Computers And Education Assignment Report
Added on 2022-09-09
24 Pages26253 Words22 Views
The effects of integrating mobile devices with teaching and
learning on students' learning performance: A meta-analysis
and research synthesis
Yao-Ting Sung a
, Kuo-En Chang b
, Tzu-Chien Liu a, *
a Department of Educational Psychology and Counseling, National Taiwan Normal University, Taiwan, ROC
b Grad. Institute of Information and Computer Education, National Taiwan Normal University, Taiwan, ROC
a r t i c l e i n f o
Article history:
Received 12 August 2015
Received in revised form 17 November 2015
Accepted 19 November 2015
Available online 23 November 2015
Keywords:
Evaluation methodologies
Pedagogical issues
Teaching/learning strategies
a b s t r a c t
Mobile devices such as laptops, personal digital assistants, and mobile phones have
become a learning tool with great potential in both classrooms and outdoor learning.
Although there have been qualitative analyses of the use of mobile devices in education,
systematic quantitative analyses of the effects of mobile-integrated education are lacking.
This study performed a meta-analysis and research synthesis of the effects of integrated
mobile devices in teaching and learning, in which 110 experimental and quasiexperimental
journal articles published during the period 1993e2013 were coded and analyzed. Overall,
there was a moderate mean effect size of 0.523 for the application of mobile devices to
education. The effect sizes of moderator variables were analyzed and the advantages and
disadvantages of mobile learning in different levels of moderator variables were synthe-
sized based on content analyses of individual studies. The results of this study and their
implications for both research and practice are discussed.
© 2015 The Authors. Published by Elsevier Ltd. This is an open access article under the CC
BY-NC-ND license (http://creativecommons.org/licenses/by-nc-nd/4.0/).
1. Introduction
1.1. Integrating mobile devices with learning and instruction
Mobile computers have gradually been introduced into educational contexts over the past 2 decades. Mobile technology
has led to most people to carry their own individual small computers that contain exceptional computing power, such as
laptops, personal digital assistants (PDAs), tablet personal computers (PCs), cell phones, and e-book readers. This large
amount of computing power and portability, combined with the wireless communication and context sensitivity tools, makes
one-to-one computing a learning tool of great potential in both traditional classrooms and outdoor informal learning.
With regard to access to computers, large-scale one-to-one computing programs have been implemented in many
countries globally (Bebell & O'Dwyer, 2010; Fleischer, 2012; Zucker & Light, 2009), such that elementary- and middle-school
students and their teachers have their own mobile devices. In addition, in terms of promoting innovation in education via
information technology, not only does mobile computing support traditional lecture-style teaching, but through convenient
* Corresponding author. Department of Educational Psychology and Counseling, National Taiwan Normal University, 162, HePing East Road, Section 1,
Taipei, Taiwan, ROC.
E-mail addresses: sungtc@ntnu.edu.tw (Y.-T. Sung), kchang@ntnu.edu.tw (K.-E. Chang), tzuchien@ntnu.edu.tw (T.-C. Liu).
Contents lists available at ScienceDirect
Computers & Education
j o u r n a l h o m e p a g e : w w w . e l s e v i e r . c o m / l o c a t e / c o m p e d u
http://dx.doi.org/10.1016/j.compedu.2015.11.008
0360-1315/© 2015 The Authors. Published by Elsevier Ltd. This is an open access article under the CC BY-NC-ND license (http://creativecommons.org/
licenses/by-nc-nd/4.0/).
Computers & Education 94 (2016) 252e275
learning on students' learning performance: A meta-analysis
and research synthesis
Yao-Ting Sung a
, Kuo-En Chang b
, Tzu-Chien Liu a, *
a Department of Educational Psychology and Counseling, National Taiwan Normal University, Taiwan, ROC
b Grad. Institute of Information and Computer Education, National Taiwan Normal University, Taiwan, ROC
a r t i c l e i n f o
Article history:
Received 12 August 2015
Received in revised form 17 November 2015
Accepted 19 November 2015
Available online 23 November 2015
Keywords:
Evaluation methodologies
Pedagogical issues
Teaching/learning strategies
a b s t r a c t
Mobile devices such as laptops, personal digital assistants, and mobile phones have
become a learning tool with great potential in both classrooms and outdoor learning.
Although there have been qualitative analyses of the use of mobile devices in education,
systematic quantitative analyses of the effects of mobile-integrated education are lacking.
This study performed a meta-analysis and research synthesis of the effects of integrated
mobile devices in teaching and learning, in which 110 experimental and quasiexperimental
journal articles published during the period 1993e2013 were coded and analyzed. Overall,
there was a moderate mean effect size of 0.523 for the application of mobile devices to
education. The effect sizes of moderator variables were analyzed and the advantages and
disadvantages of mobile learning in different levels of moderator variables were synthe-
sized based on content analyses of individual studies. The results of this study and their
implications for both research and practice are discussed.
© 2015 The Authors. Published by Elsevier Ltd. This is an open access article under the CC
BY-NC-ND license (http://creativecommons.org/licenses/by-nc-nd/4.0/).
1. Introduction
1.1. Integrating mobile devices with learning and instruction
Mobile computers have gradually been introduced into educational contexts over the past 2 decades. Mobile technology
has led to most people to carry their own individual small computers that contain exceptional computing power, such as
laptops, personal digital assistants (PDAs), tablet personal computers (PCs), cell phones, and e-book readers. This large
amount of computing power and portability, combined with the wireless communication and context sensitivity tools, makes
one-to-one computing a learning tool of great potential in both traditional classrooms and outdoor informal learning.
With regard to access to computers, large-scale one-to-one computing programs have been implemented in many
countries globally (Bebell & O'Dwyer, 2010; Fleischer, 2012; Zucker & Light, 2009), such that elementary- and middle-school
students and their teachers have their own mobile devices. In addition, in terms of promoting innovation in education via
information technology, not only does mobile computing support traditional lecture-style teaching, but through convenient
* Corresponding author. Department of Educational Psychology and Counseling, National Taiwan Normal University, 162, HePing East Road, Section 1,
Taipei, Taiwan, ROC.
E-mail addresses: sungtc@ntnu.edu.tw (Y.-T. Sung), kchang@ntnu.edu.tw (K.-E. Chang), tzuchien@ntnu.edu.tw (T.-C. Liu).
Contents lists available at ScienceDirect
Computers & Education
j o u r n a l h o m e p a g e : w w w . e l s e v i e r . c o m / l o c a t e / c o m p e d u
http://dx.doi.org/10.1016/j.compedu.2015.11.008
0360-1315/© 2015 The Authors. Published by Elsevier Ltd. This is an open access article under the CC BY-NC-ND license (http://creativecommons.org/
licenses/by-nc-nd/4.0/).
Computers & Education 94 (2016) 252e275
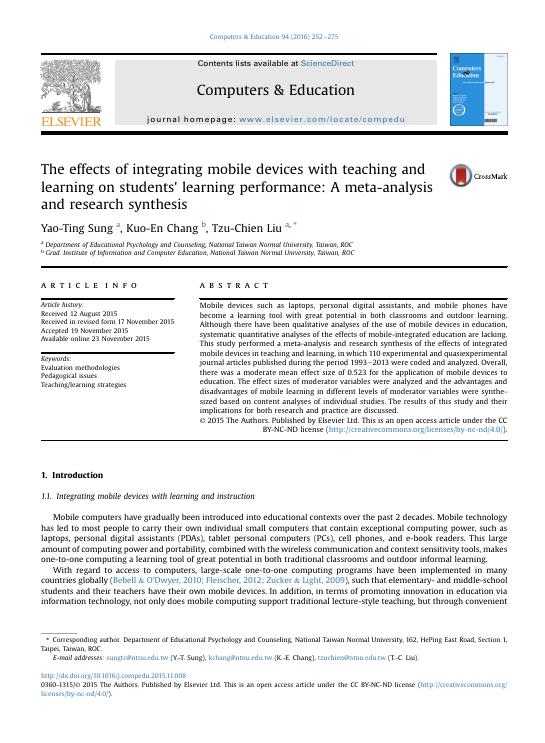
information gathering and sharing it can also promote innovative teaching methods such as cooperative learning (Lan, Sung,
& Chang, 2007; Roschelle et al., 2010), exploratory learning outside the classroom (Liu, Lin, Tsai, & Paas, 2012), and game-
based learning (Klopfer, Sheldon, Perry, & Chen, 2012). Therefore, mobile technologies have great potential for facilitating
more innovative educational methods. Simultaneously, these patterns in educational methods will likely not only help
subject content learning, but may also facilitate the development of communication, problem-solving, creativity, and other
high-level skills among students (Warschauer, 2007).
However, despite the proposed advantages of using mobile computing devices for increasing computer accessibility,
diverse teaching styles, and academic performance, currently researchers found mixed results regarding the effects of mobile-
devices (e.g., Warschauer, Zheng, Niiya, Cotten, & Farkas, 2014), and very few studies have addressed how best to use mobile
devices, and the effectiveness of doing so.
1.2. Review of the research into integrating mobile devices with teaching and learning
There are seven studies which reviewed the research into integrating mobile devices with teaching and learning and can
be divided into two types according to the devices they focused on: (1) those focused on how laptops are used in schools and
(2) those focused on the applications of various types of mobile device in education (see Appendix A).
Regarding the review of laptop-based programs, Zucker and Light (2009) believed that school programs integrating
laptops into schools have a positive impact on student learning. However, they also believed that laptop use did not achieve
the goals of increasing higher-level thinking and transformation of classroom teaching methods. Penuel (2006) reviewed
30 studies that examined the usage of laptops with wireless connectivity in one-to-one computer programs. Those studies
found that students most often used the laptops to do homework, take notes, and finish assignments. General-purpose
software such as word processors, web browsers, and presentation software were relatively common. Bebell and
O'Dwyer (2010) examined four different empirical studies of laptop programs in schools. They discovered that in most
schools participating in one-to-one programs there were significant increases in grade-point averages or standardized tests
of student achievement, relative to schools that did not provide such programs. In addition, they found that most students
used their laptops to write, browse the Internet, make presentations, do homework, or take tests. Furthermore, teachers
made more changes to their teaching methods when they had increased opportunities to use laptops. Students partici-
pating in one-to-one programs also had a deeper engagement with what they were learning when compared to control
groups.
Fleischer (2012) conducted a narrative research review of 18 different empirical studies on the usage of laptops. These
studies found a large range in the number of hours that students used laptops, from a few days to as little as 1 h per week. The
most frequently used computer functions were searches, followed by expression and communication. In most studies it was
found that students had a positive attitude toward laptops, and felt that they were more motivated and engaged in their
learning, and it was further believed that teachers conducted more student-centered learning activities. Moreover, consid-
erable differences in classroom educational practices arose from the diversity of teachers' beliefs about the usefulness of
laptops. Fleischer (2012) also found several challenges regarding the use of laptops in classrooms, such as encouraging
teachers to change their previous beliefs and teaching methods (e.g., teacher-centered lectures) in response to their students'
greater flexibility and autonomy; how to reconcile the conflict between the students' desire for independent study and the
need for teachers' guidance; and how to facilitate teachers' competence by designing an appropriate curriculum and teaching
models for laptop usage programs.
With respect to the research on the use of mobile technology in education, Hwang and Tsai (2011) provided a broad
discussion of studies on mobile and ubiquitous learning published in six journals between 2001 and 2010. In their review of
154 articles, they discovered that the use of mobile and ubiquitous learning accelerated markedly during 2008; researchers
mostly studied students of higher education, and the fields most often researched were language arts, engineering, and
computer technology. Frohberg, Goth, and Schwabe (2009) categorized 102 mobile-learning projects, and discovered that
most mobile-learning activities occurred across different settings, and took place within a physical context and an official
environment, such as a classroom or workplace. Regarding the pedagogical roles that mobile devices play in education, most
research has used mobile devices primarily as a sort of reinforcement tool to stimulate motivation and strengthen engage-
ment, and secondarily as a content-delivery tool. Few projects have used mobile devices to assist with constructive thinking
or reflection. Furthermore, most learning activities using mobile devices have been controlled by the teacher, with there being
only a handful of learner-centered projects in existence. Concerning the communication functions, very few projects have
made any use of cooperative or team communication. Moreover, the vast majority of studies have made use of novice par-
ticipants; little research has involved experienced participants. When sorted according to educational goals, it was found that
the vast majority of research has focused on lower-level knowledge and skills, and ignored higher-level tasks such as analysis
and evaluation. Wong and Looi (2011) investigated the influence of mobile devices on seamless learning. Seamless learning
refers to a learning model that students can learn whenever they want to learn in a variety of scenarios and that they can
switch from one scenario or one context to another easily and quickly (Chan et al., 2006; Wong & Looi, 2011). Wong and Looi
(2011) selected and analyzed a sample of 54 articles on the use of mobile devices to facilitate seamless learning, and found
that all 54 articles contained 10 features, including formal and informal learning, personalized and social learning, and
learning across multiple durations and locations.
Y.-T. Sung et al. / Computers & Education 94 (2016) 252e275 253
& Chang, 2007; Roschelle et al., 2010), exploratory learning outside the classroom (Liu, Lin, Tsai, & Paas, 2012), and game-
based learning (Klopfer, Sheldon, Perry, & Chen, 2012). Therefore, mobile technologies have great potential for facilitating
more innovative educational methods. Simultaneously, these patterns in educational methods will likely not only help
subject content learning, but may also facilitate the development of communication, problem-solving, creativity, and other
high-level skills among students (Warschauer, 2007).
However, despite the proposed advantages of using mobile computing devices for increasing computer accessibility,
diverse teaching styles, and academic performance, currently researchers found mixed results regarding the effects of mobile-
devices (e.g., Warschauer, Zheng, Niiya, Cotten, & Farkas, 2014), and very few studies have addressed how best to use mobile
devices, and the effectiveness of doing so.
1.2. Review of the research into integrating mobile devices with teaching and learning
There are seven studies which reviewed the research into integrating mobile devices with teaching and learning and can
be divided into two types according to the devices they focused on: (1) those focused on how laptops are used in schools and
(2) those focused on the applications of various types of mobile device in education (see Appendix A).
Regarding the review of laptop-based programs, Zucker and Light (2009) believed that school programs integrating
laptops into schools have a positive impact on student learning. However, they also believed that laptop use did not achieve
the goals of increasing higher-level thinking and transformation of classroom teaching methods. Penuel (2006) reviewed
30 studies that examined the usage of laptops with wireless connectivity in one-to-one computer programs. Those studies
found that students most often used the laptops to do homework, take notes, and finish assignments. General-purpose
software such as word processors, web browsers, and presentation software were relatively common. Bebell and
O'Dwyer (2010) examined four different empirical studies of laptop programs in schools. They discovered that in most
schools participating in one-to-one programs there were significant increases in grade-point averages or standardized tests
of student achievement, relative to schools that did not provide such programs. In addition, they found that most students
used their laptops to write, browse the Internet, make presentations, do homework, or take tests. Furthermore, teachers
made more changes to their teaching methods when they had increased opportunities to use laptops. Students partici-
pating in one-to-one programs also had a deeper engagement with what they were learning when compared to control
groups.
Fleischer (2012) conducted a narrative research review of 18 different empirical studies on the usage of laptops. These
studies found a large range in the number of hours that students used laptops, from a few days to as little as 1 h per week. The
most frequently used computer functions were searches, followed by expression and communication. In most studies it was
found that students had a positive attitude toward laptops, and felt that they were more motivated and engaged in their
learning, and it was further believed that teachers conducted more student-centered learning activities. Moreover, consid-
erable differences in classroom educational practices arose from the diversity of teachers' beliefs about the usefulness of
laptops. Fleischer (2012) also found several challenges regarding the use of laptops in classrooms, such as encouraging
teachers to change their previous beliefs and teaching methods (e.g., teacher-centered lectures) in response to their students'
greater flexibility and autonomy; how to reconcile the conflict between the students' desire for independent study and the
need for teachers' guidance; and how to facilitate teachers' competence by designing an appropriate curriculum and teaching
models for laptop usage programs.
With respect to the research on the use of mobile technology in education, Hwang and Tsai (2011) provided a broad
discussion of studies on mobile and ubiquitous learning published in six journals between 2001 and 2010. In their review of
154 articles, they discovered that the use of mobile and ubiquitous learning accelerated markedly during 2008; researchers
mostly studied students of higher education, and the fields most often researched were language arts, engineering, and
computer technology. Frohberg, Goth, and Schwabe (2009) categorized 102 mobile-learning projects, and discovered that
most mobile-learning activities occurred across different settings, and took place within a physical context and an official
environment, such as a classroom or workplace. Regarding the pedagogical roles that mobile devices play in education, most
research has used mobile devices primarily as a sort of reinforcement tool to stimulate motivation and strengthen engage-
ment, and secondarily as a content-delivery tool. Few projects have used mobile devices to assist with constructive thinking
or reflection. Furthermore, most learning activities using mobile devices have been controlled by the teacher, with there being
only a handful of learner-centered projects in existence. Concerning the communication functions, very few projects have
made any use of cooperative or team communication. Moreover, the vast majority of studies have made use of novice par-
ticipants; little research has involved experienced participants. When sorted according to educational goals, it was found that
the vast majority of research has focused on lower-level knowledge and skills, and ignored higher-level tasks such as analysis
and evaluation. Wong and Looi (2011) investigated the influence of mobile devices on seamless learning. Seamless learning
refers to a learning model that students can learn whenever they want to learn in a variety of scenarios and that they can
switch from one scenario or one context to another easily and quickly (Chan et al., 2006; Wong & Looi, 2011). Wong and Looi
(2011) selected and analyzed a sample of 54 articles on the use of mobile devices to facilitate seamless learning, and found
that all 54 articles contained 10 features, including formal and informal learning, personalized and social learning, and
learning across multiple durations and locations.
Y.-T. Sung et al. / Computers & Education 94 (2016) 252e275 253
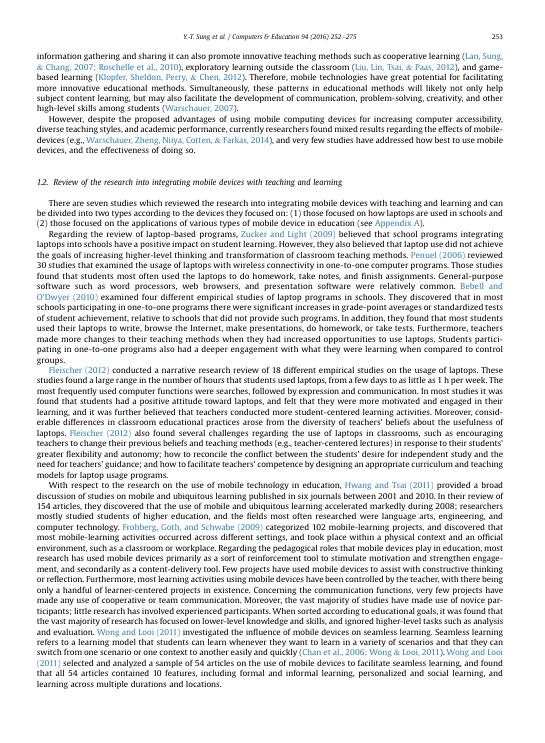
1.3. Purposes of this study
While analyzing the overall effectiveness of using mobile devices in education, the review research described above has
two major limitations. First, all of the reviews adopted a qualitative approach, which may be able to describe and summarize
how related studies were conducted and the problems encountered during their execution, but this makes it difficult to
evaluate the effects actually produced by the mobile devices in general and the specific moderator variables. Second, much of
the previous review research has focused on the usage of laptop computers as the subject of their investigation (e.g., Penuel,
2006), and most of the research participants in those reviewed articles were in primary and secondary schools. However, the
many new developments in mobile hardware have meant that diverse age groups now use different devices. Therefore, many
different moderators need to be accounted for when attempting to determine whether or not intervening variables have an
effect.
In the context of this background, the primary goal of this study was to perform a meta-analysis and research synthesis of
the research on the usage of mobile devices in education published in the last 2 decades. Specifically, the purposes of this
study were as follows:
1. To provide an overview of the status of the use of mobile devices in educational experimental studies, including who is
using them, which domain subjects are being taught, what kinds of mobile device and software are being used, where such
programs take place, how the devices are used in teaching, and the duration of the interventions.
2. To quantify the overall effectiveness of integrating mobile technologies into education on student learning achievement.
3. To determine how the moderator variables influence the effects of mobile devices on learning achievement.
4. To synthesize the advantages and disadvantages of mobile learning in levels of moderator variables based on the content
analysis of articles related with moderator variables.
2. Method
2.1. Data sources and search strategy
Journal articles published during the period 1993e2013 were searched electronically and manually, and via reference-list
checking to retrieve the relevant literature. For electronic searches, the main databases were the Education Resources In-
formation Center (ERIC) and the Social Sciences Citation Index database of the Institute of Science Index (ISI). Two sets of
keywords were searched: (1) mobile-device related keywords, including mobile, wireless, ubiquitous, wearable, portable,
handheld, cell phone, personal digital assistant, PDA, palmtop, pad, web pad, tablet PC, tablet computer, laptop, e-book, digital
pen, pocket dictionary, and classroom response system; and (2) learning-related keywords, including teaching, learning,
training, and lectures. The two sets of keywords were combined when searching the electronic databases. Manual searches
included the major journals in educational technology and e-learning, such as the Australian Journal of Educational Tech-
nology, British Journal of Educational Technology, Computers & Education, Computer Assisted Language Learning, Educa-
tional Technology Research and Development, Journal of Computer Assisted Learning, Language Learning & Technology, and
ReCall.
After collating all of the related literature, another round of searches was conducted using the reference lists found in the
literature yielded by the electronic search to find any omitted but relevant works.
2.2. Search results
2.2.1. Initial screening
The initial search yielded 4121 abstracts published between 1993 and 2013 (1718 in ERIC and 2403 in ISI) that were related
to mobile learning. Two authors read each abstract of the article and judged whether or not the article was related to teaching
and learning with a mobile device, which resulted in the selection of 925 articles.
2.2.2. Screening for experimental and quasiexperimental research
In the second stage, the studies were screened according to the research method. Experimental studies (including the
pretest-posttest equivalent-group, posttest-only equivalent-groups, and randomized matched subjects and posttest-only
control-group designs) and quasi-experimental studies (including the pretest-posttest nonequivalent-groups and counter-
balanced designs; see Ary, Jacobs, & Razavieh, 2002; Best & Kahn, 1998, for a reference) were included. Conceptual analysis or
research reviews, case studies and qualitative research, survey research, and pre-experimental studies were all excluded at
this stage. At the completion of this stage there remained 182 articles.
2.2.3. Application of inclusion and exclusion criteria for the meta-analysis
Studies were eligible for inclusion in the meta-analysis if they conformed with the following three criteria:
Y.-T. Sung et al. / Computers & Education 94 (2016) 252e275254
While analyzing the overall effectiveness of using mobile devices in education, the review research described above has
two major limitations. First, all of the reviews adopted a qualitative approach, which may be able to describe and summarize
how related studies were conducted and the problems encountered during their execution, but this makes it difficult to
evaluate the effects actually produced by the mobile devices in general and the specific moderator variables. Second, much of
the previous review research has focused on the usage of laptop computers as the subject of their investigation (e.g., Penuel,
2006), and most of the research participants in those reviewed articles were in primary and secondary schools. However, the
many new developments in mobile hardware have meant that diverse age groups now use different devices. Therefore, many
different moderators need to be accounted for when attempting to determine whether or not intervening variables have an
effect.
In the context of this background, the primary goal of this study was to perform a meta-analysis and research synthesis of
the research on the usage of mobile devices in education published in the last 2 decades. Specifically, the purposes of this
study were as follows:
1. To provide an overview of the status of the use of mobile devices in educational experimental studies, including who is
using them, which domain subjects are being taught, what kinds of mobile device and software are being used, where such
programs take place, how the devices are used in teaching, and the duration of the interventions.
2. To quantify the overall effectiveness of integrating mobile technologies into education on student learning achievement.
3. To determine how the moderator variables influence the effects of mobile devices on learning achievement.
4. To synthesize the advantages and disadvantages of mobile learning in levels of moderator variables based on the content
analysis of articles related with moderator variables.
2. Method
2.1. Data sources and search strategy
Journal articles published during the period 1993e2013 were searched electronically and manually, and via reference-list
checking to retrieve the relevant literature. For electronic searches, the main databases were the Education Resources In-
formation Center (ERIC) and the Social Sciences Citation Index database of the Institute of Science Index (ISI). Two sets of
keywords were searched: (1) mobile-device related keywords, including mobile, wireless, ubiquitous, wearable, portable,
handheld, cell phone, personal digital assistant, PDA, palmtop, pad, web pad, tablet PC, tablet computer, laptop, e-book, digital
pen, pocket dictionary, and classroom response system; and (2) learning-related keywords, including teaching, learning,
training, and lectures. The two sets of keywords were combined when searching the electronic databases. Manual searches
included the major journals in educational technology and e-learning, such as the Australian Journal of Educational Tech-
nology, British Journal of Educational Technology, Computers & Education, Computer Assisted Language Learning, Educa-
tional Technology Research and Development, Journal of Computer Assisted Learning, Language Learning & Technology, and
ReCall.
After collating all of the related literature, another round of searches was conducted using the reference lists found in the
literature yielded by the electronic search to find any omitted but relevant works.
2.2. Search results
2.2.1. Initial screening
The initial search yielded 4121 abstracts published between 1993 and 2013 (1718 in ERIC and 2403 in ISI) that were related
to mobile learning. Two authors read each abstract of the article and judged whether or not the article was related to teaching
and learning with a mobile device, which resulted in the selection of 925 articles.
2.2.2. Screening for experimental and quasiexperimental research
In the second stage, the studies were screened according to the research method. Experimental studies (including the
pretest-posttest equivalent-group, posttest-only equivalent-groups, and randomized matched subjects and posttest-only
control-group designs) and quasi-experimental studies (including the pretest-posttest nonequivalent-groups and counter-
balanced designs; see Ary, Jacobs, & Razavieh, 2002; Best & Kahn, 1998, for a reference) were included. Conceptual analysis or
research reviews, case studies and qualitative research, survey research, and pre-experimental studies were all excluded at
this stage. At the completion of this stage there remained 182 articles.
2.2.3. Application of inclusion and exclusion criteria for the meta-analysis
Studies were eligible for inclusion in the meta-analysis if they conformed with the following three criteria:
Y.-T. Sung et al. / Computers & Education 94 (2016) 252e275254
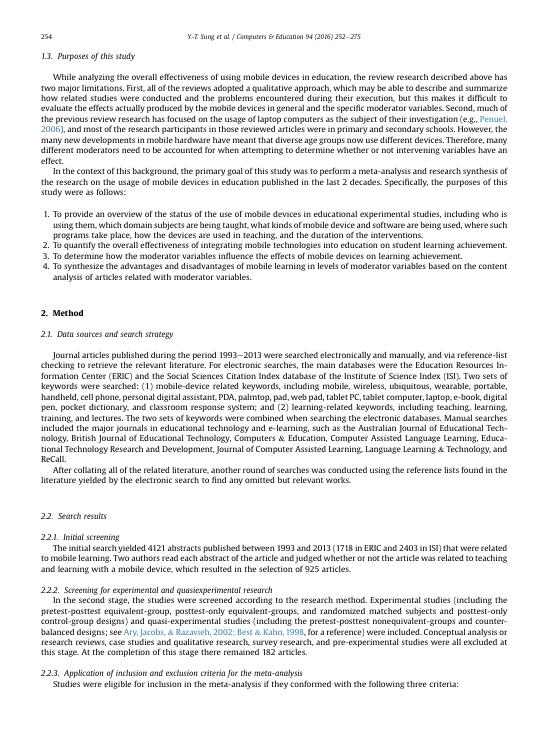
1. The application of mobile devices was the key variable of the study. The experimental group had an intervention that
used mobile devices, and was compared with a control group that used traditional learning. If both the experimental
and control groups used mobile-device interventions, and only the teaching methods were compared, then the study
was excluded (e.g., Hsu, Hwang, Chang, & Chang, 2013; Jeong & Hong, 2013; Li, Chen, & Yang, 2013; Ryu & Parsons,
2012).
2. Sufficient information was presented to calculate effect sizes, such as means, standard deviations, t, F, or c2 values, or the
number of people in each group. Articles in which the sample sizes of each group were not cited, lacked any inferential
statistical results, or had inferential statistical results but were still inadequate for calculating an effect size according to
Lipsey and Wilson (2000) were excluded (e.g., Gleaves, Walker, & Grey, 2007; Langman & Fies, 2010; Purrazzella &
Mechling, 2013; Yang et al., 2013).
3. Experimental results were presented with learning achievement as a major dependent variable measured by standardized
or researcher-constructed tests. Studies for which the results were related to affective variables (e.g., learning attitude or
learning motivation) or interaction between peers but without learning achievement were excluded (e.g., Jian, Sandnes,
Law, Huang, & Huang, 2009; Lan et al., 2007; Mouza, 2008; Siozos, Palaigeorgiou, Triantafyllakos, & Despotakis, 2009).
Application of these criteria yielded 110 articles that were acceptable for inclusion in the meta-analysis. For a complete list
of these references, please see our online supplemental archive.
2.3. Selection and coding of the outcome variables
One of the most used framework for representing the research content and dimensions is the activity theory (AT), which
uses activity as a unit for analyzing human practices (Bakhurst, 2009). Recently, several researchers have used the AT as a
theoretical basis for analyzing mobile learning studies (e.g., Frohberg et al., 2009; Sharples, Taylor, & Vavoula, 2007) or for
designing mobile learning scenarios (e.g., Zurita & Nussbaum, 2007). This study used six major components of AT to select
moderator variables and analyze mobile learning: (a) Subjects: which involve all the people who may be involved in learning
curriculums through mobile devices, such as students of different age levels or teachers of different levels of teaching
expertise. (b) Objects (or objectives) of the mobile learning, which focus on the goal such as acquiring cognitive skills or
enhancing learning motivation through mobile devices. (c) Tools/instruments in the mobile learning, which may be artifacts
(e.g., hardware and software) or learning resources (e.g., tutors). (d) Rules/control for the activity, which are norms or reg-
ulations that circumscribe the mobile activities, such as the procedure in teaching scenarios designed for the learning pace or
styles designated. (e) Context of the activity, which refers to the physical (e.g., classroom or museum) or social (e.g., ambience
of learning in a group) environments for conducting mobile learning. (f) Communication/interaction, which refers to the
method of interaction between users and mobile technologies (such as the process teachers' adaption to mobile devices) or
the communications styles among learners.
2.3.1. Research name
This refers to the first author's name, the year of publication, and the article title.
2.3.2. Research participants
In this review, for all the reviewed articles, the research participant corresponded to the “subject” of the AT framework,
and was coded by their learning stages, including kindergarten, elementary school, middle school, (senior) high school,
university, graduate school, teachers, adults, and mixed.
2.3.3. Treatments
The treatments of the reviewed articles corresponded to the “tools” component (e.g., the hardware and software), the
“rules/control” component (e.g., the teaching methods and domain subjects), and the “context” component (e.g., intervention
settings, intervention duration). The description for each of these treatment variables are as follows:
1. Hardware: Different types of mobile hardware, which comprised PDAs, laptops, tablet PCs, cell phones, iPods, MP3 players,
e-book readers, pads, digital pens, pocket dictionaries, and classroom response systems (CRSs), or any mixture of thereof.
2. Software: Different types of software, which encompassed general-purpose software and learning-oriented software
(Sung & Lesgold, 2007), the former referring to commercial software currently in circulation that was not designed
especially for teaching and learning (e.g., word processors or spreadsheets), and the latter having been designed specif-
ically for educational programs or goals.
3. Teaching method: Different teaching methods, including lectures, cooperative learning (students were divided into groups
and completed learning tasks collaboratively, e.g., Chang, Lan, Chang, & Sung, 2010; Huang, Liang, Su, & Chen, 2012),
inquiry-oriented learning (using problem-, project-, or inquiry-based methods with mobile devices for learning, e.g., Chen,
2010; Lowther, Ross, & Marrison, 2003), self-directed study (teachers/researchers did not designate or implement specific
teaching scenarios for students to follow, students use mobile devices for self-paced learning, e.g., Chen & Li, 2010; Chen,
Y.-T. Sung et al. / Computers & Education 94 (2016) 252e275 255
used mobile devices, and was compared with a control group that used traditional learning. If both the experimental
and control groups used mobile-device interventions, and only the teaching methods were compared, then the study
was excluded (e.g., Hsu, Hwang, Chang, & Chang, 2013; Jeong & Hong, 2013; Li, Chen, & Yang, 2013; Ryu & Parsons,
2012).
2. Sufficient information was presented to calculate effect sizes, such as means, standard deviations, t, F, or c2 values, or the
number of people in each group. Articles in which the sample sizes of each group were not cited, lacked any inferential
statistical results, or had inferential statistical results but were still inadequate for calculating an effect size according to
Lipsey and Wilson (2000) were excluded (e.g., Gleaves, Walker, & Grey, 2007; Langman & Fies, 2010; Purrazzella &
Mechling, 2013; Yang et al., 2013).
3. Experimental results were presented with learning achievement as a major dependent variable measured by standardized
or researcher-constructed tests. Studies for which the results were related to affective variables (e.g., learning attitude or
learning motivation) or interaction between peers but without learning achievement were excluded (e.g., Jian, Sandnes,
Law, Huang, & Huang, 2009; Lan et al., 2007; Mouza, 2008; Siozos, Palaigeorgiou, Triantafyllakos, & Despotakis, 2009).
Application of these criteria yielded 110 articles that were acceptable for inclusion in the meta-analysis. For a complete list
of these references, please see our online supplemental archive.
2.3. Selection and coding of the outcome variables
One of the most used framework for representing the research content and dimensions is the activity theory (AT), which
uses activity as a unit for analyzing human practices (Bakhurst, 2009). Recently, several researchers have used the AT as a
theoretical basis for analyzing mobile learning studies (e.g., Frohberg et al., 2009; Sharples, Taylor, & Vavoula, 2007) or for
designing mobile learning scenarios (e.g., Zurita & Nussbaum, 2007). This study used six major components of AT to select
moderator variables and analyze mobile learning: (a) Subjects: which involve all the people who may be involved in learning
curriculums through mobile devices, such as students of different age levels or teachers of different levels of teaching
expertise. (b) Objects (or objectives) of the mobile learning, which focus on the goal such as acquiring cognitive skills or
enhancing learning motivation through mobile devices. (c) Tools/instruments in the mobile learning, which may be artifacts
(e.g., hardware and software) or learning resources (e.g., tutors). (d) Rules/control for the activity, which are norms or reg-
ulations that circumscribe the mobile activities, such as the procedure in teaching scenarios designed for the learning pace or
styles designated. (e) Context of the activity, which refers to the physical (e.g., classroom or museum) or social (e.g., ambience
of learning in a group) environments for conducting mobile learning. (f) Communication/interaction, which refers to the
method of interaction between users and mobile technologies (such as the process teachers' adaption to mobile devices) or
the communications styles among learners.
2.3.1. Research name
This refers to the first author's name, the year of publication, and the article title.
2.3.2. Research participants
In this review, for all the reviewed articles, the research participant corresponded to the “subject” of the AT framework,
and was coded by their learning stages, including kindergarten, elementary school, middle school, (senior) high school,
university, graduate school, teachers, adults, and mixed.
2.3.3. Treatments
The treatments of the reviewed articles corresponded to the “tools” component (e.g., the hardware and software), the
“rules/control” component (e.g., the teaching methods and domain subjects), and the “context” component (e.g., intervention
settings, intervention duration). The description for each of these treatment variables are as follows:
1. Hardware: Different types of mobile hardware, which comprised PDAs, laptops, tablet PCs, cell phones, iPods, MP3 players,
e-book readers, pads, digital pens, pocket dictionaries, and classroom response systems (CRSs), or any mixture of thereof.
2. Software: Different types of software, which encompassed general-purpose software and learning-oriented software
(Sung & Lesgold, 2007), the former referring to commercial software currently in circulation that was not designed
especially for teaching and learning (e.g., word processors or spreadsheets), and the latter having been designed specif-
ically for educational programs or goals.
3. Teaching method: Different teaching methods, including lectures, cooperative learning (students were divided into groups
and completed learning tasks collaboratively, e.g., Chang, Lan, Chang, & Sung, 2010; Huang, Liang, Su, & Chen, 2012),
inquiry-oriented learning (using problem-, project-, or inquiry-based methods with mobile devices for learning, e.g., Chen,
2010; Lowther, Ross, & Marrison, 2003), self-directed study (teachers/researchers did not designate or implement specific
teaching scenarios for students to follow, students use mobile devices for self-paced learning, e.g., Chen & Li, 2010; Chen,
Y.-T. Sung et al. / Computers & Education 94 (2016) 252e275 255
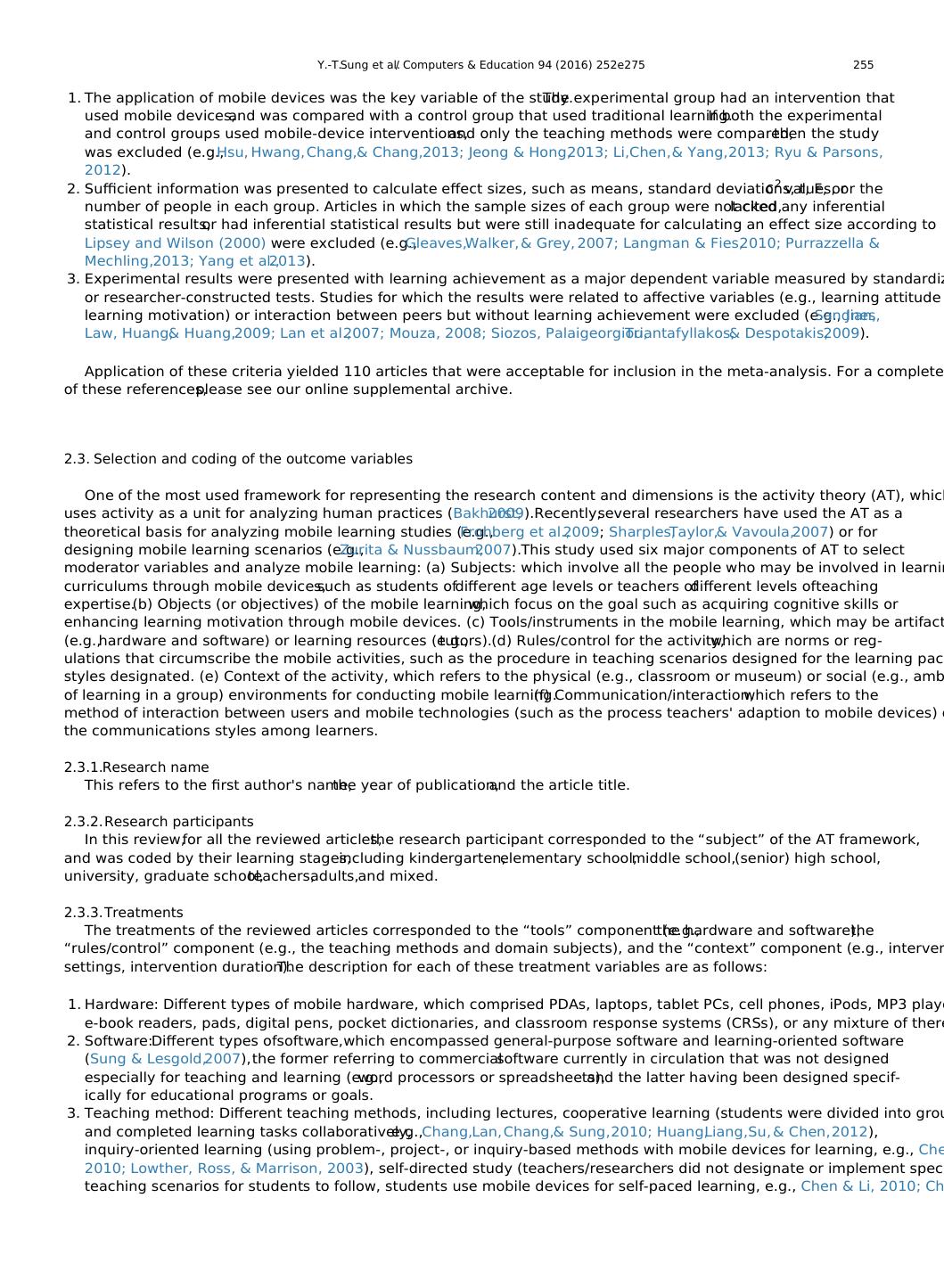
Tan, & Lo, 2013), computer-assisted testing/assessment (using mobile devices for formative assessment or quizzes in
classroom or outdoors, e.g., Agbatogun, 2012), and mixed methods thereof.
4. Domain subject: Domain subjects were analyzed to establish the relative effectiveness of mobile devices for teaching
different subjects, including language arts, social studies, science, mathematics, multidisciplinary (if the mobile devices
were used in several subjects, but measurement of the achievement was presented as a whole instead of separately, this
was coded as multidisciplinary), specific abilities (e.g., spatial ability or creativity), health-care programs, education,
psychology, and computer and information technology.
5. Implementation setting: Implementation settings were included to establish whether the impact of mobile devices on
learning differed according to the environment in which they were used, which included classrooms, outdoors (e.g., zoo or
campus gardens), museum, laboratory, workplaces, and unrestricted settings (devices may be used anywhere).
6. Intervention duration: Different periods of time for the intervention, including periods no more than four hours (4 h),
between five and 24 h (>4 and 24 h), between one day and seven days (>1 day and 7 days), between one week and four
weeks (>1 week and 4 weeks), between one month and six months (>1 month and 6 months), and more than six
months (>6 months).
2.3.4. Dependent variables
The dependent variables corresponded to the “Objective” of the AT model, including two categories: the learning
achievement dependent variables refer to measurement of cognitive outcomes such as knowledge application, retention,
problem solving...etc. The affective variables refer to measurement of motivation, interest, participation...etc.
2.4. Data analysis
2.4.1. Calculating the effect size
The following meta-analysis steps recommended by Borenstein, Hedges, Higgins, and Rothstein (2009) were employed in
this study: (a) determine the effect sizes of each article, (b) determine the weighted mean effect size across articles, (c)
calculate the confidence interval for the average effect size, and (d) determine whether the effect size of any particular group
was influenced by a moderator variable based on a heterogeneity analysis (Q B).
Two formulae were used to calculate the effect sizes of the studies. Cohen's d formula (Cohen, 1988) was used to determine
the effect size for the experimental research with random assignment and without a pretest:
d ¼ X1 X2
ffiffiffiffiffiffiffiffiffiffiffiffiffiffiffiffiffiffiffiffiffiffiffiffiffiffiffiffiffiffiffiffi
ðn1 1Þs2
1 þðn2 1Þs2
2
ðn1 þn2 2Þ
r (1)
where X1 and X2 represent the mean scores, n1 and n2 represent the sample sizes, and s2
1 and s2
2 represent the variances of the
experiment and control groups, respectively.
For experimental or quasiexperimental research with pretests, it was proposed that the pretest should be taken into
consideration instead of using the posttest in order to mitigate possible selection bias (Furtak, Seidel, Iverson, & Briggs, 2012;
Morris, 2008). Hence, the formula developed in Comprehensive Meta Analysis (version 2.0) was used to obtain effect sizes for
research with pre- and posttests:
ESPre=Post Test Two Groups ¼
X1 Post X1 Pre
X2 Post X2 Pre
SDPost
(2)
where X1 Pre and X1 Post represent the mean scores of the experimental group for the pretest and posttest, respectively, and
X2 Pre and X2 Post represent the mean scores of the control group for the pretest and posttest, respectively. SDPost can be
calculated as follows:
SDPost ¼
ffiffiffiffiffiffiffiffiffiffiffiffiffiffiffiffiffiffiffiffiffiffiffiffiffiffiffiffiffiffiffiffiffiffiffiffiffiffiffiffiffiffiffiffiffiffiffiffiffiffiffiffiffiffiffiffiffiffiffiffiffiffiffiffiffiffiffiffiffiffiffiffiffiffiffiffiffiffiffiffiffiffiffiffiffiffiffin2 Post 1s2
2 Post þ n1 Post 1s2
1 Postn2 Post þ n1 Post 2
s
(3)
where n1 Post and n2 Post represent the sample sizes of the experimental and control groups, respectively, for the posttest,
while s2
1 Post and s2
2 Post represent the variances of the experimental and control groups, respectively.
The two types of effect sizes were calibrated using the sample weights to calculate a Hedges' g according to
g ¼
1 3
4df 1
d (4)
Y.-T. Sung et al. / Computers & Education 94 (2016) 252e275256
classroom or outdoors, e.g., Agbatogun, 2012), and mixed methods thereof.
4. Domain subject: Domain subjects were analyzed to establish the relative effectiveness of mobile devices for teaching
different subjects, including language arts, social studies, science, mathematics, multidisciplinary (if the mobile devices
were used in several subjects, but measurement of the achievement was presented as a whole instead of separately, this
was coded as multidisciplinary), specific abilities (e.g., spatial ability or creativity), health-care programs, education,
psychology, and computer and information technology.
5. Implementation setting: Implementation settings were included to establish whether the impact of mobile devices on
learning differed according to the environment in which they were used, which included classrooms, outdoors (e.g., zoo or
campus gardens), museum, laboratory, workplaces, and unrestricted settings (devices may be used anywhere).
6. Intervention duration: Different periods of time for the intervention, including periods no more than four hours (4 h),
between five and 24 h (>4 and 24 h), between one day and seven days (>1 day and 7 days), between one week and four
weeks (>1 week and 4 weeks), between one month and six months (>1 month and 6 months), and more than six
months (>6 months).
2.3.4. Dependent variables
The dependent variables corresponded to the “Objective” of the AT model, including two categories: the learning
achievement dependent variables refer to measurement of cognitive outcomes such as knowledge application, retention,
problem solving...etc. The affective variables refer to measurement of motivation, interest, participation...etc.
2.4. Data analysis
2.4.1. Calculating the effect size
The following meta-analysis steps recommended by Borenstein, Hedges, Higgins, and Rothstein (2009) were employed in
this study: (a) determine the effect sizes of each article, (b) determine the weighted mean effect size across articles, (c)
calculate the confidence interval for the average effect size, and (d) determine whether the effect size of any particular group
was influenced by a moderator variable based on a heterogeneity analysis (Q B).
Two formulae were used to calculate the effect sizes of the studies. Cohen's d formula (Cohen, 1988) was used to determine
the effect size for the experimental research with random assignment and without a pretest:
d ¼ X1 X2
ffiffiffiffiffiffiffiffiffiffiffiffiffiffiffiffiffiffiffiffiffiffiffiffiffiffiffiffiffiffiffiffi
ðn1 1Þs2
1 þðn2 1Þs2
2
ðn1 þn2 2Þ
r (1)
where X1 and X2 represent the mean scores, n1 and n2 represent the sample sizes, and s2
1 and s2
2 represent the variances of the
experiment and control groups, respectively.
For experimental or quasiexperimental research with pretests, it was proposed that the pretest should be taken into
consideration instead of using the posttest in order to mitigate possible selection bias (Furtak, Seidel, Iverson, & Briggs, 2012;
Morris, 2008). Hence, the formula developed in Comprehensive Meta Analysis (version 2.0) was used to obtain effect sizes for
research with pre- and posttests:
ESPre=Post Test Two Groups ¼
X1 Post X1 Pre
X2 Post X2 Pre
SDPost
(2)
where X1 Pre and X1 Post represent the mean scores of the experimental group for the pretest and posttest, respectively, and
X2 Pre and X2 Post represent the mean scores of the control group for the pretest and posttest, respectively. SDPost can be
calculated as follows:
SDPost ¼
ffiffiffiffiffiffiffiffiffiffiffiffiffiffiffiffiffiffiffiffiffiffiffiffiffiffiffiffiffiffiffiffiffiffiffiffiffiffiffiffiffiffiffiffiffiffiffiffiffiffiffiffiffiffiffiffiffiffiffiffiffiffiffiffiffiffiffiffiffiffiffiffiffiffiffiffiffiffiffiffiffiffiffiffiffiffiffin2 Post 1s2
2 Post þ n1 Post 1s2
1 Postn2 Post þ n1 Post 2
s
(3)
where n1 Post and n2 Post represent the sample sizes of the experimental and control groups, respectively, for the posttest,
while s2
1 Post and s2
2 Post represent the variances of the experimental and control groups, respectively.
The two types of effect sizes were calibrated using the sample weights to calculate a Hedges' g according to
g ¼
1 3
4df 1
d (4)
Y.-T. Sung et al. / Computers & Education 94 (2016) 252e275256
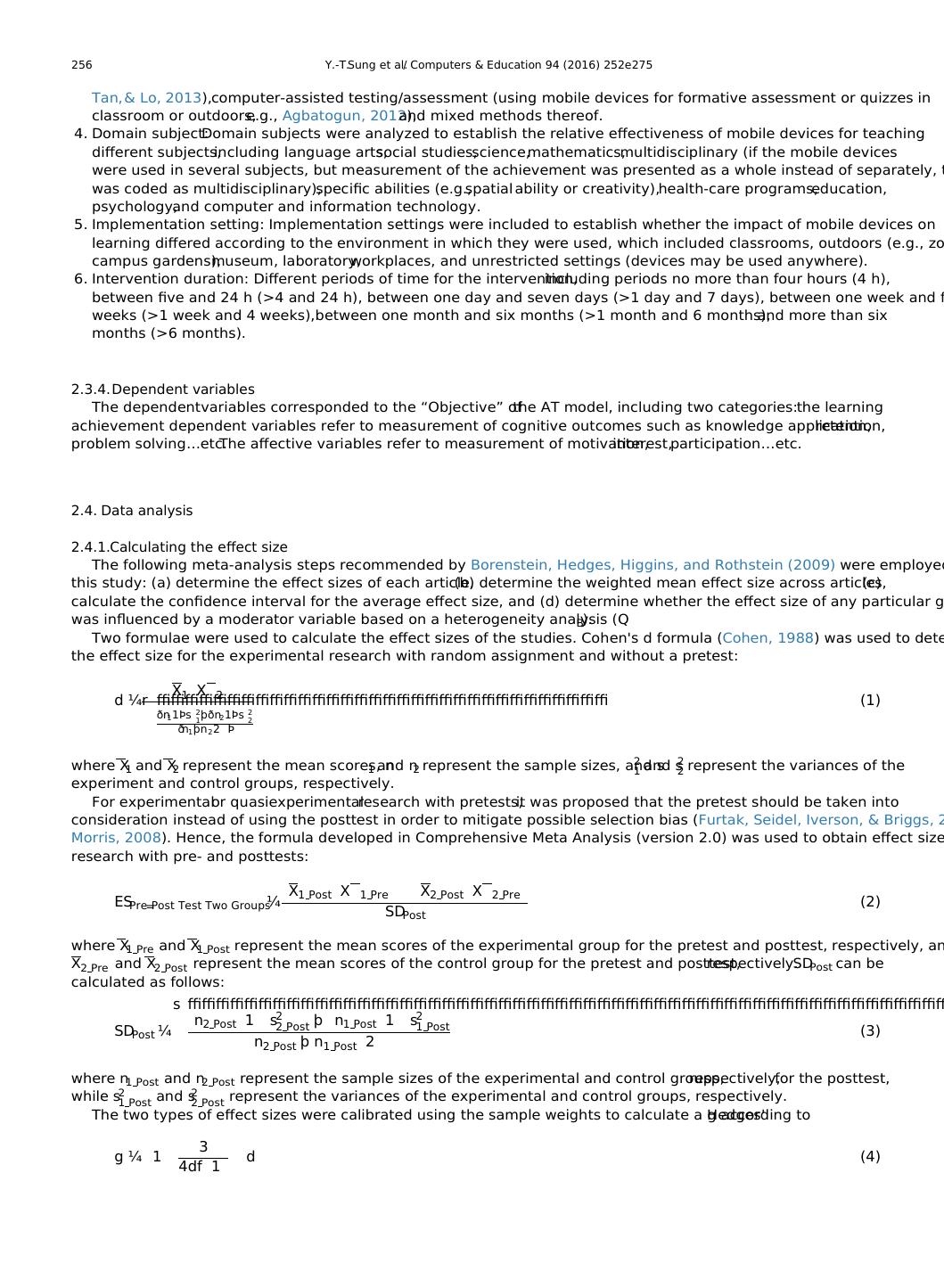
2.4.2. Evaluating publication bias
The fail-safe N (i.e., classic fail-safe N) of Rosenthal (1979) was used to estimate how many insignificant effect sizes
(unpublished data) would be necessary to reduce the overall effect size to an insignificant level. The comparison criterion was
5nþ10, where n is the number of studies included in the meta-analysis. If the fail-safe N is larger than 5nþ10, it means that the
estimated effect size of unpublished research is unlikely to influence the effect size of the meta-analysis. Moreover, the
present study also adopted Orwin's fail-safe N (Orwin, 1983) to estimate the number of missing null studies that would be
required to bring the mean effect size to a trivial level.
3. Results and discussion
3.1. Descriptive statistics information
Table 1 presents the distribution of moderator variables and their corresponding effect sizes (g). In total there were 110
articles, 419 effect sizes, and 18 749 participants. The largest proportion of studies involved the college-student-level learning
stage (38.4%); the next largest group was elementary-school students (33.9%). More studies used learning-oriented software
(62.7%) than general-purpose software (34.5%). Handheld devices (including PDA, cell phone, iPod, MP3 player, digital pen,
pocket dictionary, and classroom response system) were the most widely studied of the hardware (72.7%), followed by
laptops (21.8%, including laptop, pad, tablet PC, and e-book reader). The largest proportion of studies were set in the classroom
(50.0%), followed by outdoors (15.5%) and unrestricted settings (16.4%). For teaching methods, self-directed study (30.9%) was
the most frequently researched, and the most frequently studied intervention duration was >1 month and 6 months
(32.7%), followed by > 1 week and 4 weeks (25.5%) and 4 h (20.9%). Finally, language arts were the most often studied
domain subject (34.7%), followed by science (22.9%).
In addition, among those moderating variables, the evolution of hardware used, implementation setting, and domain
subjects may have seen the greatest amount of change during 1993e2013. The trends of those moderating variables during
the two decades are shown in Figs. 1e3. Fig. 1 shows the evolution of the use of different mobile devices. Compared with
laptop and mixed categories, handheld devices (e.g., cell phone) had been used more since 2009e2013 and showing an
obviously rising trend. Moreover, Fig. 2 shows the evolution of the use of different implementation settings. Compared with
informal settings (e.g., museums; outdoors) and unrestricted categories, formal settings (e.g., classroom; laboratories) had
been set more since 2004e2008 and showing an obviously rising trend. Finally, Fig. 3 shows the evolution of the domain
subject. Compared with other domain subjects, language arts had been studied more since 2009e2013 and showing an
obviously rising trend.
3.2. Overall effect size for learning achievement
The distribution of the effect sizes of the 110 articles is shown in Fig. 4. The forest plot of effect sizes and the 95%
confidence interval of the 110 articles are shown in Appendix B. There were two unusually large effect sizes, g ¼ 4.045 (Hsu
& Lee, 2011) and g ¼ 3.050 (Wu, Sung, Huang, Yang, & Yang, 2011), which were larger than the average effect size for the
entire collection of 110 articles (g ¼ 0.628) more than three standard deviations, and so these were not included in further
analyses (Lipsey & Wilson, 2000). Using the procedure of Lipsey and Wilson (2000) with a random-effects model to
integrate the effect sizes of the 108 articles, there was an overall moderate mean effect size of 0.523, with a 95% confidence
interval of 0.432e0.613. Researchers (e.g., McMillan, Venable, & Varier, 2013; Van der Kleij, Feskens, & Eggen, 2015) have
proposed that Hattie's (2009) criterion is appropriate for evaluating the effect sizes in educational contexts. Therefore, we
adopt Hattie's (2009) criterion to interpret the effect size of our research, in which an effect size of 0.60 is high, around
0.40 is medium, around 0.20 is low, and <0.20 is with little significant meaning. In this study it was found that using mobile
devices in education had a medium effect size for learning achievement; in other words, 69.95% of learners using a mobile
device performed significantly better in dependent variables related with cognitive achievement than those not using
mobile devices.
The Q statistics show that the effect sizes in the meta-analysis were heterogeneous (Qtotal ¼ 626.302, z ¼ 11.315, p < .001),
which indicates that there are differences among the effect sizes resulting from factors other than subject-level sampling
error, such as the diversity of the learning stage, the hardware used, and the teaching methods.
Furthermore, we also conducted an analysis for the studies related to the affective variables (such as motivation,
engagement, attitude, satisfaction, preference). The overall mean effect size of the 22 articles was 0.433 (z ¼ 6.148, p ¼ .001),
with a 95% confidence interval of 0.295e0.570. According to Hattie's criterion, there is a medium effect size for affective
variables when using mobile devices in educational context.
The overall mean effect size for learning achievement in this meta-analysis was 0.523, meaning that learning with
mobiles is significantly more effective than traditional teaching methods that only use pen-and-paper or desktop com-
puters. Compared to past comparisons of effects between using computers and not using computers in education, the effect
size of using mobile devices reported herein seems larger than those found in meta-analysis into desktop-computer-based
instruction, such as in the studies of Kulik and Kulik (1991) and Tamim, Bernard, Borokhovski, Abrami, and Schmid (2011),
who found mean effect sizes for computer-based instruction of 0.30 and 0.35, respectively. One of the reasons for the
different effect sizes may be differences in the features of desktops and mobile devices; however, there are alternative
Y.-T. Sung et al. / Computers & Education 94 (2016) 252e275 257
The fail-safe N (i.e., classic fail-safe N) of Rosenthal (1979) was used to estimate how many insignificant effect sizes
(unpublished data) would be necessary to reduce the overall effect size to an insignificant level. The comparison criterion was
5nþ10, where n is the number of studies included in the meta-analysis. If the fail-safe N is larger than 5nþ10, it means that the
estimated effect size of unpublished research is unlikely to influence the effect size of the meta-analysis. Moreover, the
present study also adopted Orwin's fail-safe N (Orwin, 1983) to estimate the number of missing null studies that would be
required to bring the mean effect size to a trivial level.
3. Results and discussion
3.1. Descriptive statistics information
Table 1 presents the distribution of moderator variables and their corresponding effect sizes (g). In total there were 110
articles, 419 effect sizes, and 18 749 participants. The largest proportion of studies involved the college-student-level learning
stage (38.4%); the next largest group was elementary-school students (33.9%). More studies used learning-oriented software
(62.7%) than general-purpose software (34.5%). Handheld devices (including PDA, cell phone, iPod, MP3 player, digital pen,
pocket dictionary, and classroom response system) were the most widely studied of the hardware (72.7%), followed by
laptops (21.8%, including laptop, pad, tablet PC, and e-book reader). The largest proportion of studies were set in the classroom
(50.0%), followed by outdoors (15.5%) and unrestricted settings (16.4%). For teaching methods, self-directed study (30.9%) was
the most frequently researched, and the most frequently studied intervention duration was >1 month and 6 months
(32.7%), followed by > 1 week and 4 weeks (25.5%) and 4 h (20.9%). Finally, language arts were the most often studied
domain subject (34.7%), followed by science (22.9%).
In addition, among those moderating variables, the evolution of hardware used, implementation setting, and domain
subjects may have seen the greatest amount of change during 1993e2013. The trends of those moderating variables during
the two decades are shown in Figs. 1e3. Fig. 1 shows the evolution of the use of different mobile devices. Compared with
laptop and mixed categories, handheld devices (e.g., cell phone) had been used more since 2009e2013 and showing an
obviously rising trend. Moreover, Fig. 2 shows the evolution of the use of different implementation settings. Compared with
informal settings (e.g., museums; outdoors) and unrestricted categories, formal settings (e.g., classroom; laboratories) had
been set more since 2004e2008 and showing an obviously rising trend. Finally, Fig. 3 shows the evolution of the domain
subject. Compared with other domain subjects, language arts had been studied more since 2009e2013 and showing an
obviously rising trend.
3.2. Overall effect size for learning achievement
The distribution of the effect sizes of the 110 articles is shown in Fig. 4. The forest plot of effect sizes and the 95%
confidence interval of the 110 articles are shown in Appendix B. There were two unusually large effect sizes, g ¼ 4.045 (Hsu
& Lee, 2011) and g ¼ 3.050 (Wu, Sung, Huang, Yang, & Yang, 2011), which were larger than the average effect size for the
entire collection of 110 articles (g ¼ 0.628) more than three standard deviations, and so these were not included in further
analyses (Lipsey & Wilson, 2000). Using the procedure of Lipsey and Wilson (2000) with a random-effects model to
integrate the effect sizes of the 108 articles, there was an overall moderate mean effect size of 0.523, with a 95% confidence
interval of 0.432e0.613. Researchers (e.g., McMillan, Venable, & Varier, 2013; Van der Kleij, Feskens, & Eggen, 2015) have
proposed that Hattie's (2009) criterion is appropriate for evaluating the effect sizes in educational contexts. Therefore, we
adopt Hattie's (2009) criterion to interpret the effect size of our research, in which an effect size of 0.60 is high, around
0.40 is medium, around 0.20 is low, and <0.20 is with little significant meaning. In this study it was found that using mobile
devices in education had a medium effect size for learning achievement; in other words, 69.95% of learners using a mobile
device performed significantly better in dependent variables related with cognitive achievement than those not using
mobile devices.
The Q statistics show that the effect sizes in the meta-analysis were heterogeneous (Qtotal ¼ 626.302, z ¼ 11.315, p < .001),
which indicates that there are differences among the effect sizes resulting from factors other than subject-level sampling
error, such as the diversity of the learning stage, the hardware used, and the teaching methods.
Furthermore, we also conducted an analysis for the studies related to the affective variables (such as motivation,
engagement, attitude, satisfaction, preference). The overall mean effect size of the 22 articles was 0.433 (z ¼ 6.148, p ¼ .001),
with a 95% confidence interval of 0.295e0.570. According to Hattie's criterion, there is a medium effect size for affective
variables when using mobile devices in educational context.
The overall mean effect size for learning achievement in this meta-analysis was 0.523, meaning that learning with
mobiles is significantly more effective than traditional teaching methods that only use pen-and-paper or desktop com-
puters. Compared to past comparisons of effects between using computers and not using computers in education, the effect
size of using mobile devices reported herein seems larger than those found in meta-analysis into desktop-computer-based
instruction, such as in the studies of Kulik and Kulik (1991) and Tamim, Bernard, Borokhovski, Abrami, and Schmid (2011),
who found mean effect sizes for computer-based instruction of 0.30 and 0.35, respectively. One of the reasons for the
different effect sizes may be differences in the features of desktops and mobile devices; however, there are alternative
Y.-T. Sung et al. / Computers & Education 94 (2016) 252e275 257
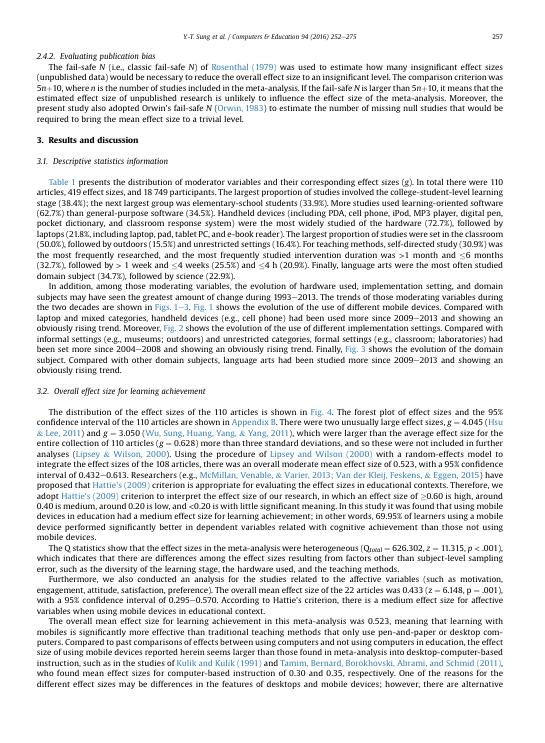
End of preview
Want to access all the pages? Upload your documents or become a member.
Related Documents
Mobile Social Media: the New Hybrid Element of Digital Marketing Communicationslg...
|9
|6236
|16
Journal of Systems and Softwarelg...
|17
|16812
|18
Managing Change in the Delivery of Complex Projectslg...
|13
|14248
|38
Journal of Systems and Software PDFlg...
|17
|16667
|20