Techniques for Data Driven Decision Making
VerifiedAdded on 2023/06/13
|11
|3211
|476
AI Summary
The report discusses four scenarios based on the usage of data analytics. It covers the concept of Big Data, different types of data studied, and how data analytics can be used to improve the performance of a company. It also explains Pearson’s correlation matrix and Regression in business decision making.
Contribute Materials
Your contribution can guide someone’s learning journey. Share your
documents today.
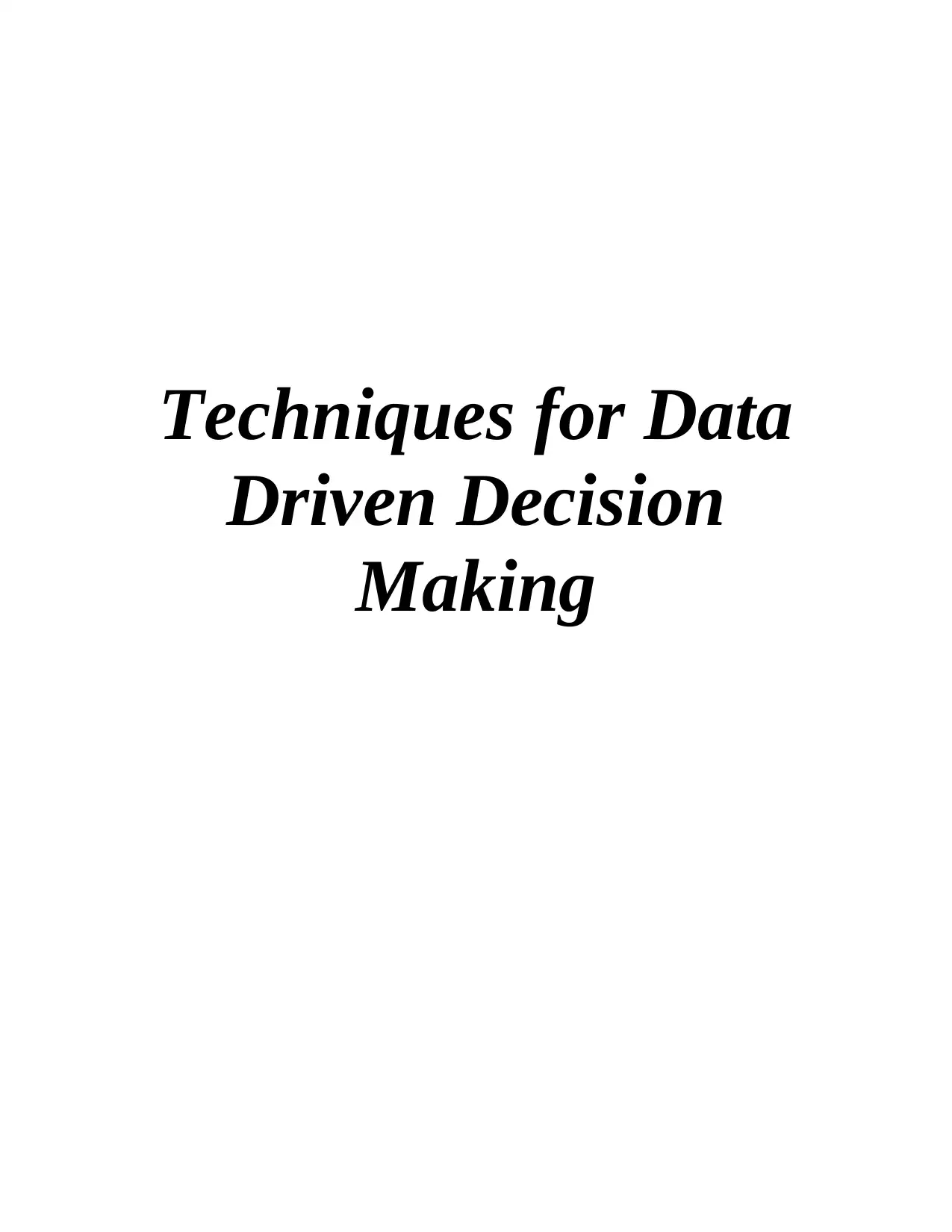
Techniques for Data
Driven Decision
Making
Driven Decision
Making
Secure Best Marks with AI Grader
Need help grading? Try our AI Grader for instant feedback on your assignments.
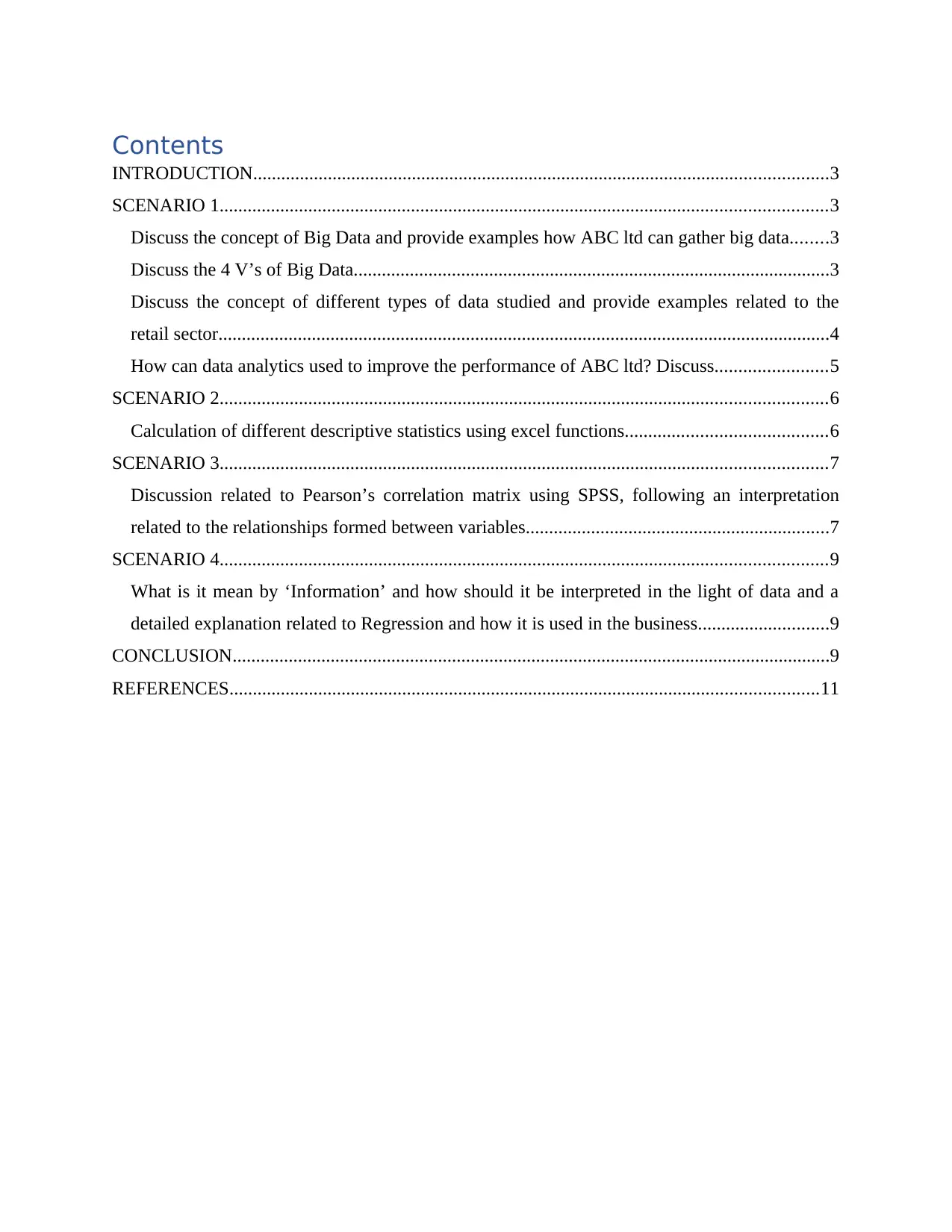
Contents
INTRODUCTION...........................................................................................................................3
SCENARIO 1..................................................................................................................................3
Discuss the concept of Big Data and provide examples how ABC ltd can gather big data........3
Discuss the 4 V’s of Big Data......................................................................................................3
Discuss the concept of different types of data studied and provide examples related to the
retail sector...................................................................................................................................4
How can data analytics used to improve the performance of ABC ltd? Discuss........................5
SCENARIO 2..................................................................................................................................6
Calculation of different descriptive statistics using excel functions...........................................6
SCENARIO 3..................................................................................................................................7
Discussion related to Pearson’s correlation matrix using SPSS, following an interpretation
related to the relationships formed between variables.................................................................7
SCENARIO 4..................................................................................................................................9
What is it mean by ‘Information’ and how should it be interpreted in the light of data and a
detailed explanation related to Regression and how it is used in the business............................9
CONCLUSION................................................................................................................................9
REFERENCES..............................................................................................................................11
INTRODUCTION...........................................................................................................................3
SCENARIO 1..................................................................................................................................3
Discuss the concept of Big Data and provide examples how ABC ltd can gather big data........3
Discuss the 4 V’s of Big Data......................................................................................................3
Discuss the concept of different types of data studied and provide examples related to the
retail sector...................................................................................................................................4
How can data analytics used to improve the performance of ABC ltd? Discuss........................5
SCENARIO 2..................................................................................................................................6
Calculation of different descriptive statistics using excel functions...........................................6
SCENARIO 3..................................................................................................................................7
Discussion related to Pearson’s correlation matrix using SPSS, following an interpretation
related to the relationships formed between variables.................................................................7
SCENARIO 4..................................................................................................................................9
What is it mean by ‘Information’ and how should it be interpreted in the light of data and a
detailed explanation related to Regression and how it is used in the business............................9
CONCLUSION................................................................................................................................9
REFERENCES..............................................................................................................................11
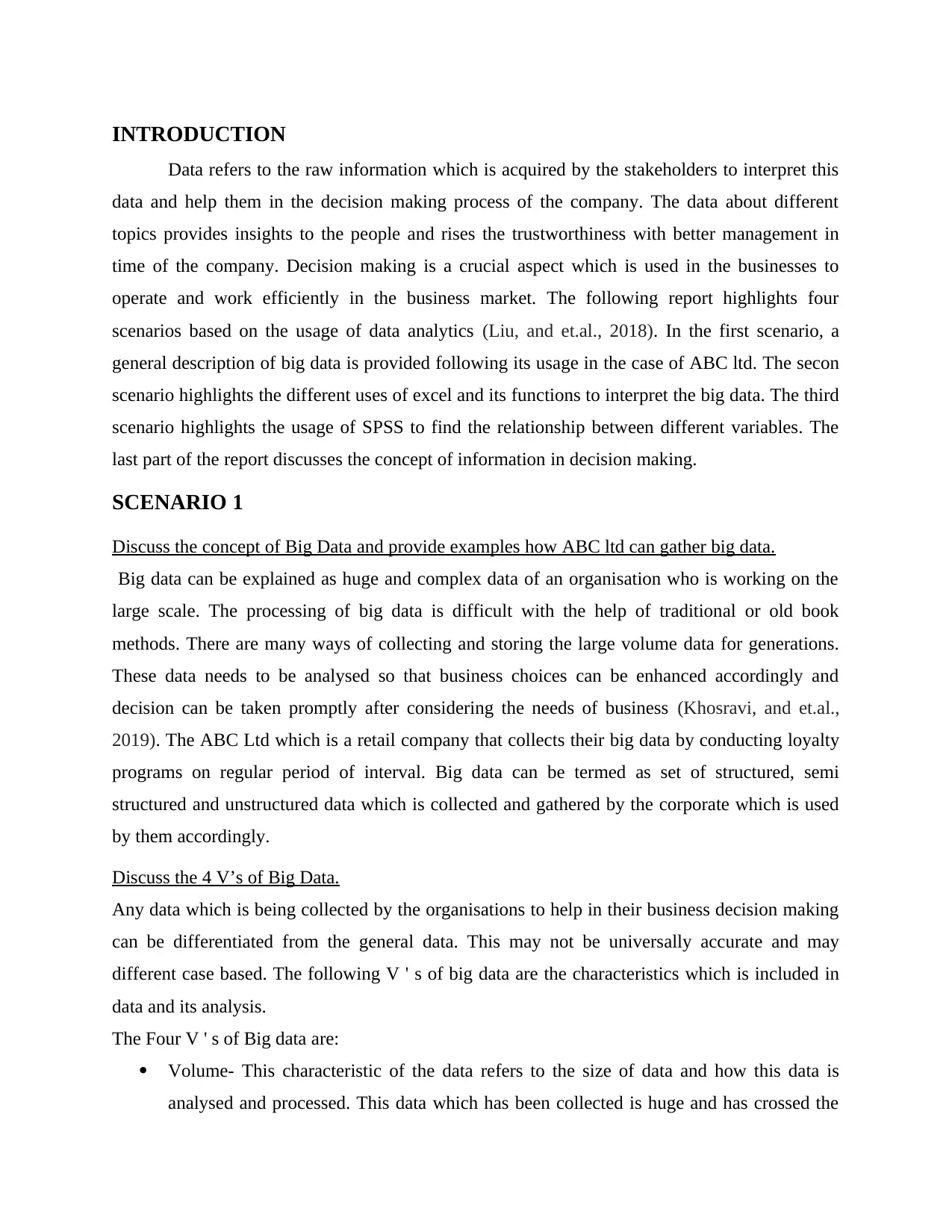
INTRODUCTION
Data refers to the raw information which is acquired by the stakeholders to interpret this
data and help them in the decision making process of the company. The data about different
topics provides insights to the people and rises the trustworthiness with better management in
time of the company. Decision making is a crucial aspect which is used in the businesses to
operate and work efficiently in the business market. The following report highlights four
scenarios based on the usage of data analytics (Liu, and et.al., 2018). In the first scenario, a
general description of big data is provided following its usage in the case of ABC ltd. The secon
scenario highlights the different uses of excel and its functions to interpret the big data. The third
scenario highlights the usage of SPSS to find the relationship between different variables. The
last part of the report discusses the concept of information in decision making.
SCENARIO 1
Discuss the concept of Big Data and provide examples how ABC ltd can gather big data.
Big data can be explained as huge and complex data of an organisation who is working on the
large scale. The processing of big data is difficult with the help of traditional or old book
methods. There are many ways of collecting and storing the large volume data for generations.
These data needs to be analysed so that business choices can be enhanced accordingly and
decision can be taken promptly after considering the needs of business (Khosravi, and et.al.,
2019). The ABC Ltd which is a retail company that collects their big data by conducting loyalty
programs on regular period of interval. Big data can be termed as set of structured, semi
structured and unstructured data which is collected and gathered by the corporate which is used
by them accordingly.
Discuss the 4 V’s of Big Data.
Any data which is being collected by the organisations to help in their business decision making
can be differentiated from the general data. This may not be universally accurate and may
different case based. The following V ' s of big data are the characteristics which is included in
data and its analysis.
The Four V ' s of Big data are:
Volume- This characteristic of the data refers to the size of data and how this data is
analysed and processed. This data which has been collected is huge and has crossed the
Data refers to the raw information which is acquired by the stakeholders to interpret this
data and help them in the decision making process of the company. The data about different
topics provides insights to the people and rises the trustworthiness with better management in
time of the company. Decision making is a crucial aspect which is used in the businesses to
operate and work efficiently in the business market. The following report highlights four
scenarios based on the usage of data analytics (Liu, and et.al., 2018). In the first scenario, a
general description of big data is provided following its usage in the case of ABC ltd. The secon
scenario highlights the different uses of excel and its functions to interpret the big data. The third
scenario highlights the usage of SPSS to find the relationship between different variables. The
last part of the report discusses the concept of information in decision making.
SCENARIO 1
Discuss the concept of Big Data and provide examples how ABC ltd can gather big data.
Big data can be explained as huge and complex data of an organisation who is working on the
large scale. The processing of big data is difficult with the help of traditional or old book
methods. There are many ways of collecting and storing the large volume data for generations.
These data needs to be analysed so that business choices can be enhanced accordingly and
decision can be taken promptly after considering the needs of business (Khosravi, and et.al.,
2019). The ABC Ltd which is a retail company that collects their big data by conducting loyalty
programs on regular period of interval. Big data can be termed as set of structured, semi
structured and unstructured data which is collected and gathered by the corporate which is used
by them accordingly.
Discuss the 4 V’s of Big Data.
Any data which is being collected by the organisations to help in their business decision making
can be differentiated from the general data. This may not be universally accurate and may
different case based. The following V ' s of big data are the characteristics which is included in
data and its analysis.
The Four V ' s of Big data are:
Volume- This characteristic of the data refers to the size of data and how this data is
analysed and processed. This data which has been collected is huge and has crossed the
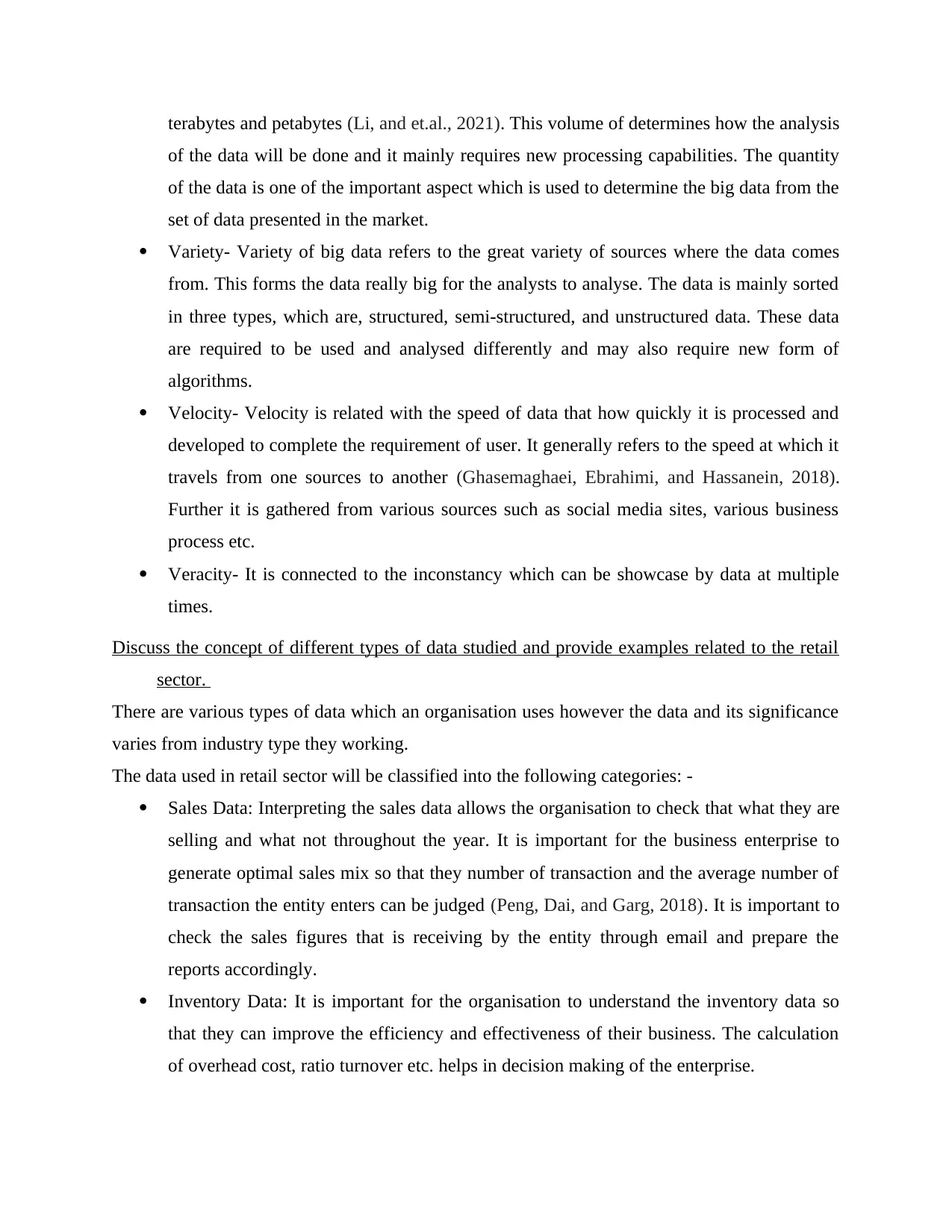
terabytes and petabytes (Li, and et.al., 2021). This volume of determines how the analysis
of the data will be done and it mainly requires new processing capabilities. The quantity
of the data is one of the important aspect which is used to determine the big data from the
set of data presented in the market.
Variety- Variety of big data refers to the great variety of sources where the data comes
from. This forms the data really big for the analysts to analyse. The data is mainly sorted
in three types, which are, structured, semi-structured, and unstructured data. These data
are required to be used and analysed differently and may also require new form of
algorithms.
Velocity- Velocity is related with the speed of data that how quickly it is processed and
developed to complete the requirement of user. It generally refers to the speed at which it
travels from one sources to another (Ghasemaghaei, Ebrahimi, and Hassanein, 2018).
Further it is gathered from various sources such as social media sites, various business
process etc.
Veracity- It is connected to the inconstancy which can be showcase by data at multiple
times.
Discuss the concept of different types of data studied and provide examples related to the retail
sector.
There are various types of data which an organisation uses however the data and its significance
varies from industry type they working.
The data used in retail sector will be classified into the following categories: -
Sales Data: Interpreting the sales data allows the organisation to check that what they are
selling and what not throughout the year. It is important for the business enterprise to
generate optimal sales mix so that they number of transaction and the average number of
transaction the entity enters can be judged (Peng, Dai, and Garg, 2018). It is important to
check the sales figures that is receiving by the entity through email and prepare the
reports accordingly.
Inventory Data: It is important for the organisation to understand the inventory data so
that they can improve the efficiency and effectiveness of their business. The calculation
of overhead cost, ratio turnover etc. helps in decision making of the enterprise.
of the data will be done and it mainly requires new processing capabilities. The quantity
of the data is one of the important aspect which is used to determine the big data from the
set of data presented in the market.
Variety- Variety of big data refers to the great variety of sources where the data comes
from. This forms the data really big for the analysts to analyse. The data is mainly sorted
in three types, which are, structured, semi-structured, and unstructured data. These data
are required to be used and analysed differently and may also require new form of
algorithms.
Velocity- Velocity is related with the speed of data that how quickly it is processed and
developed to complete the requirement of user. It generally refers to the speed at which it
travels from one sources to another (Ghasemaghaei, Ebrahimi, and Hassanein, 2018).
Further it is gathered from various sources such as social media sites, various business
process etc.
Veracity- It is connected to the inconstancy which can be showcase by data at multiple
times.
Discuss the concept of different types of data studied and provide examples related to the retail
sector.
There are various types of data which an organisation uses however the data and its significance
varies from industry type they working.
The data used in retail sector will be classified into the following categories: -
Sales Data: Interpreting the sales data allows the organisation to check that what they are
selling and what not throughout the year. It is important for the business enterprise to
generate optimal sales mix so that they number of transaction and the average number of
transaction the entity enters can be judged (Peng, Dai, and Garg, 2018). It is important to
check the sales figures that is receiving by the entity through email and prepare the
reports accordingly.
Inventory Data: It is important for the organisation to understand the inventory data so
that they can improve the efficiency and effectiveness of their business. The calculation
of overhead cost, ratio turnover etc. helps in decision making of the enterprise.
Secure Best Marks with AI Grader
Need help grading? Try our AI Grader for instant feedback on your assignments.
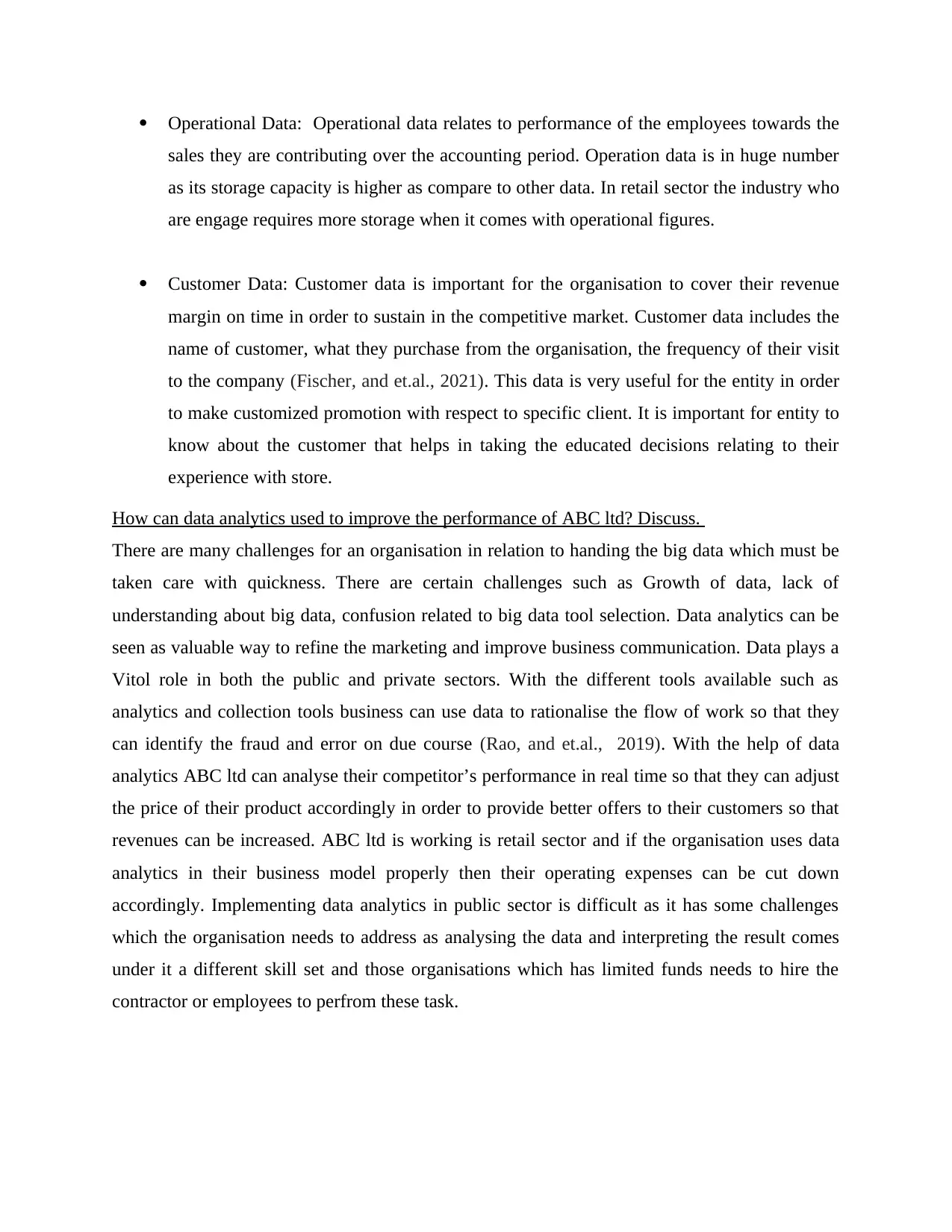
Operational Data: Operational data relates to performance of the employees towards the
sales they are contributing over the accounting period. Operation data is in huge number
as its storage capacity is higher as compare to other data. In retail sector the industry who
are engage requires more storage when it comes with operational figures.
Customer Data: Customer data is important for the organisation to cover their revenue
margin on time in order to sustain in the competitive market. Customer data includes the
name of customer, what they purchase from the organisation, the frequency of their visit
to the company (Fischer, and et.al., 2021). This data is very useful for the entity in order
to make customized promotion with respect to specific client. It is important for entity to
know about the customer that helps in taking the educated decisions relating to their
experience with store.
How can data analytics used to improve the performance of ABC ltd? Discuss.
There are many challenges for an organisation in relation to handing the big data which must be
taken care with quickness. There are certain challenges such as Growth of data, lack of
understanding about big data, confusion related to big data tool selection. Data analytics can be
seen as valuable way to refine the marketing and improve business communication. Data plays a
Vitol role in both the public and private sectors. With the different tools available such as
analytics and collection tools business can use data to rationalise the flow of work so that they
can identify the fraud and error on due course (Rao, and et.al., 2019). With the help of data
analytics ABC ltd can analyse their competitor’s performance in real time so that they can adjust
the price of their product accordingly in order to provide better offers to their customers so that
revenues can be increased. ABC ltd is working is retail sector and if the organisation uses data
analytics in their business model properly then their operating expenses can be cut down
accordingly. Implementing data analytics in public sector is difficult as it has some challenges
which the organisation needs to address as analysing the data and interpreting the result comes
under it a different skill set and those organisations which has limited funds needs to hire the
contractor or employees to perfrom these task.
sales they are contributing over the accounting period. Operation data is in huge number
as its storage capacity is higher as compare to other data. In retail sector the industry who
are engage requires more storage when it comes with operational figures.
Customer Data: Customer data is important for the organisation to cover their revenue
margin on time in order to sustain in the competitive market. Customer data includes the
name of customer, what they purchase from the organisation, the frequency of their visit
to the company (Fischer, and et.al., 2021). This data is very useful for the entity in order
to make customized promotion with respect to specific client. It is important for entity to
know about the customer that helps in taking the educated decisions relating to their
experience with store.
How can data analytics used to improve the performance of ABC ltd? Discuss.
There are many challenges for an organisation in relation to handing the big data which must be
taken care with quickness. There are certain challenges such as Growth of data, lack of
understanding about big data, confusion related to big data tool selection. Data analytics can be
seen as valuable way to refine the marketing and improve business communication. Data plays a
Vitol role in both the public and private sectors. With the different tools available such as
analytics and collection tools business can use data to rationalise the flow of work so that they
can identify the fraud and error on due course (Rao, and et.al., 2019). With the help of data
analytics ABC ltd can analyse their competitor’s performance in real time so that they can adjust
the price of their product accordingly in order to provide better offers to their customers so that
revenues can be increased. ABC ltd is working is retail sector and if the organisation uses data
analytics in their business model properly then their operating expenses can be cut down
accordingly. Implementing data analytics in public sector is difficult as it has some challenges
which the organisation needs to address as analysing the data and interpreting the result comes
under it a different skill set and those organisations which has limited funds needs to hire the
contractor or employees to perfrom these task.
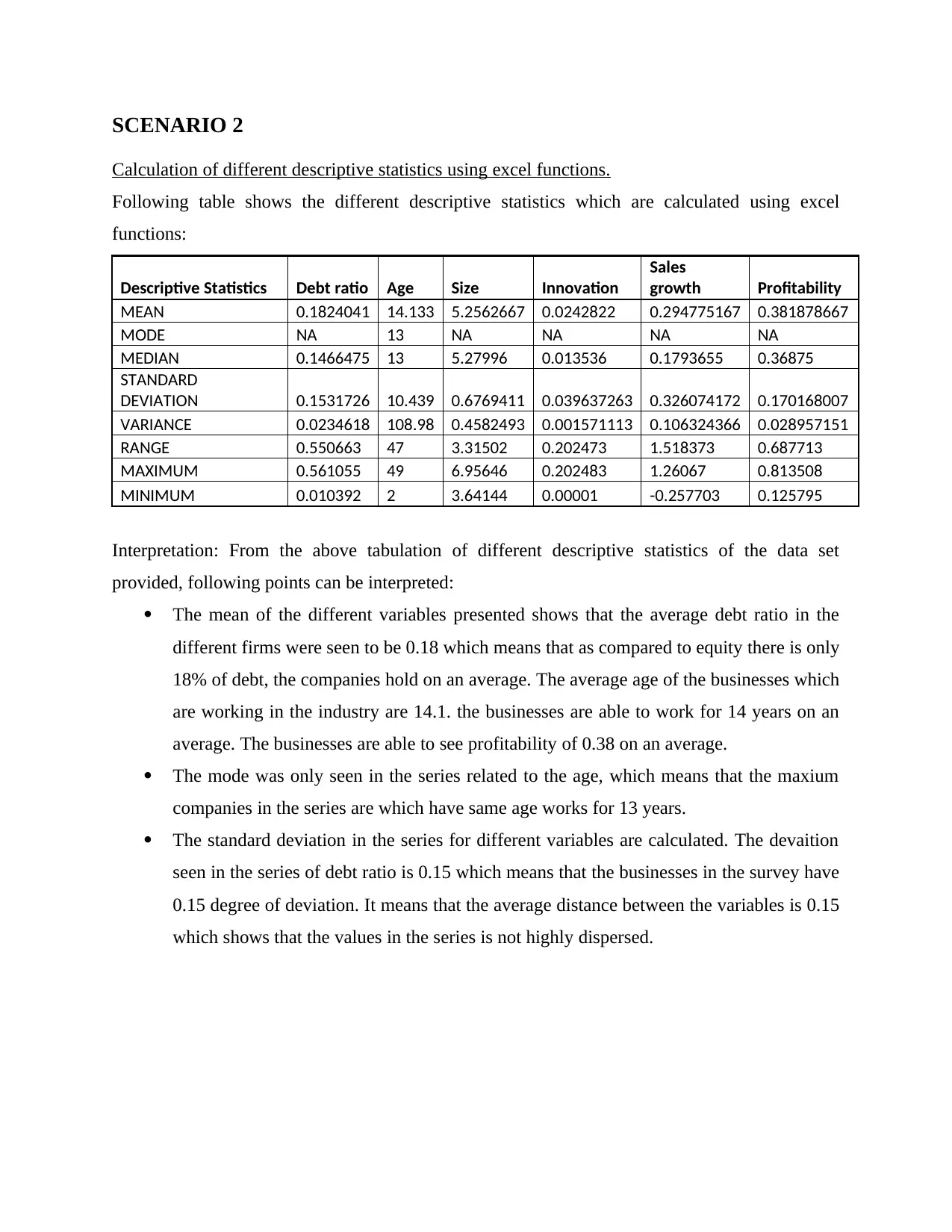
SCENARIO 2
Calculation of different descriptive statistics using excel functions.
Following table shows the different descriptive statistics which are calculated using excel
functions:
Descriptive Statistics Debt ratio Age Size Innovation
Sales
growth Profitability
MEAN 0.1824041 14.133 5.2562667 0.0242822 0.294775167 0.381878667
MODE NA 13 NA NA NA NA
MEDIAN 0.1466475 13 5.27996 0.013536 0.1793655 0.36875
STANDARD
DEVIATION 0.1531726 10.439 0.6769411 0.039637263 0.326074172 0.170168007
VARIANCE 0.0234618 108.98 0.4582493 0.001571113 0.106324366 0.028957151
RANGE 0.550663 47 3.31502 0.202473 1.518373 0.687713
MAXIMUM 0.561055 49 6.95646 0.202483 1.26067 0.813508
MINIMUM 0.010392 2 3.64144 0.00001 -0.257703 0.125795
Interpretation: From the above tabulation of different descriptive statistics of the data set
provided, following points can be interpreted:
The mean of the different variables presented shows that the average debt ratio in the
different firms were seen to be 0.18 which means that as compared to equity there is only
18% of debt, the companies hold on an average. The average age of the businesses which
are working in the industry are 14.1. the businesses are able to work for 14 years on an
average. The businesses are able to see profitability of 0.38 on an average.
The mode was only seen in the series related to the age, which means that the maxium
companies in the series are which have same age works for 13 years.
The standard deviation in the series for different variables are calculated. The devaition
seen in the series of debt ratio is 0.15 which means that the businesses in the survey have
0.15 degree of deviation. It means that the average distance between the variables is 0.15
which shows that the values in the series is not highly dispersed.
Calculation of different descriptive statistics using excel functions.
Following table shows the different descriptive statistics which are calculated using excel
functions:
Descriptive Statistics Debt ratio Age Size Innovation
Sales
growth Profitability
MEAN 0.1824041 14.133 5.2562667 0.0242822 0.294775167 0.381878667
MODE NA 13 NA NA NA NA
MEDIAN 0.1466475 13 5.27996 0.013536 0.1793655 0.36875
STANDARD
DEVIATION 0.1531726 10.439 0.6769411 0.039637263 0.326074172 0.170168007
VARIANCE 0.0234618 108.98 0.4582493 0.001571113 0.106324366 0.028957151
RANGE 0.550663 47 3.31502 0.202473 1.518373 0.687713
MAXIMUM 0.561055 49 6.95646 0.202483 1.26067 0.813508
MINIMUM 0.010392 2 3.64144 0.00001 -0.257703 0.125795
Interpretation: From the above tabulation of different descriptive statistics of the data set
provided, following points can be interpreted:
The mean of the different variables presented shows that the average debt ratio in the
different firms were seen to be 0.18 which means that as compared to equity there is only
18% of debt, the companies hold on an average. The average age of the businesses which
are working in the industry are 14.1. the businesses are able to work for 14 years on an
average. The businesses are able to see profitability of 0.38 on an average.
The mode was only seen in the series related to the age, which means that the maxium
companies in the series are which have same age works for 13 years.
The standard deviation in the series for different variables are calculated. The devaition
seen in the series of debt ratio is 0.15 which means that the businesses in the survey have
0.15 degree of deviation. It means that the average distance between the variables is 0.15
which shows that the values in the series is not highly dispersed.
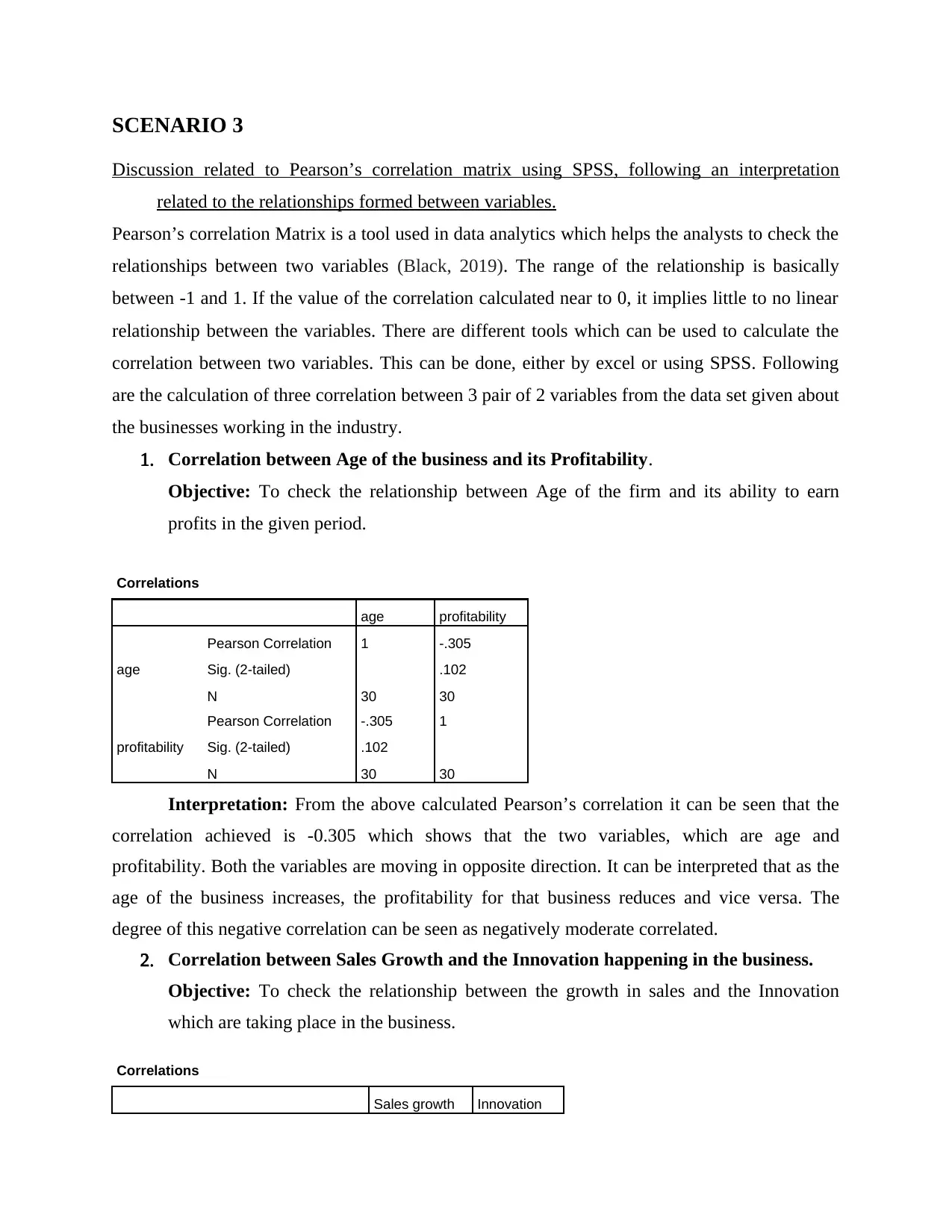
SCENARIO 3
Discussion related to Pearson’s correlation matrix using SPSS, following an interpretation
related to the relationships formed between variables.
Pearson’s correlation Matrix is a tool used in data analytics which helps the analysts to check the
relationships between two variables (Black, 2019). The range of the relationship is basically
between -1 and 1. If the value of the correlation calculated near to 0, it implies little to no linear
relationship between the variables. There are different tools which can be used to calculate the
correlation between two variables. This can be done, either by excel or using SPSS. Following
are the calculation of three correlation between 3 pair of 2 variables from the data set given about
the businesses working in the industry.
1. Correlation between Age of the business and its Profitability.
Objective: To check the relationship between Age of the firm and its ability to earn
profits in the given period.
Correlations
age profitability
age
Pearson Correlation 1 -.305
Sig. (2-tailed) .102
N 30 30
profitability
Pearson Correlation -.305 1
Sig. (2-tailed) .102
N 30 30
Interpretation: From the above calculated Pearson’s correlation it can be seen that the
correlation achieved is -0.305 which shows that the two variables, which are age and
profitability. Both the variables are moving in opposite direction. It can be interpreted that as the
age of the business increases, the profitability for that business reduces and vice versa. The
degree of this negative correlation can be seen as negatively moderate correlated.
2. Correlation between Sales Growth and the Innovation happening in the business.
Objective: To check the relationship between the growth in sales and the Innovation
which are taking place in the business.
Correlations
Sales growth Innovation
Discussion related to Pearson’s correlation matrix using SPSS, following an interpretation
related to the relationships formed between variables.
Pearson’s correlation Matrix is a tool used in data analytics which helps the analysts to check the
relationships between two variables (Black, 2019). The range of the relationship is basically
between -1 and 1. If the value of the correlation calculated near to 0, it implies little to no linear
relationship between the variables. There are different tools which can be used to calculate the
correlation between two variables. This can be done, either by excel or using SPSS. Following
are the calculation of three correlation between 3 pair of 2 variables from the data set given about
the businesses working in the industry.
1. Correlation between Age of the business and its Profitability.
Objective: To check the relationship between Age of the firm and its ability to earn
profits in the given period.
Correlations
age profitability
age
Pearson Correlation 1 -.305
Sig. (2-tailed) .102
N 30 30
profitability
Pearson Correlation -.305 1
Sig. (2-tailed) .102
N 30 30
Interpretation: From the above calculated Pearson’s correlation it can be seen that the
correlation achieved is -0.305 which shows that the two variables, which are age and
profitability. Both the variables are moving in opposite direction. It can be interpreted that as the
age of the business increases, the profitability for that business reduces and vice versa. The
degree of this negative correlation can be seen as negatively moderate correlated.
2. Correlation between Sales Growth and the Innovation happening in the business.
Objective: To check the relationship between the growth in sales and the Innovation
which are taking place in the business.
Correlations
Sales growth Innovation
Paraphrase This Document
Need a fresh take? Get an instant paraphrase of this document with our AI Paraphraser
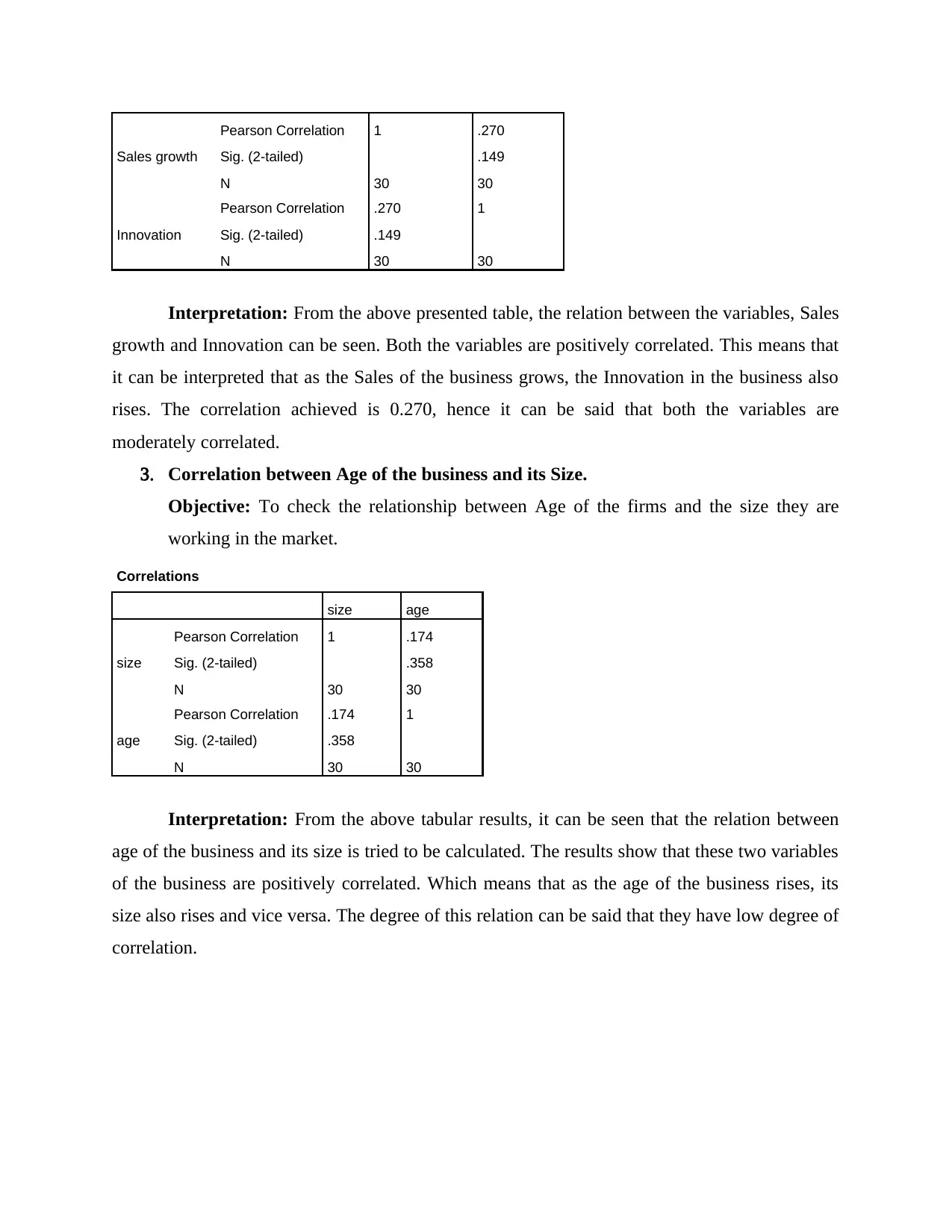
Sales growth
Pearson Correlation 1 .270
Sig. (2-tailed) .149
N 30 30
Innovation
Pearson Correlation .270 1
Sig. (2-tailed) .149
N 30 30
Interpretation: From the above presented table, the relation between the variables, Sales
growth and Innovation can be seen. Both the variables are positively correlated. This means that
it can be interpreted that as the Sales of the business grows, the Innovation in the business also
rises. The correlation achieved is 0.270, hence it can be said that both the variables are
moderately correlated.
3. Correlation between Age of the business and its Size.
Objective: To check the relationship between Age of the firms and the size they are
working in the market.
Correlations
size age
size
Pearson Correlation 1 .174
Sig. (2-tailed) .358
N 30 30
age
Pearson Correlation .174 1
Sig. (2-tailed) .358
N 30 30
Interpretation: From the above tabular results, it can be seen that the relation between
age of the business and its size is tried to be calculated. The results show that these two variables
of the business are positively correlated. Which means that as the age of the business rises, its
size also rises and vice versa. The degree of this relation can be said that they have low degree of
correlation.
Pearson Correlation 1 .270
Sig. (2-tailed) .149
N 30 30
Innovation
Pearson Correlation .270 1
Sig. (2-tailed) .149
N 30 30
Interpretation: From the above presented table, the relation between the variables, Sales
growth and Innovation can be seen. Both the variables are positively correlated. This means that
it can be interpreted that as the Sales of the business grows, the Innovation in the business also
rises. The correlation achieved is 0.270, hence it can be said that both the variables are
moderately correlated.
3. Correlation between Age of the business and its Size.
Objective: To check the relationship between Age of the firms and the size they are
working in the market.
Correlations
size age
size
Pearson Correlation 1 .174
Sig. (2-tailed) .358
N 30 30
age
Pearson Correlation .174 1
Sig. (2-tailed) .358
N 30 30
Interpretation: From the above tabular results, it can be seen that the relation between
age of the business and its size is tried to be calculated. The results show that these two variables
of the business are positively correlated. Which means that as the age of the business rises, its
size also rises and vice versa. The degree of this relation can be said that they have low degree of
correlation.
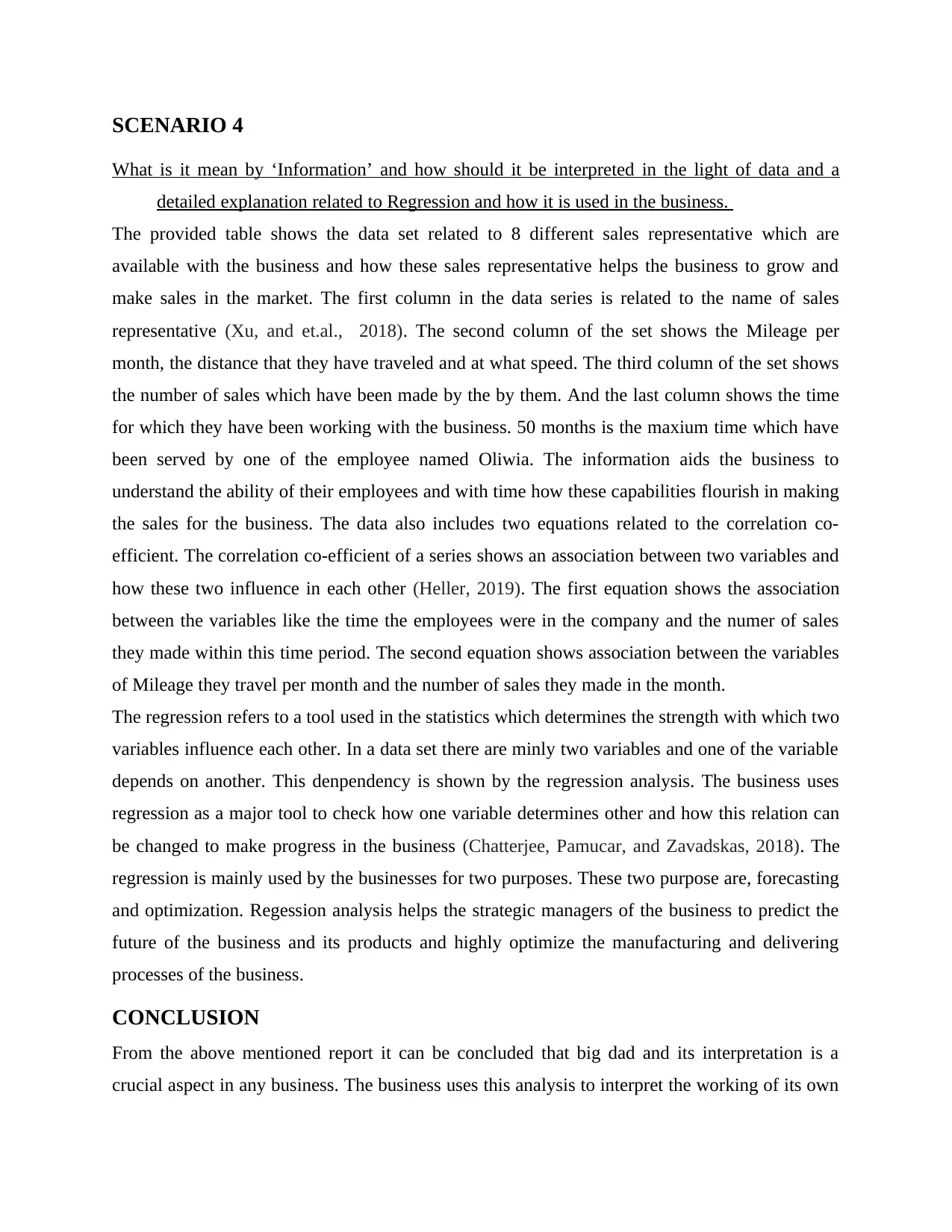
SCENARIO 4
What is it mean by ‘Information’ and how should it be interpreted in the light of data and a
detailed explanation related to Regression and how it is used in the business.
The provided table shows the data set related to 8 different sales representative which are
available with the business and how these sales representative helps the business to grow and
make sales in the market. The first column in the data series is related to the name of sales
representative (Xu, and et.al., 2018). The second column of the set shows the Mileage per
month, the distance that they have traveled and at what speed. The third column of the set shows
the number of sales which have been made by the by them. And the last column shows the time
for which they have been working with the business. 50 months is the maxium time which have
been served by one of the employee named Oliwia. The information aids the business to
understand the ability of their employees and with time how these capabilities flourish in making
the sales for the business. The data also includes two equations related to the correlation co-
efficient. The correlation co-efficient of a series shows an association between two variables and
how these two influence in each other (Heller, 2019). The first equation shows the association
between the variables like the time the employees were in the company and the numer of sales
they made within this time period. The second equation shows association between the variables
of Mileage they travel per month and the number of sales they made in the month.
The regression refers to a tool used in the statistics which determines the strength with which two
variables influence each other. In a data set there are minly two variables and one of the variable
depends on another. This denpendency is shown by the regression analysis. The business uses
regression as a major tool to check how one variable determines other and how this relation can
be changed to make progress in the business (Chatterjee, Pamucar, and Zavadskas, 2018). The
regression is mainly used by the businesses for two purposes. These two purpose are, forecasting
and optimization. Regession analysis helps the strategic managers of the business to predict the
future of the business and its products and highly optimize the manufacturing and delivering
processes of the business.
CONCLUSION
From the above mentioned report it can be concluded that big dad and its interpretation is a
crucial aspect in any business. The business uses this analysis to interpret the working of its own
What is it mean by ‘Information’ and how should it be interpreted in the light of data and a
detailed explanation related to Regression and how it is used in the business.
The provided table shows the data set related to 8 different sales representative which are
available with the business and how these sales representative helps the business to grow and
make sales in the market. The first column in the data series is related to the name of sales
representative (Xu, and et.al., 2018). The second column of the set shows the Mileage per
month, the distance that they have traveled and at what speed. The third column of the set shows
the number of sales which have been made by the by them. And the last column shows the time
for which they have been working with the business. 50 months is the maxium time which have
been served by one of the employee named Oliwia. The information aids the business to
understand the ability of their employees and with time how these capabilities flourish in making
the sales for the business. The data also includes two equations related to the correlation co-
efficient. The correlation co-efficient of a series shows an association between two variables and
how these two influence in each other (Heller, 2019). The first equation shows the association
between the variables like the time the employees were in the company and the numer of sales
they made within this time period. The second equation shows association between the variables
of Mileage they travel per month and the number of sales they made in the month.
The regression refers to a tool used in the statistics which determines the strength with which two
variables influence each other. In a data set there are minly two variables and one of the variable
depends on another. This denpendency is shown by the regression analysis. The business uses
regression as a major tool to check how one variable determines other and how this relation can
be changed to make progress in the business (Chatterjee, Pamucar, and Zavadskas, 2018). The
regression is mainly used by the businesses for two purposes. These two purpose are, forecasting
and optimization. Regession analysis helps the strategic managers of the business to predict the
future of the business and its products and highly optimize the manufacturing and delivering
processes of the business.
CONCLUSION
From the above mentioned report it can be concluded that big dad and its interpretation is a
crucial aspect in any business. The business uses this analysis to interpret the working of its own
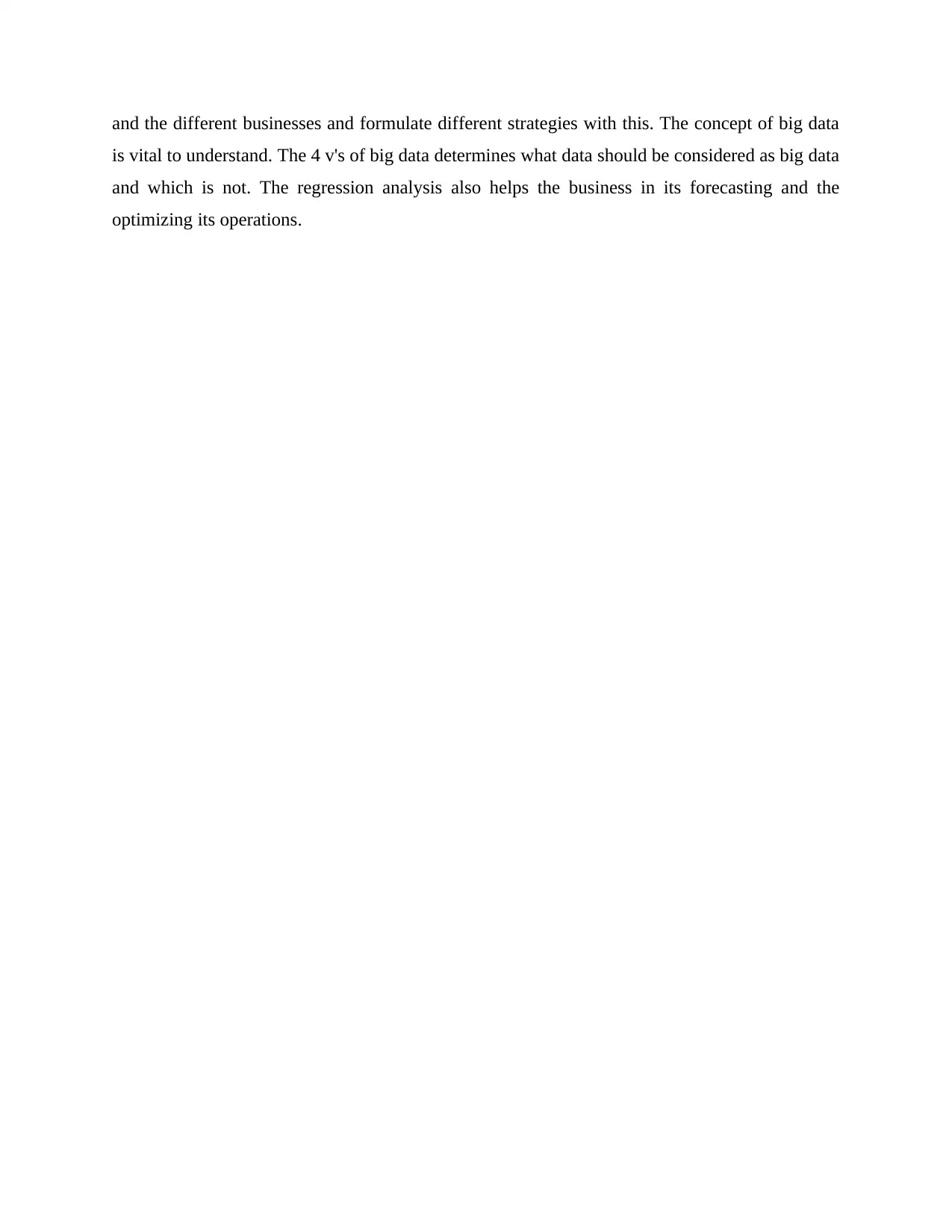
and the different businesses and formulate different strategies with this. The concept of big data
is vital to understand. The 4 v's of big data determines what data should be considered as big data
and which is not. The regression analysis also helps the business in its forecasting and the
optimizing its operations.
is vital to understand. The 4 v's of big data determines what data should be considered as big data
and which is not. The regression analysis also helps the business in its forecasting and the
optimizing its operations.
Secure Best Marks with AI Grader
Need help grading? Try our AI Grader for instant feedback on your assignments.
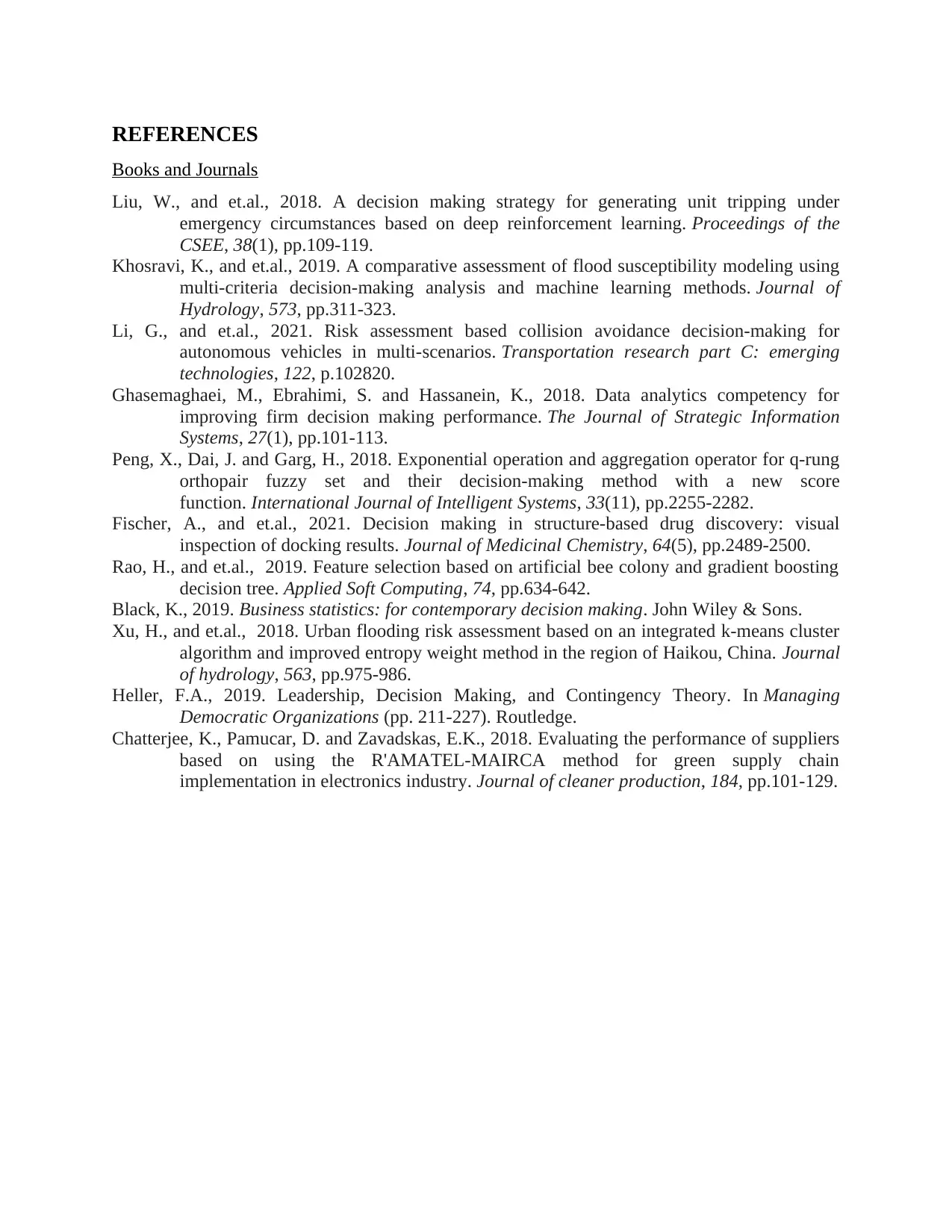
REFERENCES
Books and Journals
Liu, W., and et.al., 2018. A decision making strategy for generating unit tripping under
emergency circumstances based on deep reinforcement learning. Proceedings of the
CSEE, 38(1), pp.109-119.
Khosravi, K., and et.al., 2019. A comparative assessment of flood susceptibility modeling using
multi-criteria decision-making analysis and machine learning methods. Journal of
Hydrology, 573, pp.311-323.
Li, G., and et.al., 2021. Risk assessment based collision avoidance decision-making for
autonomous vehicles in multi-scenarios. Transportation research part C: emerging
technologies, 122, p.102820.
Ghasemaghaei, M., Ebrahimi, S. and Hassanein, K., 2018. Data analytics competency for
improving firm decision making performance. The Journal of Strategic Information
Systems, 27(1), pp.101-113.
Peng, X., Dai, J. and Garg, H., 2018. Exponential operation and aggregation operator for q‐rung
orthopair fuzzy set and their decision‐making method with a new score
function. International Journal of Intelligent Systems, 33(11), pp.2255-2282.
Fischer, A., and et.al., 2021. Decision making in structure-based drug discovery: visual
inspection of docking results. Journal of Medicinal Chemistry, 64(5), pp.2489-2500.
Rao, H., and et.al., 2019. Feature selection based on artificial bee colony and gradient boosting
decision tree. Applied Soft Computing, 74, pp.634-642.
Black, K., 2019. Business statistics: for contemporary decision making. John Wiley & Sons.
Xu, H., and et.al., 2018. Urban flooding risk assessment based on an integrated k-means cluster
algorithm and improved entropy weight method in the region of Haikou, China. Journal
of hydrology, 563, pp.975-986.
Heller, F.A., 2019. Leadership, Decision Making, and Contingency Theory. In Managing
Democratic Organizations (pp. 211-227). Routledge.
Chatterjee, K., Pamucar, D. and Zavadskas, E.K., 2018. Evaluating the performance of suppliers
based on using the R'AMATEL-MAIRCA method for green supply chain
implementation in electronics industry. Journal of cleaner production, 184, pp.101-129.
Books and Journals
Liu, W., and et.al., 2018. A decision making strategy for generating unit tripping under
emergency circumstances based on deep reinforcement learning. Proceedings of the
CSEE, 38(1), pp.109-119.
Khosravi, K., and et.al., 2019. A comparative assessment of flood susceptibility modeling using
multi-criteria decision-making analysis and machine learning methods. Journal of
Hydrology, 573, pp.311-323.
Li, G., and et.al., 2021. Risk assessment based collision avoidance decision-making for
autonomous vehicles in multi-scenarios. Transportation research part C: emerging
technologies, 122, p.102820.
Ghasemaghaei, M., Ebrahimi, S. and Hassanein, K., 2018. Data analytics competency for
improving firm decision making performance. The Journal of Strategic Information
Systems, 27(1), pp.101-113.
Peng, X., Dai, J. and Garg, H., 2018. Exponential operation and aggregation operator for q‐rung
orthopair fuzzy set and their decision‐making method with a new score
function. International Journal of Intelligent Systems, 33(11), pp.2255-2282.
Fischer, A., and et.al., 2021. Decision making in structure-based drug discovery: visual
inspection of docking results. Journal of Medicinal Chemistry, 64(5), pp.2489-2500.
Rao, H., and et.al., 2019. Feature selection based on artificial bee colony and gradient boosting
decision tree. Applied Soft Computing, 74, pp.634-642.
Black, K., 2019. Business statistics: for contemporary decision making. John Wiley & Sons.
Xu, H., and et.al., 2018. Urban flooding risk assessment based on an integrated k-means cluster
algorithm and improved entropy weight method in the region of Haikou, China. Journal
of hydrology, 563, pp.975-986.
Heller, F.A., 2019. Leadership, Decision Making, and Contingency Theory. In Managing
Democratic Organizations (pp. 211-227). Routledge.
Chatterjee, K., Pamucar, D. and Zavadskas, E.K., 2018. Evaluating the performance of suppliers
based on using the R'AMATEL-MAIRCA method for green supply chain
implementation in electronics industry. Journal of cleaner production, 184, pp.101-129.
1 out of 11
Related Documents
![[object Object]](/_next/image/?url=%2F_next%2Fstatic%2Fmedia%2Flogo.6d15ce61.png&w=640&q=75)
Your All-in-One AI-Powered Toolkit for Academic Success.
+13062052269
info@desklib.com
Available 24*7 on WhatsApp / Email
Unlock your academic potential
© 2024 | Zucol Services PVT LTD | All rights reserved.