Business Analytics: Data Analysis, Email and Interactive Dashboard Presentation
VerifiedAdded on 2023/06/12
|15
|3044
|262
AI Summary
This report provides a data analysis of Supermart's financial data, including gross profit, wastage, and online channel by location. It also includes an email from the general manager and an interactive dashboard presentation.
Contribute Materials
Your contribution can guide someone’s learning journey. Share your
documents today.
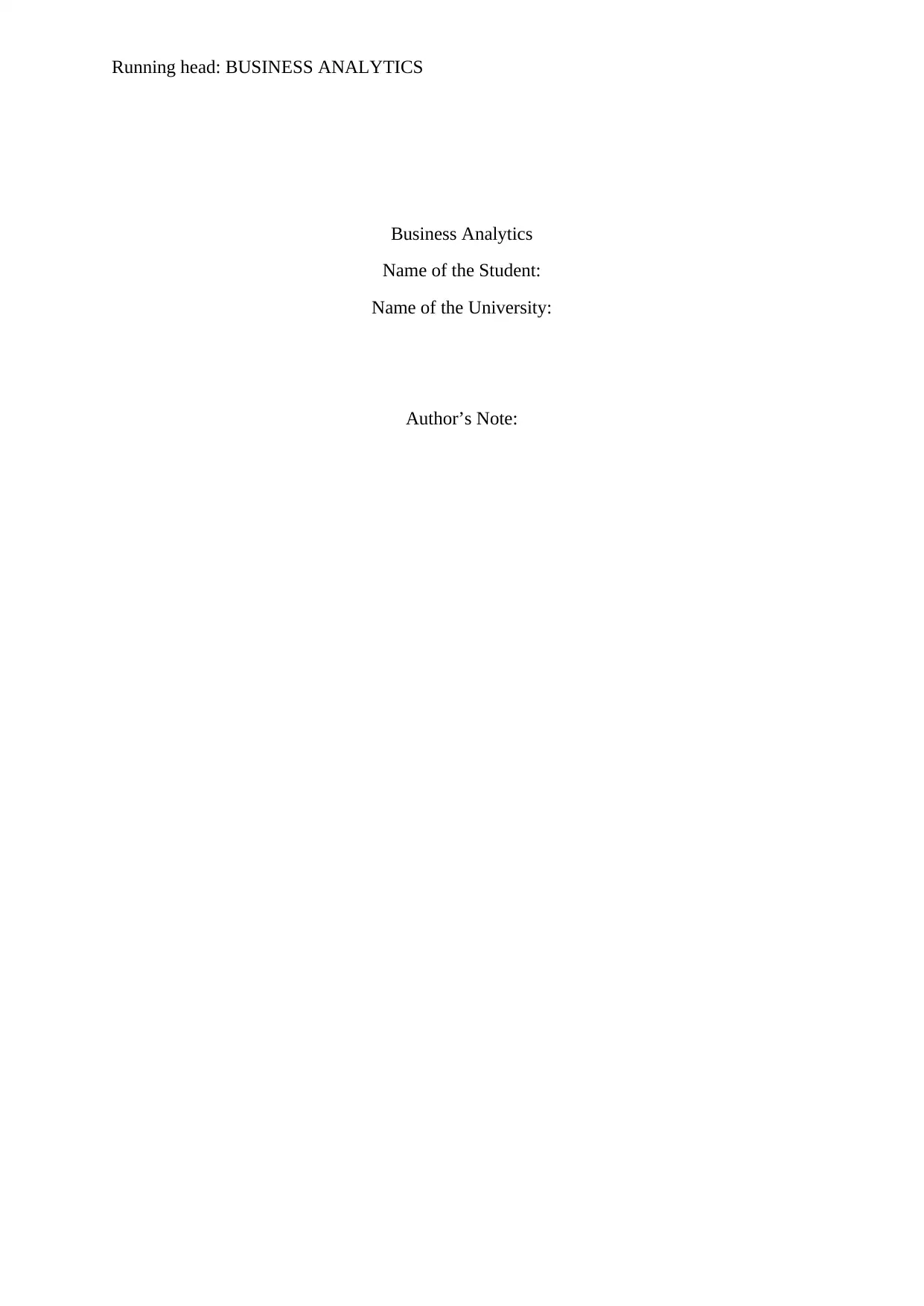
Running head: BUSINESS ANALYTICS
Business Analytics
Name of the Student:
Name of the University:
Author’s Note:
Business Analytics
Name of the Student:
Name of the University:
Author’s Note:
Secure Best Marks with AI Grader
Need help grading? Try our AI Grader for instant feedback on your assignments.
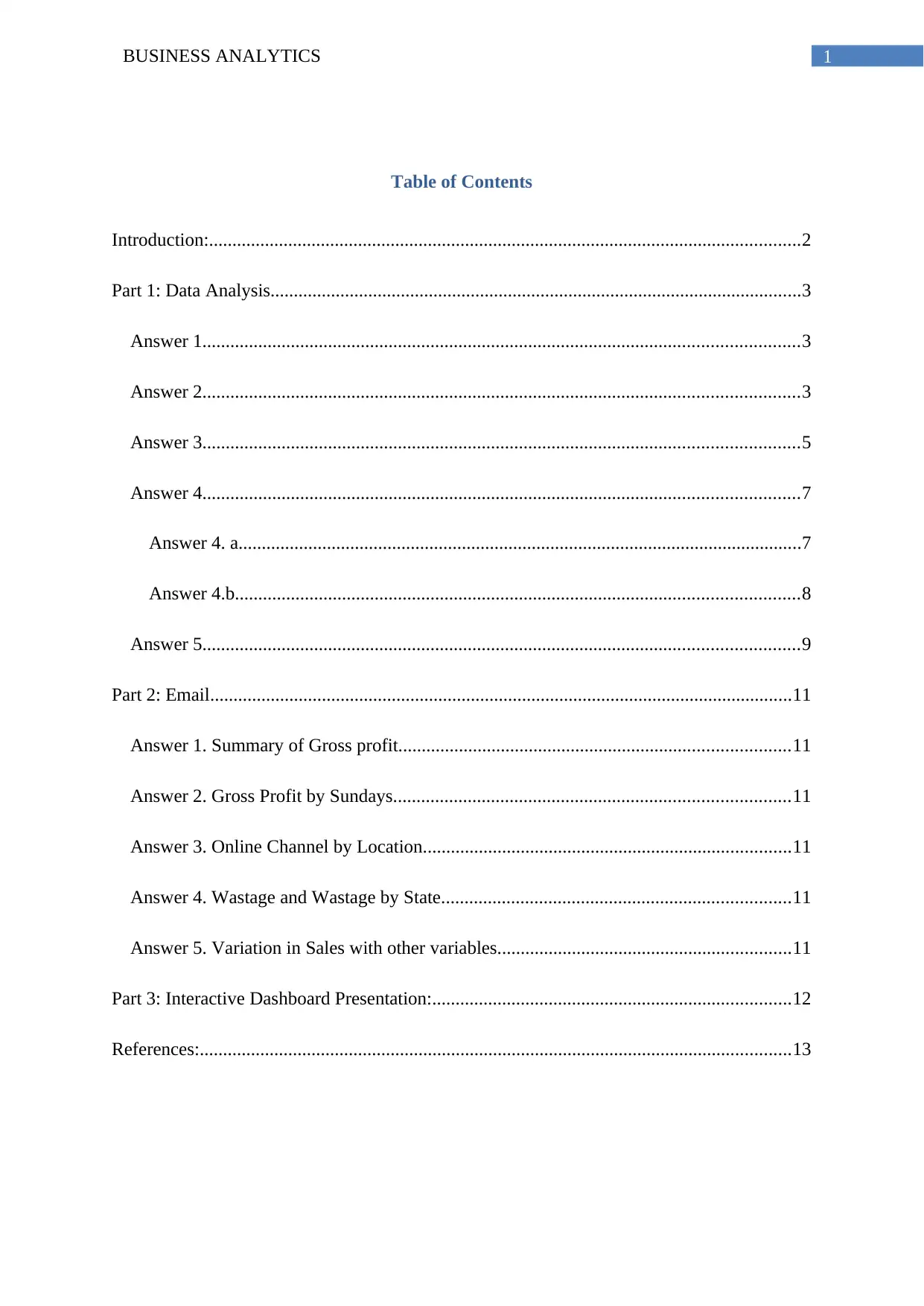
1BUSINESS ANALYTICS
Table of Contents
Introduction:...............................................................................................................................2
Part 1: Data Analysis..................................................................................................................3
Answer 1................................................................................................................................3
Answer 2................................................................................................................................3
Answer 3................................................................................................................................5
Answer 4................................................................................................................................7
Answer 4. a.........................................................................................................................7
Answer 4.b.........................................................................................................................8
Answer 5................................................................................................................................9
Part 2: Email.............................................................................................................................11
Answer 1. Summary of Gross profit....................................................................................11
Answer 2. Gross Profit by Sundays.....................................................................................11
Answer 3. Online Channel by Location...............................................................................11
Answer 4. Wastage and Wastage by State...........................................................................11
Answer 5. Variation in Sales with other variables...............................................................11
Part 3: Interactive Dashboard Presentation:.............................................................................12
References:...............................................................................................................................13
Table of Contents
Introduction:...............................................................................................................................2
Part 1: Data Analysis..................................................................................................................3
Answer 1................................................................................................................................3
Answer 2................................................................................................................................3
Answer 3................................................................................................................................5
Answer 4................................................................................................................................7
Answer 4. a.........................................................................................................................7
Answer 4.b.........................................................................................................................8
Answer 5................................................................................................................................9
Part 2: Email.............................................................................................................................11
Answer 1. Summary of Gross profit....................................................................................11
Answer 2. Gross Profit by Sundays.....................................................................................11
Answer 3. Online Channel by Location...............................................................................11
Answer 4. Wastage and Wastage by State...........................................................................11
Answer 5. Variation in Sales with other variables...............................................................11
Part 3: Interactive Dashboard Presentation:.............................................................................12
References:...............................................................................................................................13
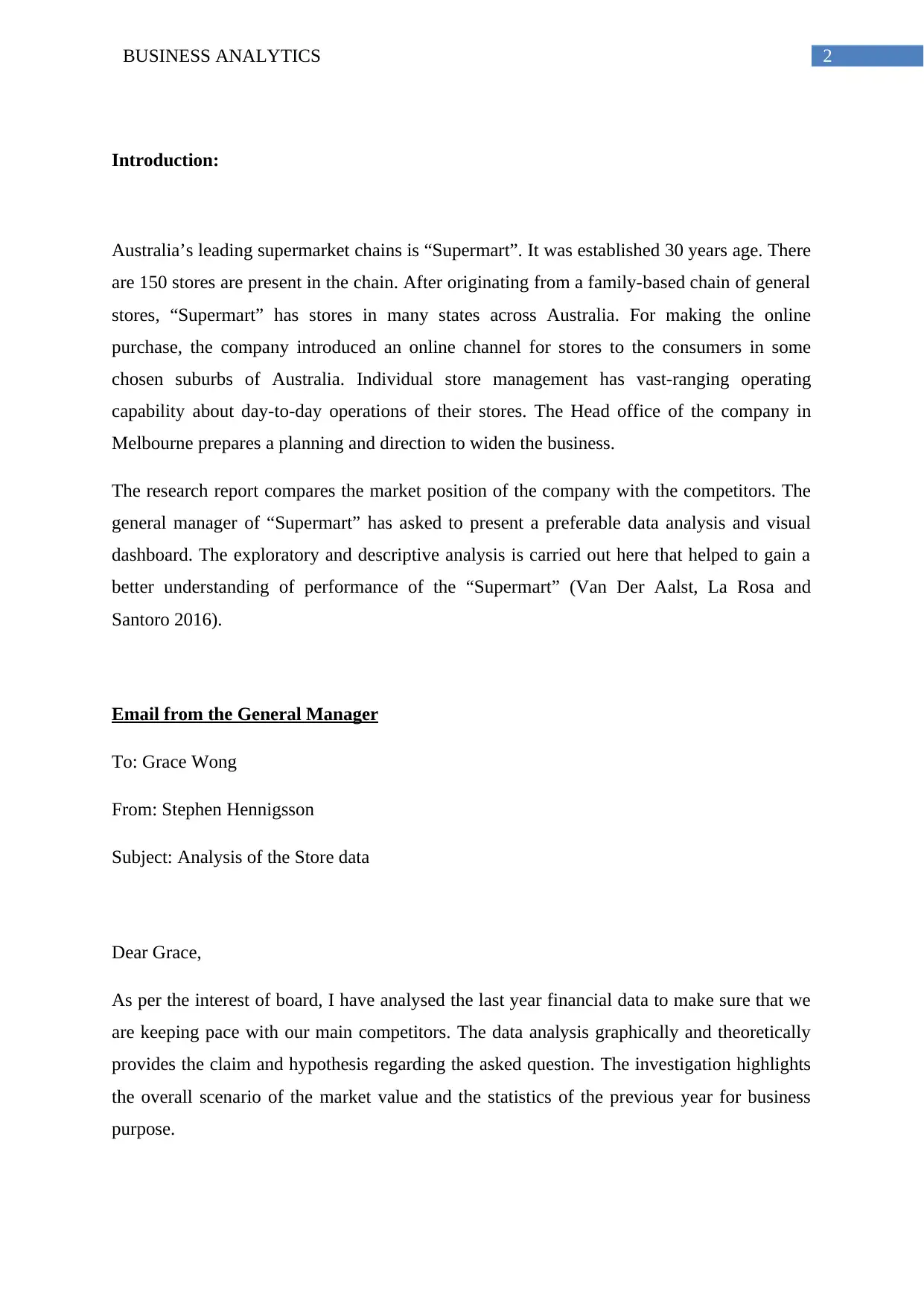
2BUSINESS ANALYTICS
Introduction:
Australia’s leading supermarket chains is “Supermart”. It was established 30 years age. There
are 150 stores are present in the chain. After originating from a family-based chain of general
stores, “Supermart” has stores in many states across Australia. For making the online
purchase, the company introduced an online channel for stores to the consumers in some
chosen suburbs of Australia. Individual store management has vast-ranging operating
capability about day-to-day operations of their stores. The Head office of the company in
Melbourne prepares a planning and direction to widen the business.
The research report compares the market position of the company with the competitors. The
general manager of “Supermart” has asked to present a preferable data analysis and visual
dashboard. The exploratory and descriptive analysis is carried out here that helped to gain a
better understanding of performance of the “Supermart” (Van Der Aalst, La Rosa and
Santoro 2016).
Email from the General Manager
To: Grace Wong
From: Stephen Hennigsson
Subject: Analysis of the Store data
Dear Grace,
As per the interest of board, I have analysed the last year financial data to make sure that we
are keeping pace with our main competitors. The data analysis graphically and theoretically
provides the claim and hypothesis regarding the asked question. The investigation highlights
the overall scenario of the market value and the statistics of the previous year for business
purpose.
Introduction:
Australia’s leading supermarket chains is “Supermart”. It was established 30 years age. There
are 150 stores are present in the chain. After originating from a family-based chain of general
stores, “Supermart” has stores in many states across Australia. For making the online
purchase, the company introduced an online channel for stores to the consumers in some
chosen suburbs of Australia. Individual store management has vast-ranging operating
capability about day-to-day operations of their stores. The Head office of the company in
Melbourne prepares a planning and direction to widen the business.
The research report compares the market position of the company with the competitors. The
general manager of “Supermart” has asked to present a preferable data analysis and visual
dashboard. The exploratory and descriptive analysis is carried out here that helped to gain a
better understanding of performance of the “Supermart” (Van Der Aalst, La Rosa and
Santoro 2016).
Email from the General Manager
To: Grace Wong
From: Stephen Hennigsson
Subject: Analysis of the Store data
Dear Grace,
As per the interest of board, I have analysed the last year financial data to make sure that we
are keeping pace with our main competitors. The data analysis graphically and theoretically
provides the claim and hypothesis regarding the asked question. The investigation highlights
the overall scenario of the market value and the statistics of the previous year for business
purpose.
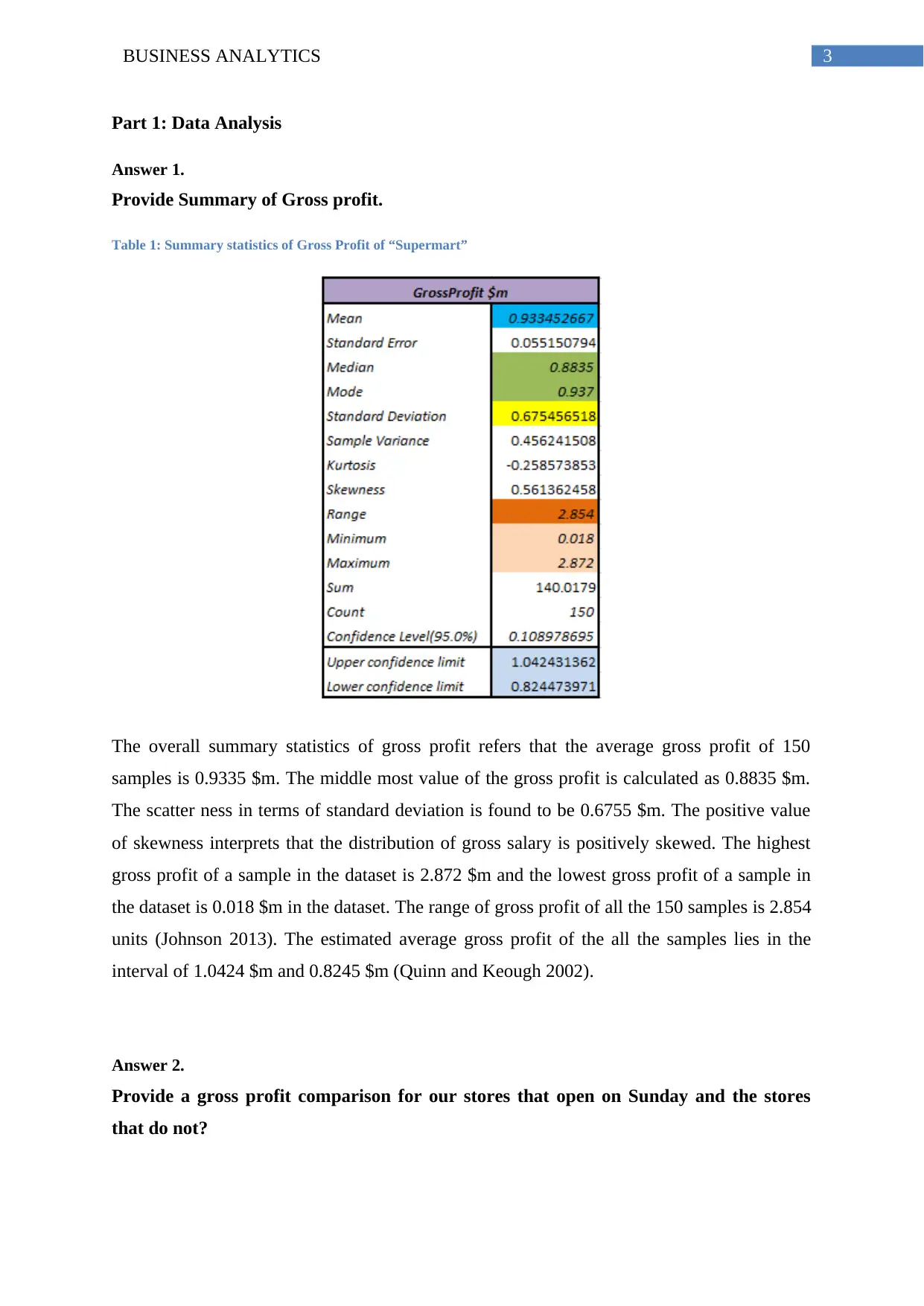
3BUSINESS ANALYTICS
Part 1: Data Analysis
Answer 1.
Provide Summary of Gross profit.
Table 1: Summary statistics of Gross Profit of “Supermart”
The overall summary statistics of gross profit refers that the average gross profit of 150
samples is 0.9335 $m. The middle most value of the gross profit is calculated as 0.8835 $m.
The scatter ness in terms of standard deviation is found to be 0.6755 $m. The positive value
of skewness interprets that the distribution of gross salary is positively skewed. The highest
gross profit of a sample in the dataset is 2.872 $m and the lowest gross profit of a sample in
the dataset is 0.018 $m in the dataset. The range of gross profit of all the 150 samples is 2.854
units (Johnson 2013). The estimated average gross profit of the all the samples lies in the
interval of 1.0424 $m and 0.8245 $m (Quinn and Keough 2002).
Answer 2.
Provide a gross profit comparison for our stores that open on Sunday and the stores
that do not?
Part 1: Data Analysis
Answer 1.
Provide Summary of Gross profit.
Table 1: Summary statistics of Gross Profit of “Supermart”
The overall summary statistics of gross profit refers that the average gross profit of 150
samples is 0.9335 $m. The middle most value of the gross profit is calculated as 0.8835 $m.
The scatter ness in terms of standard deviation is found to be 0.6755 $m. The positive value
of skewness interprets that the distribution of gross salary is positively skewed. The highest
gross profit of a sample in the dataset is 2.872 $m and the lowest gross profit of a sample in
the dataset is 0.018 $m in the dataset. The range of gross profit of all the 150 samples is 2.854
units (Johnson 2013). The estimated average gross profit of the all the samples lies in the
interval of 1.0424 $m and 0.8245 $m (Quinn and Keough 2002).
Answer 2.
Provide a gross profit comparison for our stores that open on Sunday and the stores
that do not?
Secure Best Marks with AI Grader
Need help grading? Try our AI Grader for instant feedback on your assignments.
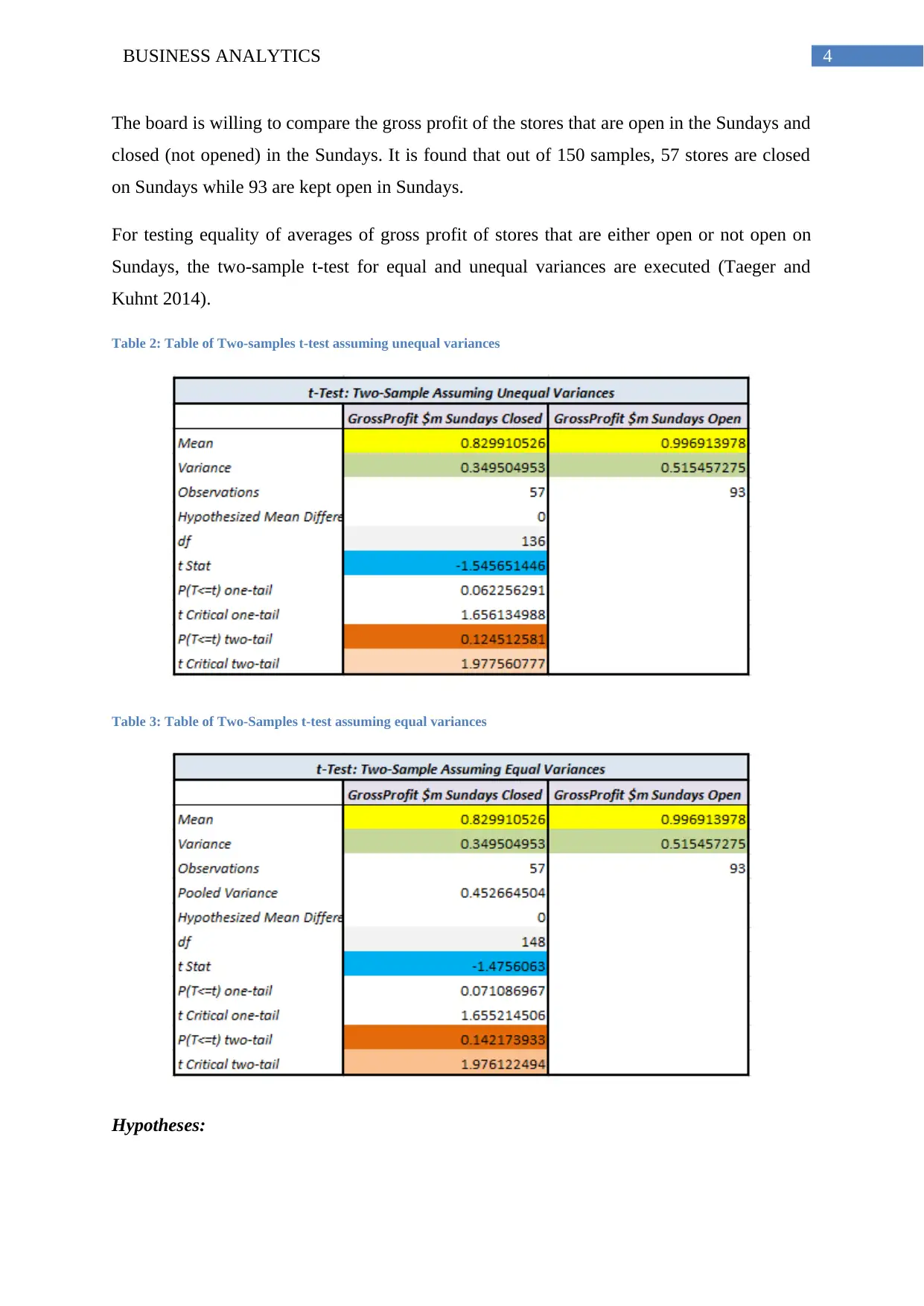
4BUSINESS ANALYTICS
The board is willing to compare the gross profit of the stores that are open in the Sundays and
closed (not opened) in the Sundays. It is found that out of 150 samples, 57 stores are closed
on Sundays while 93 are kept open in Sundays.
For testing equality of averages of gross profit of stores that are either open or not open on
Sundays, the two-sample t-test for equal and unequal variances are executed (Taeger and
Kuhnt 2014).
Table 2: Table of Two-samples t-test assuming unequal variances
Table 3: Table of Two-Samples t-test assuming equal variances
Hypotheses:
The board is willing to compare the gross profit of the stores that are open in the Sundays and
closed (not opened) in the Sundays. It is found that out of 150 samples, 57 stores are closed
on Sundays while 93 are kept open in Sundays.
For testing equality of averages of gross profit of stores that are either open or not open on
Sundays, the two-sample t-test for equal and unequal variances are executed (Taeger and
Kuhnt 2014).
Table 2: Table of Two-samples t-test assuming unequal variances
Table 3: Table of Two-Samples t-test assuming equal variances
Hypotheses:
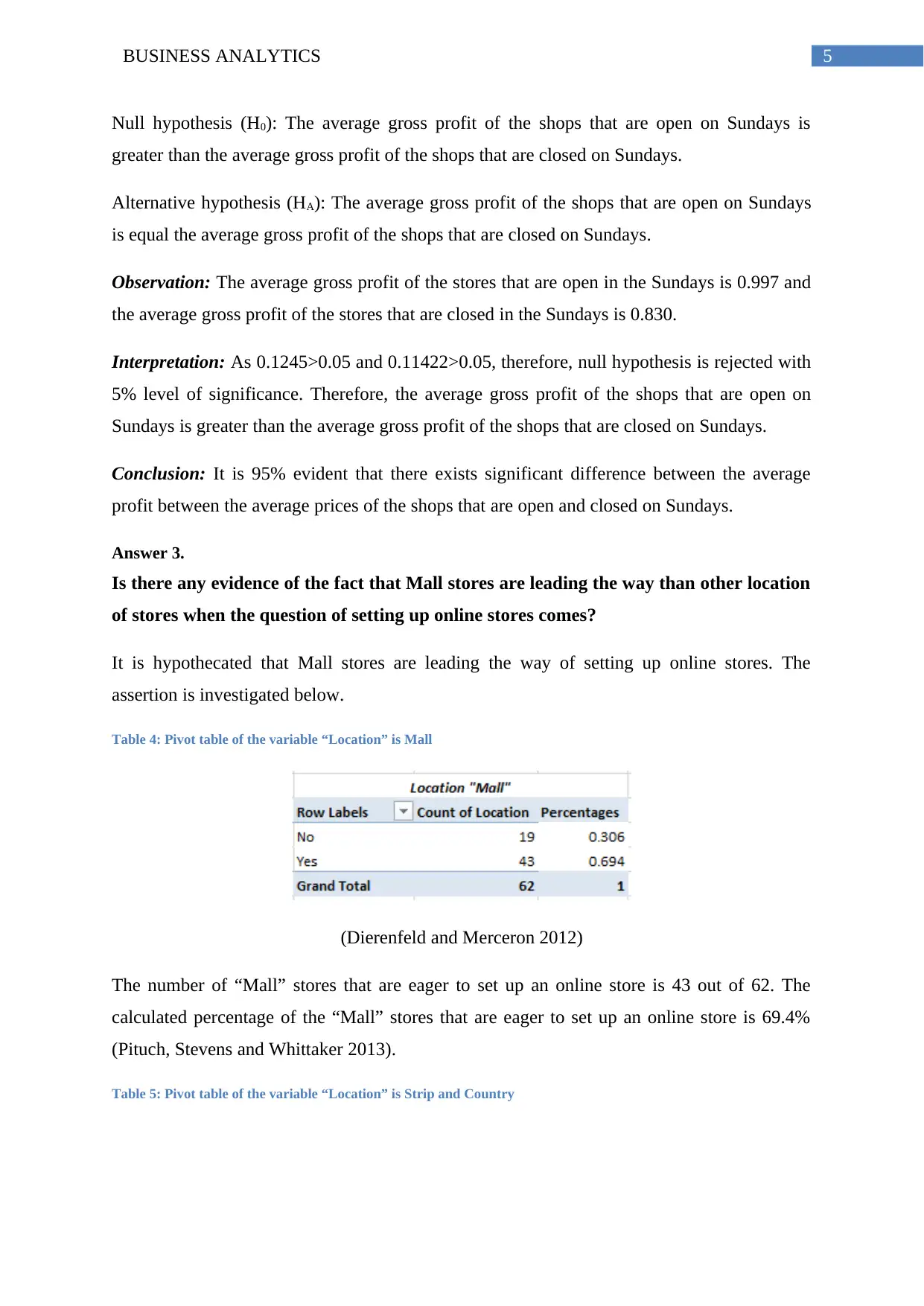
5BUSINESS ANALYTICS
Null hypothesis (H0): The average gross profit of the shops that are open on Sundays is
greater than the average gross profit of the shops that are closed on Sundays.
Alternative hypothesis (HA): The average gross profit of the shops that are open on Sundays
is equal the average gross profit of the shops that are closed on Sundays.
Observation: The average gross profit of the stores that are open in the Sundays is 0.997 and
the average gross profit of the stores that are closed in the Sundays is 0.830.
Interpretation: As 0.1245>0.05 and 0.11422>0.05, therefore, null hypothesis is rejected with
5% level of significance. Therefore, the average gross profit of the shops that are open on
Sundays is greater than the average gross profit of the shops that are closed on Sundays.
Conclusion: It is 95% evident that there exists significant difference between the average
profit between the average prices of the shops that are open and closed on Sundays.
Answer 3.
Is there any evidence of the fact that Mall stores are leading the way than other location
of stores when the question of setting up online stores comes?
It is hypothecated that Mall stores are leading the way of setting up online stores. The
assertion is investigated below.
Table 4: Pivot table of the variable “Location” is Mall
(Dierenfeld and Merceron 2012)
The number of “Mall” stores that are eager to set up an online store is 43 out of 62. The
calculated percentage of the “Mall” stores that are eager to set up an online store is 69.4%
(Pituch, Stevens and Whittaker 2013).
Table 5: Pivot table of the variable “Location” is Strip and Country
Null hypothesis (H0): The average gross profit of the shops that are open on Sundays is
greater than the average gross profit of the shops that are closed on Sundays.
Alternative hypothesis (HA): The average gross profit of the shops that are open on Sundays
is equal the average gross profit of the shops that are closed on Sundays.
Observation: The average gross profit of the stores that are open in the Sundays is 0.997 and
the average gross profit of the stores that are closed in the Sundays is 0.830.
Interpretation: As 0.1245>0.05 and 0.11422>0.05, therefore, null hypothesis is rejected with
5% level of significance. Therefore, the average gross profit of the shops that are open on
Sundays is greater than the average gross profit of the shops that are closed on Sundays.
Conclusion: It is 95% evident that there exists significant difference between the average
profit between the average prices of the shops that are open and closed on Sundays.
Answer 3.
Is there any evidence of the fact that Mall stores are leading the way than other location
of stores when the question of setting up online stores comes?
It is hypothecated that Mall stores are leading the way of setting up online stores. The
assertion is investigated below.
Table 4: Pivot table of the variable “Location” is Mall
(Dierenfeld and Merceron 2012)
The number of “Mall” stores that are eager to set up an online store is 43 out of 62. The
calculated percentage of the “Mall” stores that are eager to set up an online store is 69.4%
(Pituch, Stevens and Whittaker 2013).
Table 5: Pivot table of the variable “Location” is Strip and Country
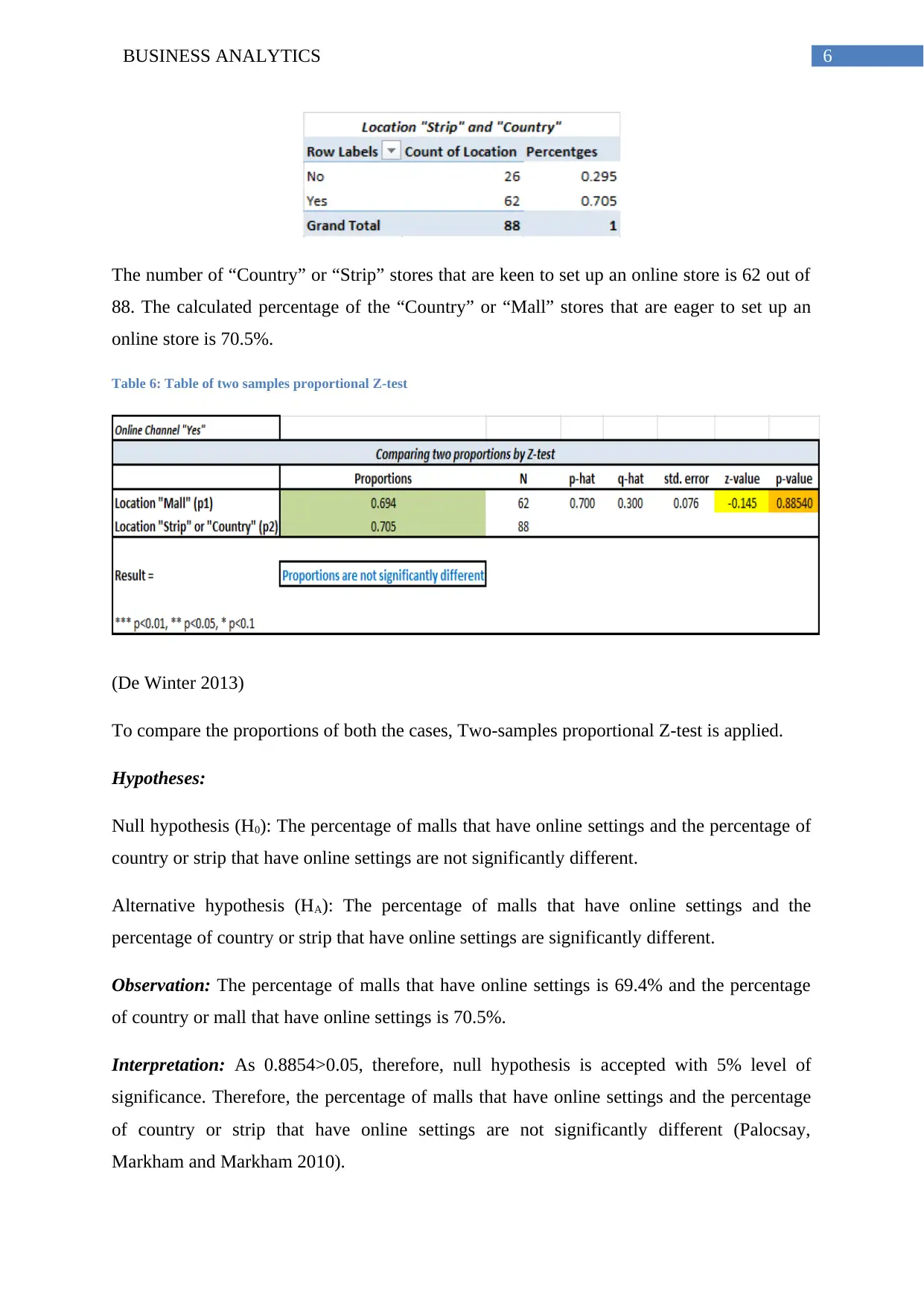
6BUSINESS ANALYTICS
The number of “Country” or “Strip” stores that are keen to set up an online store is 62 out of
88. The calculated percentage of the “Country” or “Mall” stores that are eager to set up an
online store is 70.5%.
Table 6: Table of two samples proportional Z-test
(De Winter 2013)
To compare the proportions of both the cases, Two-samples proportional Z-test is applied.
Hypotheses:
Null hypothesis (H0): The percentage of malls that have online settings and the percentage of
country or strip that have online settings are not significantly different.
Alternative hypothesis (HA): The percentage of malls that have online settings and the
percentage of country or strip that have online settings are significantly different.
Observation: The percentage of malls that have online settings is 69.4% and the percentage
of country or mall that have online settings is 70.5%.
Interpretation: As 0.8854>0.05, therefore, null hypothesis is accepted with 5% level of
significance. Therefore, the percentage of malls that have online settings and the percentage
of country or strip that have online settings are not significantly different (Palocsay,
Markham and Markham 2010).
The number of “Country” or “Strip” stores that are keen to set up an online store is 62 out of
88. The calculated percentage of the “Country” or “Mall” stores that are eager to set up an
online store is 70.5%.
Table 6: Table of two samples proportional Z-test
(De Winter 2013)
To compare the proportions of both the cases, Two-samples proportional Z-test is applied.
Hypotheses:
Null hypothesis (H0): The percentage of malls that have online settings and the percentage of
country or strip that have online settings are not significantly different.
Alternative hypothesis (HA): The percentage of malls that have online settings and the
percentage of country or strip that have online settings are significantly different.
Observation: The percentage of malls that have online settings is 69.4% and the percentage
of country or mall that have online settings is 70.5%.
Interpretation: As 0.8854>0.05, therefore, null hypothesis is accepted with 5% level of
significance. Therefore, the percentage of malls that have online settings and the percentage
of country or strip that have online settings are not significantly different (Palocsay,
Markham and Markham 2010).
Paraphrase This Document
Need a fresh take? Get an instant paraphrase of this document with our AI Paraphraser
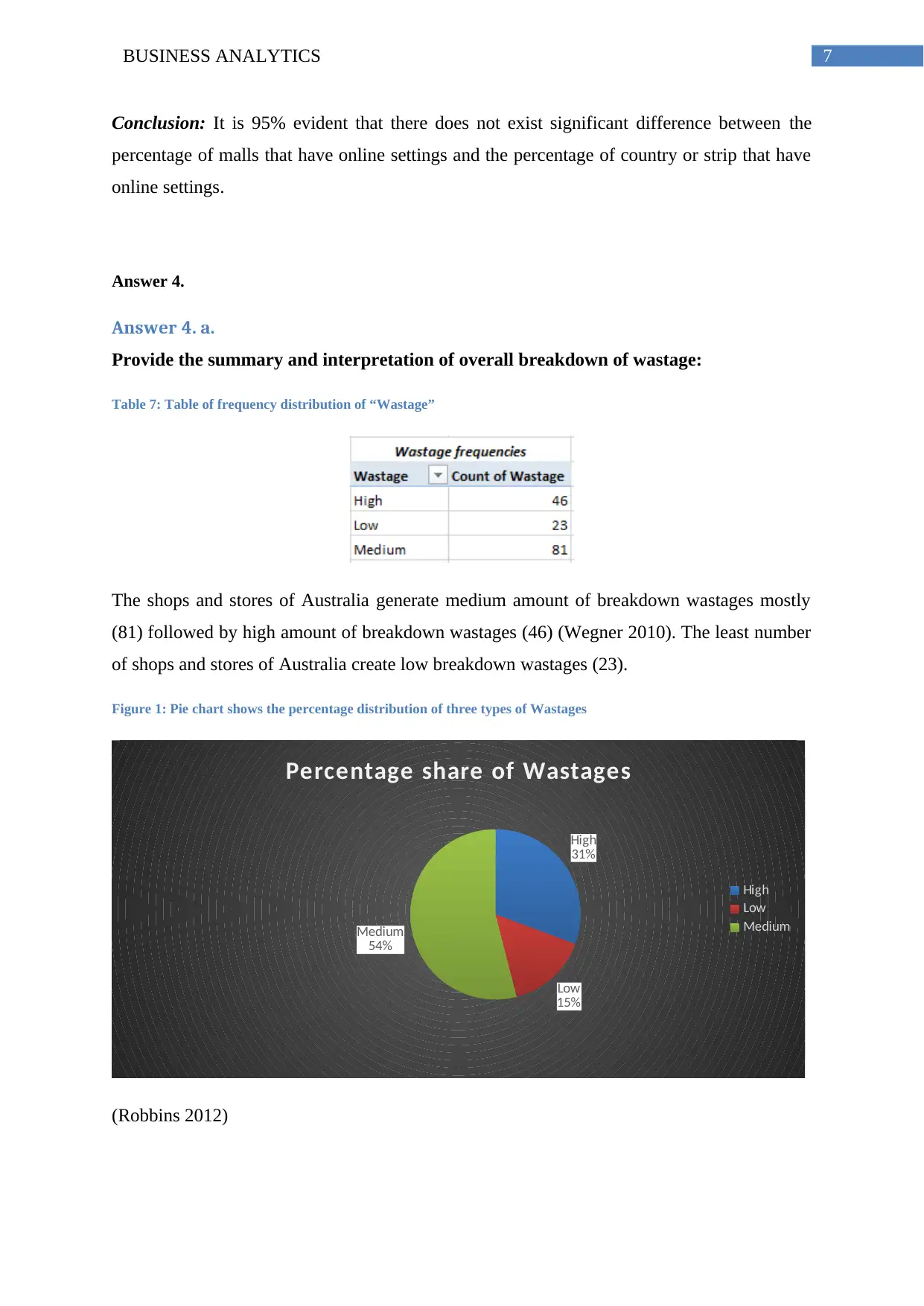
7BUSINESS ANALYTICS
Conclusion: It is 95% evident that there does not exist significant difference between the
percentage of malls that have online settings and the percentage of country or strip that have
online settings.
Answer 4.
Answer 4. a.
Provide the summary and interpretation of overall breakdown of wastage:
Table 7: Table of frequency distribution of “Wastage”
The shops and stores of Australia generate medium amount of breakdown wastages mostly
(81) followed by high amount of breakdown wastages (46) (Wegner 2010). The least number
of shops and stores of Australia create low breakdown wastages (23).
Figure 1: Pie chart shows the percentage distribution of three types of Wastages
High
31%
Low
15%
Medium
54%
Percentage share of Wastages
High
Low
Medium
(Robbins 2012)
Conclusion: It is 95% evident that there does not exist significant difference between the
percentage of malls that have online settings and the percentage of country or strip that have
online settings.
Answer 4.
Answer 4. a.
Provide the summary and interpretation of overall breakdown of wastage:
Table 7: Table of frequency distribution of “Wastage”
The shops and stores of Australia generate medium amount of breakdown wastages mostly
(81) followed by high amount of breakdown wastages (46) (Wegner 2010). The least number
of shops and stores of Australia create low breakdown wastages (23).
Figure 1: Pie chart shows the percentage distribution of three types of Wastages
High
31%
Low
15%
Medium
54%
Percentage share of Wastages
High
Low
Medium
(Robbins 2012)
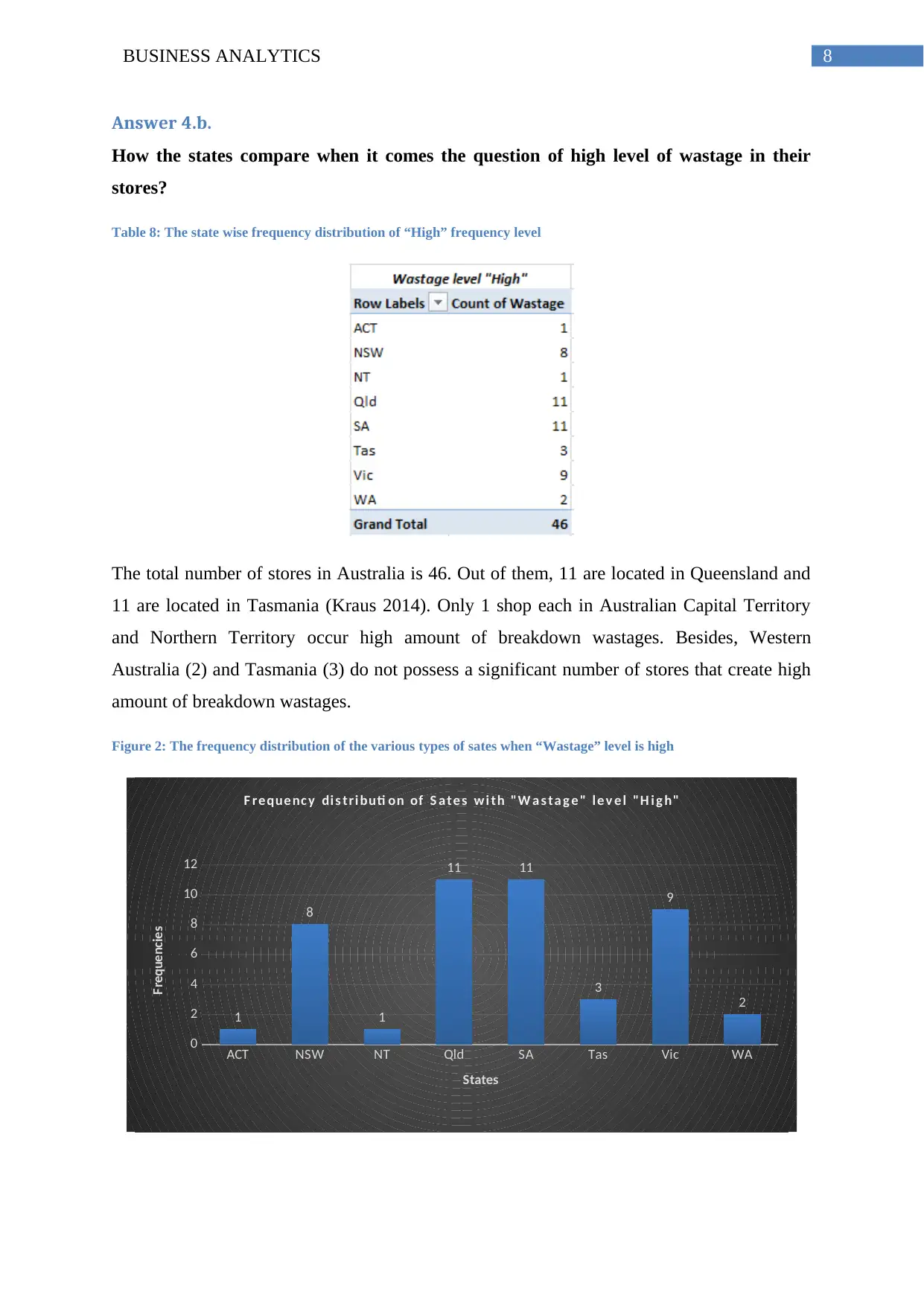
8BUSINESS ANALYTICS
Answer 4.b.
How the states compare when it comes the question of high level of wastage in their
stores?
Table 8: The state wise frequency distribution of “High” frequency level
The total number of stores in Australia is 46. Out of them, 11 are located in Queensland and
11 are located in Tasmania (Kraus 2014). Only 1 shop each in Australian Capital Territory
and Northern Territory occur high amount of breakdown wastages. Besides, Western
Australia (2) and Tasmania (3) do not possess a significant number of stores that create high
amount of breakdown wastages.
Figure 2: The frequency distribution of the various types of sates when “Wastage” level is high
ACT NSW NT Qld SA Tas Vic WA
0
2
4
6
8
10
12
1
8
1
11 11
3
9
2
F reque nc y di s tri buti on of S a te s w i th "W a s ta g e " l e v e l "H i g h"
States
Frequencies
Answer 4.b.
How the states compare when it comes the question of high level of wastage in their
stores?
Table 8: The state wise frequency distribution of “High” frequency level
The total number of stores in Australia is 46. Out of them, 11 are located in Queensland and
11 are located in Tasmania (Kraus 2014). Only 1 shop each in Australian Capital Territory
and Northern Territory occur high amount of breakdown wastages. Besides, Western
Australia (2) and Tasmania (3) do not possess a significant number of stores that create high
amount of breakdown wastages.
Figure 2: The frequency distribution of the various types of sates when “Wastage” level is high
ACT NSW NT Qld SA Tas Vic WA
0
2
4
6
8
10
12
1
8
1
11 11
3
9
2
F reque nc y di s tri buti on of S a te s w i th "W a s ta g e " l e v e l "H i g h"
States
Frequencies
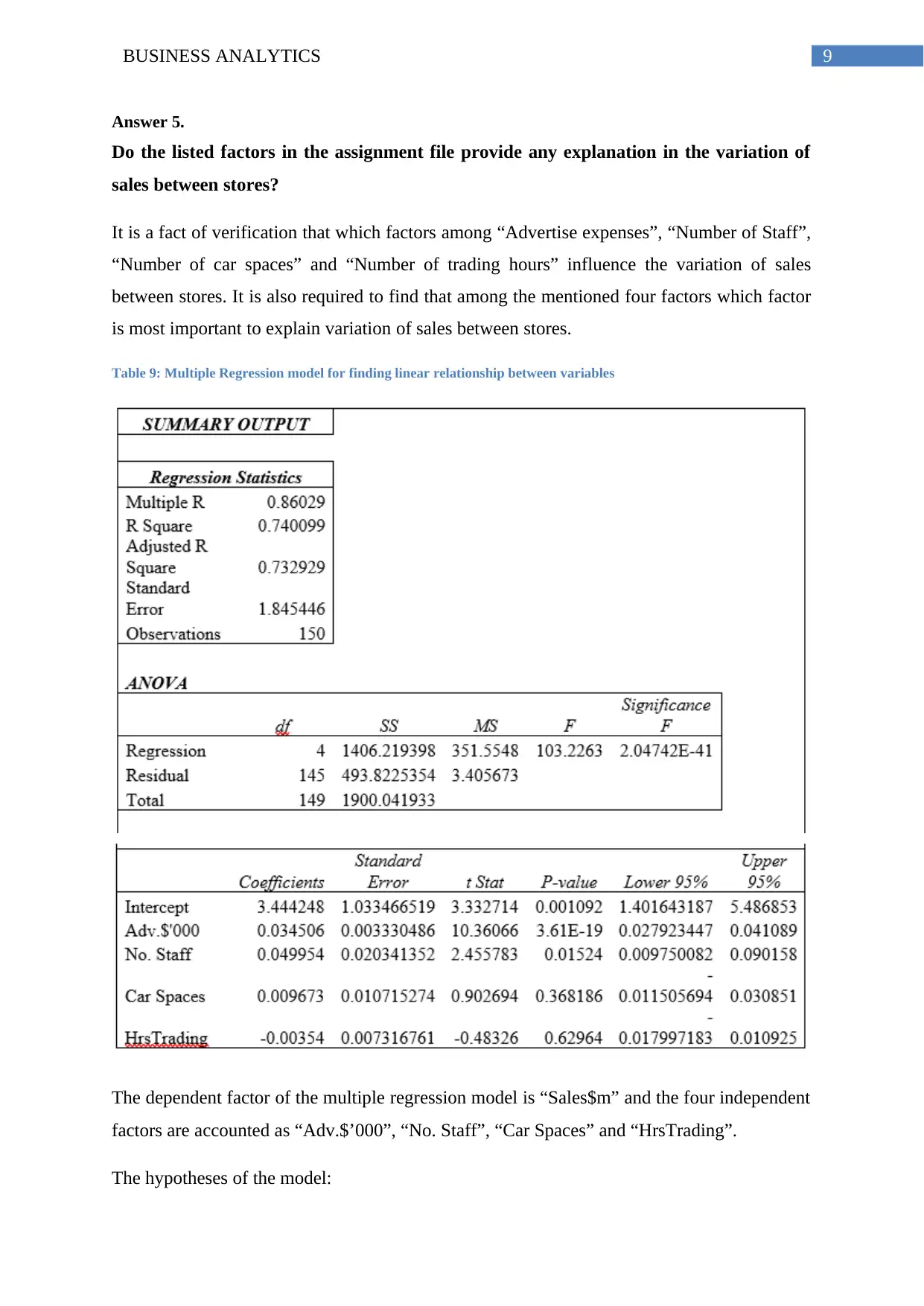
9BUSINESS ANALYTICS
Answer 5.
Do the listed factors in the assignment file provide any explanation in the variation of
sales between stores?
It is a fact of verification that which factors among “Advertise expenses”, “Number of Staff”,
“Number of car spaces” and “Number of trading hours” influence the variation of sales
between stores. It is also required to find that among the mentioned four factors which factor
is most important to explain variation of sales between stores.
Table 9: Multiple Regression model for finding linear relationship between variables
The dependent factor of the multiple regression model is “Sales$m” and the four independent
factors are accounted as “Adv.$’000”, “No. Staff”, “Car Spaces” and “HrsTrading”.
The hypotheses of the model:
Answer 5.
Do the listed factors in the assignment file provide any explanation in the variation of
sales between stores?
It is a fact of verification that which factors among “Advertise expenses”, “Number of Staff”,
“Number of car spaces” and “Number of trading hours” influence the variation of sales
between stores. It is also required to find that among the mentioned four factors which factor
is most important to explain variation of sales between stores.
Table 9: Multiple Regression model for finding linear relationship between variables
The dependent factor of the multiple regression model is “Sales$m” and the four independent
factors are accounted as “Adv.$’000”, “No. Staff”, “Car Spaces” and “HrsTrading”.
The hypotheses of the model:
Secure Best Marks with AI Grader
Need help grading? Try our AI Grader for instant feedback on your assignments.
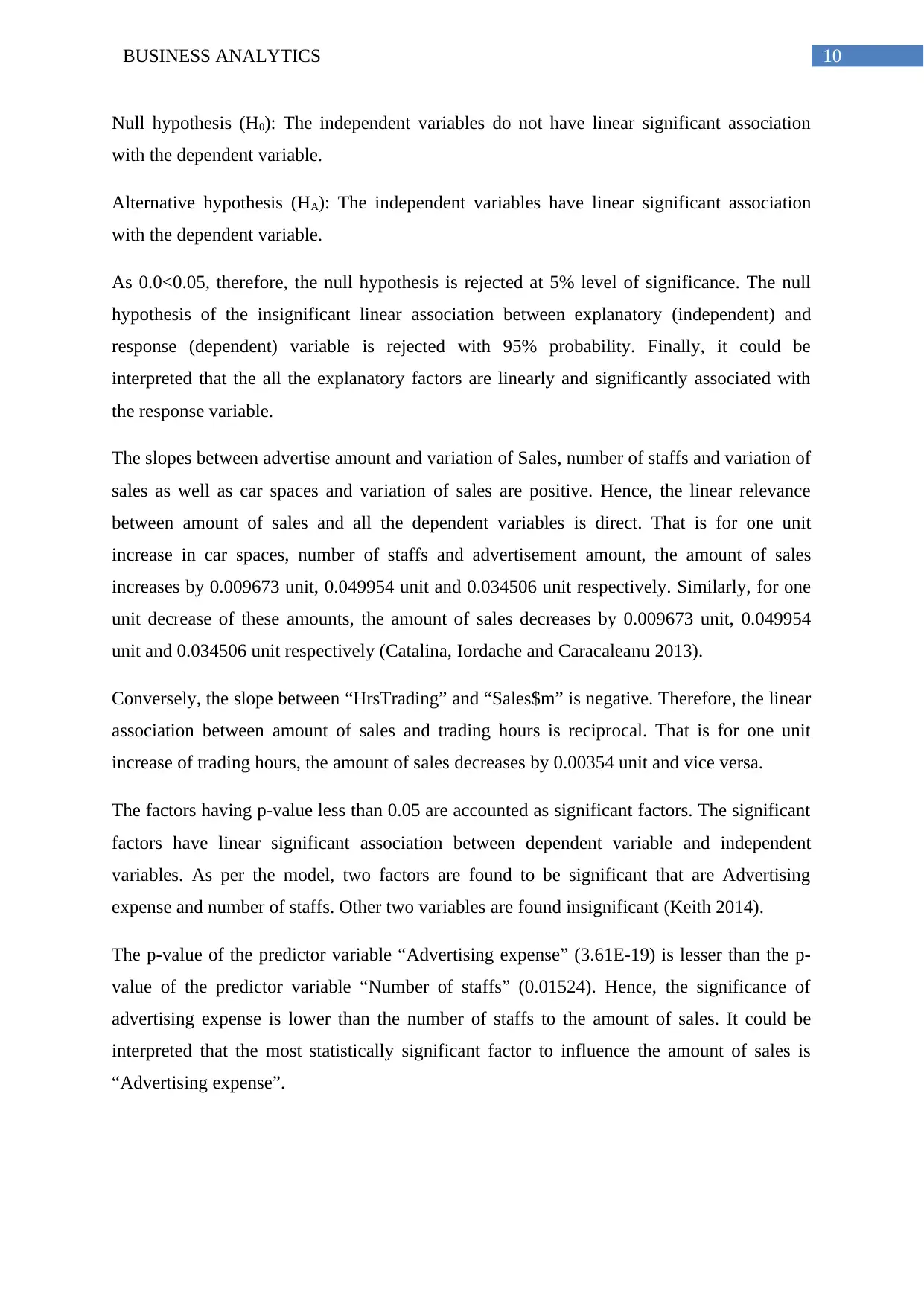
10BUSINESS ANALYTICS
Null hypothesis (H0): The independent variables do not have linear significant association
with the dependent variable.
Alternative hypothesis (HA): The independent variables have linear significant association
with the dependent variable.
As 0.0<0.05, therefore, the null hypothesis is rejected at 5% level of significance. The null
hypothesis of the insignificant linear association between explanatory (independent) and
response (dependent) variable is rejected with 95% probability. Finally, it could be
interpreted that the all the explanatory factors are linearly and significantly associated with
the response variable.
The slopes between advertise amount and variation of Sales, number of staffs and variation of
sales as well as car spaces and variation of sales are positive. Hence, the linear relevance
between amount of sales and all the dependent variables is direct. That is for one unit
increase in car spaces, number of staffs and advertisement amount, the amount of sales
increases by 0.009673 unit, 0.049954 unit and 0.034506 unit respectively. Similarly, for one
unit decrease of these amounts, the amount of sales decreases by 0.009673 unit, 0.049954
unit and 0.034506 unit respectively (Catalina, Iordache and Caracaleanu 2013).
Conversely, the slope between “HrsTrading” and “Sales$m” is negative. Therefore, the linear
association between amount of sales and trading hours is reciprocal. That is for one unit
increase of trading hours, the amount of sales decreases by 0.00354 unit and vice versa.
The factors having p-value less than 0.05 are accounted as significant factors. The significant
factors have linear significant association between dependent variable and independent
variables. As per the model, two factors are found to be significant that are Advertising
expense and number of staffs. Other two variables are found insignificant (Keith 2014).
The p-value of the predictor variable “Advertising expense” (3.61E-19) is lesser than the p-
value of the predictor variable “Number of staffs” (0.01524). Hence, the significance of
advertising expense is lower than the number of staffs to the amount of sales. It could be
interpreted that the most statistically significant factor to influence the amount of sales is
“Advertising expense”.
Null hypothesis (H0): The independent variables do not have linear significant association
with the dependent variable.
Alternative hypothesis (HA): The independent variables have linear significant association
with the dependent variable.
As 0.0<0.05, therefore, the null hypothesis is rejected at 5% level of significance. The null
hypothesis of the insignificant linear association between explanatory (independent) and
response (dependent) variable is rejected with 95% probability. Finally, it could be
interpreted that the all the explanatory factors are linearly and significantly associated with
the response variable.
The slopes between advertise amount and variation of Sales, number of staffs and variation of
sales as well as car spaces and variation of sales are positive. Hence, the linear relevance
between amount of sales and all the dependent variables is direct. That is for one unit
increase in car spaces, number of staffs and advertisement amount, the amount of sales
increases by 0.009673 unit, 0.049954 unit and 0.034506 unit respectively. Similarly, for one
unit decrease of these amounts, the amount of sales decreases by 0.009673 unit, 0.049954
unit and 0.034506 unit respectively (Catalina, Iordache and Caracaleanu 2013).
Conversely, the slope between “HrsTrading” and “Sales$m” is negative. Therefore, the linear
association between amount of sales and trading hours is reciprocal. That is for one unit
increase of trading hours, the amount of sales decreases by 0.00354 unit and vice versa.
The factors having p-value less than 0.05 are accounted as significant factors. The significant
factors have linear significant association between dependent variable and independent
variables. As per the model, two factors are found to be significant that are Advertising
expense and number of staffs. Other two variables are found insignificant (Keith 2014).
The p-value of the predictor variable “Advertising expense” (3.61E-19) is lesser than the p-
value of the predictor variable “Number of staffs” (0.01524). Hence, the significance of
advertising expense is lower than the number of staffs to the amount of sales. It could be
interpreted that the most statistically significant factor to influence the amount of sales is
“Advertising expense”.
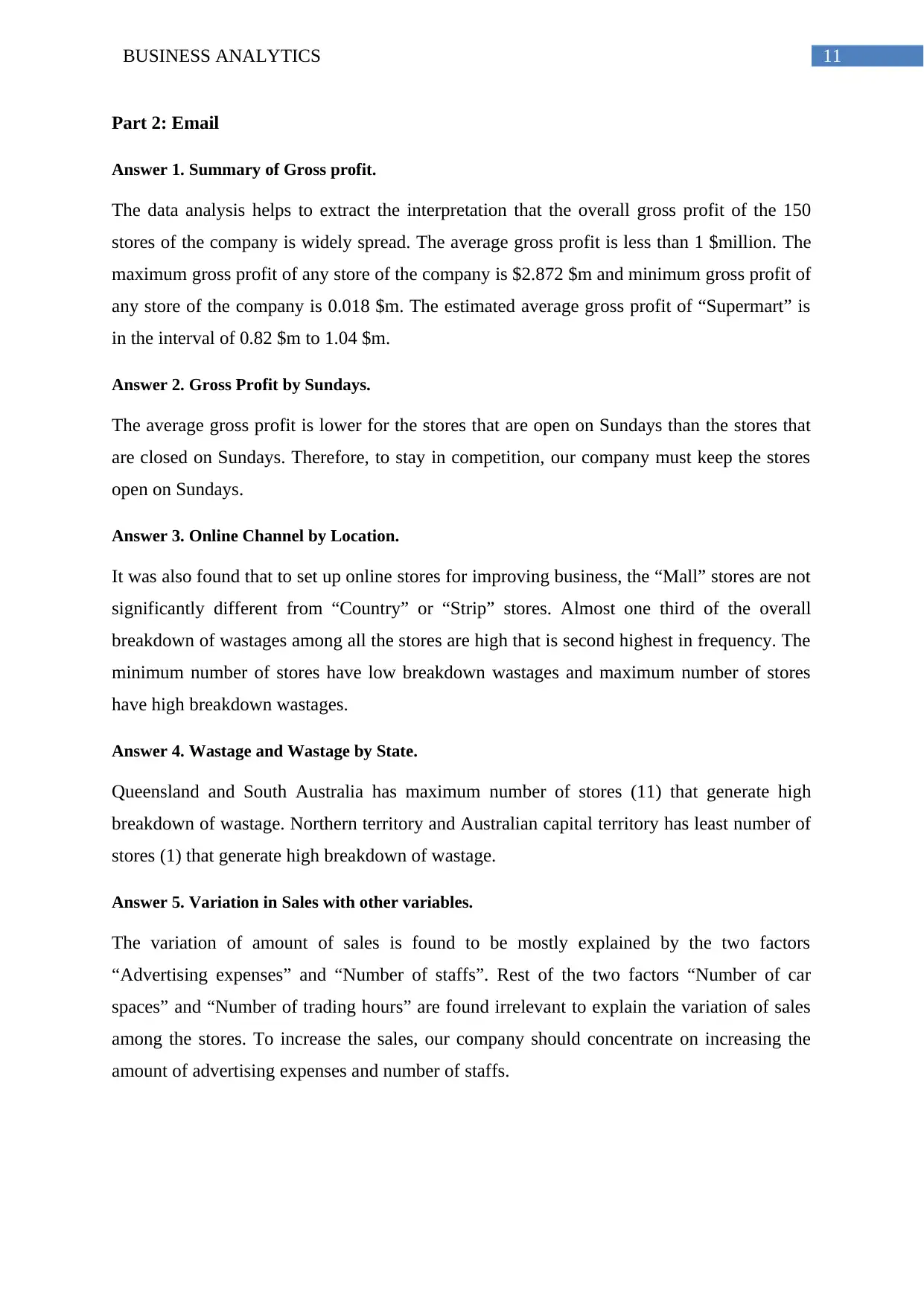
11BUSINESS ANALYTICS
Part 2: Email
Answer 1. Summary of Gross profit.
The data analysis helps to extract the interpretation that the overall gross profit of the 150
stores of the company is widely spread. The average gross profit is less than 1 $million. The
maximum gross profit of any store of the company is $2.872 $m and minimum gross profit of
any store of the company is 0.018 $m. The estimated average gross profit of “Supermart” is
in the interval of 0.82 $m to 1.04 $m.
Answer 2. Gross Profit by Sundays.
The average gross profit is lower for the stores that are open on Sundays than the stores that
are closed on Sundays. Therefore, to stay in competition, our company must keep the stores
open on Sundays.
Answer 3. Online Channel by Location.
It was also found that to set up online stores for improving business, the “Mall” stores are not
significantly different from “Country” or “Strip” stores. Almost one third of the overall
breakdown of wastages among all the stores are high that is second highest in frequency. The
minimum number of stores have low breakdown wastages and maximum number of stores
have high breakdown wastages.
Answer 4. Wastage and Wastage by State.
Queensland and South Australia has maximum number of stores (11) that generate high
breakdown of wastage. Northern territory and Australian capital territory has least number of
stores (1) that generate high breakdown of wastage.
Answer 5. Variation in Sales with other variables.
The variation of amount of sales is found to be mostly explained by the two factors
“Advertising expenses” and “Number of staffs”. Rest of the two factors “Number of car
spaces” and “Number of trading hours” are found irrelevant to explain the variation of sales
among the stores. To increase the sales, our company should concentrate on increasing the
amount of advertising expenses and number of staffs.
Part 2: Email
Answer 1. Summary of Gross profit.
The data analysis helps to extract the interpretation that the overall gross profit of the 150
stores of the company is widely spread. The average gross profit is less than 1 $million. The
maximum gross profit of any store of the company is $2.872 $m and minimum gross profit of
any store of the company is 0.018 $m. The estimated average gross profit of “Supermart” is
in the interval of 0.82 $m to 1.04 $m.
Answer 2. Gross Profit by Sundays.
The average gross profit is lower for the stores that are open on Sundays than the stores that
are closed on Sundays. Therefore, to stay in competition, our company must keep the stores
open on Sundays.
Answer 3. Online Channel by Location.
It was also found that to set up online stores for improving business, the “Mall” stores are not
significantly different from “Country” or “Strip” stores. Almost one third of the overall
breakdown of wastages among all the stores are high that is second highest in frequency. The
minimum number of stores have low breakdown wastages and maximum number of stores
have high breakdown wastages.
Answer 4. Wastage and Wastage by State.
Queensland and South Australia has maximum number of stores (11) that generate high
breakdown of wastage. Northern territory and Australian capital territory has least number of
stores (1) that generate high breakdown of wastage.
Answer 5. Variation in Sales with other variables.
The variation of amount of sales is found to be mostly explained by the two factors
“Advertising expenses” and “Number of staffs”. Rest of the two factors “Number of car
spaces” and “Number of trading hours” are found irrelevant to explain the variation of sales
among the stores. To increase the sales, our company should concentrate on increasing the
amount of advertising expenses and number of staffs.
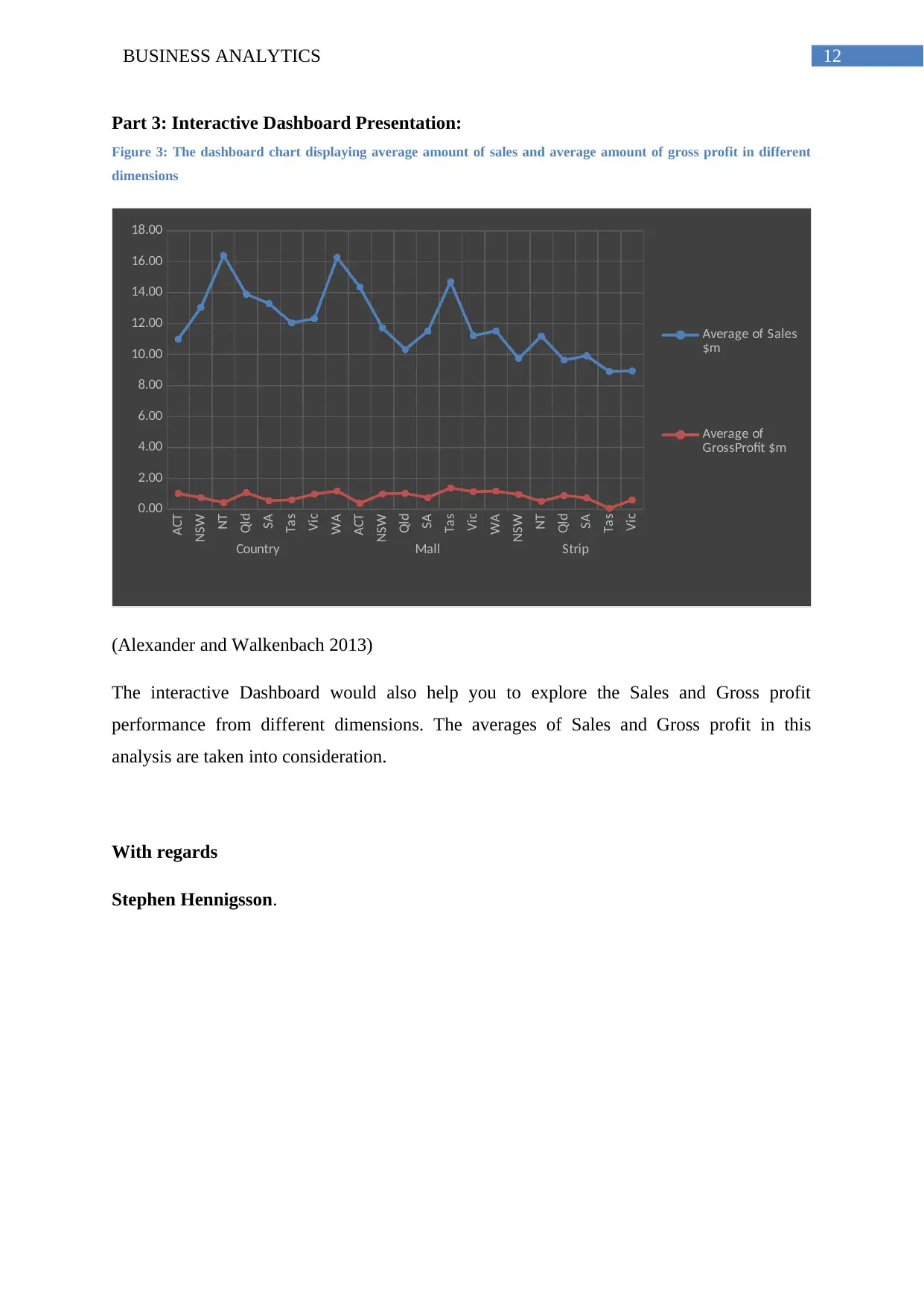
12BUSINESS ANALYTICS
Part 3: Interactive Dashboard Presentation:
Figure 3: The dashboard chart displaying average amount of sales and average amount of gross profit in different
dimensionsACT
NSW
NT
Qld
SA
Tas
Vic
WA
ACT
NSW
Qld
SA
Tas
Vic
WA
NSW
NT
Qld
SA
Tas
Vic
Country Mall Strip
0.00
2.00
4.00
6.00
8.00
10.00
12.00
14.00
16.00
18.00
Average of Sales
$m
Average of
GrossProfit $m
(Alexander and Walkenbach 2013)
The interactive Dashboard would also help you to explore the Sales and Gross profit
performance from different dimensions. The averages of Sales and Gross profit in this
analysis are taken into consideration.
With regards
Stephen Hennigsson.
Part 3: Interactive Dashboard Presentation:
Figure 3: The dashboard chart displaying average amount of sales and average amount of gross profit in different
dimensionsACT
NSW
NT
Qld
SA
Tas
Vic
WA
ACT
NSW
Qld
SA
Tas
Vic
WA
NSW
NT
Qld
SA
Tas
Vic
Country Mall Strip
0.00
2.00
4.00
6.00
8.00
10.00
12.00
14.00
16.00
18.00
Average of Sales
$m
Average of
GrossProfit $m
(Alexander and Walkenbach 2013)
The interactive Dashboard would also help you to explore the Sales and Gross profit
performance from different dimensions. The averages of Sales and Gross profit in this
analysis are taken into consideration.
With regards
Stephen Hennigsson.
Paraphrase This Document
Need a fresh take? Get an instant paraphrase of this document with our AI Paraphraser
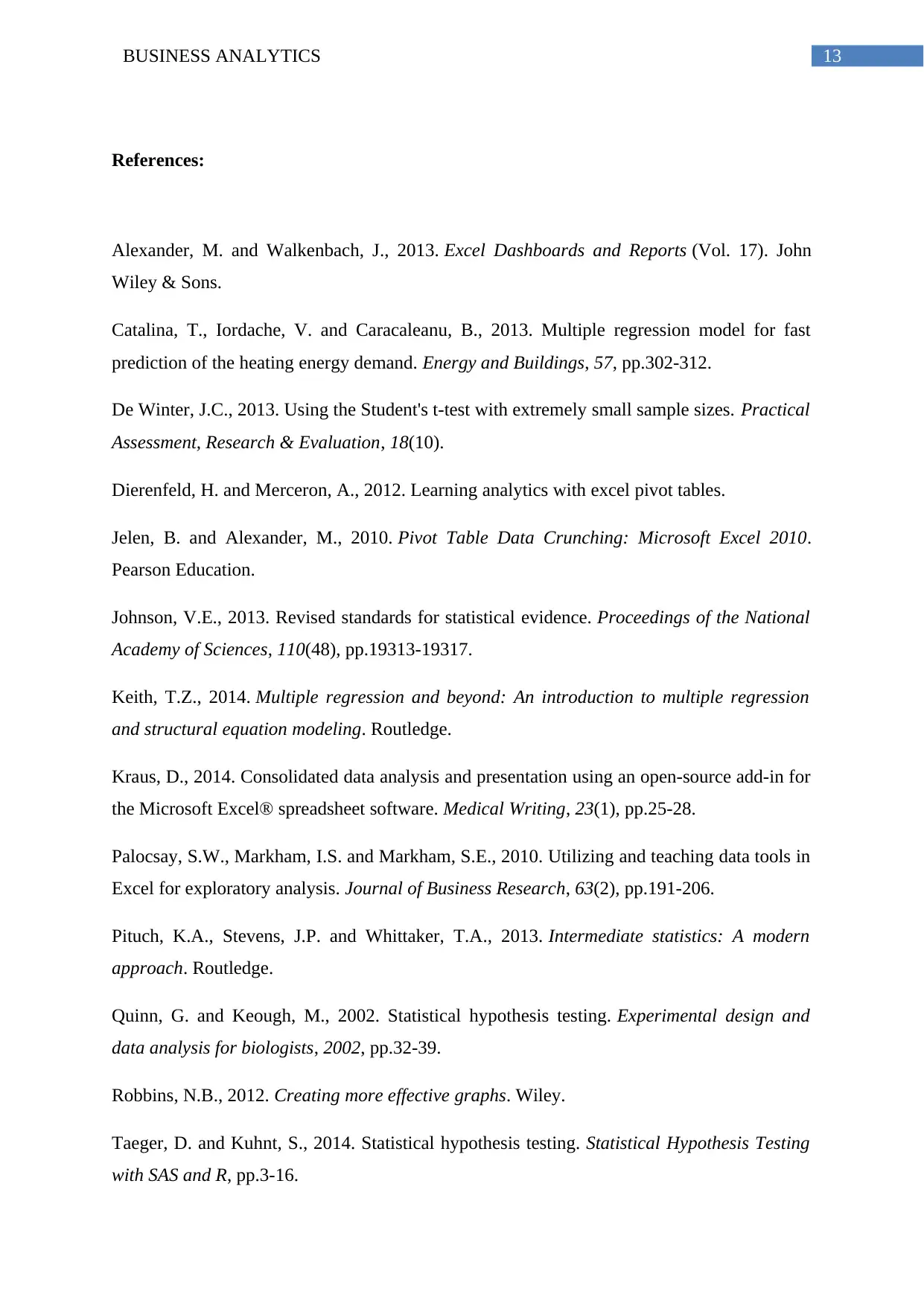
13BUSINESS ANALYTICS
References:
Alexander, M. and Walkenbach, J., 2013. Excel Dashboards and Reports (Vol. 17). John
Wiley & Sons.
Catalina, T., Iordache, V. and Caracaleanu, B., 2013. Multiple regression model for fast
prediction of the heating energy demand. Energy and Buildings, 57, pp.302-312.
De Winter, J.C., 2013. Using the Student's t-test with extremely small sample sizes. Practical
Assessment, Research & Evaluation, 18(10).
Dierenfeld, H. and Merceron, A., 2012. Learning analytics with excel pivot tables.
Jelen, B. and Alexander, M., 2010. Pivot Table Data Crunching: Microsoft Excel 2010.
Pearson Education.
Johnson, V.E., 2013. Revised standards for statistical evidence. Proceedings of the National
Academy of Sciences, 110(48), pp.19313-19317.
Keith, T.Z., 2014. Multiple regression and beyond: An introduction to multiple regression
and structural equation modeling. Routledge.
Kraus, D., 2014. Consolidated data analysis and presentation using an open-source add-in for
the Microsoft Excel® spreadsheet software. Medical Writing, 23(1), pp.25-28.
Palocsay, S.W., Markham, I.S. and Markham, S.E., 2010. Utilizing and teaching data tools in
Excel for exploratory analysis. Journal of Business Research, 63(2), pp.191-206.
Pituch, K.A., Stevens, J.P. and Whittaker, T.A., 2013. Intermediate statistics: A modern
approach. Routledge.
Quinn, G. and Keough, M., 2002. Statistical hypothesis testing. Experimental design and
data analysis for biologists, 2002, pp.32-39.
Robbins, N.B., 2012. Creating more effective graphs. Wiley.
Taeger, D. and Kuhnt, S., 2014. Statistical hypothesis testing. Statistical Hypothesis Testing
with SAS and R, pp.3-16.
References:
Alexander, M. and Walkenbach, J., 2013. Excel Dashboards and Reports (Vol. 17). John
Wiley & Sons.
Catalina, T., Iordache, V. and Caracaleanu, B., 2013. Multiple regression model for fast
prediction of the heating energy demand. Energy and Buildings, 57, pp.302-312.
De Winter, J.C., 2013. Using the Student's t-test with extremely small sample sizes. Practical
Assessment, Research & Evaluation, 18(10).
Dierenfeld, H. and Merceron, A., 2012. Learning analytics with excel pivot tables.
Jelen, B. and Alexander, M., 2010. Pivot Table Data Crunching: Microsoft Excel 2010.
Pearson Education.
Johnson, V.E., 2013. Revised standards for statistical evidence. Proceedings of the National
Academy of Sciences, 110(48), pp.19313-19317.
Keith, T.Z., 2014. Multiple regression and beyond: An introduction to multiple regression
and structural equation modeling. Routledge.
Kraus, D., 2014. Consolidated data analysis and presentation using an open-source add-in for
the Microsoft Excel® spreadsheet software. Medical Writing, 23(1), pp.25-28.
Palocsay, S.W., Markham, I.S. and Markham, S.E., 2010. Utilizing and teaching data tools in
Excel for exploratory analysis. Journal of Business Research, 63(2), pp.191-206.
Pituch, K.A., Stevens, J.P. and Whittaker, T.A., 2013. Intermediate statistics: A modern
approach. Routledge.
Quinn, G. and Keough, M., 2002. Statistical hypothesis testing. Experimental design and
data analysis for biologists, 2002, pp.32-39.
Robbins, N.B., 2012. Creating more effective graphs. Wiley.
Taeger, D. and Kuhnt, S., 2014. Statistical hypothesis testing. Statistical Hypothesis Testing
with SAS and R, pp.3-16.
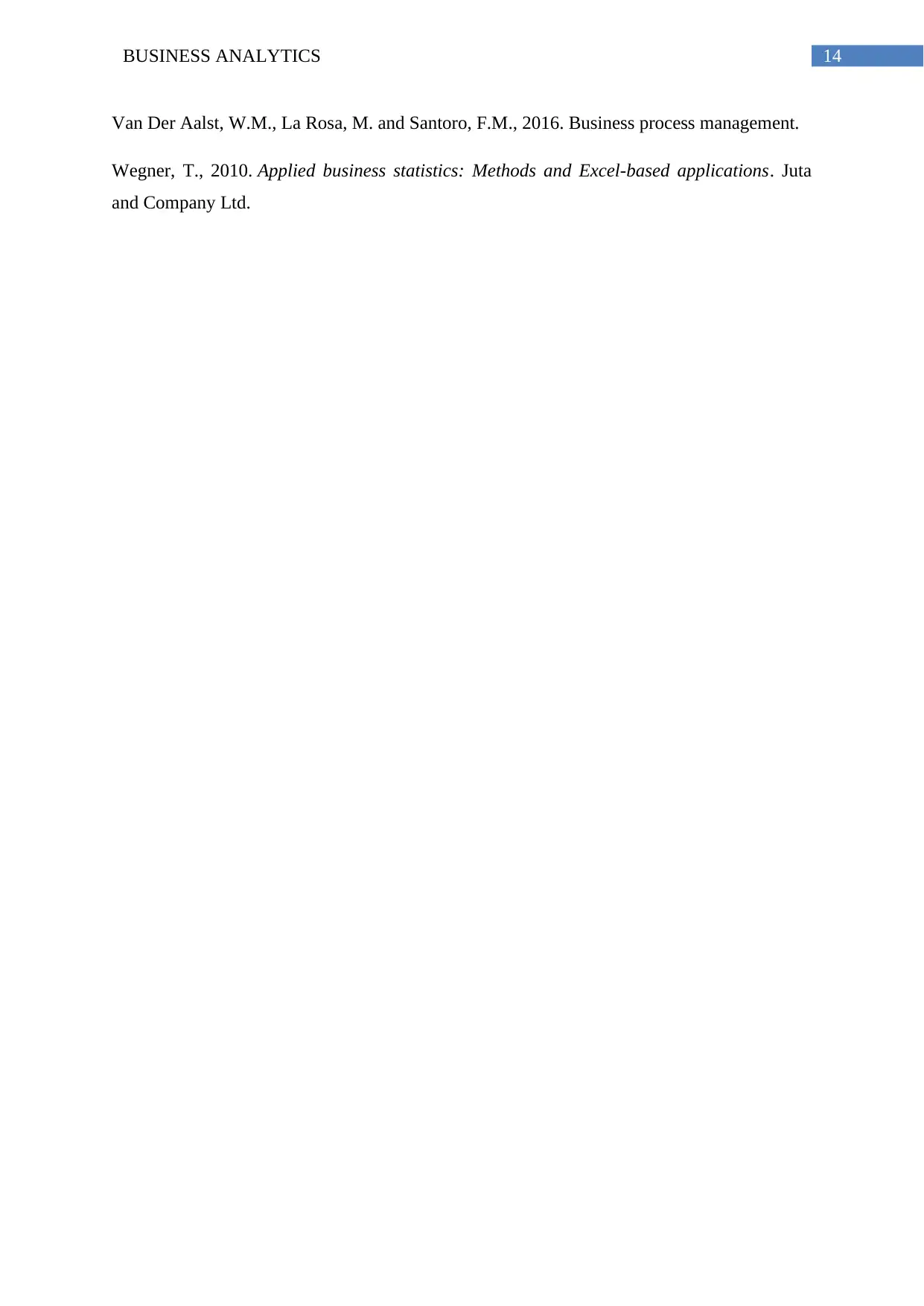
14BUSINESS ANALYTICS
Van Der Aalst, W.M., La Rosa, M. and Santoro, F.M., 2016. Business process management.
Wegner, T., 2010. Applied business statistics: Methods and Excel-based applications. Juta
and Company Ltd.
Van Der Aalst, W.M., La Rosa, M. and Santoro, F.M., 2016. Business process management.
Wegner, T., 2010. Applied business statistics: Methods and Excel-based applications. Juta
and Company Ltd.
1 out of 15
Related Documents
![[object Object]](/_next/image/?url=%2F_next%2Fstatic%2Fmedia%2Flogo.6d15ce61.png&w=640&q=75)
Your All-in-One AI-Powered Toolkit for Academic Success.
+13062052269
info@desklib.com
Available 24*7 on WhatsApp / Email
Unlock your academic potential
© 2024 | Zucol Services PVT LTD | All rights reserved.