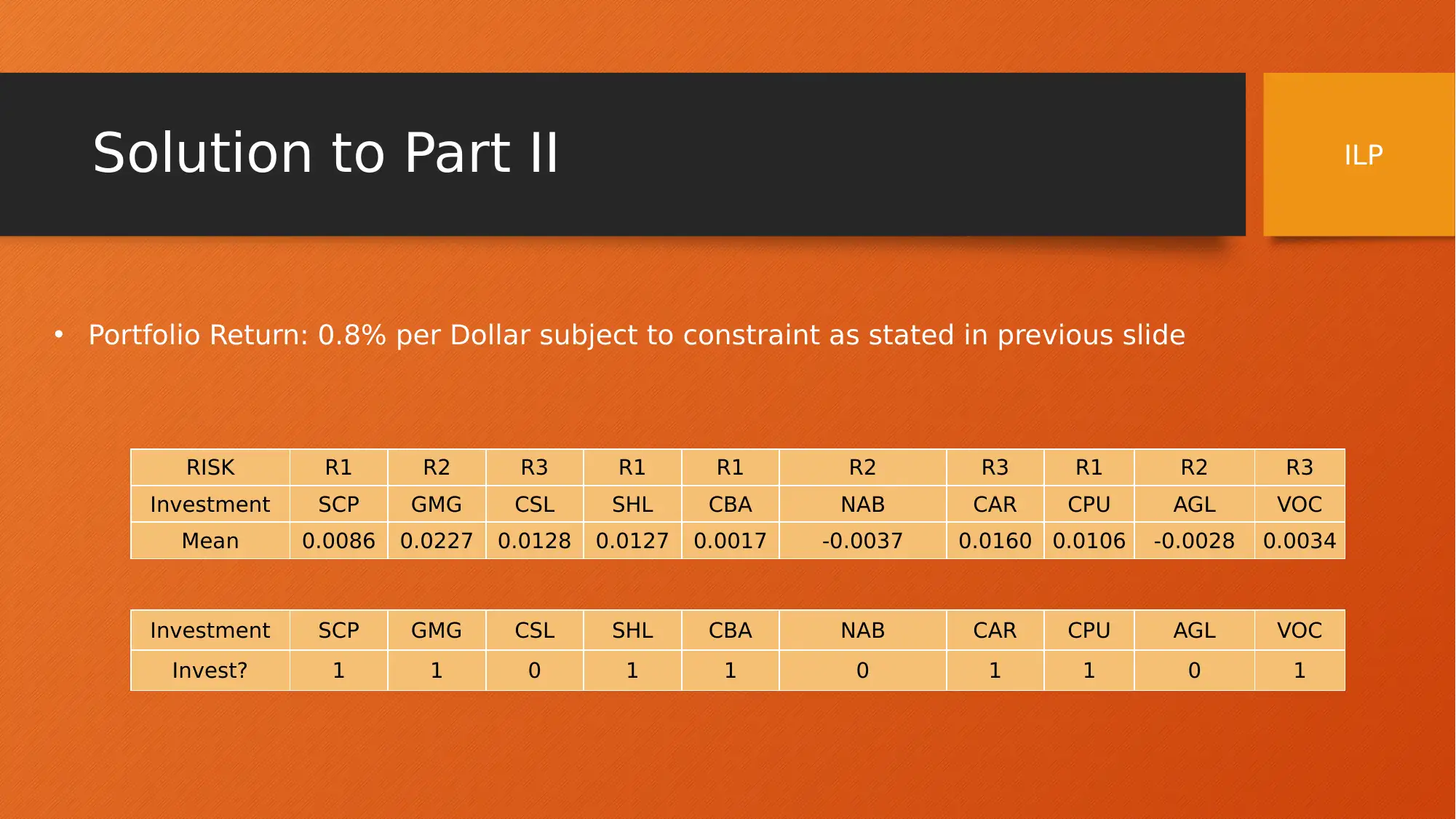
• Portfolio Return: 0.8% per Dollar subject to constraint as stated in previous slide
RISK R1 R2 R3 R1 R1 R2 R3 R1 R2 R3
Investment SCP GMG CSL SHL CBA NAB CAR CPU AGL VOC
Mean 0.0086 0.0227 0.0128 0.0127 0.0017 -0.0037 0.0160 0.0106 -0.0028 0.0034
Investment SCP GMG CSL SHL CBA NAB CAR CPU AGL VOC
Invest? 1 1 0 1 1 0 1 1 0 1
ILP
⊘ This is a preview!⊘
Do you want full access?
Subscribe today to unlock all pages.

Trusted by 1+ million students worldwide
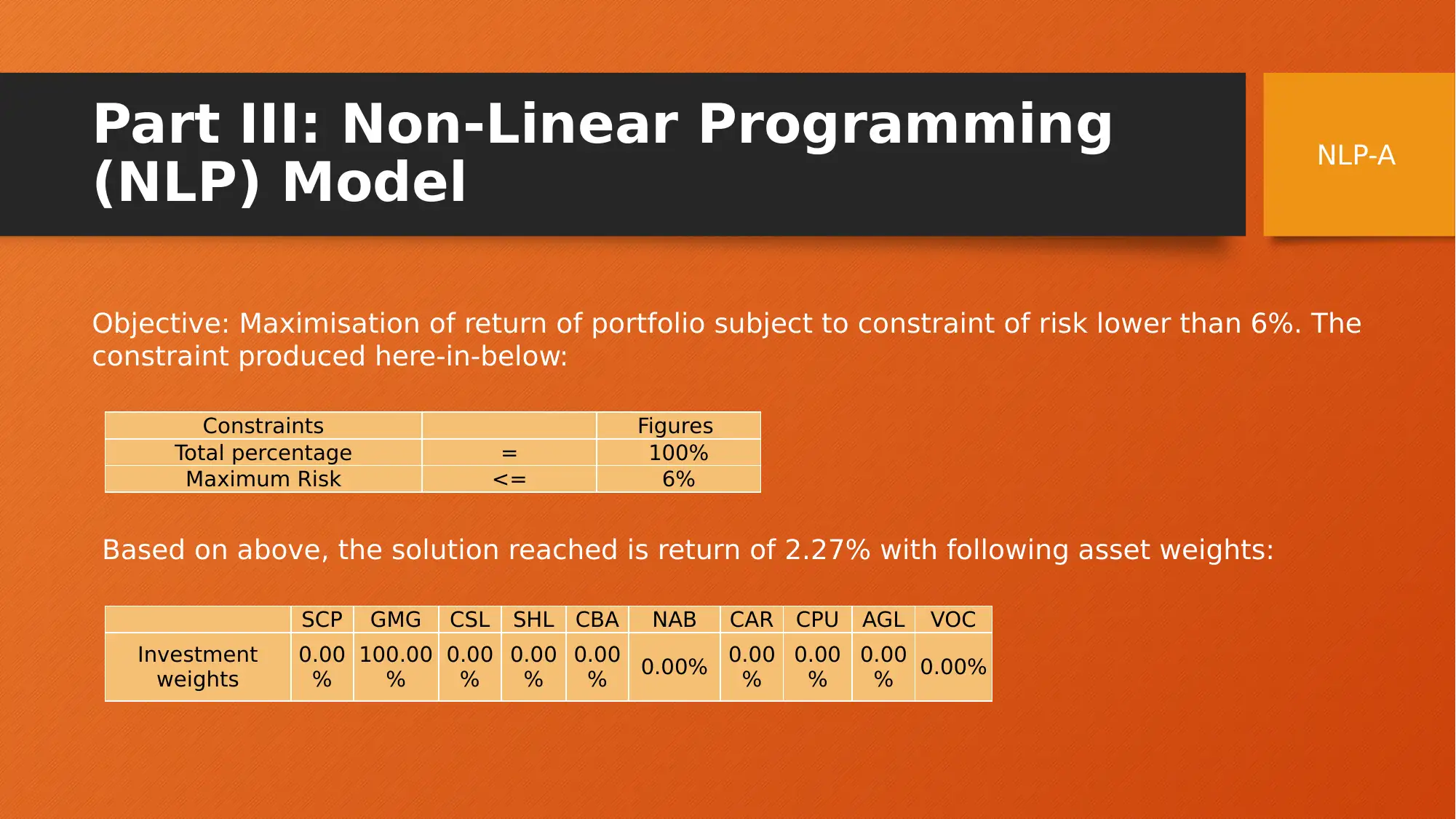
(NLP) Model
Objective: Maximisation of return of portfolio subject to constraint of risk lower than 6%. The
constraint produced here-in-below:
Constraints Figures
Total percentage = 100%
Maximum Risk <= 6%
Based on above, the solution reached is return of 2.27% with following asset weights:
SCP GMG CSL SHL CBA NAB CAR CPU AGL VOC
Investment
weights
0.00
%
100.00
%
0.00
%
0.00
%
0.00
% 0.00% 0.00
%
0.00
%
0.00
% 0.00%
NLP-A
Paraphrase This Document
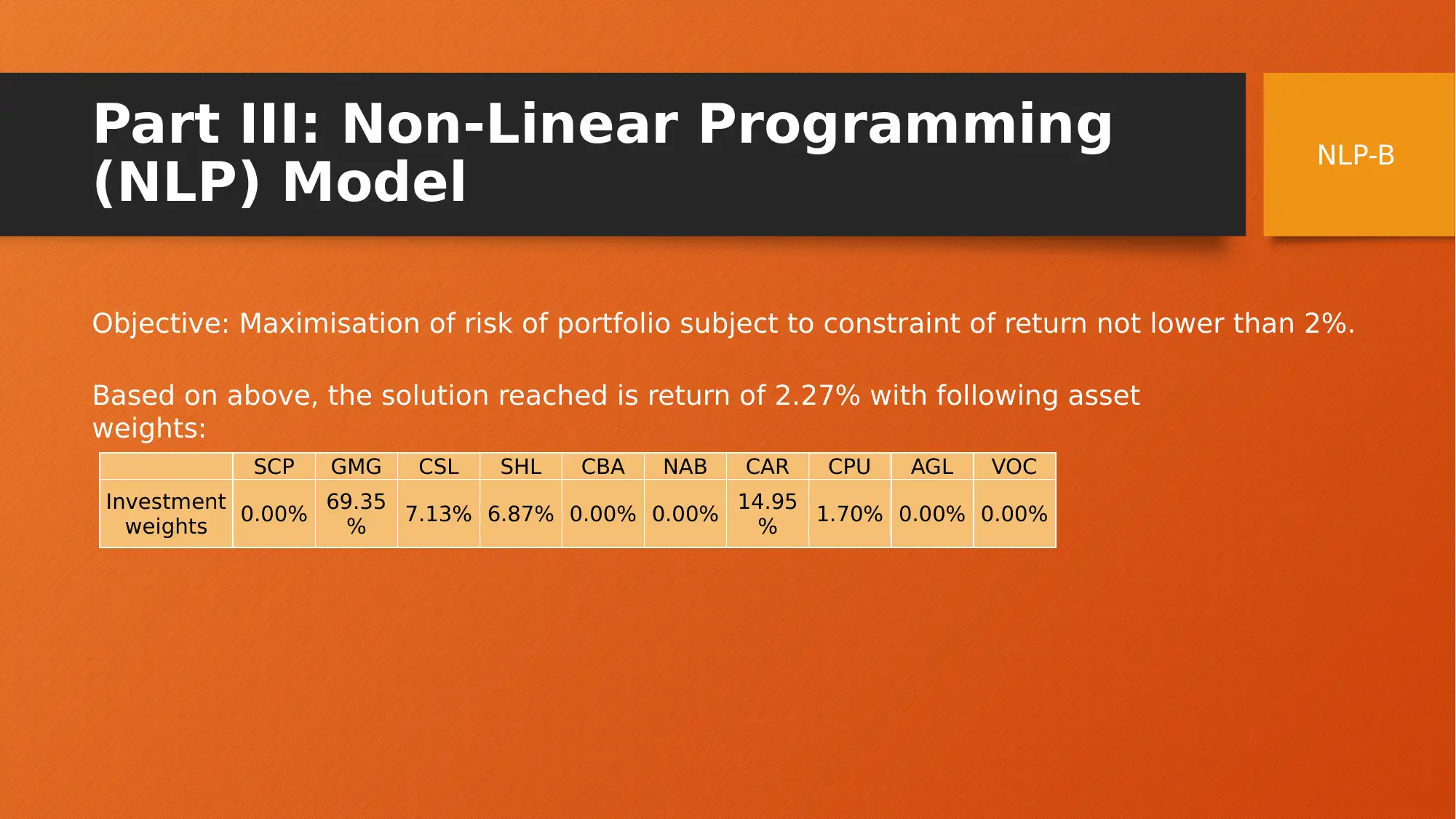
(NLP) Model
Objective: Maximisation of risk of portfolio subject to constraint of return not lower than 2%.
Based on above, the solution reached is return of 2.27% with following asset
weights:
SCP GMG CSL SHL CBA NAB CAR CPU AGL VOC
Investment
weights 0.00% 69.35
% 7.13% 6.87% 0.00% 0.00% 14.95
% 1.70% 0.00% 0.00%
NLP-B
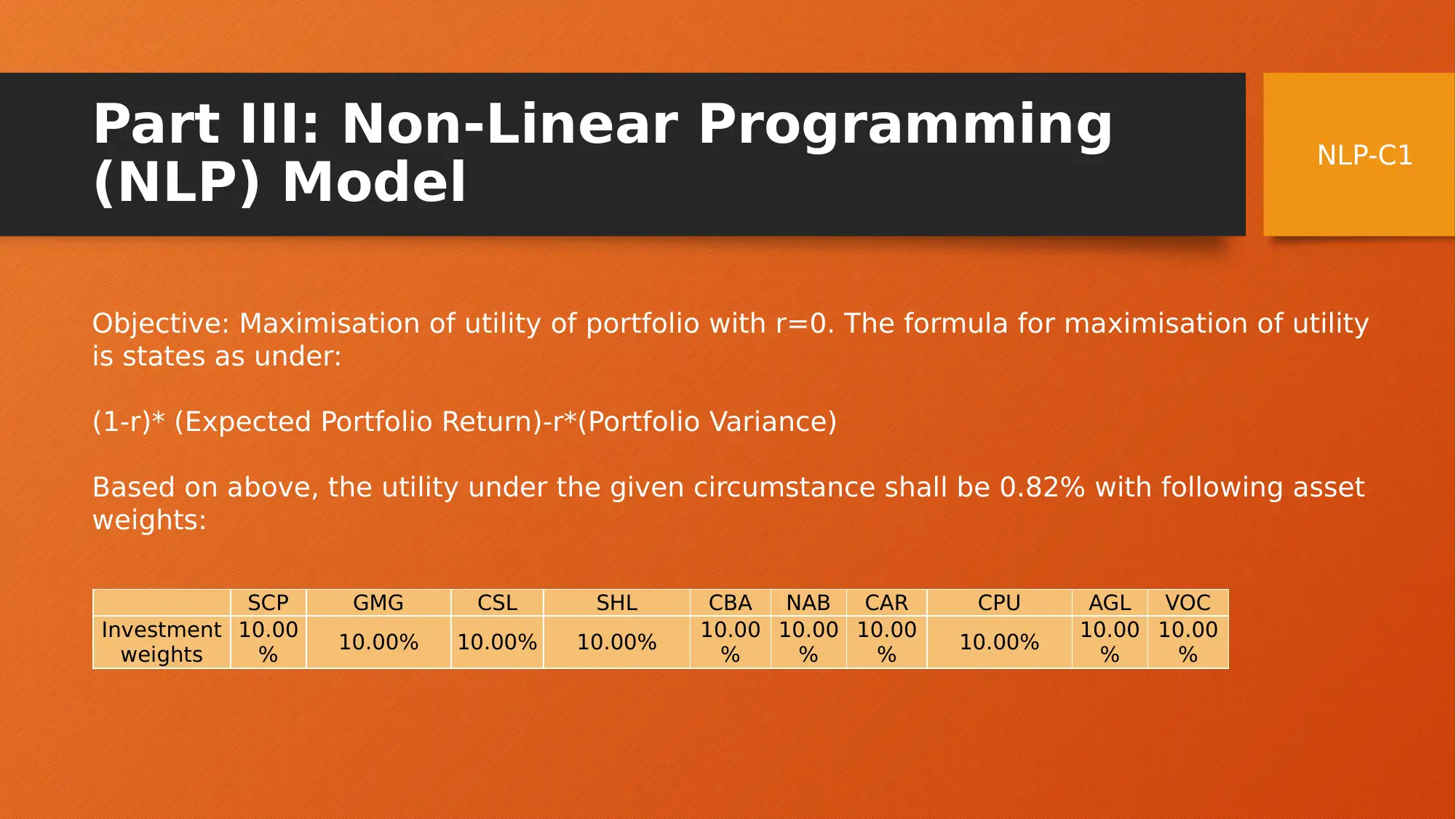
(NLP) Model
Objective: Maximisation of utility of portfolio with r=0. The formula for maximisation of utility
is states as under:
(1-r)* (Expected Portfolio Return)-r*(Portfolio Variance)
Based on above, the utility under the given circumstance shall be 0.82% with following asset
weights:
SCP GMG CSL SHL CBA NAB CAR CPU AGL VOC
Investment
weights
10.00
% 10.00% 10.00% 10.00% 10.00
%
10.00
%
10.00
% 10.00% 10.00
%
10.00
%
NLP-C1
⊘ This is a preview!⊘
Do you want full access?
Subscribe today to unlock all pages.

Trusted by 1+ million students worldwide
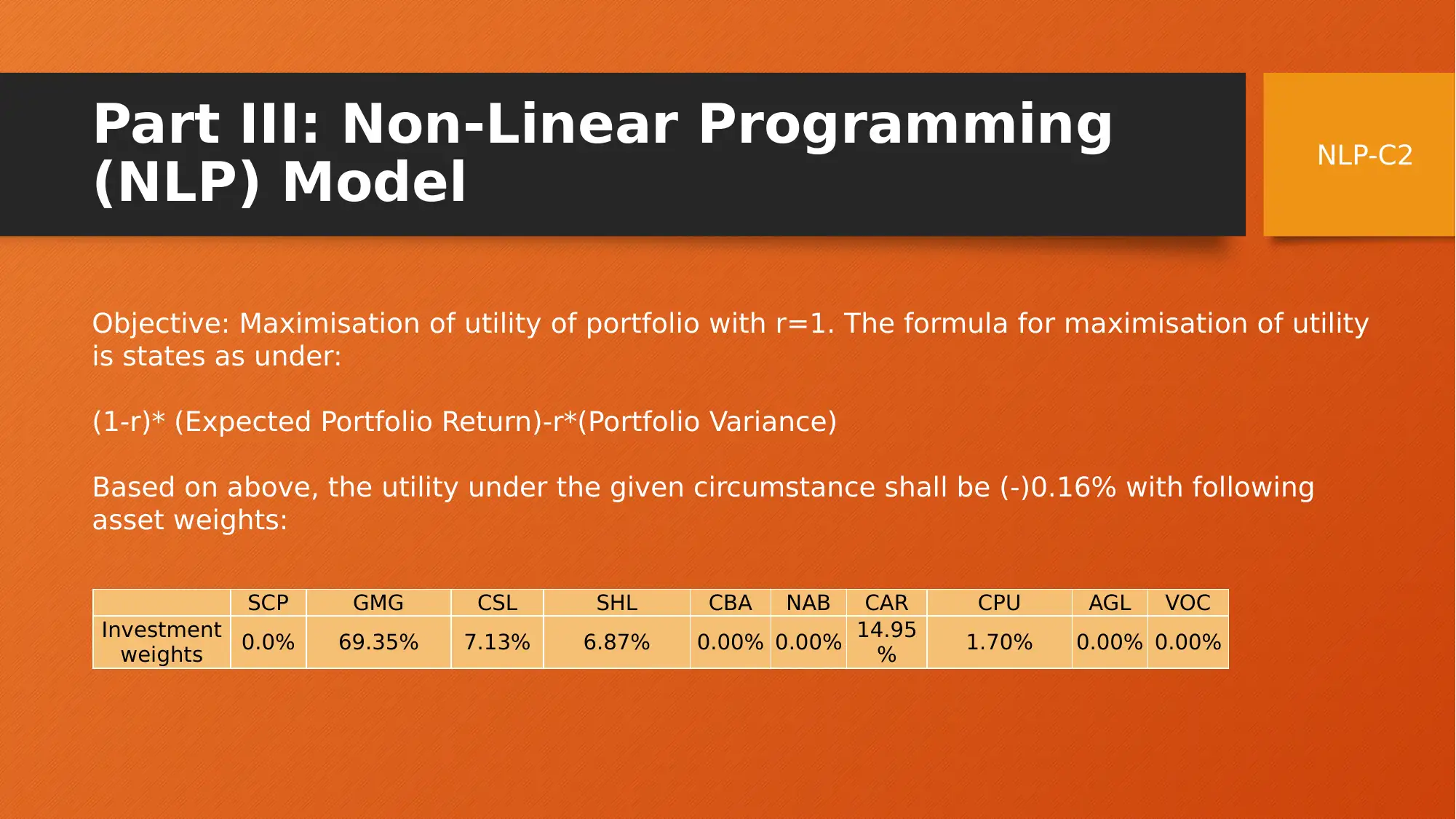
(NLP) Model
Objective: Maximisation of utility of portfolio with r=1. The formula for maximisation of utility
is states as under:
(1-r)* (Expected Portfolio Return)-r*(Portfolio Variance)
Based on above, the utility under the given circumstance shall be (-)0.16% with following
asset weights:
SCP GMG CSL SHL CBA NAB CAR CPU AGL VOC
Investment
weights 0.0% 69.35% 7.13% 6.87% 0.00% 0.00% 14.95
% 1.70% 0.00% 0.00%
NLP-C2
Paraphrase This Document
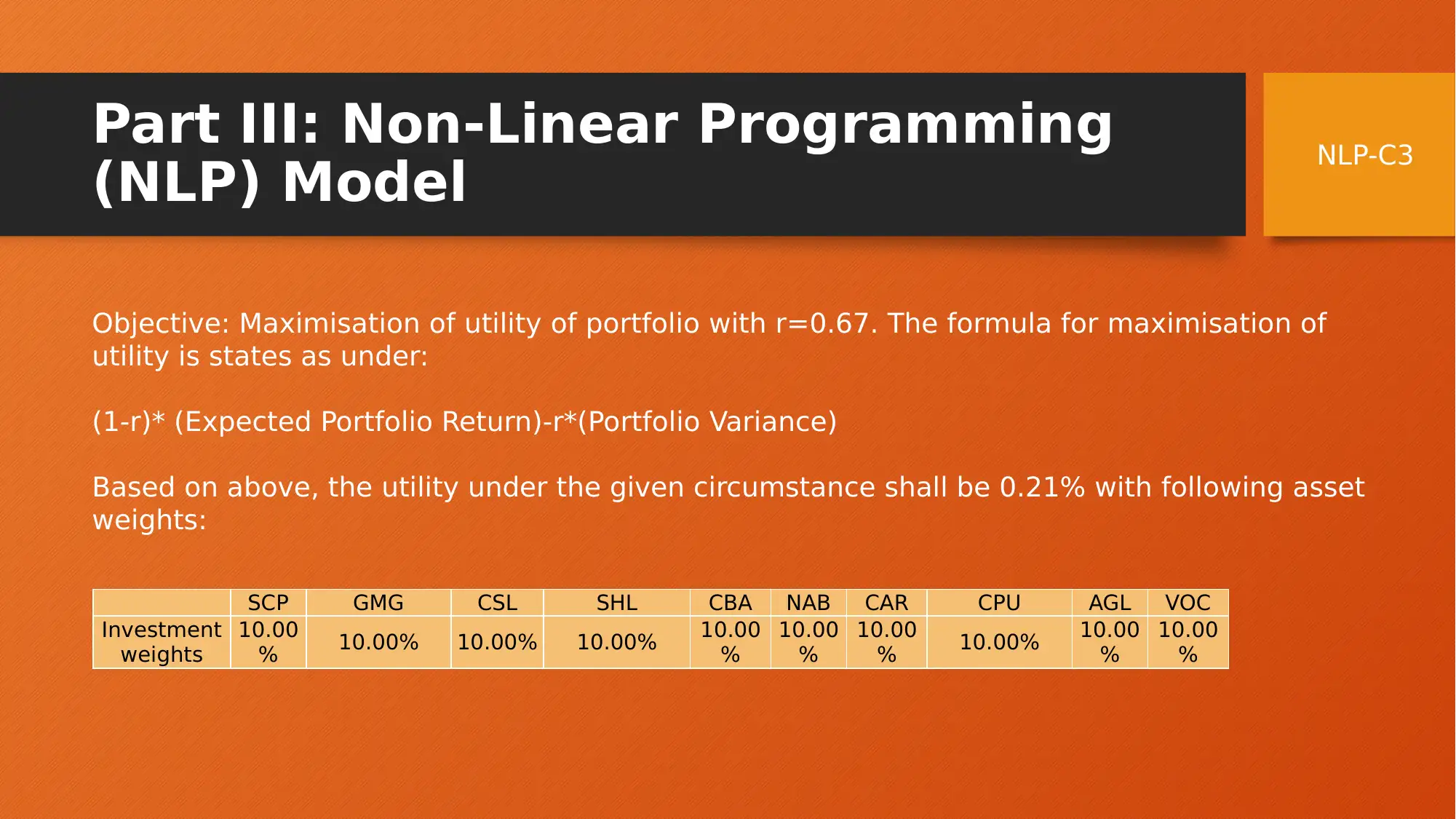
(NLP) Model
Objective: Maximisation of utility of portfolio with r=0.67. The formula for maximisation of
utility is states as under:
(1-r)* (Expected Portfolio Return)-r*(Portfolio Variance)
Based on above, the utility under the given circumstance shall be 0.21% with following asset
weights:
SCP GMG CSL SHL CBA NAB CAR CPU AGL VOC
Investment
weights
10.00
% 10.00% 10.00% 10.00% 10.00
%
10.00
%
10.00
% 10.00% 10.00
%
10.00
%
NLP-C3
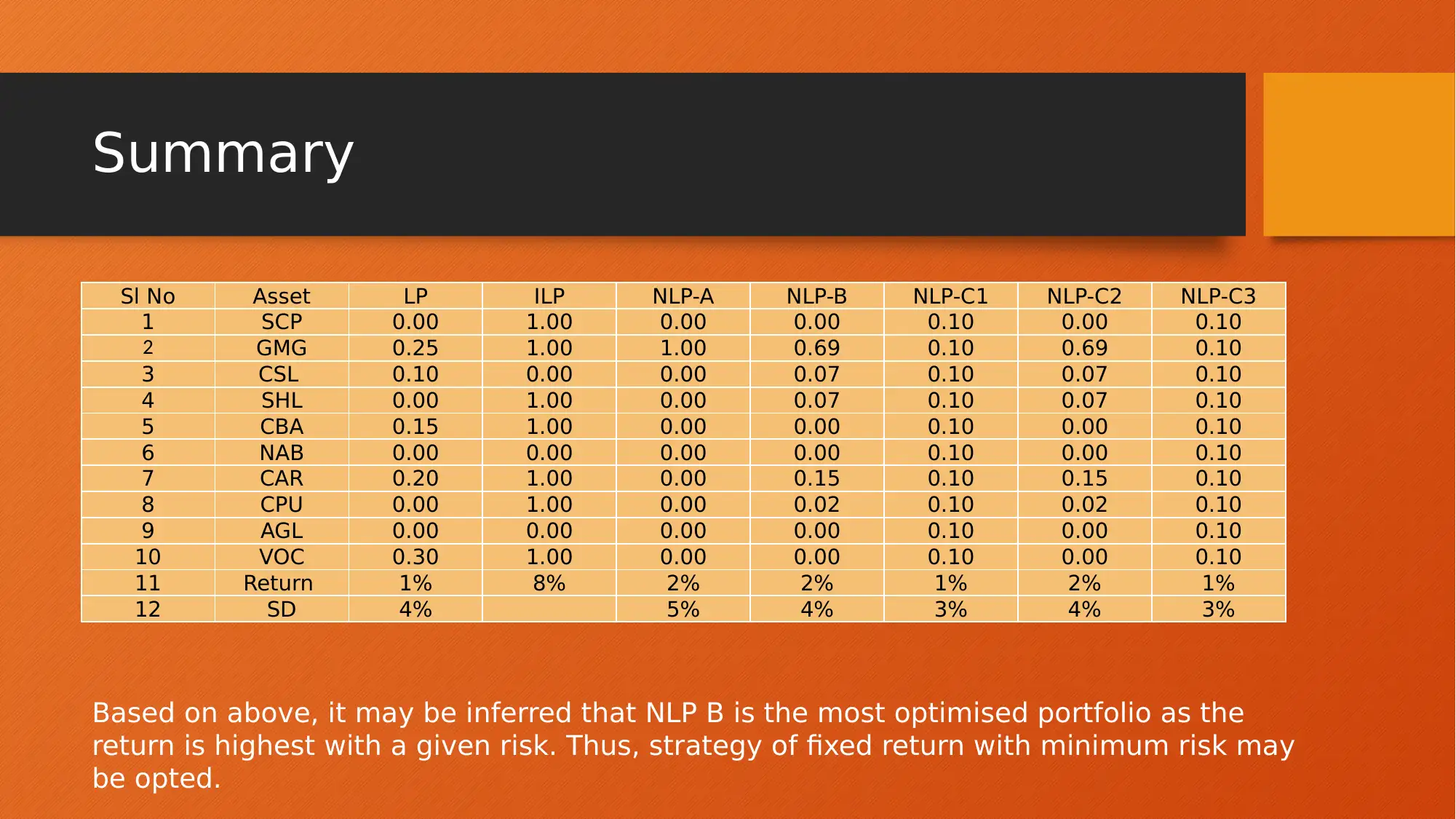
Sl No Asset LP ILP NLP-A NLP-B NLP-C1 NLP-C2 NLP-C3
1 SCP 0.00 1.00 0.00 0.00 0.10 0.00 0.10
2 GMG 0.25 1.00 1.00 0.69 0.10 0.69 0.10
3 CSL 0.10 0.00 0.00 0.07 0.10 0.07 0.10
4 SHL 0.00 1.00 0.00 0.07 0.10 0.07 0.10
5 CBA 0.15 1.00 0.00 0.00 0.10 0.00 0.10
6 NAB 0.00 0.00 0.00 0.00 0.10 0.00 0.10
7 CAR 0.20 1.00 0.00 0.15 0.10 0.15 0.10
8 CPU 0.00 1.00 0.00 0.02 0.10 0.02 0.10
9 AGL 0.00 0.00 0.00 0.00 0.10 0.00 0.10
10 VOC 0.30 1.00 0.00 0.00 0.10 0.00 0.10
11 Return 1% 8% 2% 2% 1% 2% 1%
12 SD 4% 5% 4% 3% 4% 3%
Based on above, it may be inferred that NLP B is the most optimised portfolio as the
return is highest with a given risk. Thus, strategy of fixed return with minimum risk may
be opted.
⊘ This is a preview!⊘
Do you want full access?
Subscribe today to unlock all pages.

Trusted by 1+ million students worldwide
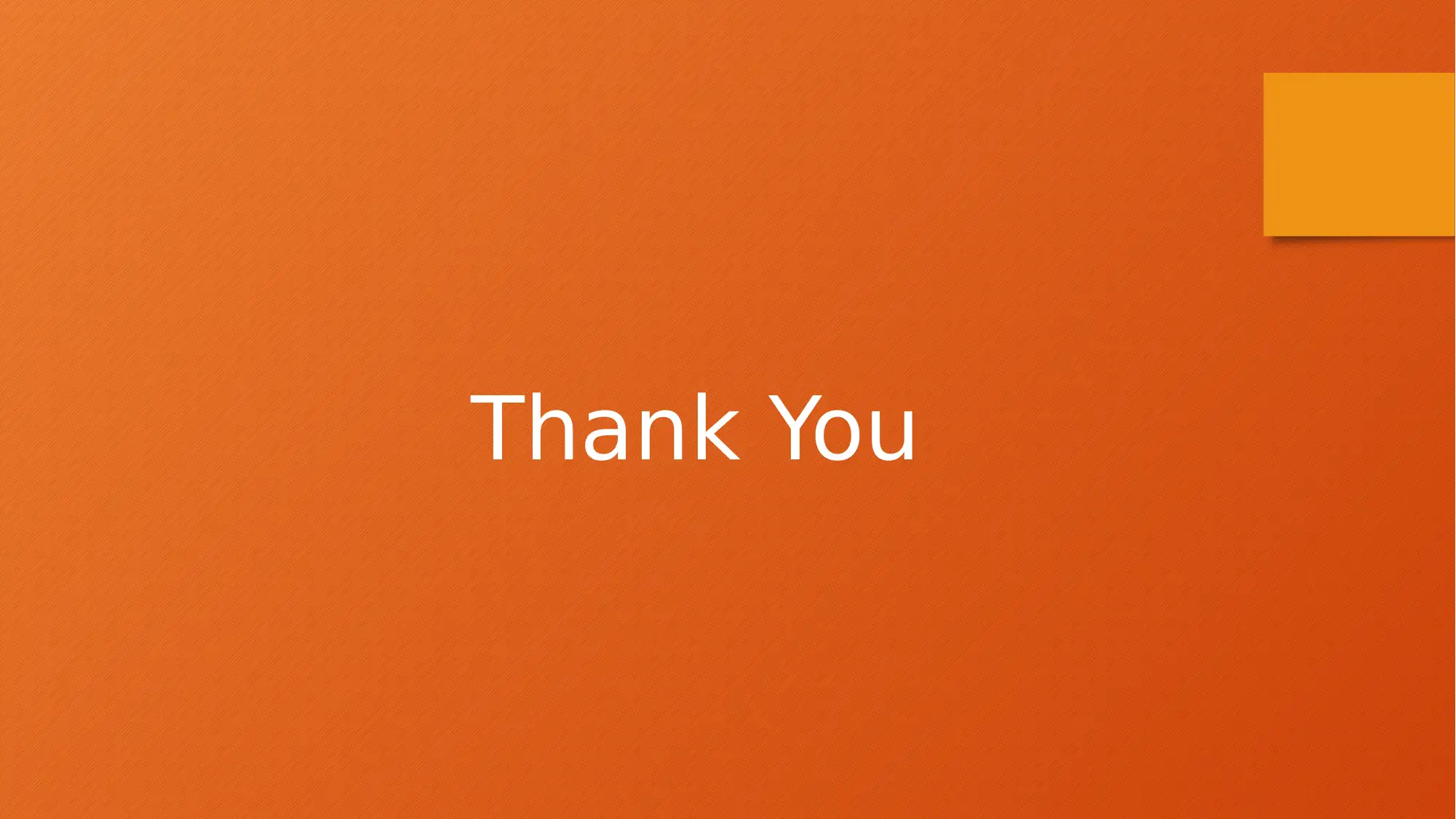

Your All-in-One AI-Powered Toolkit for Academic Success.
+13062052269
info@desklib.com
Available 24*7 on WhatsApp / Email
© 2024 | Zucol Services PVT LTD | All rights reserved.