Application of Artificial Intelligence in Business
VerifiedAdded on 2022/12/30
|12
|3762
|86
AI Summary
This paper discusses the benefits and challenges of implementing artificial intelligence (AI) in the current business environment. It explores how AI can revolutionize industries and improve decision-making. The implementation of AI is centered around natural language processing, machine learning, and intelligent processing and retrieval of data. The paper also highlights the challenges of conceptualizing and practically applying AI, as well as the ethical and legal concerns associated with AI.
Contribute Materials
Your contribution can guide someone’s learning journey. Share your
documents today.
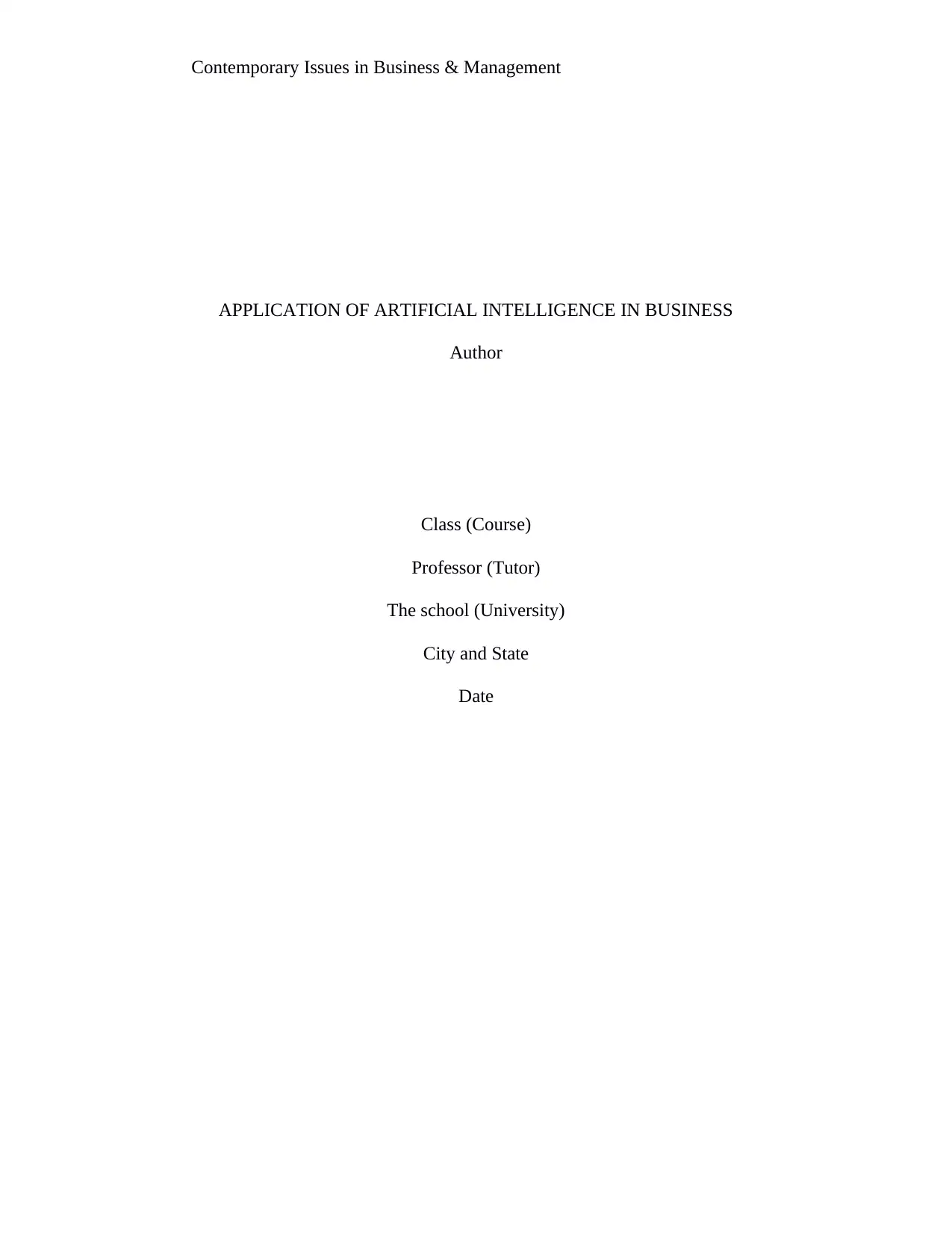
Contemporary Issues in Business & Management
APPLICATION OF ARTIFICIAL INTELLIGENCE IN BUSINESS
Author
Class (Course)
Professor (Tutor)
The school (University)
City and State
Date
APPLICATION OF ARTIFICIAL INTELLIGENCE IN BUSINESS
Author
Class (Course)
Professor (Tutor)
The school (University)
City and State
Date
Secure Best Marks with AI Grader
Need help grading? Try our AI Grader for instant feedback on your assignments.
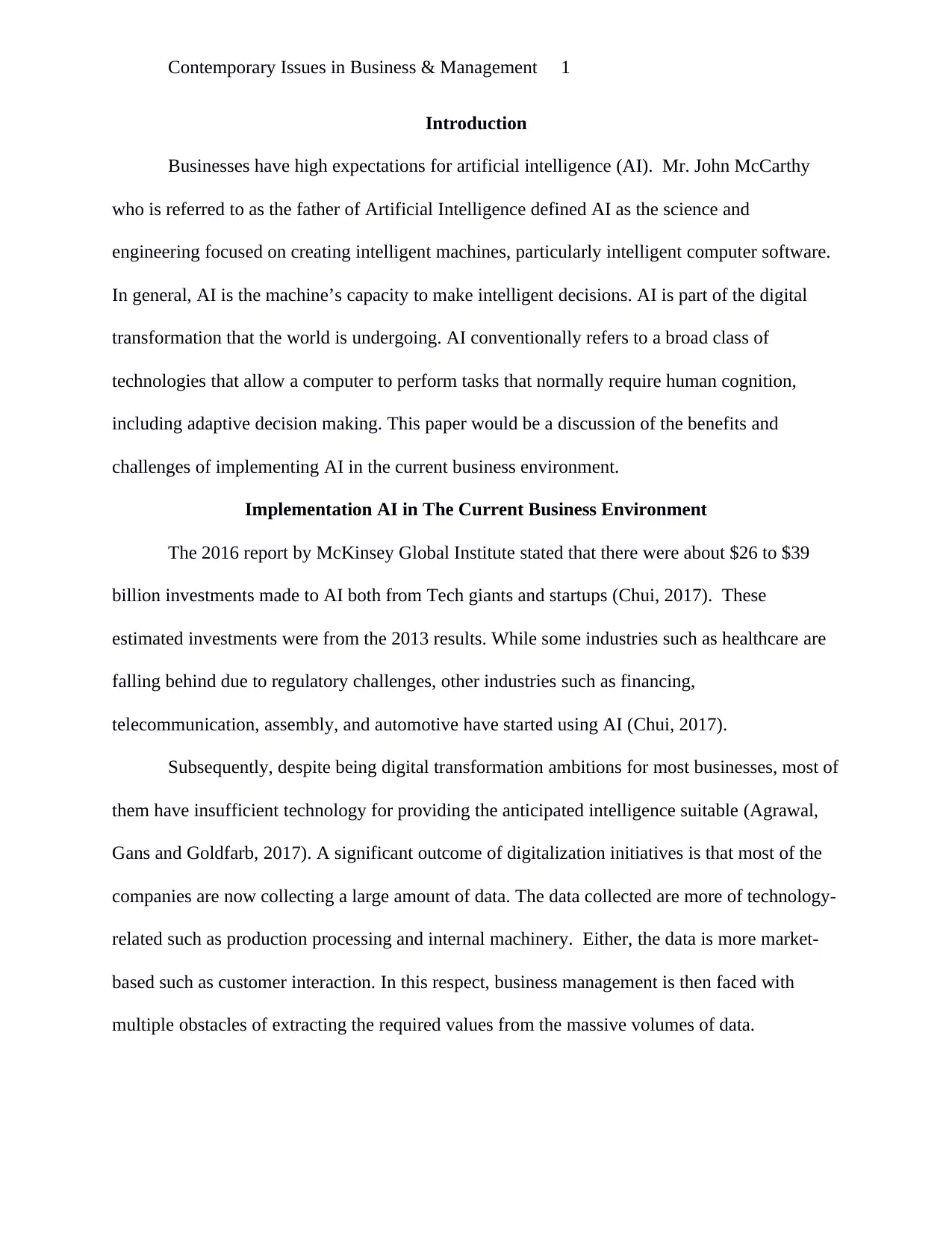
Contemporary Issues in Business & Management 1
Introduction
Businesses have high expectations for artificial intelligence (AI). Mr. John McCarthy
who is referred to as the father of Artificial Intelligence defined AI as the science and
engineering focused on creating intelligent machines, particularly intelligent computer software.
In general, AI is the machine’s capacity to make intelligent decisions. AI is part of the digital
transformation that the world is undergoing. AI conventionally refers to a broad class of
technologies that allow a computer to perform tasks that normally require human cognition,
including adaptive decision making. This paper would be a discussion of the benefits and
challenges of implementing AI in the current business environment.
Implementation AI in The Current Business Environment
The 2016 report by McKinsey Global Institute stated that there were about $26 to $39
billion investments made to AI both from Tech giants and startups (Chui, 2017). These
estimated investments were from the 2013 results. While some industries such as healthcare are
falling behind due to regulatory challenges, other industries such as financing,
telecommunication, assembly, and automotive have started using AI (Chui, 2017).
Subsequently, despite being digital transformation ambitions for most businesses, most of
them have insufficient technology for providing the anticipated intelligence suitable (Agrawal,
Gans and Goldfarb, 2017). A significant outcome of digitalization initiatives is that most of the
companies are now collecting a large amount of data. The data collected are more of technology-
related such as production processing and internal machinery. Either, the data is more market-
based such as customer interaction. In this respect, business management is then faced with
multiple obstacles of extracting the required values from the massive volumes of data.
Introduction
Businesses have high expectations for artificial intelligence (AI). Mr. John McCarthy
who is referred to as the father of Artificial Intelligence defined AI as the science and
engineering focused on creating intelligent machines, particularly intelligent computer software.
In general, AI is the machine’s capacity to make intelligent decisions. AI is part of the digital
transformation that the world is undergoing. AI conventionally refers to a broad class of
technologies that allow a computer to perform tasks that normally require human cognition,
including adaptive decision making. This paper would be a discussion of the benefits and
challenges of implementing AI in the current business environment.
Implementation AI in The Current Business Environment
The 2016 report by McKinsey Global Institute stated that there were about $26 to $39
billion investments made to AI both from Tech giants and startups (Chui, 2017). These
estimated investments were from the 2013 results. While some industries such as healthcare are
falling behind due to regulatory challenges, other industries such as financing,
telecommunication, assembly, and automotive have started using AI (Chui, 2017).
Subsequently, despite being digital transformation ambitions for most businesses, most of
them have insufficient technology for providing the anticipated intelligence suitable (Agrawal,
Gans and Goldfarb, 2017). A significant outcome of digitalization initiatives is that most of the
companies are now collecting a large amount of data. The data collected are more of technology-
related such as production processing and internal machinery. Either, the data is more market-
based such as customer interaction. In this respect, business management is then faced with
multiple obstacles of extracting the required values from the massive volumes of data.
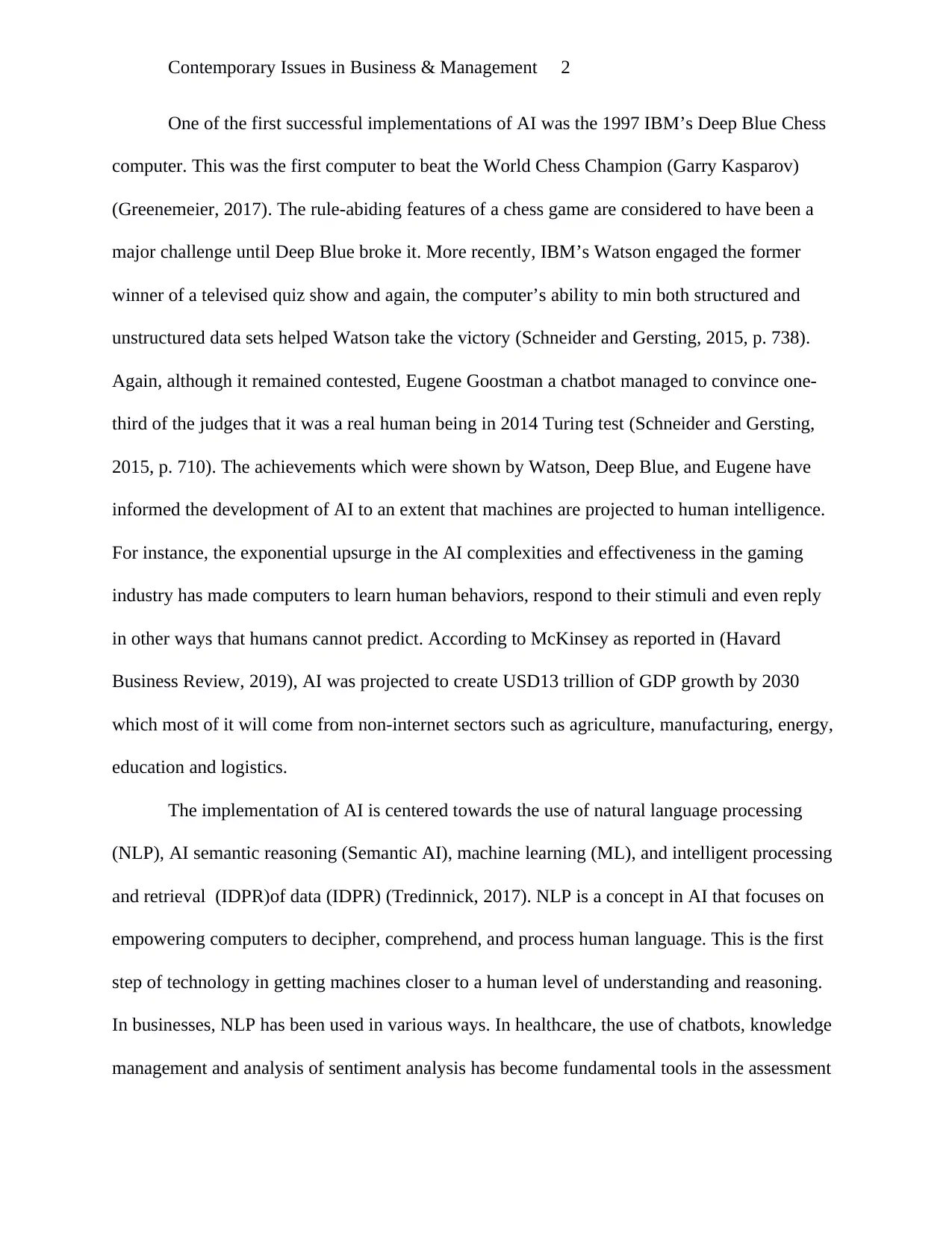
Contemporary Issues in Business & Management 2
One of the first successful implementations of AI was the 1997 IBM’s Deep Blue Chess
computer. This was the first computer to beat the World Chess Champion (Garry Kasparov)
(Greenemeier, 2017). The rule-abiding features of a chess game are considered to have been a
major challenge until Deep Blue broke it. More recently, IBM’s Watson engaged the former
winner of a televised quiz show and again, the computer’s ability to min both structured and
unstructured data sets helped Watson take the victory (Schneider and Gersting, 2015, p. 738).
Again, although it remained contested, Eugene Goostman a chatbot managed to convince one-
third of the judges that it was a real human being in 2014 Turing test (Schneider and Gersting,
2015, p. 710). The achievements which were shown by Watson, Deep Blue, and Eugene have
informed the development of AI to an extent that machines are projected to human intelligence.
For instance, the exponential upsurge in the AI complexities and effectiveness in the gaming
industry has made computers to learn human behaviors, respond to their stimuli and even reply
in other ways that humans cannot predict. According to McKinsey as reported in (Havard
Business Review, 2019), AI was projected to create USD13 trillion of GDP growth by 2030
which most of it will come from non-internet sectors such as agriculture, manufacturing, energy,
education and logistics.
The implementation of AI is centered towards the use of natural language processing
(NLP), AI semantic reasoning (Semantic AI), machine learning (ML), and intelligent processing
and retrieval (IDPR)of data (IDPR) (Tredinnick, 2017). NLP is a concept in AI that focuses on
empowering computers to decipher, comprehend, and process human language. This is the first
step of technology in getting machines closer to a human level of understanding and reasoning.
In businesses, NLP has been used in various ways. In healthcare, the use of chatbots, knowledge
management and analysis of sentiment analysis has become fundamental tools in the assessment
One of the first successful implementations of AI was the 1997 IBM’s Deep Blue Chess
computer. This was the first computer to beat the World Chess Champion (Garry Kasparov)
(Greenemeier, 2017). The rule-abiding features of a chess game are considered to have been a
major challenge until Deep Blue broke it. More recently, IBM’s Watson engaged the former
winner of a televised quiz show and again, the computer’s ability to min both structured and
unstructured data sets helped Watson take the victory (Schneider and Gersting, 2015, p. 738).
Again, although it remained contested, Eugene Goostman a chatbot managed to convince one-
third of the judges that it was a real human being in 2014 Turing test (Schneider and Gersting,
2015, p. 710). The achievements which were shown by Watson, Deep Blue, and Eugene have
informed the development of AI to an extent that machines are projected to human intelligence.
For instance, the exponential upsurge in the AI complexities and effectiveness in the gaming
industry has made computers to learn human behaviors, respond to their stimuli and even reply
in other ways that humans cannot predict. According to McKinsey as reported in (Havard
Business Review, 2019), AI was projected to create USD13 trillion of GDP growth by 2030
which most of it will come from non-internet sectors such as agriculture, manufacturing, energy,
education and logistics.
The implementation of AI is centered towards the use of natural language processing
(NLP), AI semantic reasoning (Semantic AI), machine learning (ML), and intelligent processing
and retrieval (IDPR)of data (IDPR) (Tredinnick, 2017). NLP is a concept in AI that focuses on
empowering computers to decipher, comprehend, and process human language. This is the first
step of technology in getting machines closer to a human level of understanding and reasoning.
In businesses, NLP has been used in various ways. In healthcare, the use of chatbots, knowledge
management and analysis of sentiment analysis has become fundamental tools in the assessment
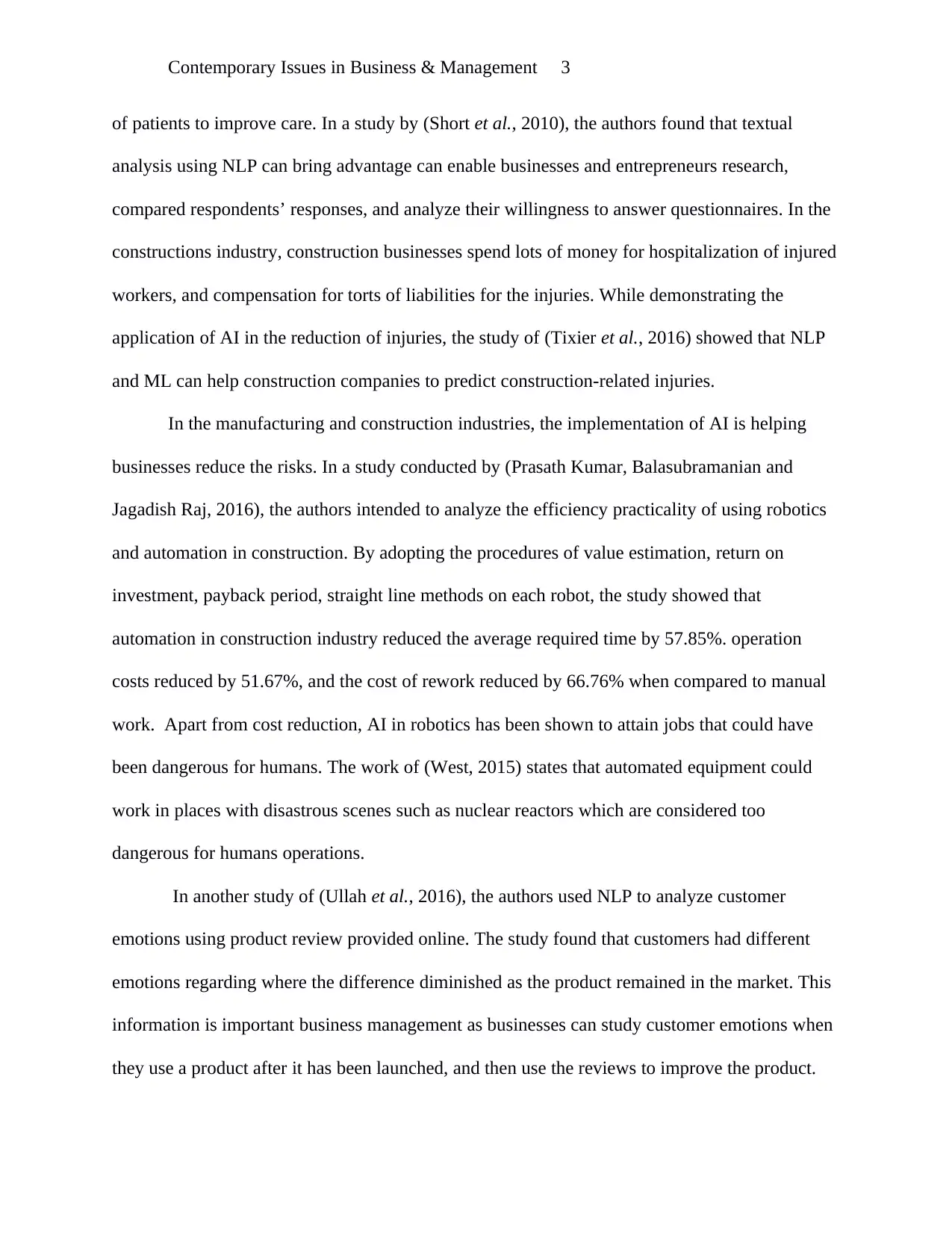
Contemporary Issues in Business & Management 3
of patients to improve care. In a study by (Short et al., 2010), the authors found that textual
analysis using NLP can bring advantage can enable businesses and entrepreneurs research,
compared respondents’ responses, and analyze their willingness to answer questionnaires. In the
constructions industry, construction businesses spend lots of money for hospitalization of injured
workers, and compensation for torts of liabilities for the injuries. While demonstrating the
application of AI in the reduction of injuries, the study of (Tixier et al., 2016) showed that NLP
and ML can help construction companies to predict construction-related injuries.
In the manufacturing and construction industries, the implementation of AI is helping
businesses reduce the risks. In a study conducted by (Prasath Kumar, Balasubramanian and
Jagadish Raj, 2016), the authors intended to analyze the efficiency practicality of using robotics
and automation in construction. By adopting the procedures of value estimation, return on
investment, payback period, straight line methods on each robot, the study showed that
automation in construction industry reduced the average required time by 57.85%. operation
costs reduced by 51.67%, and the cost of rework reduced by 66.76% when compared to manual
work. Apart from cost reduction, AI in robotics has been shown to attain jobs that could have
been dangerous for humans. The work of (West, 2015) states that automated equipment could
work in places with disastrous scenes such as nuclear reactors which are considered too
dangerous for humans operations.
In another study of (Ullah et al., 2016), the authors used NLP to analyze customer
emotions using product review provided online. The study found that customers had different
emotions regarding where the difference diminished as the product remained in the market. This
information is important business management as businesses can study customer emotions when
they use a product after it has been launched, and then use the reviews to improve the product.
of patients to improve care. In a study by (Short et al., 2010), the authors found that textual
analysis using NLP can bring advantage can enable businesses and entrepreneurs research,
compared respondents’ responses, and analyze their willingness to answer questionnaires. In the
constructions industry, construction businesses spend lots of money for hospitalization of injured
workers, and compensation for torts of liabilities for the injuries. While demonstrating the
application of AI in the reduction of injuries, the study of (Tixier et al., 2016) showed that NLP
and ML can help construction companies to predict construction-related injuries.
In the manufacturing and construction industries, the implementation of AI is helping
businesses reduce the risks. In a study conducted by (Prasath Kumar, Balasubramanian and
Jagadish Raj, 2016), the authors intended to analyze the efficiency practicality of using robotics
and automation in construction. By adopting the procedures of value estimation, return on
investment, payback period, straight line methods on each robot, the study showed that
automation in construction industry reduced the average required time by 57.85%. operation
costs reduced by 51.67%, and the cost of rework reduced by 66.76% when compared to manual
work. Apart from cost reduction, AI in robotics has been shown to attain jobs that could have
been dangerous for humans. The work of (West, 2015) states that automated equipment could
work in places with disastrous scenes such as nuclear reactors which are considered too
dangerous for humans operations.
In another study of (Ullah et al., 2016), the authors used NLP to analyze customer
emotions using product review provided online. The study found that customers had different
emotions regarding where the difference diminished as the product remained in the market. This
information is important business management as businesses can study customer emotions when
they use a product after it has been launched, and then use the reviews to improve the product.
Secure Best Marks with AI Grader
Need help grading? Try our AI Grader for instant feedback on your assignments.
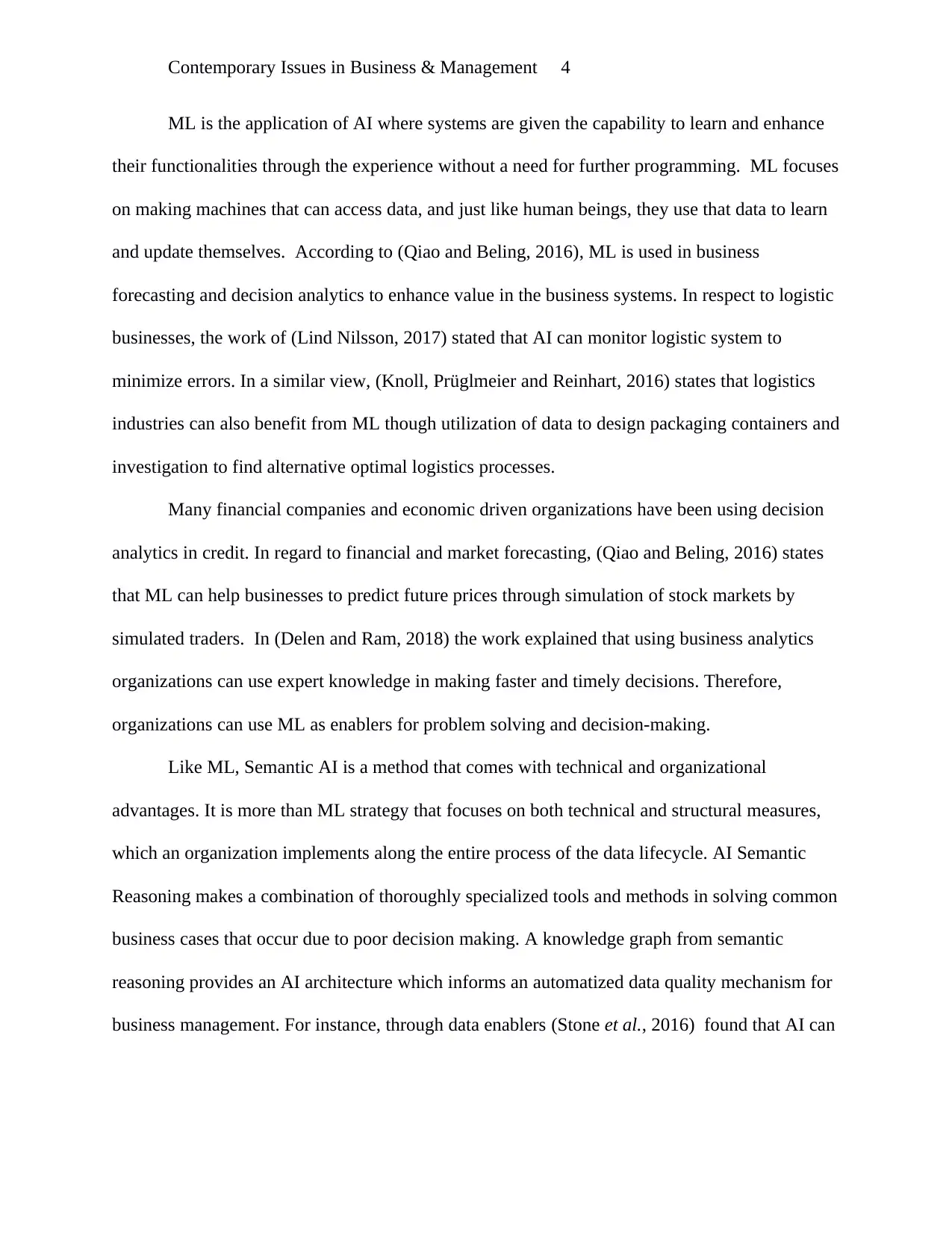
Contemporary Issues in Business & Management 4
ML is the application of AI where systems are given the capability to learn and enhance
their functionalities through the experience without a need for further programming. ML focuses
on making machines that can access data, and just like human beings, they use that data to learn
and update themselves. According to (Qiao and Beling, 2016), ML is used in business
forecasting and decision analytics to enhance value in the business systems. In respect to logistic
businesses, the work of (Lind Nilsson, 2017) stated that AI can monitor logistic system to
minimize errors. In a similar view, (Knoll, Prüglmeier and Reinhart, 2016) states that logistics
industries can also benefit from ML though utilization of data to design packaging containers and
investigation to find alternative optimal logistics processes.
Many financial companies and economic driven organizations have been using decision
analytics in credit. In regard to financial and market forecasting, (Qiao and Beling, 2016) states
that ML can help businesses to predict future prices through simulation of stock markets by
simulated traders. In (Delen and Ram, 2018) the work explained that using business analytics
organizations can use expert knowledge in making faster and timely decisions. Therefore,
organizations can use ML as enablers for problem solving and decision-making.
Like ML, Semantic AI is a method that comes with technical and organizational
advantages. It is more than ML strategy that focuses on both technical and structural measures,
which an organization implements along the entire process of the data lifecycle. AI Semantic
Reasoning makes a combination of thoroughly specialized tools and methods in solving common
business cases that occur due to poor decision making. A knowledge graph from semantic
reasoning provides an AI architecture which informs an automatized data quality mechanism for
business management. For instance, through data enablers (Stone et al., 2016) found that AI can
ML is the application of AI where systems are given the capability to learn and enhance
their functionalities through the experience without a need for further programming. ML focuses
on making machines that can access data, and just like human beings, they use that data to learn
and update themselves. According to (Qiao and Beling, 2016), ML is used in business
forecasting and decision analytics to enhance value in the business systems. In respect to logistic
businesses, the work of (Lind Nilsson, 2017) stated that AI can monitor logistic system to
minimize errors. In a similar view, (Knoll, Prüglmeier and Reinhart, 2016) states that logistics
industries can also benefit from ML though utilization of data to design packaging containers and
investigation to find alternative optimal logistics processes.
Many financial companies and economic driven organizations have been using decision
analytics in credit. In regard to financial and market forecasting, (Qiao and Beling, 2016) states
that ML can help businesses to predict future prices through simulation of stock markets by
simulated traders. In (Delen and Ram, 2018) the work explained that using business analytics
organizations can use expert knowledge in making faster and timely decisions. Therefore,
organizations can use ML as enablers for problem solving and decision-making.
Like ML, Semantic AI is a method that comes with technical and organizational
advantages. It is more than ML strategy that focuses on both technical and structural measures,
which an organization implements along the entire process of the data lifecycle. AI Semantic
Reasoning makes a combination of thoroughly specialized tools and methods in solving common
business cases that occur due to poor decision making. A knowledge graph from semantic
reasoning provides an AI architecture which informs an automatized data quality mechanism for
business management. For instance, through data enablers (Stone et al., 2016) found that AI can
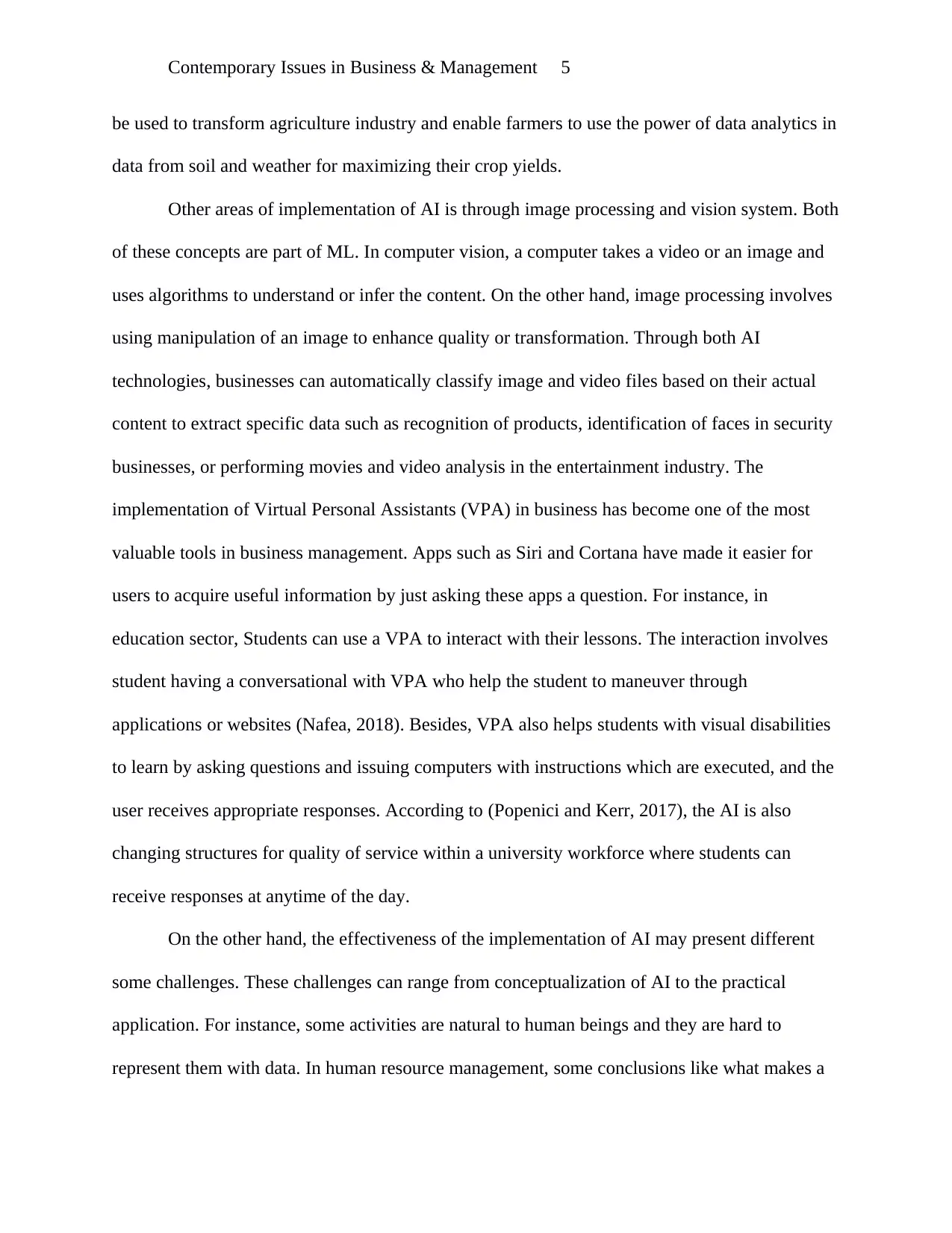
Contemporary Issues in Business & Management 5
be used to transform agriculture industry and enable farmers to use the power of data analytics in
data from soil and weather for maximizing their crop yields.
Other areas of implementation of AI is through image processing and vision system. Both
of these concepts are part of ML. In computer vision, a computer takes a video or an image and
uses algorithms to understand or infer the content. On the other hand, image processing involves
using manipulation of an image to enhance quality or transformation. Through both AI
technologies, businesses can automatically classify image and video files based on their actual
content to extract specific data such as recognition of products, identification of faces in security
businesses, or performing movies and video analysis in the entertainment industry. The
implementation of Virtual Personal Assistants (VPA) in business has become one of the most
valuable tools in business management. Apps such as Siri and Cortana have made it easier for
users to acquire useful information by just asking these apps a question. For instance, in
education sector, Students can use a VPA to interact with their lessons. The interaction involves
student having a conversational with VPA who help the student to maneuver through
applications or websites (Nafea, 2018). Besides, VPA also helps students with visual disabilities
to learn by asking questions and issuing computers with instructions which are executed, and the
user receives appropriate responses. According to (Popenici and Kerr, 2017), the AI is also
changing structures for quality of service within a university workforce where students can
receive responses at anytime of the day.
On the other hand, the effectiveness of the implementation of AI may present different
some challenges. These challenges can range from conceptualization of AI to the practical
application. For instance, some activities are natural to human beings and they are hard to
represent them with data. In human resource management, some conclusions like what makes a
be used to transform agriculture industry and enable farmers to use the power of data analytics in
data from soil and weather for maximizing their crop yields.
Other areas of implementation of AI is through image processing and vision system. Both
of these concepts are part of ML. In computer vision, a computer takes a video or an image and
uses algorithms to understand or infer the content. On the other hand, image processing involves
using manipulation of an image to enhance quality or transformation. Through both AI
technologies, businesses can automatically classify image and video files based on their actual
content to extract specific data such as recognition of products, identification of faces in security
businesses, or performing movies and video analysis in the entertainment industry. The
implementation of Virtual Personal Assistants (VPA) in business has become one of the most
valuable tools in business management. Apps such as Siri and Cortana have made it easier for
users to acquire useful information by just asking these apps a question. For instance, in
education sector, Students can use a VPA to interact with their lessons. The interaction involves
student having a conversational with VPA who help the student to maneuver through
applications or websites (Nafea, 2018). Besides, VPA also helps students with visual disabilities
to learn by asking questions and issuing computers with instructions which are executed, and the
user receives appropriate responses. According to (Popenici and Kerr, 2017), the AI is also
changing structures for quality of service within a university workforce where students can
receive responses at anytime of the day.
On the other hand, the effectiveness of the implementation of AI may present different
some challenges. These challenges can range from conceptualization of AI to the practical
application. For instance, some activities are natural to human beings and they are hard to
represent them with data. In human resource management, some conclusions like what makes a
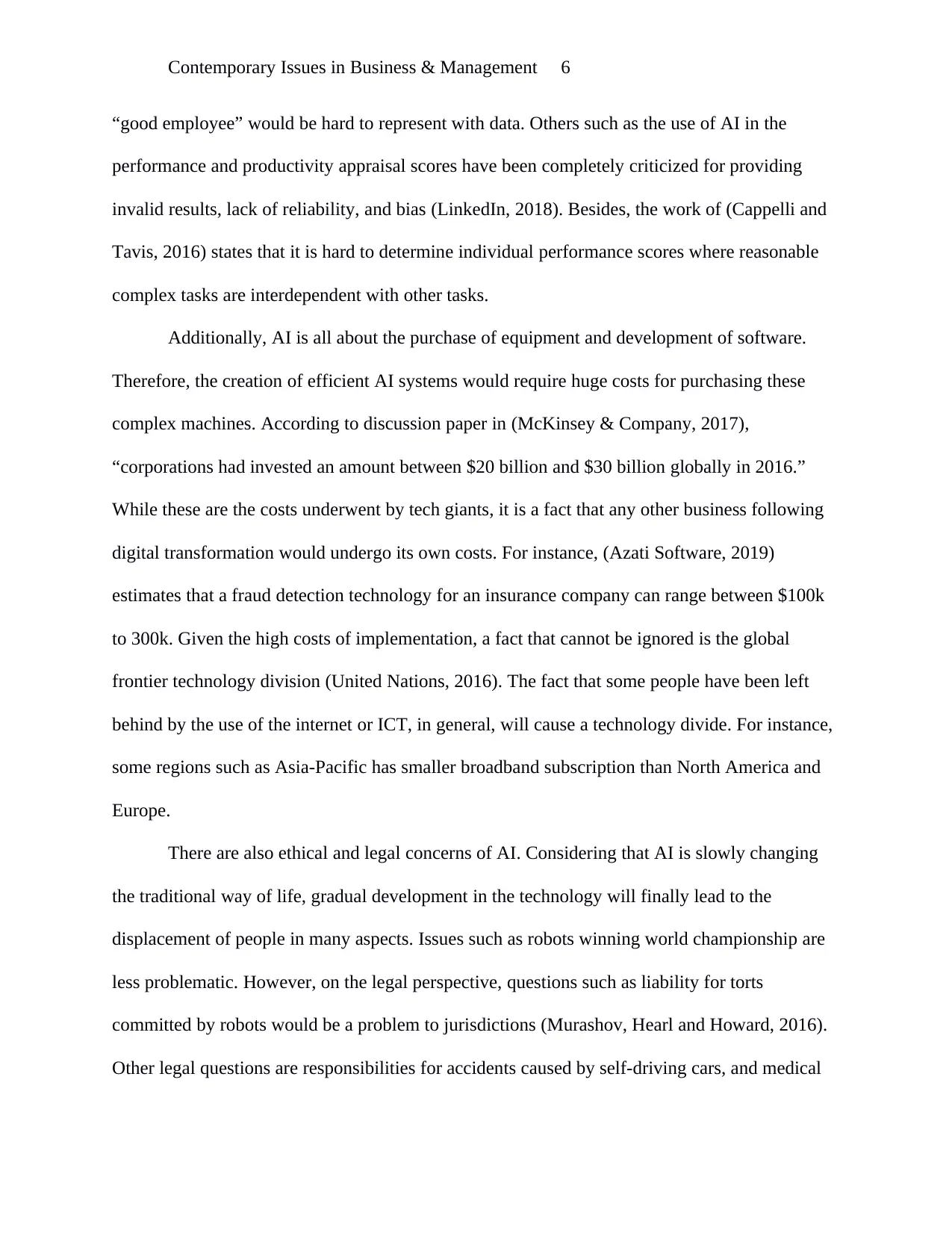
Contemporary Issues in Business & Management 6
“good employee” would be hard to represent with data. Others such as the use of AI in the
performance and productivity appraisal scores have been completely criticized for providing
invalid results, lack of reliability, and bias (LinkedIn, 2018). Besides, the work of (Cappelli and
Tavis, 2016) states that it is hard to determine individual performance scores where reasonable
complex tasks are interdependent with other tasks.
Additionally, AI is all about the purchase of equipment and development of software.
Therefore, the creation of efficient AI systems would require huge costs for purchasing these
complex machines. According to discussion paper in (McKinsey & Company, 2017),
“corporations had invested an amount between $20 billion and $30 billion globally in 2016.”
While these are the costs underwent by tech giants, it is a fact that any other business following
digital transformation would undergo its own costs. For instance, (Azati Software, 2019)
estimates that a fraud detection technology for an insurance company can range between $100k
to 300k. Given the high costs of implementation, a fact that cannot be ignored is the global
frontier technology division (United Nations, 2016). The fact that some people have been left
behind by the use of the internet or ICT, in general, will cause a technology divide. For instance,
some regions such as Asia-Pacific has smaller broadband subscription than North America and
Europe.
There are also ethical and legal concerns of AI. Considering that AI is slowly changing
the traditional way of life, gradual development in the technology will finally lead to the
displacement of people in many aspects. Issues such as robots winning world championship are
less problematic. However, on the legal perspective, questions such as liability for torts
committed by robots would be a problem to jurisdictions (Murashov, Hearl and Howard, 2016).
Other legal questions are responsibilities for accidents caused by self-driving cars, and medical
“good employee” would be hard to represent with data. Others such as the use of AI in the
performance and productivity appraisal scores have been completely criticized for providing
invalid results, lack of reliability, and bias (LinkedIn, 2018). Besides, the work of (Cappelli and
Tavis, 2016) states that it is hard to determine individual performance scores where reasonable
complex tasks are interdependent with other tasks.
Additionally, AI is all about the purchase of equipment and development of software.
Therefore, the creation of efficient AI systems would require huge costs for purchasing these
complex machines. According to discussion paper in (McKinsey & Company, 2017),
“corporations had invested an amount between $20 billion and $30 billion globally in 2016.”
While these are the costs underwent by tech giants, it is a fact that any other business following
digital transformation would undergo its own costs. For instance, (Azati Software, 2019)
estimates that a fraud detection technology for an insurance company can range between $100k
to 300k. Given the high costs of implementation, a fact that cannot be ignored is the global
frontier technology division (United Nations, 2016). The fact that some people have been left
behind by the use of the internet or ICT, in general, will cause a technology divide. For instance,
some regions such as Asia-Pacific has smaller broadband subscription than North America and
Europe.
There are also ethical and legal concerns of AI. Considering that AI is slowly changing
the traditional way of life, gradual development in the technology will finally lead to the
displacement of people in many aspects. Issues such as robots winning world championship are
less problematic. However, on the legal perspective, questions such as liability for torts
committed by robots would be a problem to jurisdictions (Murashov, Hearl and Howard, 2016).
Other legal questions are responsibilities for accidents caused by self-driving cars, and medical
Paraphrase This Document
Need a fresh take? Get an instant paraphrase of this document with our AI Paraphraser
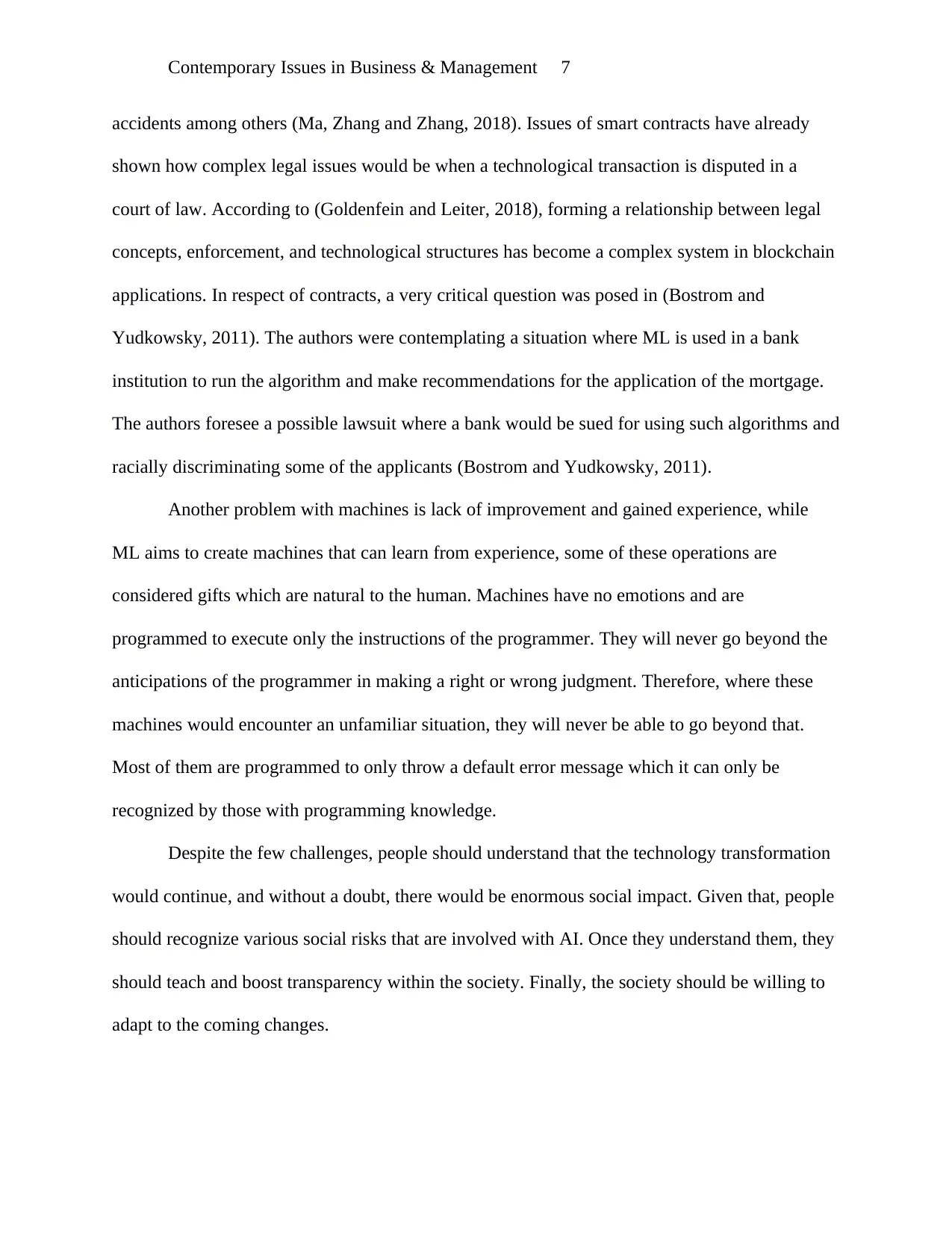
Contemporary Issues in Business & Management 7
accidents among others (Ma, Zhang and Zhang, 2018). Issues of smart contracts have already
shown how complex legal issues would be when a technological transaction is disputed in a
court of law. According to (Goldenfein and Leiter, 2018), forming a relationship between legal
concepts, enforcement, and technological structures has become a complex system in blockchain
applications. In respect of contracts, a very critical question was posed in (Bostrom and
Yudkowsky, 2011). The authors were contemplating a situation where ML is used in a bank
institution to run the algorithm and make recommendations for the application of the mortgage.
The authors foresee a possible lawsuit where a bank would be sued for using such algorithms and
racially discriminating some of the applicants (Bostrom and Yudkowsky, 2011).
Another problem with machines is lack of improvement and gained experience, while
ML aims to create machines that can learn from experience, some of these operations are
considered gifts which are natural to the human. Machines have no emotions and are
programmed to execute only the instructions of the programmer. They will never go beyond the
anticipations of the programmer in making a right or wrong judgment. Therefore, where these
machines would encounter an unfamiliar situation, they will never be able to go beyond that.
Most of them are programmed to only throw a default error message which it can only be
recognized by those with programming knowledge.
Despite the few challenges, people should understand that the technology transformation
would continue, and without a doubt, there would be enormous social impact. Given that, people
should recognize various social risks that are involved with AI. Once they understand them, they
should teach and boost transparency within the society. Finally, the society should be willing to
adapt to the coming changes.
accidents among others (Ma, Zhang and Zhang, 2018). Issues of smart contracts have already
shown how complex legal issues would be when a technological transaction is disputed in a
court of law. According to (Goldenfein and Leiter, 2018), forming a relationship between legal
concepts, enforcement, and technological structures has become a complex system in blockchain
applications. In respect of contracts, a very critical question was posed in (Bostrom and
Yudkowsky, 2011). The authors were contemplating a situation where ML is used in a bank
institution to run the algorithm and make recommendations for the application of the mortgage.
The authors foresee a possible lawsuit where a bank would be sued for using such algorithms and
racially discriminating some of the applicants (Bostrom and Yudkowsky, 2011).
Another problem with machines is lack of improvement and gained experience, while
ML aims to create machines that can learn from experience, some of these operations are
considered gifts which are natural to the human. Machines have no emotions and are
programmed to execute only the instructions of the programmer. They will never go beyond the
anticipations of the programmer in making a right or wrong judgment. Therefore, where these
machines would encounter an unfamiliar situation, they will never be able to go beyond that.
Most of them are programmed to only throw a default error message which it can only be
recognized by those with programming knowledge.
Despite the few challenges, people should understand that the technology transformation
would continue, and without a doubt, there would be enormous social impact. Given that, people
should recognize various social risks that are involved with AI. Once they understand them, they
should teach and boost transparency within the society. Finally, the society should be willing to
adapt to the coming changes.
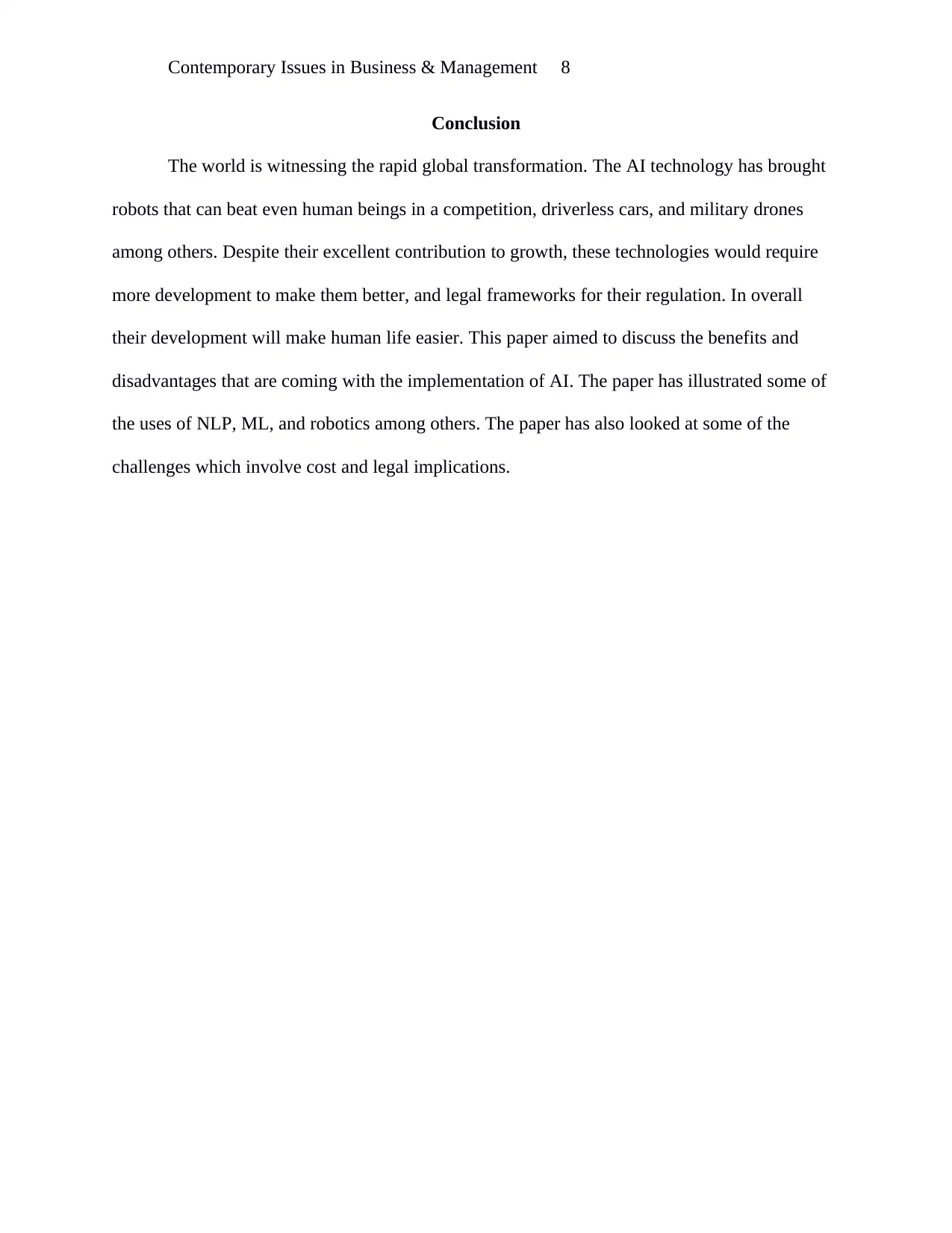
Contemporary Issues in Business & Management 8
Conclusion
The world is witnessing the rapid global transformation. The AI technology has brought
robots that can beat even human beings in a competition, driverless cars, and military drones
among others. Despite their excellent contribution to growth, these technologies would require
more development to make them better, and legal frameworks for their regulation. In overall
their development will make human life easier. This paper aimed to discuss the benefits and
disadvantages that are coming with the implementation of AI. The paper has illustrated some of
the uses of NLP, ML, and robotics among others. The paper has also looked at some of the
challenges which involve cost and legal implications.
Conclusion
The world is witnessing the rapid global transformation. The AI technology has brought
robots that can beat even human beings in a competition, driverless cars, and military drones
among others. Despite their excellent contribution to growth, these technologies would require
more development to make them better, and legal frameworks for their regulation. In overall
their development will make human life easier. This paper aimed to discuss the benefits and
disadvantages that are coming with the implementation of AI. The paper has illustrated some of
the uses of NLP, ML, and robotics among others. The paper has also looked at some of the
challenges which involve cost and legal implications.
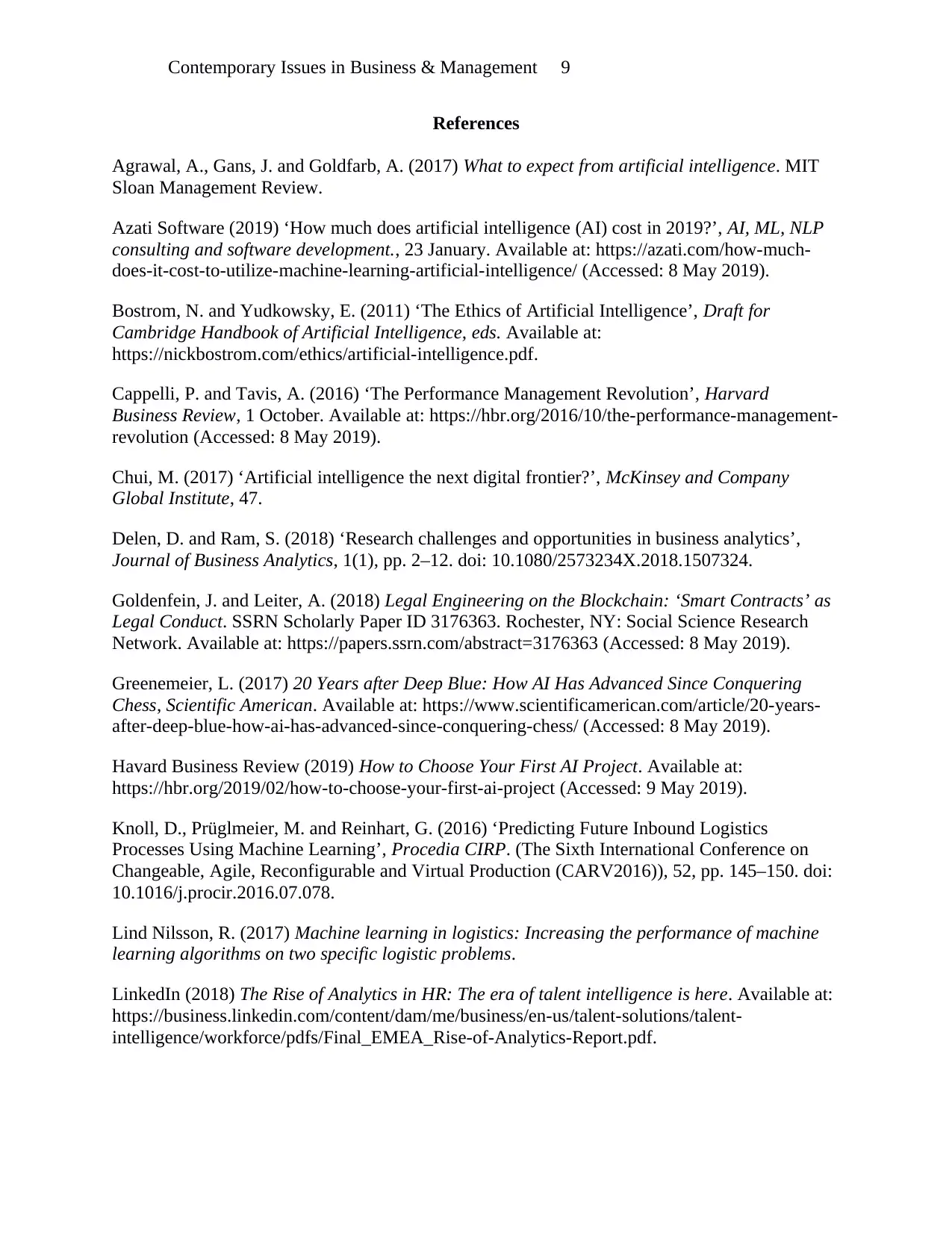
Contemporary Issues in Business & Management 9
References
Agrawal, A., Gans, J. and Goldfarb, A. (2017) What to expect from artificial intelligence. MIT
Sloan Management Review.
Azati Software (2019) ‘How much does artificial intelligence (AI) cost in 2019?’, AI, ML, NLP
consulting and software development., 23 January. Available at: https://azati.com/how-much-
does-it-cost-to-utilize-machine-learning-artificial-intelligence/ (Accessed: 8 May 2019).
Bostrom, N. and Yudkowsky, E. (2011) ‘The Ethics of Artificial Intelligence’, Draft for
Cambridge Handbook of Artificial Intelligence, eds. Available at:
https://nickbostrom.com/ethics/artificial-intelligence.pdf.
Cappelli, P. and Tavis, A. (2016) ‘The Performance Management Revolution’, Harvard
Business Review, 1 October. Available at: https://hbr.org/2016/10/the-performance-management-
revolution (Accessed: 8 May 2019).
Chui, M. (2017) ‘Artificial intelligence the next digital frontier?’, McKinsey and Company
Global Institute, 47.
Delen, D. and Ram, S. (2018) ‘Research challenges and opportunities in business analytics’,
Journal of Business Analytics, 1(1), pp. 2–12. doi: 10.1080/2573234X.2018.1507324.
Goldenfein, J. and Leiter, A. (2018) Legal Engineering on the Blockchain: ‘Smart Contracts’ as
Legal Conduct. SSRN Scholarly Paper ID 3176363. Rochester, NY: Social Science Research
Network. Available at: https://papers.ssrn.com/abstract=3176363 (Accessed: 8 May 2019).
Greenemeier, L. (2017) 20 Years after Deep Blue: How AI Has Advanced Since Conquering
Chess, Scientific American. Available at: https://www.scientificamerican.com/article/20-years-
after-deep-blue-how-ai-has-advanced-since-conquering-chess/ (Accessed: 8 May 2019).
Havard Business Review (2019) How to Choose Your First AI Project. Available at:
https://hbr.org/2019/02/how-to-choose-your-first-ai-project (Accessed: 9 May 2019).
Knoll, D., Prüglmeier, M. and Reinhart, G. (2016) ‘Predicting Future Inbound Logistics
Processes Using Machine Learning’, Procedia CIRP. (The Sixth International Conference on
Changeable, Agile, Reconfigurable and Virtual Production (CARV2016)), 52, pp. 145–150. doi:
10.1016/j.procir.2016.07.078.
Lind Nilsson, R. (2017) Machine learning in logistics: Increasing the performance of machine
learning algorithms on two specific logistic problems.
LinkedIn (2018) The Rise of Analytics in HR: The era of talent intelligence is here. Available at:
https://business.linkedin.com/content/dam/me/business/en-us/talent-solutions/talent-
intelligence/workforce/pdfs/Final_EMEA_Rise-of-Analytics-Report.pdf.
References
Agrawal, A., Gans, J. and Goldfarb, A. (2017) What to expect from artificial intelligence. MIT
Sloan Management Review.
Azati Software (2019) ‘How much does artificial intelligence (AI) cost in 2019?’, AI, ML, NLP
consulting and software development., 23 January. Available at: https://azati.com/how-much-
does-it-cost-to-utilize-machine-learning-artificial-intelligence/ (Accessed: 8 May 2019).
Bostrom, N. and Yudkowsky, E. (2011) ‘The Ethics of Artificial Intelligence’, Draft for
Cambridge Handbook of Artificial Intelligence, eds. Available at:
https://nickbostrom.com/ethics/artificial-intelligence.pdf.
Cappelli, P. and Tavis, A. (2016) ‘The Performance Management Revolution’, Harvard
Business Review, 1 October. Available at: https://hbr.org/2016/10/the-performance-management-
revolution (Accessed: 8 May 2019).
Chui, M. (2017) ‘Artificial intelligence the next digital frontier?’, McKinsey and Company
Global Institute, 47.
Delen, D. and Ram, S. (2018) ‘Research challenges and opportunities in business analytics’,
Journal of Business Analytics, 1(1), pp. 2–12. doi: 10.1080/2573234X.2018.1507324.
Goldenfein, J. and Leiter, A. (2018) Legal Engineering on the Blockchain: ‘Smart Contracts’ as
Legal Conduct. SSRN Scholarly Paper ID 3176363. Rochester, NY: Social Science Research
Network. Available at: https://papers.ssrn.com/abstract=3176363 (Accessed: 8 May 2019).
Greenemeier, L. (2017) 20 Years after Deep Blue: How AI Has Advanced Since Conquering
Chess, Scientific American. Available at: https://www.scientificamerican.com/article/20-years-
after-deep-blue-how-ai-has-advanced-since-conquering-chess/ (Accessed: 8 May 2019).
Havard Business Review (2019) How to Choose Your First AI Project. Available at:
https://hbr.org/2019/02/how-to-choose-your-first-ai-project (Accessed: 9 May 2019).
Knoll, D., Prüglmeier, M. and Reinhart, G. (2016) ‘Predicting Future Inbound Logistics
Processes Using Machine Learning’, Procedia CIRP. (The Sixth International Conference on
Changeable, Agile, Reconfigurable and Virtual Production (CARV2016)), 52, pp. 145–150. doi:
10.1016/j.procir.2016.07.078.
Lind Nilsson, R. (2017) Machine learning in logistics: Increasing the performance of machine
learning algorithms on two specific logistic problems.
LinkedIn (2018) The Rise of Analytics in HR: The era of talent intelligence is here. Available at:
https://business.linkedin.com/content/dam/me/business/en-us/talent-solutions/talent-
intelligence/workforce/pdfs/Final_EMEA_Rise-of-Analytics-Report.pdf.
Secure Best Marks with AI Grader
Need help grading? Try our AI Grader for instant feedback on your assignments.
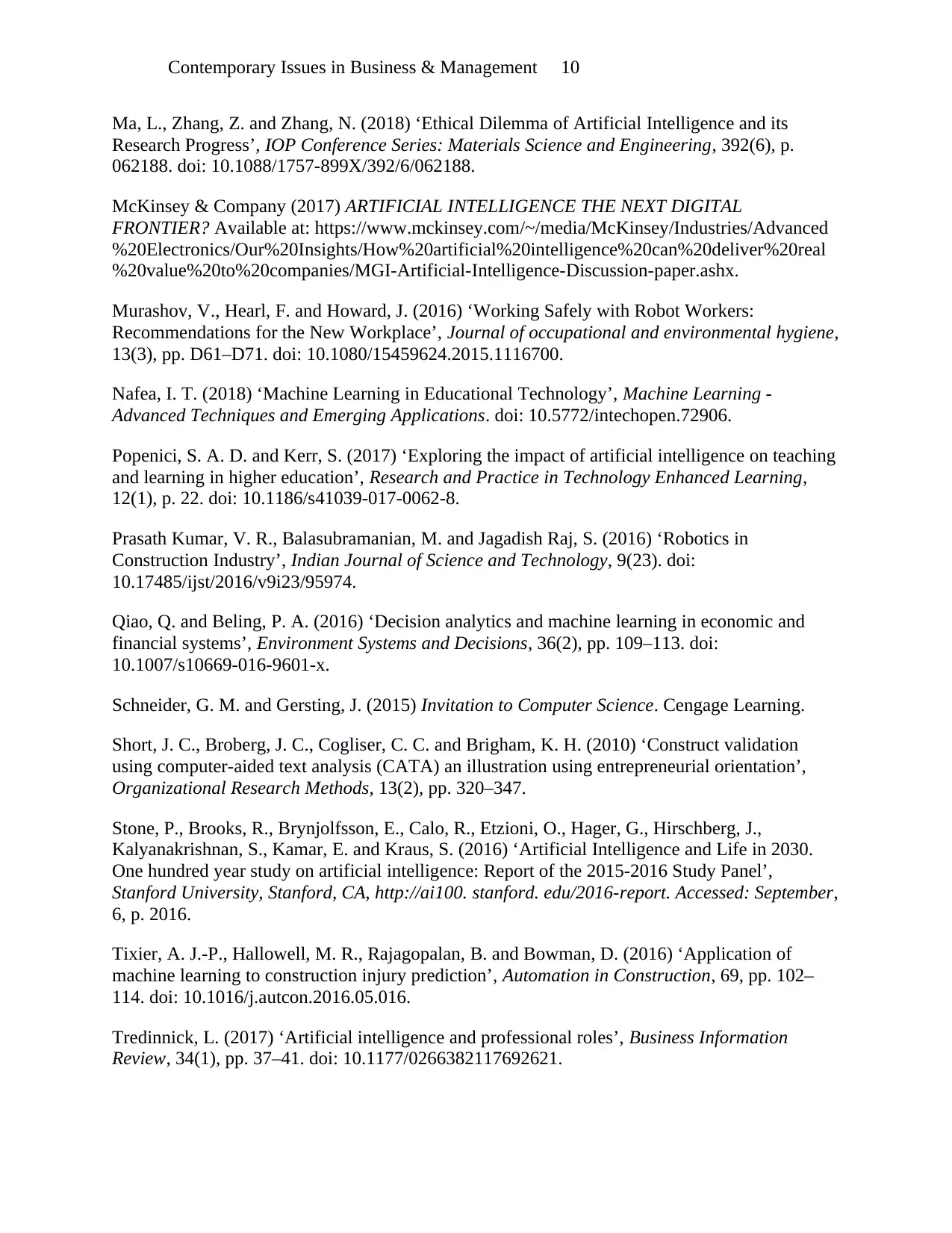
Contemporary Issues in Business & Management 10
Ma, L., Zhang, Z. and Zhang, N. (2018) ‘Ethical Dilemma of Artificial Intelligence and its
Research Progress’, IOP Conference Series: Materials Science and Engineering, 392(6), p.
062188. doi: 10.1088/1757-899X/392/6/062188.
McKinsey & Company (2017) ARTIFICIAL INTELLIGENCE THE NEXT DIGITAL
FRONTIER? Available at: https://www.mckinsey.com/~/media/McKinsey/Industries/Advanced
%20Electronics/Our%20Insights/How%20artificial%20intelligence%20can%20deliver%20real
%20value%20to%20companies/MGI-Artificial-Intelligence-Discussion-paper.ashx.
Murashov, V., Hearl, F. and Howard, J. (2016) ‘Working Safely with Robot Workers:
Recommendations for the New Workplace’, Journal of occupational and environmental hygiene,
13(3), pp. D61–D71. doi: 10.1080/15459624.2015.1116700.
Nafea, I. T. (2018) ‘Machine Learning in Educational Technology’, Machine Learning -
Advanced Techniques and Emerging Applications. doi: 10.5772/intechopen.72906.
Popenici, S. A. D. and Kerr, S. (2017) ‘Exploring the impact of artificial intelligence on teaching
and learning in higher education’, Research and Practice in Technology Enhanced Learning,
12(1), p. 22. doi: 10.1186/s41039-017-0062-8.
Prasath Kumar, V. R., Balasubramanian, M. and Jagadish Raj, S. (2016) ‘Robotics in
Construction Industry’, Indian Journal of Science and Technology, 9(23). doi:
10.17485/ijst/2016/v9i23/95974.
Qiao, Q. and Beling, P. A. (2016) ‘Decision analytics and machine learning in economic and
financial systems’, Environment Systems and Decisions, 36(2), pp. 109–113. doi:
10.1007/s10669-016-9601-x.
Schneider, G. M. and Gersting, J. (2015) Invitation to Computer Science. Cengage Learning.
Short, J. C., Broberg, J. C., Cogliser, C. C. and Brigham, K. H. (2010) ‘Construct validation
using computer-aided text analysis (CATA) an illustration using entrepreneurial orientation’,
Organizational Research Methods, 13(2), pp. 320–347.
Stone, P., Brooks, R., Brynjolfsson, E., Calo, R., Etzioni, O., Hager, G., Hirschberg, J.,
Kalyanakrishnan, S., Kamar, E. and Kraus, S. (2016) ‘Artificial Intelligence and Life in 2030.
One hundred year study on artificial intelligence: Report of the 2015-2016 Study Panel’,
Stanford University, Stanford, CA, http://ai100. stanford. edu/2016-report. Accessed: September,
6, p. 2016.
Tixier, A. J.-P., Hallowell, M. R., Rajagopalan, B. and Bowman, D. (2016) ‘Application of
machine learning to construction injury prediction’, Automation in Construction, 69, pp. 102–
114. doi: 10.1016/j.autcon.2016.05.016.
Tredinnick, L. (2017) ‘Artificial intelligence and professional roles’, Business Information
Review, 34(1), pp. 37–41. doi: 10.1177/0266382117692621.
Ma, L., Zhang, Z. and Zhang, N. (2018) ‘Ethical Dilemma of Artificial Intelligence and its
Research Progress’, IOP Conference Series: Materials Science and Engineering, 392(6), p.
062188. doi: 10.1088/1757-899X/392/6/062188.
McKinsey & Company (2017) ARTIFICIAL INTELLIGENCE THE NEXT DIGITAL
FRONTIER? Available at: https://www.mckinsey.com/~/media/McKinsey/Industries/Advanced
%20Electronics/Our%20Insights/How%20artificial%20intelligence%20can%20deliver%20real
%20value%20to%20companies/MGI-Artificial-Intelligence-Discussion-paper.ashx.
Murashov, V., Hearl, F. and Howard, J. (2016) ‘Working Safely with Robot Workers:
Recommendations for the New Workplace’, Journal of occupational and environmental hygiene,
13(3), pp. D61–D71. doi: 10.1080/15459624.2015.1116700.
Nafea, I. T. (2018) ‘Machine Learning in Educational Technology’, Machine Learning -
Advanced Techniques and Emerging Applications. doi: 10.5772/intechopen.72906.
Popenici, S. A. D. and Kerr, S. (2017) ‘Exploring the impact of artificial intelligence on teaching
and learning in higher education’, Research and Practice in Technology Enhanced Learning,
12(1), p. 22. doi: 10.1186/s41039-017-0062-8.
Prasath Kumar, V. R., Balasubramanian, M. and Jagadish Raj, S. (2016) ‘Robotics in
Construction Industry’, Indian Journal of Science and Technology, 9(23). doi:
10.17485/ijst/2016/v9i23/95974.
Qiao, Q. and Beling, P. A. (2016) ‘Decision analytics and machine learning in economic and
financial systems’, Environment Systems and Decisions, 36(2), pp. 109–113. doi:
10.1007/s10669-016-9601-x.
Schneider, G. M. and Gersting, J. (2015) Invitation to Computer Science. Cengage Learning.
Short, J. C., Broberg, J. C., Cogliser, C. C. and Brigham, K. H. (2010) ‘Construct validation
using computer-aided text analysis (CATA) an illustration using entrepreneurial orientation’,
Organizational Research Methods, 13(2), pp. 320–347.
Stone, P., Brooks, R., Brynjolfsson, E., Calo, R., Etzioni, O., Hager, G., Hirschberg, J.,
Kalyanakrishnan, S., Kamar, E. and Kraus, S. (2016) ‘Artificial Intelligence and Life in 2030.
One hundred year study on artificial intelligence: Report of the 2015-2016 Study Panel’,
Stanford University, Stanford, CA, http://ai100. stanford. edu/2016-report. Accessed: September,
6, p. 2016.
Tixier, A. J.-P., Hallowell, M. R., Rajagopalan, B. and Bowman, D. (2016) ‘Application of
machine learning to construction injury prediction’, Automation in Construction, 69, pp. 102–
114. doi: 10.1016/j.autcon.2016.05.016.
Tredinnick, L. (2017) ‘Artificial intelligence and professional roles’, Business Information
Review, 34(1), pp. 37–41. doi: 10.1177/0266382117692621.
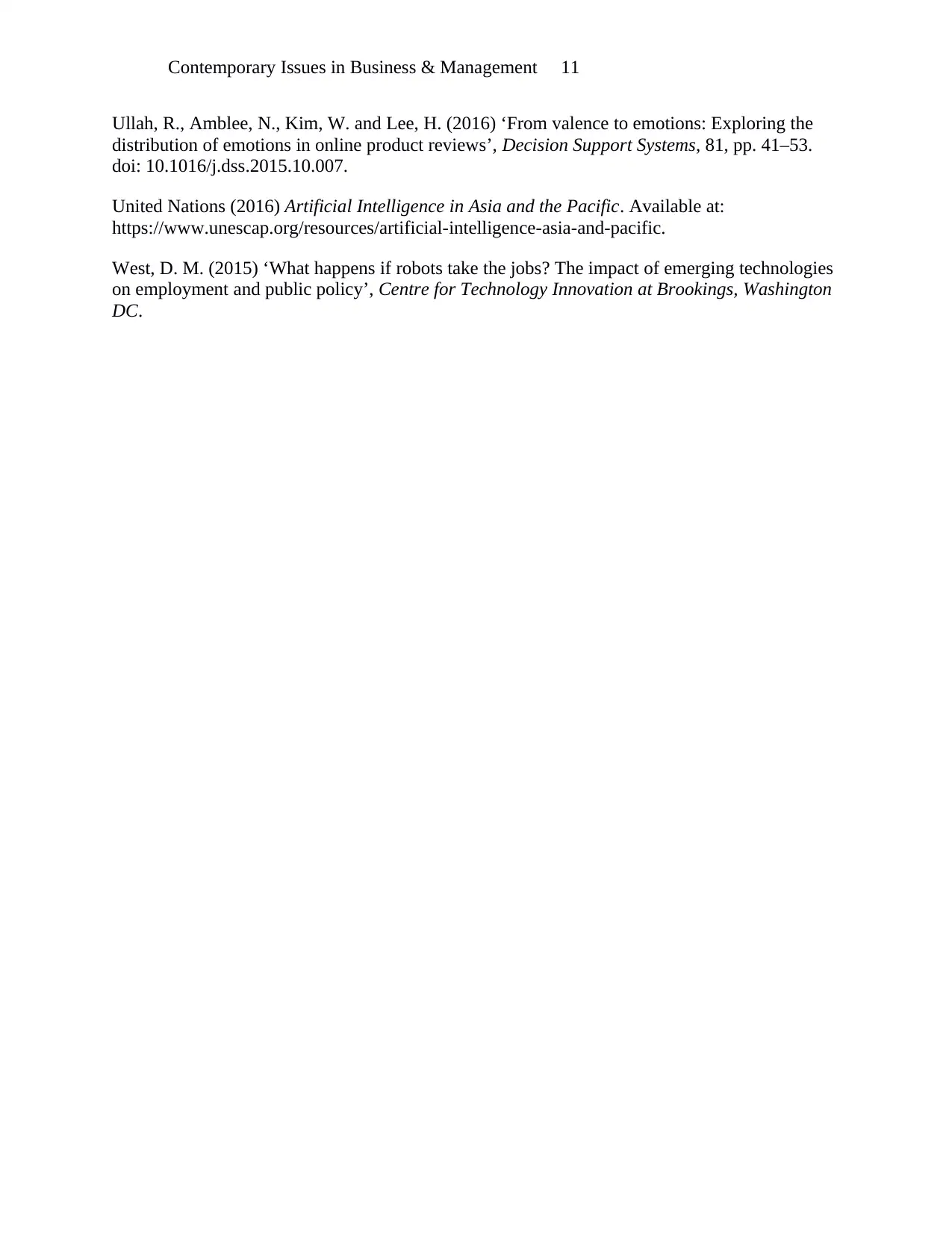
Contemporary Issues in Business & Management 11
Ullah, R., Amblee, N., Kim, W. and Lee, H. (2016) ‘From valence to emotions: Exploring the
distribution of emotions in online product reviews’, Decision Support Systems, 81, pp. 41–53.
doi: 10.1016/j.dss.2015.10.007.
United Nations (2016) Artificial Intelligence in Asia and the Pacific. Available at:
https://www.unescap.org/resources/artificial-intelligence-asia-and-pacific.
West, D. M. (2015) ‘What happens if robots take the jobs? The impact of emerging technologies
on employment and public policy’, Centre for Technology Innovation at Brookings, Washington
DC.
Ullah, R., Amblee, N., Kim, W. and Lee, H. (2016) ‘From valence to emotions: Exploring the
distribution of emotions in online product reviews’, Decision Support Systems, 81, pp. 41–53.
doi: 10.1016/j.dss.2015.10.007.
United Nations (2016) Artificial Intelligence in Asia and the Pacific. Available at:
https://www.unescap.org/resources/artificial-intelligence-asia-and-pacific.
West, D. M. (2015) ‘What happens if robots take the jobs? The impact of emerging technologies
on employment and public policy’, Centre for Technology Innovation at Brookings, Washington
DC.
1 out of 12
![[object Object]](/_next/image/?url=%2F_next%2Fstatic%2Fmedia%2Flogo.6d15ce61.png&w=640&q=75)
Your All-in-One AI-Powered Toolkit for Academic Success.
+13062052269
info@desklib.com
Available 24*7 on WhatsApp / Email
Unlock your academic potential
© 2024 | Zucol Services PVT LTD | All rights reserved.