Detailed Regression Model Analysis for Business Statistics Assignment
VerifiedAdded on 2021/02/19
|19
|2073
|110
Homework Assignment
AI Summary
This assignment delves into the application of regression models within the context of business statistics, specifically focusing on the Japanese electronic industry. The analysis begins with an introduction to the regression model, explaining its use in investigating relationships between variables, such as operating revenue, shareholders' funds, debtors, intangible assets, and other fixed assets. The assignment utilizes SPSS for data analysis, including the creation of scatter plots to visualize relationships between variables. It then proceeds to evaluate the significance of the initial model through ANOVA analysis, rejecting or accepting null hypotheses based on p-values. The document also includes a re-estimated model, comparing the initial and re-estimated models and interpreting the estimated coefficients. The study concludes by emphasizing the importance of business statistics in decision-making, highlighting the effective use of statistical tools and techniques in areas like finance and research.
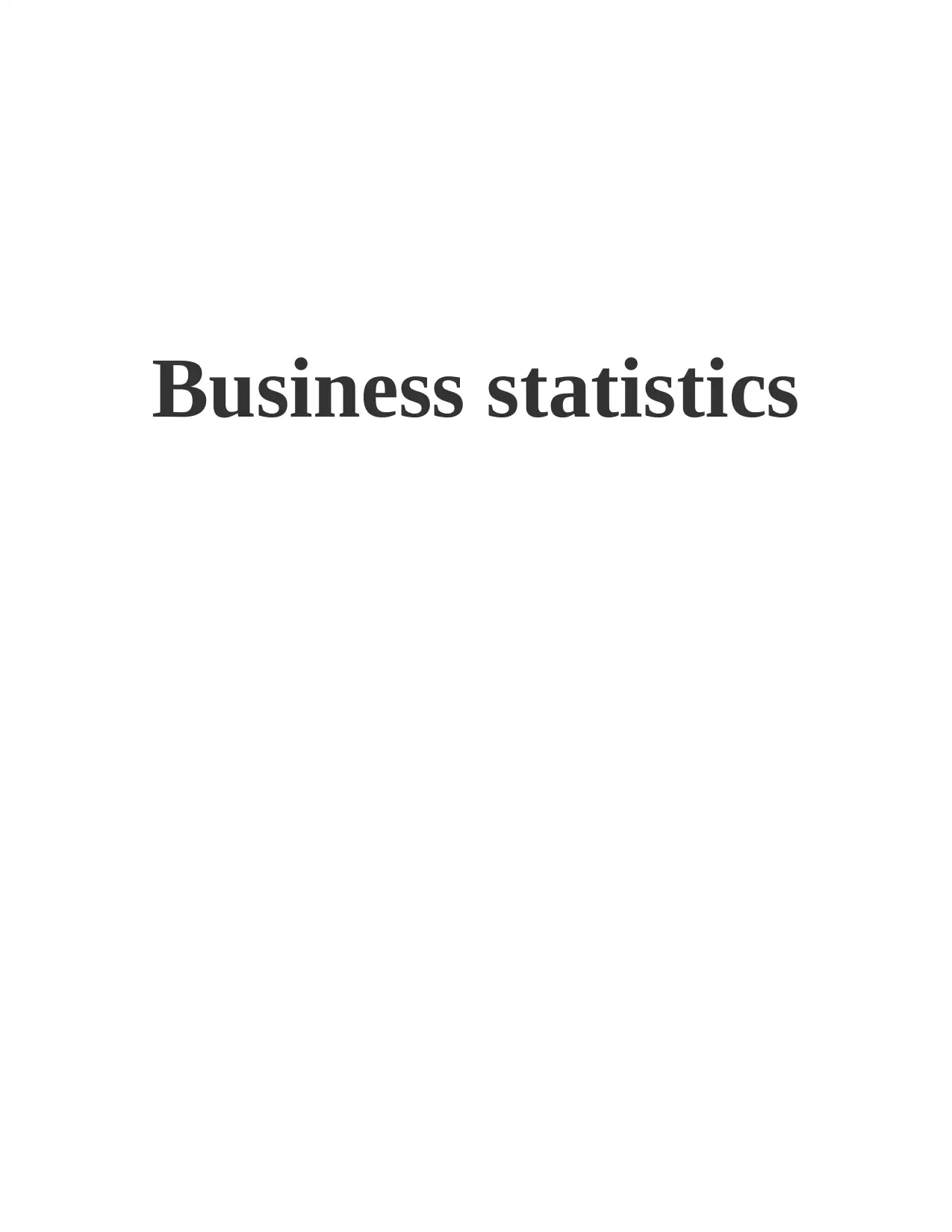
Business statistics
Paraphrase This Document
Need a fresh take? Get an instant paraphrase of this document with our AI Paraphraser
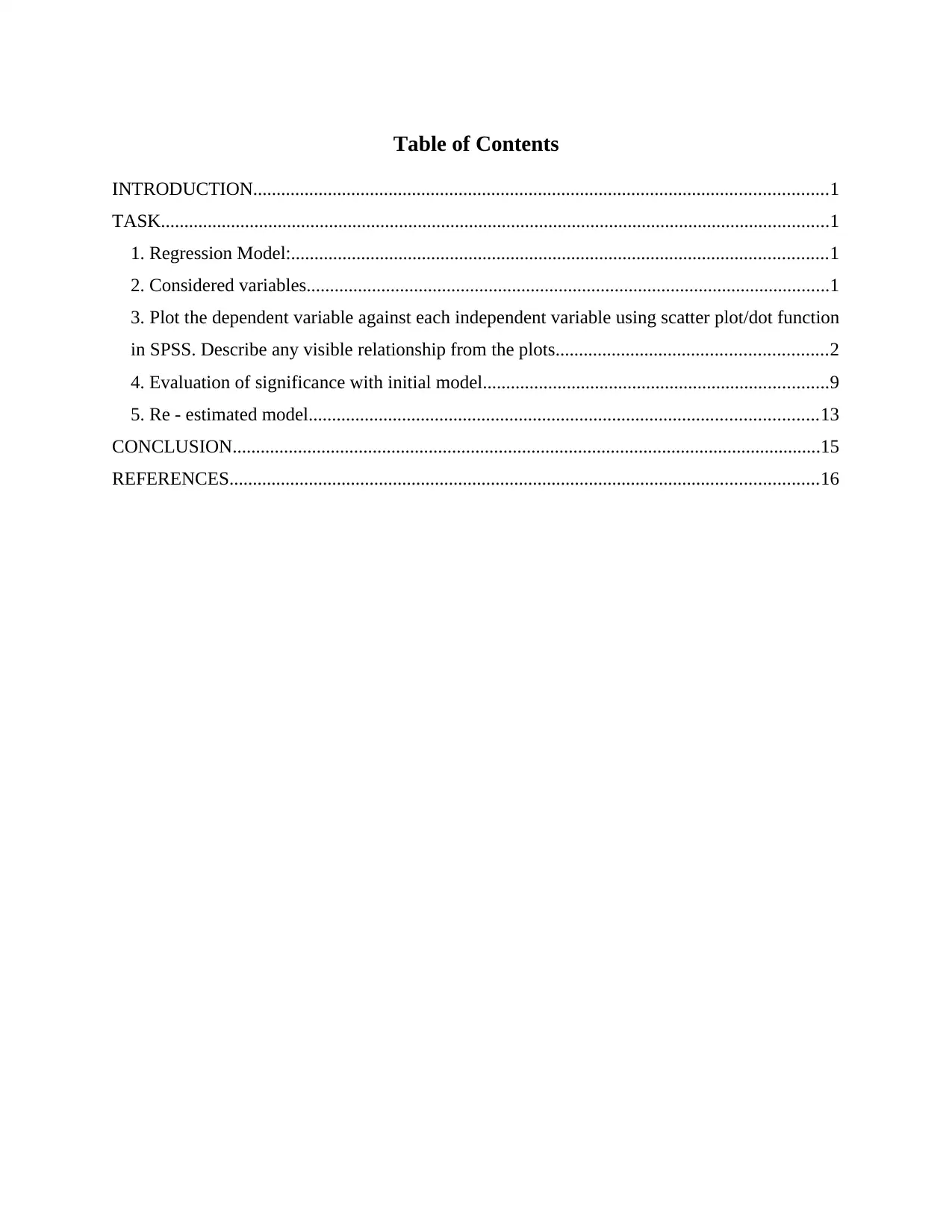
Table of Contents
INTRODUCTION...........................................................................................................................1
TASK...............................................................................................................................................1
1. Regression Model:...................................................................................................................1
2. Considered variables................................................................................................................1
3. Plot the dependent variable against each independent variable using scatter plot/dot function
in SPSS. Describe any visible relationship from the plots..........................................................2
4. Evaluation of significance with initial model..........................................................................9
5. Re - estimated model.............................................................................................................13
CONCLUSION..............................................................................................................................15
REFERENCES..............................................................................................................................16
INTRODUCTION...........................................................................................................................1
TASK...............................................................................................................................................1
1. Regression Model:...................................................................................................................1
2. Considered variables................................................................................................................1
3. Plot the dependent variable against each independent variable using scatter plot/dot function
in SPSS. Describe any visible relationship from the plots..........................................................2
4. Evaluation of significance with initial model..........................................................................9
5. Re - estimated model.............................................................................................................13
CONCLUSION..............................................................................................................................15
REFERENCES..............................................................................................................................16
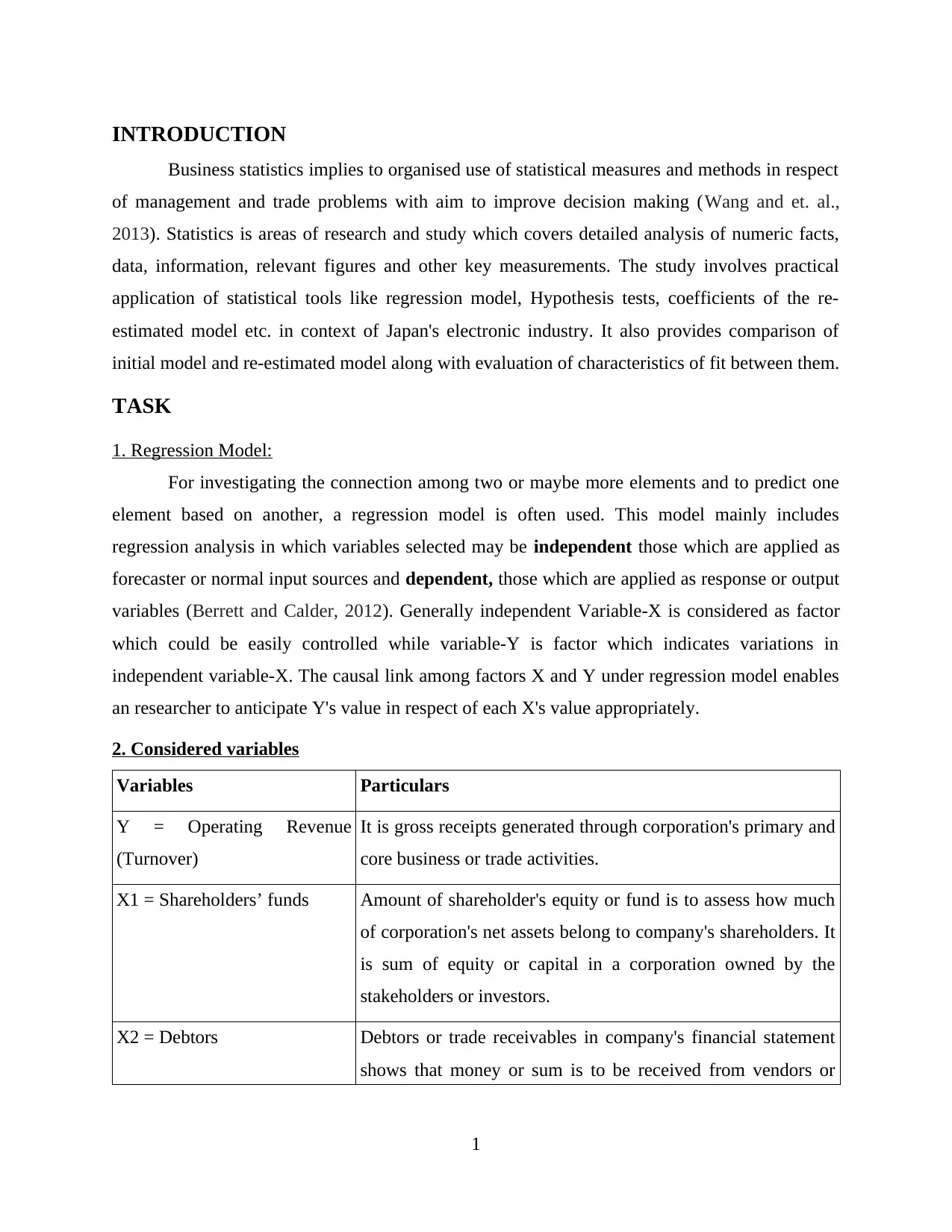
INTRODUCTION
Business statistics implies to organised use of statistical measures and methods in respect
of management and trade problems with aim to improve decision making (Wang and et. al.,
2013). Statistics is areas of research and study which covers detailed analysis of numeric facts,
data, information, relevant figures and other key measurements. The study involves practical
application of statistical tools like regression model, Hypothesis tests, coefficients of the re-
estimated model etc. in context of Japan's electronic industry. It also provides comparison of
initial model and re-estimated model along with evaluation of characteristics of fit between them.
TASK
1. Regression Model:
For investigating the connection among two or maybe more elements and to predict one
element based on another, a regression model is often used. This model mainly includes
regression analysis in which variables selected may be independent those which are applied as
forecaster or normal input sources and dependent, those which are applied as response or output
variables (Berrett and Calder, 2012). Generally independent Variable-X is considered as factor
which could be easily controlled while variable-Y is factor which indicates variations in
independent variable-X. The causal link among factors X and Y under regression model enables
an researcher to anticipate Y's value in respect of each X's value appropriately.
2. Considered variables
Variables Particulars
Y = Operating Revenue
(Turnover)
It is gross receipts generated through corporation's primary and
core business or trade activities.
X1 = Shareholders’ funds Amount of shareholder's equity or fund is to assess how much
of corporation's net assets belong to company's shareholders. It
is sum of equity or capital in a corporation owned by the
stakeholders or investors.
X2 = Debtors Debtors or trade receivables in company's financial statement
shows that money or sum is to be received from vendors or
1
Business statistics implies to organised use of statistical measures and methods in respect
of management and trade problems with aim to improve decision making (Wang and et. al.,
2013). Statistics is areas of research and study which covers detailed analysis of numeric facts,
data, information, relevant figures and other key measurements. The study involves practical
application of statistical tools like regression model, Hypothesis tests, coefficients of the re-
estimated model etc. in context of Japan's electronic industry. It also provides comparison of
initial model and re-estimated model along with evaluation of characteristics of fit between them.
TASK
1. Regression Model:
For investigating the connection among two or maybe more elements and to predict one
element based on another, a regression model is often used. This model mainly includes
regression analysis in which variables selected may be independent those which are applied as
forecaster or normal input sources and dependent, those which are applied as response or output
variables (Berrett and Calder, 2012). Generally independent Variable-X is considered as factor
which could be easily controlled while variable-Y is factor which indicates variations in
independent variable-X. The causal link among factors X and Y under regression model enables
an researcher to anticipate Y's value in respect of each X's value appropriately.
2. Considered variables
Variables Particulars
Y = Operating Revenue
(Turnover)
It is gross receipts generated through corporation's primary and
core business or trade activities.
X1 = Shareholders’ funds Amount of shareholder's equity or fund is to assess how much
of corporation's net assets belong to company's shareholders. It
is sum of equity or capital in a corporation owned by the
stakeholders or investors.
X2 = Debtors Debtors or trade receivables in company's financial statement
shows that money or sum is to be received from vendors or
1
You're viewing a preview
Unlock full access by subscribing today!
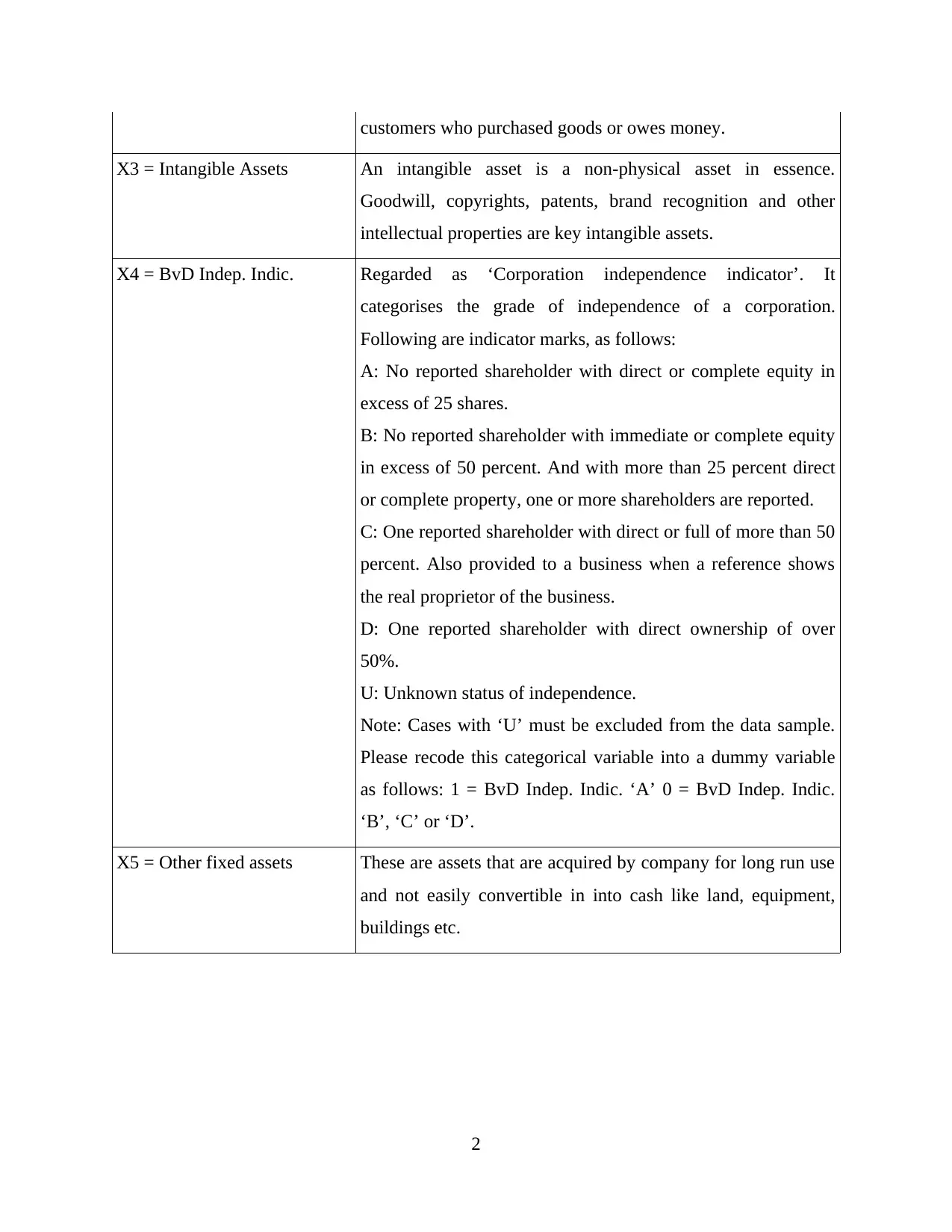
customers who purchased goods or owes money.
X3 = Intangible Assets An intangible asset is a non-physical asset in essence.
Goodwill, copyrights, patents, brand recognition and other
intellectual properties are key intangible assets.
X4 = BvD Indep. Indic. Regarded as ‘Corporation independence indicator’. It
categorises the grade of independence of a corporation.
Following are indicator marks, as follows:
A: No reported shareholder with direct or complete equity in
excess of 25 shares.
B: No reported shareholder with immediate or complete equity
in excess of 50 percent. And with more than 25 percent direct
or complete property, one or more shareholders are reported.
C: One reported shareholder with direct or full of more than 50
percent. Also provided to a business when a reference shows
the real proprietor of the business.
D: One reported shareholder with direct ownership of over
50%.
U: Unknown status of independence.
Note: Cases with ‘U’ must be excluded from the data sample.
Please recode this categorical variable into a dummy variable
as follows: 1 = BvD Indep. Indic. ‘A’ 0 = BvD Indep. Indic.
‘B’, ‘C’ or ‘D’.
X5 = Other fixed assets These are assets that are acquired by company for long run use
and not easily convertible in into cash like land, equipment,
buildings etc.
2
X3 = Intangible Assets An intangible asset is a non-physical asset in essence.
Goodwill, copyrights, patents, brand recognition and other
intellectual properties are key intangible assets.
X4 = BvD Indep. Indic. Regarded as ‘Corporation independence indicator’. It
categorises the grade of independence of a corporation.
Following are indicator marks, as follows:
A: No reported shareholder with direct or complete equity in
excess of 25 shares.
B: No reported shareholder with immediate or complete equity
in excess of 50 percent. And with more than 25 percent direct
or complete property, one or more shareholders are reported.
C: One reported shareholder with direct or full of more than 50
percent. Also provided to a business when a reference shows
the real proprietor of the business.
D: One reported shareholder with direct ownership of over
50%.
U: Unknown status of independence.
Note: Cases with ‘U’ must be excluded from the data sample.
Please recode this categorical variable into a dummy variable
as follows: 1 = BvD Indep. Indic. ‘A’ 0 = BvD Indep. Indic.
‘B’, ‘C’ or ‘D’.
X5 = Other fixed assets These are assets that are acquired by company for long run use
and not easily convertible in into cash like land, equipment,
buildings etc.
2
Paraphrase This Document
Need a fresh take? Get an instant paraphrase of this document with our AI Paraphraser
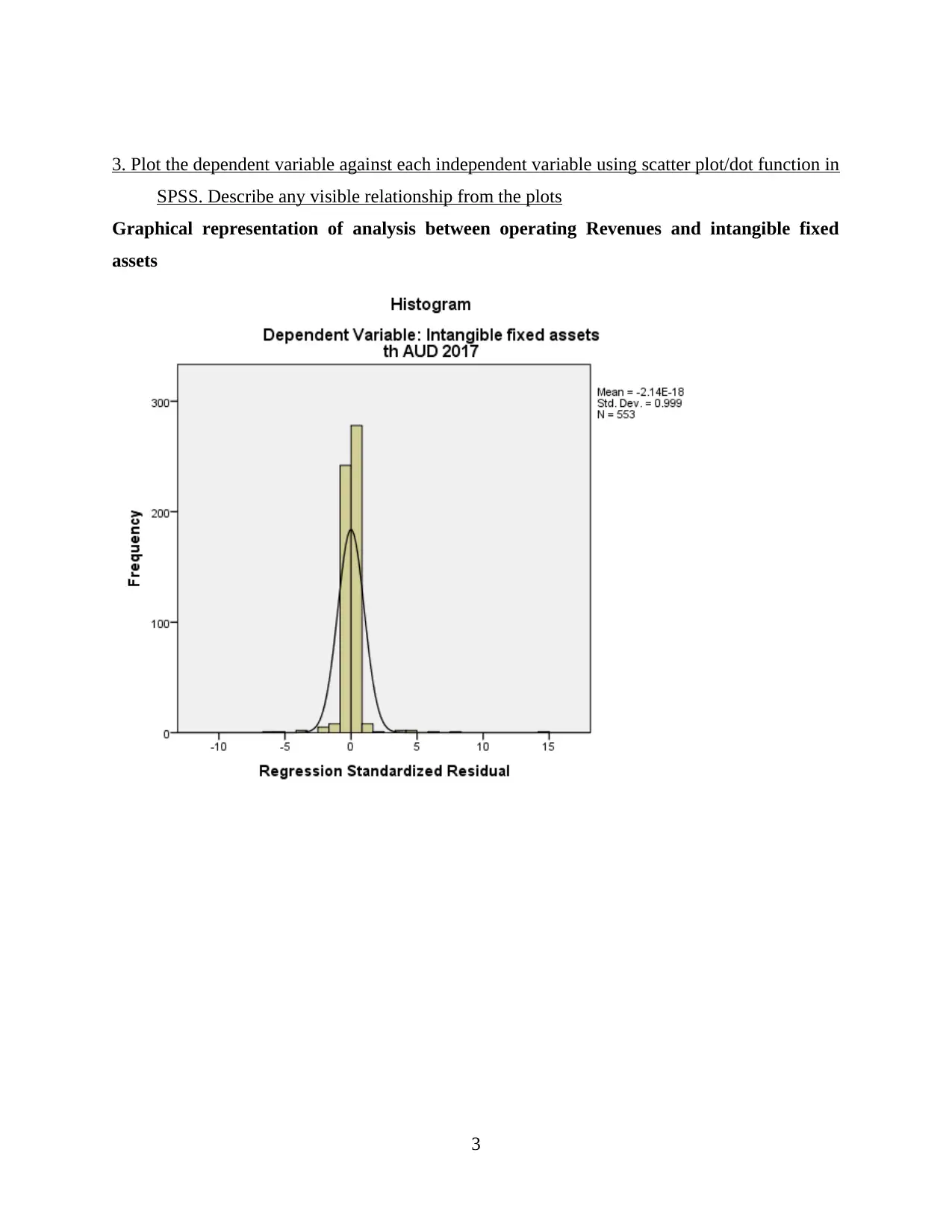
3. Plot the dependent variable against each independent variable using scatter plot/dot function in
SPSS. Describe any visible relationship from the plots
Graphical representation of analysis between operating Revenues and intangible fixed
assets
3
SPSS. Describe any visible relationship from the plots
Graphical representation of analysis between operating Revenues and intangible fixed
assets
3
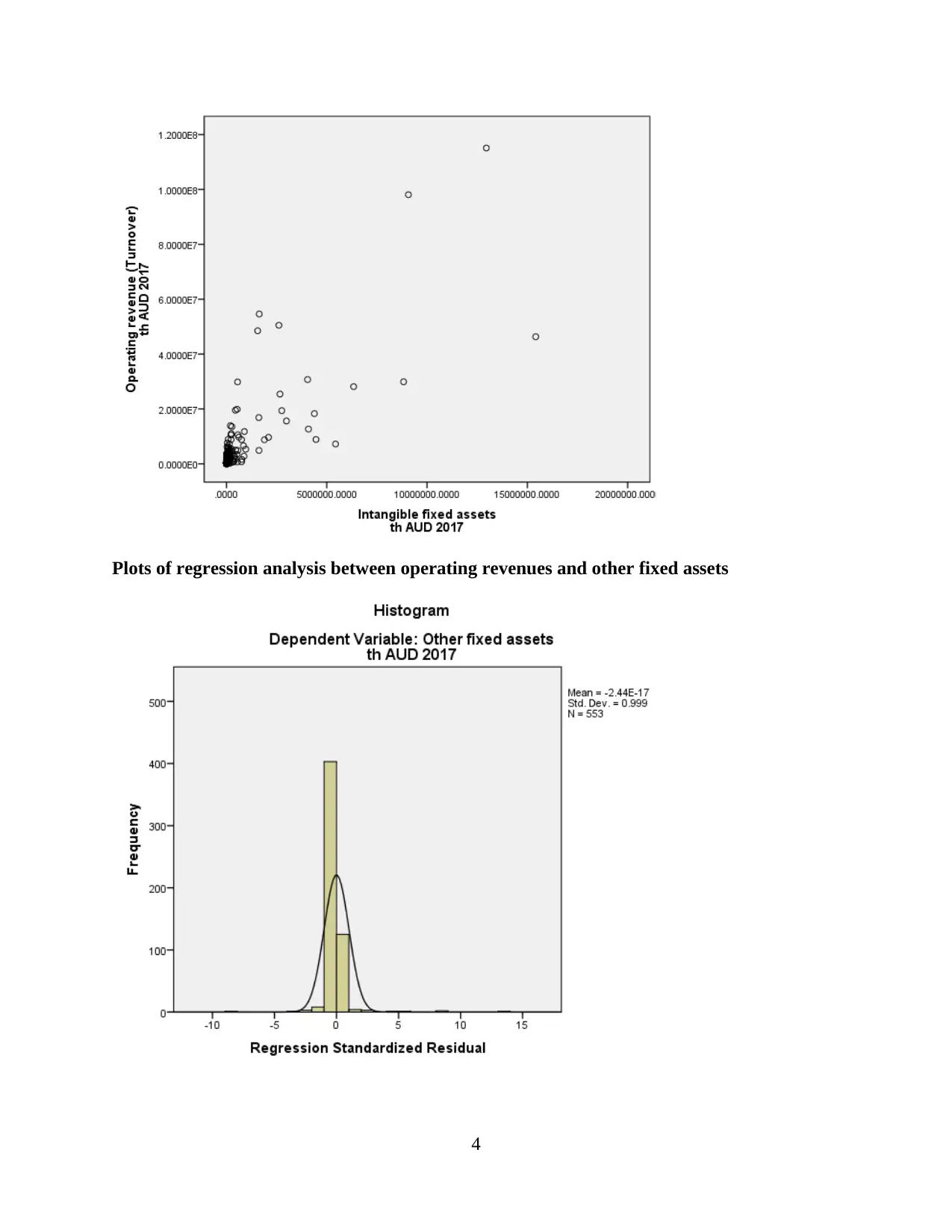
Plots of regression analysis between operating revenues and other fixed assets
4
4
You're viewing a preview
Unlock full access by subscribing today!
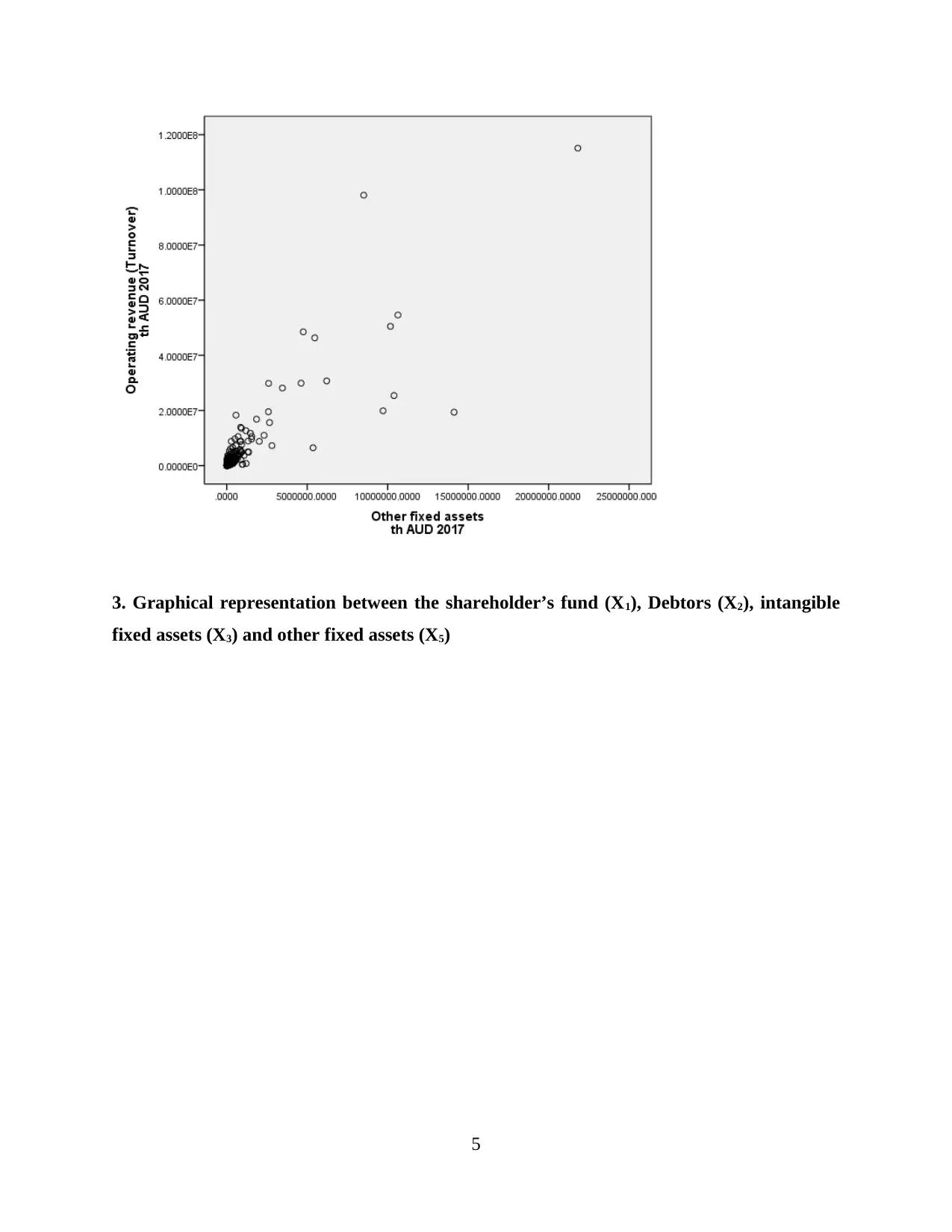
3. Graphical representation between the shareholder’s fund (X1), Debtors (X2), intangible
fixed assets (X3) and other fixed assets (X5)
5
fixed assets (X3) and other fixed assets (X5)
5
Paraphrase This Document
Need a fresh take? Get an instant paraphrase of this document with our AI Paraphraser
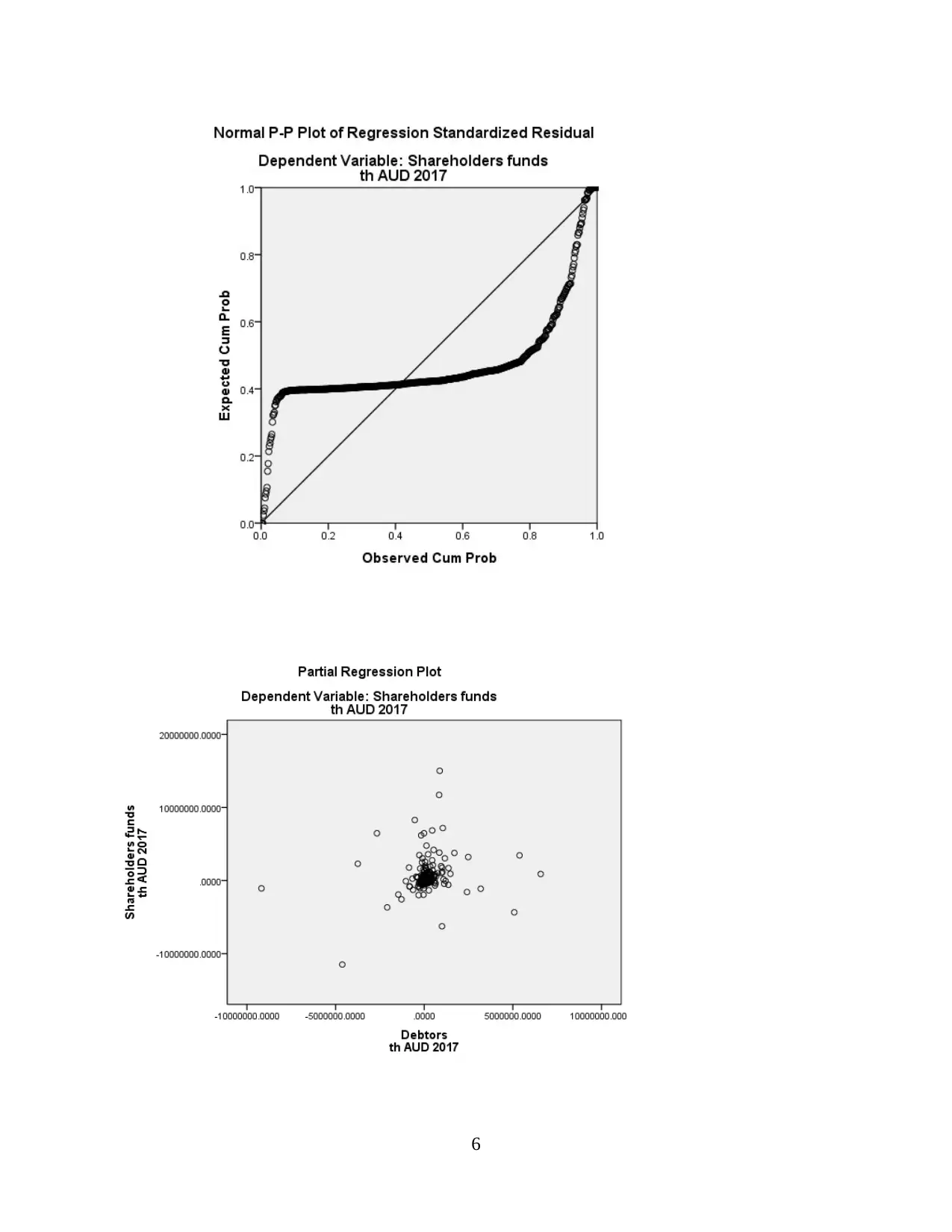
6
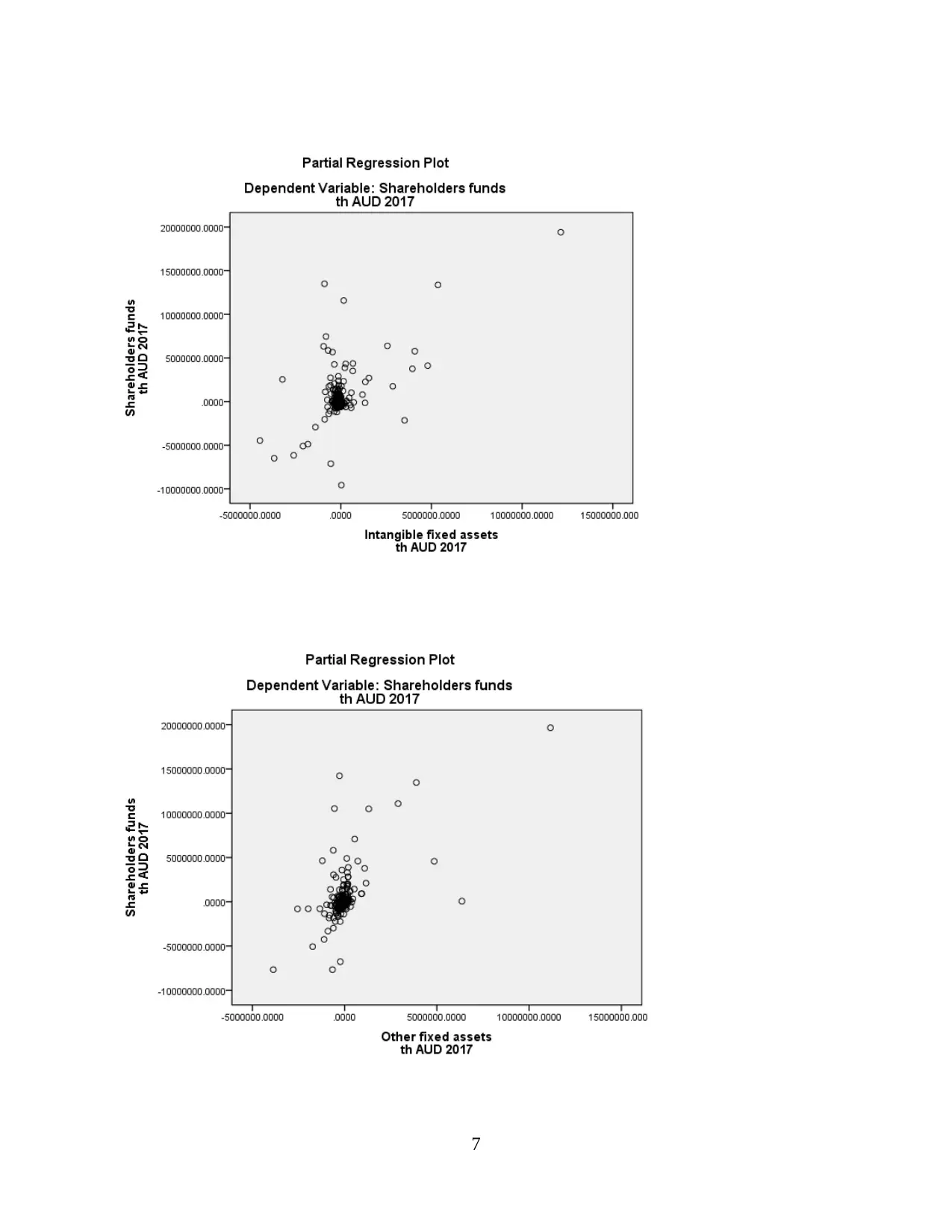
7
You're viewing a preview
Unlock full access by subscribing today!
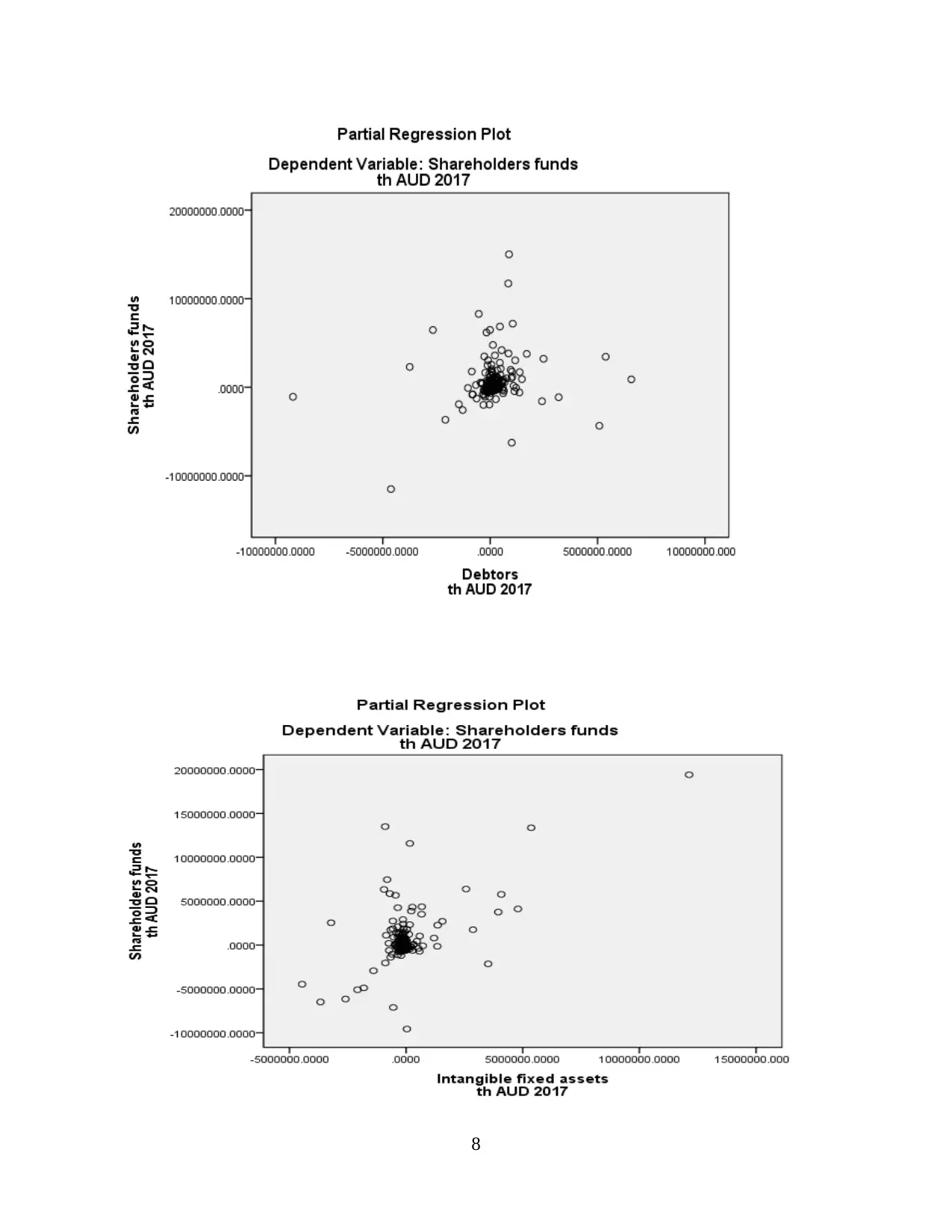
8
Paraphrase This Document
Need a fresh take? Get an instant paraphrase of this document with our AI Paraphraser
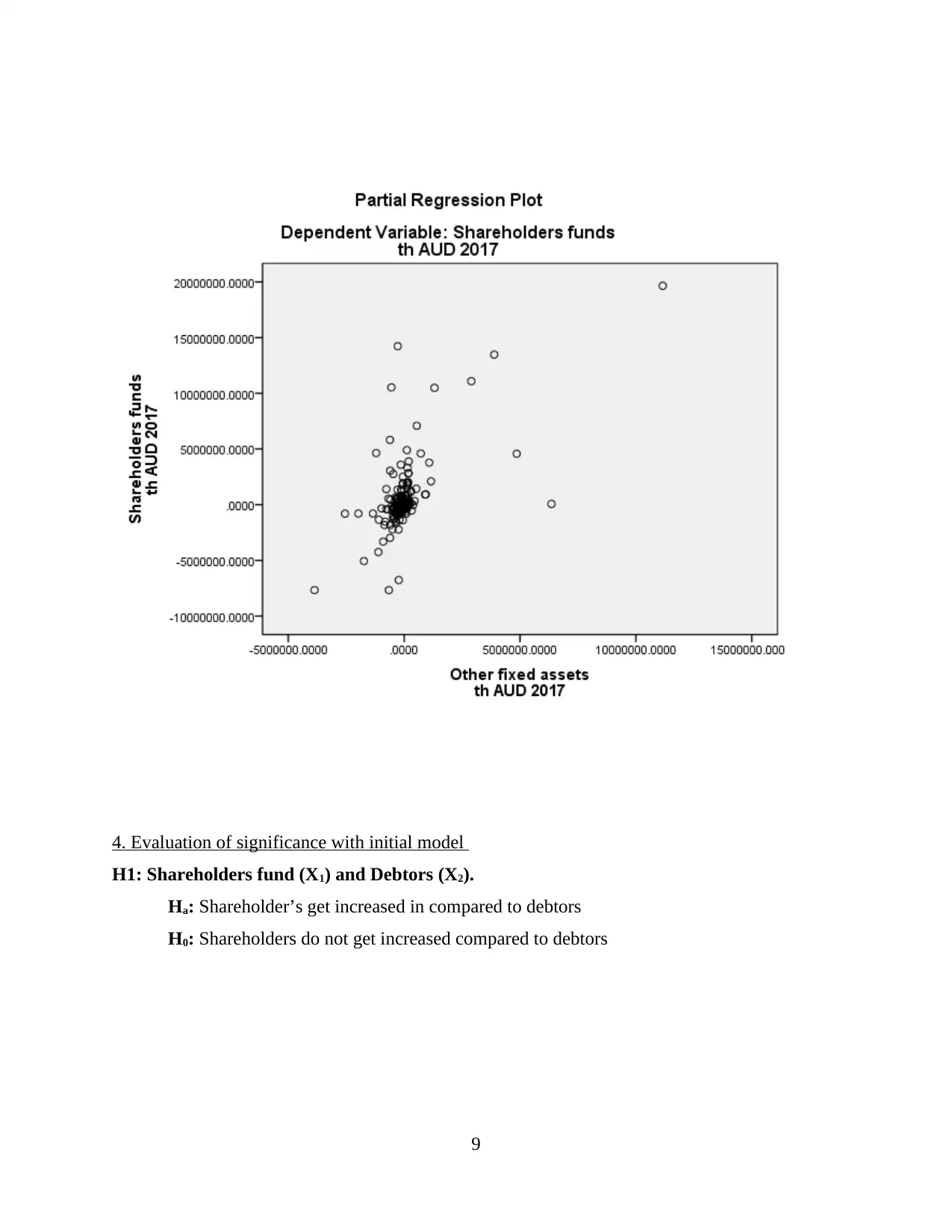
4. Evaluation of significance with initial model
H1: Shareholders fund (X1) and Debtors (X2).
Ha: Shareholder’s get increased in compared to debtors
H0: Shareholders do not get increased compared to debtors
9
H1: Shareholders fund (X1) and Debtors (X2).
Ha: Shareholder’s get increased in compared to debtors
H0: Shareholders do not get increased compared to debtors
9
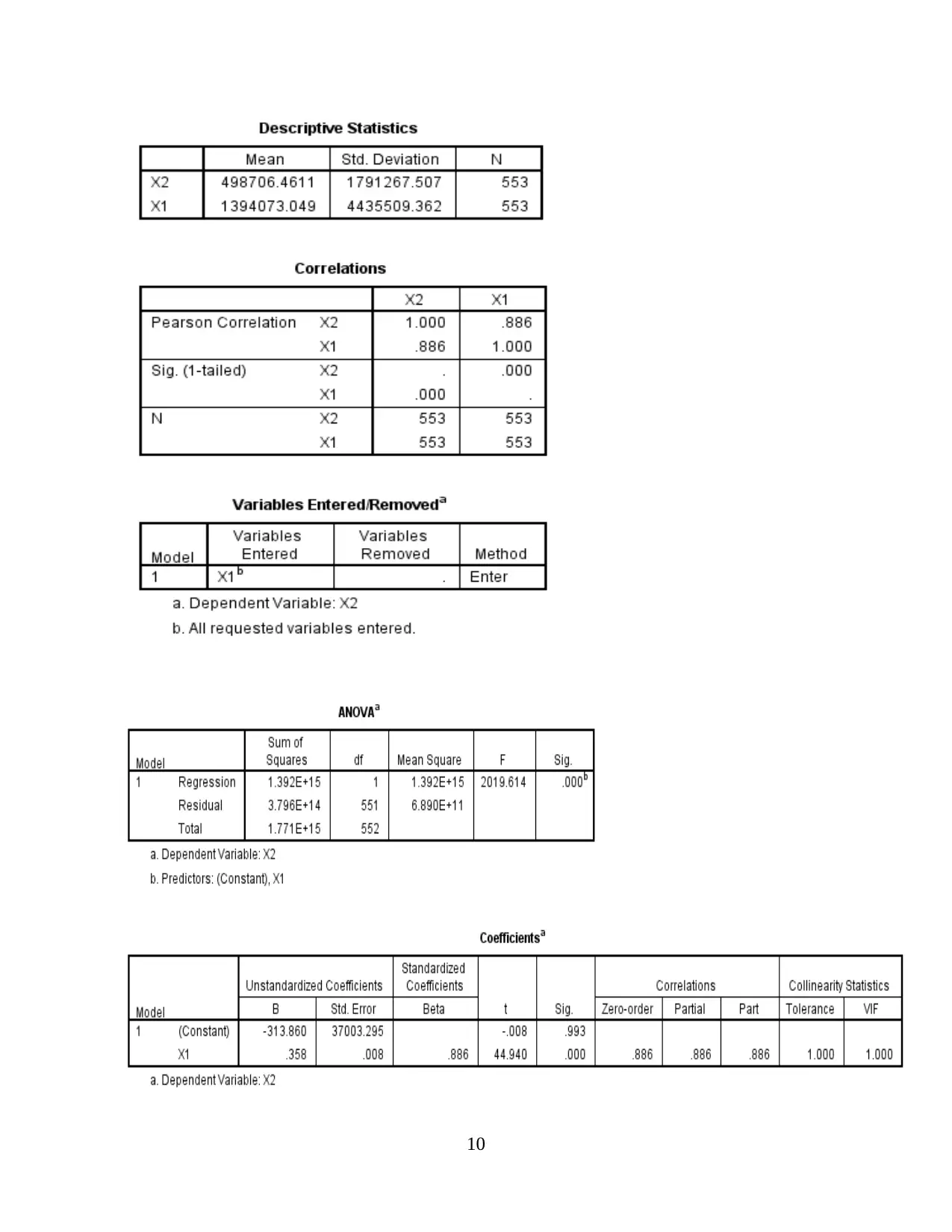
10
You're viewing a preview
Unlock full access by subscribing today!
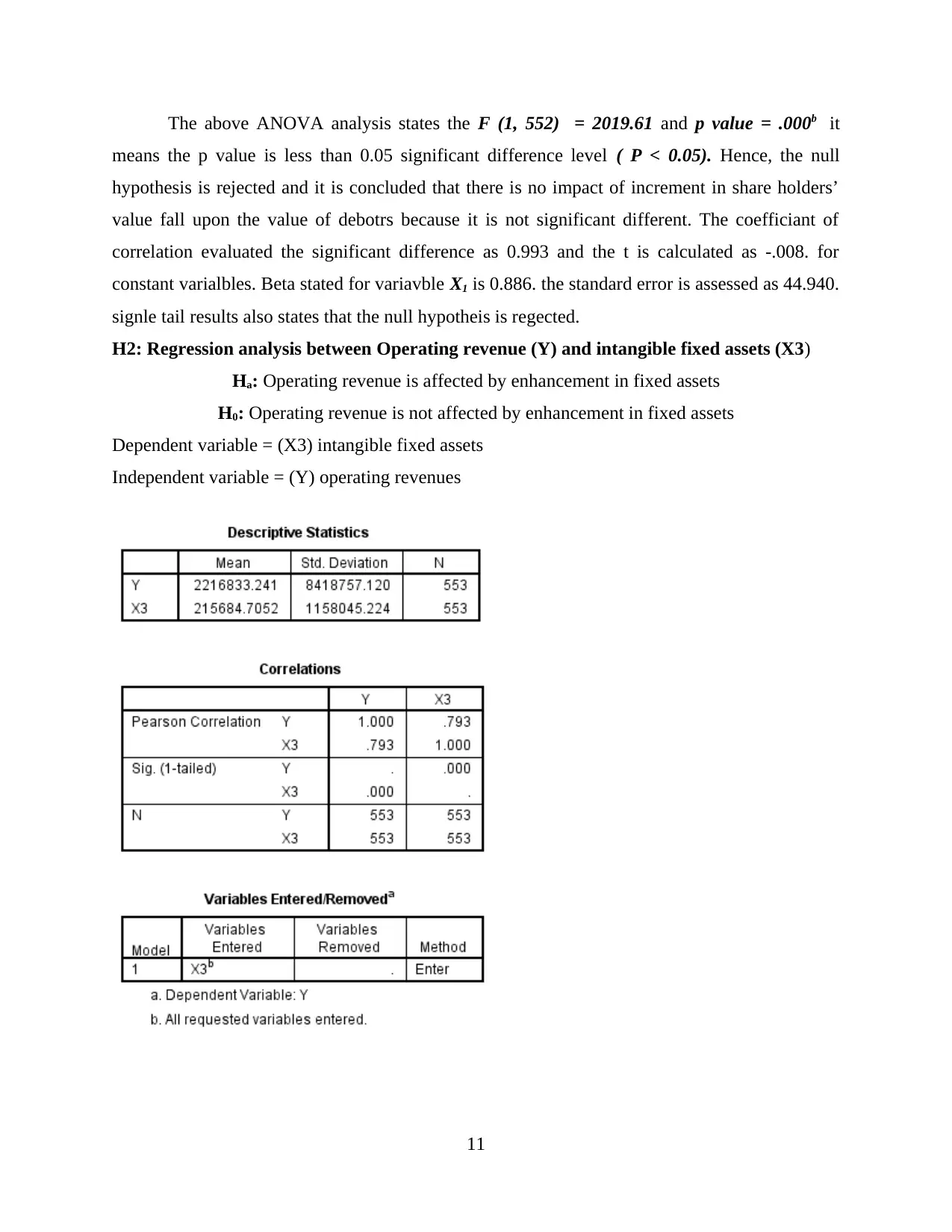
The above ANOVA analysis states the F (1, 552) = 2019.61 and p value = .000b it
means the p value is less than 0.05 significant difference level ( P < 0.05). Hence, the null
hypothesis is rejected and it is concluded that there is no impact of increment in share holders’
value fall upon the value of debotrs because it is not significant different. The coefficiant of
correlation evaluated the significant difference as 0.993 and the t is calculated as -.008. for
constant varialbles. Beta stated for variavble X1 is 0.886. the standard error is assessed as 44.940.
signle tail results also states that the null hypotheis is regected.
H2: Regression analysis between Operating revenue (Y) and intangible fixed assets (X3)
Ha: Operating revenue is affected by enhancement in fixed assets
H0: Operating revenue is not affected by enhancement in fixed assets
Dependent variable = (X3) intangible fixed assets
Independent variable = (Y) operating revenues
11
means the p value is less than 0.05 significant difference level ( P < 0.05). Hence, the null
hypothesis is rejected and it is concluded that there is no impact of increment in share holders’
value fall upon the value of debotrs because it is not significant different. The coefficiant of
correlation evaluated the significant difference as 0.993 and the t is calculated as -.008. for
constant varialbles. Beta stated for variavble X1 is 0.886. the standard error is assessed as 44.940.
signle tail results also states that the null hypotheis is regected.
H2: Regression analysis between Operating revenue (Y) and intangible fixed assets (X3)
Ha: Operating revenue is affected by enhancement in fixed assets
H0: Operating revenue is not affected by enhancement in fixed assets
Dependent variable = (X3) intangible fixed assets
Independent variable = (Y) operating revenues
11
Paraphrase This Document
Need a fresh take? Get an instant paraphrase of this document with our AI Paraphraser
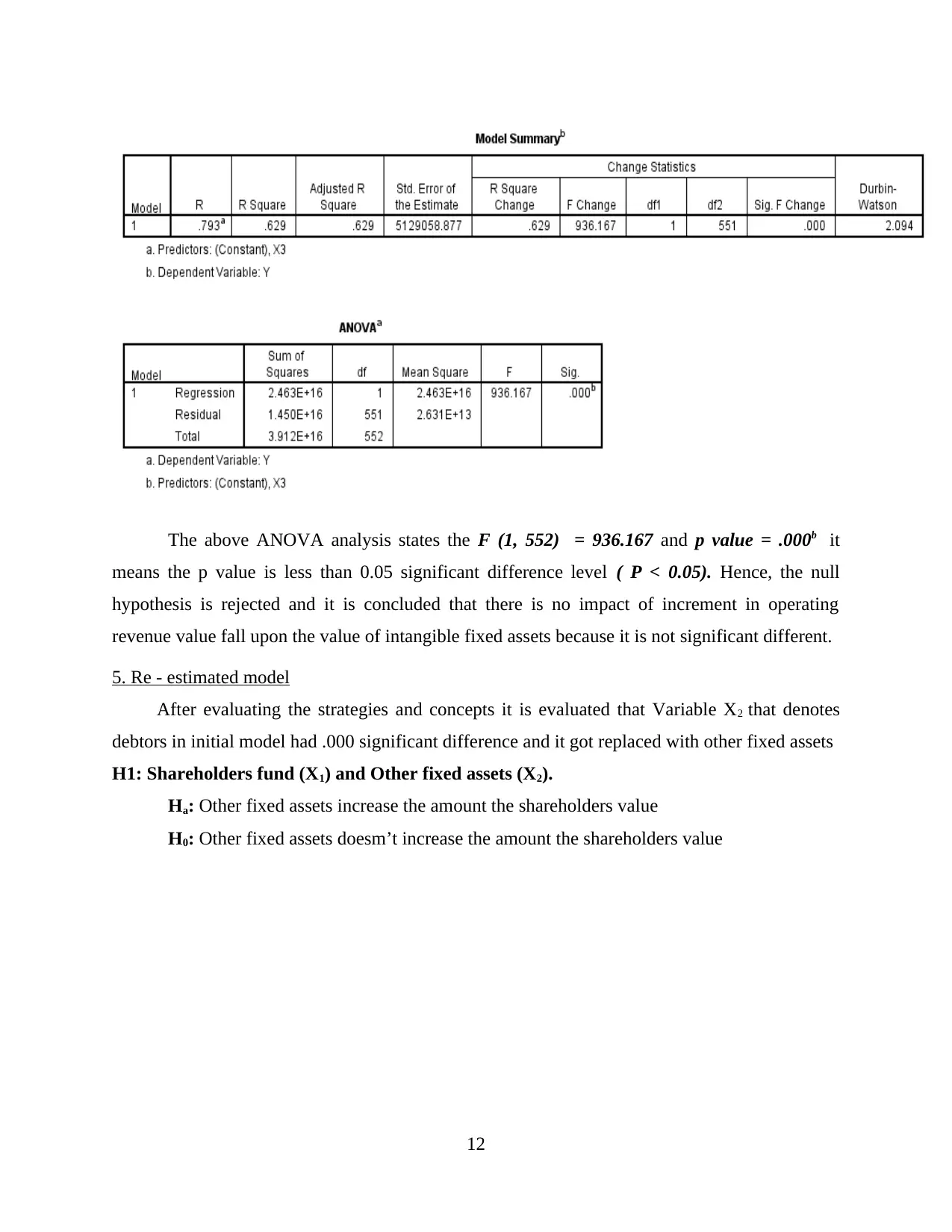
The above ANOVA analysis states the F (1, 552) = 936.167 and p value = .000b it
means the p value is less than 0.05 significant difference level ( P < 0.05). Hence, the null
hypothesis is rejected and it is concluded that there is no impact of increment in operating
revenue value fall upon the value of intangible fixed assets because it is not significant different.
5. Re - estimated model
After evaluating the strategies and concepts it is evaluated that Variable X2 that denotes
debtors in initial model had .000 significant difference and it got replaced with other fixed assets
H1: Shareholders fund (X1) and Other fixed assets (X2).
Ha: Other fixed assets increase the amount the shareholders value
H0: Other fixed assets doesm’t increase the amount the shareholders value
12
means the p value is less than 0.05 significant difference level ( P < 0.05). Hence, the null
hypothesis is rejected and it is concluded that there is no impact of increment in operating
revenue value fall upon the value of intangible fixed assets because it is not significant different.
5. Re - estimated model
After evaluating the strategies and concepts it is evaluated that Variable X2 that denotes
debtors in initial model had .000 significant difference and it got replaced with other fixed assets
H1: Shareholders fund (X1) and Other fixed assets (X2).
Ha: Other fixed assets increase the amount the shareholders value
H0: Other fixed assets doesm’t increase the amount the shareholders value
12
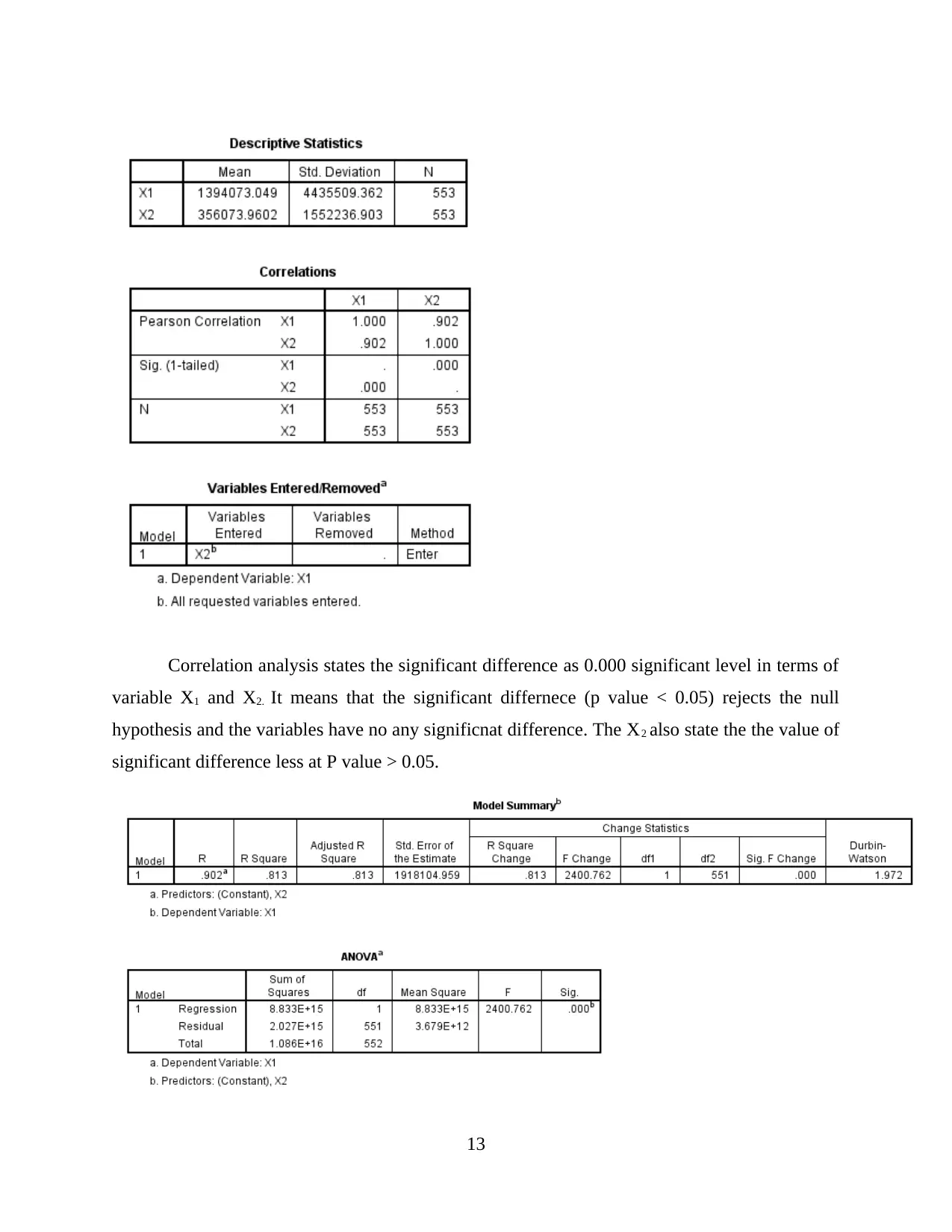
Correlation analysis states the significant difference as 0.000 significant level in terms of
variable X1 and X2. It means that the significant differnece (p value < 0.05) rejects the null
hypothesis and the variables have no any significnat difference. The X2 also state the the value of
significant difference less at P value > 0.05.
13
variable X1 and X2. It means that the significant differnece (p value < 0.05) rejects the null
hypothesis and the variables have no any significnat difference. The X2 also state the the value of
significant difference less at P value > 0.05.
13
You're viewing a preview
Unlock full access by subscribing today!
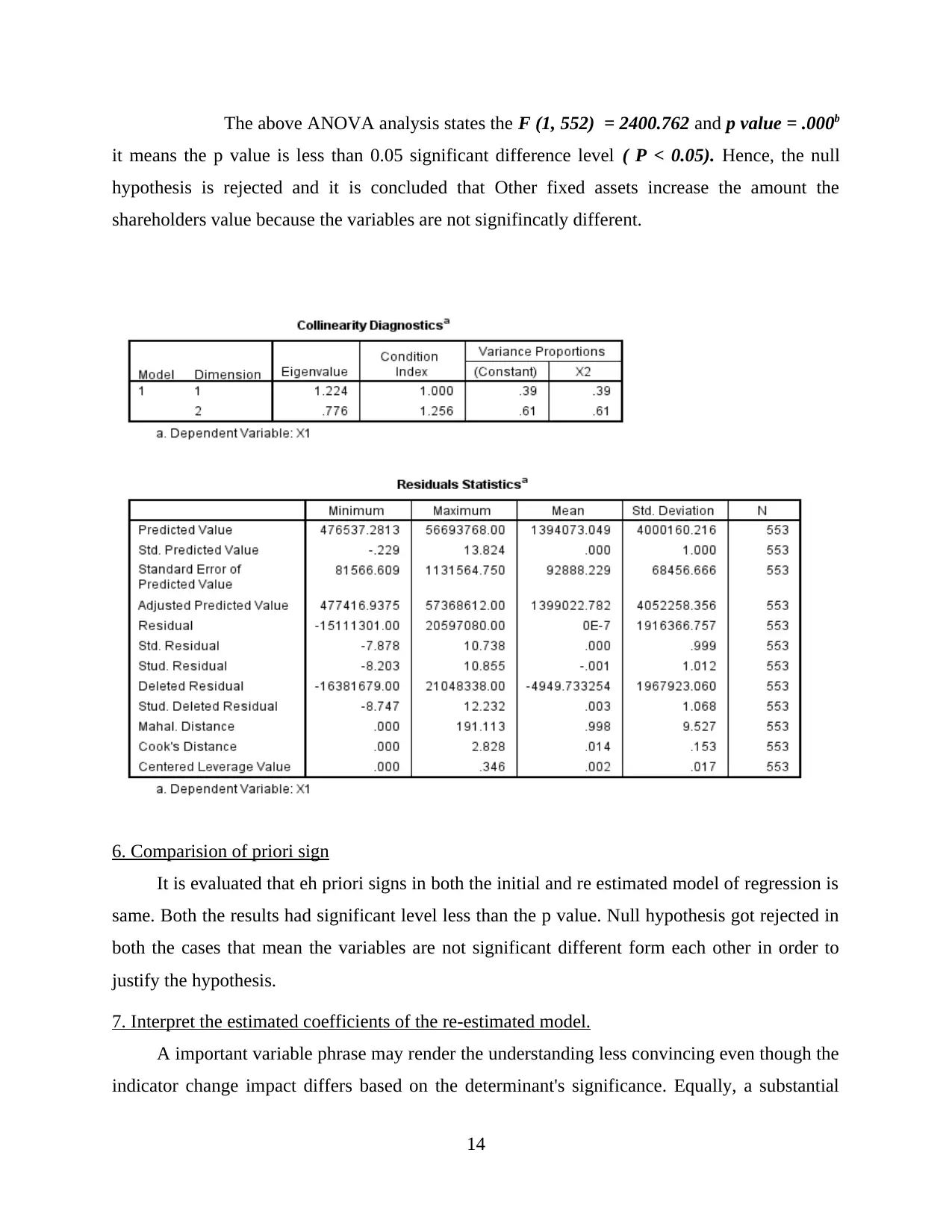
The above ANOVA analysis states the F (1, 552) = 2400.762 and p value = .000b
it means the p value is less than 0.05 significant difference level ( P < 0.05). Hence, the null
hypothesis is rejected and it is concluded that Other fixed assets increase the amount the
shareholders value because the variables are not signifincatly different.
6. Comparision of priori sign
It is evaluated that eh priori signs in both the initial and re estimated model of regression is
same. Both the results had significant level less than the p value. Null hypothesis got rejected in
both the cases that mean the variables are not significant different form each other in order to
justify the hypothesis.
7. Interpret the estimated coefficients of the re-estimated model.
A important variable phrase may render the understanding less convincing even though the
indicator change impact differs based on the determinant's significance. Equally, a substantial
14
it means the p value is less than 0.05 significant difference level ( P < 0.05). Hence, the null
hypothesis is rejected and it is concluded that Other fixed assets increase the amount the
shareholders value because the variables are not signifincatly different.
6. Comparision of priori sign
It is evaluated that eh priori signs in both the initial and re estimated model of regression is
same. Both the results had significant level less than the p value. Null hypothesis got rejected in
both the cases that mean the variables are not significant different form each other in order to
justify the hypothesis.
7. Interpret the estimated coefficients of the re-estimated model.
A important variable phrase may render the understanding less convincing even though the
indicator change impact differs based on the determinant's significance. Equally, a substantial
14
Paraphrase This Document
Need a fresh take? Get an instant paraphrase of this document with our AI Paraphraser
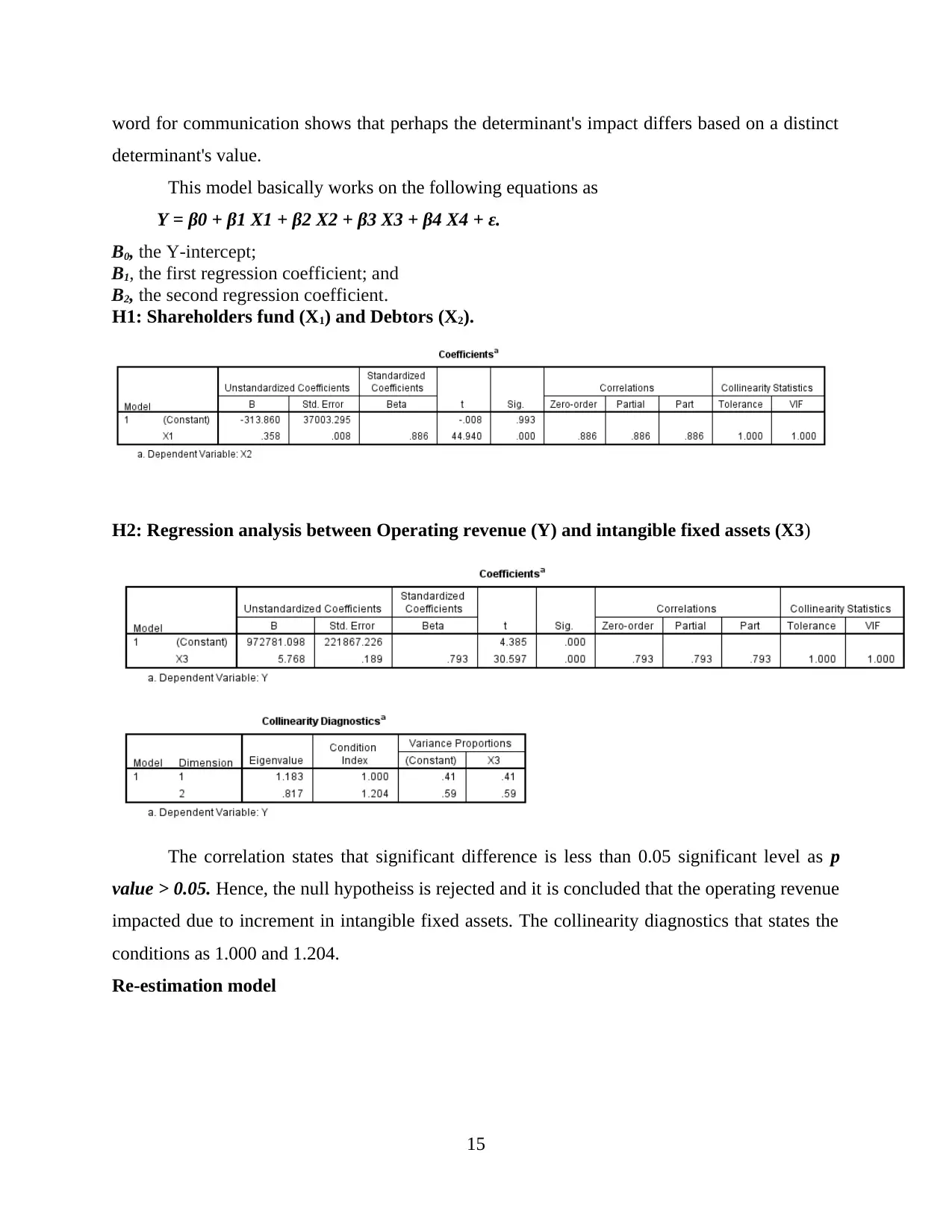
word for communication shows that perhaps the determinant's impact differs based on a distinct
determinant's value.
This model basically works on the following equations as
Y = β0 + β1 X1 + β2 X2 + β3 X3 + β4 X4 + ε.
B0, the Y-intercept;
B1, the first regression coefficient; and
B2, the second regression coefficient.
H1: Shareholders fund (X1) and Debtors (X2).
H2: Regression analysis between Operating revenue (Y) and intangible fixed assets (X3)
The correlation states that significant difference is less than 0.05 significant level as p
value > 0.05. Hence, the null hypotheiss is rejected and it is concluded that the operating revenue
impacted due to increment in intangible fixed assets. The collinearity diagnostics that states the
conditions as 1.000 and 1.204.
Re-estimation model
15
determinant's value.
This model basically works on the following equations as
Y = β0 + β1 X1 + β2 X2 + β3 X3 + β4 X4 + ε.
B0, the Y-intercept;
B1, the first regression coefficient; and
B2, the second regression coefficient.
H1: Shareholders fund (X1) and Debtors (X2).
H2: Regression analysis between Operating revenue (Y) and intangible fixed assets (X3)
The correlation states that significant difference is less than 0.05 significant level as p
value > 0.05. Hence, the null hypotheiss is rejected and it is concluded that the operating revenue
impacted due to increment in intangible fixed assets. The collinearity diagnostics that states the
conditions as 1.000 and 1.204.
Re-estimation model
15
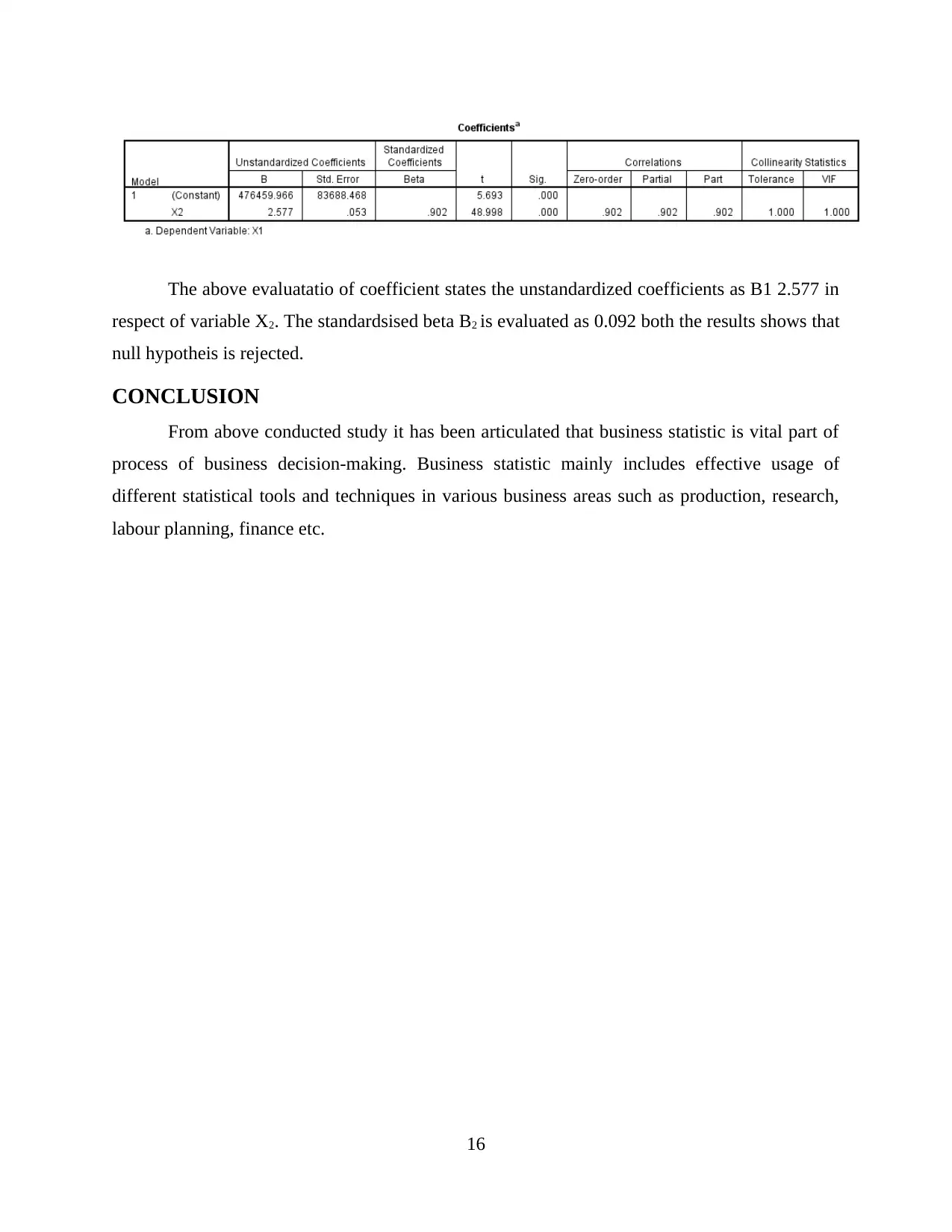
The above evaluatatio of coefficient states the unstandardized coefficients as B1 2.577 in
respect of variable X2. The standardsised beta B2 is evaluated as 0.092 both the results shows that
null hypotheis is rejected.
CONCLUSION
From above conducted study it has been articulated that business statistic is vital part of
process of business decision-making. Business statistic mainly includes effective usage of
different statistical tools and techniques in various business areas such as production, research,
labour planning, finance etc.
16
respect of variable X2. The standardsised beta B2 is evaluated as 0.092 both the results shows that
null hypotheis is rejected.
CONCLUSION
From above conducted study it has been articulated that business statistic is vital part of
process of business decision-making. Business statistic mainly includes effective usage of
different statistical tools and techniques in various business areas such as production, research,
labour planning, finance etc.
16
You're viewing a preview
Unlock full access by subscribing today!
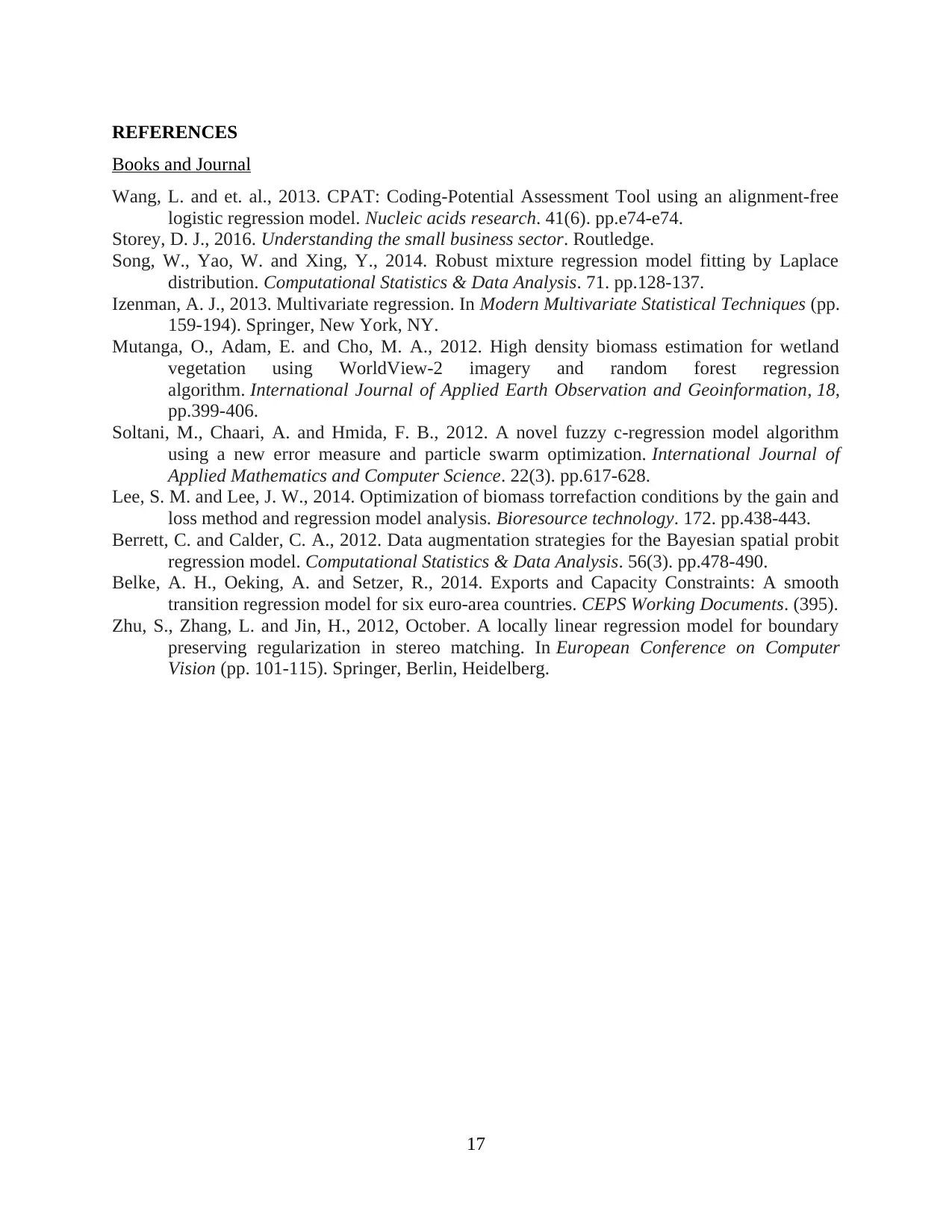
REFERENCES
Books and Journal
Wang, L. and et. al., 2013. CPAT: Coding-Potential Assessment Tool using an alignment-free
logistic regression model. Nucleic acids research. 41(6). pp.e74-e74.
Storey, D. J., 2016. Understanding the small business sector. Routledge.
Song, W., Yao, W. and Xing, Y., 2014. Robust mixture regression model fitting by Laplace
distribution. Computational Statistics & Data Analysis. 71. pp.128-137.
Izenman, A. J., 2013. Multivariate regression. In Modern Multivariate Statistical Techniques (pp.
159-194). Springer, New York, NY.
Mutanga, O., Adam, E. and Cho, M. A., 2012. High density biomass estimation for wetland
vegetation using WorldView-2 imagery and random forest regression
algorithm. International Journal of Applied Earth Observation and Geoinformation, 18,
pp.399-406.
Soltani, M., Chaari, A. and Hmida, F. B., 2012. A novel fuzzy c-regression model algorithm
using a new error measure and particle swarm optimization. International Journal of
Applied Mathematics and Computer Science. 22(3). pp.617-628.
Lee, S. M. and Lee, J. W., 2014. Optimization of biomass torrefaction conditions by the gain and
loss method and regression model analysis. Bioresource technology. 172. pp.438-443.
Berrett, C. and Calder, C. A., 2012. Data augmentation strategies for the Bayesian spatial probit
regression model. Computational Statistics & Data Analysis. 56(3). pp.478-490.
Belke, A. H., Oeking, A. and Setzer, R., 2014. Exports and Capacity Constraints: A smooth
transition regression model for six euro-area countries. CEPS Working Documents. (395).
Zhu, S., Zhang, L. and Jin, H., 2012, October. A locally linear regression model for boundary
preserving regularization in stereo matching. In European Conference on Computer
Vision (pp. 101-115). Springer, Berlin, Heidelberg.
17
Books and Journal
Wang, L. and et. al., 2013. CPAT: Coding-Potential Assessment Tool using an alignment-free
logistic regression model. Nucleic acids research. 41(6). pp.e74-e74.
Storey, D. J., 2016. Understanding the small business sector. Routledge.
Song, W., Yao, W. and Xing, Y., 2014. Robust mixture regression model fitting by Laplace
distribution. Computational Statistics & Data Analysis. 71. pp.128-137.
Izenman, A. J., 2013. Multivariate regression. In Modern Multivariate Statistical Techniques (pp.
159-194). Springer, New York, NY.
Mutanga, O., Adam, E. and Cho, M. A., 2012. High density biomass estimation for wetland
vegetation using WorldView-2 imagery and random forest regression
algorithm. International Journal of Applied Earth Observation and Geoinformation, 18,
pp.399-406.
Soltani, M., Chaari, A. and Hmida, F. B., 2012. A novel fuzzy c-regression model algorithm
using a new error measure and particle swarm optimization. International Journal of
Applied Mathematics and Computer Science. 22(3). pp.617-628.
Lee, S. M. and Lee, J. W., 2014. Optimization of biomass torrefaction conditions by the gain and
loss method and regression model analysis. Bioresource technology. 172. pp.438-443.
Berrett, C. and Calder, C. A., 2012. Data augmentation strategies for the Bayesian spatial probit
regression model. Computational Statistics & Data Analysis. 56(3). pp.478-490.
Belke, A. H., Oeking, A. and Setzer, R., 2014. Exports and Capacity Constraints: A smooth
transition regression model for six euro-area countries. CEPS Working Documents. (395).
Zhu, S., Zhang, L. and Jin, H., 2012, October. A locally linear regression model for boundary
preserving regularization in stereo matching. In European Conference on Computer
Vision (pp. 101-115). Springer, Berlin, Heidelberg.
17
1 out of 19
Related Documents

Your All-in-One AI-Powered Toolkit for Academic Success.
+13062052269
info@desklib.com
Available 24*7 on WhatsApp / Email
Unlock your academic potential
© 2024 | Zucol Services PVT LTD | All rights reserved.