
Business analytics
Name:
Institution:
14th March 2019
Name:
Institution:
14th March 2019
Paraphrase This Document
Need a fresh take? Get an instant paraphrase of this document with our AI Paraphraser

Applications of Business Analytics in Healthcare
Introduction
In today's world, most healthcare organizations are utilizing business analytics to analyze the big
data that they collect to improve business and clinical activities. Predictive and historical
analytics are the inspiration to guarantee essential health data is reaching the ideal individuals at
the perfect time. This empowers healthcare providers to keep track of performance much better,
recognize patterns, forecast outcomes, and ensure efficient patient care is delivered
(Djurdjanovic, et al., 2013).
Business Analytics for Performance
Clearly, the focal point of a healthcare organization is to commit 100% on ensuring that the
patients receive premium treatment and consideration while intently monitoring the business side
(Gaeth, 2018). This frequently involves settling on hard hit choices – whether workforce or
financial related – concerning resources and giving priority to initiatives.
Business analytics give managers the information they require to observe how results have
changed over a period of time, in various regions, and all through differing service categories.
With this information, managers are prepared to adequately target advertising programs, come up
with new service lines, and lift up productivity by streamlining workflow and doing away with
inefficiencies (Moreira-Matias, et al., 2016).
Moreover, business analytics enables healthcare organizations to control on costs by highlighting
and decreasing unanticipated changes in resources, volumes, contracts, and measures related to
quality. They are additionally at a point of easily identifying and executing methods that will
improve the patient's clinical outcomes (Nigrini, 2011).
Introduction
In today's world, most healthcare organizations are utilizing business analytics to analyze the big
data that they collect to improve business and clinical activities. Predictive and historical
analytics are the inspiration to guarantee essential health data is reaching the ideal individuals at
the perfect time. This empowers healthcare providers to keep track of performance much better,
recognize patterns, forecast outcomes, and ensure efficient patient care is delivered
(Djurdjanovic, et al., 2013).
Business Analytics for Performance
Clearly, the focal point of a healthcare organization is to commit 100% on ensuring that the
patients receive premium treatment and consideration while intently monitoring the business side
(Gaeth, 2018). This frequently involves settling on hard hit choices – whether workforce or
financial related – concerning resources and giving priority to initiatives.
Business analytics give managers the information they require to observe how results have
changed over a period of time, in various regions, and all through differing service categories.
With this information, managers are prepared to adequately target advertising programs, come up
with new service lines, and lift up productivity by streamlining workflow and doing away with
inefficiencies (Moreira-Matias, et al., 2016).
Moreover, business analytics enables healthcare organizations to control on costs by highlighting
and decreasing unanticipated changes in resources, volumes, contracts, and measures related to
quality. They are additionally at a point of easily identifying and executing methods that will
improve the patient's clinical outcomes (Nigrini, 2011).

Predictive Analytics
Doctors are astute, all around prepared people and we generally expect them to be aware of the
absolute best consideration for any kind of sickness or infection in the event that we or a friend
or family member get ill. Sensibly, doctors are individuals who, much the same as us, can't in
any way, know everything regardless of the amount they endeavor to stay aware of the most
recent research.
Predictive analytics utilizes innovation and statistical strategies to look through huge amounts of
data to examine it and in this way predict individual's outcomes. The data can incorporate
historical information from prior earlier treatments or admissions as well as the latest research
that are published in healthcare databases and journals.
An enormous advantage of predictive analytics is that it helps doctors with individual patients.
The believed and known treatment approach may not work best for someone in particular and
predictive analytics can enable a doctor to choose the precise treatment for such person. It isn't
astute, and can be risky, to give medications that are not required and won't work (Dhar, et al.,
2010). By giving better diagnoses and treatment focused to the individual, increasingly positive
results are obtained and less resources are utilized – including the doctor's time.
Doctors are astute, all around prepared people and we generally expect them to be aware of the
absolute best consideration for any kind of sickness or infection in the event that we or a friend
or family member get ill. Sensibly, doctors are individuals who, much the same as us, can't in
any way, know everything regardless of the amount they endeavor to stay aware of the most
recent research.
Predictive analytics utilizes innovation and statistical strategies to look through huge amounts of
data to examine it and in this way predict individual's outcomes. The data can incorporate
historical information from prior earlier treatments or admissions as well as the latest research
that are published in healthcare databases and journals.
An enormous advantage of predictive analytics is that it helps doctors with individual patients.
The believed and known treatment approach may not work best for someone in particular and
predictive analytics can enable a doctor to choose the precise treatment for such person. It isn't
astute, and can be risky, to give medications that are not required and won't work (Dhar, et al.,
2010). By giving better diagnoses and treatment focused to the individual, increasingly positive
results are obtained and less resources are utilized – including the doctor's time.
⊘ This is a preview!⊘
Do you want full access?
Subscribe today to unlock all pages.

Trusted by 1+ million students worldwide

Analysis of the data
First we look at the distribution of the victims in terms of gender. A pie chart of victim’s gender
is presented below (figure 1). As can be seen, majority of the victims were males (52%, n =
473,779) while the female victims were represented by 48% (n = 434,334).
Figure 1: Pie chart of the gender of the victims
The second analysis is on the association between gender and description status of the victims.
As can be seen in table 1 below, there seems to be slight differences in the description status of
the victims based on their gender. Majority of the two genders (male and female) were under the
category Invest Continued with the female victims under this category being 71.67% (n = 43895)
while the male victims under this category being 78.78% (n = 235386).
Table 1: Cross tabulation of gender versus description status
Status Gender Grand Total
Female Male
Adult Arrest 31272 (11.35%) 30442 (10.19%) 61714 (10.74%)
Adult Other 43895 (15.93%) 29955 (10.03%) 73850 (12.86%)
Invest Cont 197497 (71.67%) 235386 (78.78%) 432883 (75.37%)
Juv Arrest 1940 (0.70%) 2427 (0.81%) 4367 (0.76%)
Juv Other 976 (0.35%) 570 (0.19%) 1546 (0.27%)
UNK 1 (0.00%) 6 (0.00%) 7 (0.00%)
First we look at the distribution of the victims in terms of gender. A pie chart of victim’s gender
is presented below (figure 1). As can be seen, majority of the victims were males (52%, n =
473,779) while the female victims were represented by 48% (n = 434,334).
Figure 1: Pie chart of the gender of the victims
The second analysis is on the association between gender and description status of the victims.
As can be seen in table 1 below, there seems to be slight differences in the description status of
the victims based on their gender. Majority of the two genders (male and female) were under the
category Invest Continued with the female victims under this category being 71.67% (n = 43895)
while the male victims under this category being 78.78% (n = 235386).
Table 1: Cross tabulation of gender versus description status
Status Gender Grand Total
Female Male
Adult Arrest 31272 (11.35%) 30442 (10.19%) 61714 (10.74%)
Adult Other 43895 (15.93%) 29955 (10.03%) 73850 (12.86%)
Invest Cont 197497 (71.67%) 235386 (78.78%) 432883 (75.37%)
Juv Arrest 1940 (0.70%) 2427 (0.81%) 4367 (0.76%)
Juv Other 976 (0.35%) 570 (0.19%) 1546 (0.27%)
UNK 1 (0.00%) 6 (0.00%) 7 (0.00%)
Paraphrase This Document
Need a fresh take? Get an instant paraphrase of this document with our AI Paraphraser
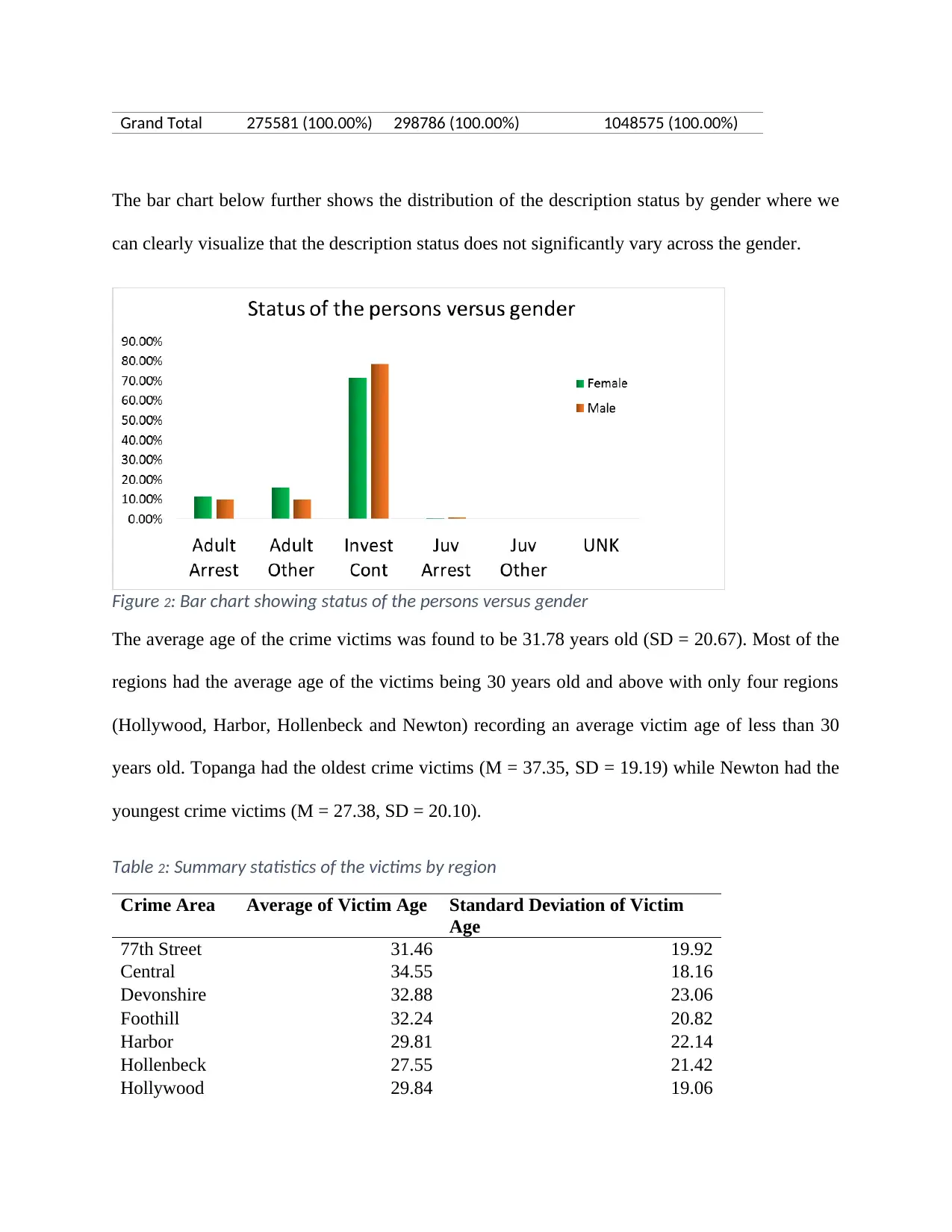
Grand Total 275581 (100.00%) 298786 (100.00%) 1048575 (100.00%)
The bar chart below further shows the distribution of the description status by gender where we
can clearly visualize that the description status does not significantly vary across the gender.
Figure 2: Bar chart showing status of the persons versus gender
The average age of the crime victims was found to be 31.78 years old (SD = 20.67). Most of the
regions had the average age of the victims being 30 years old and above with only four regions
(Hollywood, Harbor, Hollenbeck and Newton) recording an average victim age of less than 30
years old. Topanga had the oldest crime victims (M = 37.35, SD = 19.19) while Newton had the
youngest crime victims (M = 27.38, SD = 20.10).
Table 2: Summary statistics of the victims by region
Crime Area Average of Victim Age Standard Deviation of Victim
Age
77th Street 31.46 19.92
Central 34.55 18.16
Devonshire 32.88 23.06
Foothill 32.24 20.82
Harbor 29.81 22.14
Hollenbeck 27.55 21.42
Hollywood 29.84 19.06
The bar chart below further shows the distribution of the description status by gender where we
can clearly visualize that the description status does not significantly vary across the gender.
Figure 2: Bar chart showing status of the persons versus gender
The average age of the crime victims was found to be 31.78 years old (SD = 20.67). Most of the
regions had the average age of the victims being 30 years old and above with only four regions
(Hollywood, Harbor, Hollenbeck and Newton) recording an average victim age of less than 30
years old. Topanga had the oldest crime victims (M = 37.35, SD = 19.19) while Newton had the
youngest crime victims (M = 27.38, SD = 20.10).
Table 2: Summary statistics of the victims by region
Crime Area Average of Victim Age Standard Deviation of Victim
Age
77th Street 31.46 19.92
Central 34.55 18.16
Devonshire 32.88 23.06
Foothill 32.24 20.82
Harbor 29.81 22.14
Hollenbeck 27.55 21.42
Hollywood 29.84 19.06

Mission 30.77 20.37
N Hollywood 31.10 21.21
Newton 27.38 20.10
Northeast 30.99 21.42
Olympic 30.47 20.55
Pacific 32.23 21.58
Rampart 30.10 19.35
Southeast 30.44 19.64
Southwest 31.69 19.39
Topanga 37.35 19.19
Van Nuys 33.15 20.25
West LA 36.29 21.84
West Valley 34.18 22.01
Wilshire 33.30 20.45
Grand Total 31.78 20.67
The bar chart presented in figure 2 below, shows the average age of the victims by region. As
can be seen, there is no much difference in the victims age based on the region where the crime
was committed. However, it can be seen that Topanga had the oldest victims while Newton had
the youngest victims by age.
Figure 3: Bar chart on average age of the victims by region
N Hollywood 31.10 21.21
Newton 27.38 20.10
Northeast 30.99 21.42
Olympic 30.47 20.55
Pacific 32.23 21.58
Rampart 30.10 19.35
Southeast 30.44 19.64
Southwest 31.69 19.39
Topanga 37.35 19.19
Van Nuys 33.15 20.25
West LA 36.29 21.84
West Valley 34.18 22.01
Wilshire 33.30 20.45
Grand Total 31.78 20.67
The bar chart presented in figure 2 below, shows the average age of the victims by region. As
can be seen, there is no much difference in the victims age based on the region where the crime
was committed. However, it can be seen that Topanga had the oldest victims while Newton had
the youngest victims by age.
Figure 3: Bar chart on average age of the victims by region
⊘ This is a preview!⊘
Do you want full access?
Subscribe today to unlock all pages.

Trusted by 1+ million students worldwide

References
Dhar, V., Chou, D. & Provost , F., 2010. Discovering Interesting Patterns in Investment Decision
Making with GLOWER – A Genetic Learning Algorithm Overlaid With Entropy Reduction.
Data Mining and Knowledge Discovery, 4(4), pp. 75-89.
Djurdjanovic, D., Lee, J. & Ni, J., 2013. Watchdog Agent—an infotronics-based prognostics
approach for product performance degradation assessment and prediction. Advanced
Engineering Informatics, 17 ((3–4)), p. 109–125.
Gaeth, A., 2018. Evaluating Predictive Analytics for Capacity Planning. Journal of Planning,
5(3), pp. 78-91.
Moreira-Matias, L., Gama, J. & Ferreira, M., 2016. Time-evolving O-D matrix estimation using
high-speed GPS data streams. Expert Systems with Applications, 44(6), p. 275–288.
Nigrini, M., 2011. Forensic Analytics: Methods and Techniques for Forensic Accounting
Investigations. Journal of Accounting and Finance, 4(1), pp. 45-61.
Dhar, V., Chou, D. & Provost , F., 2010. Discovering Interesting Patterns in Investment Decision
Making with GLOWER – A Genetic Learning Algorithm Overlaid With Entropy Reduction.
Data Mining and Knowledge Discovery, 4(4), pp. 75-89.
Djurdjanovic, D., Lee, J. & Ni, J., 2013. Watchdog Agent—an infotronics-based prognostics
approach for product performance degradation assessment and prediction. Advanced
Engineering Informatics, 17 ((3–4)), p. 109–125.
Gaeth, A., 2018. Evaluating Predictive Analytics for Capacity Planning. Journal of Planning,
5(3), pp. 78-91.
Moreira-Matias, L., Gama, J. & Ferreira, M., 2016. Time-evolving O-D matrix estimation using
high-speed GPS data streams. Expert Systems with Applications, 44(6), p. 275–288.
Nigrini, M., 2011. Forensic Analytics: Methods and Techniques for Forensic Accounting
Investigations. Journal of Accounting and Finance, 4(1), pp. 45-61.
1 out of 7
Related Documents

Your All-in-One AI-Powered Toolkit for Academic Success.
+13062052269
info@desklib.com
Available 24*7 on WhatsApp / Email
Unlock your academic potential
© 2024 | Zucol Services PVT LTD | All rights reserved.