Data Analysis Using Rapid Miner | Report
VerifiedAdded on  2022/08/21
|11
|821
|15
AI Summary
Contribute Materials
Your contribution can guide someone’s learning journey. Share your
documents today.
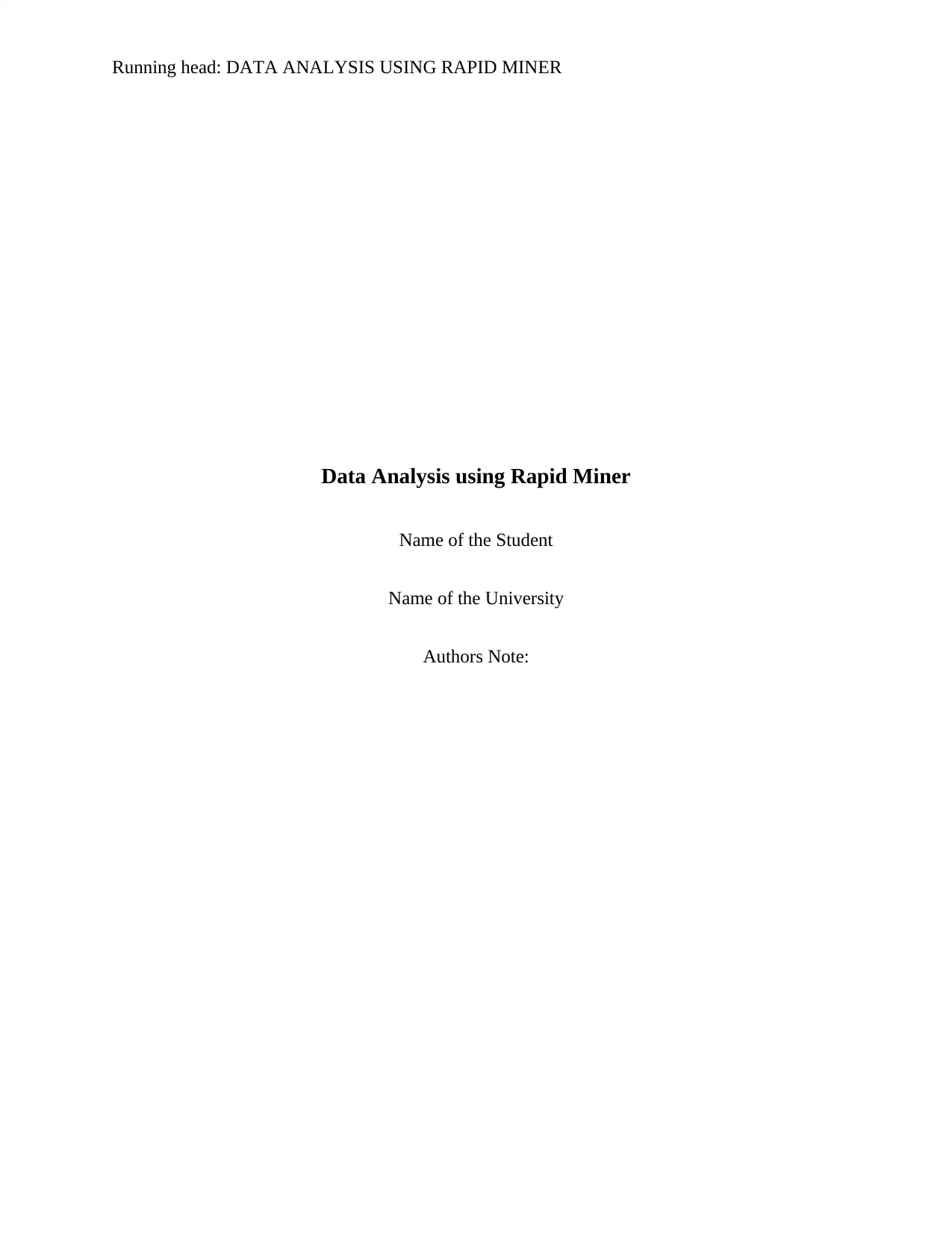
Running head: DATA ANALYSIS USING RAPID MINER
Data Analysis using Rapid Miner
Name of the Student
Name of the University
Authors Note:
Data Analysis using Rapid Miner
Name of the Student
Name of the University
Authors Note:
Secure Best Marks with AI Grader
Need help grading? Try our AI Grader for instant feedback on your assignments.
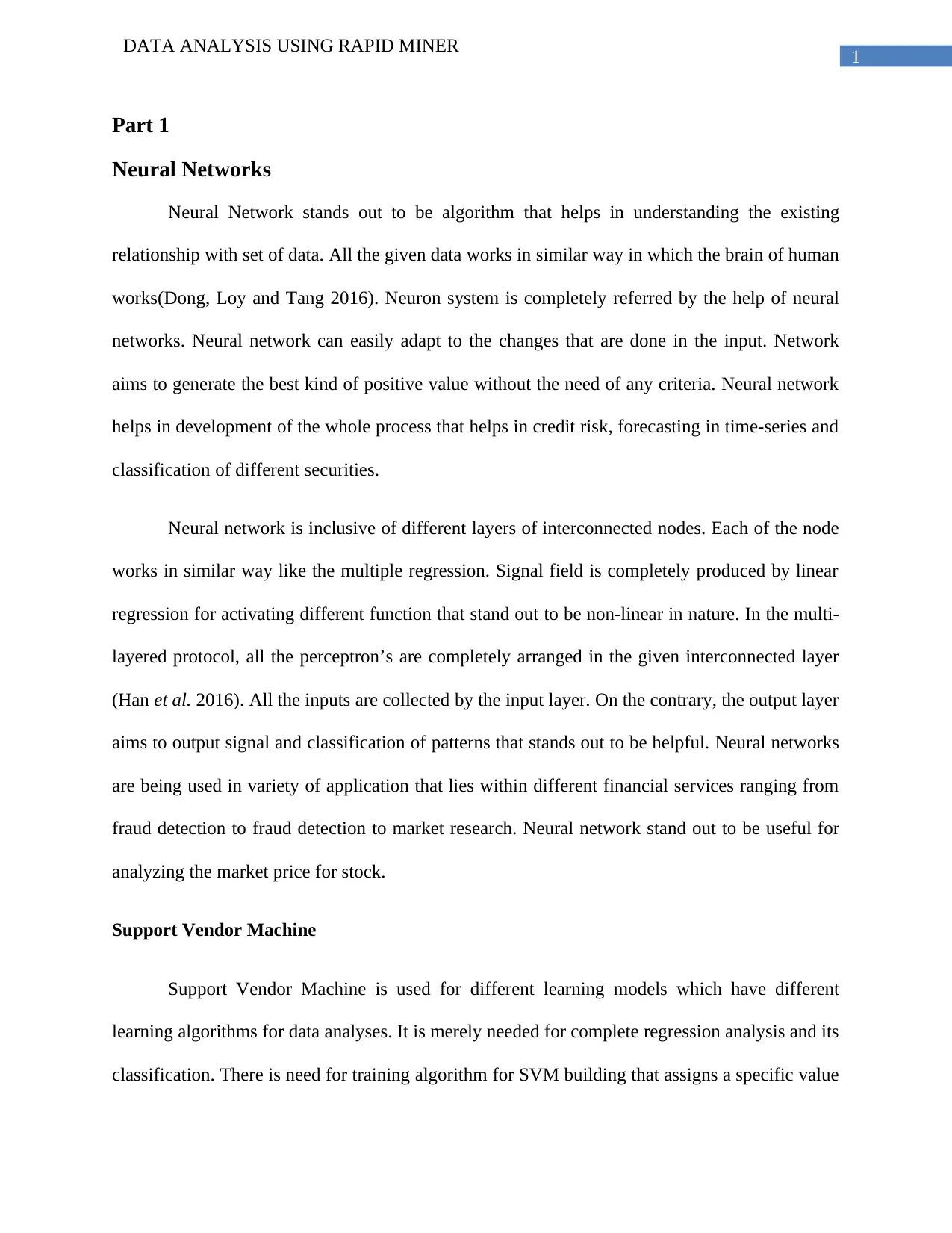
1
DATA ANALYSIS USING RAPID MINER
Part 1
Neural Networks
Neural Network stands out to be algorithm that helps in understanding the existing
relationship with set of data. All the given data works in similar way in which the brain of human
works(Dong, Loy and Tang 2016). Neuron system is completely referred by the help of neural
networks. Neural network can easily adapt to the changes that are done in the input. Network
aims to generate the best kind of positive value without the need of any criteria. Neural network
helps in development of the whole process that helps in credit risk, forecasting in time-series and
classification of different securities.
Neural network is inclusive of different layers of interconnected nodes. Each of the node
works in similar way like the multiple regression. Signal field is completely produced by linear
regression for activating different function that stand out to be non-linear in nature. In the multi-
layered protocol, all the perceptron’s are completely arranged in the given interconnected layer
(Han et al. 2016). All the inputs are collected by the input layer. On the contrary, the output layer
aims to output signal and classification of patterns that stands out to be helpful. Neural networks
are being used in variety of application that lies within different financial services ranging from
fraud detection to fraud detection to market research. Neural network stand out to be useful for
analyzing the market price for stock.
Support Vendor Machine
Support Vendor Machine is used for different learning models which have different
learning algorithms for data analyses. It is merely needed for complete regression analysis and its
classification. There is need for training algorithm for SVM building that assigns a specific value
DATA ANALYSIS USING RAPID MINER
Part 1
Neural Networks
Neural Network stands out to be algorithm that helps in understanding the existing
relationship with set of data. All the given data works in similar way in which the brain of human
works(Dong, Loy and Tang 2016). Neuron system is completely referred by the help of neural
networks. Neural network can easily adapt to the changes that are done in the input. Network
aims to generate the best kind of positive value without the need of any criteria. Neural network
helps in development of the whole process that helps in credit risk, forecasting in time-series and
classification of different securities.
Neural network is inclusive of different layers of interconnected nodes. Each of the node
works in similar way like the multiple regression. Signal field is completely produced by linear
regression for activating different function that stand out to be non-linear in nature. In the multi-
layered protocol, all the perceptron’s are completely arranged in the given interconnected layer
(Han et al. 2016). All the inputs are collected by the input layer. On the contrary, the output layer
aims to output signal and classification of patterns that stands out to be helpful. Neural networks
are being used in variety of application that lies within different financial services ranging from
fraud detection to fraud detection to market research. Neural network stand out to be useful for
analyzing the market price for stock.
Support Vendor Machine
Support Vendor Machine is used for different learning models which have different
learning algorithms for data analyses. It is merely needed for complete regression analysis and its
classification. There is need for training algorithm for SVM building that assigns a specific value
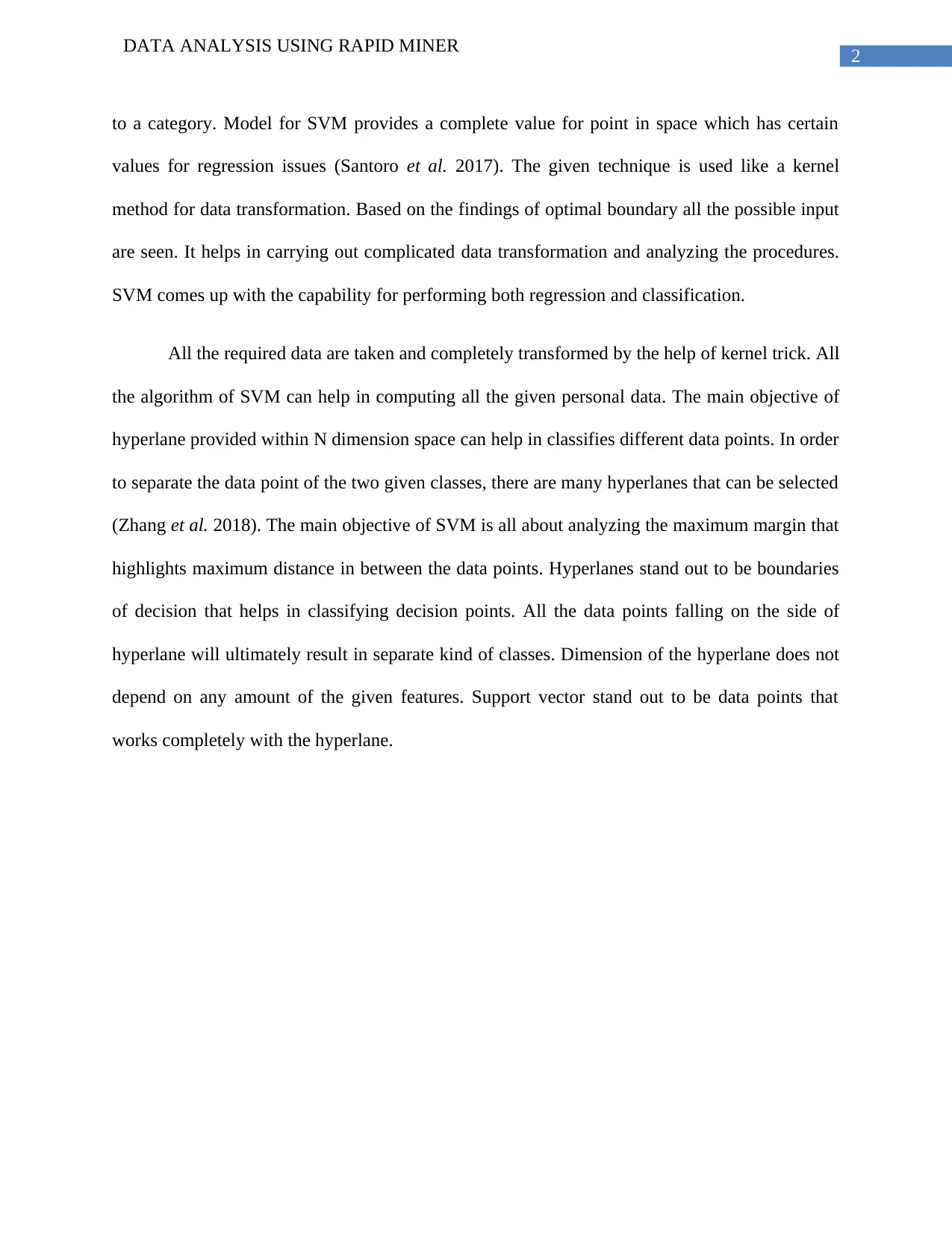
2
DATA ANALYSIS USING RAPID MINER
to a category. Model for SVM provides a complete value for point in space which has certain
values for regression issues (Santoro et al. 2017). The given technique is used like a kernel
method for data transformation. Based on the findings of optimal boundary all the possible input
are seen. It helps in carrying out complicated data transformation and analyzing the procedures.
SVM comes up with the capability for performing both regression and classification.
All the required data are taken and completely transformed by the help of kernel trick. All
the algorithm of SVM can help in computing all the given personal data. The main objective of
hyperlane provided within N dimension space can help in classifies different data points. In order
to separate the data point of the two given classes, there are many hyperlanes that can be selected
(Zhang et al. 2018). The main objective of SVM is all about analyzing the maximum margin that
highlights maximum distance in between the data points. Hyperlanes stand out to be boundaries
of decision that helps in classifying decision points. All the data points falling on the side of
hyperlane will ultimately result in separate kind of classes. Dimension of the hyperlane does not
depend on any amount of the given features. Support vector stand out to be data points that
works completely with the hyperlane.
DATA ANALYSIS USING RAPID MINER
to a category. Model for SVM provides a complete value for point in space which has certain
values for regression issues (Santoro et al. 2017). The given technique is used like a kernel
method for data transformation. Based on the findings of optimal boundary all the possible input
are seen. It helps in carrying out complicated data transformation and analyzing the procedures.
SVM comes up with the capability for performing both regression and classification.
All the required data are taken and completely transformed by the help of kernel trick. All
the algorithm of SVM can help in computing all the given personal data. The main objective of
hyperlane provided within N dimension space can help in classifies different data points. In order
to separate the data point of the two given classes, there are many hyperlanes that can be selected
(Zhang et al. 2018). The main objective of SVM is all about analyzing the maximum margin that
highlights maximum distance in between the data points. Hyperlanes stand out to be boundaries
of decision that helps in classifying decision points. All the data points falling on the side of
hyperlane will ultimately result in separate kind of classes. Dimension of the hyperlane does not
depend on any amount of the given features. Support vector stand out to be data points that
works completely with the hyperlane.
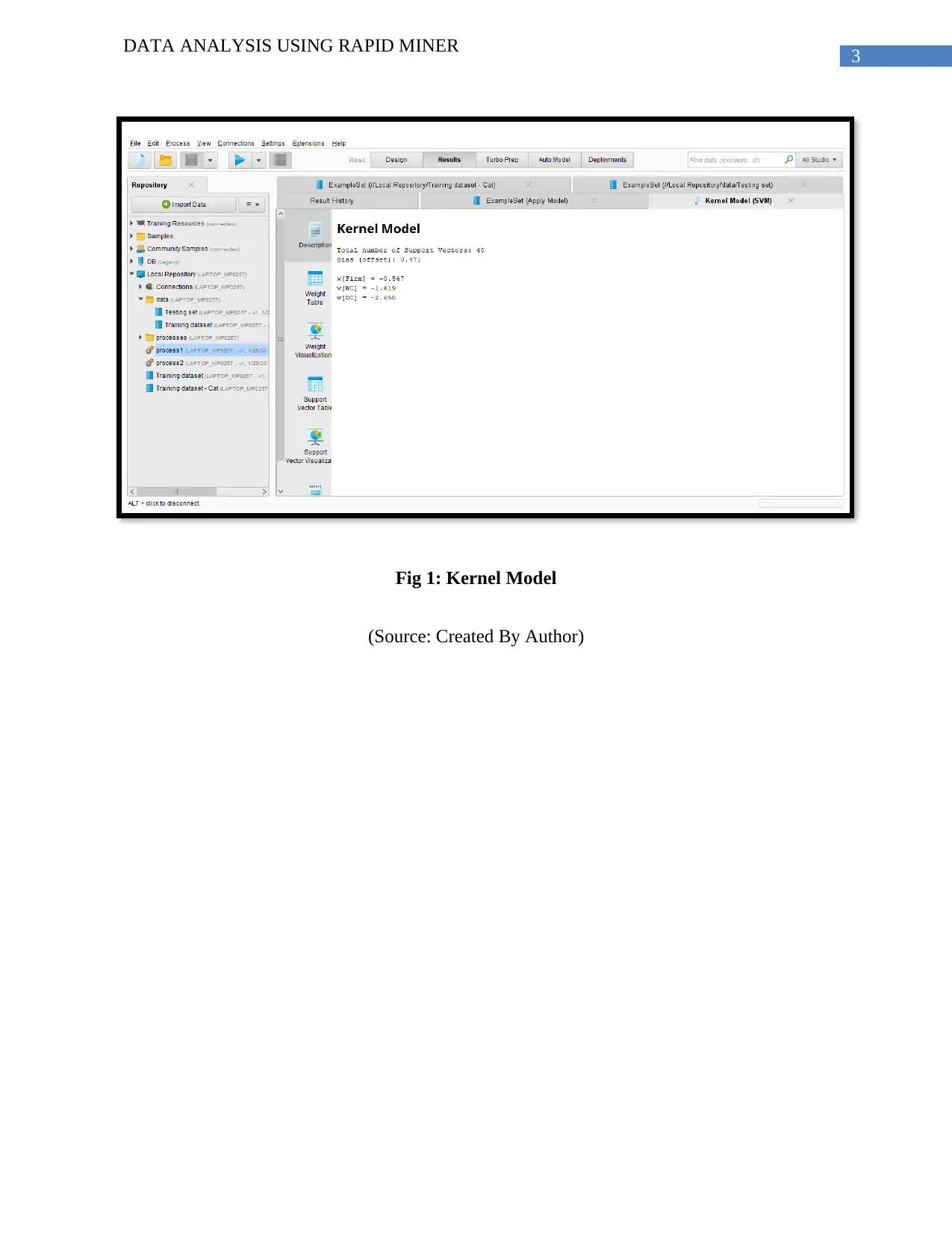
3
DATA ANALYSIS USING RAPID MINER
Fig 1: Kernel Model
(Source: Created By Author)
DATA ANALYSIS USING RAPID MINER
Fig 1: Kernel Model
(Source: Created By Author)
Secure Best Marks with AI Grader
Need help grading? Try our AI Grader for instant feedback on your assignments.
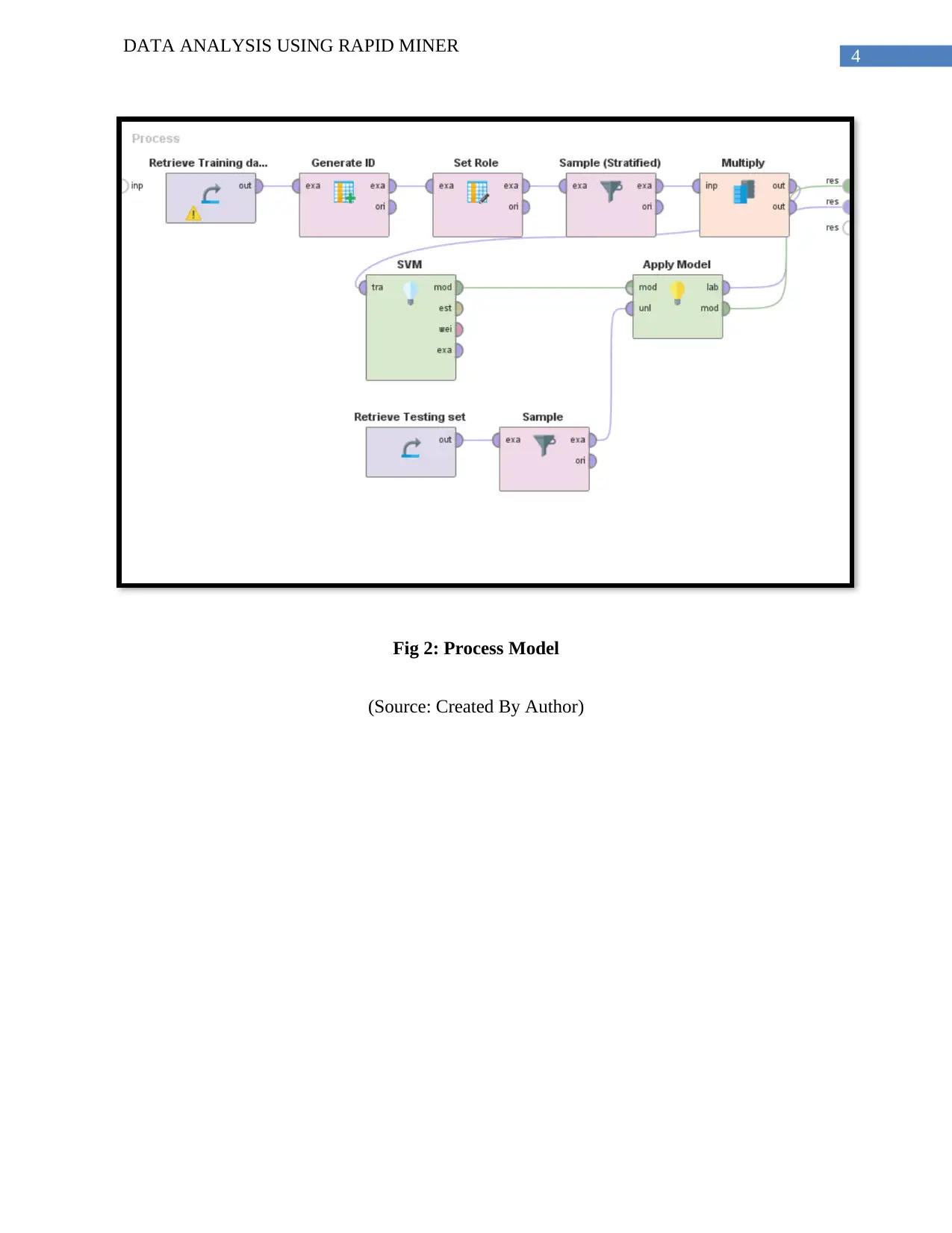
4
DATA ANALYSIS USING RAPID MINER
Fig 2: Process Model
(Source: Created By Author)
DATA ANALYSIS USING RAPID MINER
Fig 2: Process Model
(Source: Created By Author)
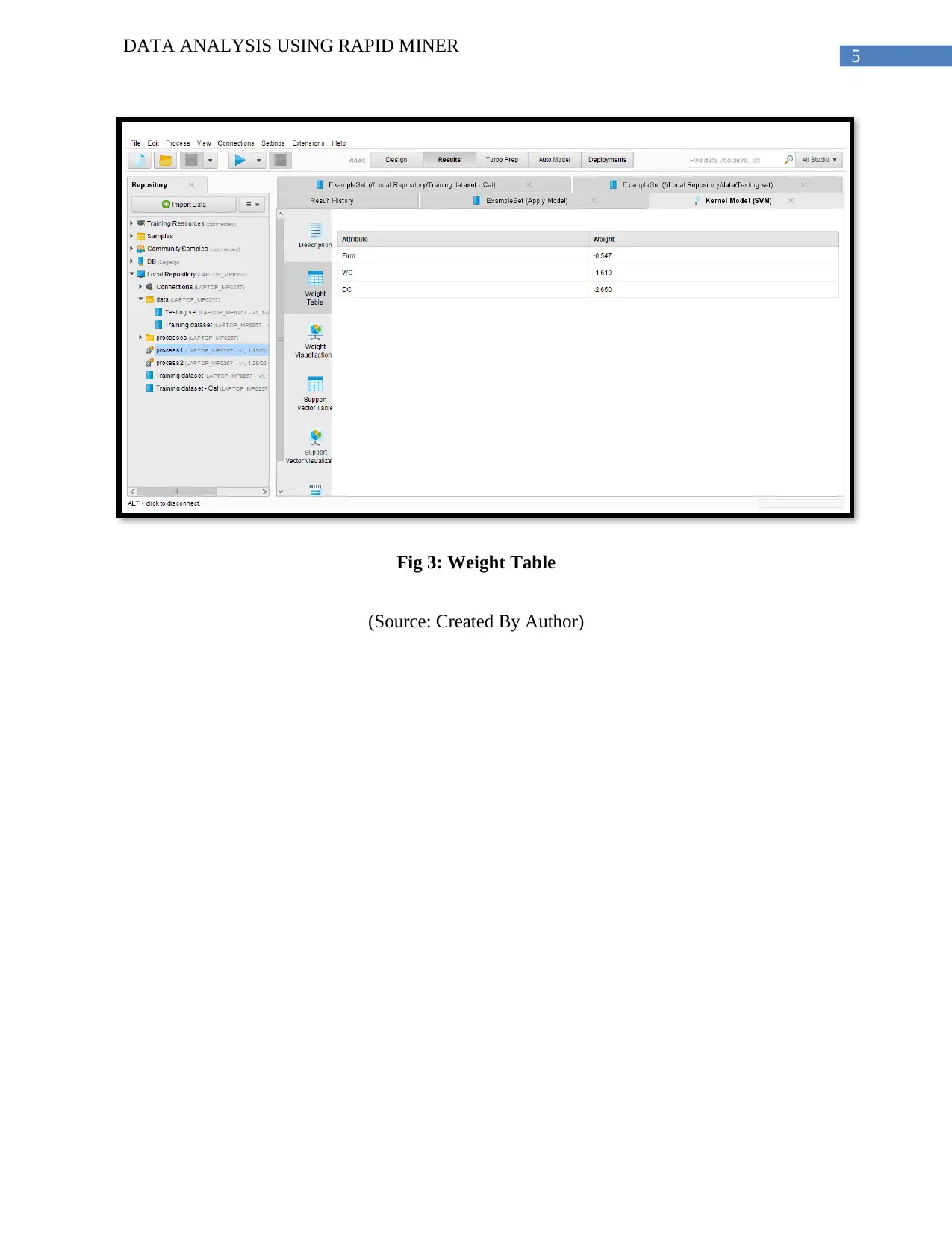
5
DATA ANALYSIS USING RAPID MINER
Fig 3: Weight Table
(Source: Created By Author)
DATA ANALYSIS USING RAPID MINER
Fig 3: Weight Table
(Source: Created By Author)
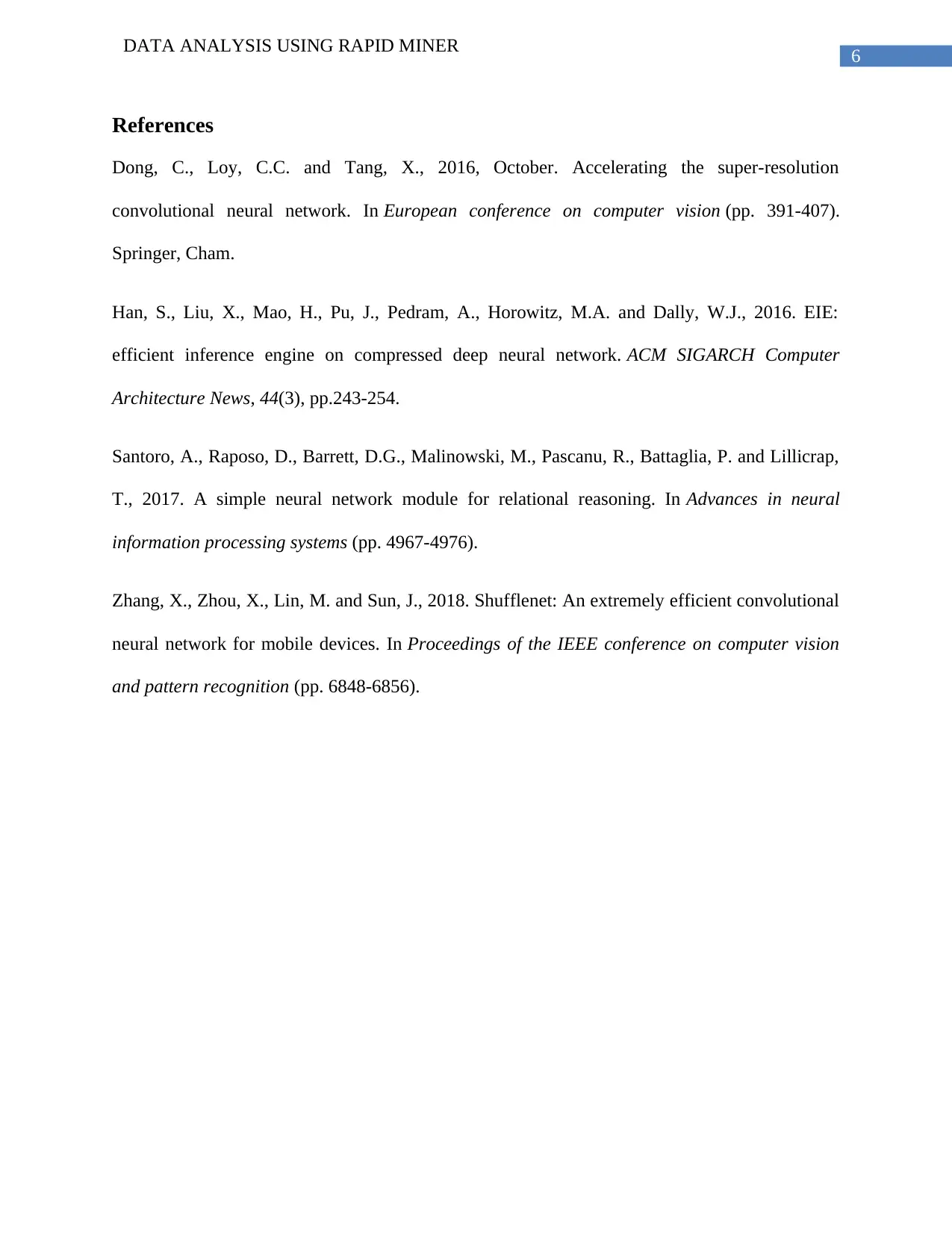
6
DATA ANALYSIS USING RAPID MINER
References
Dong, C., Loy, C.C. and Tang, X., 2016, October. Accelerating the super-resolution
convolutional neural network. In European conference on computer vision (pp. 391-407).
Springer, Cham.
Han, S., Liu, X., Mao, H., Pu, J., Pedram, A., Horowitz, M.A. and Dally, W.J., 2016. EIE:
efficient inference engine on compressed deep neural network. ACM SIGARCH Computer
Architecture News, 44(3), pp.243-254.
Santoro, A., Raposo, D., Barrett, D.G., Malinowski, M., Pascanu, R., Battaglia, P. and Lillicrap,
T., 2017. A simple neural network module for relational reasoning. In Advances in neural
information processing systems (pp. 4967-4976).
Zhang, X., Zhou, X., Lin, M. and Sun, J., 2018. Shufflenet: An extremely efficient convolutional
neural network for mobile devices. In Proceedings of the IEEE conference on computer vision
and pattern recognition (pp. 6848-6856).
DATA ANALYSIS USING RAPID MINER
References
Dong, C., Loy, C.C. and Tang, X., 2016, October. Accelerating the super-resolution
convolutional neural network. In European conference on computer vision (pp. 391-407).
Springer, Cham.
Han, S., Liu, X., Mao, H., Pu, J., Pedram, A., Horowitz, M.A. and Dally, W.J., 2016. EIE:
efficient inference engine on compressed deep neural network. ACM SIGARCH Computer
Architecture News, 44(3), pp.243-254.
Santoro, A., Raposo, D., Barrett, D.G., Malinowski, M., Pascanu, R., Battaglia, P. and Lillicrap,
T., 2017. A simple neural network module for relational reasoning. In Advances in neural
information processing systems (pp. 4967-4976).
Zhang, X., Zhou, X., Lin, M. and Sun, J., 2018. Shufflenet: An extremely efficient convolutional
neural network for mobile devices. In Proceedings of the IEEE conference on computer vision
and pattern recognition (pp. 6848-6856).
Paraphrase This Document
Need a fresh take? Get an instant paraphrase of this document with our AI Paraphraser
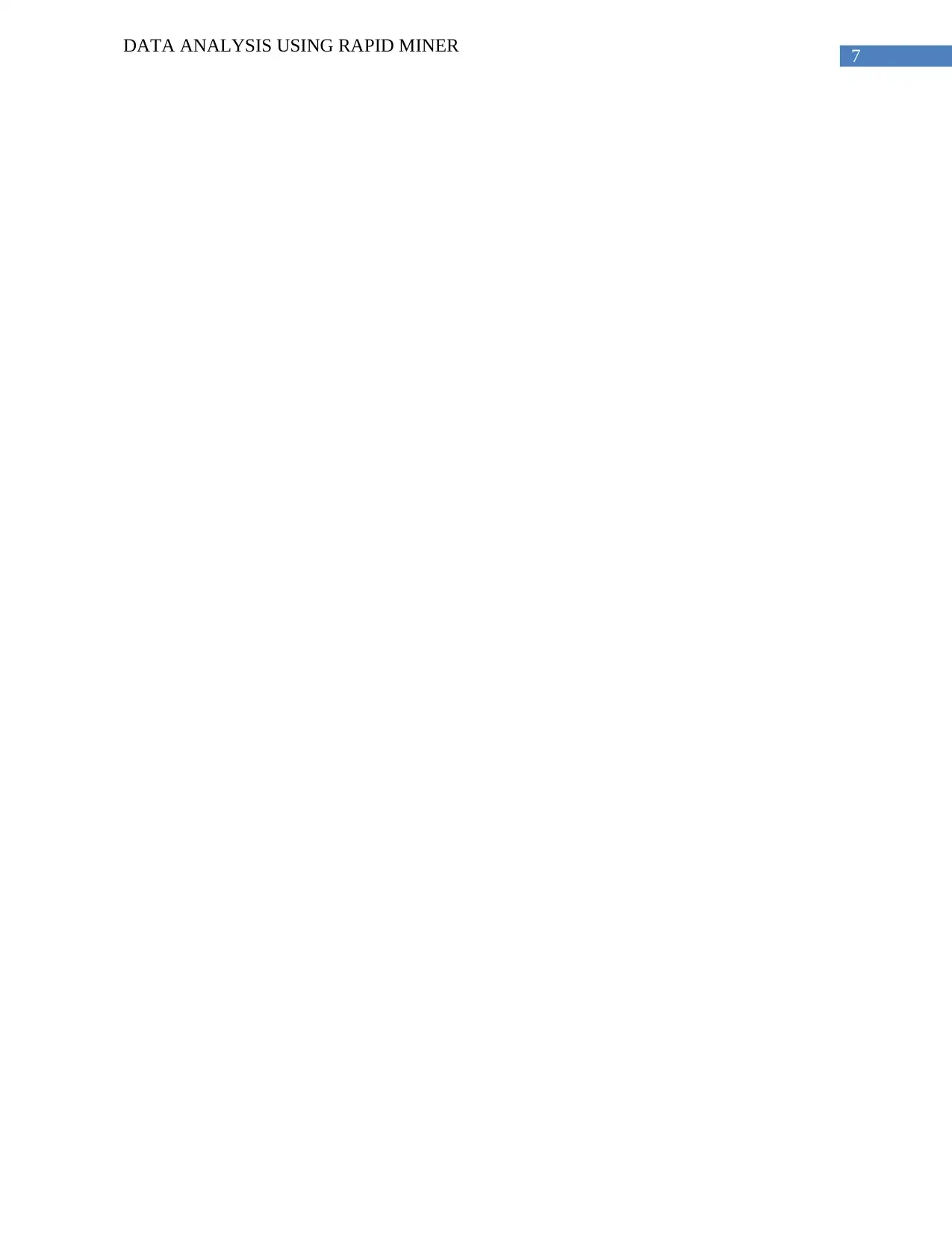
7
DATA ANALYSIS USING RAPID MINER
DATA ANALYSIS USING RAPID MINER
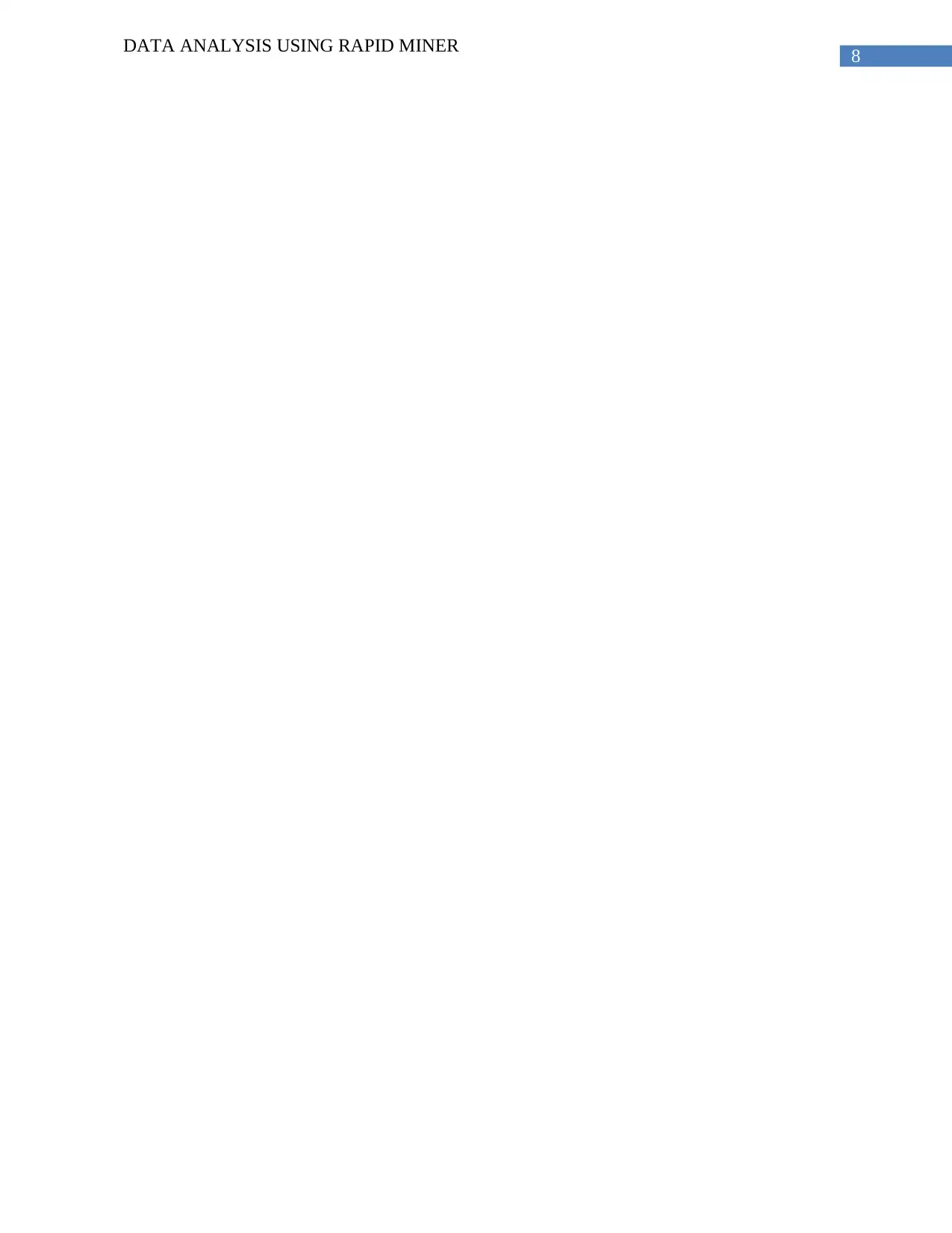
8
DATA ANALYSIS USING RAPID MINER
DATA ANALYSIS USING RAPID MINER
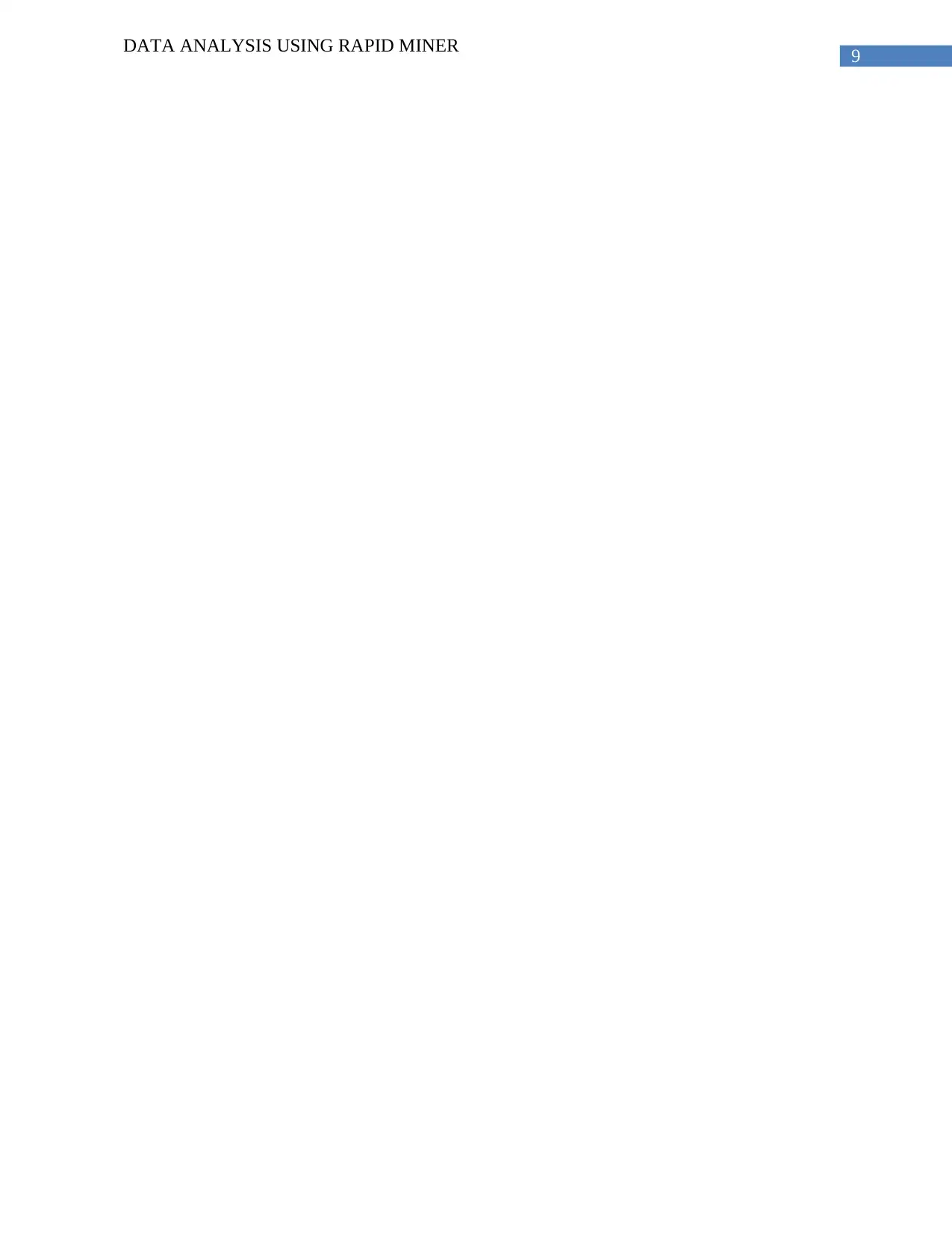
9
DATA ANALYSIS USING RAPID MINER
DATA ANALYSIS USING RAPID MINER
Secure Best Marks with AI Grader
Need help grading? Try our AI Grader for instant feedback on your assignments.
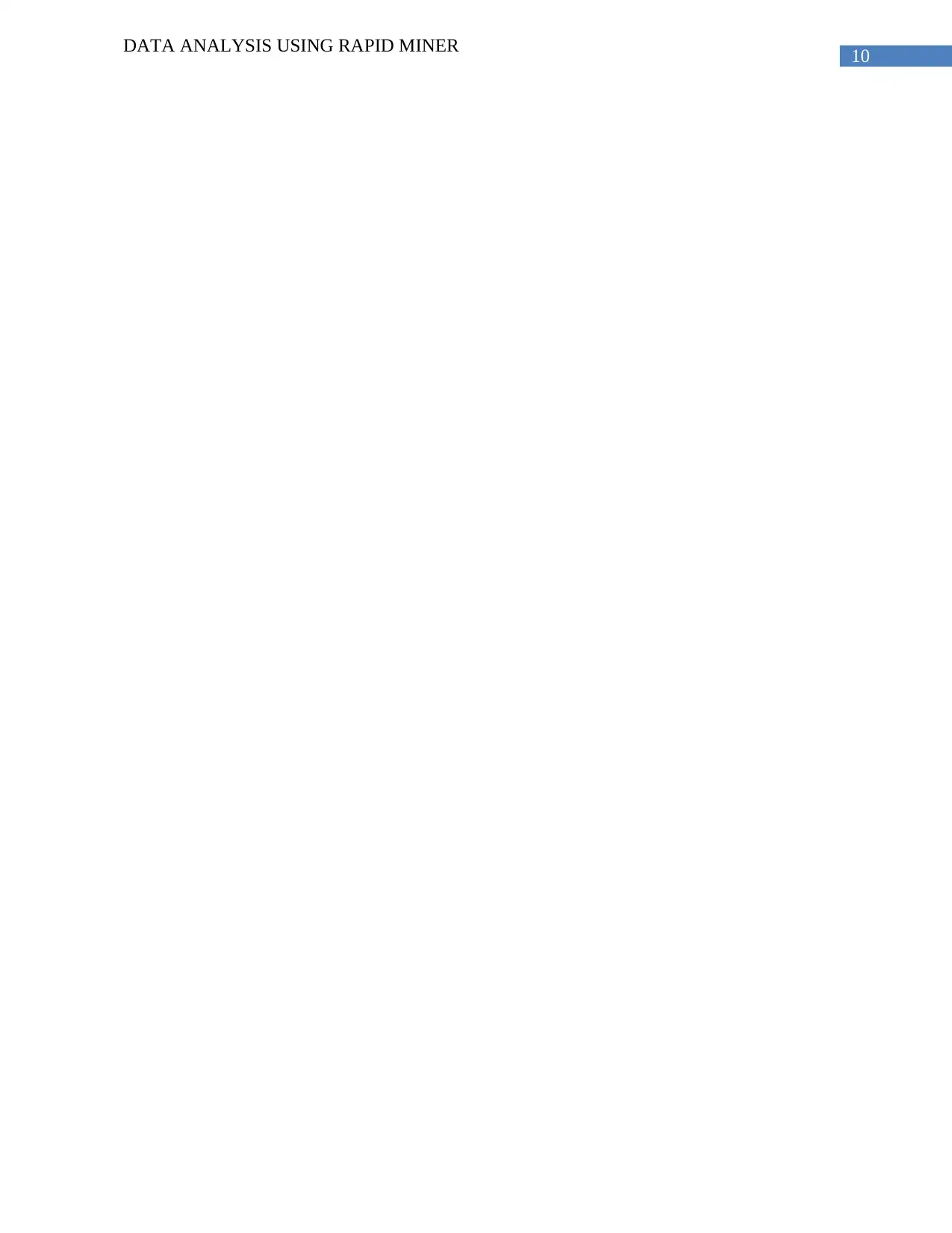
10
DATA ANALYSIS USING RAPID MINER
DATA ANALYSIS USING RAPID MINER
1 out of 11
Related Documents
![[object Object]](/_next/image/?url=%2F_next%2Fstatic%2Fmedia%2Flogo.6d15ce61.png&w=640&q=75)
Your All-in-One AI-Powered Toolkit for Academic Success.
 +13062052269
info@desklib.com
Available 24*7 on WhatsApp / Email
Unlock your academic potential
© 2024  |  Zucol Services PVT LTD  |  All rights reserved.