Exploring Trends in Data Handling and Business Intelligence
VerifiedAdded on 2023/01/11
|7
|1754
|88
AI Summary
This report explores the latest trends in data handling, business intelligence, and data mining. It discusses the importance of business intelligence and the impact of data warehousing. It also highlights the role of predictive analytics in decision-making.
Contribute Materials
Your contribution can guide someone’s learning journey. Share your
documents today.
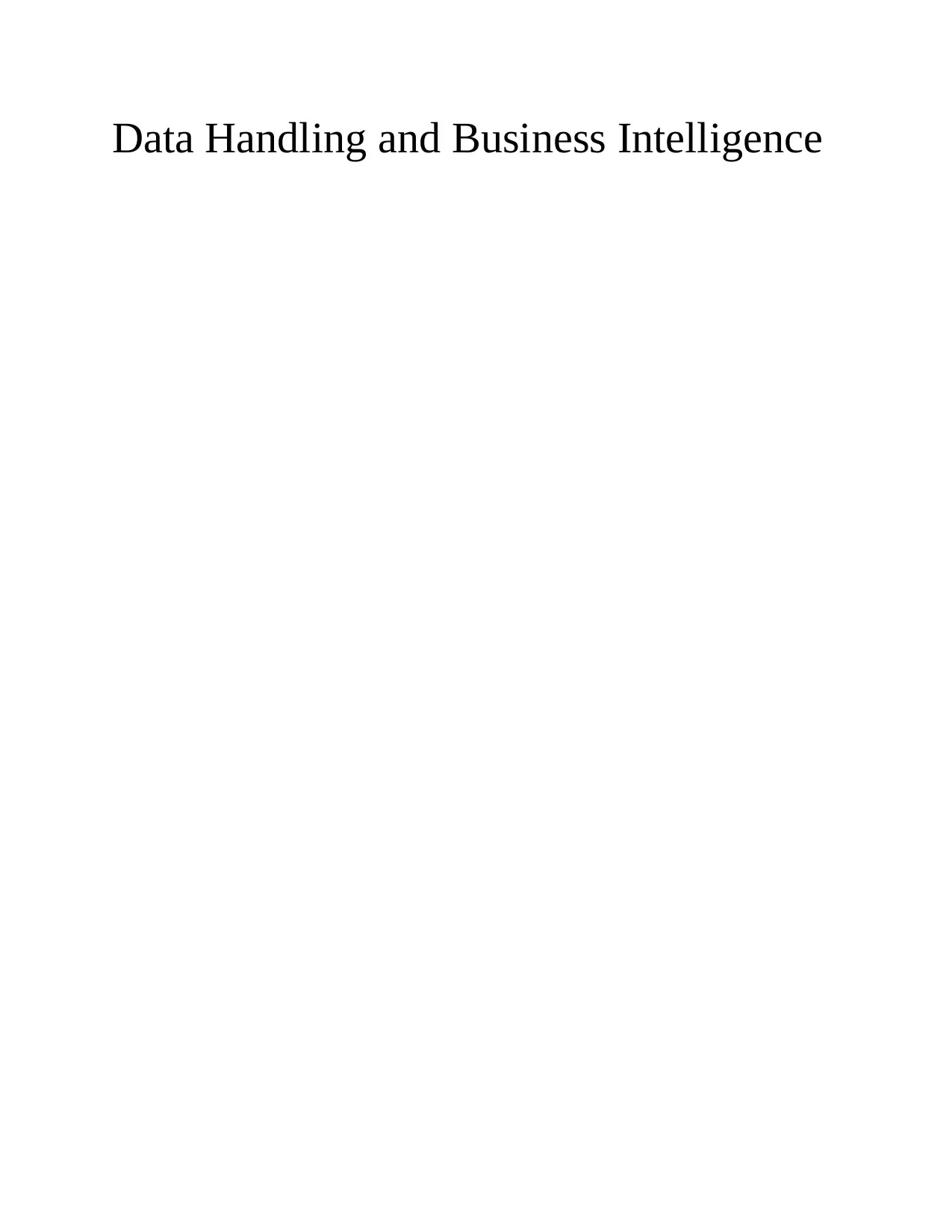
Data Handling and Business Intelligence
Secure Best Marks with AI Grader
Need help grading? Try our AI Grader for instant feedback on your assignments.
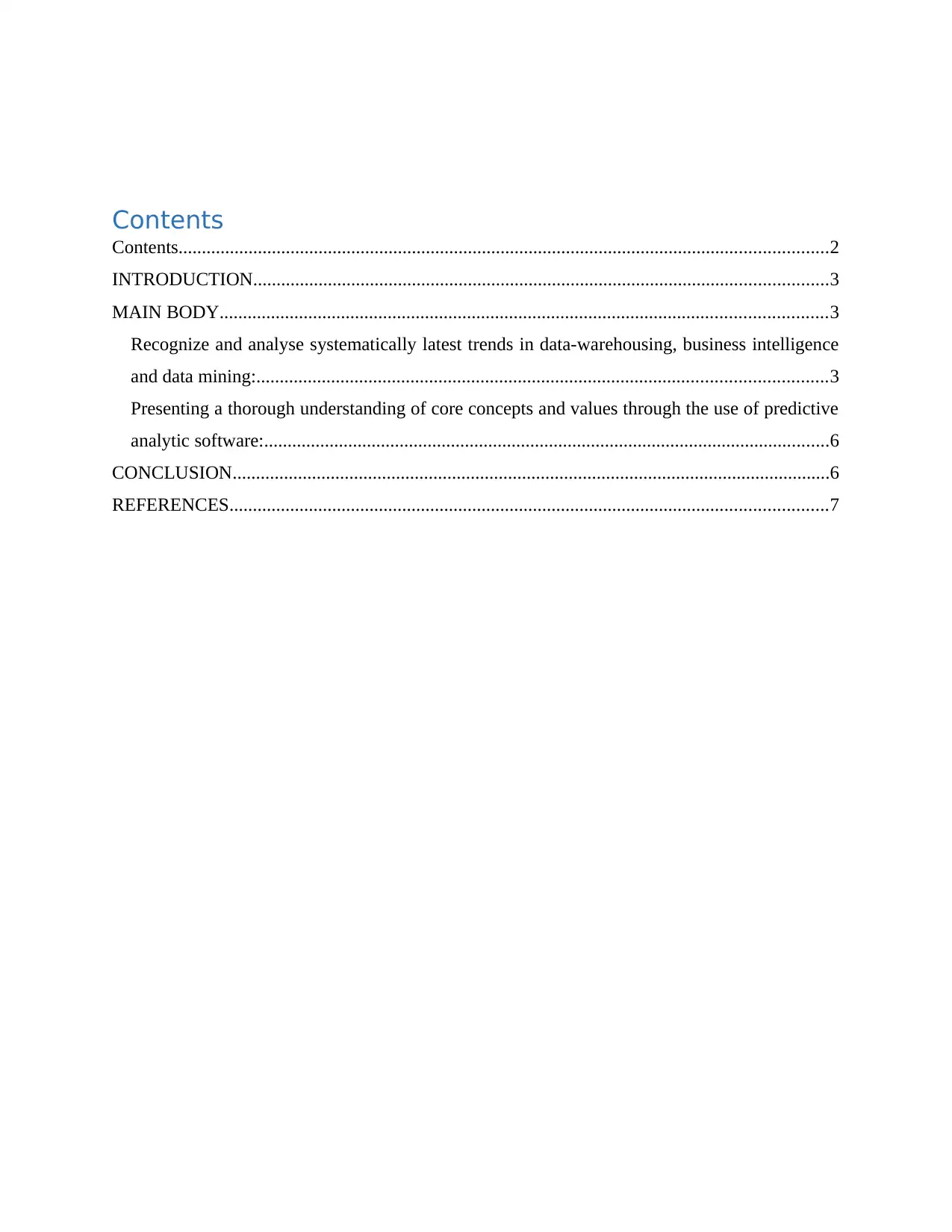
Contents
Contents...........................................................................................................................................2
INTRODUCTION...........................................................................................................................3
MAIN BODY..................................................................................................................................3
Recognize and analyse systematically latest trends in data-warehousing, business intelligence
and data mining:..........................................................................................................................3
Presenting a thorough understanding of core concepts and values through the use of predictive
analytic software:.........................................................................................................................6
CONCLUSION................................................................................................................................6
REFERENCES................................................................................................................................7
Contents...........................................................................................................................................2
INTRODUCTION...........................................................................................................................3
MAIN BODY..................................................................................................................................3
Recognize and analyse systematically latest trends in data-warehousing, business intelligence
and data mining:..........................................................................................................................3
Presenting a thorough understanding of core concepts and values through the use of predictive
analytic software:.........................................................................................................................6
CONCLUSION................................................................................................................................6
REFERENCES................................................................................................................................7
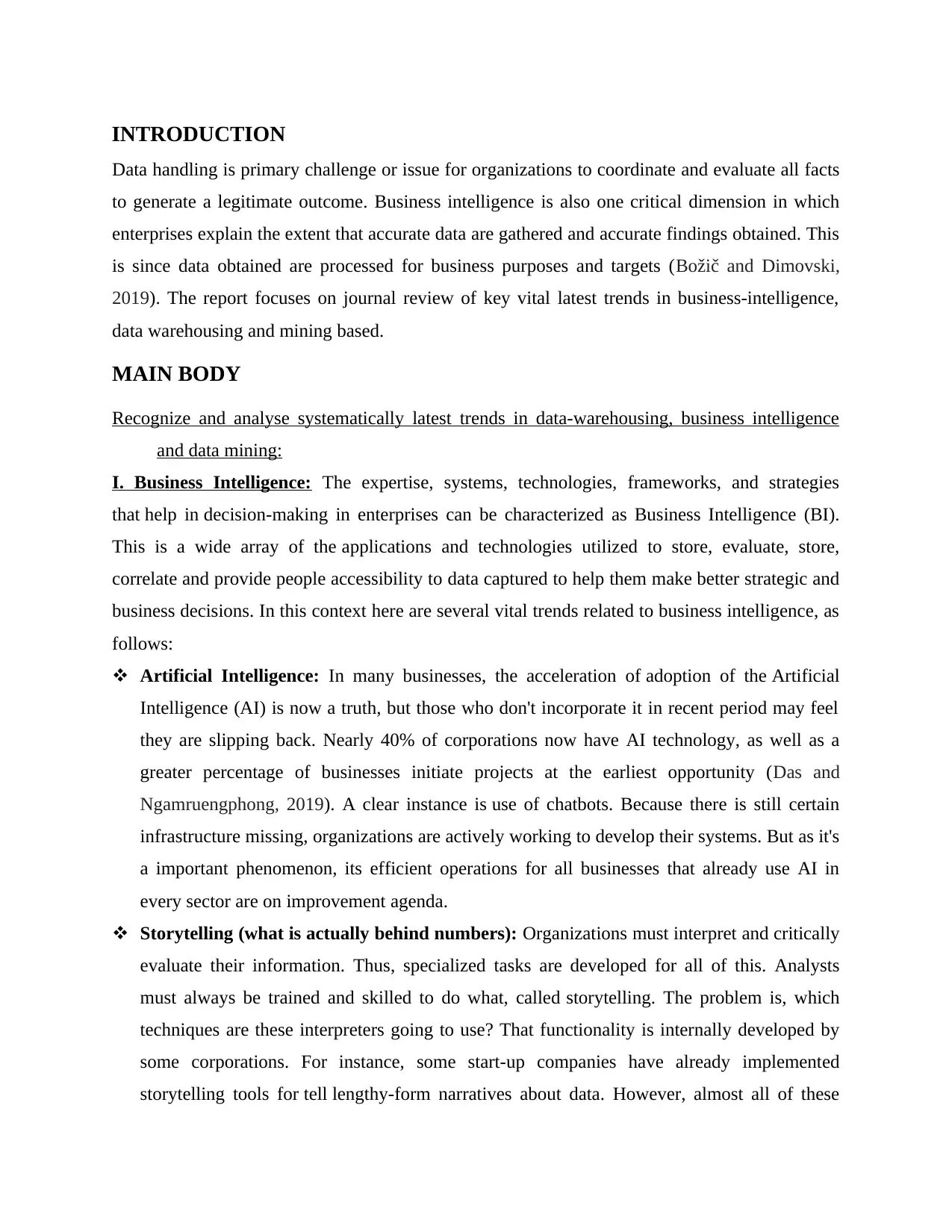
INTRODUCTION
Data handling is primary challenge or issue for organizations to coordinate and evaluate all facts
to generate a legitimate outcome. Business intelligence is also one critical dimension in which
enterprises explain the extent that accurate data are gathered and accurate findings obtained. This
is since data obtained are processed for business purposes and targets (Božič and Dimovski,
2019). The report focuses on journal review of key vital latest trends in business-intelligence,
data warehousing and mining based.
MAIN BODY
Recognize and analyse systematically latest trends in data-warehousing, business intelligence
and data mining:
I. Business Intelligence: The expertise, systems, technologies, frameworks, and strategies
that help in decision-making in enterprises can be characterized as Business Intelligence (BI).
This is a wide array of the applications and technologies utilized to store, evaluate, store,
correlate and provide people accessibility to data captured to help them make better strategic and
business decisions. In this context here are several vital trends related to business intelligence, as
follows:
Artificial Intelligence: In many businesses, the acceleration of adoption of the Artificial
Intelligence (AI) is now a truth, but those who don't incorporate it in recent period may feel
they are slipping back. Nearly 40% of corporations now have AI technology, as well as a
greater percentage of businesses initiate projects at the earliest opportunity (Das and
Ngamruengphong, 2019). A clear instance is use of chatbots. Because there is still certain
infrastructure missing, organizations are actively working to develop their systems. But as it's
a important phenomenon, its efficient operations for all businesses that already use AI in
every sector are on improvement agenda.
Storytelling (what is actually behind numbers): Organizations must interpret and critically
evaluate their information. Thus, specialized tasks are developed for all of this. Analysts
must always be trained and skilled to do what, called storytelling. The problem is, which
techniques are these interpreters going to use? That functionality is internally developed by
some corporations. For instance, some start-up companies have already implemented
storytelling tools for tell lengthy-form narratives about data. However, almost all of these
Data handling is primary challenge or issue for organizations to coordinate and evaluate all facts
to generate a legitimate outcome. Business intelligence is also one critical dimension in which
enterprises explain the extent that accurate data are gathered and accurate findings obtained. This
is since data obtained are processed for business purposes and targets (Božič and Dimovski,
2019). The report focuses on journal review of key vital latest trends in business-intelligence,
data warehousing and mining based.
MAIN BODY
Recognize and analyse systematically latest trends in data-warehousing, business intelligence
and data mining:
I. Business Intelligence: The expertise, systems, technologies, frameworks, and strategies
that help in decision-making in enterprises can be characterized as Business Intelligence (BI).
This is a wide array of the applications and technologies utilized to store, evaluate, store,
correlate and provide people accessibility to data captured to help them make better strategic and
business decisions. In this context here are several vital trends related to business intelligence, as
follows:
Artificial Intelligence: In many businesses, the acceleration of adoption of the Artificial
Intelligence (AI) is now a truth, but those who don't incorporate it in recent period may feel
they are slipping back. Nearly 40% of corporations now have AI technology, as well as a
greater percentage of businesses initiate projects at the earliest opportunity (Das and
Ngamruengphong, 2019). A clear instance is use of chatbots. Because there is still certain
infrastructure missing, organizations are actively working to develop their systems. But as it's
a important phenomenon, its efficient operations for all businesses that already use AI in
every sector are on improvement agenda.
Storytelling (what is actually behind numbers): Organizations must interpret and critically
evaluate their information. Thus, specialized tasks are developed for all of this. Analysts
must always be trained and skilled to do what, called storytelling. The problem is, which
techniques are these interpreters going to use? That functionality is internally developed by
some corporations. For instance, some start-up companies have already implemented
storytelling tools for tell lengthy-form narratives about data. However, almost all of these
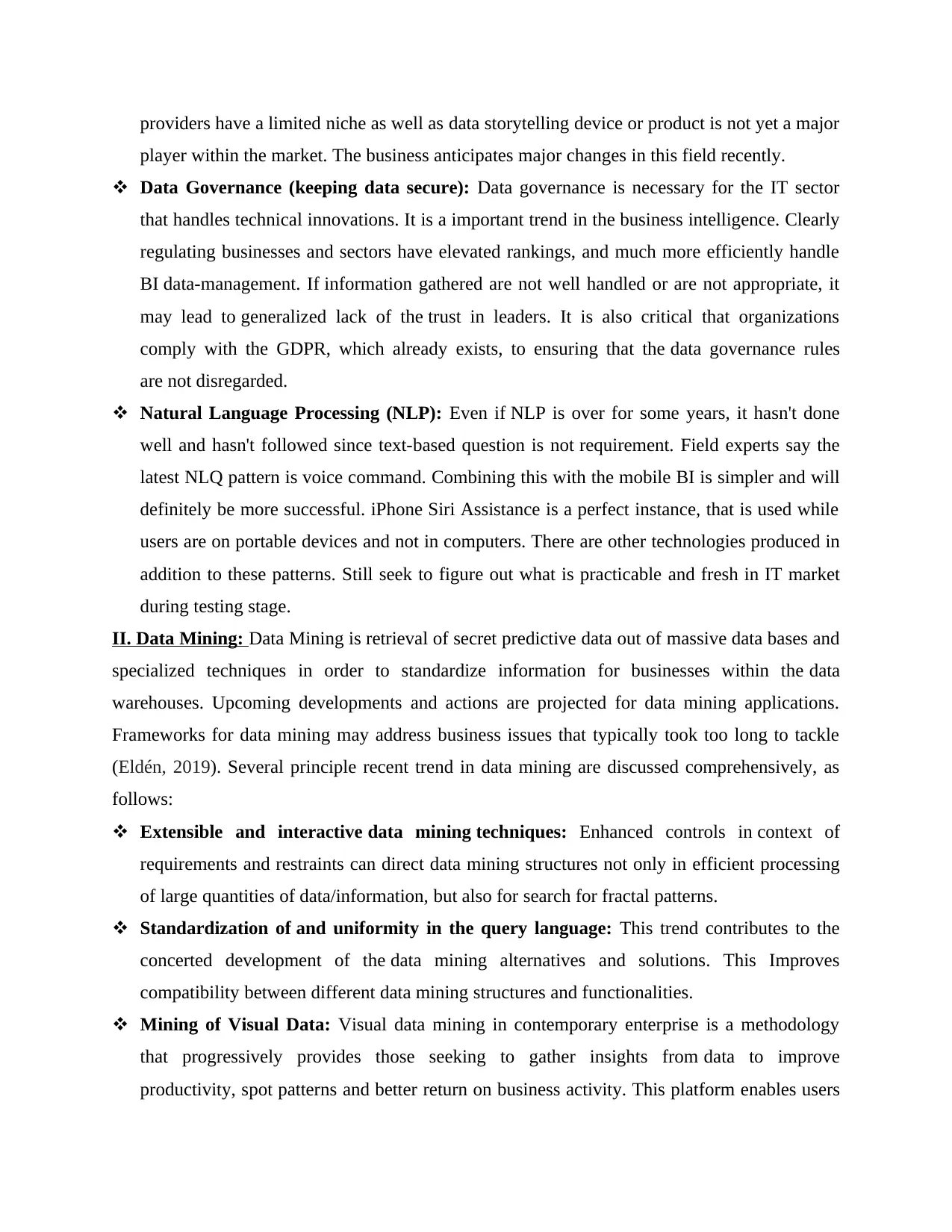
providers have a limited niche as well as data storytelling device or product is not yet a major
player within the market. The business anticipates major changes in this field recently.
Data Governance (keeping data secure): Data governance is necessary for the IT sector
that handles technical innovations. It is a important trend in the business intelligence. Clearly
regulating businesses and sectors have elevated rankings, and much more efficiently handle
BI data-management. If information gathered are not well handled or are not appropriate, it
may lead to generalized lack of the trust in leaders. It is also critical that organizations
comply with the GDPR, which already exists, to ensuring that the data governance rules
are not disregarded.
Natural Language Processing (NLP): Even if NLP is over for some years, it hasn't done
well and hasn't followed since text-based question is not requirement. Field experts say the
latest NLQ pattern is voice command. Combining this with the mobile BI is simpler and will
definitely be more successful. iPhone Siri Assistance is a perfect instance, that is used while
users are on portable devices and not in computers. There are other technologies produced in
addition to these patterns. Still seek to figure out what is practicable and fresh in IT market
during testing stage.
II. Data Mining: Data Mining is retrieval of secret predictive data out of massive data bases and
specialized techniques in order to standardize information for businesses within the data
warehouses. Upcoming developments and actions are projected for data mining applications.
Frameworks for data mining may address business issues that typically took too long to tackle
(Eldén, 2019). Several principle recent trend in data mining are discussed comprehensively, as
follows:
Extensible and interactive data mining techniques: Enhanced controls in context of
requirements and restraints can direct data mining structures not only in efficient processing
of large quantities of data/information, but also for search for fractal patterns.
Standardization of and uniformity in the query language: This trend contributes to the
concerted development of the data mining alternatives and solutions. This Improves
compatibility between different data mining structures and functionalities.
Mining of Visual Data: Visual data mining in contemporary enterprise is a methodology
that progressively provides those seeking to gather insights from data to improve
productivity, spot patterns and better return on business activity. This platform enables users
player within the market. The business anticipates major changes in this field recently.
Data Governance (keeping data secure): Data governance is necessary for the IT sector
that handles technical innovations. It is a important trend in the business intelligence. Clearly
regulating businesses and sectors have elevated rankings, and much more efficiently handle
BI data-management. If information gathered are not well handled or are not appropriate, it
may lead to generalized lack of the trust in leaders. It is also critical that organizations
comply with the GDPR, which already exists, to ensuring that the data governance rules
are not disregarded.
Natural Language Processing (NLP): Even if NLP is over for some years, it hasn't done
well and hasn't followed since text-based question is not requirement. Field experts say the
latest NLQ pattern is voice command. Combining this with the mobile BI is simpler and will
definitely be more successful. iPhone Siri Assistance is a perfect instance, that is used while
users are on portable devices and not in computers. There are other technologies produced in
addition to these patterns. Still seek to figure out what is practicable and fresh in IT market
during testing stage.
II. Data Mining: Data Mining is retrieval of secret predictive data out of massive data bases and
specialized techniques in order to standardize information for businesses within the data
warehouses. Upcoming developments and actions are projected for data mining applications.
Frameworks for data mining may address business issues that typically took too long to tackle
(Eldén, 2019). Several principle recent trend in data mining are discussed comprehensively, as
follows:
Extensible and interactive data mining techniques: Enhanced controls in context of
requirements and restraints can direct data mining structures not only in efficient processing
of large quantities of data/information, but also for search for fractal patterns.
Standardization of and uniformity in the query language: This trend contributes to the
concerted development of the data mining alternatives and solutions. This Improves
compatibility between different data mining structures and functionalities.
Mining of Visual Data: Visual data mining in contemporary enterprise is a methodology
that progressively provides those seeking to gather insights from data to improve
productivity, spot patterns and better return on business activity. This platform enables users
Secure Best Marks with AI Grader
Need help grading? Try our AI Grader for instant feedback on your assignments.
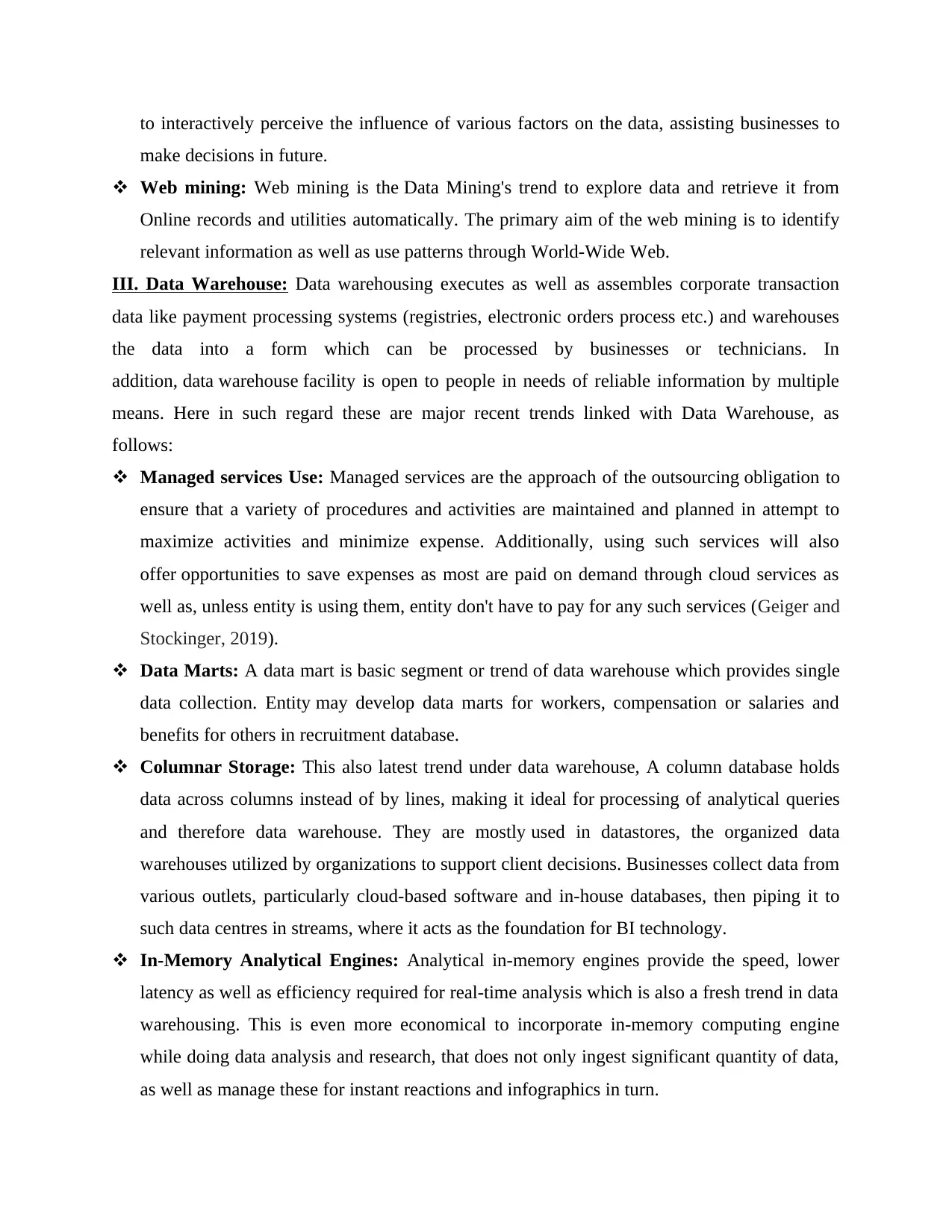
to interactively perceive the influence of various factors on the data, assisting businesses to
make decisions in future.
Web mining: Web mining is the Data Mining's trend to explore data and retrieve it from
Online records and utilities automatically. The primary aim of the web mining is to identify
relevant information as well as use patterns through World-Wide Web.
III. Data Warehouse: Data warehousing executes as well as assembles corporate transaction
data like payment processing systems (registries, electronic orders process etc.) and warehouses
the data into a form which can be processed by businesses or technicians. In
addition, data warehouse facility is open to people in needs of reliable information by multiple
means. Here in such regard these are major recent trends linked with Data Warehouse, as
follows:
Managed services Use: Managed services are the approach of the outsourcing obligation to
ensure that a variety of procedures and activities are maintained and planned in attempt to
maximize activities and minimize expense. Additionally, using such services will also
offer opportunities to save expenses as most are paid on demand through cloud services as
well as, unless entity is using them, entity don't have to pay for any such services (Geiger and
Stockinger, 2019).
Data Marts: A data mart is basic segment or trend of data warehouse which provides single
data collection. Entity may develop data marts for workers, compensation or salaries and
benefits for others in recruitment database.
Columnar Storage: This also latest trend under data warehouse, A column database holds
data across columns instead of by lines, making it ideal for processing of analytical queries
and therefore data warehouse. They are mostly used in datastores, the organized data
warehouses utilized by organizations to support client decisions. Businesses collect data from
various outlets, particularly cloud-based software and in-house databases, then piping it to
such data centres in streams, where it acts as the foundation for BI technology.
In-Memory Analytical Engines: Analytical in-memory engines provide the speed, lower
latency as well as efficiency required for real-time analysis which is also a fresh trend in data
warehousing. This is even more economical to incorporate in-memory computing engine
while doing data analysis and research, that does not only ingest significant quantity of data,
as well as manage these for instant reactions and infographics in turn.
make decisions in future.
Web mining: Web mining is the Data Mining's trend to explore data and retrieve it from
Online records and utilities automatically. The primary aim of the web mining is to identify
relevant information as well as use patterns through World-Wide Web.
III. Data Warehouse: Data warehousing executes as well as assembles corporate transaction
data like payment processing systems (registries, electronic orders process etc.) and warehouses
the data into a form which can be processed by businesses or technicians. In
addition, data warehouse facility is open to people in needs of reliable information by multiple
means. Here in such regard these are major recent trends linked with Data Warehouse, as
follows:
Managed services Use: Managed services are the approach of the outsourcing obligation to
ensure that a variety of procedures and activities are maintained and planned in attempt to
maximize activities and minimize expense. Additionally, using such services will also
offer opportunities to save expenses as most are paid on demand through cloud services as
well as, unless entity is using them, entity don't have to pay for any such services (Geiger and
Stockinger, 2019).
Data Marts: A data mart is basic segment or trend of data warehouse which provides single
data collection. Entity may develop data marts for workers, compensation or salaries and
benefits for others in recruitment database.
Columnar Storage: This also latest trend under data warehouse, A column database holds
data across columns instead of by lines, making it ideal for processing of analytical queries
and therefore data warehouse. They are mostly used in datastores, the organized data
warehouses utilized by organizations to support client decisions. Businesses collect data from
various outlets, particularly cloud-based software and in-house databases, then piping it to
such data centres in streams, where it acts as the foundation for BI technology.
In-Memory Analytical Engines: Analytical in-memory engines provide the speed, lower
latency as well as efficiency required for real-time analysis which is also a fresh trend in data
warehousing. This is even more economical to incorporate in-memory computing engine
while doing data analysis and research, that does not only ingest significant quantity of data,
as well as manage these for instant reactions and infographics in turn.
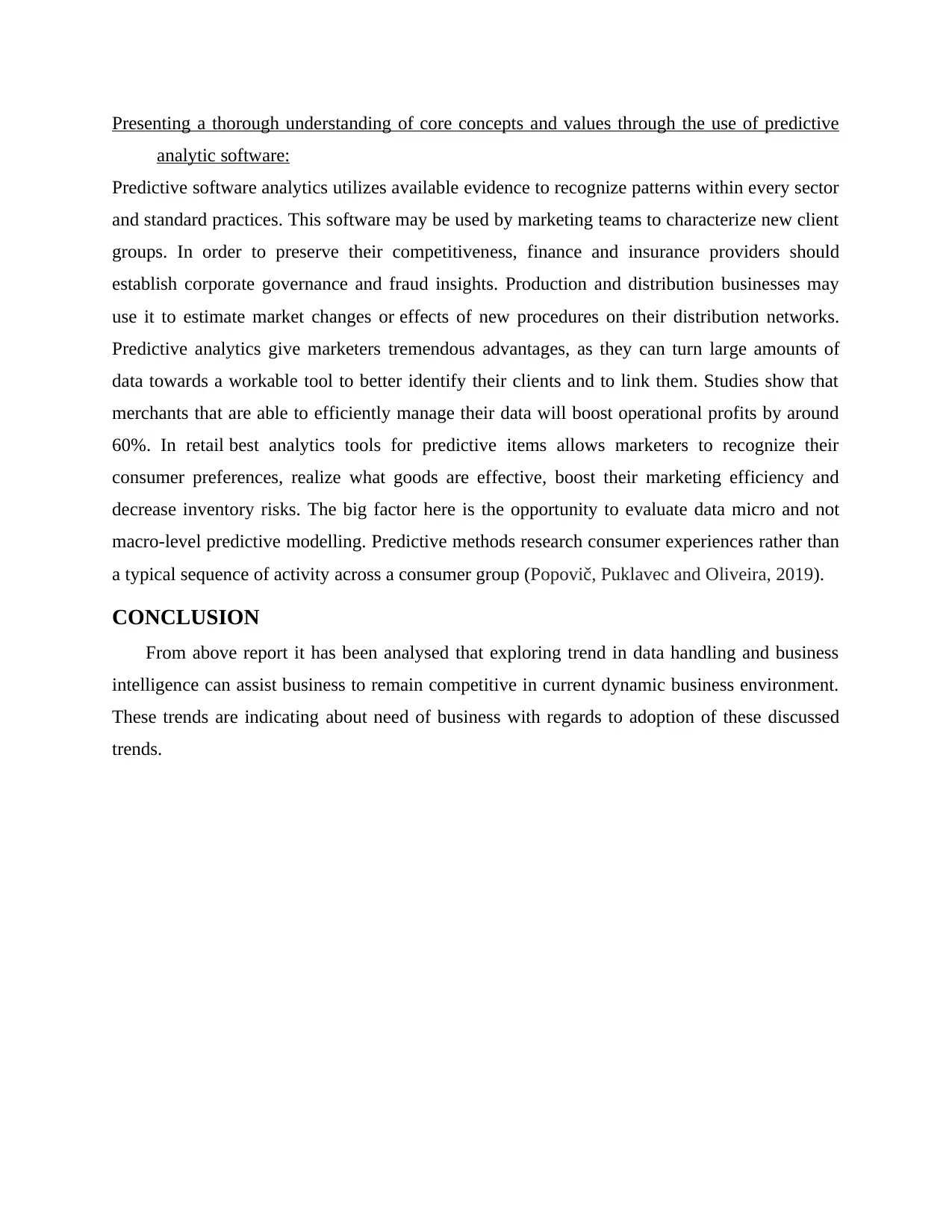
Presenting a thorough understanding of core concepts and values through the use of predictive
analytic software:
Predictive software analytics utilizes available evidence to recognize patterns within every sector
and standard practices. This software may be used by marketing teams to characterize new client
groups. In order to preserve their competitiveness, finance and insurance providers should
establish corporate governance and fraud insights. Production and distribution businesses may
use it to estimate market changes or effects of new procedures on their distribution networks.
Predictive analytics give marketers tremendous advantages, as they can turn large amounts of
data towards a workable tool to better identify their clients and to link them. Studies show that
merchants that are able to efficiently manage their data will boost operational profits by around
60%. In retail best analytics tools for predictive items allows marketers to recognize their
consumer preferences, realize what goods are effective, boost their marketing efficiency and
decrease inventory risks. The big factor here is the opportunity to evaluate data micro and not
macro-level predictive modelling. Predictive methods research consumer experiences rather than
a typical sequence of activity across a consumer group (Popovič, Puklavec and Oliveira, 2019).
CONCLUSION
From above report it has been analysed that exploring trend in data handling and business
intelligence can assist business to remain competitive in current dynamic business environment.
These trends are indicating about need of business with regards to adoption of these discussed
trends.
analytic software:
Predictive software analytics utilizes available evidence to recognize patterns within every sector
and standard practices. This software may be used by marketing teams to characterize new client
groups. In order to preserve their competitiveness, finance and insurance providers should
establish corporate governance and fraud insights. Production and distribution businesses may
use it to estimate market changes or effects of new procedures on their distribution networks.
Predictive analytics give marketers tremendous advantages, as they can turn large amounts of
data towards a workable tool to better identify their clients and to link them. Studies show that
merchants that are able to efficiently manage their data will boost operational profits by around
60%. In retail best analytics tools for predictive items allows marketers to recognize their
consumer preferences, realize what goods are effective, boost their marketing efficiency and
decrease inventory risks. The big factor here is the opportunity to evaluate data micro and not
macro-level predictive modelling. Predictive methods research consumer experiences rather than
a typical sequence of activity across a consumer group (Popovič, Puklavec and Oliveira, 2019).
CONCLUSION
From above report it has been analysed that exploring trend in data handling and business
intelligence can assist business to remain competitive in current dynamic business environment.
These trends are indicating about need of business with regards to adoption of these discussed
trends.
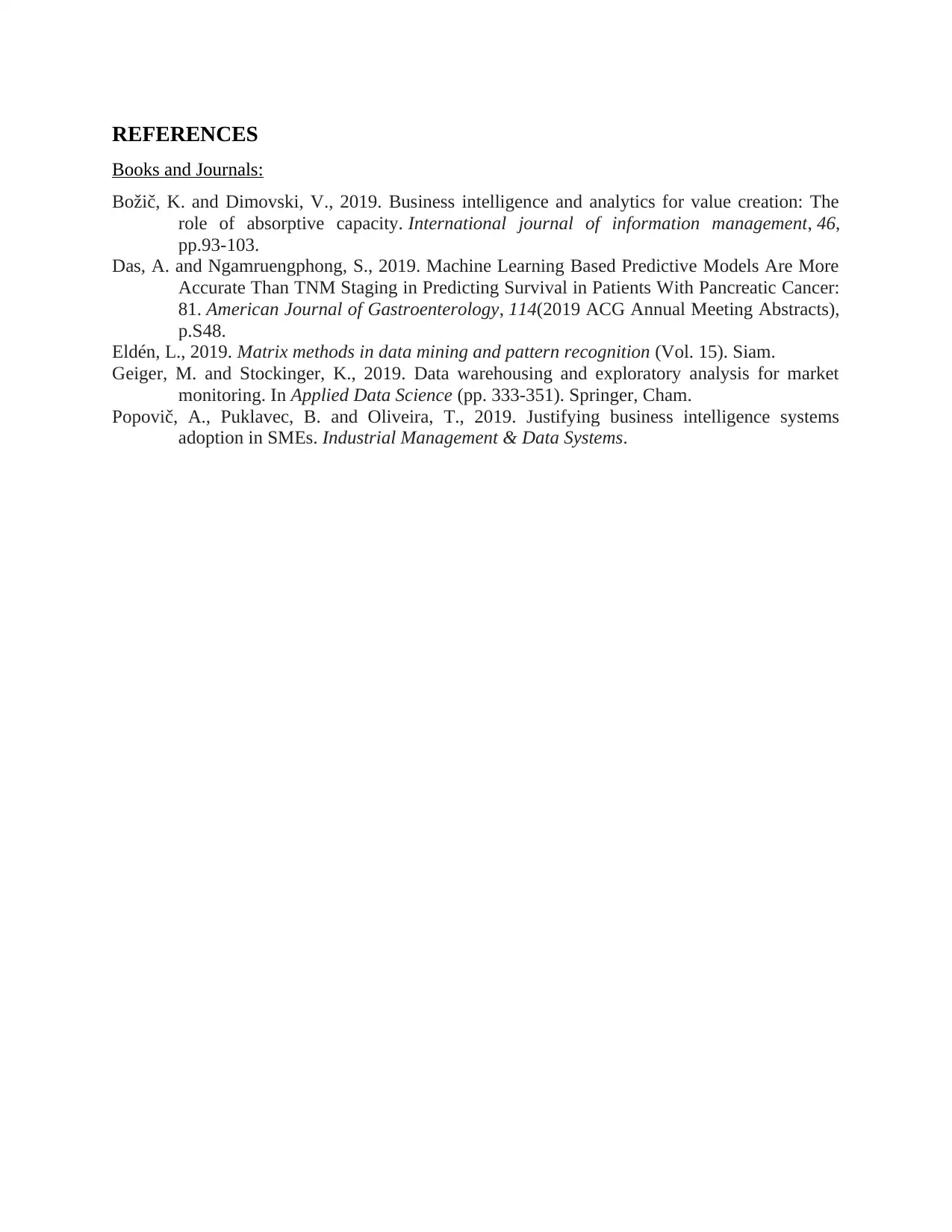
REFERENCES
Books and Journals:
Božič, K. and Dimovski, V., 2019. Business intelligence and analytics for value creation: The
role of absorptive capacity. International journal of information management, 46,
pp.93-103.
Das, A. and Ngamruengphong, S., 2019. Machine Learning Based Predictive Models Are More
Accurate Than TNM Staging in Predicting Survival in Patients With Pancreatic Cancer:
81. American Journal of Gastroenterology, 114(2019 ACG Annual Meeting Abstracts),
p.S48.
Eldén, L., 2019. Matrix methods in data mining and pattern recognition (Vol. 15). Siam.
Geiger, M. and Stockinger, K., 2019. Data warehousing and exploratory analysis for market
monitoring. In Applied Data Science (pp. 333-351). Springer, Cham.
Popovič, A., Puklavec, B. and Oliveira, T., 2019. Justifying business intelligence systems
adoption in SMEs. Industrial Management & Data Systems.
Books and Journals:
Božič, K. and Dimovski, V., 2019. Business intelligence and analytics for value creation: The
role of absorptive capacity. International journal of information management, 46,
pp.93-103.
Das, A. and Ngamruengphong, S., 2019. Machine Learning Based Predictive Models Are More
Accurate Than TNM Staging in Predicting Survival in Patients With Pancreatic Cancer:
81. American Journal of Gastroenterology, 114(2019 ACG Annual Meeting Abstracts),
p.S48.
Eldén, L., 2019. Matrix methods in data mining and pattern recognition (Vol. 15). Siam.
Geiger, M. and Stockinger, K., 2019. Data warehousing and exploratory analysis for market
monitoring. In Applied Data Science (pp. 333-351). Springer, Cham.
Popovič, A., Puklavec, B. and Oliveira, T., 2019. Justifying business intelligence systems
adoption in SMEs. Industrial Management & Data Systems.
1 out of 7
Related Documents
![[object Object]](/_next/image/?url=%2F_next%2Fstatic%2Fmedia%2Flogo.6d15ce61.png&w=640&q=75)
Your All-in-One AI-Powered Toolkit for Academic Success.
+13062052269
info@desklib.com
Available 24*7 on WhatsApp / Email
Unlock your academic potential
© 2024 | Zucol Services PVT LTD | All rights reserved.