Business Intelligence and Data Warehousing
VerifiedAdded on 2023/01/23
|17
|1282
|22
Presentation
AI Summary
This presentation provides an introduction to business intelligence and data warehousing. It explains the importance of data management and analysis for business decision making. The presentation also explores the application of business intelligence and data warehousing in the transportation, banking, and manufacturing industries. It discusses the impact and challenges of data analysis in these industries and concludes with the advantages of using business intelligence and data warehousing for competitive advantage.
Contribute Materials
Your contribution can guide someone’s learning journey. Share your
documents today.
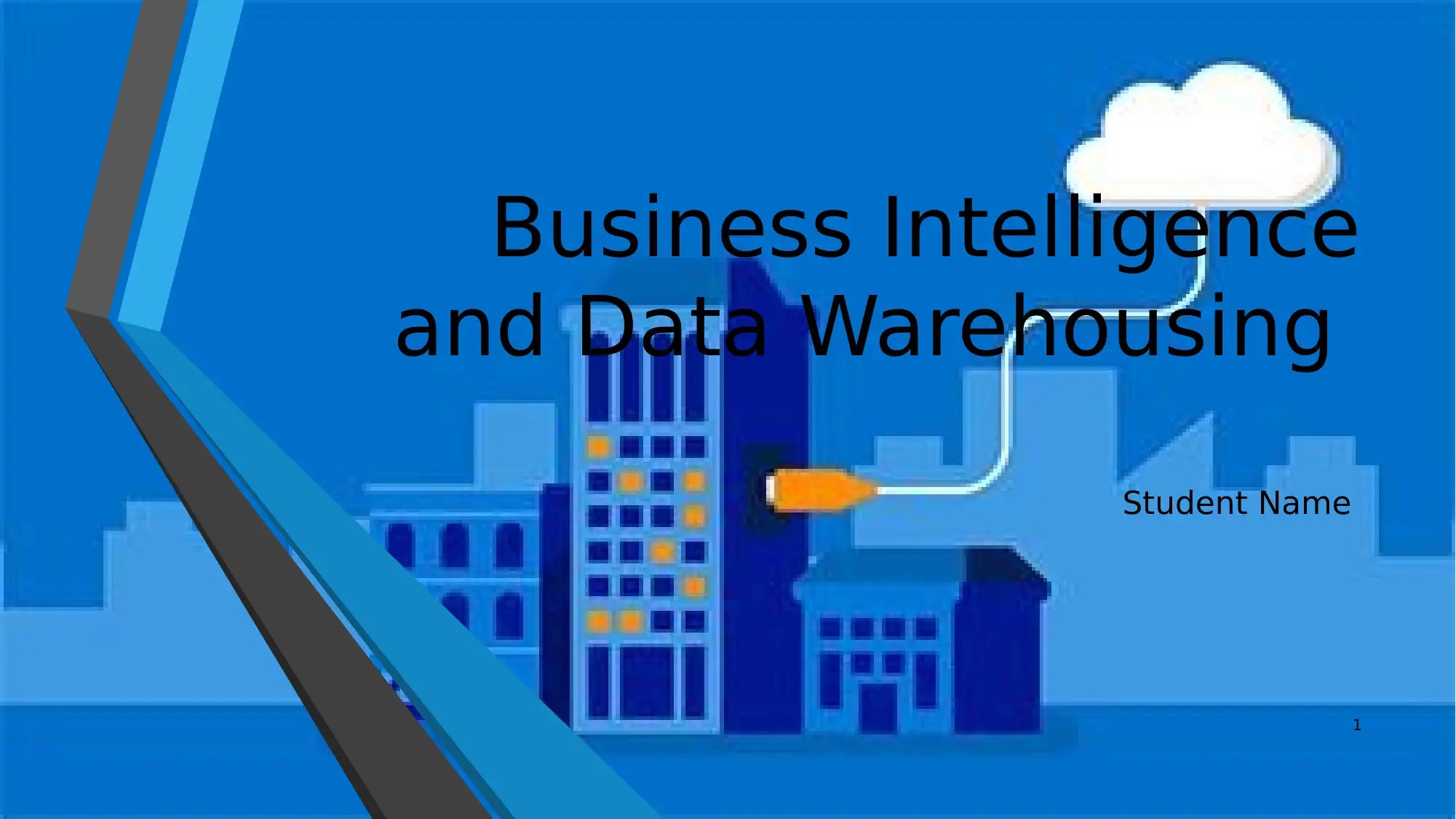
1
Business Intelligence
and Data Warehousing
Student Name
Business Intelligence
and Data Warehousing
Student Name
Secure Best Marks with AI Grader
Need help grading? Try our AI Grader for instant feedback on your assignments.
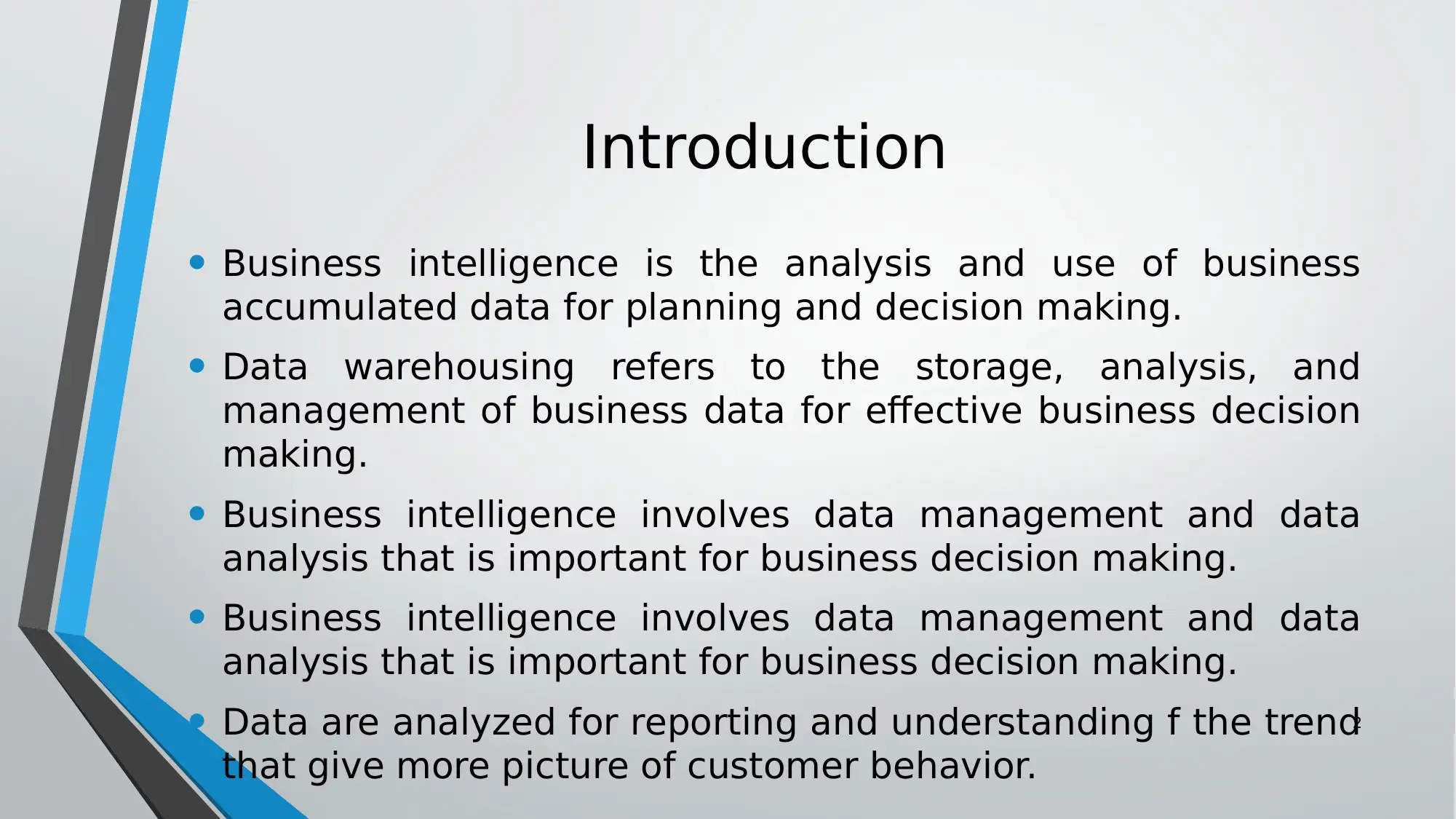
2
Introduction
• Business intelligence is the analysis and use of business
accumulated data for planning and decision making.
• Data warehousing refers to the storage, analysis, and
management of business data for effective business decision
making.
• Business intelligence involves data management and data
analysis that is important for business decision making.
• Business intelligence involves data management and data
analysis that is important for business decision making.
• Data are analyzed for reporting and understanding f the trend
that give more picture of customer behavior.
Introduction
• Business intelligence is the analysis and use of business
accumulated data for planning and decision making.
• Data warehousing refers to the storage, analysis, and
management of business data for effective business decision
making.
• Business intelligence involves data management and data
analysis that is important for business decision making.
• Business intelligence involves data management and data
analysis that is important for business decision making.
• Data are analyzed for reporting and understanding f the trend
that give more picture of customer behavior.
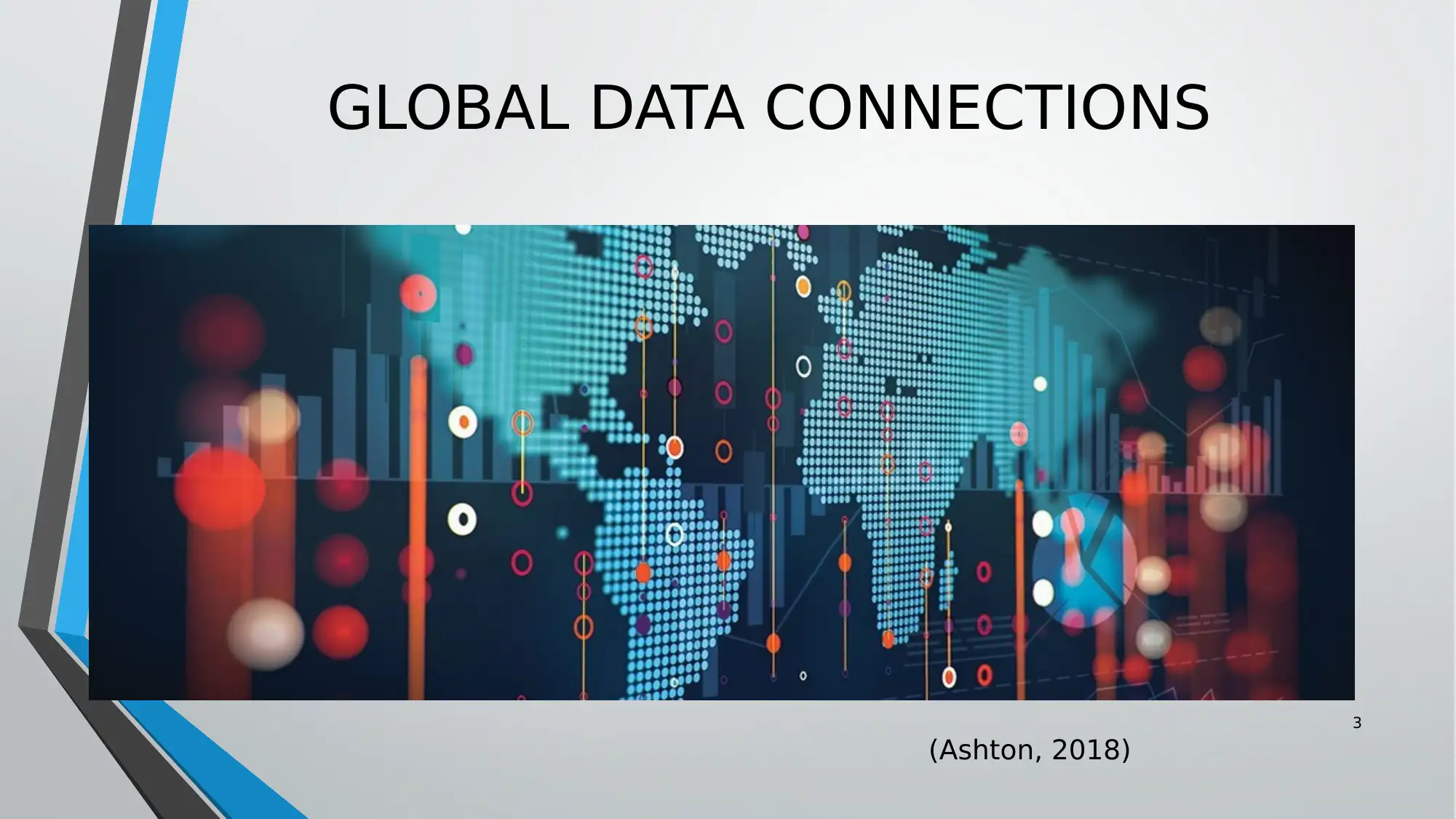
3
GLOBAL DATA CONNECTIONS
(Ashton, 2018)
GLOBAL DATA CONNECTIONS
(Ashton, 2018)
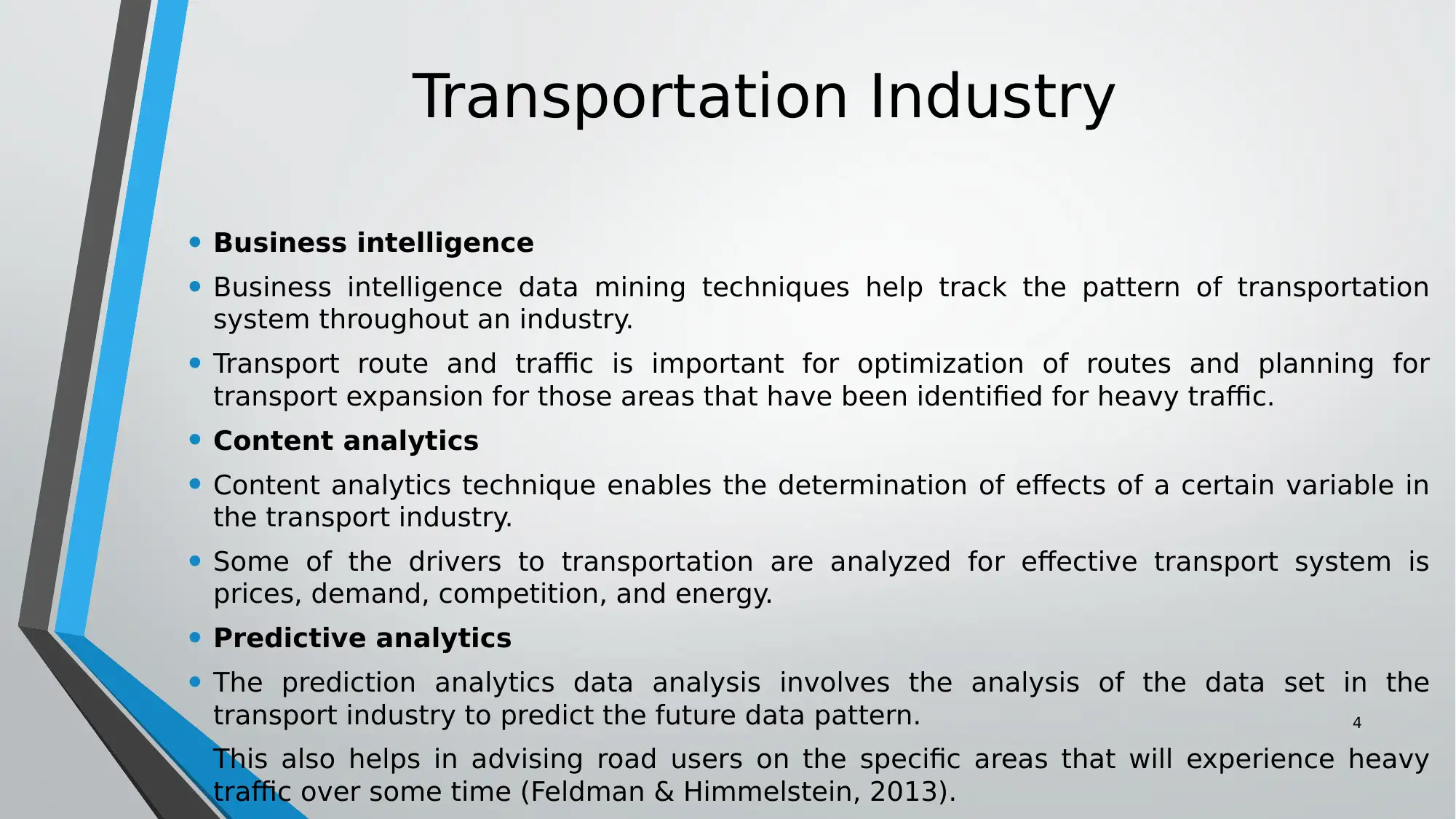
4
Transportation Industry
• Business intelligence
• Business intelligence data mining techniques help track the pattern of transportation
system throughout an industry.
• Transport route and traffic is important for optimization of routes and planning for
transport expansion for those areas that have been identified for heavy traffic.
• Content analytics
• Content analytics technique enables the determination of effects of a certain variable in
the transport industry.
• Some of the drivers to transportation are analyzed for effective transport system is
prices, demand, competition, and energy.
• Predictive analytics
• The prediction analytics data analysis involves the analysis of the data set in the
transport industry to predict the future data pattern.
• This also helps in advising road users on the specific areas that will experience heavy
traffic over some time (Feldman & Himmelstein, 2013).
Transportation Industry
• Business intelligence
• Business intelligence data mining techniques help track the pattern of transportation
system throughout an industry.
• Transport route and traffic is important for optimization of routes and planning for
transport expansion for those areas that have been identified for heavy traffic.
• Content analytics
• Content analytics technique enables the determination of effects of a certain variable in
the transport industry.
• Some of the drivers to transportation are analyzed for effective transport system is
prices, demand, competition, and energy.
• Predictive analytics
• The prediction analytics data analysis involves the analysis of the data set in the
transport industry to predict the future data pattern.
• This also helps in advising road users on the specific areas that will experience heavy
traffic over some time (Feldman & Himmelstein, 2013).
Secure Best Marks with AI Grader
Need help grading? Try our AI Grader for instant feedback on your assignments.
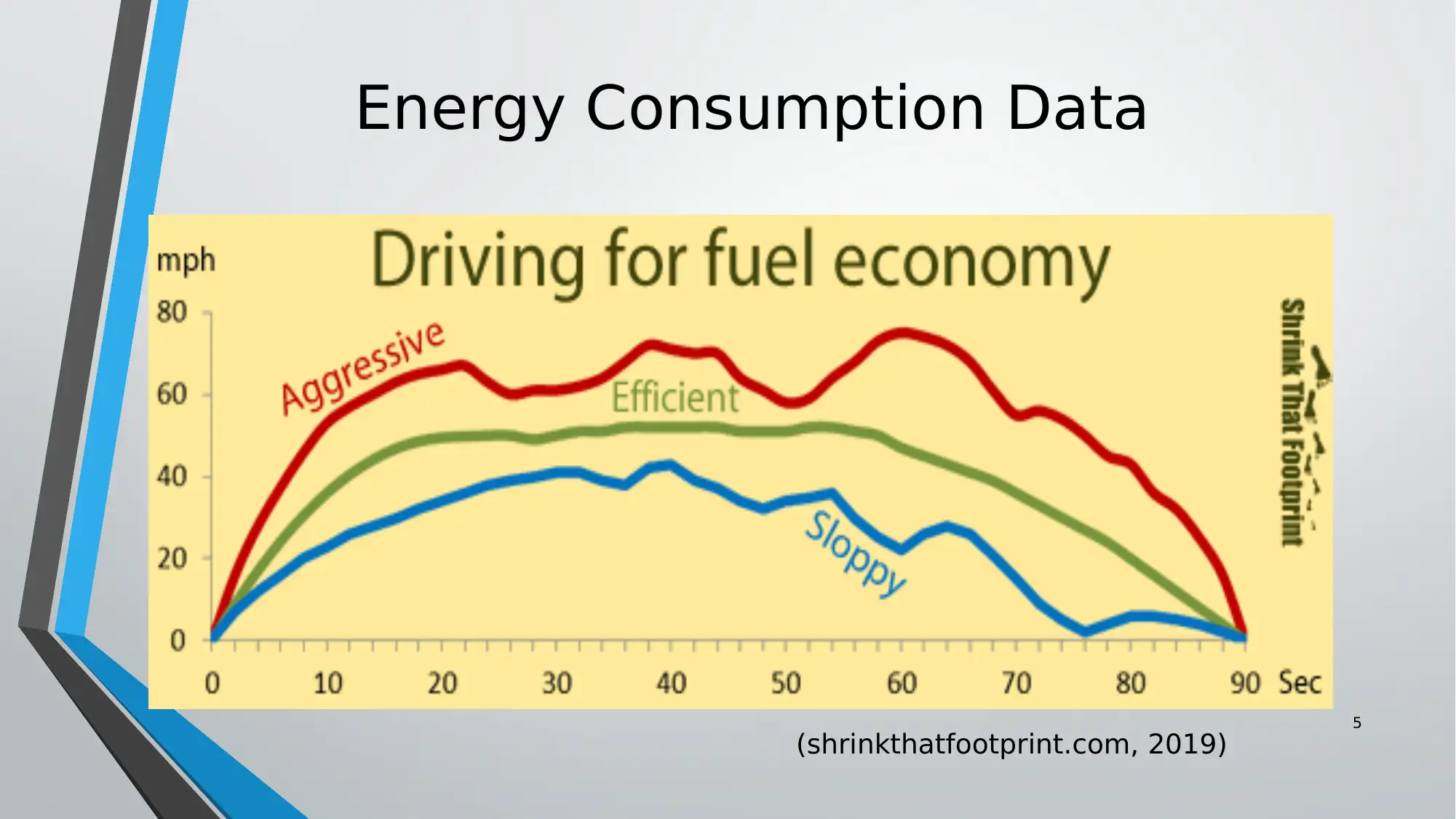
5
Energy Consumption Data
(shrinkthatfootprint.com, 2019)
Energy Consumption Data
(shrinkthatfootprint.com, 2019)
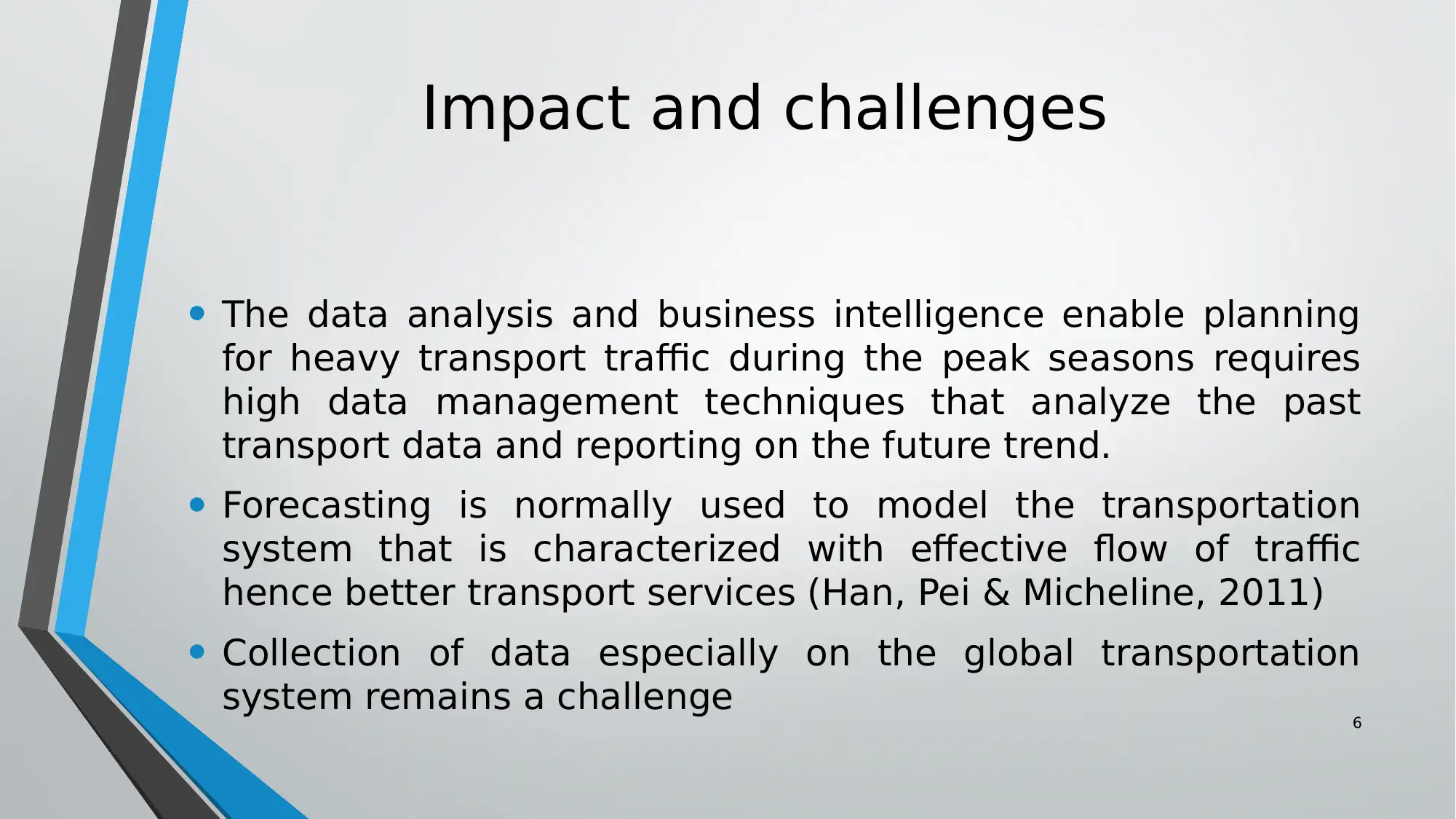
6
Impact and challenges
• The data analysis and business intelligence enable planning
for heavy transport traffic during the peak seasons requires
high data management techniques that analyze the past
transport data and reporting on the future trend.
• Forecasting is normally used to model the transportation
system that is characterized with effective flow of traffic
hence better transport services (Han, Pei & Micheline, 2011)
• Collection of data especially on the global transportation
system remains a challenge
Impact and challenges
• The data analysis and business intelligence enable planning
for heavy transport traffic during the peak seasons requires
high data management techniques that analyze the past
transport data and reporting on the future trend.
• Forecasting is normally used to model the transportation
system that is characterized with effective flow of traffic
hence better transport services (Han, Pei & Micheline, 2011)
• Collection of data especially on the global transportation
system remains a challenge
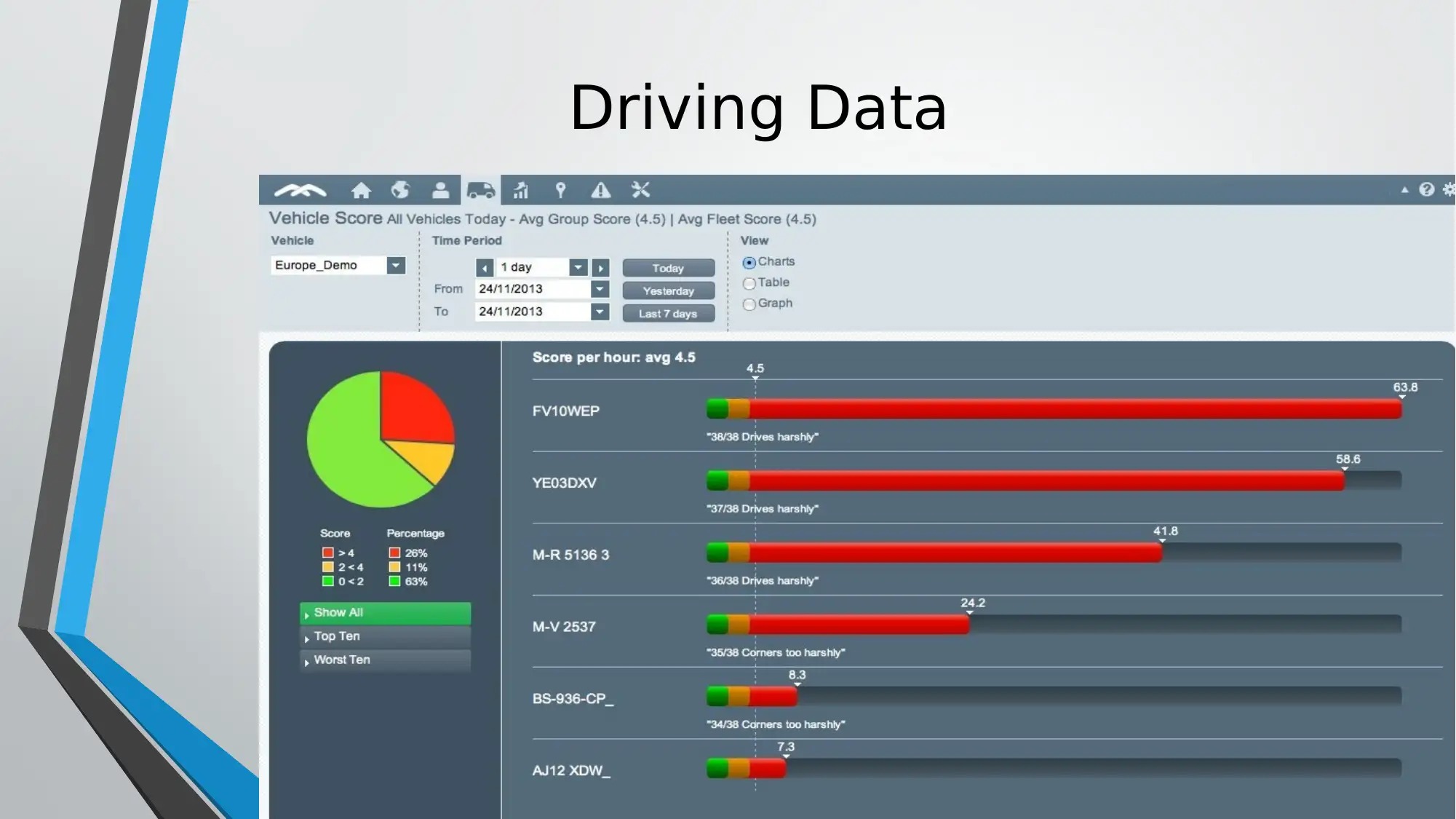
7
Driving Data
Driving Data
Paraphrase This Document
Need a fresh take? Get an instant paraphrase of this document with our AI Paraphraser
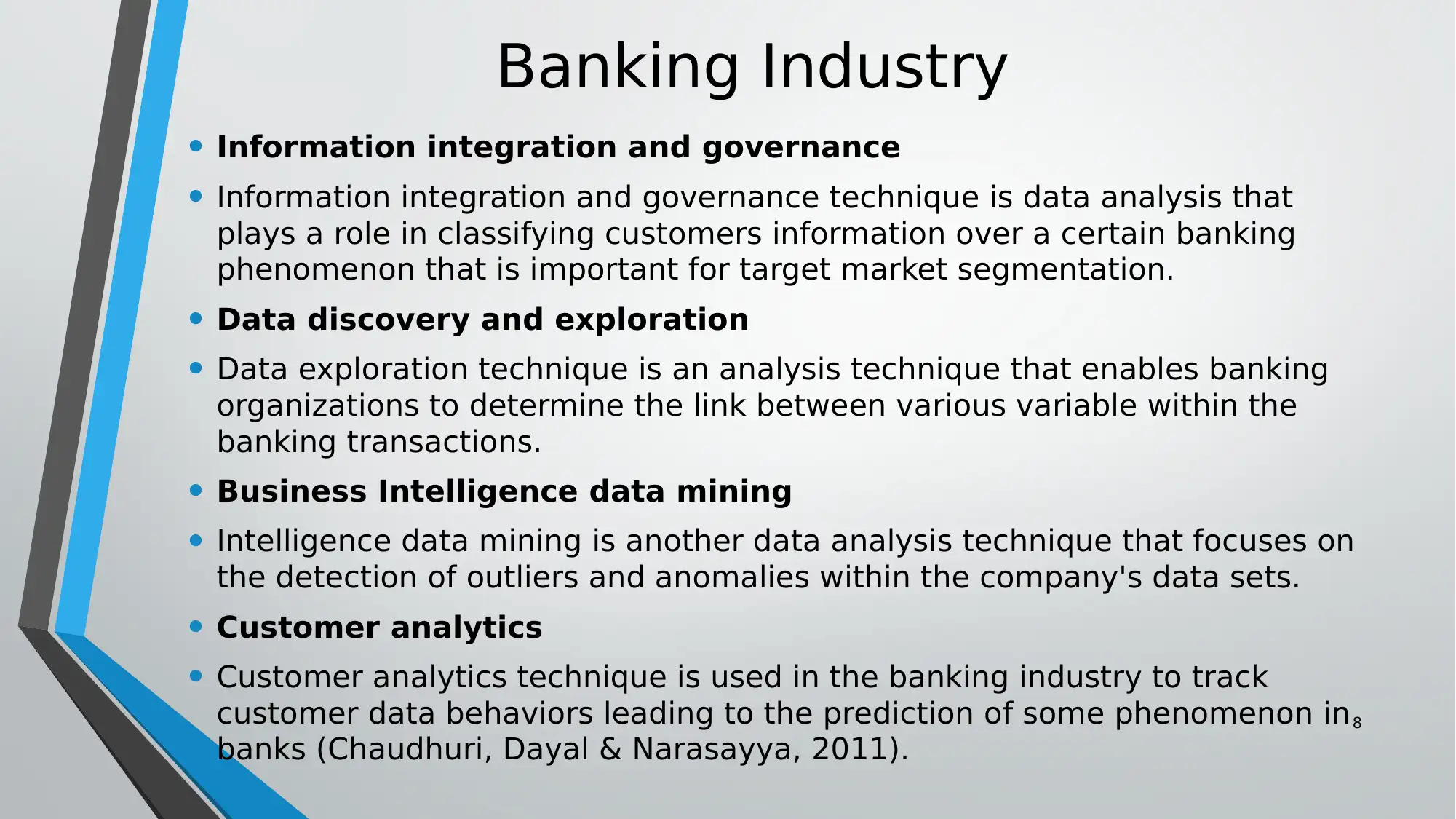
8
Banking Industry
• Information integration and governance
• Information integration and governance technique is data analysis that
plays a role in classifying customers information over a certain banking
phenomenon that is important for target market segmentation.
• Data discovery and exploration
• Data exploration technique is an analysis technique that enables banking
organizations to determine the link between various variable within the
banking transactions.
• Business Intelligence data mining
• Intelligence data mining is another data analysis technique that focuses on
the detection of outliers and anomalies within the company's data sets.
• Customer analytics
• Customer analytics technique is used in the banking industry to track
customer data behaviors leading to the prediction of some phenomenon in
banks (Chaudhuri, Dayal & Narasayya, 2011).
Banking Industry
• Information integration and governance
• Information integration and governance technique is data analysis that
plays a role in classifying customers information over a certain banking
phenomenon that is important for target market segmentation.
• Data discovery and exploration
• Data exploration technique is an analysis technique that enables banking
organizations to determine the link between various variable within the
banking transactions.
• Business Intelligence data mining
• Intelligence data mining is another data analysis technique that focuses on
the detection of outliers and anomalies within the company's data sets.
• Customer analytics
• Customer analytics technique is used in the banking industry to track
customer data behaviors leading to the prediction of some phenomenon in
banks (Chaudhuri, Dayal & Narasayya, 2011).
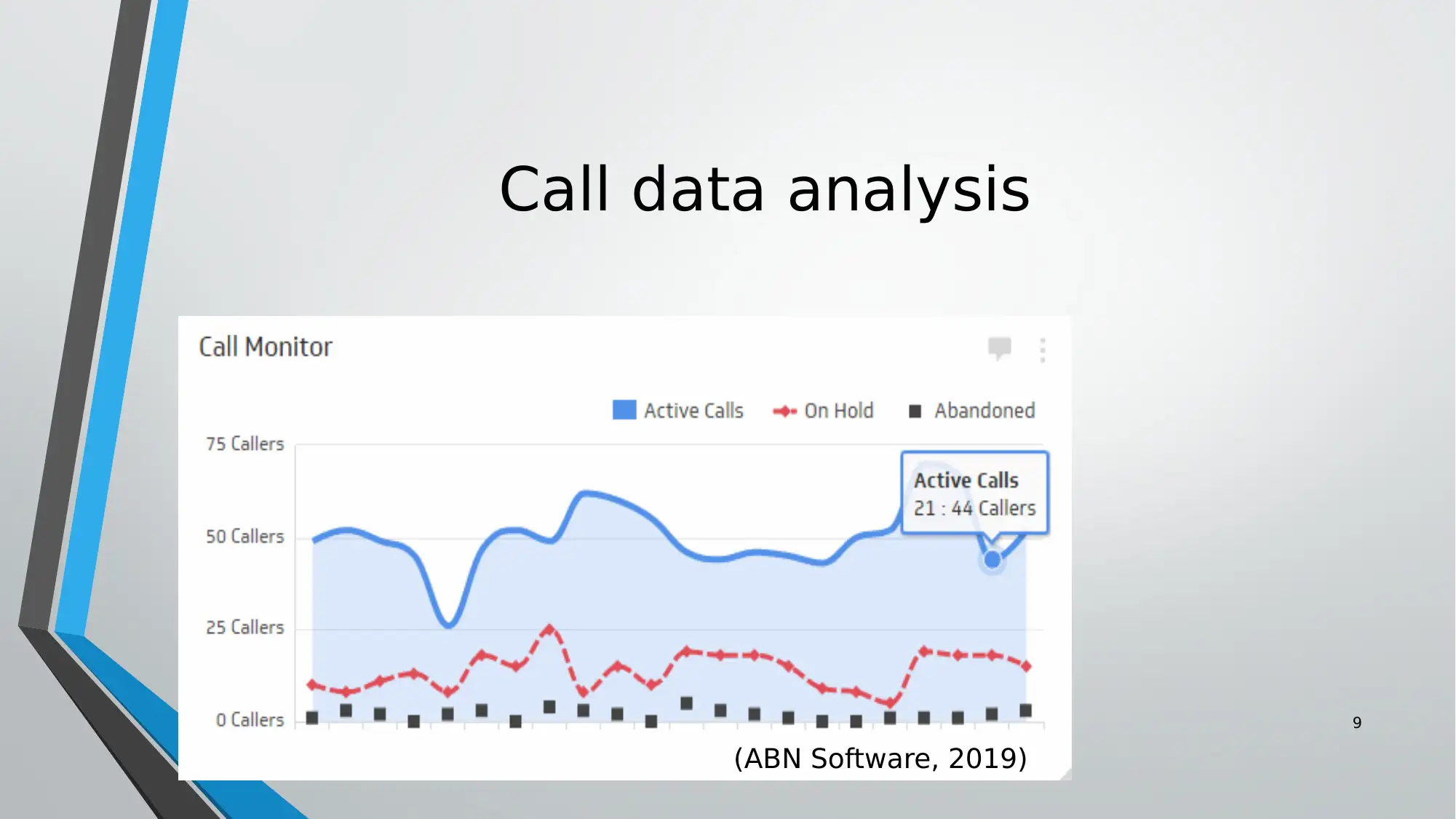
9
Call data analysis
(ABN Software, 2019)
Call data analysis
(ABN Software, 2019)
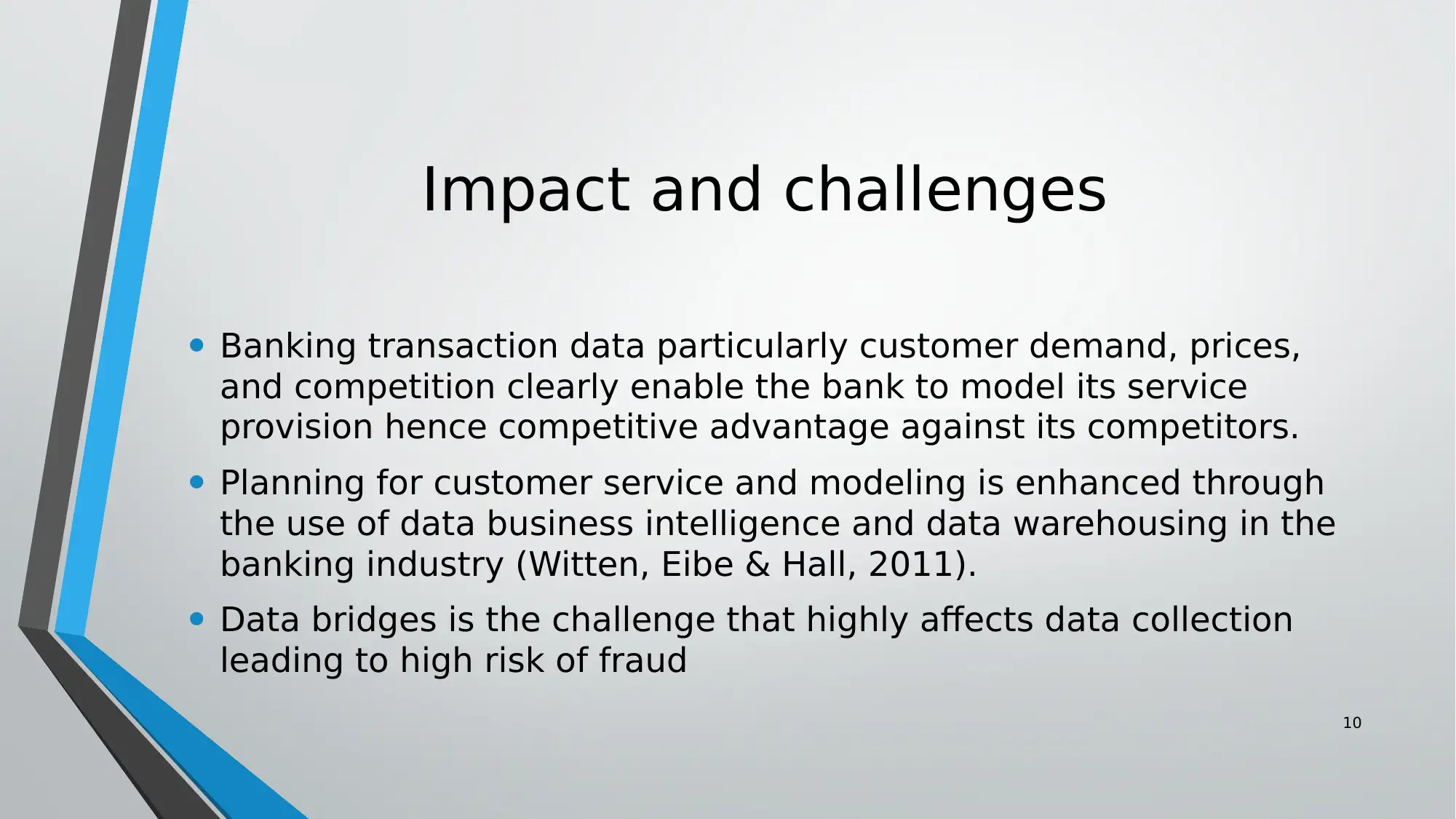
10
Impact and challenges
• Banking transaction data particularly customer demand, prices,
and competition clearly enable the bank to model its service
provision hence competitive advantage against its competitors.
• Planning for customer service and modeling is enhanced through
the use of data business intelligence and data warehousing in the
banking industry (Witten, Eibe & Hall, 2011).
• Data bridges is the challenge that highly affects data collection
leading to high risk of fraud
Impact and challenges
• Banking transaction data particularly customer demand, prices,
and competition clearly enable the bank to model its service
provision hence competitive advantage against its competitors.
• Planning for customer service and modeling is enhanced through
the use of data business intelligence and data warehousing in the
banking industry (Witten, Eibe & Hall, 2011).
• Data bridges is the challenge that highly affects data collection
leading to high risk of fraud
Secure Best Marks with AI Grader
Need help grading? Try our AI Grader for instant feedback on your assignments.
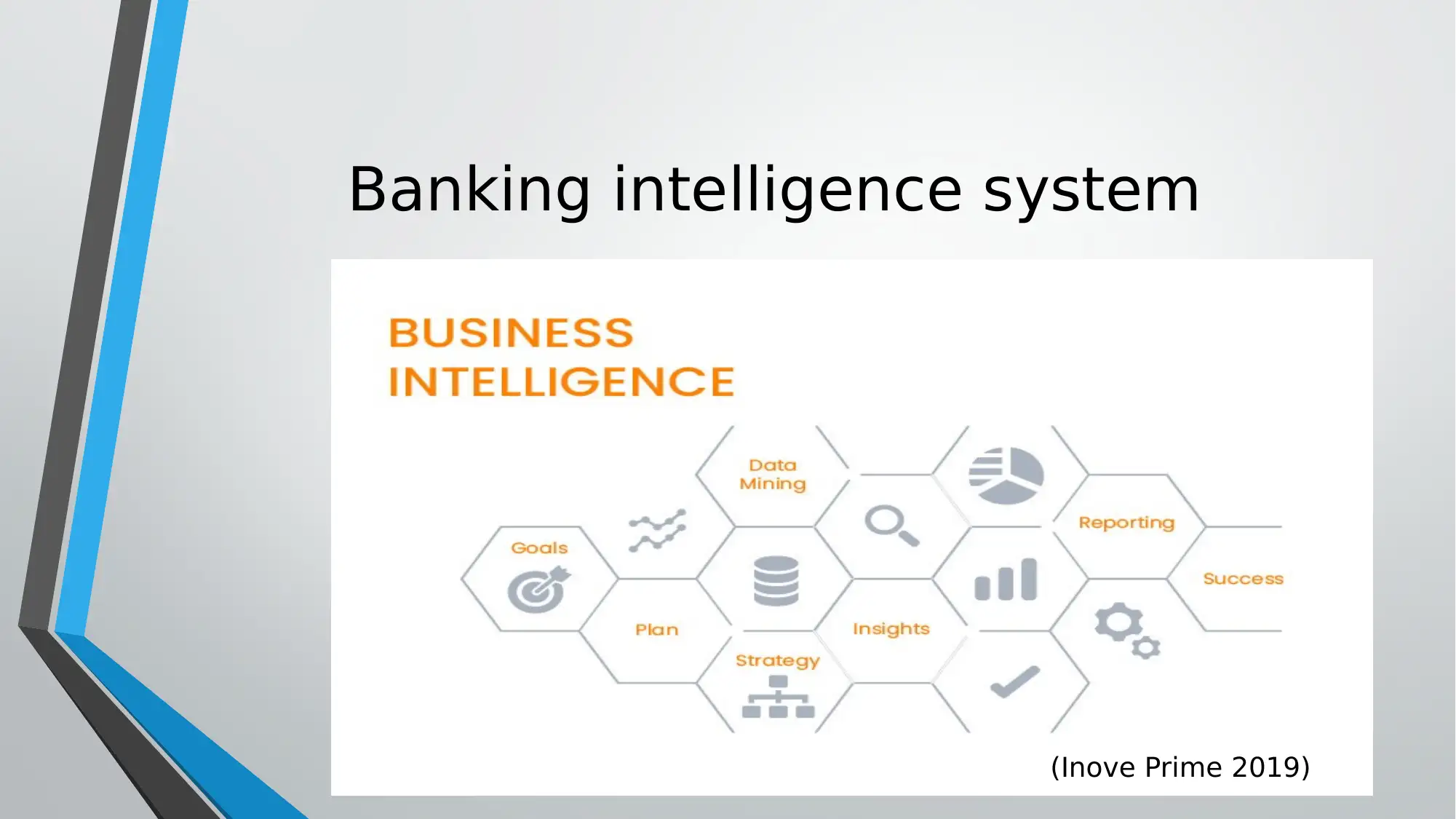
11
Banking intelligence system
(Inove Prime 2019)
Banking intelligence system
(Inove Prime 2019)
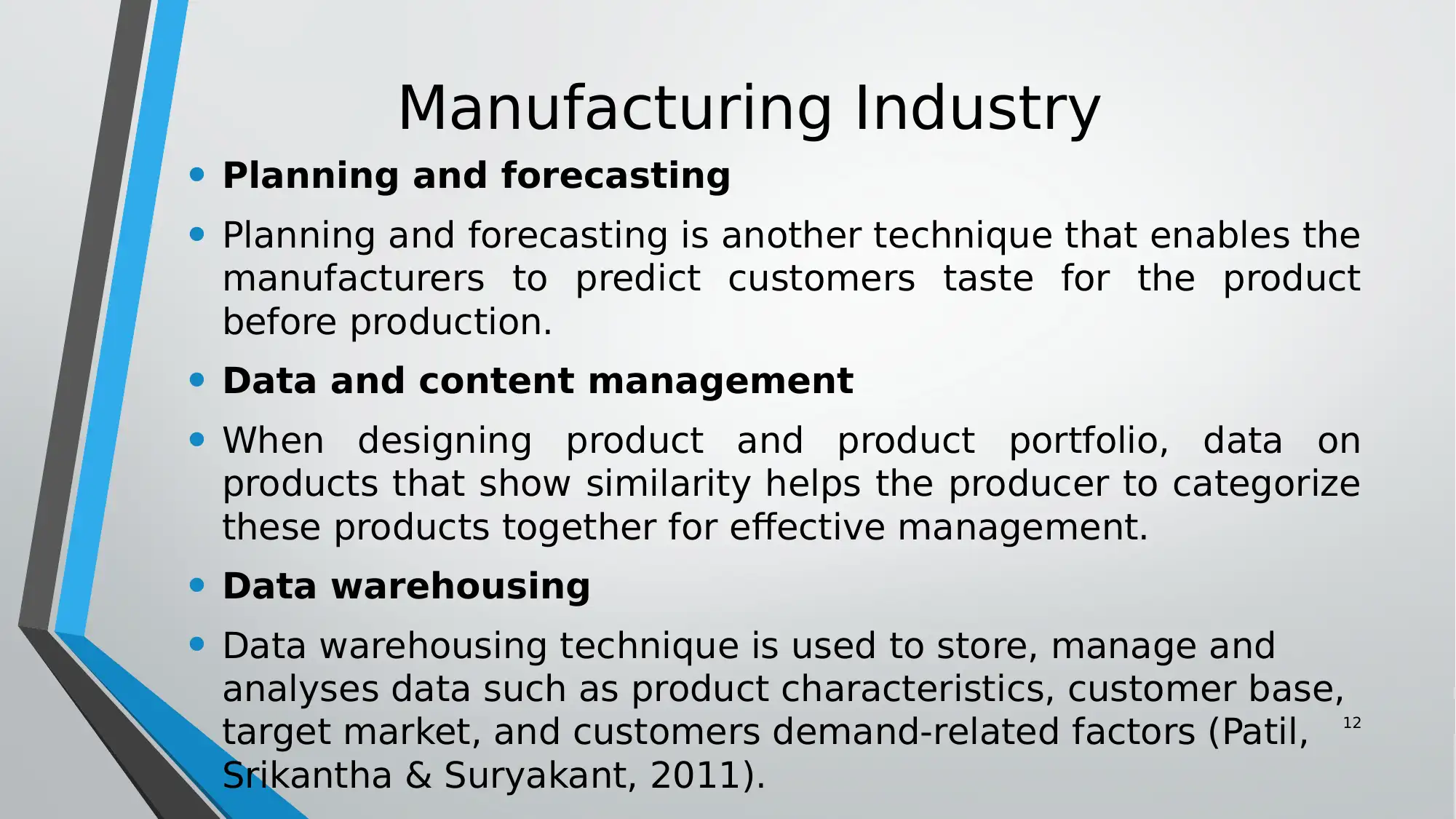
12
Manufacturing Industry
• Planning and forecasting
• Planning and forecasting is another technique that enables the
manufacturers to predict customers taste for the product
before production.
• Data and content management
• When designing product and product portfolio, data on
products that show similarity helps the producer to categorize
these products together for effective management.
• Data warehousing
• Data warehousing technique is used to store, manage and
analyses data such as product characteristics, customer base,
target market, and customers demand-related factors (Patil,
Srikantha & Suryakant, 2011).
Manufacturing Industry
• Planning and forecasting
• Planning and forecasting is another technique that enables the
manufacturers to predict customers taste for the product
before production.
• Data and content management
• When designing product and product portfolio, data on
products that show similarity helps the producer to categorize
these products together for effective management.
• Data warehousing
• Data warehousing technique is used to store, manage and
analyses data such as product characteristics, customer base,
target market, and customers demand-related factors (Patil,
Srikantha & Suryakant, 2011).
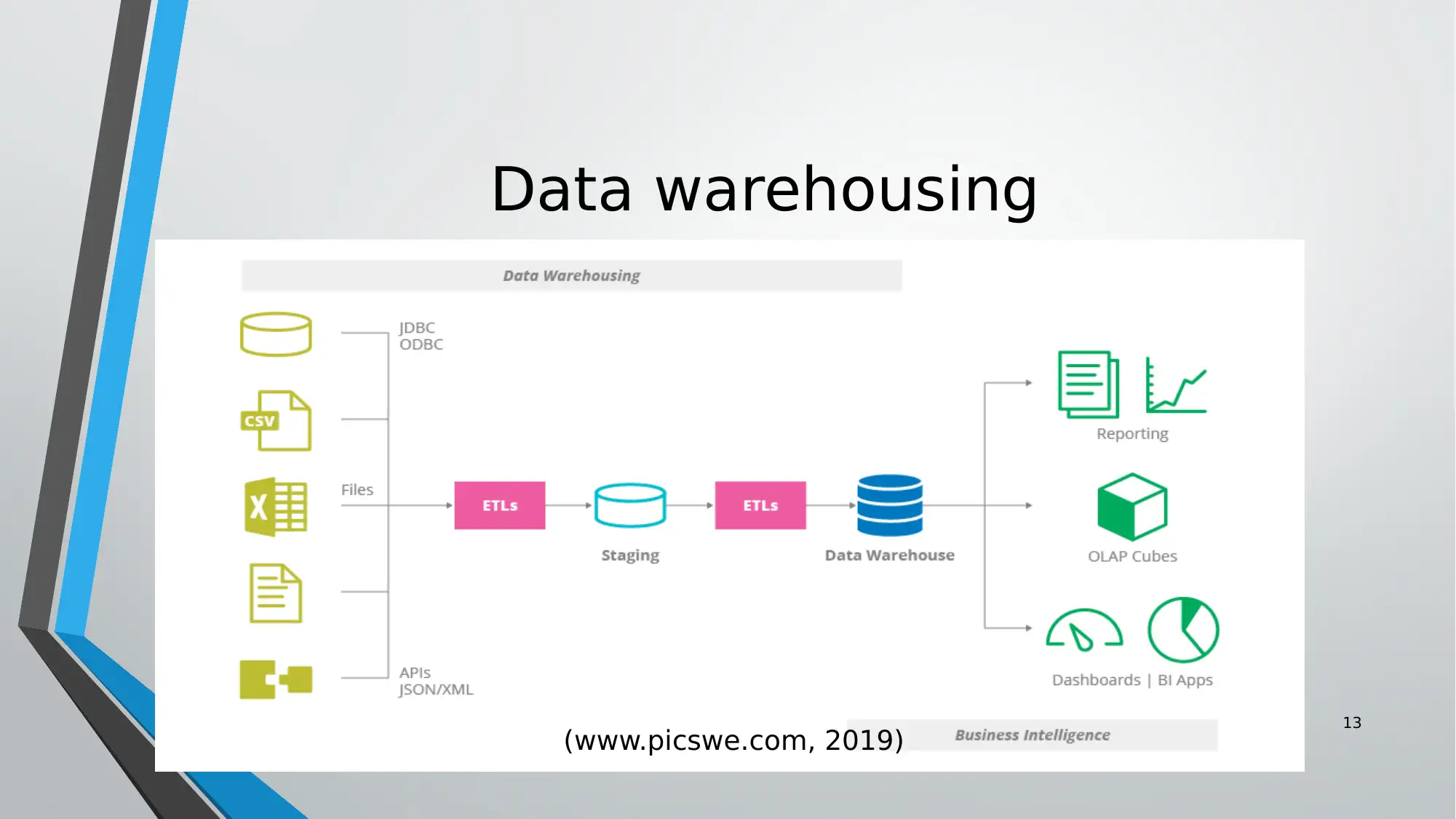
13
Data warehousing
(www.picswe.com, 2019)
Data warehousing
(www.picswe.com, 2019)
Paraphrase This Document
Need a fresh take? Get an instant paraphrase of this document with our AI Paraphraser
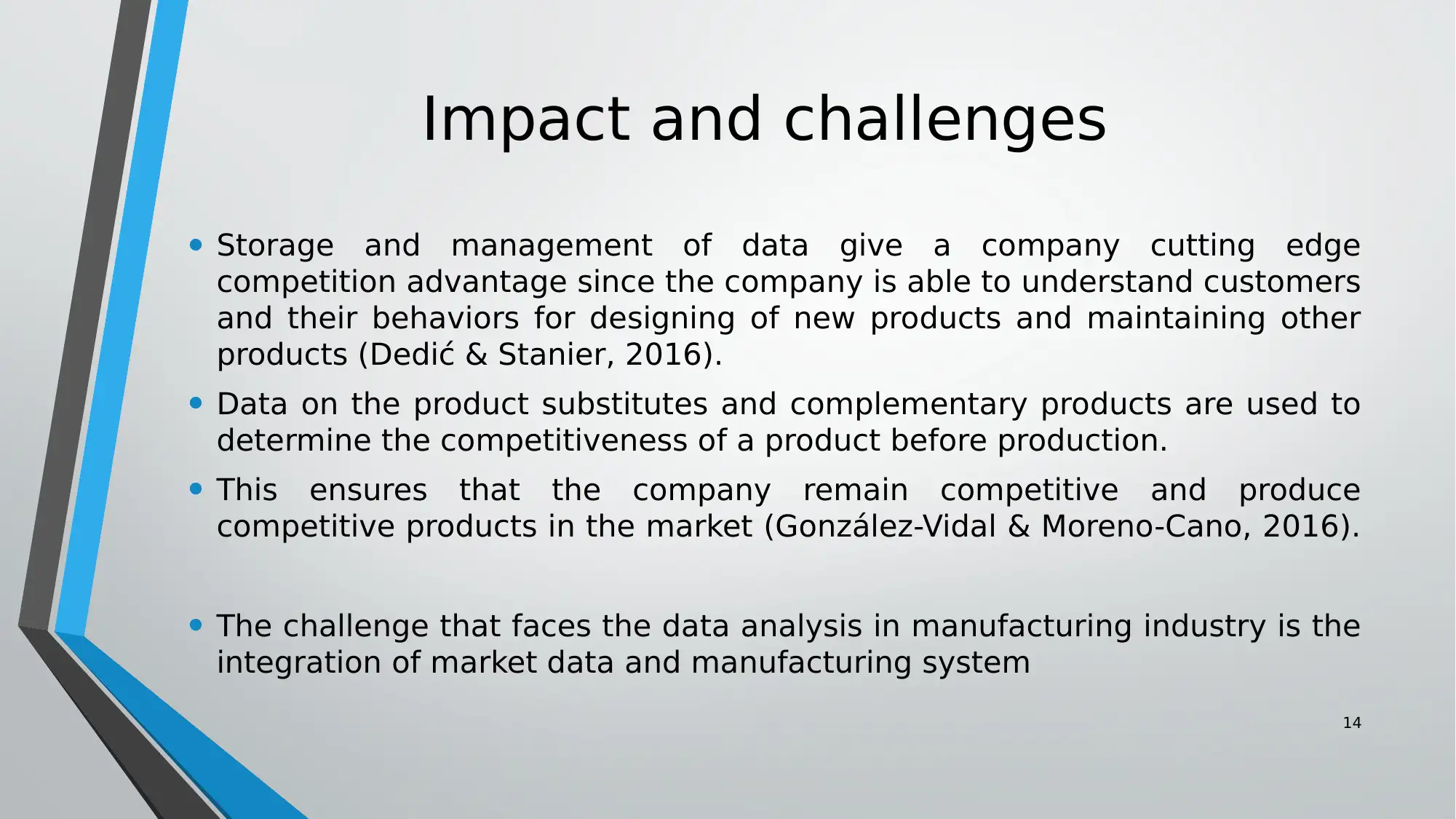
14
Impact and challenges
• Storage and management of data give a company cutting edge
competition advantage since the company is able to understand customers
and their behaviors for designing of new products and maintaining other
products (Dedić & Stanier, 2016).
• Data on the product substitutes and complementary products are used to
determine the competitiveness of a product before production.
• This ensures that the company remain competitive and produce
competitive products in the market (González-Vidal & Moreno-Cano, 2016).
• The challenge that faces the data analysis in manufacturing industry is the
integration of market data and manufacturing system
Impact and challenges
• Storage and management of data give a company cutting edge
competition advantage since the company is able to understand customers
and their behaviors for designing of new products and maintaining other
products (Dedić & Stanier, 2016).
• Data on the product substitutes and complementary products are used to
determine the competitiveness of a product before production.
• This ensures that the company remain competitive and produce
competitive products in the market (González-Vidal & Moreno-Cano, 2016).
• The challenge that faces the data analysis in manufacturing industry is the
integration of market data and manufacturing system
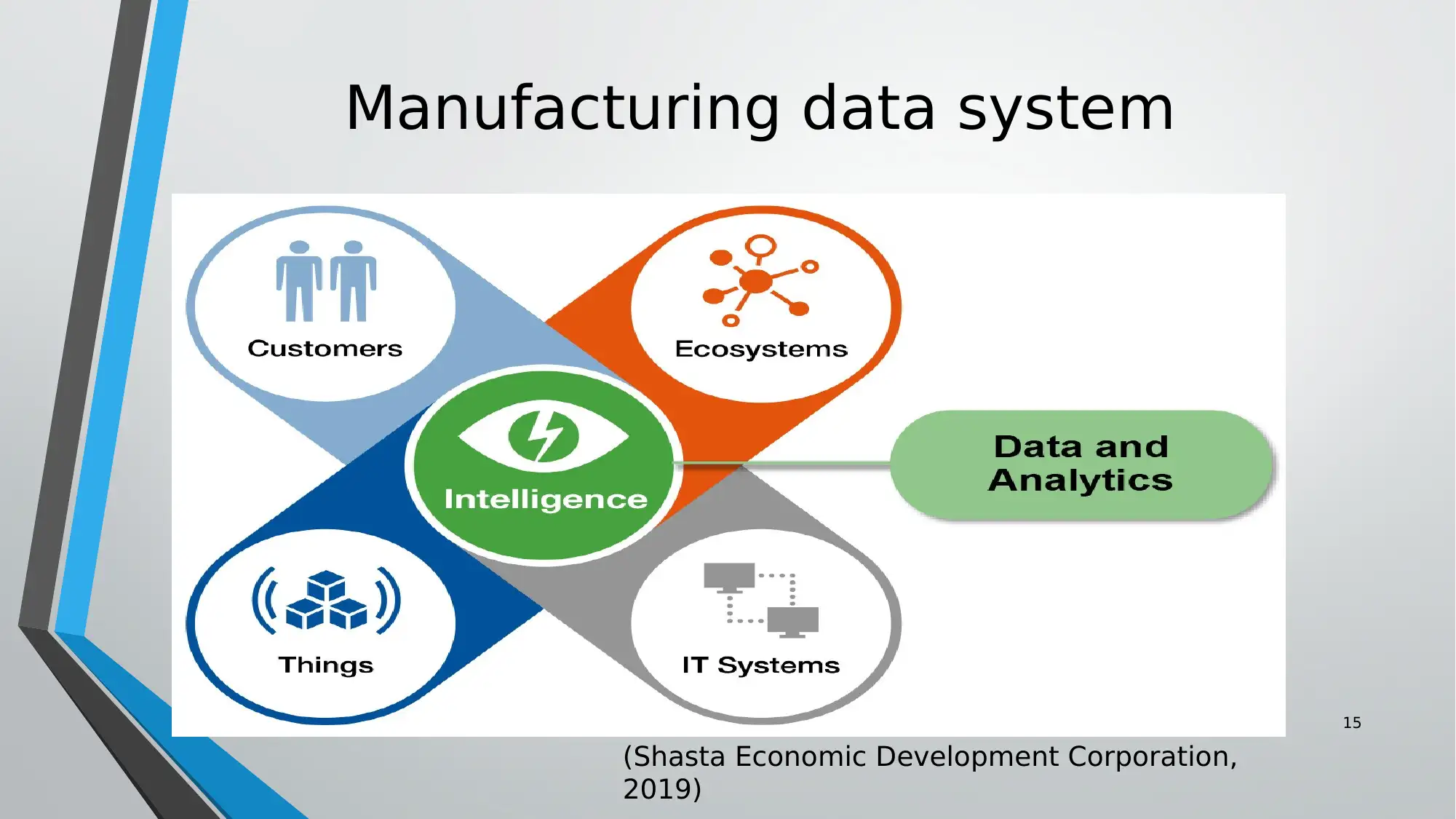
15
Manufacturing data system
(Shasta Economic Development Corporation,
2019)
Manufacturing data system
(Shasta Economic Development Corporation,
2019)
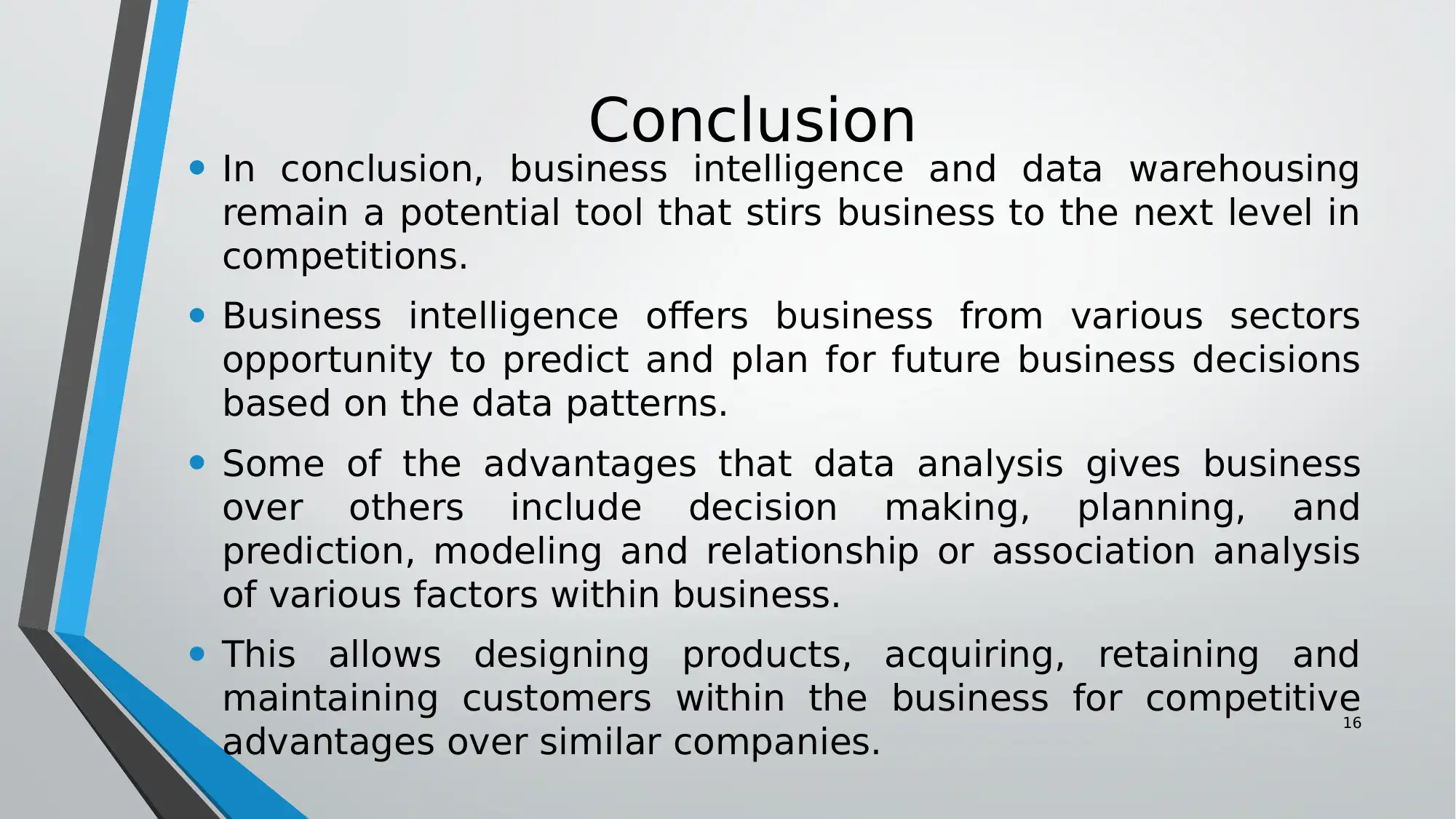
16
Conclusion
• In conclusion, business intelligence and data warehousing
remain a potential tool that stirs business to the next level in
competitions.
• Business intelligence offers business from various sectors
opportunity to predict and plan for future business decisions
based on the data patterns.
• Some of the advantages that data analysis gives business
over others include decision making, planning, and
prediction, modeling and relationship or association analysis
of various factors within business.
• This allows designing products, acquiring, retaining and
maintaining customers within the business for competitive
advantages over similar companies.
Conclusion
• In conclusion, business intelligence and data warehousing
remain a potential tool that stirs business to the next level in
competitions.
• Business intelligence offers business from various sectors
opportunity to predict and plan for future business decisions
based on the data patterns.
• Some of the advantages that data analysis gives business
over others include decision making, planning, and
prediction, modeling and relationship or association analysis
of various factors within business.
• This allows designing products, acquiring, retaining and
maintaining customers within the business for competitive
advantages over similar companies.
Secure Best Marks with AI Grader
Need help grading? Try our AI Grader for instant feedback on your assignments.
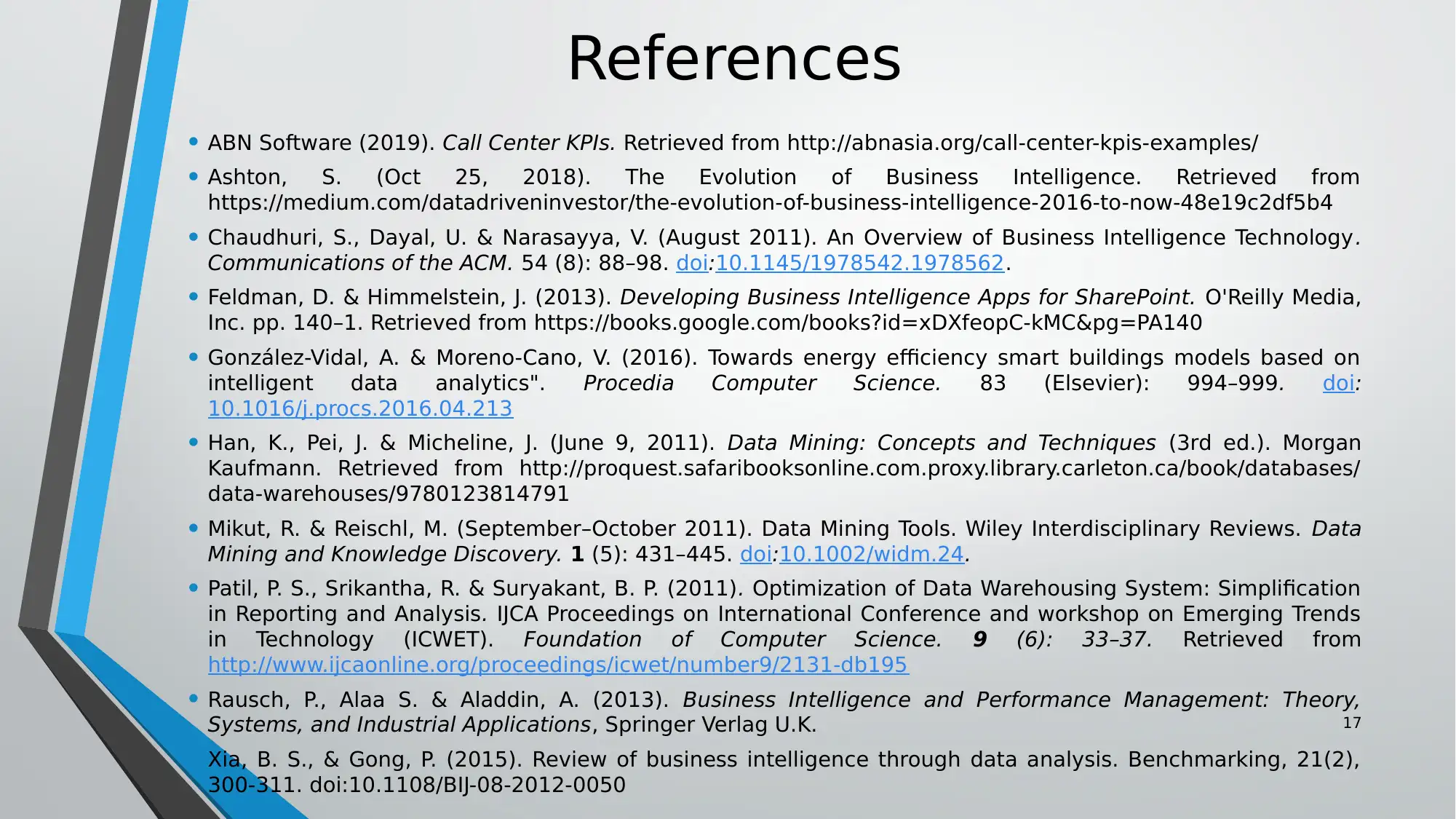
17
References
• ABN Software (2019). Call Center KPIs. Retrieved from http://abnasia.org/call-center-kpis-examples/
• Ashton, S. (Oct 25, 2018). The Evolution of Business Intelligence. Retrieved from
https://medium.com/datadriveninvestor/the-evolution-of-business-intelligence-2016-to-now-48e19c2df5b4
• Chaudhuri, S., Dayal, U. & Narasayya, V. (August 2011). An Overview of Business Intelligence Technology.
Communications of the ACM. 54 (8): 88–98. doi:10.1145/1978542.1978562.
• Feldman, D. & Himmelstein, J. (2013). Developing Business Intelligence Apps for SharePoint. O'Reilly Media,
Inc. pp. 140–1. Retrieved from https://books.google.com/books?id=xDXfeopC-kMC&pg=PA140
• González-Vidal, A. & Moreno-Cano, V. (2016). Towards energy efficiency smart buildings models based on
intelligent data analytics". Procedia Computer Science. 83 (Elsevier): 994–999. doi:
10.1016/j.procs.2016.04.213
• Han, K., Pei, J. & Micheline, J. (June 9, 2011). Data Mining: Concepts and Techniques (3rd ed.). Morgan
Kaufmann. Retrieved from http://proquest.safaribooksonline.com.proxy.library.carleton.ca/book/databases/
data-warehouses/9780123814791
• Mikut, R. & Reischl, M. (September–October 2011). Data Mining Tools. Wiley Interdisciplinary Reviews. Data
Mining and Knowledge Discovery. 1 (5): 431–445. doi:10.1002/widm.24.
• Patil, P. S., Srikantha, R. & Suryakant, B. P. (2011). Optimization of Data Warehousing System: Simplification
in Reporting and Analysis. IJCA Proceedings on International Conference and workshop on Emerging Trends
in Technology (ICWET). Foundation of Computer Science. 9 (6): 33–37. Retrieved from
http://www.ijcaonline.org/proceedings/icwet/number9/2131-db195
• Rausch, P., Alaa S. & Aladdin, A. (2013). Business Intelligence and Performance Management: Theory,
Systems, and Industrial Applications, Springer Verlag U.K.
• Xia, B. S., & Gong, P. (2015). Review of business intelligence through data analysis. Benchmarking, 21(2),
300-311. doi:10.1108/BIJ-08-2012-0050
References
• ABN Software (2019). Call Center KPIs. Retrieved from http://abnasia.org/call-center-kpis-examples/
• Ashton, S. (Oct 25, 2018). The Evolution of Business Intelligence. Retrieved from
https://medium.com/datadriveninvestor/the-evolution-of-business-intelligence-2016-to-now-48e19c2df5b4
• Chaudhuri, S., Dayal, U. & Narasayya, V. (August 2011). An Overview of Business Intelligence Technology.
Communications of the ACM. 54 (8): 88–98. doi:10.1145/1978542.1978562.
• Feldman, D. & Himmelstein, J. (2013). Developing Business Intelligence Apps for SharePoint. O'Reilly Media,
Inc. pp. 140–1. Retrieved from https://books.google.com/books?id=xDXfeopC-kMC&pg=PA140
• González-Vidal, A. & Moreno-Cano, V. (2016). Towards energy efficiency smart buildings models based on
intelligent data analytics". Procedia Computer Science. 83 (Elsevier): 994–999. doi:
10.1016/j.procs.2016.04.213
• Han, K., Pei, J. & Micheline, J. (June 9, 2011). Data Mining: Concepts and Techniques (3rd ed.). Morgan
Kaufmann. Retrieved from http://proquest.safaribooksonline.com.proxy.library.carleton.ca/book/databases/
data-warehouses/9780123814791
• Mikut, R. & Reischl, M. (September–October 2011). Data Mining Tools. Wiley Interdisciplinary Reviews. Data
Mining and Knowledge Discovery. 1 (5): 431–445. doi:10.1002/widm.24.
• Patil, P. S., Srikantha, R. & Suryakant, B. P. (2011). Optimization of Data Warehousing System: Simplification
in Reporting and Analysis. IJCA Proceedings on International Conference and workshop on Emerging Trends
in Technology (ICWET). Foundation of Computer Science. 9 (6): 33–37. Retrieved from
http://www.ijcaonline.org/proceedings/icwet/number9/2131-db195
• Rausch, P., Alaa S. & Aladdin, A. (2013). Business Intelligence and Performance Management: Theory,
Systems, and Industrial Applications, Springer Verlag U.K.
• Xia, B. S., & Gong, P. (2015). Review of business intelligence through data analysis. Benchmarking, 21(2),
300-311. doi:10.1108/BIJ-08-2012-0050
1 out of 17
Related Documents
![[object Object]](/_next/image/?url=%2F_next%2Fstatic%2Fmedia%2Flogo.6d15ce61.png&w=640&q=75)
Your All-in-One AI-Powered Toolkit for Academic Success.
+13062052269
info@desklib.com
Available 24*7 on WhatsApp / Email
Unlock your academic potential
© 2024 | Zucol Services PVT LTD | All rights reserved.