Convolution Neural Networks Research 2022
VerifiedAdded on 2022/09/16
|10
|2688
|35
AI Summary
Contribute Materials
Your contribution can guide someone’s learning journey. Share your
documents today.
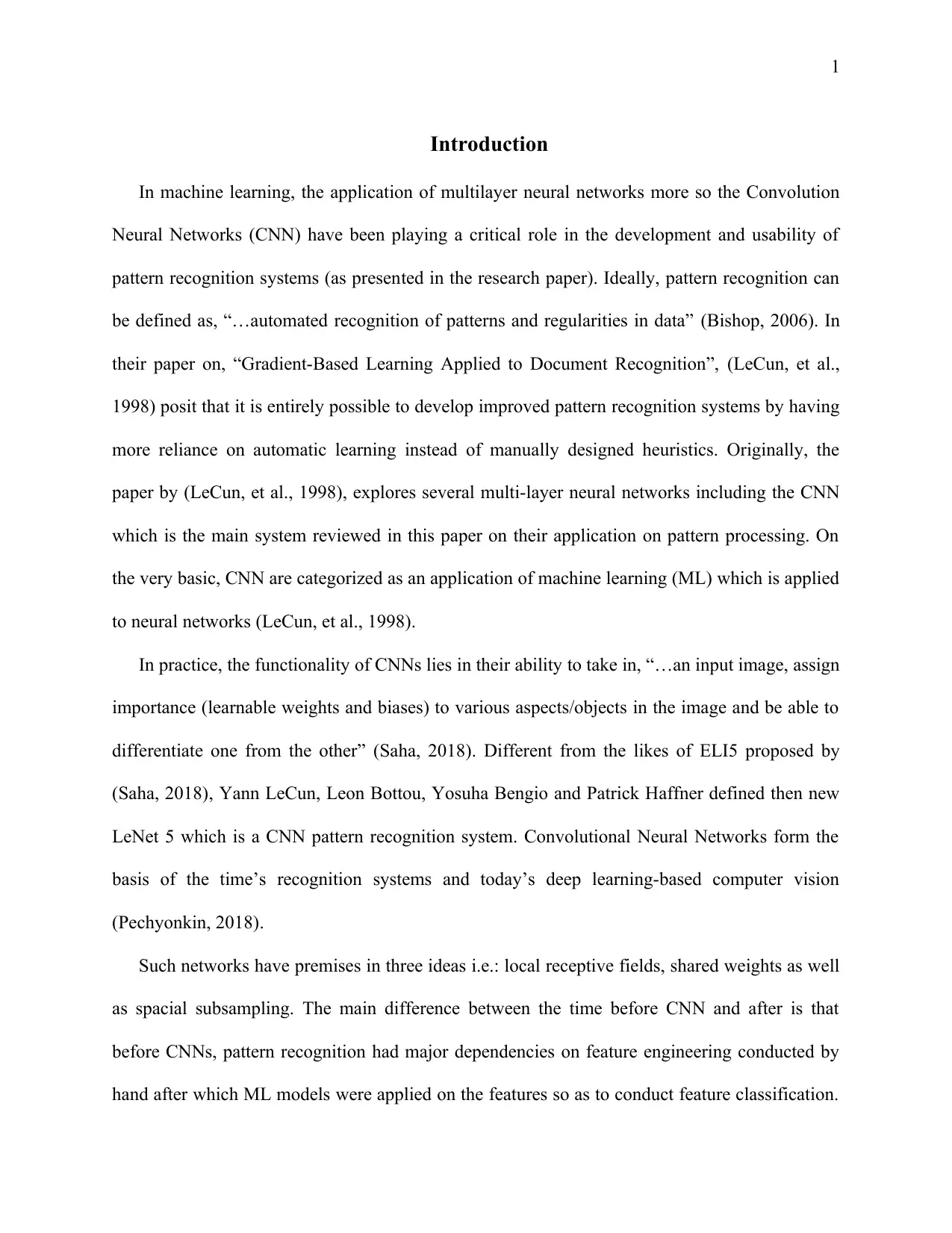
1
Introduction
In machine learning, the application of multilayer neural networks more so the Convolution
Neural Networks (CNN) have been playing a critical role in the development and usability of
pattern recognition systems (as presented in the research paper). Ideally, pattern recognition can
be defined as, “…automated recognition of patterns and regularities in data” (Bishop, 2006). In
their paper on, “Gradient-Based Learning Applied to Document Recognition”, (LeCun, et al.,
1998) posit that it is entirely possible to develop improved pattern recognition systems by having
more reliance on automatic learning instead of manually designed heuristics. Originally, the
paper by (LeCun, et al., 1998), explores several multi-layer neural networks including the CNN
which is the main system reviewed in this paper on their application on pattern processing. On
the very basic, CNN are categorized as an application of machine learning (ML) which is applied
to neural networks (LeCun, et al., 1998).
In practice, the functionality of CNNs lies in their ability to take in, “…an input image, assign
importance (learnable weights and biases) to various aspects/objects in the image and be able to
differentiate one from the other” (Saha, 2018). Different from the likes of ELI5 proposed by
(Saha, 2018), Yann LeCun, Leon Bottou, Yosuha Bengio and Patrick Haffner defined then new
LeNet 5 which is a CNN pattern recognition system. Convolutional Neural Networks form the
basis of the time’s recognition systems and today’s deep learning-based computer vision
(Pechyonkin, 2018).
Such networks have premises in three ideas i.e.: local receptive fields, shared weights as well
as spacial subsampling. The main difference between the time before CNN and after is that
before CNNs, pattern recognition had major dependencies on feature engineering conducted by
hand after which ML models were applied on the features so as to conduct feature classification.
Introduction
In machine learning, the application of multilayer neural networks more so the Convolution
Neural Networks (CNN) have been playing a critical role in the development and usability of
pattern recognition systems (as presented in the research paper). Ideally, pattern recognition can
be defined as, “…automated recognition of patterns and regularities in data” (Bishop, 2006). In
their paper on, “Gradient-Based Learning Applied to Document Recognition”, (LeCun, et al.,
1998) posit that it is entirely possible to develop improved pattern recognition systems by having
more reliance on automatic learning instead of manually designed heuristics. Originally, the
paper by (LeCun, et al., 1998), explores several multi-layer neural networks including the CNN
which is the main system reviewed in this paper on their application on pattern processing. On
the very basic, CNN are categorized as an application of machine learning (ML) which is applied
to neural networks (LeCun, et al., 1998).
In practice, the functionality of CNNs lies in their ability to take in, “…an input image, assign
importance (learnable weights and biases) to various aspects/objects in the image and be able to
differentiate one from the other” (Saha, 2018). Different from the likes of ELI5 proposed by
(Saha, 2018), Yann LeCun, Leon Bottou, Yosuha Bengio and Patrick Haffner defined then new
LeNet 5 which is a CNN pattern recognition system. Convolutional Neural Networks form the
basis of the time’s recognition systems and today’s deep learning-based computer vision
(Pechyonkin, 2018).
Such networks have premises in three ideas i.e.: local receptive fields, shared weights as well
as spacial subsampling. The main difference between the time before CNN and after is that
before CNNs, pattern recognition had major dependencies on feature engineering conducted by
hand after which ML models were applied on the features so as to conduct feature classification.
Secure Best Marks with AI Grader
Need help grading? Try our AI Grader for instant feedback on your assignments.
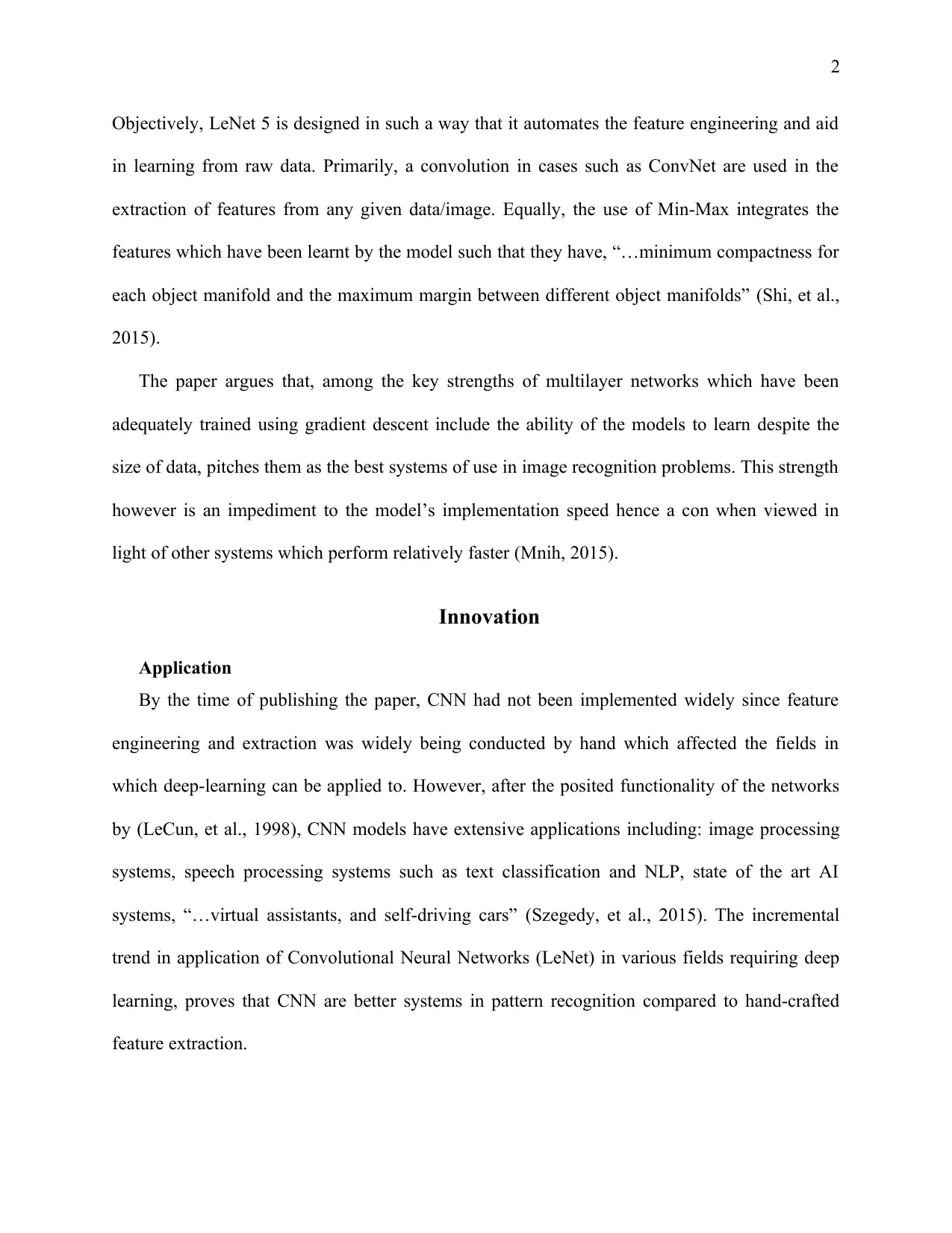
2
Objectively, LeNet 5 is designed in such a way that it automates the feature engineering and aid
in learning from raw data. Primarily, a convolution in cases such as ConvNet are used in the
extraction of features from any given data/image. Equally, the use of Min-Max integrates the
features which have been learnt by the model such that they have, “…minimum compactness for
each object manifold and the maximum margin between different object manifolds” (Shi, et al.,
2015).
The paper argues that, among the key strengths of multilayer networks which have been
adequately trained using gradient descent include the ability of the models to learn despite the
size of data, pitches them as the best systems of use in image recognition problems. This strength
however is an impediment to the model’s implementation speed hence a con when viewed in
light of other systems which perform relatively faster (Mnih, 2015).
Innovation
Application
By the time of publishing the paper, CNN had not been implemented widely since feature
engineering and extraction was widely being conducted by hand which affected the fields in
which deep-learning can be applied to. However, after the posited functionality of the networks
by (LeCun, et al., 1998), CNN models have extensive applications including: image processing
systems, speech processing systems such as text classification and NLP, state of the art AI
systems, “…virtual assistants, and self-driving cars” (Szegedy, et al., 2015). The incremental
trend in application of Convolutional Neural Networks (LeNet) in various fields requiring deep
learning, proves that CNN are better systems in pattern recognition compared to hand-crafted
feature extraction.
Objectively, LeNet 5 is designed in such a way that it automates the feature engineering and aid
in learning from raw data. Primarily, a convolution in cases such as ConvNet are used in the
extraction of features from any given data/image. Equally, the use of Min-Max integrates the
features which have been learnt by the model such that they have, “…minimum compactness for
each object manifold and the maximum margin between different object manifolds” (Shi, et al.,
2015).
The paper argues that, among the key strengths of multilayer networks which have been
adequately trained using gradient descent include the ability of the models to learn despite the
size of data, pitches them as the best systems of use in image recognition problems. This strength
however is an impediment to the model’s implementation speed hence a con when viewed in
light of other systems which perform relatively faster (Mnih, 2015).
Innovation
Application
By the time of publishing the paper, CNN had not been implemented widely since feature
engineering and extraction was widely being conducted by hand which affected the fields in
which deep-learning can be applied to. However, after the posited functionality of the networks
by (LeCun, et al., 1998), CNN models have extensive applications including: image processing
systems, speech processing systems such as text classification and NLP, state of the art AI
systems, “…virtual assistants, and self-driving cars” (Szegedy, et al., 2015). The incremental
trend in application of Convolutional Neural Networks (LeNet) in various fields requiring deep
learning, proves that CNN are better systems in pattern recognition compared to hand-crafted
feature extraction.
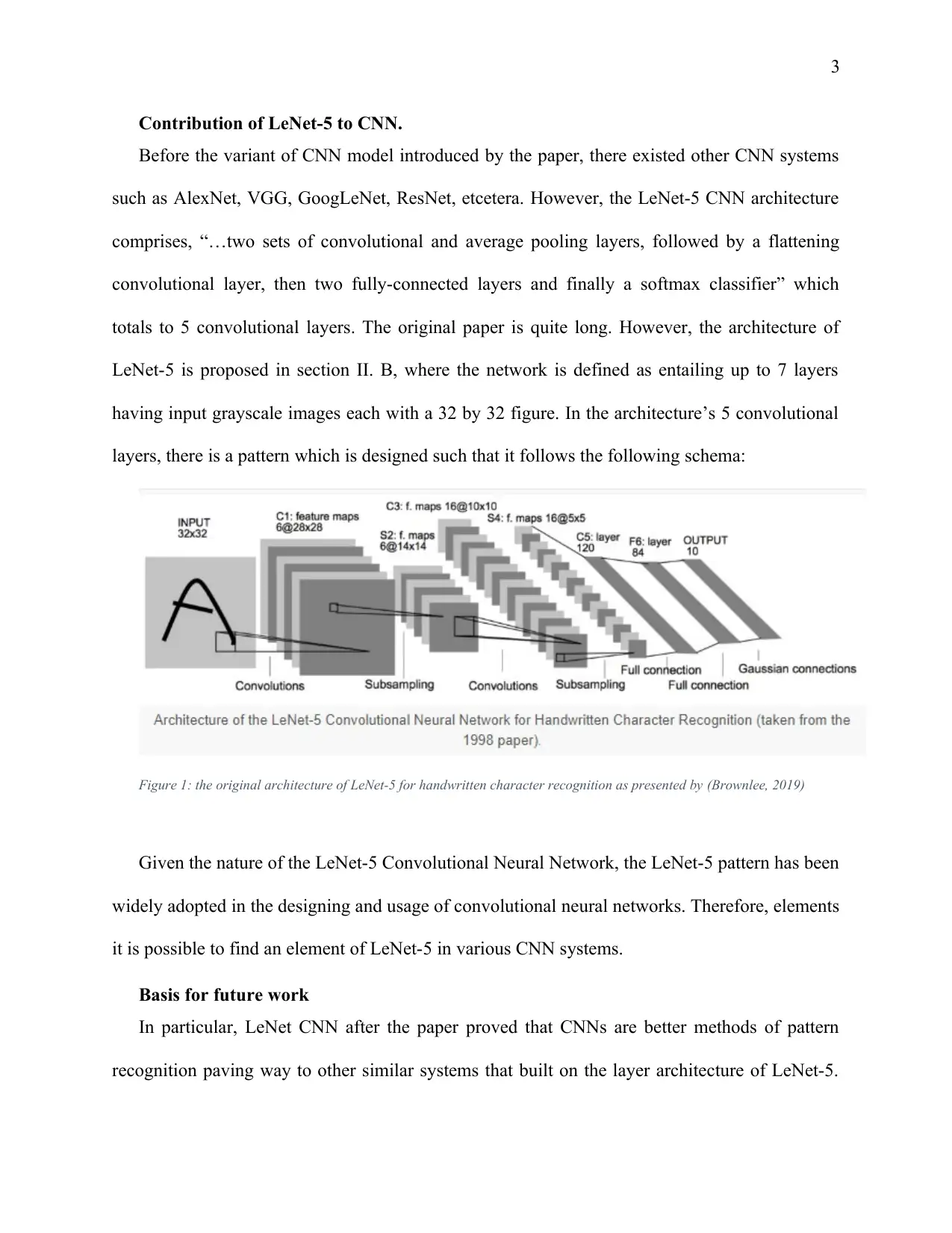
3
Contribution of LeNet-5 to CNN.
Before the variant of CNN model introduced by the paper, there existed other CNN systems
such as AlexNet, VGG, GoogLeNet, ResNet, etcetera. However, the LeNet-5 CNN architecture
comprises, “…two sets of convolutional and average pooling layers, followed by a flattening
convolutional layer, then two fully-connected layers and finally a softmax classifier” which
totals to 5 convolutional layers. The original paper is quite long. However, the architecture of
LeNet-5 is proposed in section II. B, where the network is defined as entailing up to 7 layers
having input grayscale images each with a 32 by 32 figure. In the architecture’s 5 convolutional
layers, there is a pattern which is designed such that it follows the following schema:
Figure 1: the original architecture of LeNet-5 for handwritten character recognition as presented by (Brownlee, 2019)
Given the nature of the LeNet-5 Convolutional Neural Network, the LeNet-5 pattern has been
widely adopted in the designing and usage of convolutional neural networks. Therefore, elements
it is possible to find an element of LeNet-5 in various CNN systems.
Basis for future work
In particular, LeNet CNN after the paper proved that CNNs are better methods of pattern
recognition paving way to other similar systems that built on the layer architecture of LeNet-5.
Contribution of LeNet-5 to CNN.
Before the variant of CNN model introduced by the paper, there existed other CNN systems
such as AlexNet, VGG, GoogLeNet, ResNet, etcetera. However, the LeNet-5 CNN architecture
comprises, “…two sets of convolutional and average pooling layers, followed by a flattening
convolutional layer, then two fully-connected layers and finally a softmax classifier” which
totals to 5 convolutional layers. The original paper is quite long. However, the architecture of
LeNet-5 is proposed in section II. B, where the network is defined as entailing up to 7 layers
having input grayscale images each with a 32 by 32 figure. In the architecture’s 5 convolutional
layers, there is a pattern which is designed such that it follows the following schema:
Figure 1: the original architecture of LeNet-5 for handwritten character recognition as presented by (Brownlee, 2019)
Given the nature of the LeNet-5 Convolutional Neural Network, the LeNet-5 pattern has been
widely adopted in the designing and usage of convolutional neural networks. Therefore, elements
it is possible to find an element of LeNet-5 in various CNN systems.
Basis for future work
In particular, LeNet CNN after the paper proved that CNNs are better methods of pattern
recognition paving way to other similar systems that built on the layer architecture of LeNet-5.
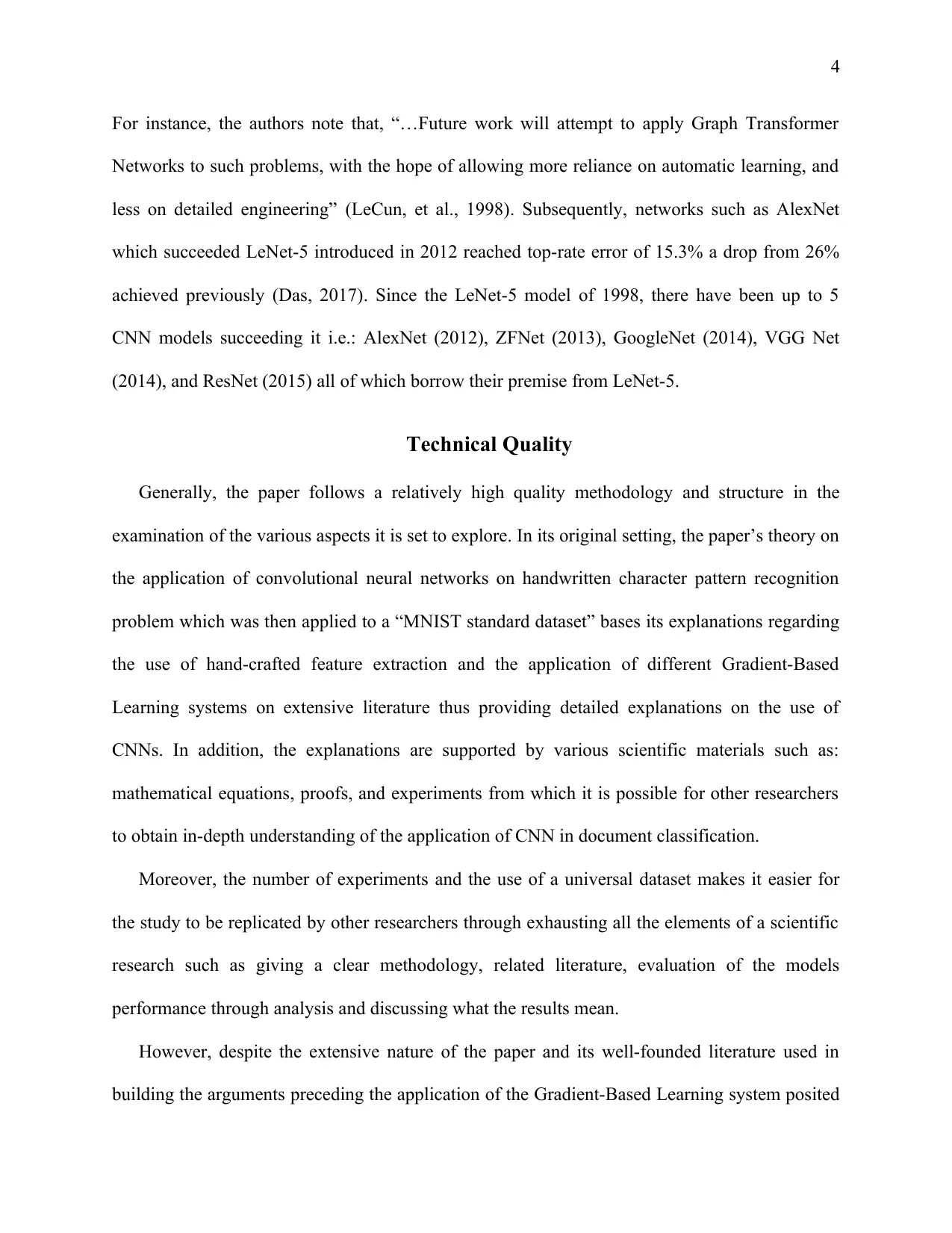
4
For instance, the authors note that, “…Future work will attempt to apply Graph Transformer
Networks to such problems, with the hope of allowing more reliance on automatic learning, and
less on detailed engineering” (LeCun, et al., 1998). Subsequently, networks such as AlexNet
which succeeded LeNet-5 introduced in 2012 reached top-rate error of 15.3% a drop from 26%
achieved previously (Das, 2017). Since the LeNet-5 model of 1998, there have been up to 5
CNN models succeeding it i.e.: AlexNet (2012), ZFNet (2013), GoogleNet (2014), VGG Net
(2014), and ResNet (2015) all of which borrow their premise from LeNet-5.
Technical Quality
Generally, the paper follows a relatively high quality methodology and structure in the
examination of the various aspects it is set to explore. In its original setting, the paper’s theory on
the application of convolutional neural networks on handwritten character pattern recognition
problem which was then applied to a “MNIST standard dataset” bases its explanations regarding
the use of hand-crafted feature extraction and the application of different Gradient-Based
Learning systems on extensive literature thus providing detailed explanations on the use of
CNNs. In addition, the explanations are supported by various scientific materials such as:
mathematical equations, proofs, and experiments from which it is possible for other researchers
to obtain in-depth understanding of the application of CNN in document classification.
Moreover, the number of experiments and the use of a universal dataset makes it easier for
the study to be replicated by other researchers through exhausting all the elements of a scientific
research such as giving a clear methodology, related literature, evaluation of the models
performance through analysis and discussing what the results mean.
However, despite the extensive nature of the paper and its well-founded literature used in
building the arguments preceding the application of the Gradient-Based Learning system posited
For instance, the authors note that, “…Future work will attempt to apply Graph Transformer
Networks to such problems, with the hope of allowing more reliance on automatic learning, and
less on detailed engineering” (LeCun, et al., 1998). Subsequently, networks such as AlexNet
which succeeded LeNet-5 introduced in 2012 reached top-rate error of 15.3% a drop from 26%
achieved previously (Das, 2017). Since the LeNet-5 model of 1998, there have been up to 5
CNN models succeeding it i.e.: AlexNet (2012), ZFNet (2013), GoogleNet (2014), VGG Net
(2014), and ResNet (2015) all of which borrow their premise from LeNet-5.
Technical Quality
Generally, the paper follows a relatively high quality methodology and structure in the
examination of the various aspects it is set to explore. In its original setting, the paper’s theory on
the application of convolutional neural networks on handwritten character pattern recognition
problem which was then applied to a “MNIST standard dataset” bases its explanations regarding
the use of hand-crafted feature extraction and the application of different Gradient-Based
Learning systems on extensive literature thus providing detailed explanations on the use of
CNNs. In addition, the explanations are supported by various scientific materials such as:
mathematical equations, proofs, and experiments from which it is possible for other researchers
to obtain in-depth understanding of the application of CNN in document classification.
Moreover, the number of experiments and the use of a universal dataset makes it easier for
the study to be replicated by other researchers through exhausting all the elements of a scientific
research such as giving a clear methodology, related literature, evaluation of the models
performance through analysis and discussing what the results mean.
However, despite the extensive nature of the paper and its well-founded literature used in
building the arguments preceding the application of the Gradient-Based Learning system posited
Secure Best Marks with AI Grader
Need help grading? Try our AI Grader for instant feedback on your assignments.
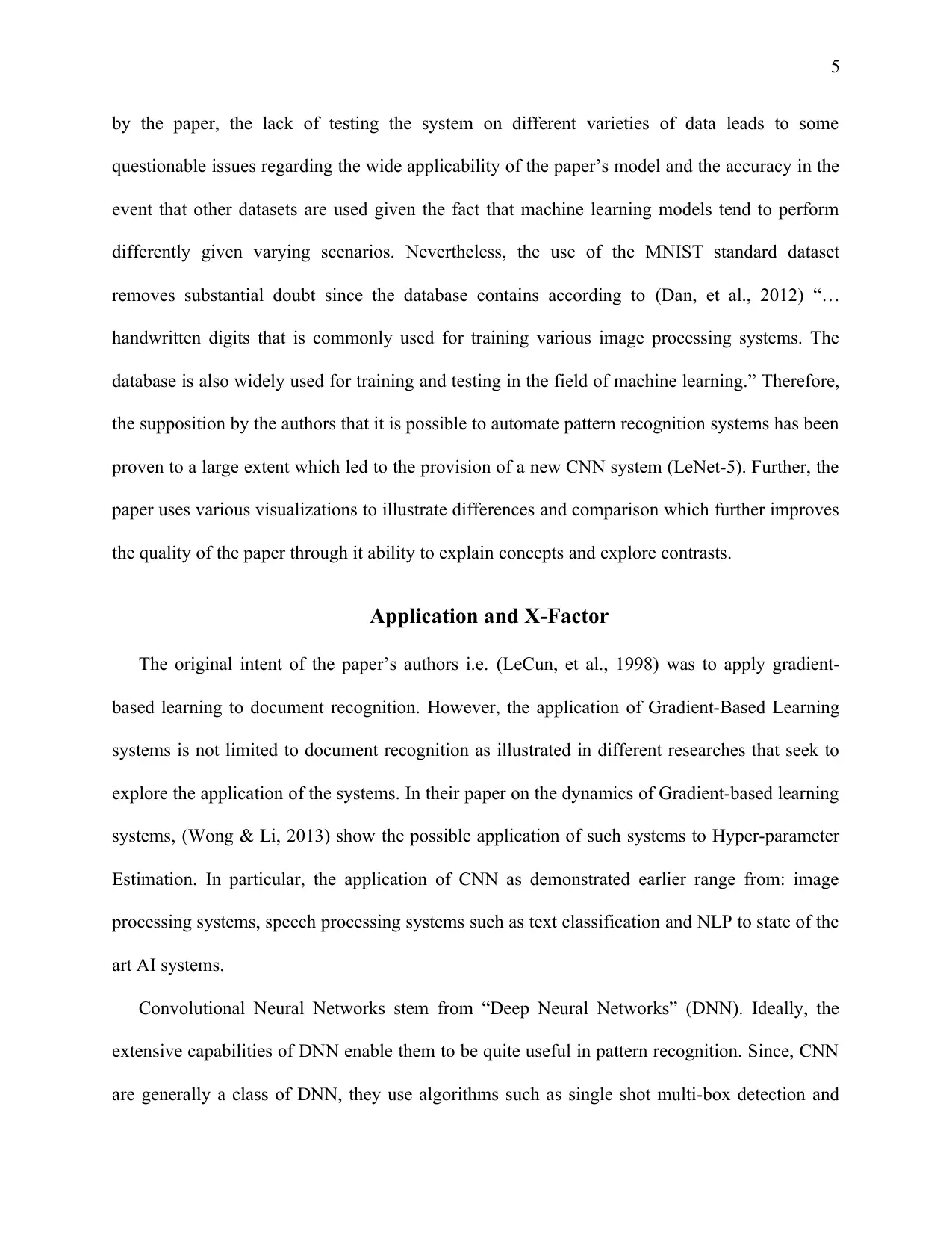
5
by the paper, the lack of testing the system on different varieties of data leads to some
questionable issues regarding the wide applicability of the paper’s model and the accuracy in the
event that other datasets are used given the fact that machine learning models tend to perform
differently given varying scenarios. Nevertheless, the use of the MNIST standard dataset
removes substantial doubt since the database contains according to (Dan, et al., 2012) “…
handwritten digits that is commonly used for training various image processing systems. The
database is also widely used for training and testing in the field of machine learning.” Therefore,
the supposition by the authors that it is possible to automate pattern recognition systems has been
proven to a large extent which led to the provision of a new CNN system (LeNet-5). Further, the
paper uses various visualizations to illustrate differences and comparison which further improves
the quality of the paper through it ability to explain concepts and explore contrasts.
Application and X-Factor
The original intent of the paper’s authors i.e. (LeCun, et al., 1998) was to apply gradient-
based learning to document recognition. However, the application of Gradient-Based Learning
systems is not limited to document recognition as illustrated in different researches that seek to
explore the application of the systems. In their paper on the dynamics of Gradient-based learning
systems, (Wong & Li, 2013) show the possible application of such systems to Hyper-parameter
Estimation. In particular, the application of CNN as demonstrated earlier range from: image
processing systems, speech processing systems such as text classification and NLP to state of the
art AI systems.
Convolutional Neural Networks stem from “Deep Neural Networks” (DNN). Ideally, the
extensive capabilities of DNN enable them to be quite useful in pattern recognition. Since, CNN
are generally a class of DNN, they use algorithms such as single shot multi-box detection and
by the paper, the lack of testing the system on different varieties of data leads to some
questionable issues regarding the wide applicability of the paper’s model and the accuracy in the
event that other datasets are used given the fact that machine learning models tend to perform
differently given varying scenarios. Nevertheless, the use of the MNIST standard dataset
removes substantial doubt since the database contains according to (Dan, et al., 2012) “…
handwritten digits that is commonly used for training various image processing systems. The
database is also widely used for training and testing in the field of machine learning.” Therefore,
the supposition by the authors that it is possible to automate pattern recognition systems has been
proven to a large extent which led to the provision of a new CNN system (LeNet-5). Further, the
paper uses various visualizations to illustrate differences and comparison which further improves
the quality of the paper through it ability to explain concepts and explore contrasts.
Application and X-Factor
The original intent of the paper’s authors i.e. (LeCun, et al., 1998) was to apply gradient-
based learning to document recognition. However, the application of Gradient-Based Learning
systems is not limited to document recognition as illustrated in different researches that seek to
explore the application of the systems. In their paper on the dynamics of Gradient-based learning
systems, (Wong & Li, 2013) show the possible application of such systems to Hyper-parameter
Estimation. In particular, the application of CNN as demonstrated earlier range from: image
processing systems, speech processing systems such as text classification and NLP to state of the
art AI systems.
Convolutional Neural Networks stem from “Deep Neural Networks” (DNN). Ideally, the
extensive capabilities of DNN enable them to be quite useful in pattern recognition. Since, CNN
are generally a class of DNN, they use algorithms such as single shot multi-box detection and
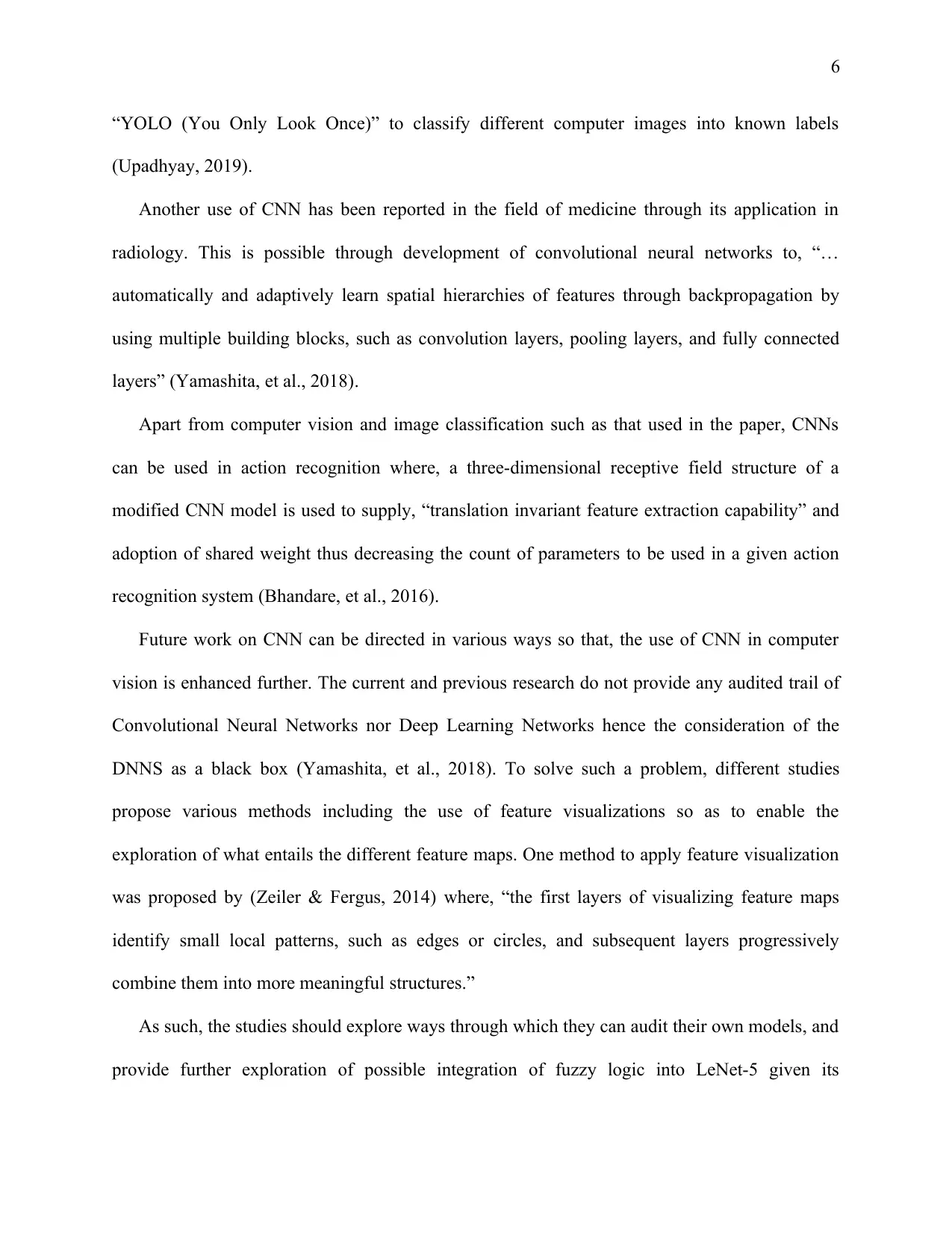
6
“YOLO (You Only Look Once)” to classify different computer images into known labels
(Upadhyay, 2019).
Another use of CNN has been reported in the field of medicine through its application in
radiology. This is possible through development of convolutional neural networks to, “…
automatically and adaptively learn spatial hierarchies of features through backpropagation by
using multiple building blocks, such as convolution layers, pooling layers, and fully connected
layers” (Yamashita, et al., 2018).
Apart from computer vision and image classification such as that used in the paper, CNNs
can be used in action recognition where, a three-dimensional receptive field structure of a
modified CNN model is used to supply, “translation invariant feature extraction capability” and
adoption of shared weight thus decreasing the count of parameters to be used in a given action
recognition system (Bhandare, et al., 2016).
Future work on CNN can be directed in various ways so that, the use of CNN in computer
vision is enhanced further. The current and previous research do not provide any audited trail of
Convolutional Neural Networks nor Deep Learning Networks hence the consideration of the
DNNS as a black box (Yamashita, et al., 2018). To solve such a problem, different studies
propose various methods including the use of feature visualizations so as to enable the
exploration of what entails the different feature maps. One method to apply feature visualization
was proposed by (Zeiler & Fergus, 2014) where, “the first layers of visualizing feature maps
identify small local patterns, such as edges or circles, and subsequent layers progressively
combine them into more meaningful structures.”
As such, the studies should explore ways through which they can audit their own models, and
provide further exploration of possible integration of fuzzy logic into LeNet-5 given its
“YOLO (You Only Look Once)” to classify different computer images into known labels
(Upadhyay, 2019).
Another use of CNN has been reported in the field of medicine through its application in
radiology. This is possible through development of convolutional neural networks to, “…
automatically and adaptively learn spatial hierarchies of features through backpropagation by
using multiple building blocks, such as convolution layers, pooling layers, and fully connected
layers” (Yamashita, et al., 2018).
Apart from computer vision and image classification such as that used in the paper, CNNs
can be used in action recognition where, a three-dimensional receptive field structure of a
modified CNN model is used to supply, “translation invariant feature extraction capability” and
adoption of shared weight thus decreasing the count of parameters to be used in a given action
recognition system (Bhandare, et al., 2016).
Future work on CNN can be directed in various ways so that, the use of CNN in computer
vision is enhanced further. The current and previous research do not provide any audited trail of
Convolutional Neural Networks nor Deep Learning Networks hence the consideration of the
DNNS as a black box (Yamashita, et al., 2018). To solve such a problem, different studies
propose various methods including the use of feature visualizations so as to enable the
exploration of what entails the different feature maps. One method to apply feature visualization
was proposed by (Zeiler & Fergus, 2014) where, “the first layers of visualizing feature maps
identify small local patterns, such as edges or circles, and subsequent layers progressively
combine them into more meaningful structures.”
As such, the studies should explore ways through which they can audit their own models, and
provide further exploration of possible integration of fuzzy logic into LeNet-5 given its
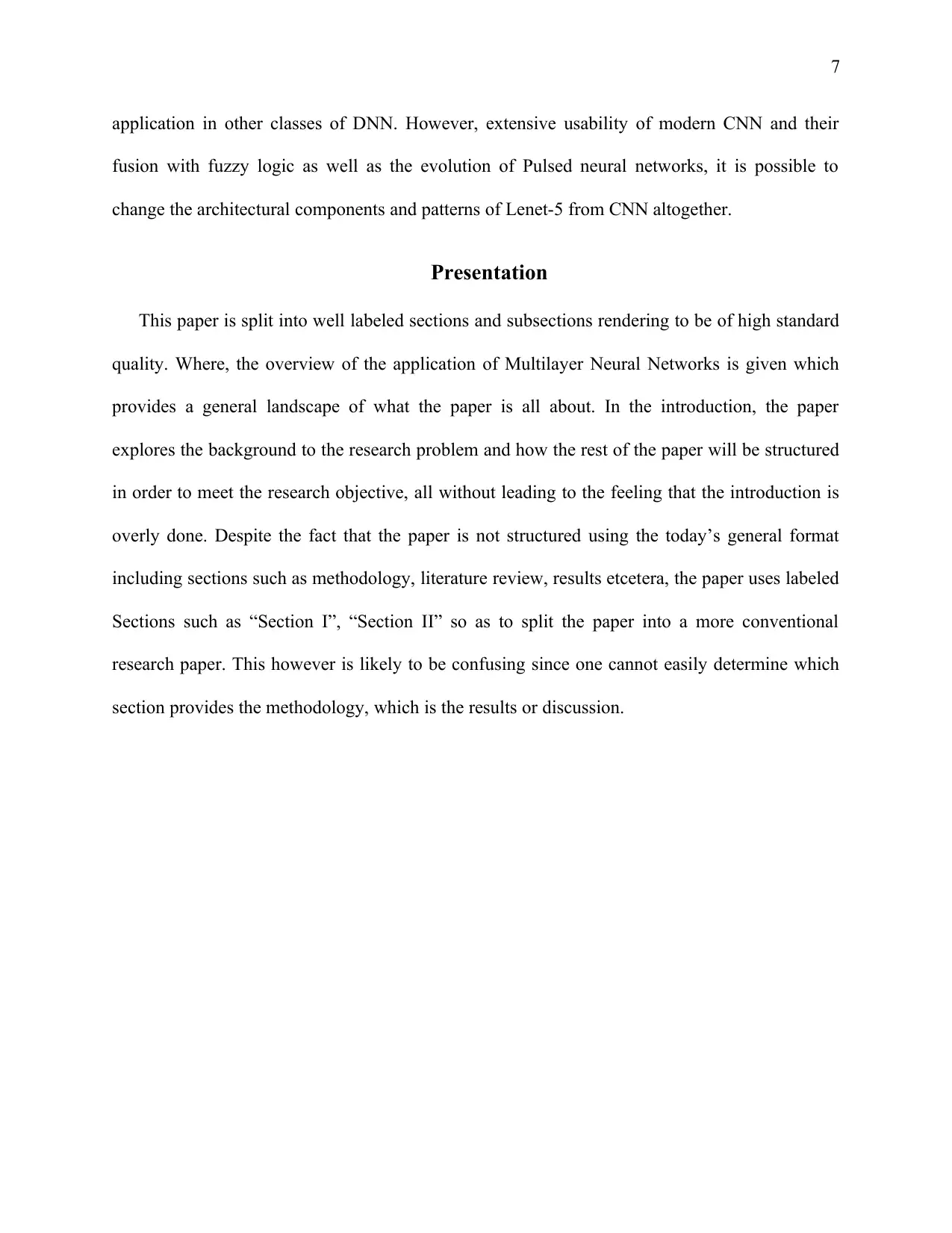
7
application in other classes of DNN. However, extensive usability of modern CNN and their
fusion with fuzzy logic as well as the evolution of Pulsed neural networks, it is possible to
change the architectural components and patterns of Lenet-5 from CNN altogether.
Presentation
This paper is split into well labeled sections and subsections rendering to be of high standard
quality. Where, the overview of the application of Multilayer Neural Networks is given which
provides a general landscape of what the paper is all about. In the introduction, the paper
explores the background to the research problem and how the rest of the paper will be structured
in order to meet the research objective, all without leading to the feeling that the introduction is
overly done. Despite the fact that the paper is not structured using the today’s general format
including sections such as methodology, literature review, results etcetera, the paper uses labeled
Sections such as “Section I”, “Section II” so as to split the paper into a more conventional
research paper. This however is likely to be confusing since one cannot easily determine which
section provides the methodology, which is the results or discussion.
application in other classes of DNN. However, extensive usability of modern CNN and their
fusion with fuzzy logic as well as the evolution of Pulsed neural networks, it is possible to
change the architectural components and patterns of Lenet-5 from CNN altogether.
Presentation
This paper is split into well labeled sections and subsections rendering to be of high standard
quality. Where, the overview of the application of Multilayer Neural Networks is given which
provides a general landscape of what the paper is all about. In the introduction, the paper
explores the background to the research problem and how the rest of the paper will be structured
in order to meet the research objective, all without leading to the feeling that the introduction is
overly done. Despite the fact that the paper is not structured using the today’s general format
including sections such as methodology, literature review, results etcetera, the paper uses labeled
Sections such as “Section I”, “Section II” so as to split the paper into a more conventional
research paper. This however is likely to be confusing since one cannot easily determine which
section provides the methodology, which is the results or discussion.
Paraphrase This Document
Need a fresh take? Get an instant paraphrase of this document with our AI Paraphraser
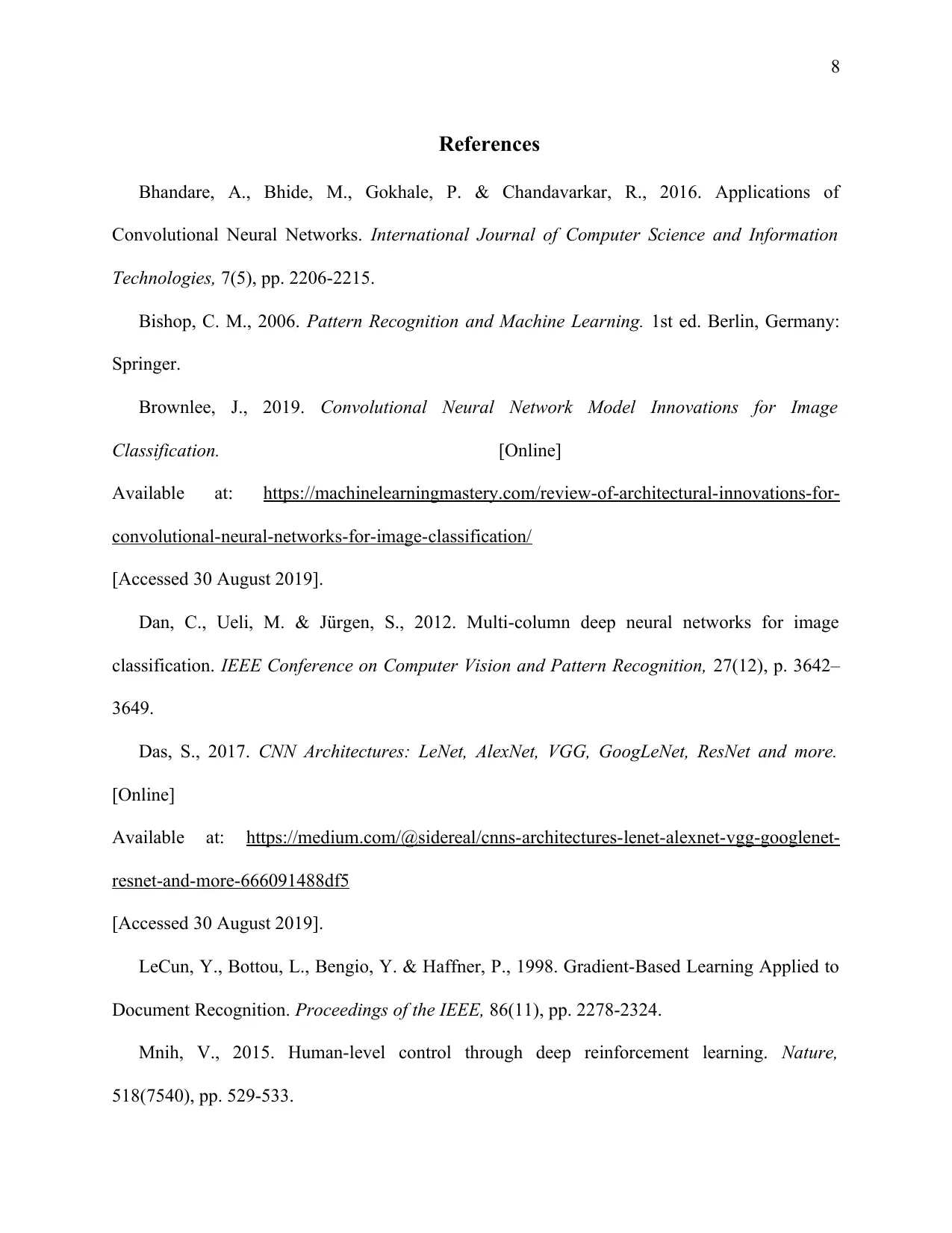
8
References
Bhandare, A., Bhide, M., Gokhale, P. & Chandavarkar, R., 2016. Applications of
Convolutional Neural Networks. International Journal of Computer Science and Information
Technologies, 7(5), pp. 2206-2215.
Bishop, C. M., 2006. Pattern Recognition and Machine Learning. 1st ed. Berlin, Germany:
Springer.
Brownlee, J., 2019. Convolutional Neural Network Model Innovations for Image
Classification. [Online]
Available at: https://machinelearningmastery.com/review-of-architectural-innovations-for-
convolutional-neural-networks-for-image-classification/
[Accessed 30 August 2019].
Dan, C., Ueli, M. & Jürgen, S., 2012. Multi-column deep neural networks for image
classification. IEEE Conference on Computer Vision and Pattern Recognition, 27(12), p. 3642–
3649.
Das, S., 2017. CNN Architectures: LeNet, AlexNet, VGG, GoogLeNet, ResNet and more.
[Online]
Available at: https://medium.com/@sidereal/cnns-architectures-lenet-alexnet-vgg-googlenet-
resnet-and-more-666091488df5
[Accessed 30 August 2019].
LeCun, Y., Bottou, L., Bengio, Y. & Haffner, P., 1998. Gradient-Based Learning Applied to
Document Recognition. Proceedings of the IEEE, 86(11), pp. 2278-2324.
Mnih, V., 2015. Human-level control through deep reinforcement learning. Nature,
518(7540), pp. 529-533.
References
Bhandare, A., Bhide, M., Gokhale, P. & Chandavarkar, R., 2016. Applications of
Convolutional Neural Networks. International Journal of Computer Science and Information
Technologies, 7(5), pp. 2206-2215.
Bishop, C. M., 2006. Pattern Recognition and Machine Learning. 1st ed. Berlin, Germany:
Springer.
Brownlee, J., 2019. Convolutional Neural Network Model Innovations for Image
Classification. [Online]
Available at: https://machinelearningmastery.com/review-of-architectural-innovations-for-
convolutional-neural-networks-for-image-classification/
[Accessed 30 August 2019].
Dan, C., Ueli, M. & Jürgen, S., 2012. Multi-column deep neural networks for image
classification. IEEE Conference on Computer Vision and Pattern Recognition, 27(12), p. 3642–
3649.
Das, S., 2017. CNN Architectures: LeNet, AlexNet, VGG, GoogLeNet, ResNet and more.
[Online]
Available at: https://medium.com/@sidereal/cnns-architectures-lenet-alexnet-vgg-googlenet-
resnet-and-more-666091488df5
[Accessed 30 August 2019].
LeCun, Y., Bottou, L., Bengio, Y. & Haffner, P., 1998. Gradient-Based Learning Applied to
Document Recognition. Proceedings of the IEEE, 86(11), pp. 2278-2324.
Mnih, V., 2015. Human-level control through deep reinforcement learning. Nature,
518(7540), pp. 529-533.
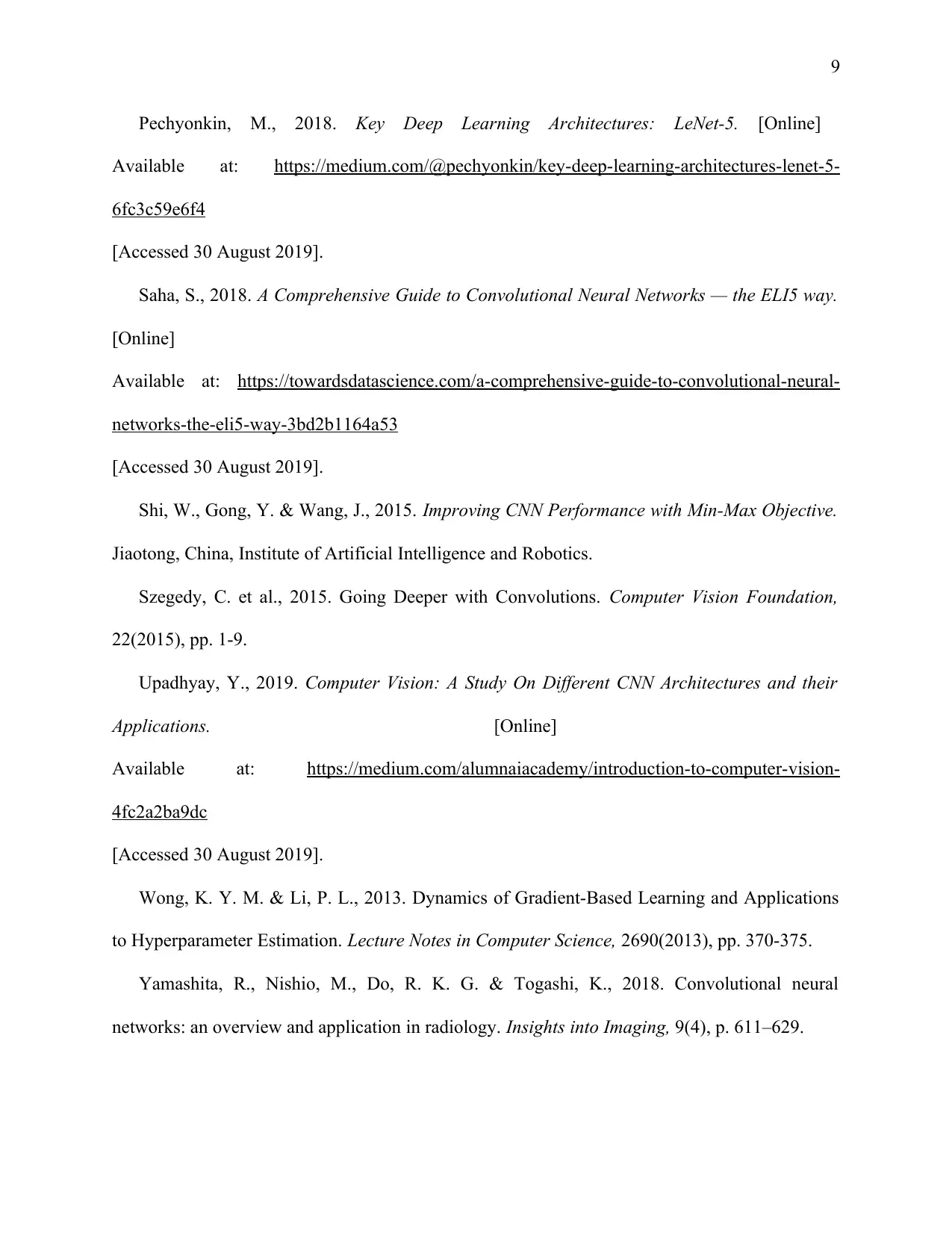
9
Pechyonkin, M., 2018. Key Deep Learning Architectures: LeNet-5. [Online]
Available at: https://medium.com/@pechyonkin/key-deep-learning-architectures-lenet-5-
6fc3c59e6f4
[Accessed 30 August 2019].
Saha, S., 2018. A Comprehensive Guide to Convolutional Neural Networks — the ELI5 way.
[Online]
Available at: https://towardsdatascience.com/a-comprehensive-guide-to-convolutional-neural-
networks-the-eli5-way-3bd2b1164a53
[Accessed 30 August 2019].
Shi, W., Gong, Y. & Wang, J., 2015. Improving CNN Performance with Min-Max Objective.
Jiaotong, China, Institute of Artificial Intelligence and Robotics.
Szegedy, C. et al., 2015. Going Deeper with Convolutions. Computer Vision Foundation,
22(2015), pp. 1-9.
Upadhyay, Y., 2019. Computer Vision: A Study On Different CNN Architectures and their
Applications. [Online]
Available at: https://medium.com/alumnaiacademy/introduction-to-computer-vision-
4fc2a2ba9dc
[Accessed 30 August 2019].
Wong, K. Y. M. & Li, P. L., 2013. Dynamics of Gradient-Based Learning and Applications
to Hyperparameter Estimation. Lecture Notes in Computer Science, 2690(2013), pp. 370-375.
Yamashita, R., Nishio, M., Do, R. K. G. & Togashi, K., 2018. Convolutional neural
networks: an overview and application in radiology. Insights into Imaging, 9(4), p. 611–629.
Pechyonkin, M., 2018. Key Deep Learning Architectures: LeNet-5. [Online]
Available at: https://medium.com/@pechyonkin/key-deep-learning-architectures-lenet-5-
6fc3c59e6f4
[Accessed 30 August 2019].
Saha, S., 2018. A Comprehensive Guide to Convolutional Neural Networks — the ELI5 way.
[Online]
Available at: https://towardsdatascience.com/a-comprehensive-guide-to-convolutional-neural-
networks-the-eli5-way-3bd2b1164a53
[Accessed 30 August 2019].
Shi, W., Gong, Y. & Wang, J., 2015. Improving CNN Performance with Min-Max Objective.
Jiaotong, China, Institute of Artificial Intelligence and Robotics.
Szegedy, C. et al., 2015. Going Deeper with Convolutions. Computer Vision Foundation,
22(2015), pp. 1-9.
Upadhyay, Y., 2019. Computer Vision: A Study On Different CNN Architectures and their
Applications. [Online]
Available at: https://medium.com/alumnaiacademy/introduction-to-computer-vision-
4fc2a2ba9dc
[Accessed 30 August 2019].
Wong, K. Y. M. & Li, P. L., 2013. Dynamics of Gradient-Based Learning and Applications
to Hyperparameter Estimation. Lecture Notes in Computer Science, 2690(2013), pp. 370-375.
Yamashita, R., Nishio, M., Do, R. K. G. & Togashi, K., 2018. Convolutional neural
networks: an overview and application in radiology. Insights into Imaging, 9(4), p. 611–629.
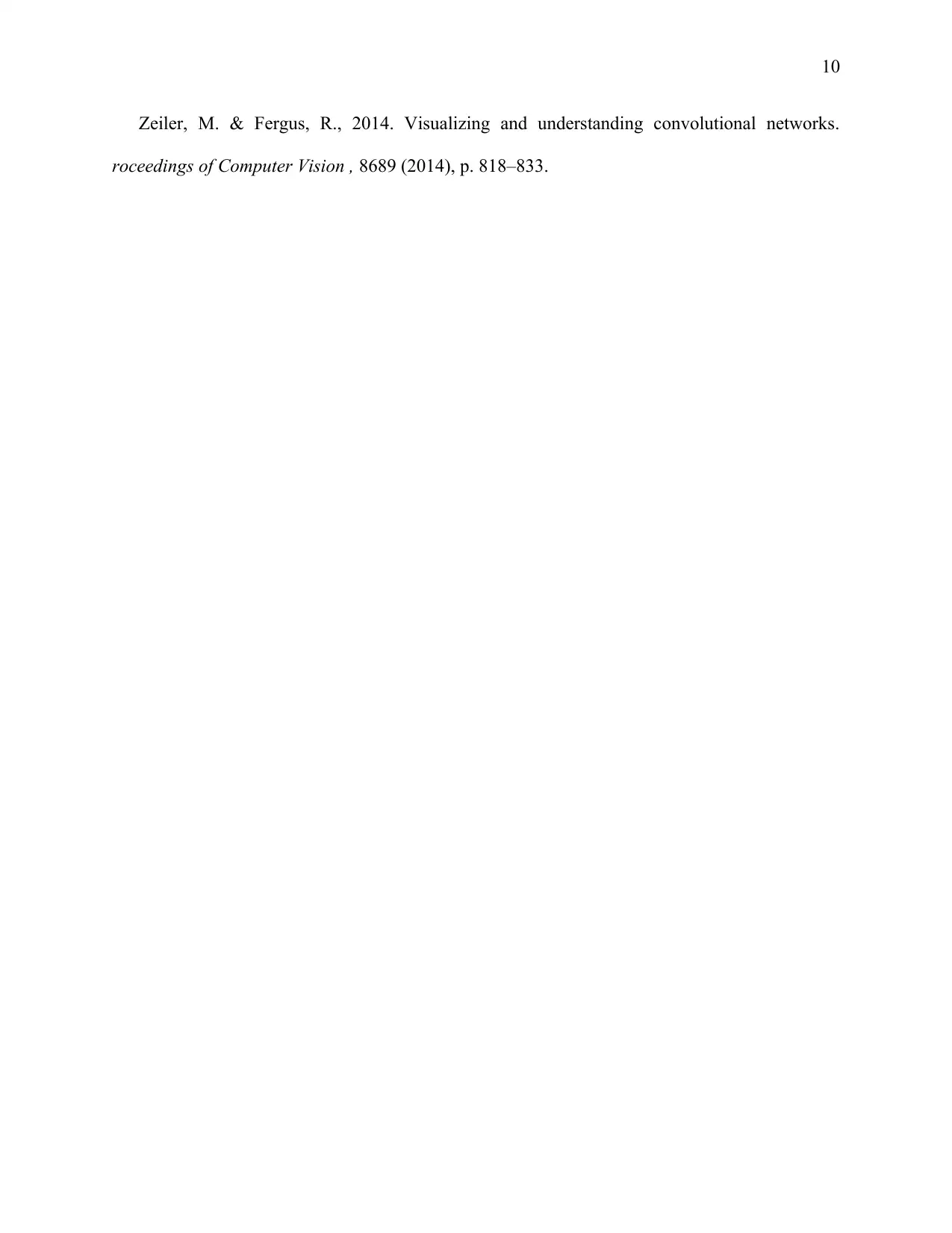
10
Zeiler, M. & Fergus, R., 2014. Visualizing and understanding convolutional networks.
roceedings of Computer Vision , 8689 (2014), p. 818–833.
Zeiler, M. & Fergus, R., 2014. Visualizing and understanding convolutional networks.
roceedings of Computer Vision , 8689 (2014), p. 818–833.
1 out of 10
Related Documents
![[object Object]](/_next/image/?url=%2F_next%2Fstatic%2Fmedia%2Flogo.6d15ce61.png&w=640&q=75)
Your All-in-One AI-Powered Toolkit for Academic Success.
+13062052269
info@desklib.com
Available 24*7 on WhatsApp / Email
Unlock your academic potential
© 2024 | Zucol Services PVT LTD | All rights reserved.