Data Handling and Business Intelligence
VerifiedAdded on 2023/01/03
|8
|1696
|24
AI Summary
This article discusses the current trends in data warehousing, business intelligence, and data mining. It also explores the application of predictive analytics in business intelligence.
Contribute Materials
Your contribution can guide someone’s learning journey. Share your
documents today.
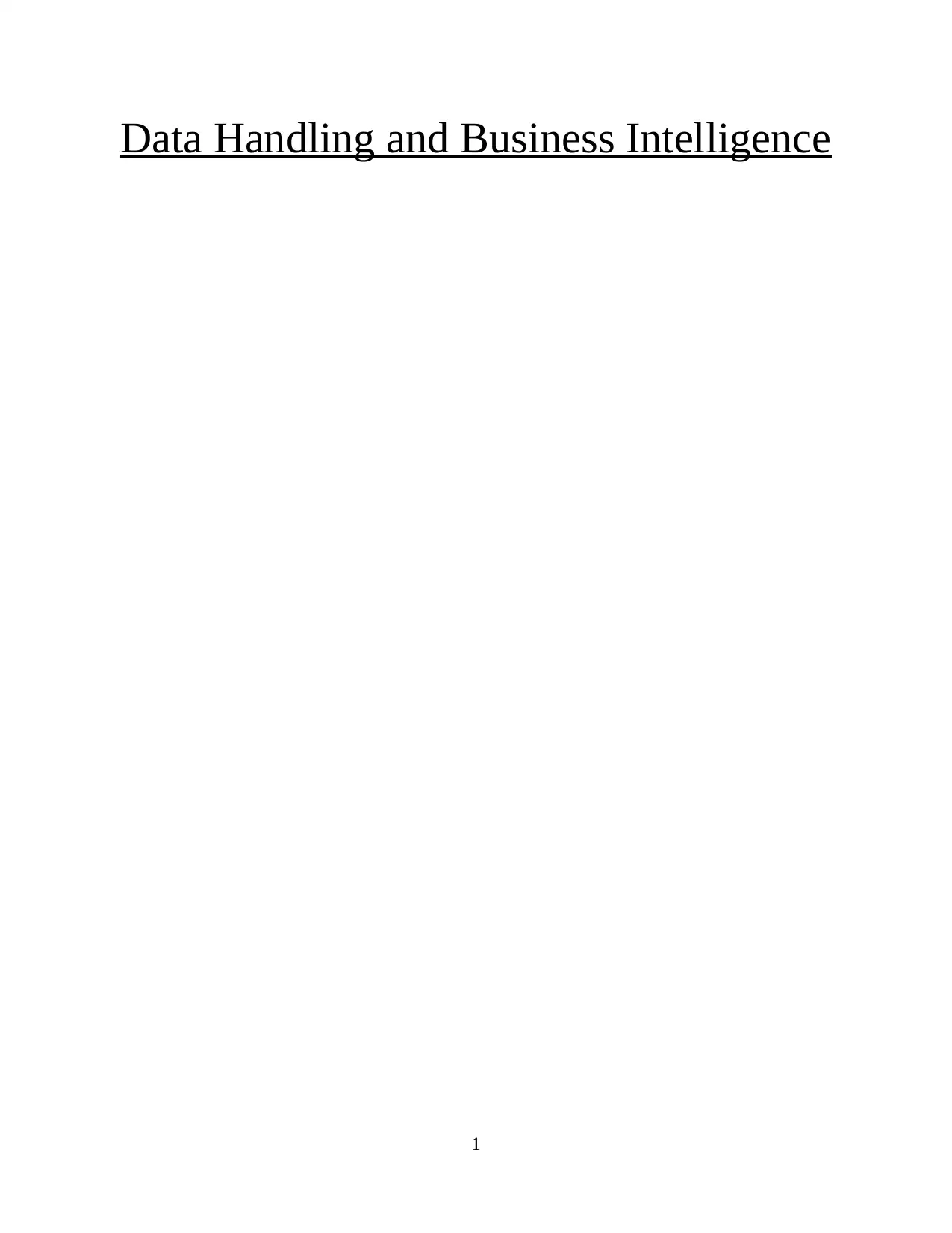
Data Handling and Business Intelligence
1
1
Secure Best Marks with AI Grader
Need help grading? Try our AI Grader for instant feedback on your assignments.
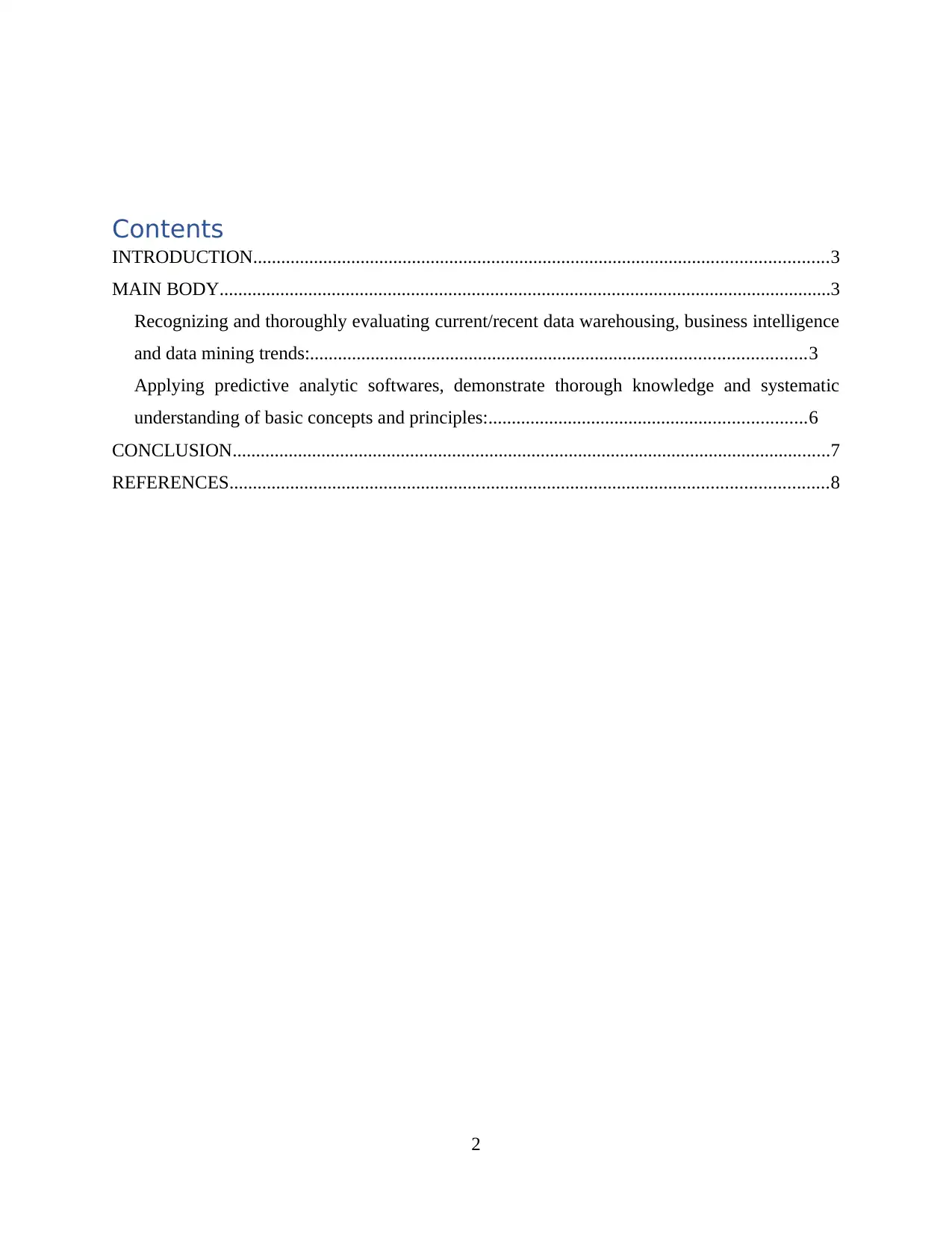
Contents
INTRODUCTION...........................................................................................................................3
MAIN BODY...................................................................................................................................3
Recognizing and thoroughly evaluating current/recent data warehousing, business intelligence
and data mining trends:..........................................................................................................3
Applying predictive analytic softwares, demonstrate thorough knowledge and systematic
understanding of basic concepts and principles:....................................................................6
CONCLUSION................................................................................................................................7
REFERENCES................................................................................................................................8
2
INTRODUCTION...........................................................................................................................3
MAIN BODY...................................................................................................................................3
Recognizing and thoroughly evaluating current/recent data warehousing, business intelligence
and data mining trends:..........................................................................................................3
Applying predictive analytic softwares, demonstrate thorough knowledge and systematic
understanding of basic concepts and principles:....................................................................6
CONCLUSION................................................................................................................................7
REFERENCES................................................................................................................................8
2
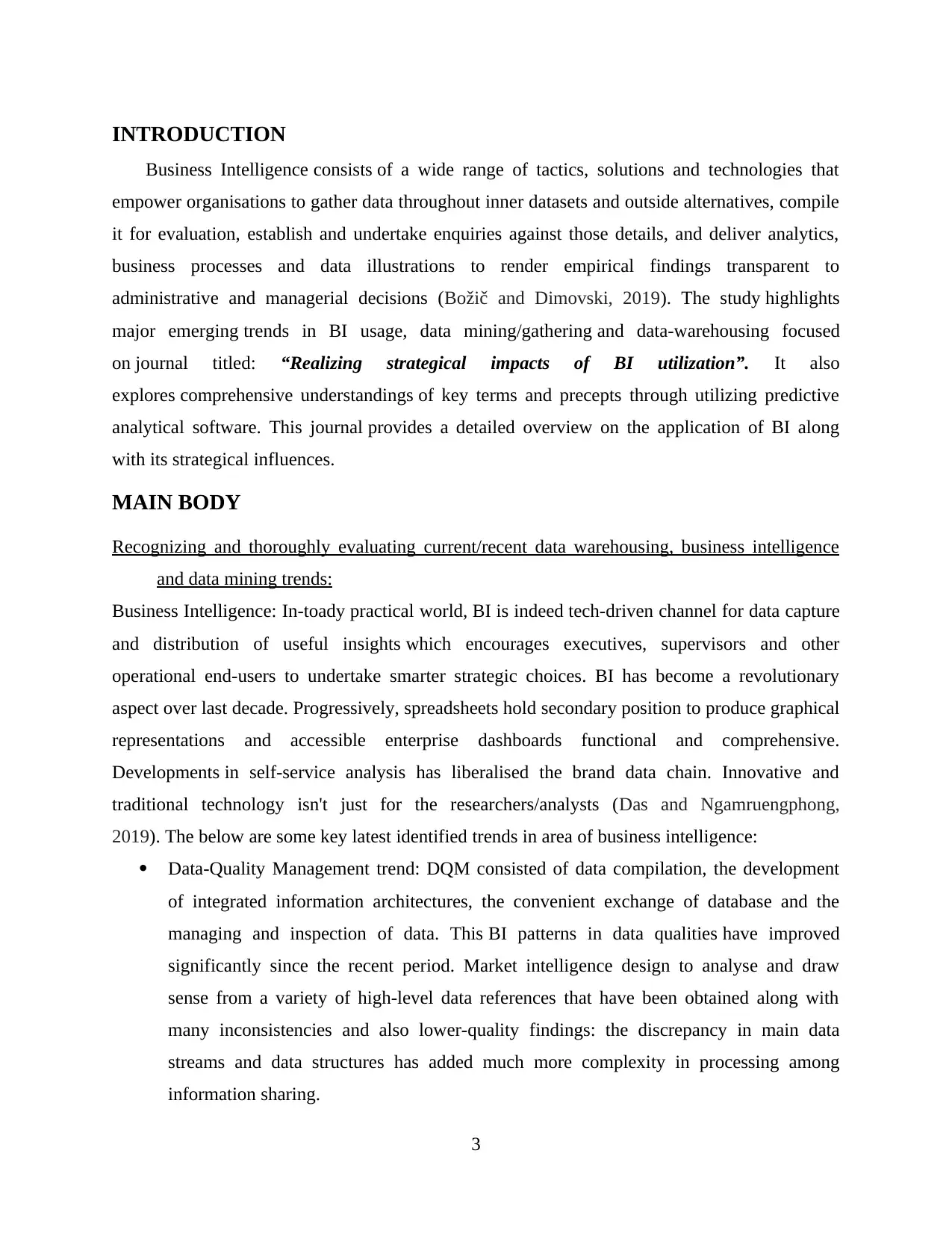
INTRODUCTION
Business Intelligence consists of a wide range of tactics, solutions and technologies that
empower organisations to gather data throughout inner datasets and outside alternatives, compile
it for evaluation, establish and undertake enquiries against those details, and deliver analytics,
business processes and data illustrations to render empirical findings transparent to
administrative and managerial decisions (Božič and Dimovski, 2019). The study highlights
major emerging trends in BI usage, data mining/gathering and data-warehousing focused
on journal titled: “Realizing strategical impacts of BI utilization”. It also
explores comprehensive understandings of key terms and precepts through utilizing predictive
analytical software. This journal provides a detailed overview on the application of BI along
with its strategical influences.
MAIN BODY
Recognizing and thoroughly evaluating current/recent data warehousing, business intelligence
and data mining trends:
Business Intelligence: In-toady practical world, BI is indeed tech-driven channel for data capture
and distribution of useful insights which encourages executives, supervisors and other
operational end-users to undertake smarter strategic choices. BI has become a revolutionary
aspect over last decade. Progressively, spreadsheets hold secondary position to produce graphical
representations and accessible enterprise dashboards functional and comprehensive.
Developments in self-service analysis has liberalised the brand data chain. Innovative and
traditional technology isn't just for the researchers/analysts (Das and Ngamruengphong,
2019). The below are some key latest identified trends in area of business intelligence:
Data-Quality Management trend: DQM consisted of data compilation, the development
of integrated information architectures, the convenient exchange of database and the
managing and inspection of data. This BI patterns in data qualities have improved
significantly since the recent period. Market intelligence design to analyse and draw
sense from a variety of high-level data references that have been obtained along with
many inconsistencies and also lower-quality findings: the discrepancy in main data
streams and data structures has added much more complexity in processing among
information sharing.
3
Business Intelligence consists of a wide range of tactics, solutions and technologies that
empower organisations to gather data throughout inner datasets and outside alternatives, compile
it for evaluation, establish and undertake enquiries against those details, and deliver analytics,
business processes and data illustrations to render empirical findings transparent to
administrative and managerial decisions (Božič and Dimovski, 2019). The study highlights
major emerging trends in BI usage, data mining/gathering and data-warehousing focused
on journal titled: “Realizing strategical impacts of BI utilization”. It also
explores comprehensive understandings of key terms and precepts through utilizing predictive
analytical software. This journal provides a detailed overview on the application of BI along
with its strategical influences.
MAIN BODY
Recognizing and thoroughly evaluating current/recent data warehousing, business intelligence
and data mining trends:
Business Intelligence: In-toady practical world, BI is indeed tech-driven channel for data capture
and distribution of useful insights which encourages executives, supervisors and other
operational end-users to undertake smarter strategic choices. BI has become a revolutionary
aspect over last decade. Progressively, spreadsheets hold secondary position to produce graphical
representations and accessible enterprise dashboards functional and comprehensive.
Developments in self-service analysis has liberalised the brand data chain. Innovative and
traditional technology isn't just for the researchers/analysts (Das and Ngamruengphong,
2019). The below are some key latest identified trends in area of business intelligence:
Data-Quality Management trend: DQM consisted of data compilation, the development
of integrated information architectures, the convenient exchange of database and the
managing and inspection of data. This BI patterns in data qualities have improved
significantly since the recent period. Market intelligence design to analyse and draw
sense from a variety of high-level data references that have been obtained along with
many inconsistencies and also lower-quality findings: the discrepancy in main data
streams and data structures has added much more complexity in processing among
information sharing.
3
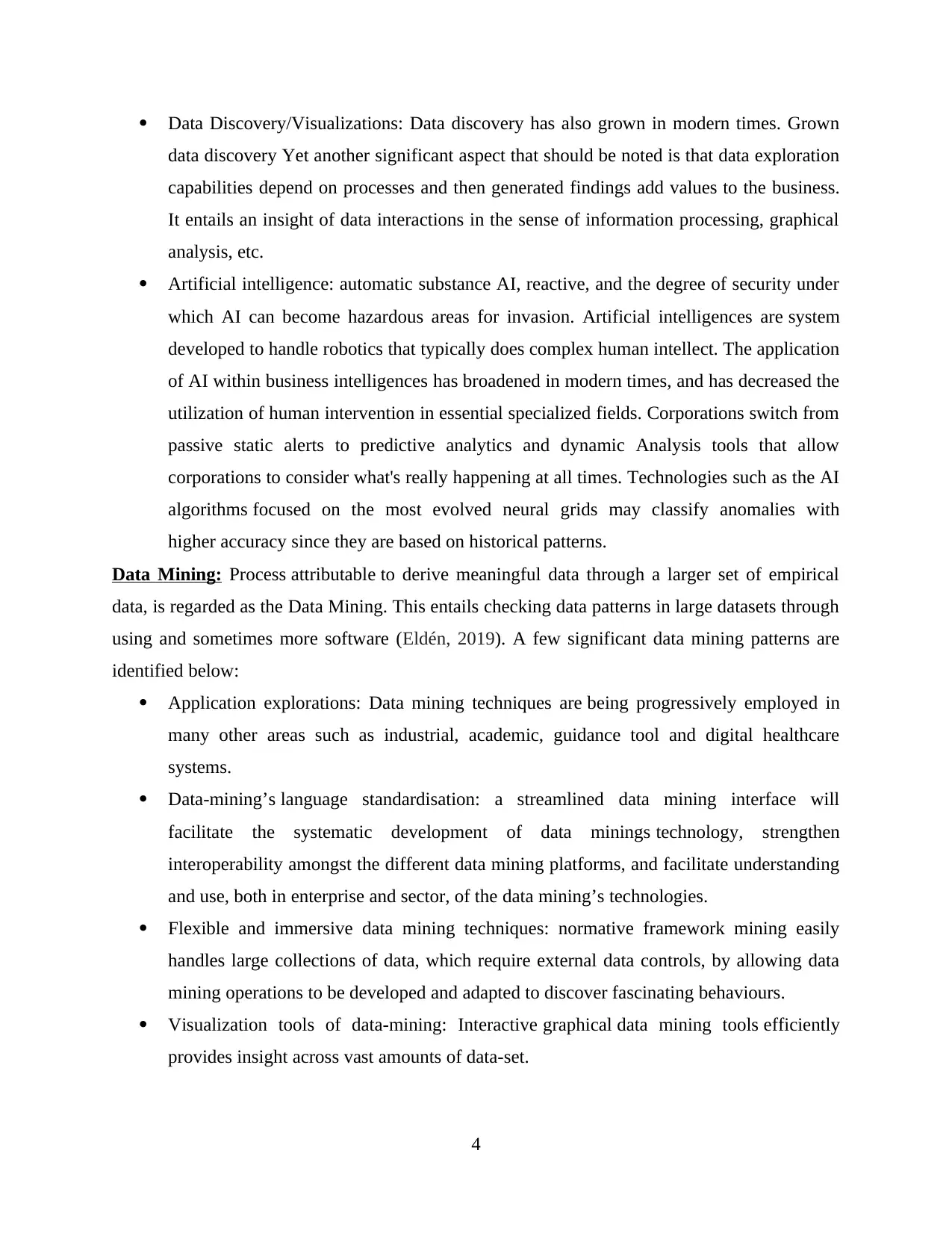
Data Discovery/Visualizations: Data discovery has also grown in modern times. Grown
data discovery Yet another significant aspect that should be noted is that data exploration
capabilities depend on processes and then generated findings add values to the business.
It entails an insight of data interactions in the sense of information processing, graphical
analysis, etc.
Artificial intelligence: automatic substance AI, reactive, and the degree of security under
which AI can become hazardous areas for invasion. Artificial intelligences are system
developed to handle robotics that typically does complex human intellect. The application
of AI within business intelligences has broadened in modern times, and has decreased the
utilization of human intervention in essential specialized fields. Corporations switch from
passive static alerts to predictive analytics and dynamic Analysis tools that allow
corporations to consider what's really happening at all times. Technologies such as the AI
algorithms focused on the most evolved neural grids may classify anomalies with
higher accuracy since they are based on historical patterns.
Data Mining: Process attributable to derive meaningful data through a larger set of empirical
data, is regarded as the Data Mining. This entails checking data patterns in large datasets through
using and sometimes more software (Eldén, 2019). A few significant data mining patterns are
identified below:
Application explorations: Data mining techniques are being progressively employed in
many other areas such as industrial, academic, guidance tool and digital healthcare
systems.
Data-mining’s language standardisation: a streamlined data mining interface will
facilitate the systematic development of data minings technology, strengthen
interoperability amongst the different data mining platforms, and facilitate understanding
and use, both in enterprise and sector, of the data mining’s technologies.
Flexible and immersive data mining techniques: normative framework mining easily
handles large collections of data, which require external data controls, by allowing data
mining operations to be developed and adapted to discover fascinating behaviours.
Visualization tools of data-mining: Interactive graphical data mining tools efficiently
provides insight across vast amounts of data-set.
4
data discovery Yet another significant aspect that should be noted is that data exploration
capabilities depend on processes and then generated findings add values to the business.
It entails an insight of data interactions in the sense of information processing, graphical
analysis, etc.
Artificial intelligence: automatic substance AI, reactive, and the degree of security under
which AI can become hazardous areas for invasion. Artificial intelligences are system
developed to handle robotics that typically does complex human intellect. The application
of AI within business intelligences has broadened in modern times, and has decreased the
utilization of human intervention in essential specialized fields. Corporations switch from
passive static alerts to predictive analytics and dynamic Analysis tools that allow
corporations to consider what's really happening at all times. Technologies such as the AI
algorithms focused on the most evolved neural grids may classify anomalies with
higher accuracy since they are based on historical patterns.
Data Mining: Process attributable to derive meaningful data through a larger set of empirical
data, is regarded as the Data Mining. This entails checking data patterns in large datasets through
using and sometimes more software (Eldén, 2019). A few significant data mining patterns are
identified below:
Application explorations: Data mining techniques are being progressively employed in
many other areas such as industrial, academic, guidance tool and digital healthcare
systems.
Data-mining’s language standardisation: a streamlined data mining interface will
facilitate the systematic development of data minings technology, strengthen
interoperability amongst the different data mining platforms, and facilitate understanding
and use, both in enterprise and sector, of the data mining’s technologies.
Flexible and immersive data mining techniques: normative framework mining easily
handles large collections of data, which require external data controls, by allowing data
mining operations to be developed and adapted to discover fascinating behaviours.
Visualization tools of data-mining: Interactive graphical data mining tools efficiently
provides insight across vast amounts of data-set.
4
Secure Best Marks with AI Grader
Need help grading? Try our AI Grader for instant feedback on your assignments.
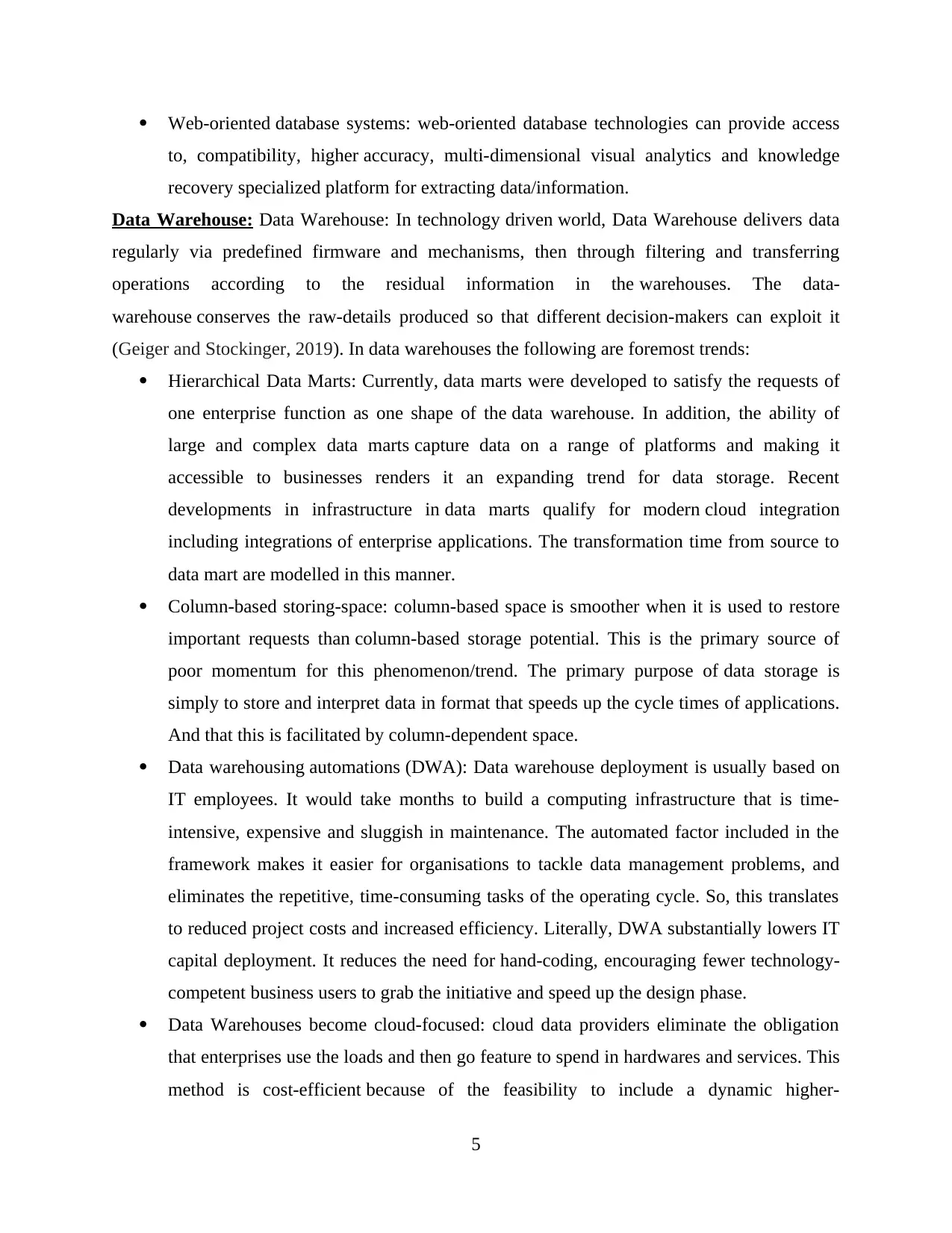
Web-oriented database systems: web-oriented database technologies can provide access
to, compatibility, higher accuracy, multi-dimensional visual analytics and knowledge
recovery specialized platform for extracting data/information.
Data Warehouse: Data Warehouse: In technology driven world, Data Warehouse delivers data
regularly via predefined firmware and mechanisms, then through filtering and transferring
operations according to the residual information in the warehouses. The data-
warehouse conserves the raw-details produced so that different decision-makers can exploit it
(Geiger and Stockinger, 2019). In data warehouses the following are foremost trends:
Hierarchical Data Marts: Currently, data marts were developed to satisfy the requests of
one enterprise function as one shape of the data warehouse. In addition, the ability of
large and complex data marts capture data on a range of platforms and making it
accessible to businesses renders it an expanding trend for data storage. Recent
developments in infrastructure in data marts qualify for modern cloud integration
including integrations of enterprise applications. The transformation time from source to
data mart are modelled in this manner.
Column-based storing-space: column-based space is smoother when it is used to restore
important requests than column-based storage potential. This is the primary source of
poor momentum for this phenomenon/trend. The primary purpose of data storage is
simply to store and interpret data in format that speeds up the cycle times of applications.
And that this is facilitated by column-dependent space.
Data warehousing automations (DWA): Data warehouse deployment is usually based on
IT employees. It would take months to build a computing infrastructure that is time-
intensive, expensive and sluggish in maintenance. The automated factor included in the
framework makes it easier for organisations to tackle data management problems, and
eliminates the repetitive, time-consuming tasks of the operating cycle. So, this translates
to reduced project costs and increased efficiency. Literally, DWA substantially lowers IT
capital deployment. It reduces the need for hand-coding, encouraging fewer technology-
competent business users to grab the initiative and speed up the design phase.
Data Warehouses become cloud-focused: cloud data providers eliminate the obligation
that enterprises use the loads and then go feature to spend in hardwares and services. This
method is cost-efficient because of the feasibility to include a dynamic higher-
5
to, compatibility, higher accuracy, multi-dimensional visual analytics and knowledge
recovery specialized platform for extracting data/information.
Data Warehouse: Data Warehouse: In technology driven world, Data Warehouse delivers data
regularly via predefined firmware and mechanisms, then through filtering and transferring
operations according to the residual information in the warehouses. The data-
warehouse conserves the raw-details produced so that different decision-makers can exploit it
(Geiger and Stockinger, 2019). In data warehouses the following are foremost trends:
Hierarchical Data Marts: Currently, data marts were developed to satisfy the requests of
one enterprise function as one shape of the data warehouse. In addition, the ability of
large and complex data marts capture data on a range of platforms and making it
accessible to businesses renders it an expanding trend for data storage. Recent
developments in infrastructure in data marts qualify for modern cloud integration
including integrations of enterprise applications. The transformation time from source to
data mart are modelled in this manner.
Column-based storing-space: column-based space is smoother when it is used to restore
important requests than column-based storage potential. This is the primary source of
poor momentum for this phenomenon/trend. The primary purpose of data storage is
simply to store and interpret data in format that speeds up the cycle times of applications.
And that this is facilitated by column-dependent space.
Data warehousing automations (DWA): Data warehouse deployment is usually based on
IT employees. It would take months to build a computing infrastructure that is time-
intensive, expensive and sluggish in maintenance. The automated factor included in the
framework makes it easier for organisations to tackle data management problems, and
eliminates the repetitive, time-consuming tasks of the operating cycle. So, this translates
to reduced project costs and increased efficiency. Literally, DWA substantially lowers IT
capital deployment. It reduces the need for hand-coding, encouraging fewer technology-
competent business users to grab the initiative and speed up the design phase.
Data Warehouses become cloud-focused: cloud data providers eliminate the obligation
that enterprises use the loads and then go feature to spend in hardwares and services. This
method is cost-efficient because of the feasibility to include a dynamic higher-
5
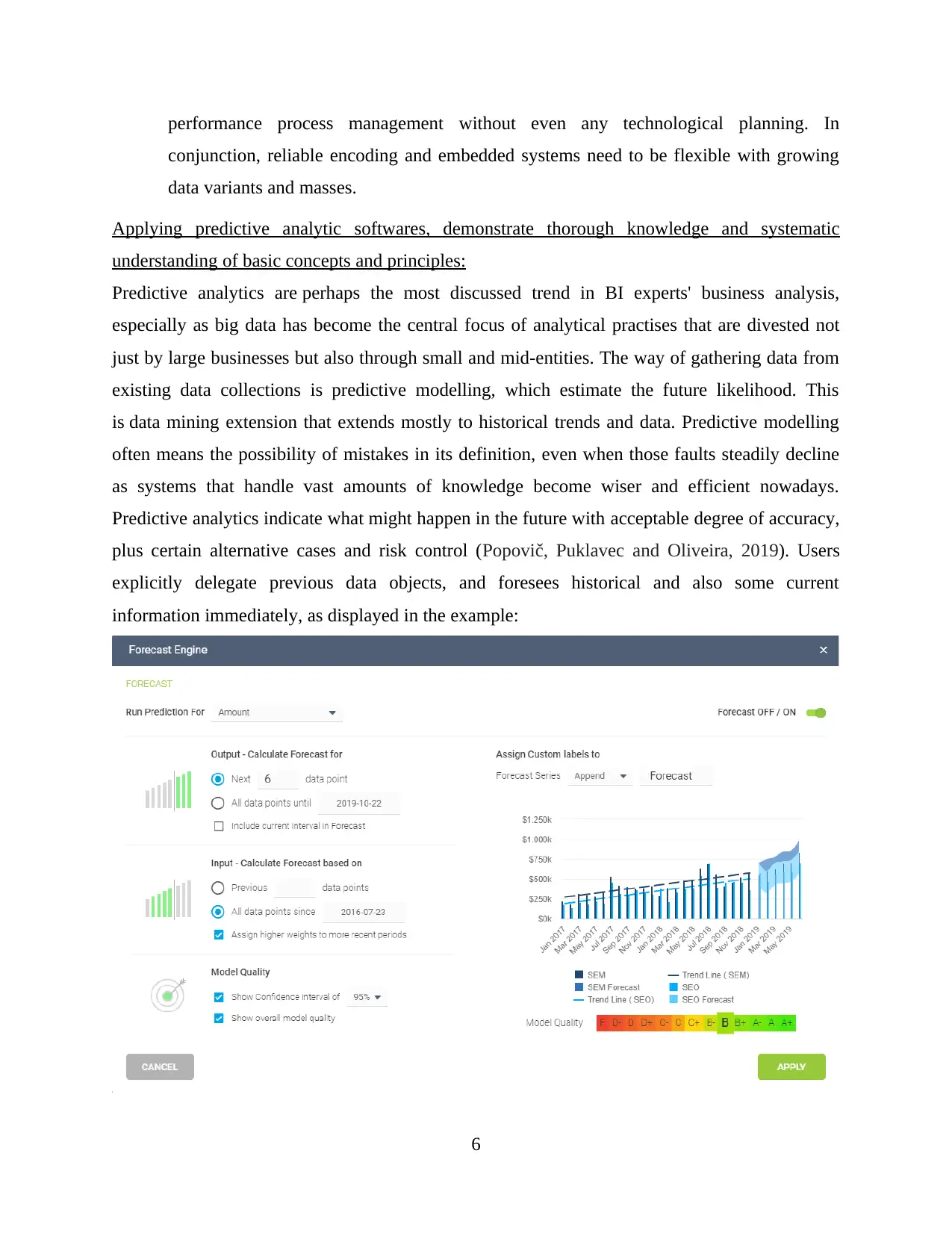
performance process management without even any technological planning. In
conjunction, reliable encoding and embedded systems need to be flexible with growing
data variants and masses.
Applying predictive analytic softwares, demonstrate thorough knowledge and systematic
understanding of basic concepts and principles:
Predictive analytics are perhaps the most discussed trend in BI experts' business analysis,
especially as big data has become the central focus of analytical practises that are divested not
just by large businesses but also through small and mid-entities. The way of gathering data from
existing data collections is predictive modelling, which estimate the future likelihood. This
is data mining extension that extends mostly to historical trends and data. Predictive modelling
often means the possibility of mistakes in its definition, even when those faults steadily decline
as systems that handle vast amounts of knowledge become wiser and efficient nowadays.
Predictive analytics indicate what might happen in the future with acceptable degree of accuracy,
plus certain alternative cases and risk control (Popovič, Puklavec and Oliveira, 2019). Users
explicitly delegate previous data objects, and foresees historical and also some current
information immediately, as displayed in the example:
6
conjunction, reliable encoding and embedded systems need to be flexible with growing
data variants and masses.
Applying predictive analytic softwares, demonstrate thorough knowledge and systematic
understanding of basic concepts and principles:
Predictive analytics are perhaps the most discussed trend in BI experts' business analysis,
especially as big data has become the central focus of analytical practises that are divested not
just by large businesses but also through small and mid-entities. The way of gathering data from
existing data collections is predictive modelling, which estimate the future likelihood. This
is data mining extension that extends mostly to historical trends and data. Predictive modelling
often means the possibility of mistakes in its definition, even when those faults steadily decline
as systems that handle vast amounts of knowledge become wiser and efficient nowadays.
Predictive analytics indicate what might happen in the future with acceptable degree of accuracy,
plus certain alternative cases and risk control (Popovič, Puklavec and Oliveira, 2019). Users
explicitly delegate previous data objects, and foresees historical and also some current
information immediately, as displayed in the example:
6
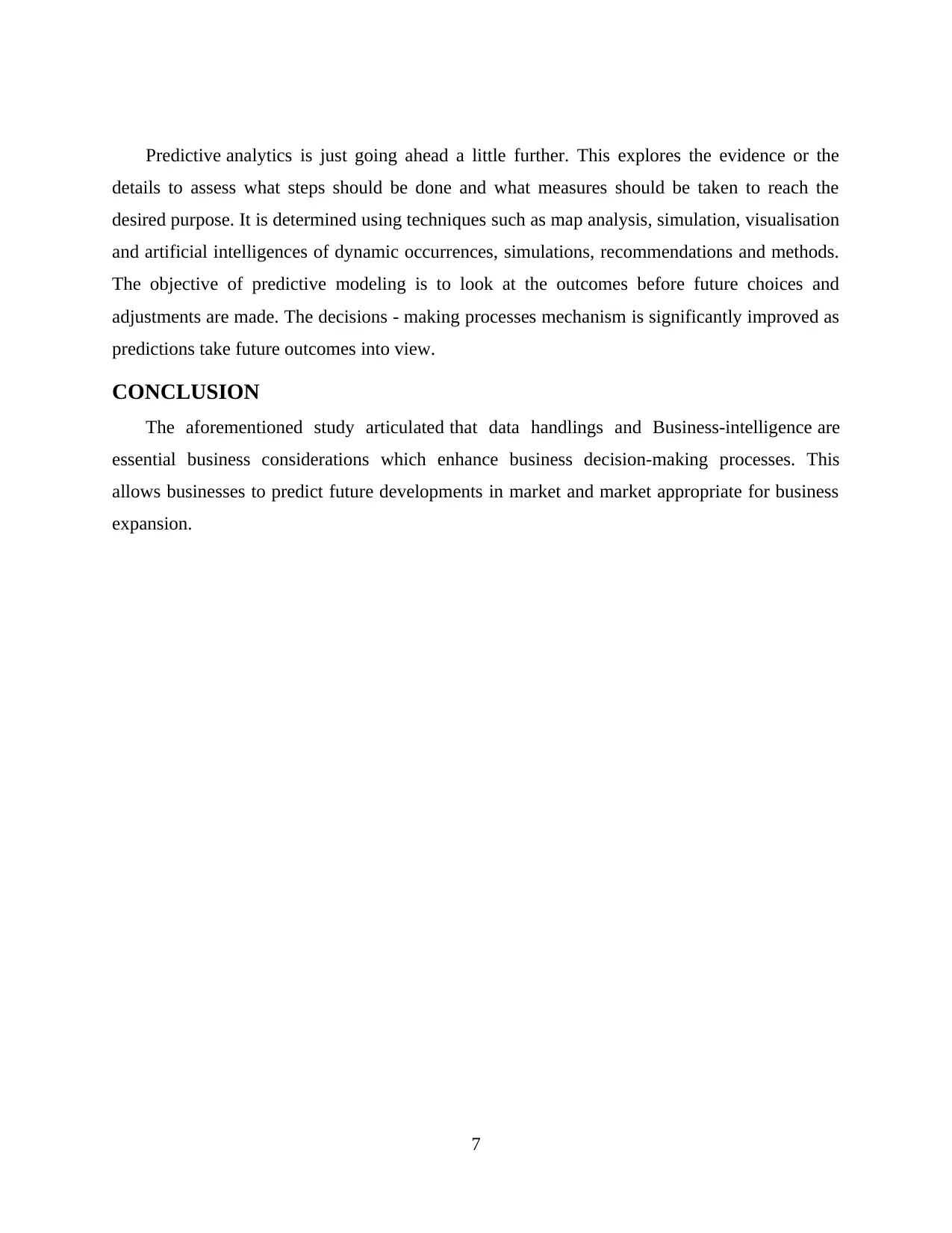
Predictive analytics is just going ahead a little further. This explores the evidence or the
details to assess what steps should be done and what measures should be taken to reach the
desired purpose. It is determined using techniques such as map analysis, simulation, visualisation
and artificial intelligences of dynamic occurrences, simulations, recommendations and methods.
The objective of predictive modeling is to look at the outcomes before future choices and
adjustments are made. The decisions - making processes mechanism is significantly improved as
predictions take future outcomes into view.
CONCLUSION
The aforementioned study articulated that data handlings and Business-intelligence are
essential business considerations which enhance business decision-making processes. This
allows businesses to predict future developments in market and market appropriate for business
expansion.
7
details to assess what steps should be done and what measures should be taken to reach the
desired purpose. It is determined using techniques such as map analysis, simulation, visualisation
and artificial intelligences of dynamic occurrences, simulations, recommendations and methods.
The objective of predictive modeling is to look at the outcomes before future choices and
adjustments are made. The decisions - making processes mechanism is significantly improved as
predictions take future outcomes into view.
CONCLUSION
The aforementioned study articulated that data handlings and Business-intelligence are
essential business considerations which enhance business decision-making processes. This
allows businesses to predict future developments in market and market appropriate for business
expansion.
7
Paraphrase This Document
Need a fresh take? Get an instant paraphrase of this document with our AI Paraphraser
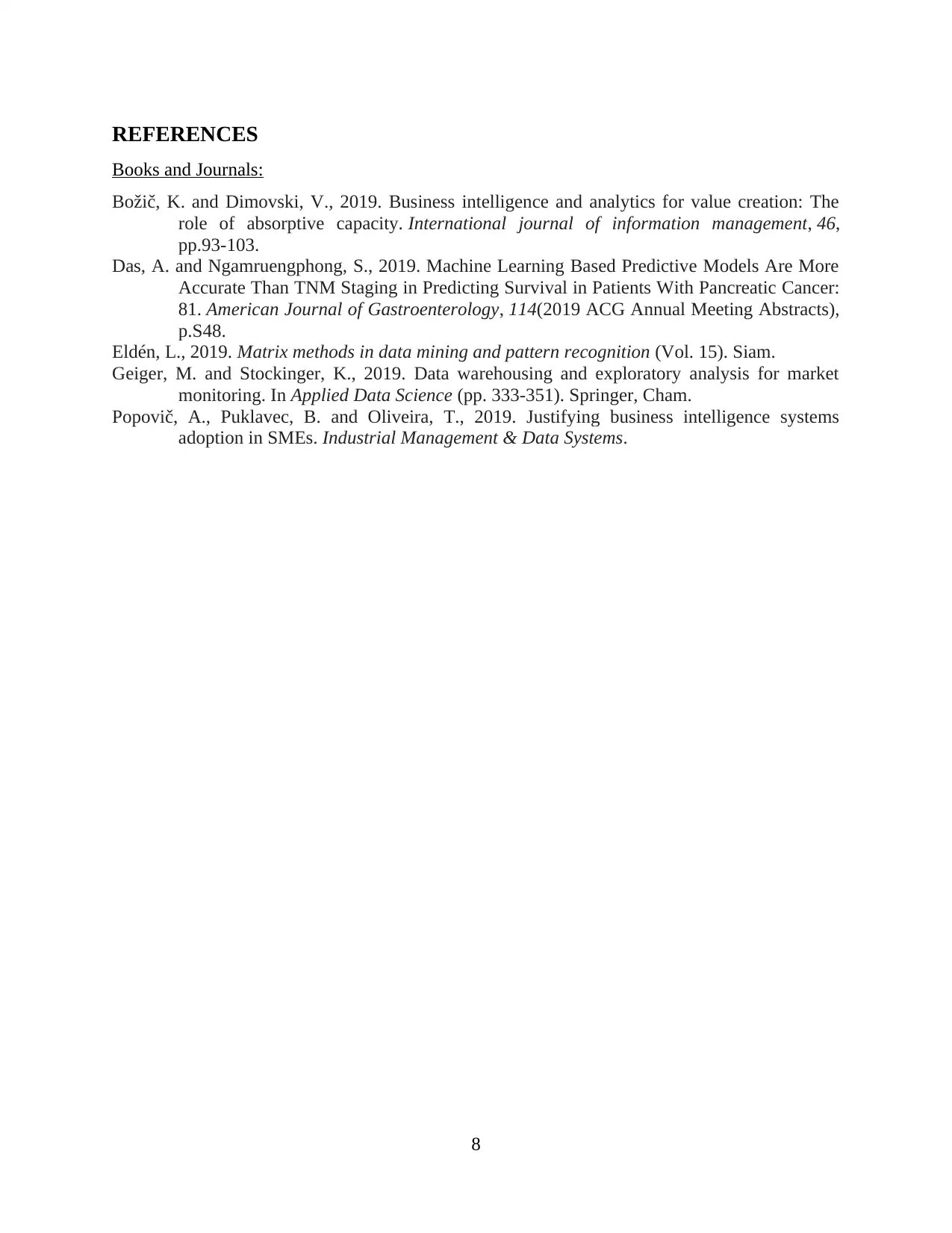
REFERENCES
Books and Journals:
Božič, K. and Dimovski, V., 2019. Business intelligence and analytics for value creation: The
role of absorptive capacity. International journal of information management, 46,
pp.93-103.
Das, A. and Ngamruengphong, S., 2019. Machine Learning Based Predictive Models Are More
Accurate Than TNM Staging in Predicting Survival in Patients With Pancreatic Cancer:
81. American Journal of Gastroenterology, 114(2019 ACG Annual Meeting Abstracts),
p.S48.
Eldén, L., 2019. Matrix methods in data mining and pattern recognition (Vol. 15). Siam.
Geiger, M. and Stockinger, K., 2019. Data warehousing and exploratory analysis for market
monitoring. In Applied Data Science (pp. 333-351). Springer, Cham.
Popovič, A., Puklavec, B. and Oliveira, T., 2019. Justifying business intelligence systems
adoption in SMEs. Industrial Management & Data Systems.
8
Books and Journals:
Božič, K. and Dimovski, V., 2019. Business intelligence and analytics for value creation: The
role of absorptive capacity. International journal of information management, 46,
pp.93-103.
Das, A. and Ngamruengphong, S., 2019. Machine Learning Based Predictive Models Are More
Accurate Than TNM Staging in Predicting Survival in Patients With Pancreatic Cancer:
81. American Journal of Gastroenterology, 114(2019 ACG Annual Meeting Abstracts),
p.S48.
Eldén, L., 2019. Matrix methods in data mining and pattern recognition (Vol. 15). Siam.
Geiger, M. and Stockinger, K., 2019. Data warehousing and exploratory analysis for market
monitoring. In Applied Data Science (pp. 333-351). Springer, Cham.
Popovič, A., Puklavec, B. and Oliveira, T., 2019. Justifying business intelligence systems
adoption in SMEs. Industrial Management & Data Systems.
8
1 out of 8
Related Documents
![[object Object]](/_next/image/?url=%2F_next%2Fstatic%2Fmedia%2Flogo.6d15ce61.png&w=640&q=75)
Your All-in-One AI-Powered Toolkit for Academic Success.
+13062052269
info@desklib.com
Available 24*7 on WhatsApp / Email
Unlock your academic potential
© 2024 | Zucol Services PVT LTD | All rights reserved.