Business Analytics Report: Analysis of Clinic Data, NIT3171
VerifiedAdded on 2022/08/13
|16
|2184
|11
Report
AI Summary
This report presents a comprehensive business analytics analysis of clinic data from multiple clinics in Australia. It begins with a descriptive analysis, examining patient demographics such as cities, ethnic backgrounds, gender, and age, as well as the most frequent descriptions, prescribed drugs, and reasons for visits. The descriptive section includes tables and figures to visualize the data and identify key trends, such as the top cities and ethnic groups of patients. The report then delves into predictive analysis, using regression to forecast annual company income and a non-linear model to predict cold and flu cases. It also investigates associations between different diseases like heart disease, obesity, hypertension, and diabetes. Finally, the report concludes with a prescriptive analysis, offering recommendations for business growth, such as opening new clinics in high-patient-volume cities and hiring staff that align with the patient demographics, including the analysis of attributes that affect patient regularity and a discussion of probabilities related to patient data. The overall goal is to provide actionable insights to improve the company's performance and strategic decision-making.
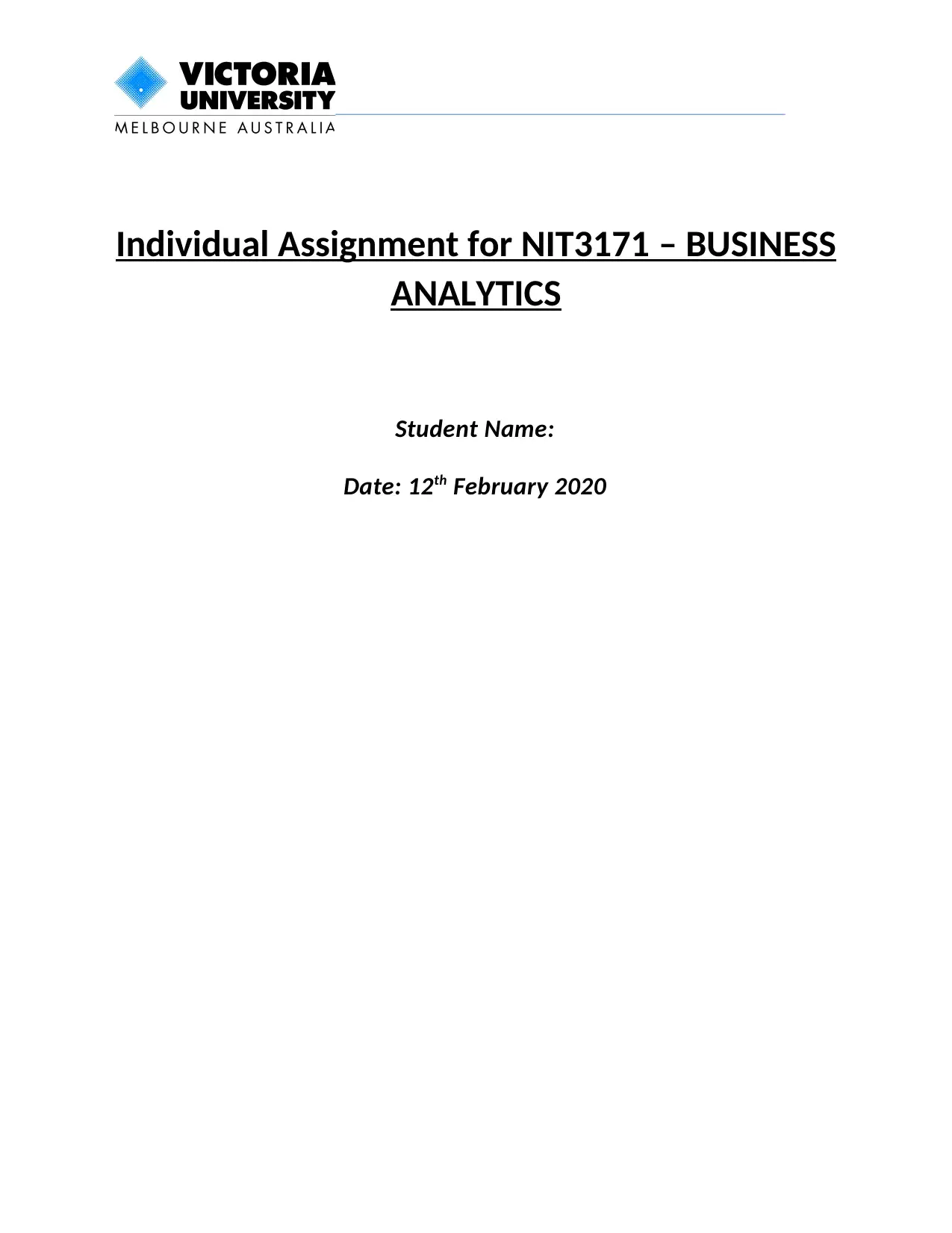
Individual Assignment for NIT3171 – BUSINESS
ANALYTICS
Student Name:
Date: 12th February 2020
ANALYTICS
Student Name:
Date: 12th February 2020
Paraphrase This Document
Need a fresh take? Get an instant paraphrase of this document with our AI Paraphraser
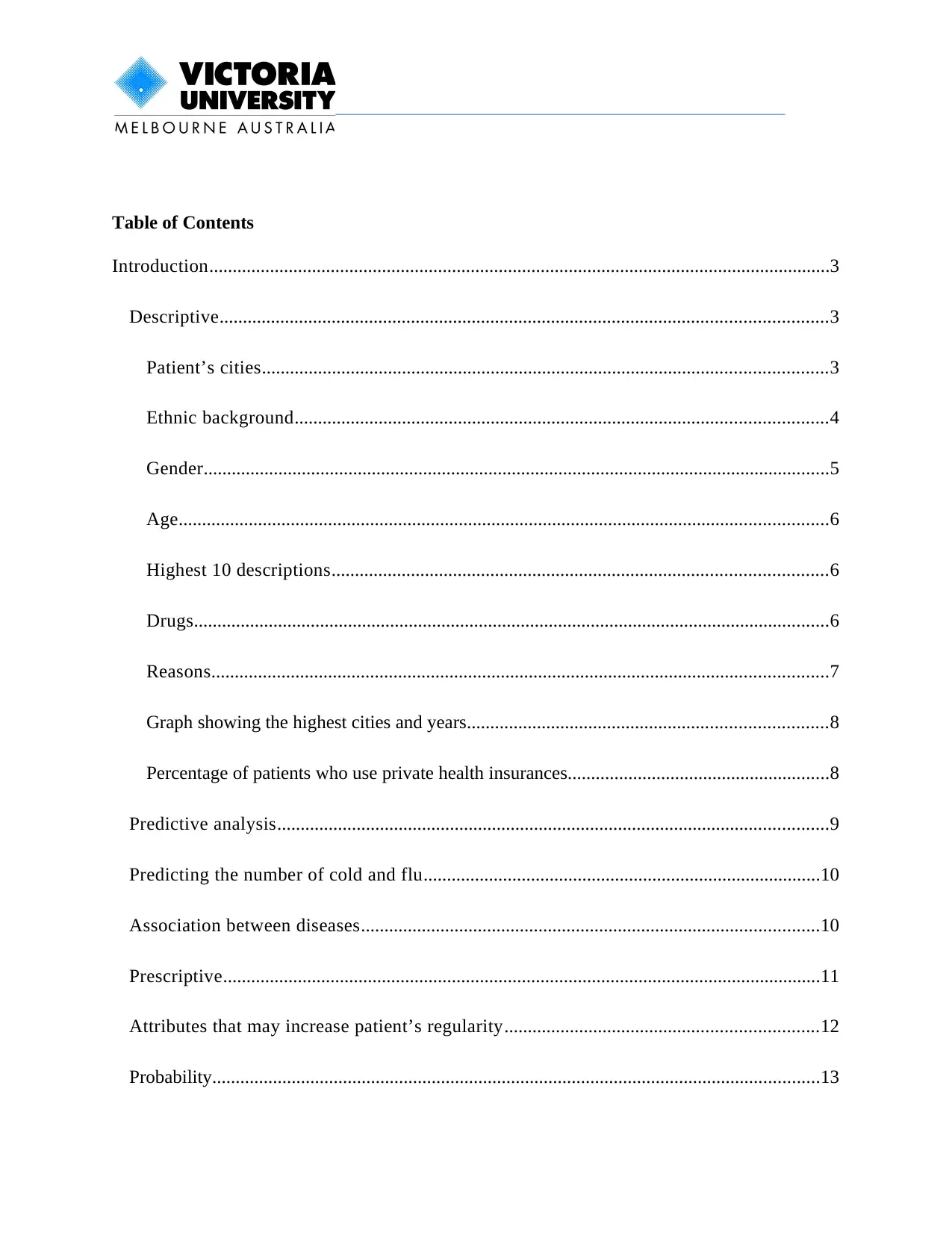
Table of Contents
Introduction.....................................................................................................................................3
Descriptive..................................................................................................................................3
Patient’s cities.........................................................................................................................3
Ethnic background..................................................................................................................4
Gender......................................................................................................................................5
Age...........................................................................................................................................6
Highest 10 descriptions..........................................................................................................6
Drugs........................................................................................................................................6
Reasons....................................................................................................................................7
Graph showing the highest cities and years.............................................................................8
Percentage of patients who use private health insurances........................................................8
Predictive analysis......................................................................................................................9
Predicting the number of cold and flu.....................................................................................10
Association between diseases..................................................................................................10
Prescriptive................................................................................................................................11
Attributes that may increase patient’s regularity...................................................................12
Probability..................................................................................................................................13
Introduction.....................................................................................................................................3
Descriptive..................................................................................................................................3
Patient’s cities.........................................................................................................................3
Ethnic background..................................................................................................................4
Gender......................................................................................................................................5
Age...........................................................................................................................................6
Highest 10 descriptions..........................................................................................................6
Drugs........................................................................................................................................6
Reasons....................................................................................................................................7
Graph showing the highest cities and years.............................................................................8
Percentage of patients who use private health insurances........................................................8
Predictive analysis......................................................................................................................9
Predicting the number of cold and flu.....................................................................................10
Association between diseases..................................................................................................10
Prescriptive................................................................................................................................11
Attributes that may increase patient’s regularity...................................................................12
Probability..................................................................................................................................13
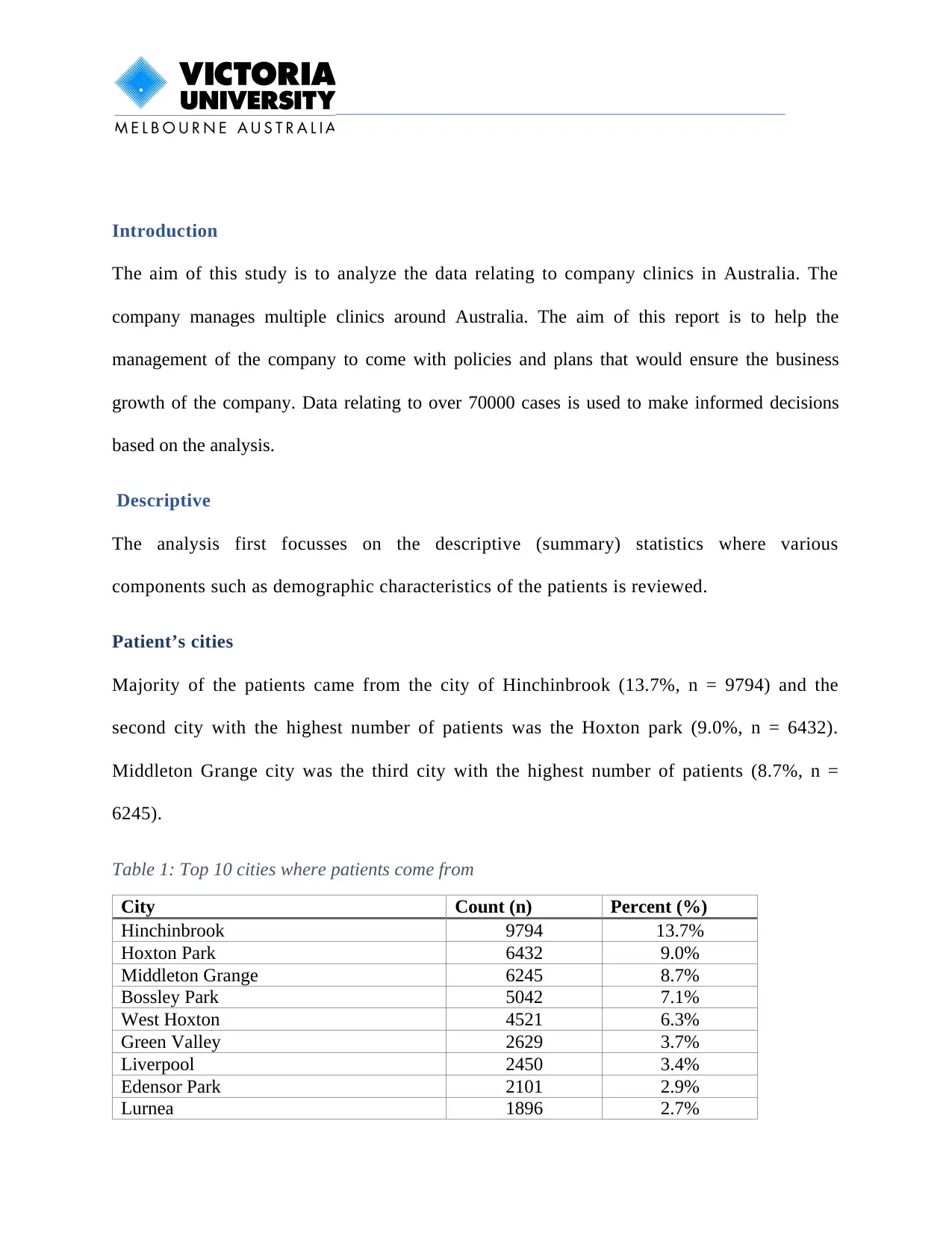
Introduction
The aim of this study is to analyze the data relating to company clinics in Australia. The
company manages multiple clinics around Australia. The aim of this report is to help the
management of the company to come with policies and plans that would ensure the business
growth of the company. Data relating to over 70000 cases is used to make informed decisions
based on the analysis.
Descriptive
The analysis first focusses on the descriptive (summary) statistics where various
components such as demographic characteristics of the patients is reviewed.
Patient’s cities
Majority of the patients came from the city of Hinchinbrook (13.7%, n = 9794) and the
second city with the highest number of patients was the Hoxton park (9.0%, n = 6432).
Middleton Grange city was the third city with the highest number of patients (8.7%, n =
6245).
Table 1: Top 10 cities where patients come from
City Count (n) Percent (%)
Hinchinbrook 9794 13.7%
Hoxton Park 6432 9.0%
Middleton Grange 6245 8.7%
Bossley Park 5042 7.1%
West Hoxton 4521 6.3%
Green Valley 2629 3.7%
Liverpool 2450 3.4%
Edensor Park 2101 2.9%
Lurnea 1896 2.7%
The aim of this study is to analyze the data relating to company clinics in Australia. The
company manages multiple clinics around Australia. The aim of this report is to help the
management of the company to come with policies and plans that would ensure the business
growth of the company. Data relating to over 70000 cases is used to make informed decisions
based on the analysis.
Descriptive
The analysis first focusses on the descriptive (summary) statistics where various
components such as demographic characteristics of the patients is reviewed.
Patient’s cities
Majority of the patients came from the city of Hinchinbrook (13.7%, n = 9794) and the
second city with the highest number of patients was the Hoxton park (9.0%, n = 6432).
Middleton Grange city was the third city with the highest number of patients (8.7%, n =
6245).
Table 1: Top 10 cities where patients come from
City Count (n) Percent (%)
Hinchinbrook 9794 13.7%
Hoxton Park 6432 9.0%
Middleton Grange 6245 8.7%
Bossley Park 5042 7.1%
West Hoxton 4521 6.3%
Green Valley 2629 3.7%
Liverpool 2450 3.4%
Edensor Park 2101 2.9%
Lurnea 1896 2.7%
⊘ This is a preview!⊘
Do you want full access?
Subscribe today to unlock all pages.

Trusted by 1+ million students worldwide
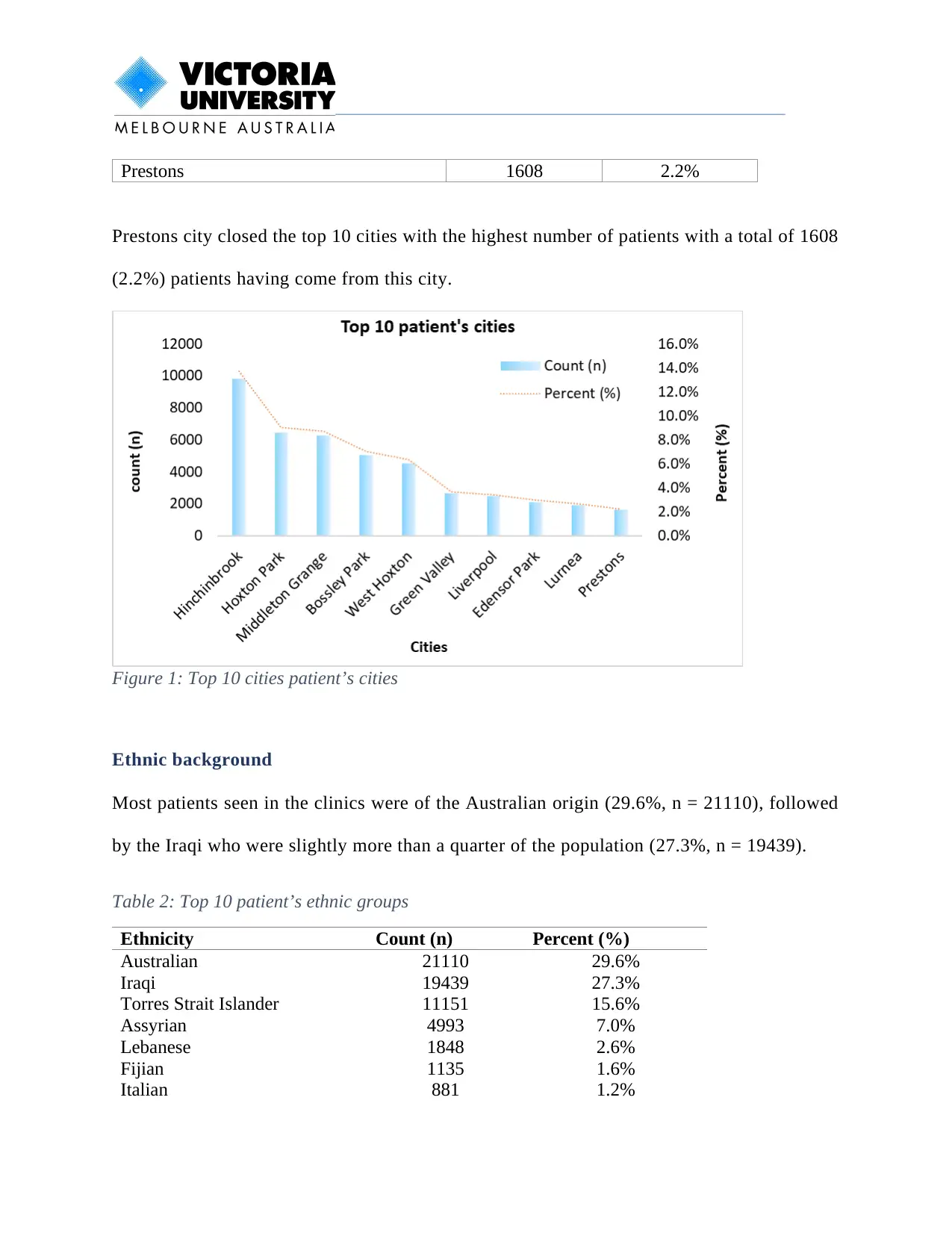
Prestons 1608 2.2%
Prestons city closed the top 10 cities with the highest number of patients with a total of 1608
(2.2%) patients having come from this city.
Figure 1: Top 10 cities patient’s cities
Ethnic background
Most patients seen in the clinics were of the Australian origin (29.6%, n = 21110), followed
by the Iraqi who were slightly more than a quarter of the population (27.3%, n = 19439).
Table 2: Top 10 patient’s ethnic groups
Ethnicity Count (n) Percent (%)
Australian 21110 29.6%
Iraqi 19439 27.3%
Torres Strait Islander 11151 15.6%
Assyrian 4993 7.0%
Lebanese 1848 2.6%
Fijian 1135 1.6%
Italian 881 1.2%
Prestons city closed the top 10 cities with the highest number of patients with a total of 1608
(2.2%) patients having come from this city.
Figure 1: Top 10 cities patient’s cities
Ethnic background
Most patients seen in the clinics were of the Australian origin (29.6%, n = 21110), followed
by the Iraqi who were slightly more than a quarter of the population (27.3%, n = 19439).
Table 2: Top 10 patient’s ethnic groups
Ethnicity Count (n) Percent (%)
Australian 21110 29.6%
Iraqi 19439 27.3%
Torres Strait Islander 11151 15.6%
Assyrian 4993 7.0%
Lebanese 1848 2.6%
Fijian 1135 1.6%
Italian 881 1.2%
Paraphrase This Document
Need a fresh take? Get an instant paraphrase of this document with our AI Paraphraser
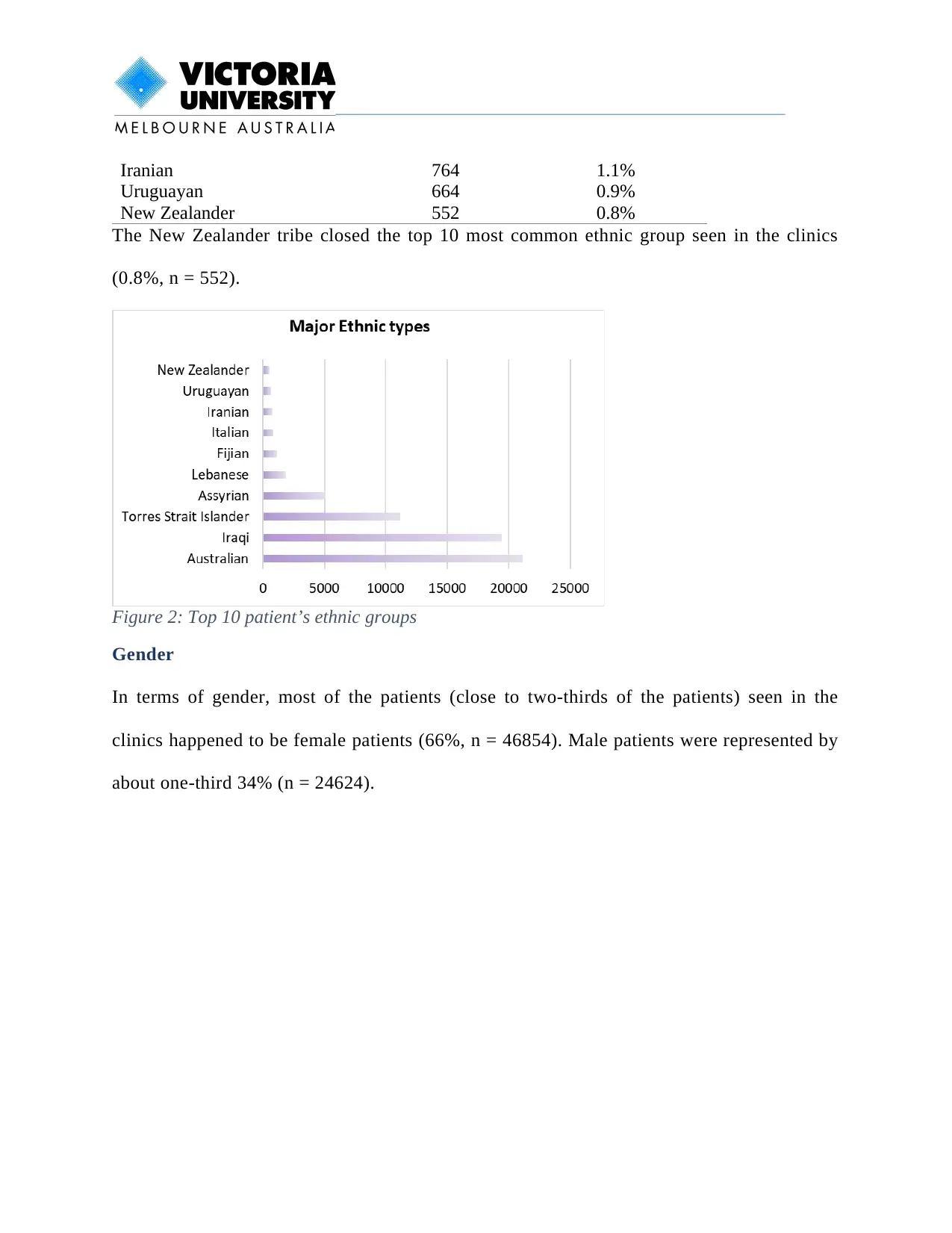
Iranian 764 1.1%
Uruguayan 664 0.9%
New Zealander 552 0.8%
The New Zealander tribe closed the top 10 most common ethnic group seen in the clinics
(0.8%, n = 552).
Figure 2: Top 10 patient’s ethnic groups
Gender
In terms of gender, most of the patients (close to two-thirds of the patients) seen in the
clinics happened to be female patients (66%, n = 46854). Male patients were represented by
about one-third 34% (n = 24624).
Uruguayan 664 0.9%
New Zealander 552 0.8%
The New Zealander tribe closed the top 10 most common ethnic group seen in the clinics
(0.8%, n = 552).
Figure 2: Top 10 patient’s ethnic groups
Gender
In terms of gender, most of the patients (close to two-thirds of the patients) seen in the
clinics happened to be female patients (66%, n = 46854). Male patients were represented by
about one-third 34% (n = 24624).
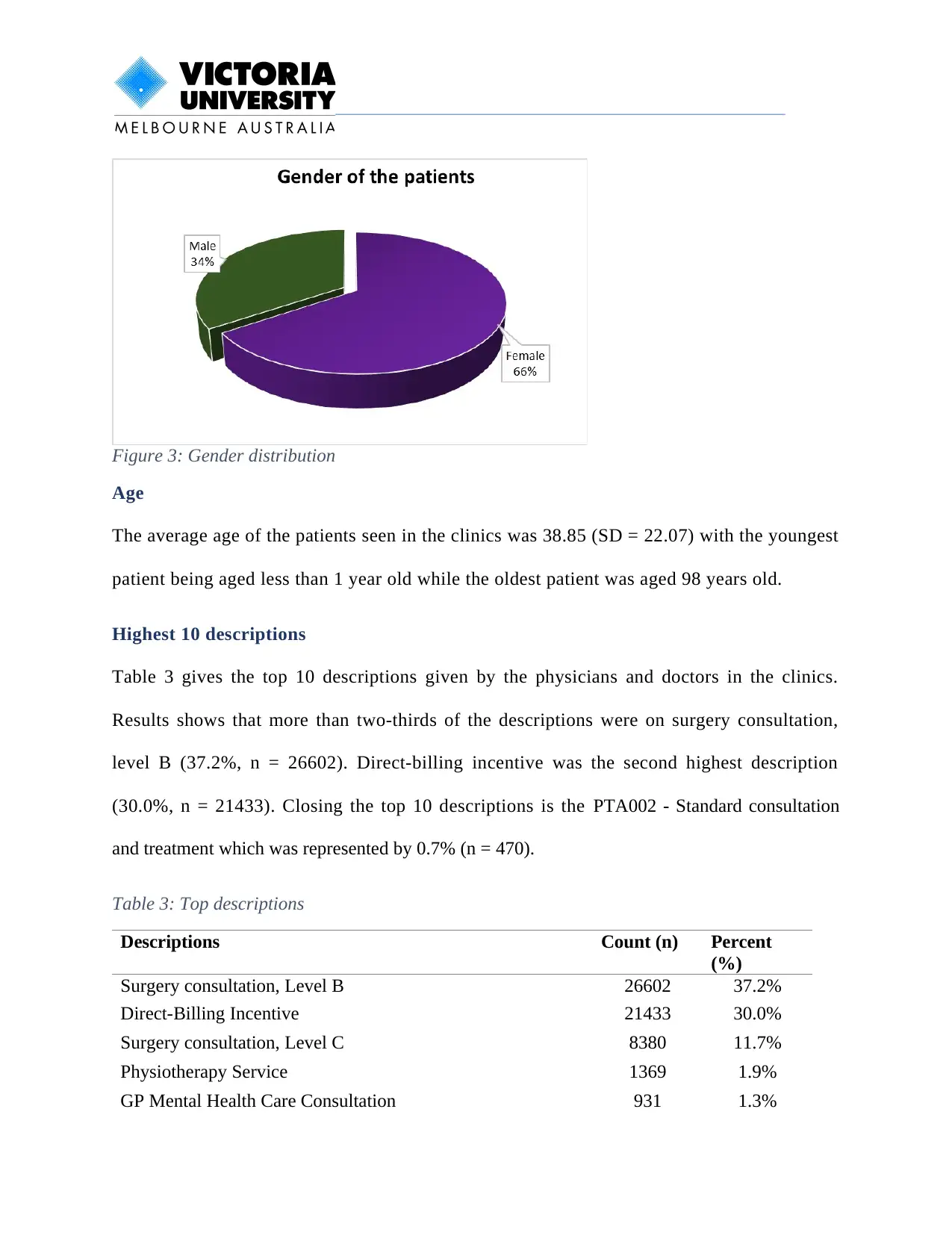
Figure 3: Gender distribution
Age
The average age of the patients seen in the clinics was 38.85 (SD = 22.07) with the youngest
patient being aged less than 1 year old while the oldest patient was aged 98 years old.
Highest 10 descriptions
Table 3 gives the top 10 descriptions given by the physicians and doctors in the clinics.
Results shows that more than two-thirds of the descriptions were on surgery consultation,
level B (37.2%, n = 26602). Direct-billing incentive was the second highest description
(30.0%, n = 21433). Closing the top 10 descriptions is the PTA002 - Standard consultation
and treatment which was represented by 0.7% (n = 470).
Table 3: Top descriptions
Descriptions Count (n) Percent
(%)
Surgery consultation, Level B 26602 37.2%
Direct-Billing Incentive 21433 30.0%
Surgery consultation, Level C 8380 11.7%
Physiotherapy Service 1369 1.9%
GP Mental Health Care Consultation 931 1.3%
Age
The average age of the patients seen in the clinics was 38.85 (SD = 22.07) with the youngest
patient being aged less than 1 year old while the oldest patient was aged 98 years old.
Highest 10 descriptions
Table 3 gives the top 10 descriptions given by the physicians and doctors in the clinics.
Results shows that more than two-thirds of the descriptions were on surgery consultation,
level B (37.2%, n = 26602). Direct-billing incentive was the second highest description
(30.0%, n = 21433). Closing the top 10 descriptions is the PTA002 - Standard consultation
and treatment which was represented by 0.7% (n = 470).
Table 3: Top descriptions
Descriptions Count (n) Percent
(%)
Surgery consultation, Level B 26602 37.2%
Direct-Billing Incentive 21433 30.0%
Surgery consultation, Level C 8380 11.7%
Physiotherapy Service 1369 1.9%
GP Mental Health Care Consultation 931 1.3%
⊘ This is a preview!⊘
Do you want full access?
Subscribe today to unlock all pages.

Trusted by 1+ million students worldwide
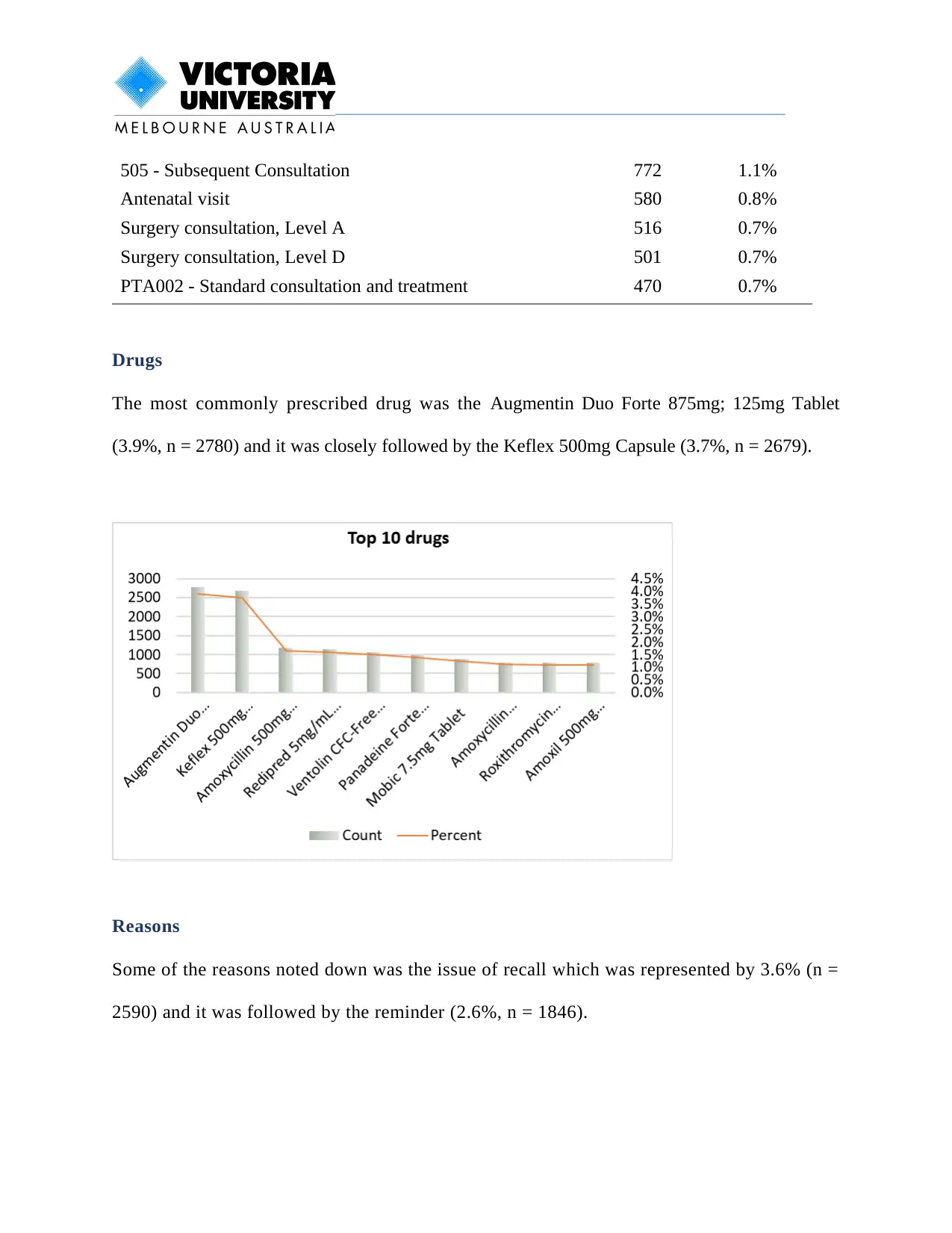
505 - Subsequent Consultation 772 1.1%
Antenatal visit 580 0.8%
Surgery consultation, Level A 516 0.7%
Surgery consultation, Level D 501 0.7%
PTA002 - Standard consultation and treatment 470 0.7%
Drugs
The most commonly prescribed drug was the Augmentin Duo Forte 875mg; 125mg Tablet
(3.9%, n = 2780) and it was closely followed by the Keflex 500mg Capsule (3.7%, n = 2679).
Reasons
Some of the reasons noted down was the issue of recall which was represented by 3.6% (n =
2590) and it was followed by the reminder (2.6%, n = 1846).
Antenatal visit 580 0.8%
Surgery consultation, Level A 516 0.7%
Surgery consultation, Level D 501 0.7%
PTA002 - Standard consultation and treatment 470 0.7%
Drugs
The most commonly prescribed drug was the Augmentin Duo Forte 875mg; 125mg Tablet
(3.9%, n = 2780) and it was closely followed by the Keflex 500mg Capsule (3.7%, n = 2679).
Reasons
Some of the reasons noted down was the issue of recall which was represented by 3.6% (n =
2590) and it was followed by the reminder (2.6%, n = 1846).
Paraphrase This Document
Need a fresh take? Get an instant paraphrase of this document with our AI Paraphraser
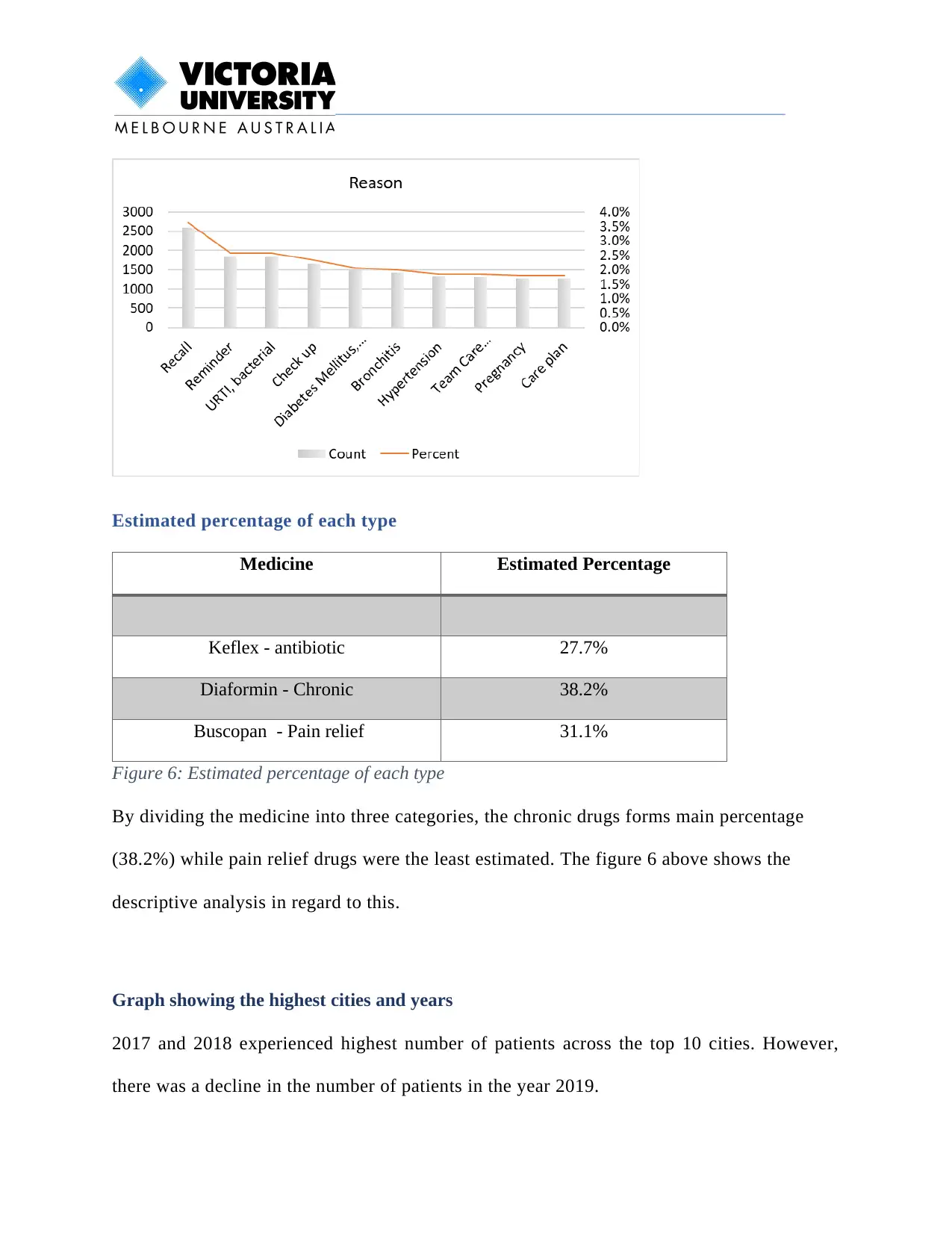
Estimated percentage of each type
Medicine Estimated Percentage
Keflex - antibiotic 27.7%
Diaformin - Chronic 38.2%
Buscopan - Pain relief 31.1%
Figure 6: Estimated percentage of each type
By dividing the medicine into three categories, the chronic drugs forms main percentage
(38.2%) while pain relief drugs were the least estimated. The figure 6 above shows the
descriptive analysis in regard to this.
Graph showing the highest cities and years
2017 and 2018 experienced highest number of patients across the top 10 cities. However,
there was a decline in the number of patients in the year 2019.
Medicine Estimated Percentage
Keflex - antibiotic 27.7%
Diaformin - Chronic 38.2%
Buscopan - Pain relief 31.1%
Figure 6: Estimated percentage of each type
By dividing the medicine into three categories, the chronic drugs forms main percentage
(38.2%) while pain relief drugs were the least estimated. The figure 6 above shows the
descriptive analysis in regard to this.
Graph showing the highest cities and years
2017 and 2018 experienced highest number of patients across the top 10 cities. However,
there was a decline in the number of patients in the year 2019.
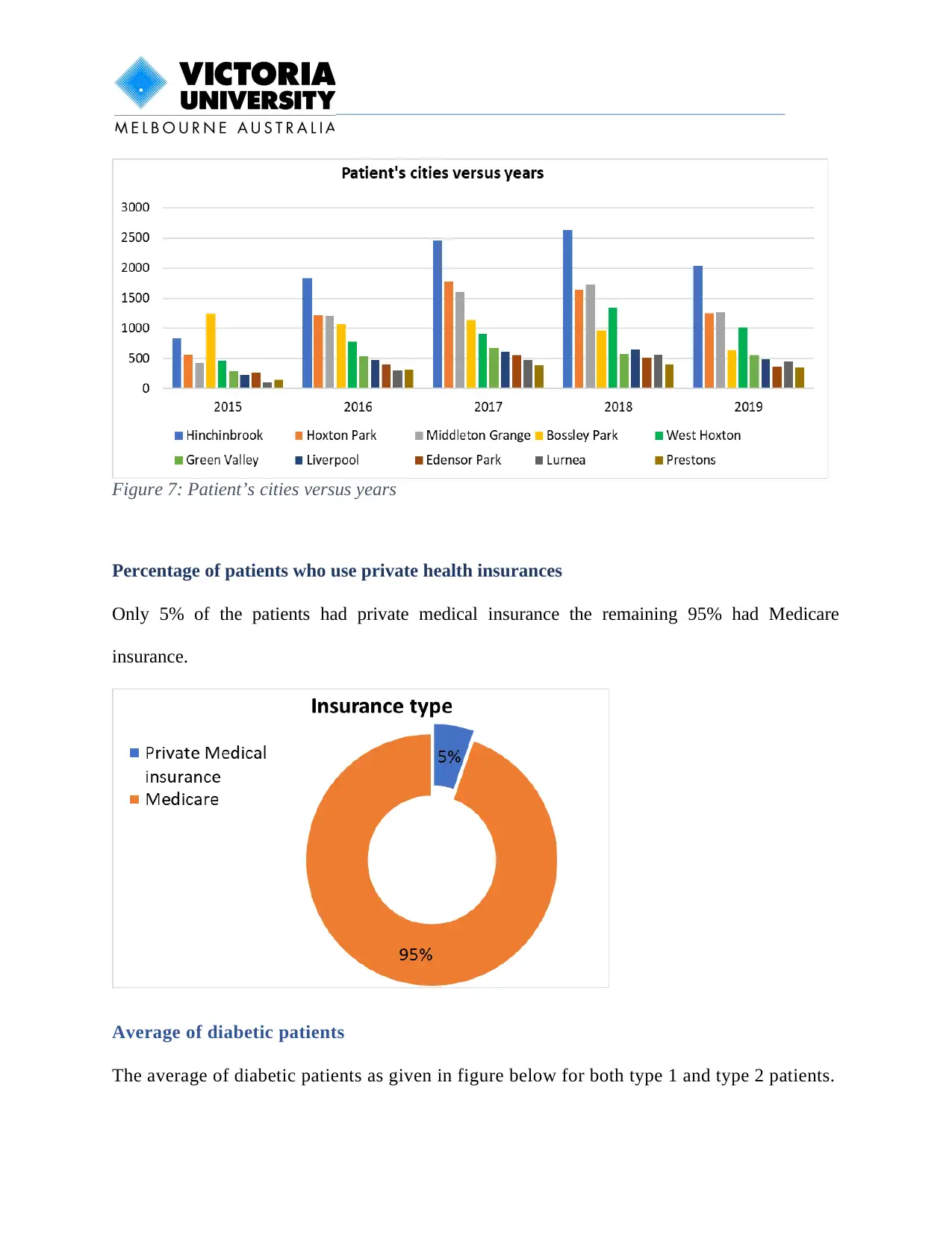
Figure 7: Patient’s cities versus years
Percentage of patients who use private health insurances
Only 5% of the patients had private medical insurance the remaining 95% had Medicare
insurance.
Average of diabetic patients
The average of diabetic patients as given in figure below for both type 1 and type 2 patients.
Percentage of patients who use private health insurances
Only 5% of the patients had private medical insurance the remaining 95% had Medicare
insurance.
Average of diabetic patients
The average of diabetic patients as given in figure below for both type 1 and type 2 patients.
⊘ This is a preview!⊘
Do you want full access?
Subscribe today to unlock all pages.

Trusted by 1+ million students worldwide
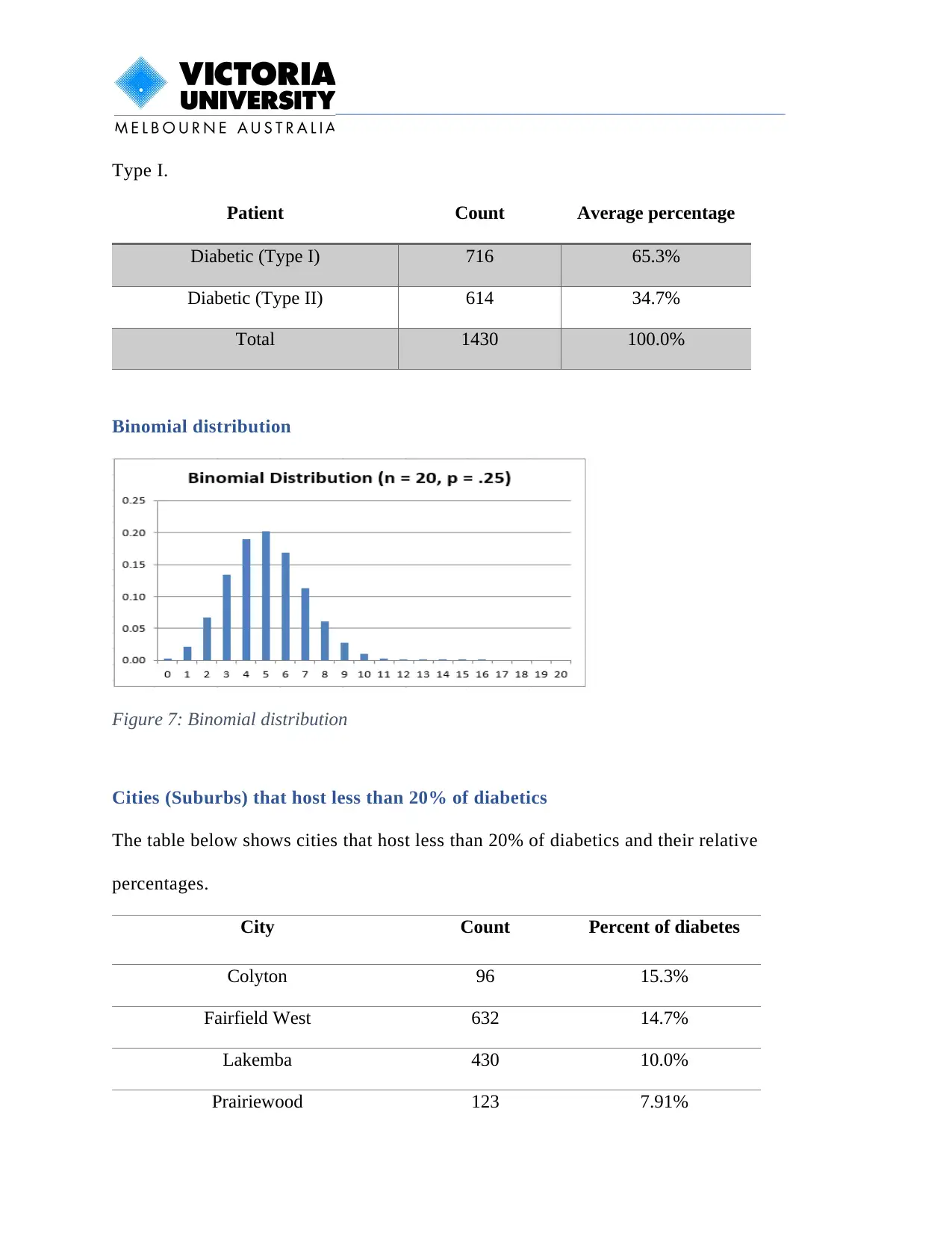
Type I.
Patient Count Average percentage
Diabetic (Type I) 716 65.3%
Diabetic (Type II) 614 34.7%
Total 1430 100.0%
Binomial distribution
Figure 7: Binomial distribution
Cities (Suburbs) that host less than 20% of diabetics
The table below shows cities that host less than 20% of diabetics and their relative
percentages.
City Count Percent of diabetes
Colyton 96 15.3%
Fairfield West 632 14.7%
Lakemba 430 10.0%
Prairiewood 123 7.91%
Patient Count Average percentage
Diabetic (Type I) 716 65.3%
Diabetic (Type II) 614 34.7%
Total 1430 100.0%
Binomial distribution
Figure 7: Binomial distribution
Cities (Suburbs) that host less than 20% of diabetics
The table below shows cities that host less than 20% of diabetics and their relative
percentages.
City Count Percent of diabetes
Colyton 96 15.3%
Fairfield West 632 14.7%
Lakemba 430 10.0%
Prairiewood 123 7.91%
Paraphrase This Document
Need a fresh take? Get an instant paraphrase of this document with our AI Paraphraser
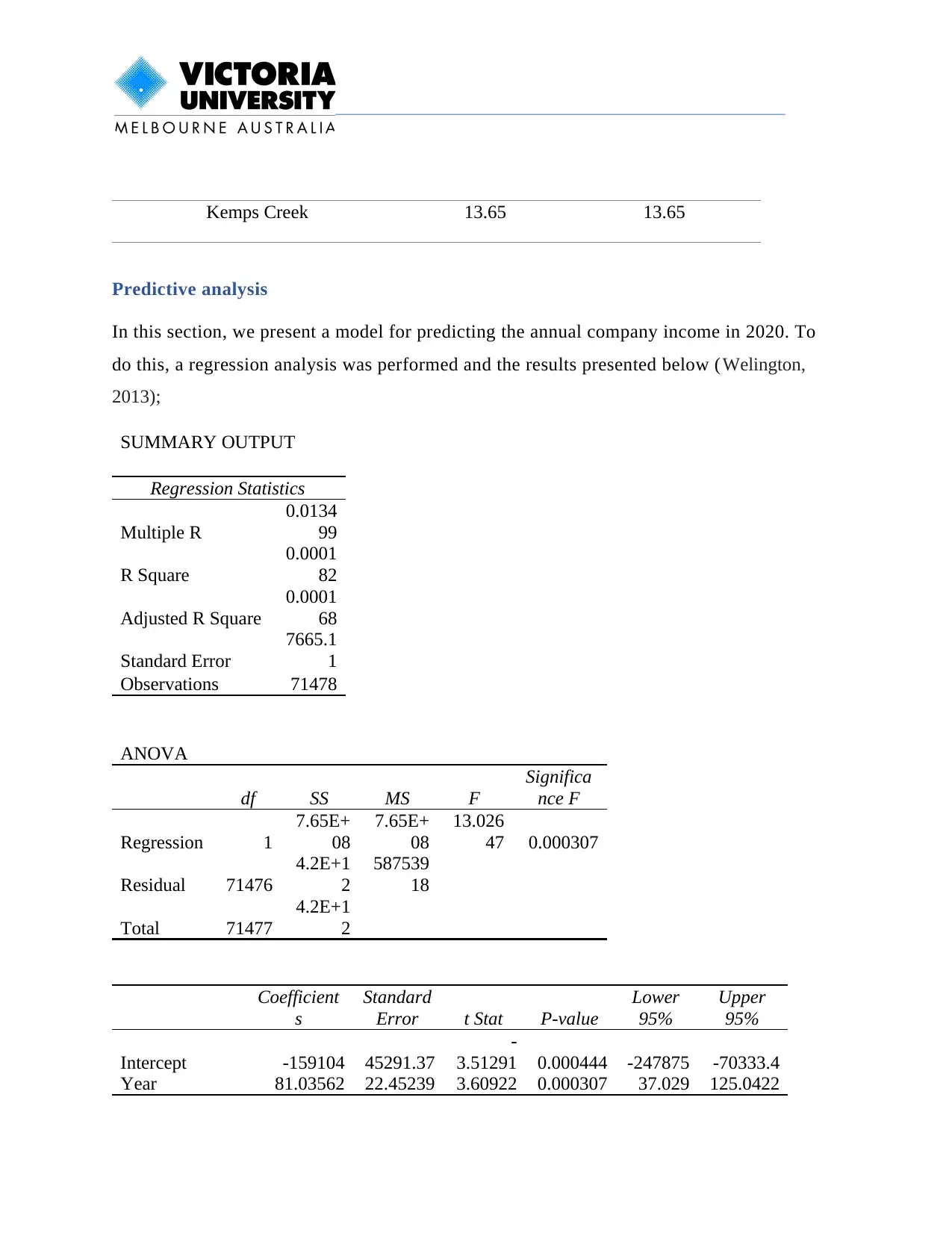
Kemps Creek 13.65 13.65
Predictive analysis
In this section, we present a model for predicting the annual company income in 2020. To
do this, a regression analysis was performed and the results presented below (Welington,
2013);
SUMMARY OUTPUT
Regression Statistics
Multiple R
0.0134
99
R Square
0.0001
82
Adjusted R Square
0.0001
68
Standard Error
7665.1
1
Observations 71478
ANOVA
df SS MS F
Significa
nce F
Regression 1
7.65E+
08
7.65E+
08
13.026
47 0.000307
Residual 71476
4.2E+1
2
587539
18
Total 71477
4.2E+1
2
Coefficient
s
Standard
Error t Stat P-value
Lower
95%
Upper
95%
Intercept -159104 45291.37
-
3.51291 0.000444 -247875 -70333.4
Year 81.03562 22.45239 3.60922 0.000307 37.029 125.0422
Predictive analysis
In this section, we present a model for predicting the annual company income in 2020. To
do this, a regression analysis was performed and the results presented below (Welington,
2013);
SUMMARY OUTPUT
Regression Statistics
Multiple R
0.0134
99
R Square
0.0001
82
Adjusted R Square
0.0001
68
Standard Error
7665.1
1
Observations 71478
ANOVA
df SS MS F
Significa
nce F
Regression 1
7.65E+
08
7.65E+
08
13.026
47 0.000307
Residual 71476
4.2E+1
2
587539
18
Total 71477
4.2E+1
2
Coefficient
s
Standard
Error t Stat P-value
Lower
95%
Upper
95%
Intercept -159104 45291.37
-
3.51291 0.000444 -247875 -70333.4
Year 81.03562 22.45239 3.60922 0.000307 37.029 125.0422
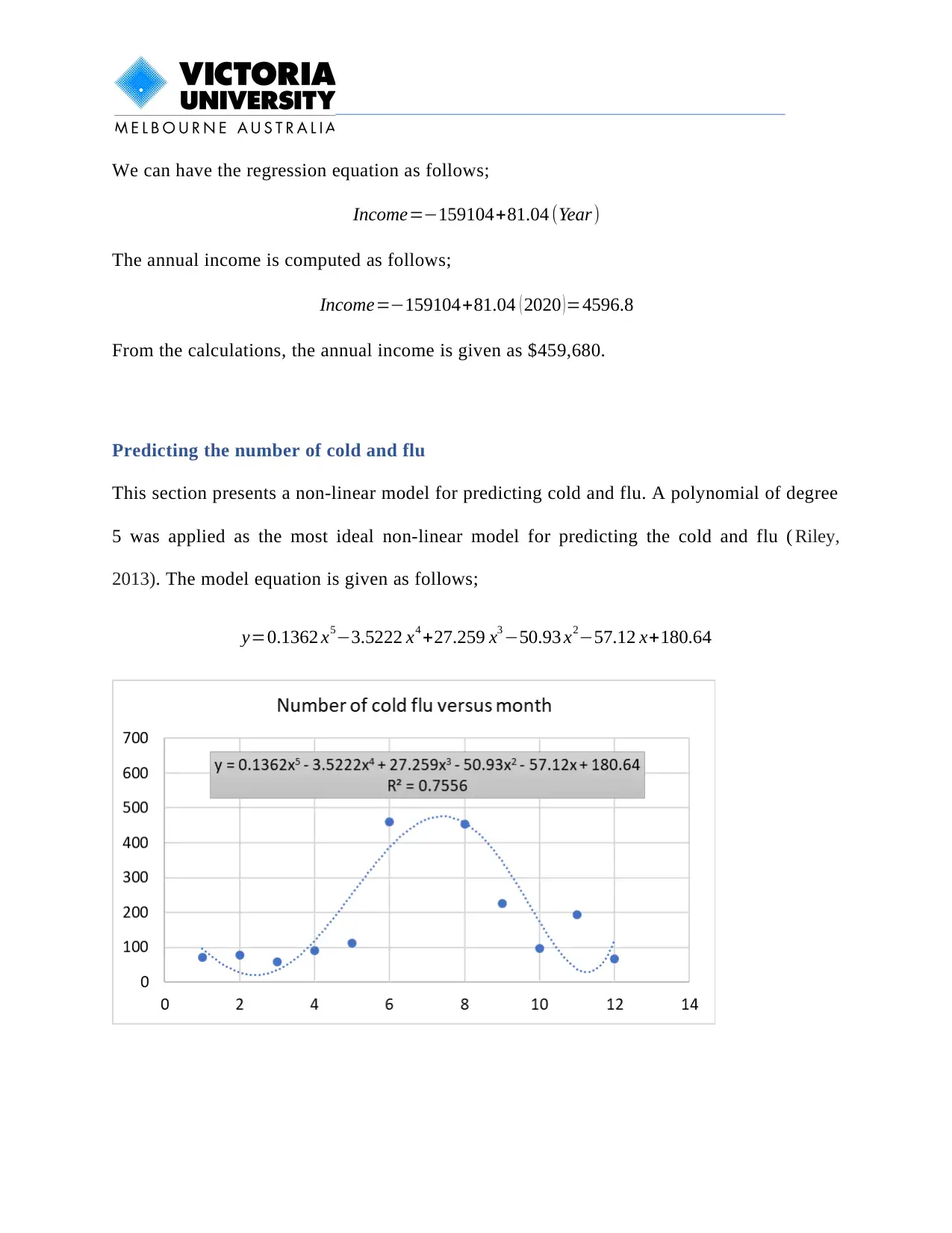
We can have the regression equation as follows;
Income=−159104+81.04 (Year)
The annual income is computed as follows;
Income=−159104+81.04 ( 2020 )=4596.8
From the calculations, the annual income is given as $459,680.
Predicting the number of cold and flu
This section presents a non-linear model for predicting cold and flu. A polynomial of degree
5 was applied as the most ideal non-linear model for predicting the cold and flu ( Riley,
2013). The model equation is given as follows;
y=0.1362 x5−3.5222 x4 +27.259 x3 −50.93 x2−57.12 x+180.64
Income=−159104+81.04 (Year)
The annual income is computed as follows;
Income=−159104+81.04 ( 2020 )=4596.8
From the calculations, the annual income is given as $459,680.
Predicting the number of cold and flu
This section presents a non-linear model for predicting cold and flu. A polynomial of degree
5 was applied as the most ideal non-linear model for predicting the cold and flu ( Riley,
2013). The model equation is given as follows;
y=0.1362 x5−3.5222 x4 +27.259 x3 −50.93 x2−57.12 x+180.64
⊘ This is a preview!⊘
Do you want full access?
Subscribe today to unlock all pages.

Trusted by 1+ million students worldwide
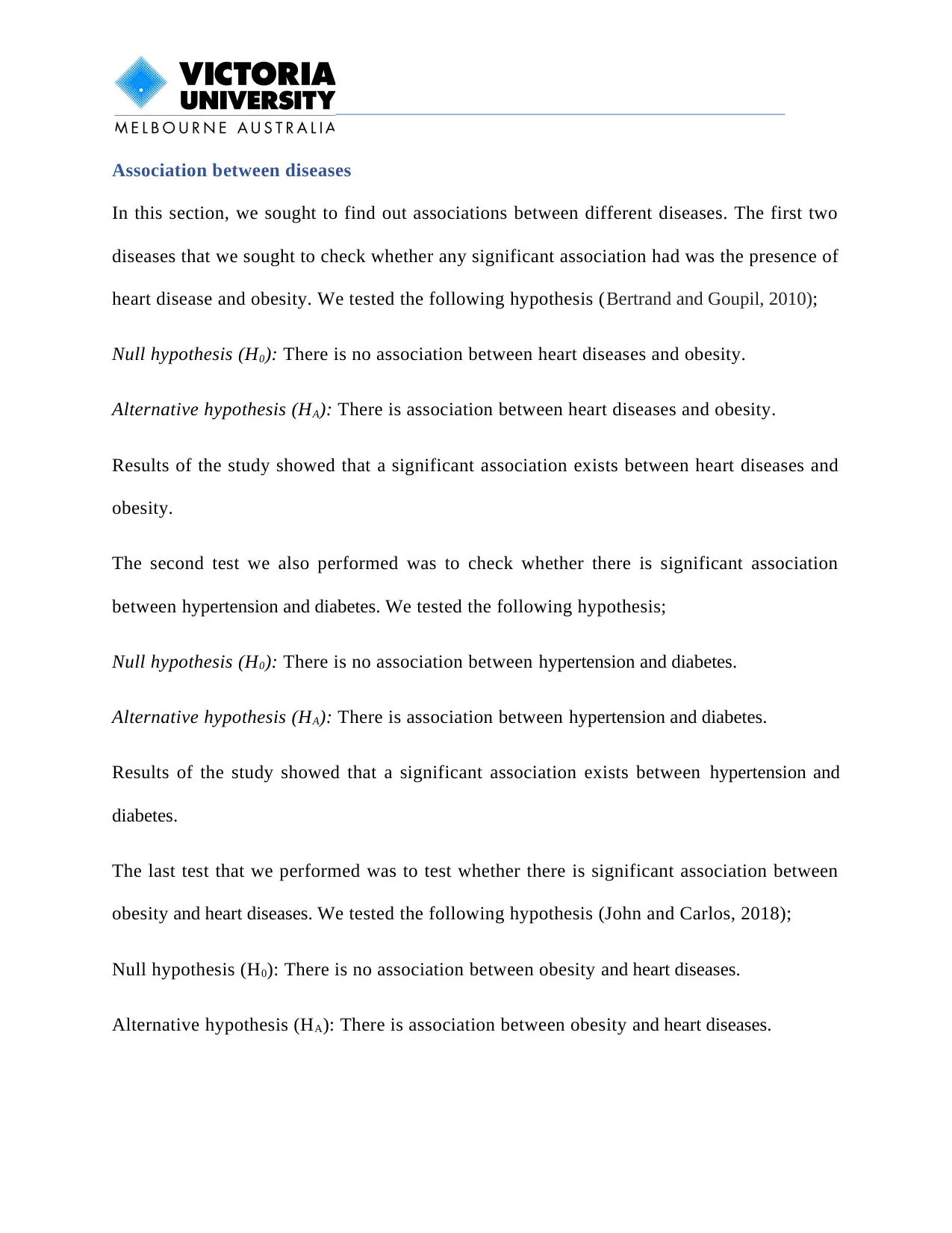
Association between diseases
In this section, we sought to find out associations between different diseases. The first two
diseases that we sought to check whether any significant association had was the presence of
heart disease and obesity. We tested the following hypothesis (Bertrand and Goupil, 2010);
Null hypothesis (H0): There is no association between heart diseases and obesity.
Alternative hypothesis (HA): There is association between heart diseases and obesity.
Results of the study showed that a significant association exists between heart diseases and
obesity.
The second test we also performed was to check whether there is significant association
between hypertension and diabetes. We tested the following hypothesis;
Null hypothesis (H0): There is no association between hypertension and diabetes.
Alternative hypothesis (HA): There is association between hypertension and diabetes.
Results of the study showed that a significant association exists between hypertension and
diabetes.
The last test that we performed was to test whether there is significant association between
obesity and heart diseases. We tested the following hypothesis (John and Carlos, 2018);
Null hypothesis (H0): There is no association between obesity and heart diseases.
Alternative hypothesis (HA): There is association between obesity and heart diseases.
In this section, we sought to find out associations between different diseases. The first two
diseases that we sought to check whether any significant association had was the presence of
heart disease and obesity. We tested the following hypothesis (Bertrand and Goupil, 2010);
Null hypothesis (H0): There is no association between heart diseases and obesity.
Alternative hypothesis (HA): There is association between heart diseases and obesity.
Results of the study showed that a significant association exists between heart diseases and
obesity.
The second test we also performed was to check whether there is significant association
between hypertension and diabetes. We tested the following hypothesis;
Null hypothesis (H0): There is no association between hypertension and diabetes.
Alternative hypothesis (HA): There is association between hypertension and diabetes.
Results of the study showed that a significant association exists between hypertension and
diabetes.
The last test that we performed was to test whether there is significant association between
obesity and heart diseases. We tested the following hypothesis (John and Carlos, 2018);
Null hypothesis (H0): There is no association between obesity and heart diseases.
Alternative hypothesis (HA): There is association between obesity and heart diseases.
Paraphrase This Document
Need a fresh take? Get an instant paraphrase of this document with our AI Paraphraser
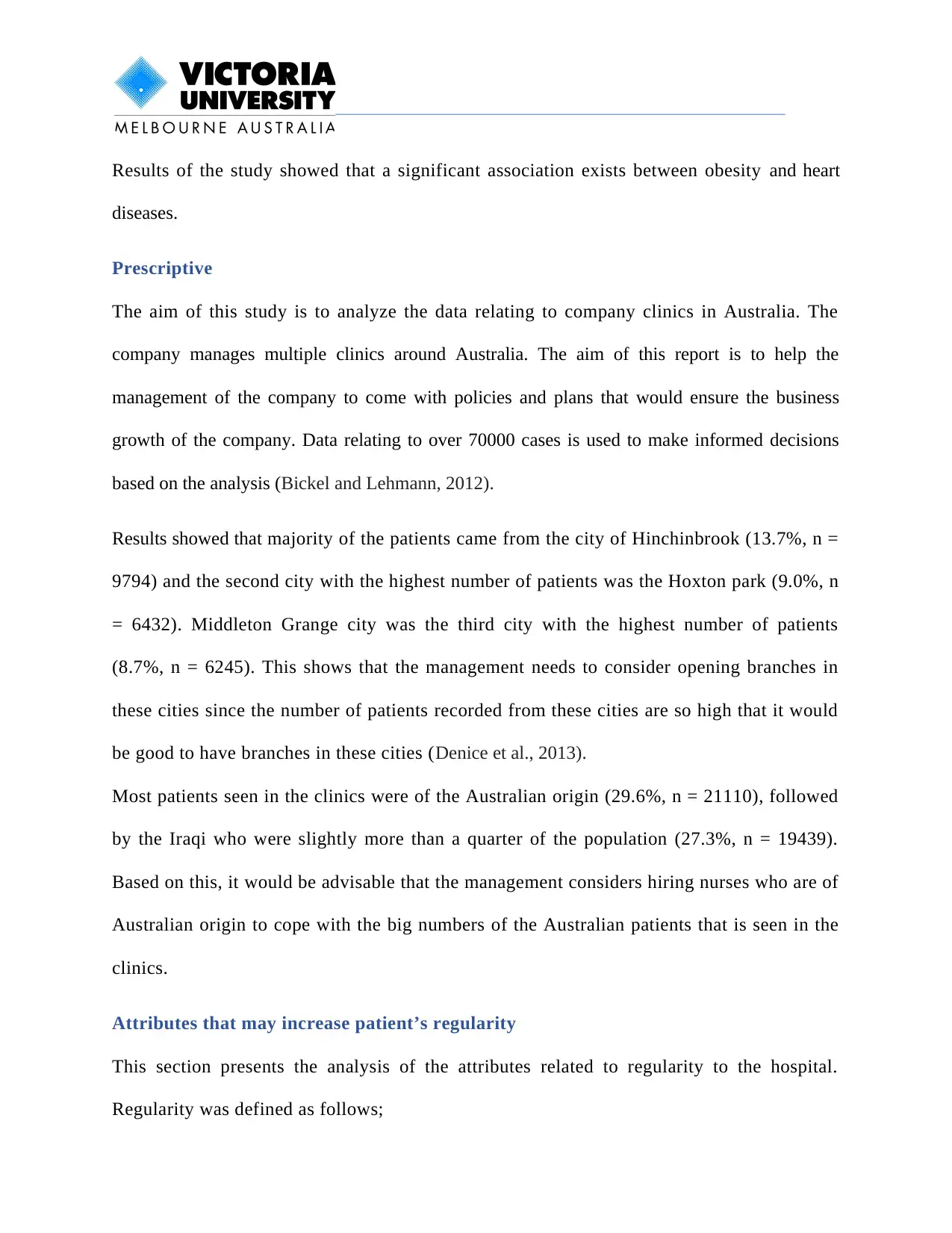
Results of the study showed that a significant association exists between obesity and heart
diseases.
Prescriptive
The aim of this study is to analyze the data relating to company clinics in Australia. The
company manages multiple clinics around Australia. The aim of this report is to help the
management of the company to come with policies and plans that would ensure the business
growth of the company. Data relating to over 70000 cases is used to make informed decisions
based on the analysis (Bickel and Lehmann, 2012).
Results showed that majority of the patients came from the city of Hinchinbrook (13.7%, n =
9794) and the second city with the highest number of patients was the Hoxton park (9.0%, n
= 6432). Middleton Grange city was the third city with the highest number of patients
(8.7%, n = 6245). This shows that the management needs to consider opening branches in
these cities since the number of patients recorded from these cities are so high that it would
be good to have branches in these cities (Denice et al., 2013).
Most patients seen in the clinics were of the Australian origin (29.6%, n = 21110), followed
by the Iraqi who were slightly more than a quarter of the population (27.3%, n = 19439).
Based on this, it would be advisable that the management considers hiring nurses who are of
Australian origin to cope with the big numbers of the Australian patients that is seen in the
clinics.
Attributes that may increase patient’s regularity
This section presents the analysis of the attributes related to regularity to the hospital.
Regularity was defined as follows;
diseases.
Prescriptive
The aim of this study is to analyze the data relating to company clinics in Australia. The
company manages multiple clinics around Australia. The aim of this report is to help the
management of the company to come with policies and plans that would ensure the business
growth of the company. Data relating to over 70000 cases is used to make informed decisions
based on the analysis (Bickel and Lehmann, 2012).
Results showed that majority of the patients came from the city of Hinchinbrook (13.7%, n =
9794) and the second city with the highest number of patients was the Hoxton park (9.0%, n
= 6432). Middleton Grange city was the third city with the highest number of patients
(8.7%, n = 6245). This shows that the management needs to consider opening branches in
these cities since the number of patients recorded from these cities are so high that it would
be good to have branches in these cities (Denice et al., 2013).
Most patients seen in the clinics were of the Australian origin (29.6%, n = 21110), followed
by the Iraqi who were slightly more than a quarter of the population (27.3%, n = 19439).
Based on this, it would be advisable that the management considers hiring nurses who are of
Australian origin to cope with the big numbers of the Australian patients that is seen in the
clinics.
Attributes that may increase patient’s regularity
This section presents the analysis of the attributes related to regularity to the hospital.
Regularity was defined as follows;
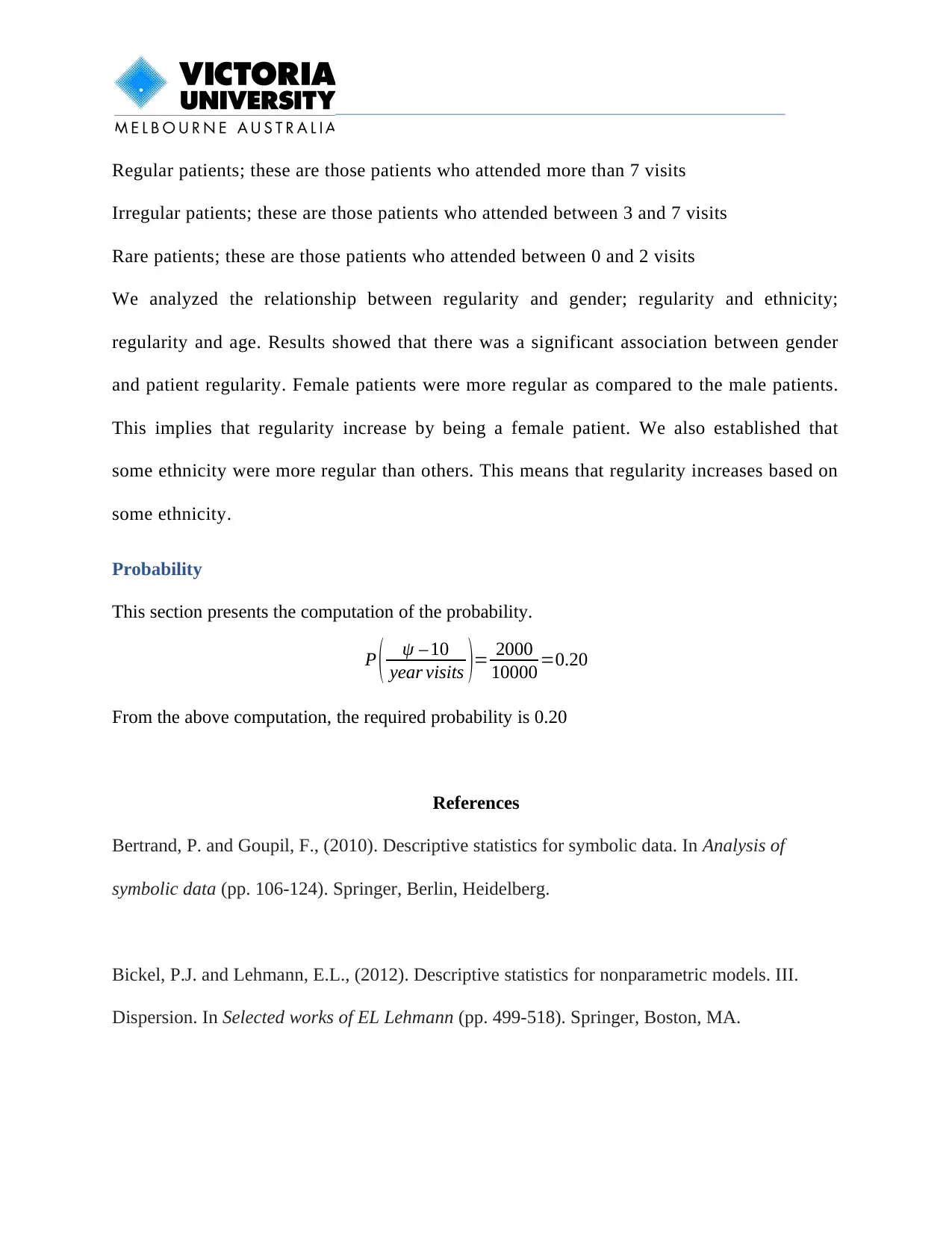
Regular patients; these are those patients who attended more than 7 visits
Irregular patients; these are those patients who attended between 3 and 7 visits
Rare patients; these are those patients who attended between 0 and 2 visits
We analyzed the relationship between regularity and gender; regularity and ethnicity;
regularity and age. Results showed that there was a significant association between gender
and patient regularity. Female patients were more regular as compared to the male patients.
This implies that regularity increase by being a female patient. We also established that
some ethnicity were more regular than others. This means that regularity increases based on
some ethnicity.
Probability
This section presents the computation of the probability.
P ( ψ – 10
year visits )= 2000
10000 =0.20
From the above computation, the required probability is 0.20
References
Bertrand, P. and Goupil, F., (2010). Descriptive statistics for symbolic data. In Analysis of
symbolic data (pp. 106-124). Springer, Berlin, Heidelberg.
Bickel, P.J. and Lehmann, E.L., (2012). Descriptive statistics for nonparametric models. III.
Dispersion. In Selected works of EL Lehmann (pp. 499-518). Springer, Boston, MA.
Irregular patients; these are those patients who attended between 3 and 7 visits
Rare patients; these are those patients who attended between 0 and 2 visits
We analyzed the relationship between regularity and gender; regularity and ethnicity;
regularity and age. Results showed that there was a significant association between gender
and patient regularity. Female patients were more regular as compared to the male patients.
This implies that regularity increase by being a female patient. We also established that
some ethnicity were more regular than others. This means that regularity increases based on
some ethnicity.
Probability
This section presents the computation of the probability.
P ( ψ – 10
year visits )= 2000
10000 =0.20
From the above computation, the required probability is 0.20
References
Bertrand, P. and Goupil, F., (2010). Descriptive statistics for symbolic data. In Analysis of
symbolic data (pp. 106-124). Springer, Berlin, Heidelberg.
Bickel, P.J. and Lehmann, E.L., (2012). Descriptive statistics for nonparametric models. III.
Dispersion. In Selected works of EL Lehmann (pp. 499-518). Springer, Boston, MA.
⊘ This is a preview!⊘
Do you want full access?
Subscribe today to unlock all pages.

Trusted by 1+ million students worldwide
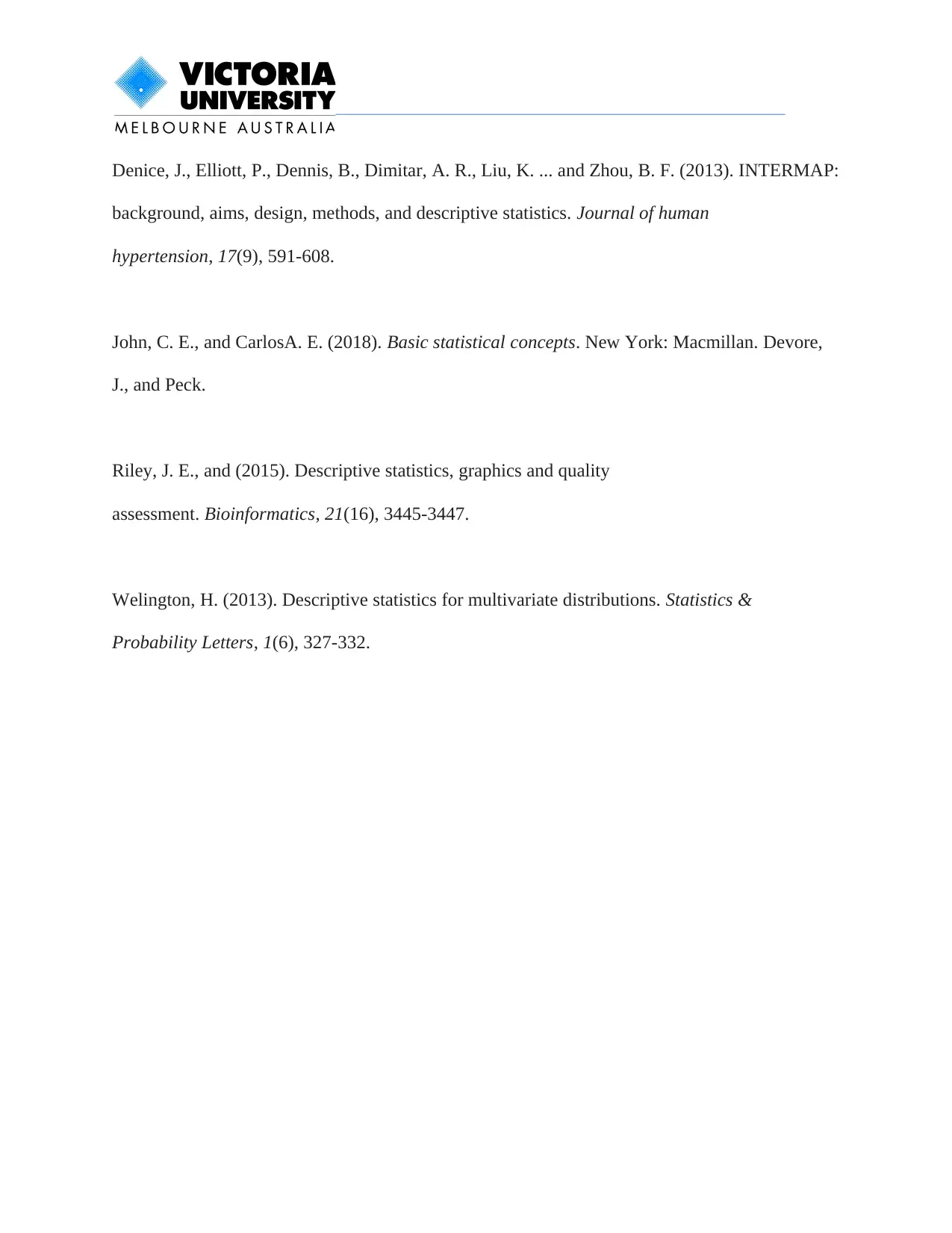
Denice, J., Elliott, P., Dennis, B., Dimitar, A. R., Liu, K. ... and Zhou, B. F. (2013). INTERMAP:
background, aims, design, methods, and descriptive statistics. Journal of human
hypertension, 17(9), 591-608.
John, C. E., and CarlosA. E. (2018). Basic statistical concepts. New York: Macmillan. Devore,
J., and Peck.
Riley, J. E., and (2015). Descriptive statistics, graphics and quality
assessment. Bioinformatics, 21(16), 3445-3447.
Welington, H. (2013). Descriptive statistics for multivariate distributions. Statistics &
Probability Letters, 1(6), 327-332.
background, aims, design, methods, and descriptive statistics. Journal of human
hypertension, 17(9), 591-608.
John, C. E., and CarlosA. E. (2018). Basic statistical concepts. New York: Macmillan. Devore,
J., and Peck.
Riley, J. E., and (2015). Descriptive statistics, graphics and quality
assessment. Bioinformatics, 21(16), 3445-3447.
Welington, H. (2013). Descriptive statistics for multivariate distributions. Statistics &
Probability Letters, 1(6), 327-332.
1 out of 16
Related Documents

Your All-in-One AI-Powered Toolkit for Academic Success.
+13062052269
info@desklib.com
Available 24*7 on WhatsApp / Email
Unlock your academic potential
© 2024 | Zucol Services PVT LTD | All rights reserved.