Analyzing Supermarket Sales and Profit Data with Excel and SPSS
VerifiedAdded on 2022/12/29
|17
|3178
|89
Report
AI Summary
This report presents an analysis of supermarket sales and profit data, utilizing Microsoft Excel for data preprocessing, analysis, and visualization. The report focuses on a four-year sales trend, employing pivot tables to interpret sales and profit figures across different modes of transport. It explores dat...
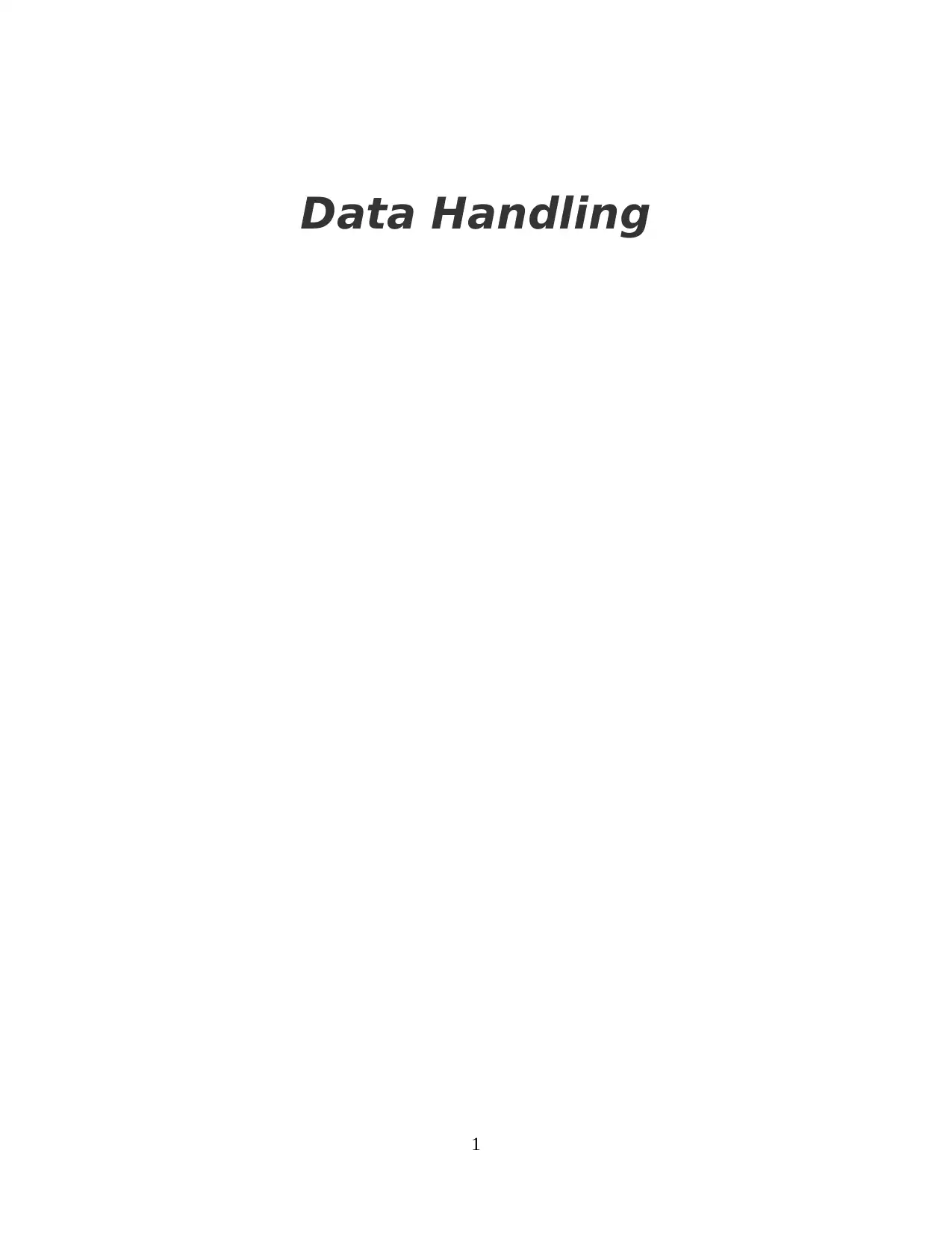
Data Handling
1
1
Paraphrase This Document
Need a fresh take? Get an instant paraphrase of this document with our AI Paraphraser
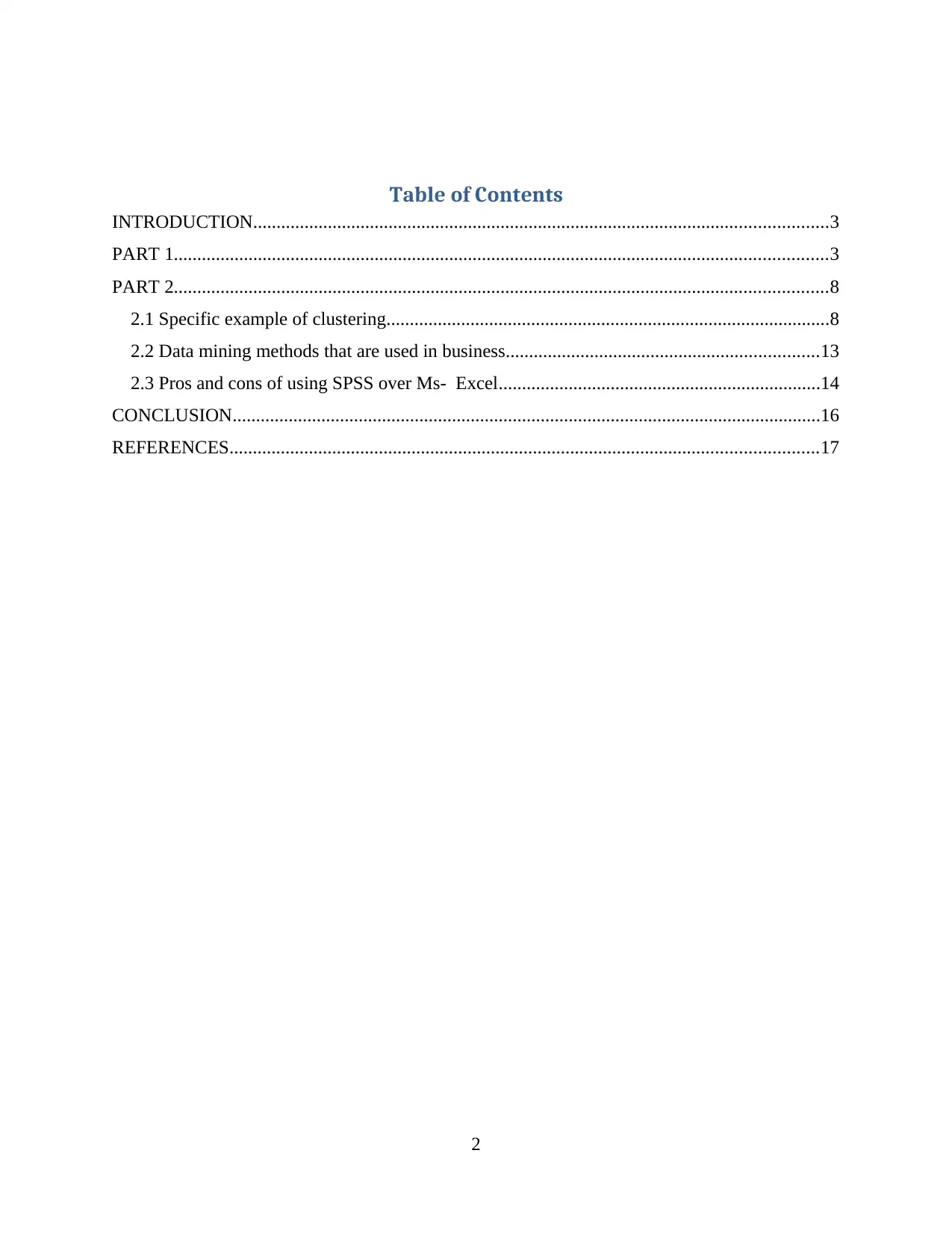
Table of Contents
INTRODUCTION...........................................................................................................................3
PART 1............................................................................................................................................3
PART 2............................................................................................................................................8
2.1 Specific example of clustering...............................................................................................8
2.2 Data mining methods that are used in business...................................................................13
2.3 Pros and cons of using SPSS over Ms- Excel.....................................................................14
CONCLUSION..............................................................................................................................16
REFERENCES..............................................................................................................................17
2
INTRODUCTION...........................................................................................................................3
PART 1............................................................................................................................................3
PART 2............................................................................................................................................8
2.1 Specific example of clustering...............................................................................................8
2.2 Data mining methods that are used in business...................................................................13
2.3 Pros and cons of using SPSS over Ms- Excel.....................................................................14
CONCLUSION..............................................................................................................................16
REFERENCES..............................................................................................................................17
2
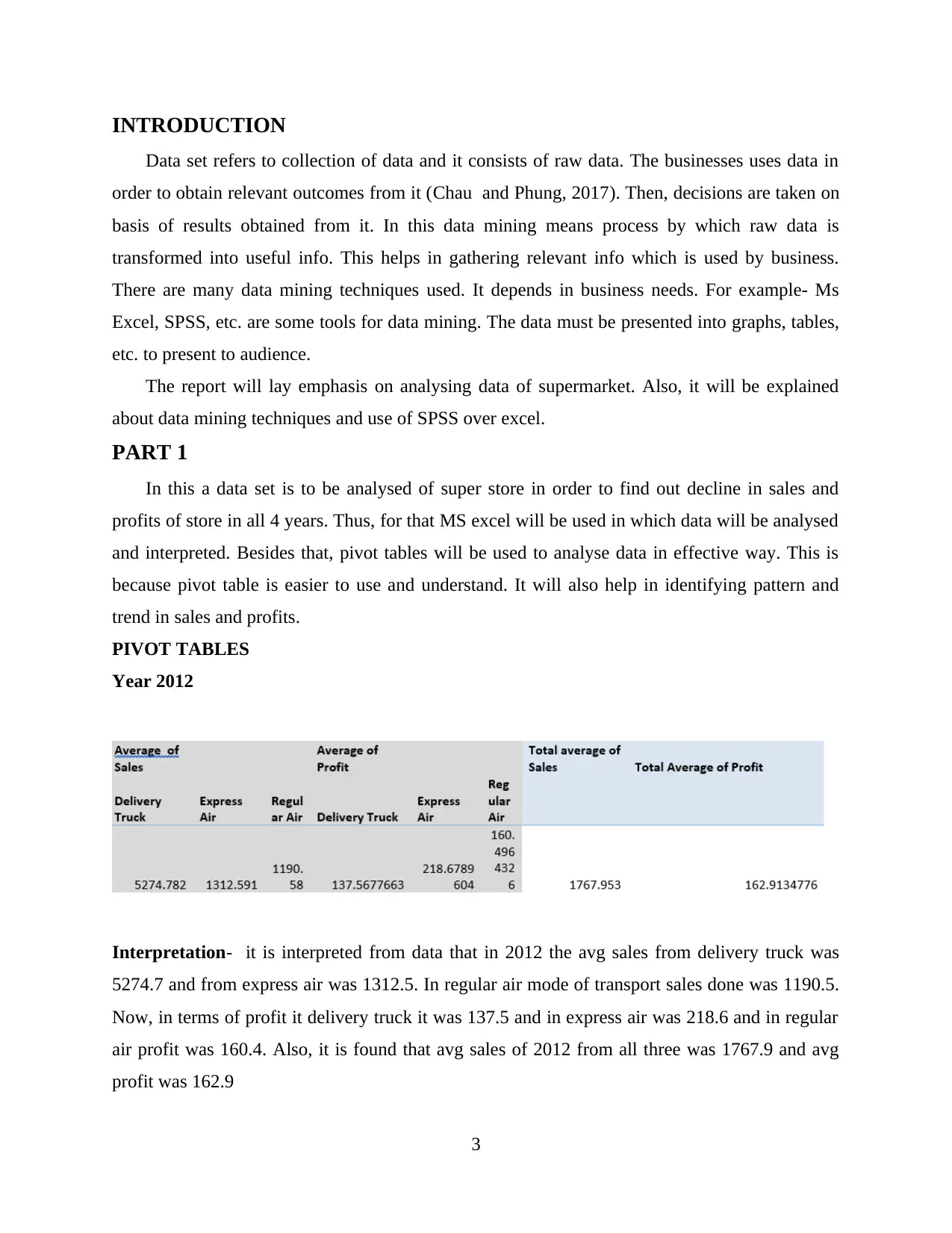
INTRODUCTION
Data set refers to collection of data and it consists of raw data. The businesses uses data in
order to obtain relevant outcomes from it (Chau and Phung, 2017). Then, decisions are taken on
basis of results obtained from it. In this data mining means process by which raw data is
transformed into useful info. This helps in gathering relevant info which is used by business.
There are many data mining techniques used. It depends in business needs. For example- Ms
Excel, SPSS, etc. are some tools for data mining. The data must be presented into graphs, tables,
etc. to present to audience.
The report will lay emphasis on analysing data of supermarket. Also, it will be explained
about data mining techniques and use of SPSS over excel.
PART 1
In this a data set is to be analysed of super store in order to find out decline in sales and
profits of store in all 4 years. Thus, for that MS excel will be used in which data will be analysed
and interpreted. Besides that, pivot tables will be used to analyse data in effective way. This is
because pivot table is easier to use and understand. It will also help in identifying pattern and
trend in sales and profits.
PIVOT TABLES
Year 2012
Interpretation- it is interpreted from data that in 2012 the avg sales from delivery truck was
5274.7 and from express air was 1312.5. In regular air mode of transport sales done was 1190.5.
Now, in terms of profit it delivery truck it was 137.5 and in express air was 218.6 and in regular
air profit was 160.4. Also, it is found that avg sales of 2012 from all three was 1767.9 and avg
profit was 162.9
3
Data set refers to collection of data and it consists of raw data. The businesses uses data in
order to obtain relevant outcomes from it (Chau and Phung, 2017). Then, decisions are taken on
basis of results obtained from it. In this data mining means process by which raw data is
transformed into useful info. This helps in gathering relevant info which is used by business.
There are many data mining techniques used. It depends in business needs. For example- Ms
Excel, SPSS, etc. are some tools for data mining. The data must be presented into graphs, tables,
etc. to present to audience.
The report will lay emphasis on analysing data of supermarket. Also, it will be explained
about data mining techniques and use of SPSS over excel.
PART 1
In this a data set is to be analysed of super store in order to find out decline in sales and
profits of store in all 4 years. Thus, for that MS excel will be used in which data will be analysed
and interpreted. Besides that, pivot tables will be used to analyse data in effective way. This is
because pivot table is easier to use and understand. It will also help in identifying pattern and
trend in sales and profits.
PIVOT TABLES
Year 2012
Interpretation- it is interpreted from data that in 2012 the avg sales from delivery truck was
5274.7 and from express air was 1312.5. In regular air mode of transport sales done was 1190.5.
Now, in terms of profit it delivery truck it was 137.5 and in express air was 218.6 and in regular
air profit was 160.4. Also, it is found that avg sales of 2012 from all three was 1767.9 and avg
profit was 162.9
3
⊘ This is a preview!⊘
Do you want full access?
Subscribe today to unlock all pages.

Trusted by 1+ million students worldwide
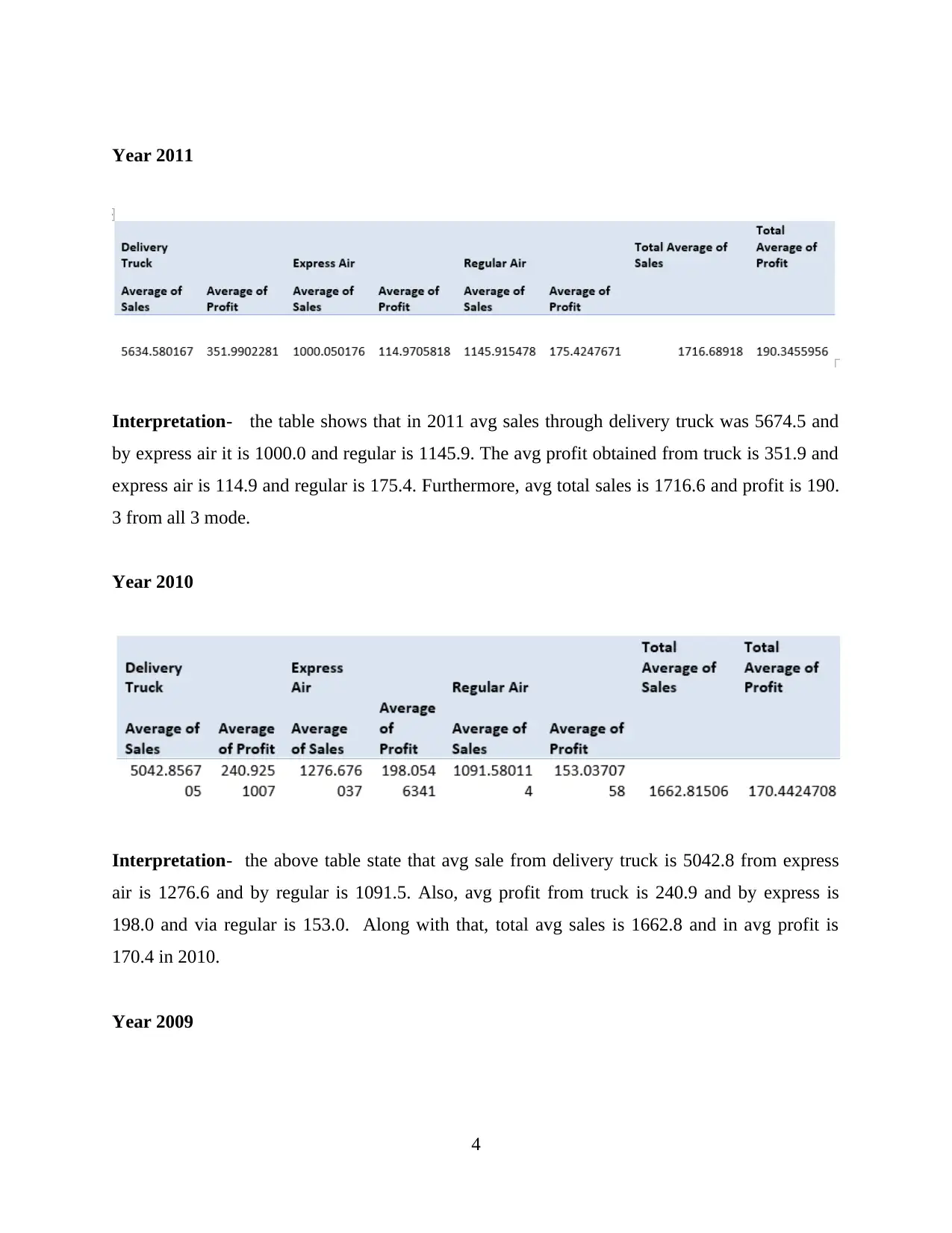
Year 2011
Interpretation- the table shows that in 2011 avg sales through delivery truck was 5674.5 and
by express air it is 1000.0 and regular is 1145.9. The avg profit obtained from truck is 351.9 and
express air is 114.9 and regular is 175.4. Furthermore, avg total sales is 1716.6 and profit is 190.
3 from all 3 mode.
Year 2010
Interpretation- the above table state that avg sale from delivery truck is 5042.8 from express
air is 1276.6 and by regular is 1091.5. Also, avg profit from truck is 240.9 and by express is
198.0 and via regular is 153.0. Along with that, total avg sales is 1662.8 and in avg profit is
170.4 in 2010.
Year 2009
4
Interpretation- the table shows that in 2011 avg sales through delivery truck was 5674.5 and
by express air it is 1000.0 and regular is 1145.9. The avg profit obtained from truck is 351.9 and
express air is 114.9 and regular is 175.4. Furthermore, avg total sales is 1716.6 and profit is 190.
3 from all 3 mode.
Year 2010
Interpretation- the above table state that avg sale from delivery truck is 5042.8 from express
air is 1276.6 and by regular is 1091.5. Also, avg profit from truck is 240.9 and by express is
198.0 and via regular is 153.0. Along with that, total avg sales is 1662.8 and in avg profit is
170.4 in 2010.
Year 2009
4
Paraphrase This Document
Need a fresh take? Get an instant paraphrase of this document with our AI Paraphraser
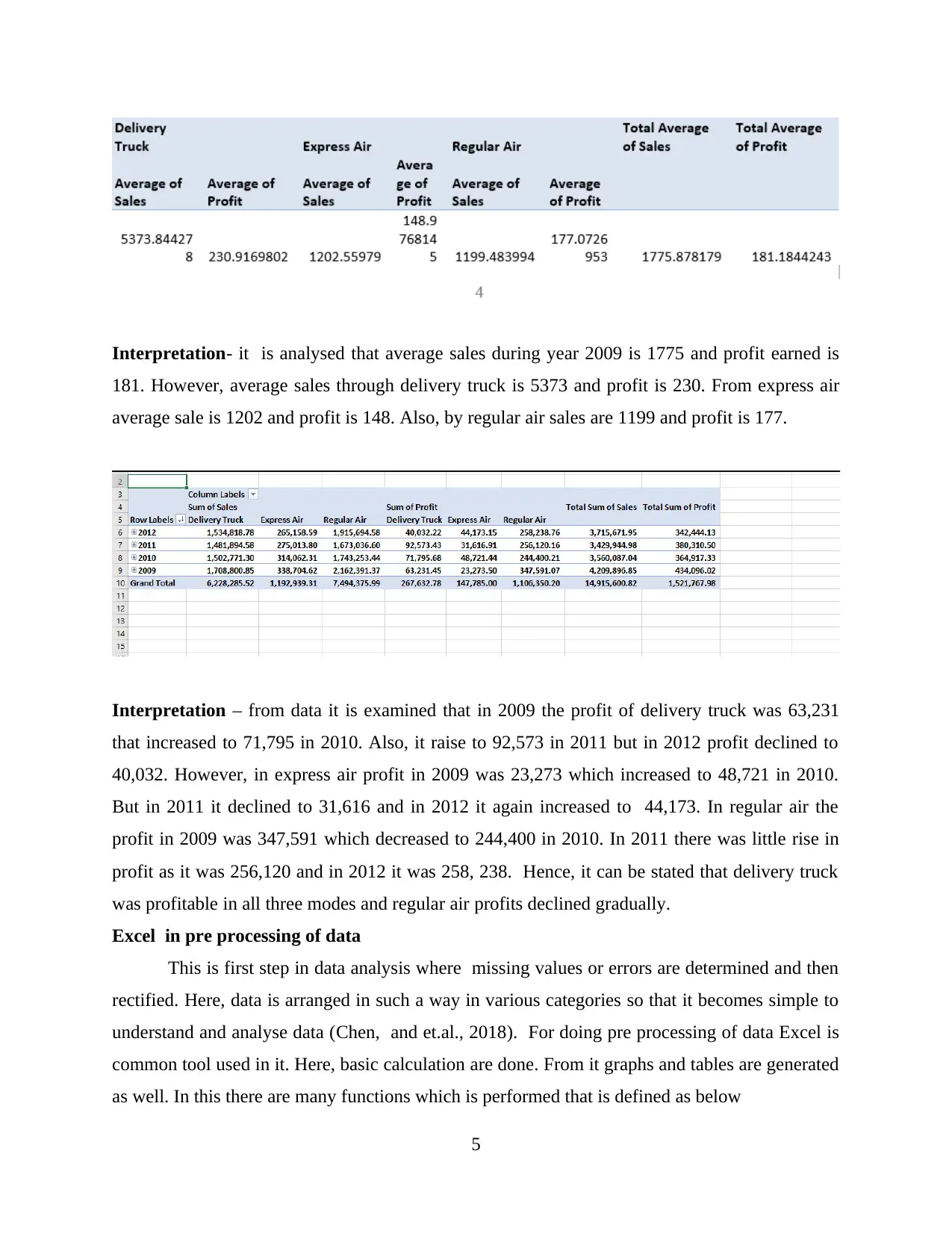
Interpretation- it is analysed that average sales during year 2009 is 1775 and profit earned is
181. However, average sales through delivery truck is 5373 and profit is 230. From express air
average sale is 1202 and profit is 148. Also, by regular air sales are 1199 and profit is 177.
Interpretation – from data it is examined that in 2009 the profit of delivery truck was 63,231
that increased to 71,795 in 2010. Also, it raise to 92,573 in 2011 but in 2012 profit declined to
40,032. However, in express air profit in 2009 was 23,273 which increased to 48,721 in 2010.
But in 2011 it declined to 31,616 and in 2012 it again increased to 44,173. In regular air the
profit in 2009 was 347,591 which decreased to 244,400 in 2010. In 2011 there was little rise in
profit as it was 256,120 and in 2012 it was 258, 238. Hence, it can be stated that delivery truck
was profitable in all three modes and regular air profits declined gradually.
Excel in pre processing of data
This is first step in data analysis where missing values or errors are determined and then
rectified. Here, data is arranged in such a way in various categories so that it becomes simple to
understand and analyse data (Chen, and et.al., 2018). For doing pre processing of data Excel is
common tool used in it. Here, basic calculation are done. From it graphs and tables are generated
as well. In this there are many functions which is performed that is defined as below
5
181. However, average sales through delivery truck is 5373 and profit is 230. From express air
average sale is 1202 and profit is 148. Also, by regular air sales are 1199 and profit is 177.
Interpretation – from data it is examined that in 2009 the profit of delivery truck was 63,231
that increased to 71,795 in 2010. Also, it raise to 92,573 in 2011 but in 2012 profit declined to
40,032. However, in express air profit in 2009 was 23,273 which increased to 48,721 in 2010.
But in 2011 it declined to 31,616 and in 2012 it again increased to 44,173. In regular air the
profit in 2009 was 347,591 which decreased to 244,400 in 2010. In 2011 there was little rise in
profit as it was 256,120 and in 2012 it was 258, 238. Hence, it can be stated that delivery truck
was profitable in all three modes and regular air profits declined gradually.
Excel in pre processing of data
This is first step in data analysis where missing values or errors are determined and then
rectified. Here, data is arranged in such a way in various categories so that it becomes simple to
understand and analyse data (Chen, and et.al., 2018). For doing pre processing of data Excel is
common tool used in it. Here, basic calculation are done. From it graphs and tables are generated
as well. In this there are many functions which is performed that is defined as below
5
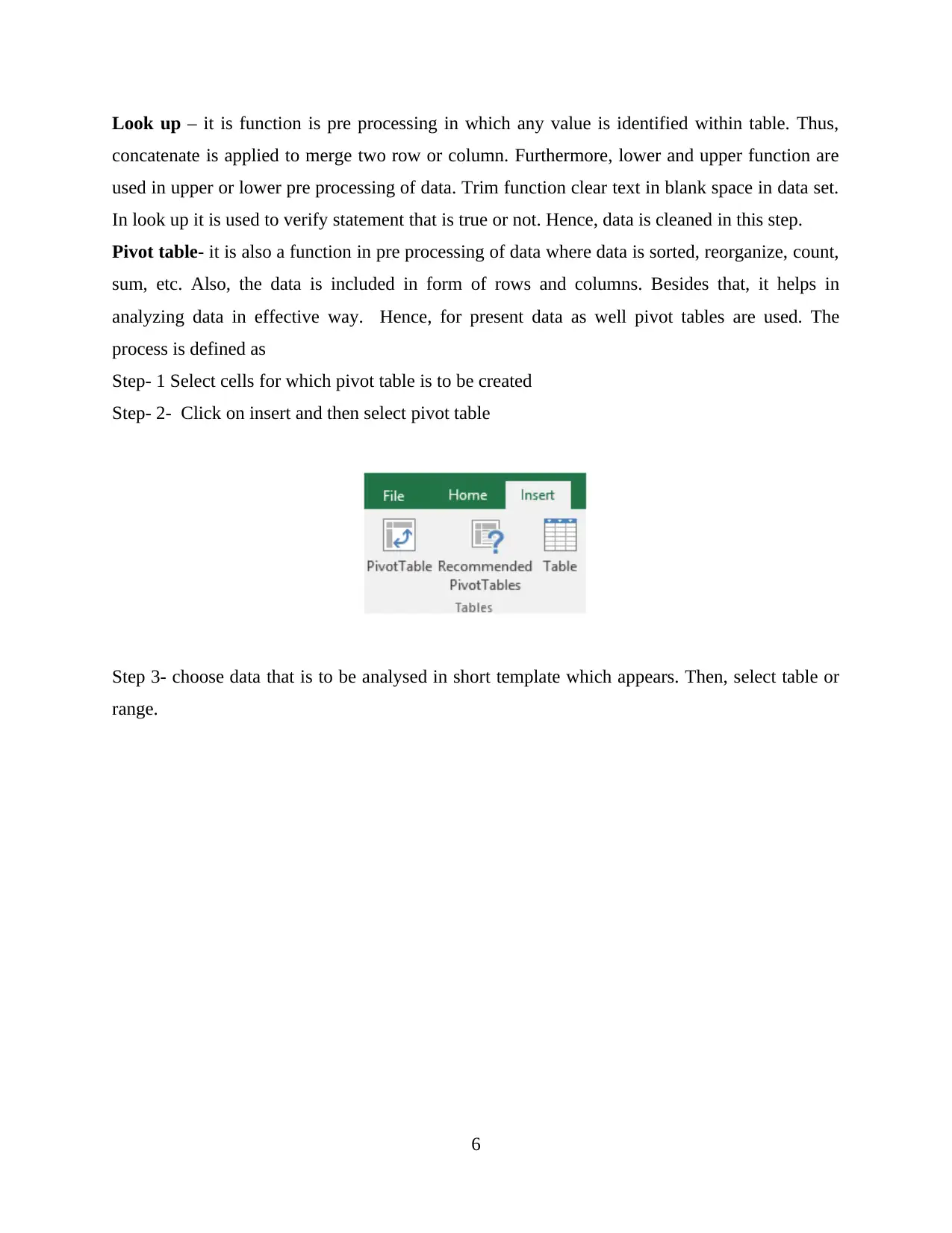
Look up – it is function is pre processing in which any value is identified within table. Thus,
concatenate is applied to merge two row or column. Furthermore, lower and upper function are
used in upper or lower pre processing of data. Trim function clear text in blank space in data set.
In look up it is used to verify statement that is true or not. Hence, data is cleaned in this step.
Pivot table- it is also a function in pre processing of data where data is sorted, reorganize, count,
sum, etc. Also, the data is included in form of rows and columns. Besides that, it helps in
analyzing data in effective way. Hence, for present data as well pivot tables are used. The
process is defined as
Step- 1 Select cells for which pivot table is to be created
Step- 2- Click on insert and then select pivot table
Step 3- choose data that is to be analysed in short template which appears. Then, select table or
range.
6
concatenate is applied to merge two row or column. Furthermore, lower and upper function are
used in upper or lower pre processing of data. Trim function clear text in blank space in data set.
In look up it is used to verify statement that is true or not. Hence, data is cleaned in this step.
Pivot table- it is also a function in pre processing of data where data is sorted, reorganize, count,
sum, etc. Also, the data is included in form of rows and columns. Besides that, it helps in
analyzing data in effective way. Hence, for present data as well pivot tables are used. The
process is defined as
Step- 1 Select cells for which pivot table is to be created
Step- 2- Click on insert and then select pivot table
Step 3- choose data that is to be analysed in short template which appears. Then, select table or
range.
6
⊘ This is a preview!⊘
Do you want full access?
Subscribe today to unlock all pages.

Trusted by 1+ million students worldwide
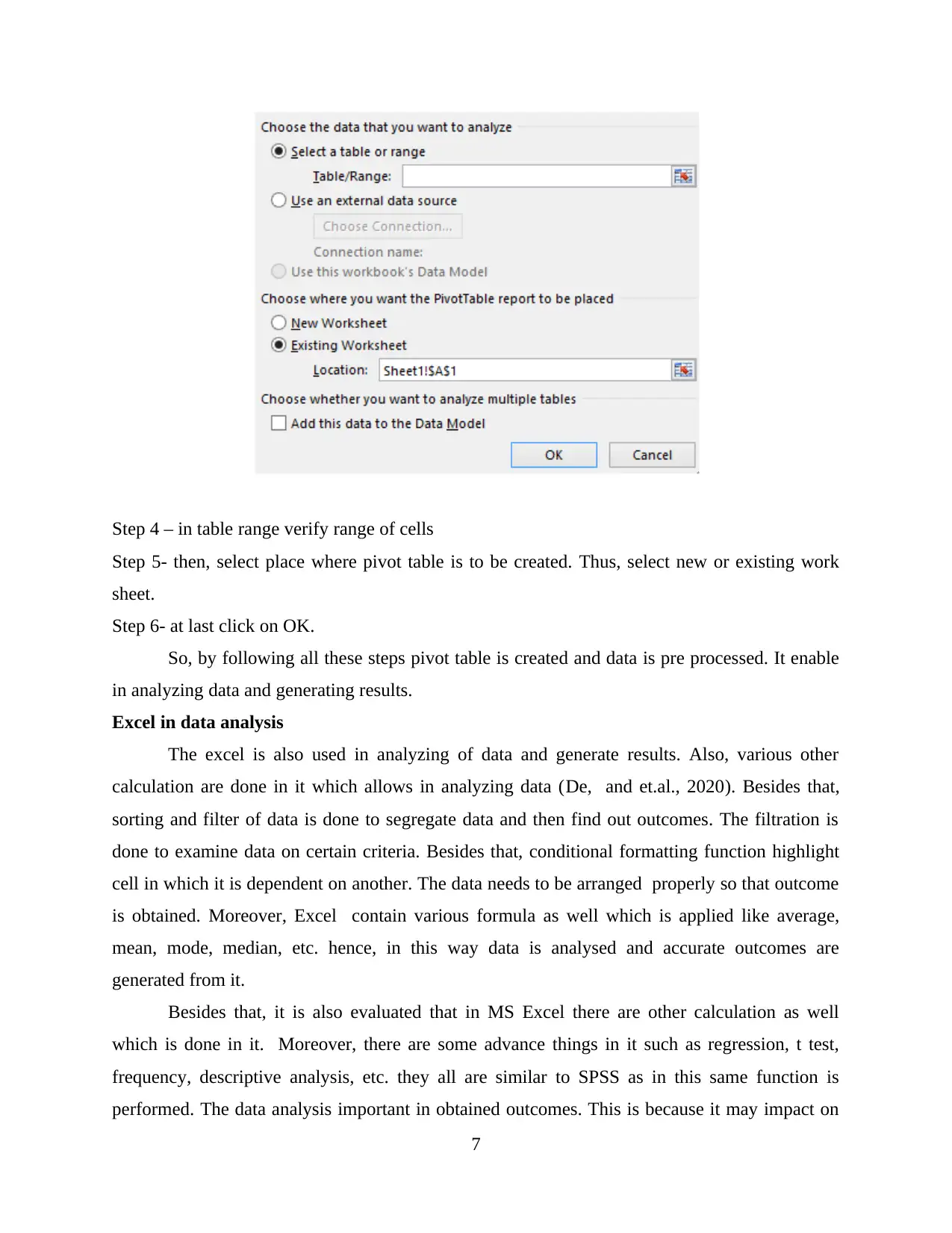
Step 4 – in table range verify range of cells
Step 5- then, select place where pivot table is to be created. Thus, select new or existing work
sheet.
Step 6- at last click on OK.
So, by following all these steps pivot table is created and data is pre processed. It enable
in analyzing data and generating results.
Excel in data analysis
The excel is also used in analyzing of data and generate results. Also, various other
calculation are done in it which allows in analyzing data (De, and et.al., 2020). Besides that,
sorting and filter of data is done to segregate data and then find out outcomes. The filtration is
done to examine data on certain criteria. Besides that, conditional formatting function highlight
cell in which it is dependent on another. The data needs to be arranged properly so that outcome
is obtained. Moreover, Excel contain various formula as well which is applied like average,
mean, mode, median, etc. hence, in this way data is analysed and accurate outcomes are
generated from it.
Besides that, it is also evaluated that in MS Excel there are other calculation as well
which is done in it. Moreover, there are some advance things in it such as regression, t test,
frequency, descriptive analysis, etc. they all are similar to SPSS as in this same function is
performed. The data analysis important in obtained outcomes. This is because it may impact on
7
Step 5- then, select place where pivot table is to be created. Thus, select new or existing work
sheet.
Step 6- at last click on OK.
So, by following all these steps pivot table is created and data is pre processed. It enable
in analyzing data and generating results.
Excel in data analysis
The excel is also used in analyzing of data and generate results. Also, various other
calculation are done in it which allows in analyzing data (De, and et.al., 2020). Besides that,
sorting and filter of data is done to segregate data and then find out outcomes. The filtration is
done to examine data on certain criteria. Besides that, conditional formatting function highlight
cell in which it is dependent on another. The data needs to be arranged properly so that outcome
is obtained. Moreover, Excel contain various formula as well which is applied like average,
mean, mode, median, etc. hence, in this way data is analysed and accurate outcomes are
generated from it.
Besides that, it is also evaluated that in MS Excel there are other calculation as well
which is done in it. Moreover, there are some advance things in it such as regression, t test,
frequency, descriptive analysis, etc. they all are similar to SPSS as in this same function is
performed. The data analysis important in obtained outcomes. This is because it may impact on
7
Paraphrase This Document
Need a fresh take? Get an instant paraphrase of this document with our AI Paraphraser
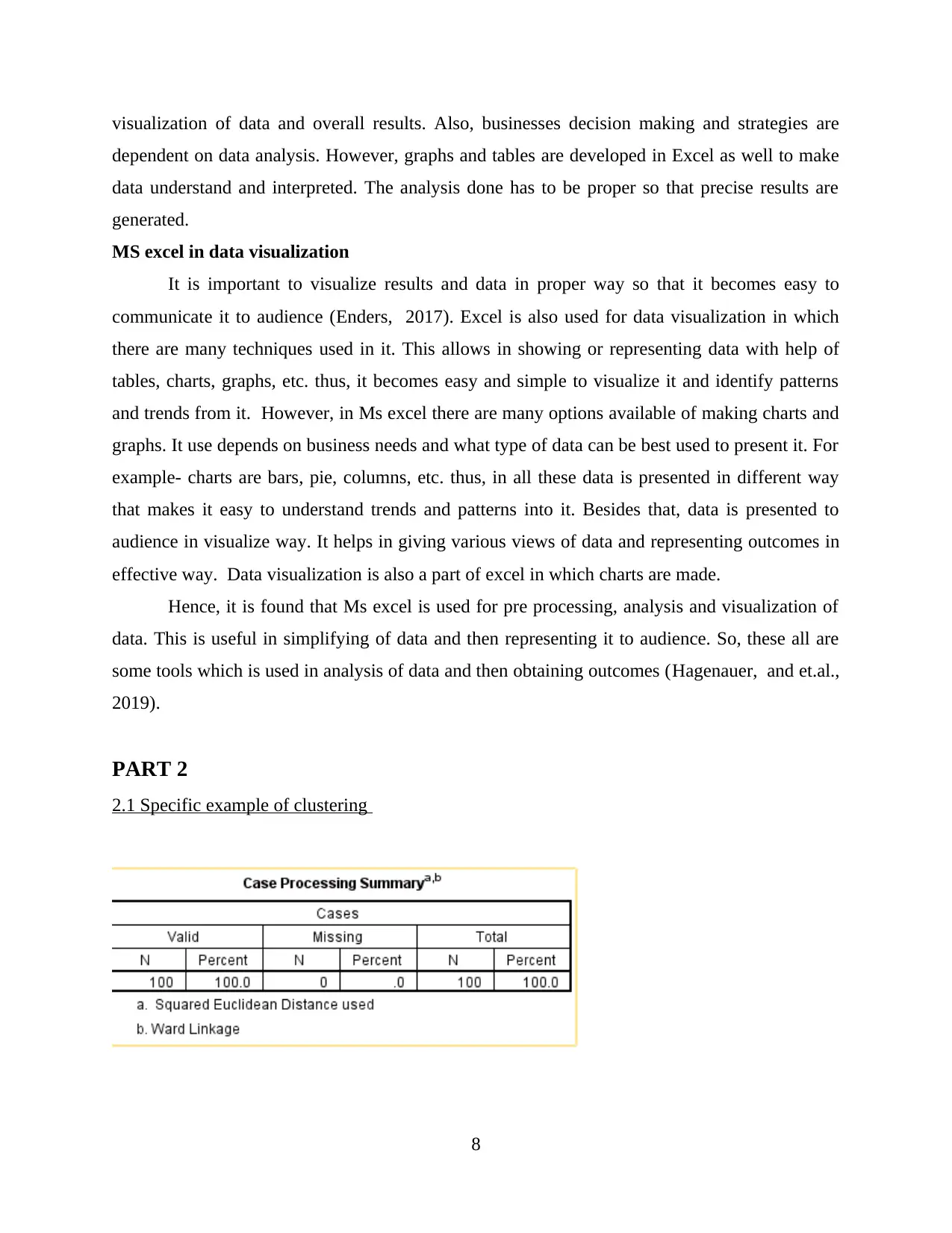
visualization of data and overall results. Also, businesses decision making and strategies are
dependent on data analysis. However, graphs and tables are developed in Excel as well to make
data understand and interpreted. The analysis done has to be proper so that precise results are
generated.
MS excel in data visualization
It is important to visualize results and data in proper way so that it becomes easy to
communicate it to audience (Enders, 2017). Excel is also used for data visualization in which
there are many techniques used in it. This allows in showing or representing data with help of
tables, charts, graphs, etc. thus, it becomes easy and simple to visualize it and identify patterns
and trends from it. However, in Ms excel there are many options available of making charts and
graphs. It use depends on business needs and what type of data can be best used to present it. For
example- charts are bars, pie, columns, etc. thus, in all these data is presented in different way
that makes it easy to understand trends and patterns into it. Besides that, data is presented to
audience in visualize way. It helps in giving various views of data and representing outcomes in
effective way. Data visualization is also a part of excel in which charts are made.
Hence, it is found that Ms excel is used for pre processing, analysis and visualization of
data. This is useful in simplifying of data and then representing it to audience. So, these all are
some tools which is used in analysis of data and then obtaining outcomes (Hagenauer, and et.al.,
2019).
PART 2
2.1 Specific example of clustering
8
dependent on data analysis. However, graphs and tables are developed in Excel as well to make
data understand and interpreted. The analysis done has to be proper so that precise results are
generated.
MS excel in data visualization
It is important to visualize results and data in proper way so that it becomes easy to
communicate it to audience (Enders, 2017). Excel is also used for data visualization in which
there are many techniques used in it. This allows in showing or representing data with help of
tables, charts, graphs, etc. thus, it becomes easy and simple to visualize it and identify patterns
and trends from it. However, in Ms excel there are many options available of making charts and
graphs. It use depends on business needs and what type of data can be best used to present it. For
example- charts are bars, pie, columns, etc. thus, in all these data is presented in different way
that makes it easy to understand trends and patterns into it. Besides that, data is presented to
audience in visualize way. It helps in giving various views of data and representing outcomes in
effective way. Data visualization is also a part of excel in which charts are made.
Hence, it is found that Ms excel is used for pre processing, analysis and visualization of
data. This is useful in simplifying of data and then representing it to audience. So, these all are
some tools which is used in analysis of data and then obtaining outcomes (Hagenauer, and et.al.,
2019).
PART 2
2.1 Specific example of clustering
8
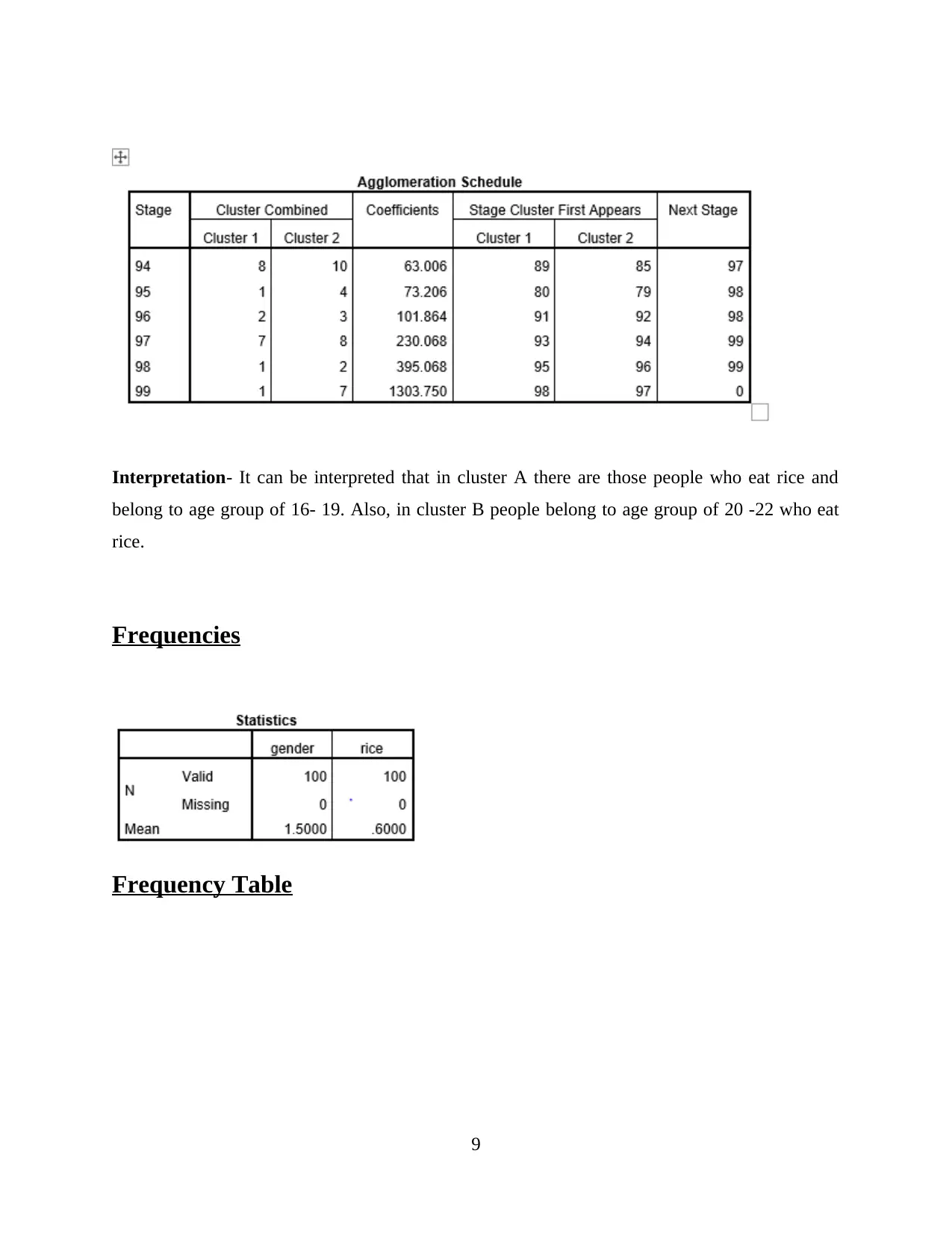
Interpretation- It can be interpreted that in cluster A there are those people who eat rice and
belong to age group of 16- 19. Also, in cluster B people belong to age group of 20 -22 who eat
rice.
Frequencies
Frequency Table
9
belong to age group of 16- 19. Also, in cluster B people belong to age group of 20 -22 who eat
rice.
Frequencies
Frequency Table
9
⊘ This is a preview!⊘
Do you want full access?
Subscribe today to unlock all pages.

Trusted by 1+ million students worldwide
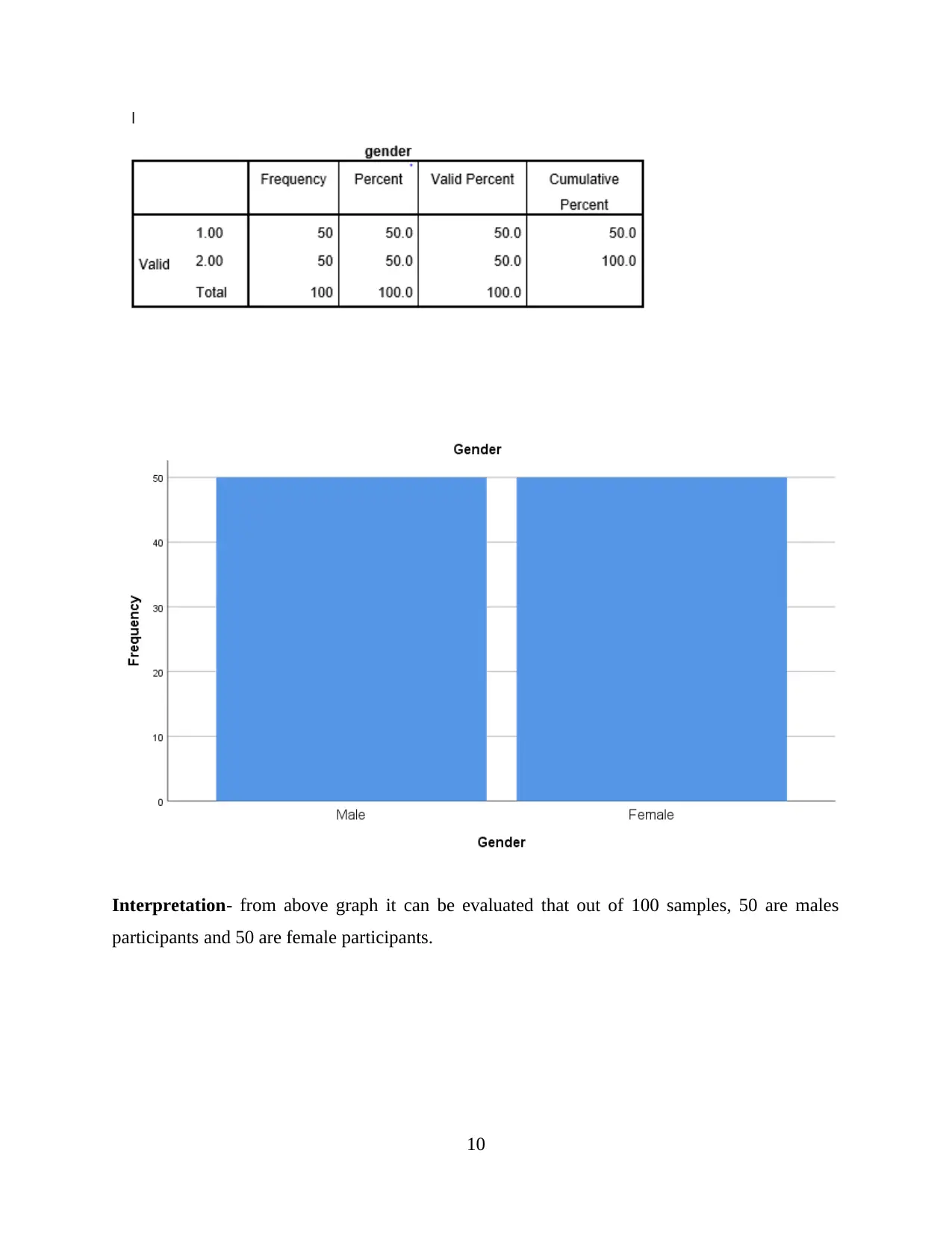
Interpretation- from above graph it can be evaluated that out of 100 samples, 50 are males
participants and 50 are female participants.
10
participants and 50 are female participants.
10
Paraphrase This Document
Need a fresh take? Get an instant paraphrase of this document with our AI Paraphraser
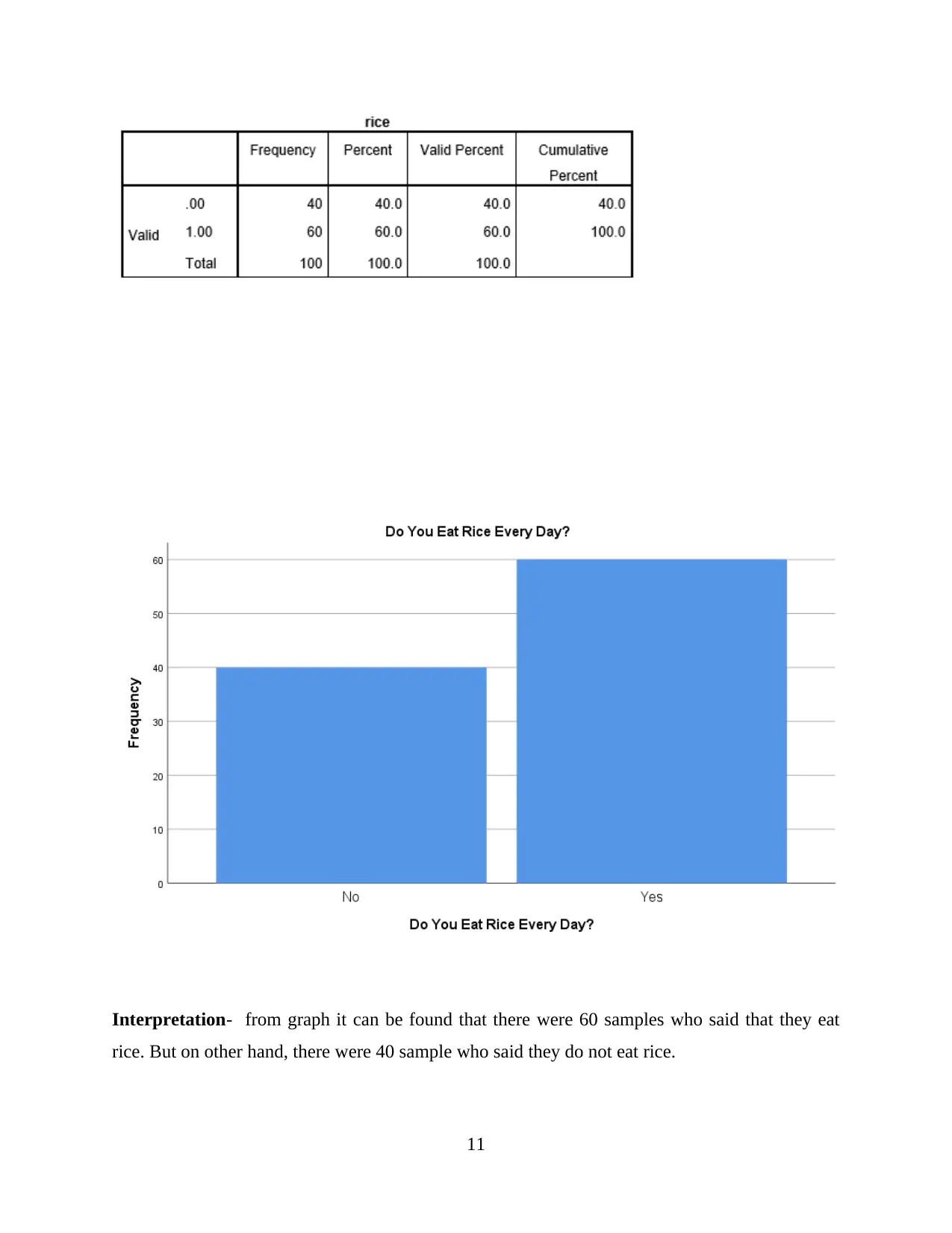
Interpretation- from graph it can be found that there were 60 samples who said that they eat
rice. But on other hand, there were 40 sample who said they do not eat rice.
11
rice. But on other hand, there were 40 sample who said they do not eat rice.
11
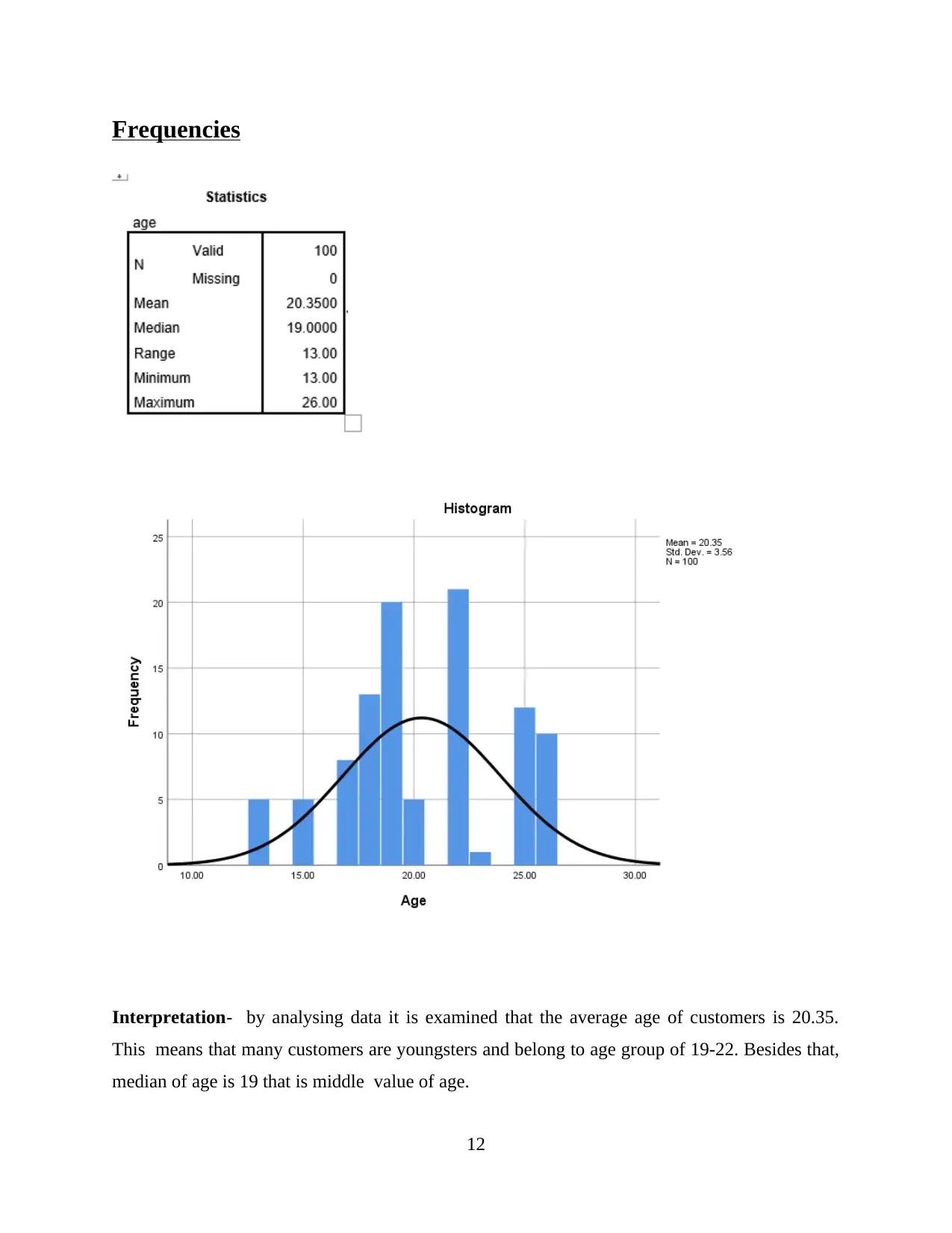
Frequencies
Interpretation- by analysing data it is examined that the average age of customers is 20.35.
This means that many customers are youngsters and belong to age group of 19-22. Besides that,
median of age is 19 that is middle value of age.
12
Interpretation- by analysing data it is examined that the average age of customers is 20.35.
This means that many customers are youngsters and belong to age group of 19-22. Besides that,
median of age is 19 that is middle value of age.
12
⊘ This is a preview!⊘
Do you want full access?
Subscribe today to unlock all pages.

Trusted by 1+ million students worldwide
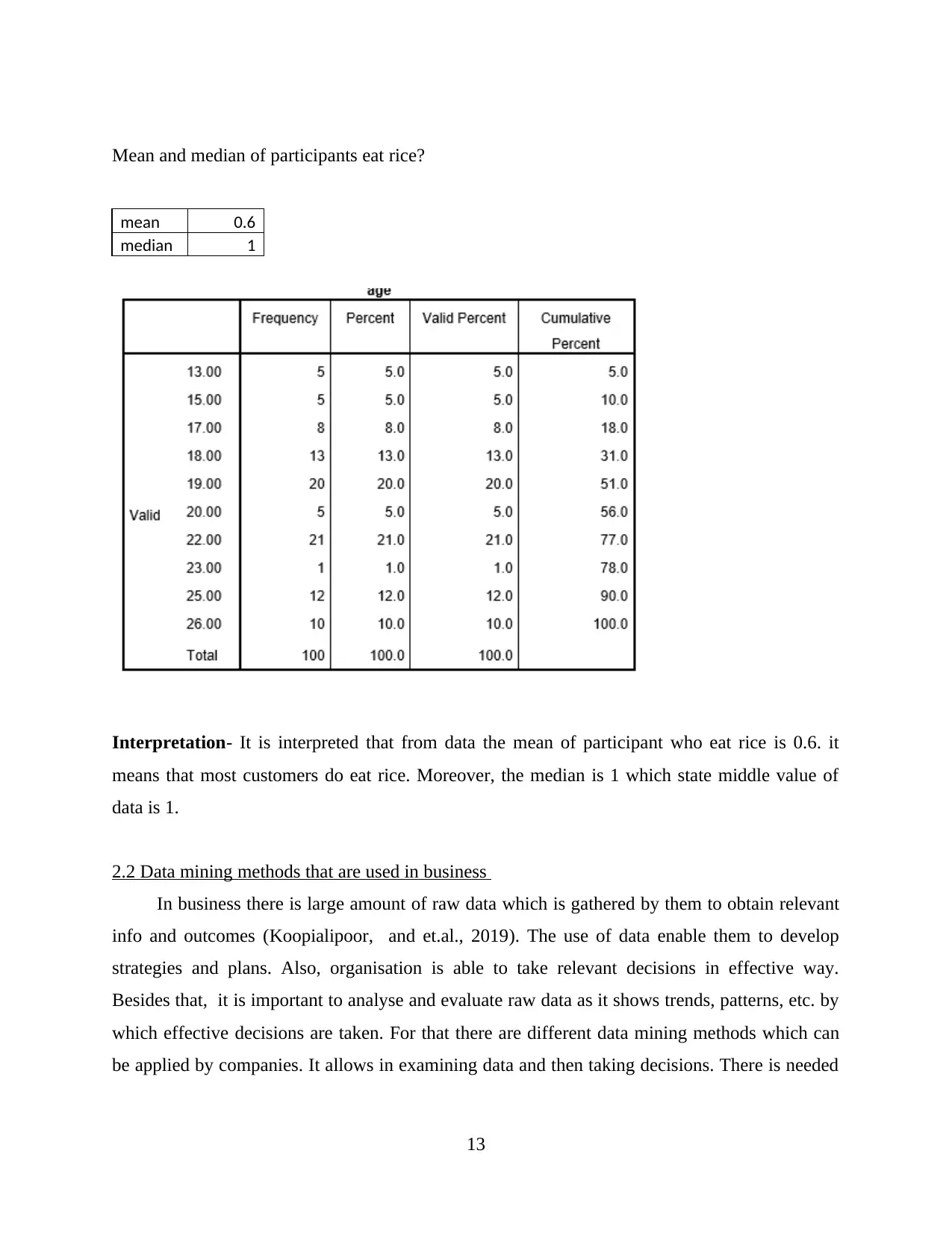
Mean and median of participants eat rice?
mean 0.6
median 1
Interpretation- It is interpreted that from data the mean of participant who eat rice is 0.6. it
means that most customers do eat rice. Moreover, the median is 1 which state middle value of
data is 1.
2.2 Data mining methods that are used in business
In business there is large amount of raw data which is gathered by them to obtain relevant
info and outcomes (Koopialipoor, and et.al., 2019). The use of data enable them to develop
strategies and plans. Also, organisation is able to take relevant decisions in effective way.
Besides that, it is important to analyse and evaluate raw data as it shows trends, patterns, etc. by
which effective decisions are taken. For that there are different data mining methods which can
be applied by companies. It allows in examining data and then taking decisions. There is needed
13
mean 0.6
median 1
Interpretation- It is interpreted that from data the mean of participant who eat rice is 0.6. it
means that most customers do eat rice. Moreover, the median is 1 which state middle value of
data is 1.
2.2 Data mining methods that are used in business
In business there is large amount of raw data which is gathered by them to obtain relevant
info and outcomes (Koopialipoor, and et.al., 2019). The use of data enable them to develop
strategies and plans. Also, organisation is able to take relevant decisions in effective way.
Besides that, it is important to analyse and evaluate raw data as it shows trends, patterns, etc. by
which effective decisions are taken. For that there are different data mining methods which can
be applied by companies. It allows in examining data and then taking decisions. There is needed
13
Paraphrase This Document
Need a fresh take? Get an instant paraphrase of this document with our AI Paraphraser
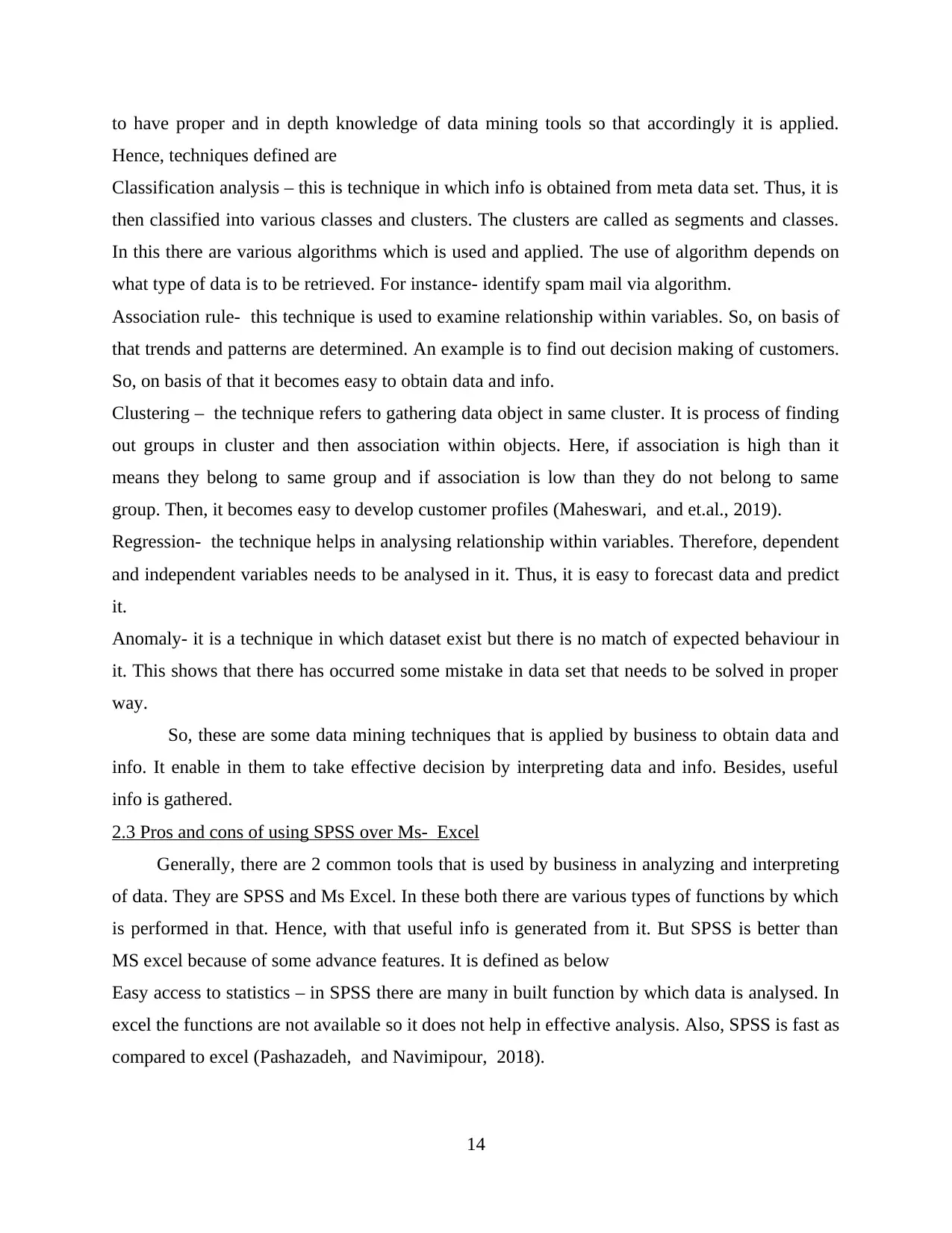
to have proper and in depth knowledge of data mining tools so that accordingly it is applied.
Hence, techniques defined are
Classification analysis – this is technique in which info is obtained from meta data set. Thus, it is
then classified into various classes and clusters. The clusters are called as segments and classes.
In this there are various algorithms which is used and applied. The use of algorithm depends on
what type of data is to be retrieved. For instance- identify spam mail via algorithm.
Association rule- this technique is used to examine relationship within variables. So, on basis of
that trends and patterns are determined. An example is to find out decision making of customers.
So, on basis of that it becomes easy to obtain data and info.
Clustering – the technique refers to gathering data object in same cluster. It is process of finding
out groups in cluster and then association within objects. Here, if association is high than it
means they belong to same group and if association is low than they do not belong to same
group. Then, it becomes easy to develop customer profiles (Maheswari, and et.al., 2019).
Regression- the technique helps in analysing relationship within variables. Therefore, dependent
and independent variables needs to be analysed in it. Thus, it is easy to forecast data and predict
it.
Anomaly- it is a technique in which dataset exist but there is no match of expected behaviour in
it. This shows that there has occurred some mistake in data set that needs to be solved in proper
way.
So, these are some data mining techniques that is applied by business to obtain data and
info. It enable in them to take effective decision by interpreting data and info. Besides, useful
info is gathered.
2.3 Pros and cons of using SPSS over Ms- Excel
Generally, there are 2 common tools that is used by business in analyzing and interpreting
of data. They are SPSS and Ms Excel. In these both there are various types of functions by which
is performed in that. Hence, with that useful info is generated from it. But SPSS is better than
MS excel because of some advance features. It is defined as below
Easy access to statistics – in SPSS there are many in built function by which data is analysed. In
excel the functions are not available so it does not help in effective analysis. Also, SPSS is fast as
compared to excel (Pashazadeh, and Navimipour, 2018).
14
Hence, techniques defined are
Classification analysis – this is technique in which info is obtained from meta data set. Thus, it is
then classified into various classes and clusters. The clusters are called as segments and classes.
In this there are various algorithms which is used and applied. The use of algorithm depends on
what type of data is to be retrieved. For instance- identify spam mail via algorithm.
Association rule- this technique is used to examine relationship within variables. So, on basis of
that trends and patterns are determined. An example is to find out decision making of customers.
So, on basis of that it becomes easy to obtain data and info.
Clustering – the technique refers to gathering data object in same cluster. It is process of finding
out groups in cluster and then association within objects. Here, if association is high than it
means they belong to same group and if association is low than they do not belong to same
group. Then, it becomes easy to develop customer profiles (Maheswari, and et.al., 2019).
Regression- the technique helps in analysing relationship within variables. Therefore, dependent
and independent variables needs to be analysed in it. Thus, it is easy to forecast data and predict
it.
Anomaly- it is a technique in which dataset exist but there is no match of expected behaviour in
it. This shows that there has occurred some mistake in data set that needs to be solved in proper
way.
So, these are some data mining techniques that is applied by business to obtain data and
info. It enable in them to take effective decision by interpreting data and info. Besides, useful
info is gathered.
2.3 Pros and cons of using SPSS over Ms- Excel
Generally, there are 2 common tools that is used by business in analyzing and interpreting
of data. They are SPSS and Ms Excel. In these both there are various types of functions by which
is performed in that. Hence, with that useful info is generated from it. But SPSS is better than
MS excel because of some advance features. It is defined as below
Easy access to statistics – in SPSS there are many in built function by which data is analysed. In
excel the functions are not available so it does not help in effective analysis. Also, SPSS is fast as
compared to excel (Pashazadeh, and Navimipour, 2018).
14
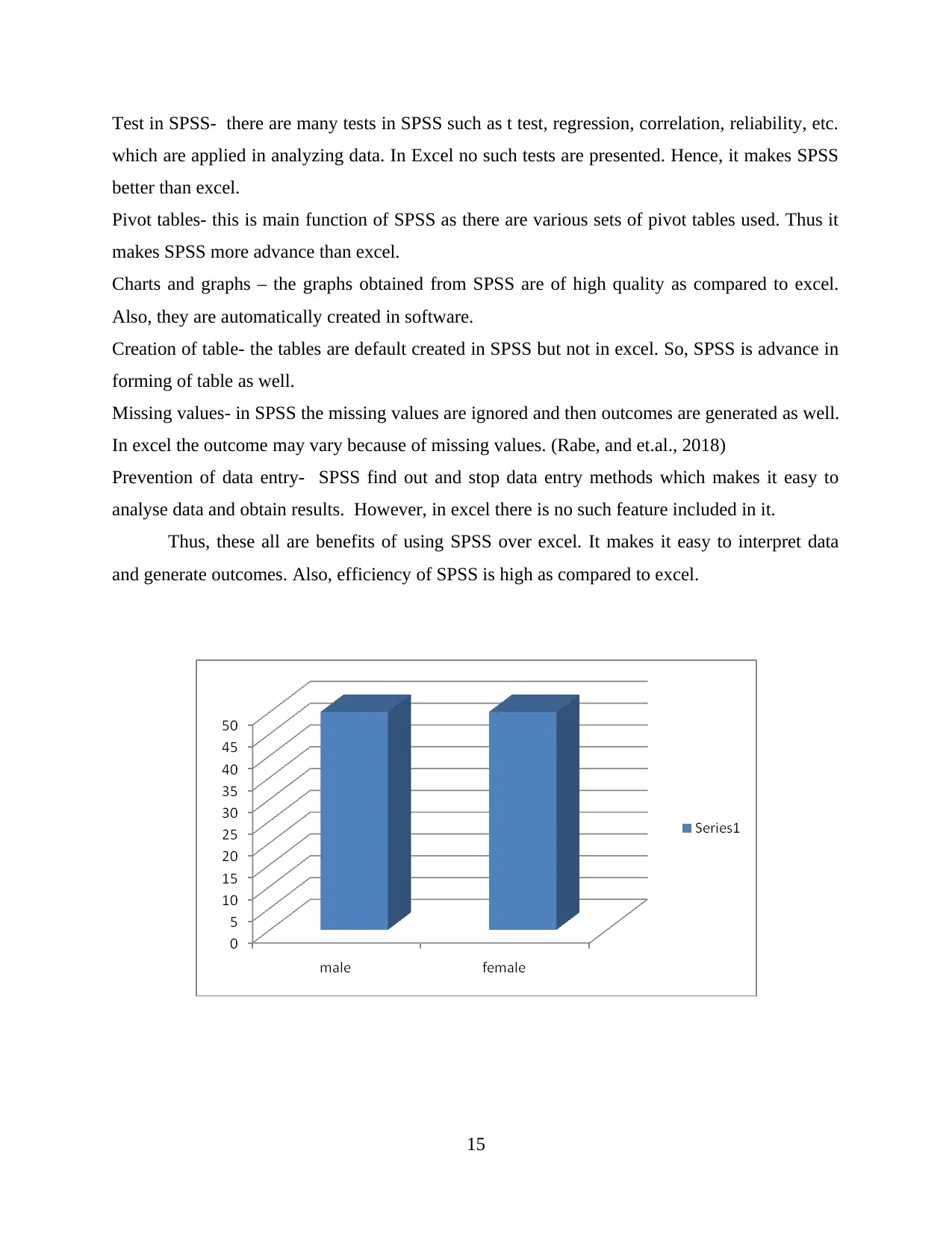
Test in SPSS- there are many tests in SPSS such as t test, regression, correlation, reliability, etc.
which are applied in analyzing data. In Excel no such tests are presented. Hence, it makes SPSS
better than excel.
Pivot tables- this is main function of SPSS as there are various sets of pivot tables used. Thus it
makes SPSS more advance than excel.
Charts and graphs – the graphs obtained from SPSS are of high quality as compared to excel.
Also, they are automatically created in software.
Creation of table- the tables are default created in SPSS but not in excel. So, SPSS is advance in
forming of table as well.
Missing values- in SPSS the missing values are ignored and then outcomes are generated as well.
In excel the outcome may vary because of missing values. (Rabe, and et.al., 2018)
Prevention of data entry- SPSS find out and stop data entry methods which makes it easy to
analyse data and obtain results. However, in excel there is no such feature included in it.
Thus, these all are benefits of using SPSS over excel. It makes it easy to interpret data
and generate outcomes. Also, efficiency of SPSS is high as compared to excel.
15
which are applied in analyzing data. In Excel no such tests are presented. Hence, it makes SPSS
better than excel.
Pivot tables- this is main function of SPSS as there are various sets of pivot tables used. Thus it
makes SPSS more advance than excel.
Charts and graphs – the graphs obtained from SPSS are of high quality as compared to excel.
Also, they are automatically created in software.
Creation of table- the tables are default created in SPSS but not in excel. So, SPSS is advance in
forming of table as well.
Missing values- in SPSS the missing values are ignored and then outcomes are generated as well.
In excel the outcome may vary because of missing values. (Rabe, and et.al., 2018)
Prevention of data entry- SPSS find out and stop data entry methods which makes it easy to
analyse data and obtain results. However, in excel there is no such feature included in it.
Thus, these all are benefits of using SPSS over excel. It makes it easy to interpret data
and generate outcomes. Also, efficiency of SPSS is high as compared to excel.
15
⊘ This is a preview!⊘
Do you want full access?
Subscribe today to unlock all pages.

Trusted by 1+ million students worldwide
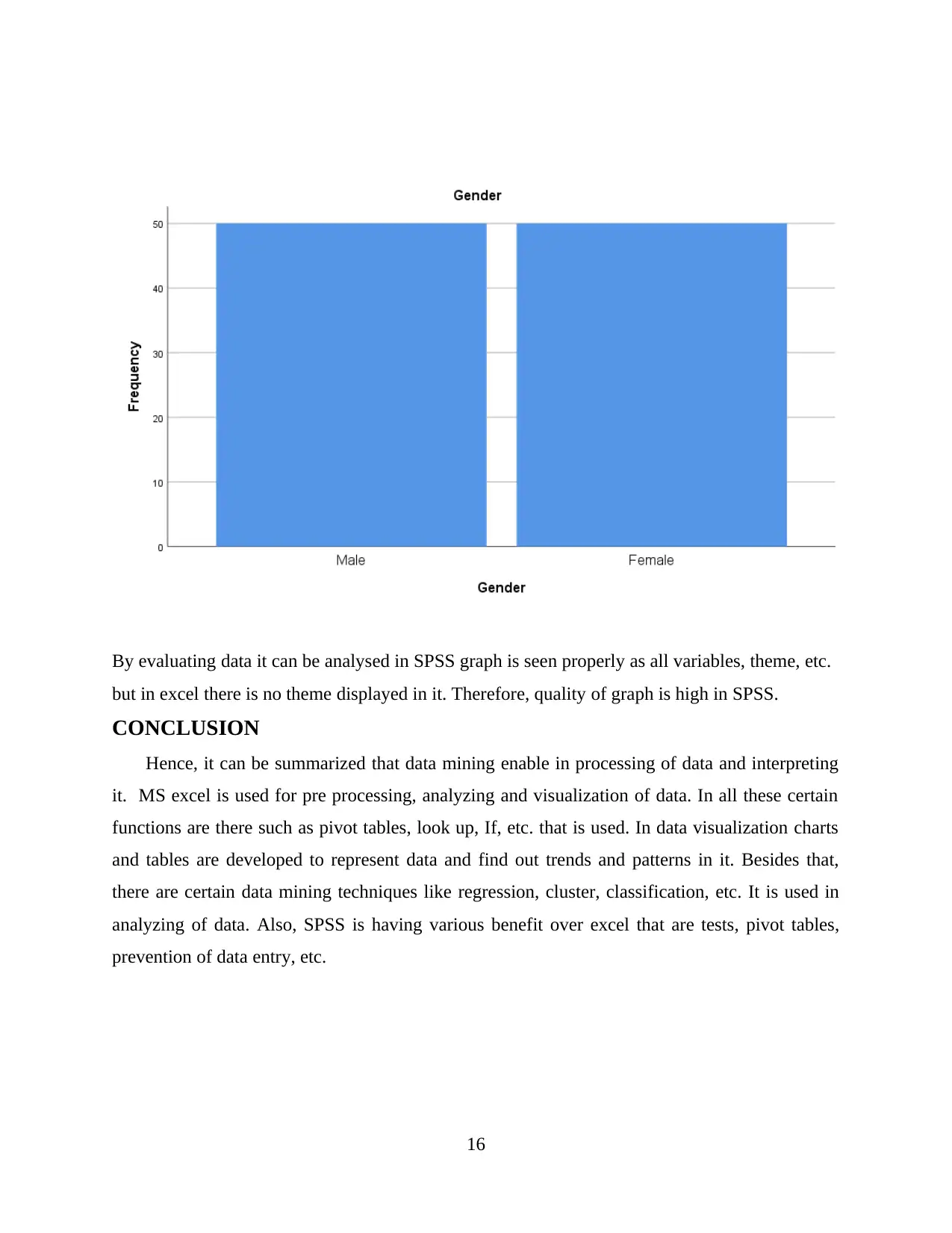
By evaluating data it can be analysed in SPSS graph is seen properly as all variables, theme, etc.
but in excel there is no theme displayed in it. Therefore, quality of graph is high in SPSS.
CONCLUSION
Hence, it can be summarized that data mining enable in processing of data and interpreting
it. MS excel is used for pre processing, analyzing and visualization of data. In all these certain
functions are there such as pivot tables, look up, If, etc. that is used. In data visualization charts
and tables are developed to represent data and find out trends and patterns in it. Besides that,
there are certain data mining techniques like regression, cluster, classification, etc. It is used in
analyzing of data. Also, SPSS is having various benefit over excel that are tests, pivot tables,
prevention of data entry, etc.
16
but in excel there is no theme displayed in it. Therefore, quality of graph is high in SPSS.
CONCLUSION
Hence, it can be summarized that data mining enable in processing of data and interpreting
it. MS excel is used for pre processing, analyzing and visualization of data. In all these certain
functions are there such as pivot tables, look up, If, etc. that is used. In data visualization charts
and tables are developed to represent data and find out trends and patterns in it. Besides that,
there are certain data mining techniques like regression, cluster, classification, etc. It is used in
analyzing of data. Also, SPSS is having various benefit over excel that are tests, pivot tables,
prevention of data entry, etc.
16
Paraphrase This Document
Need a fresh take? Get an instant paraphrase of this document with our AI Paraphraser
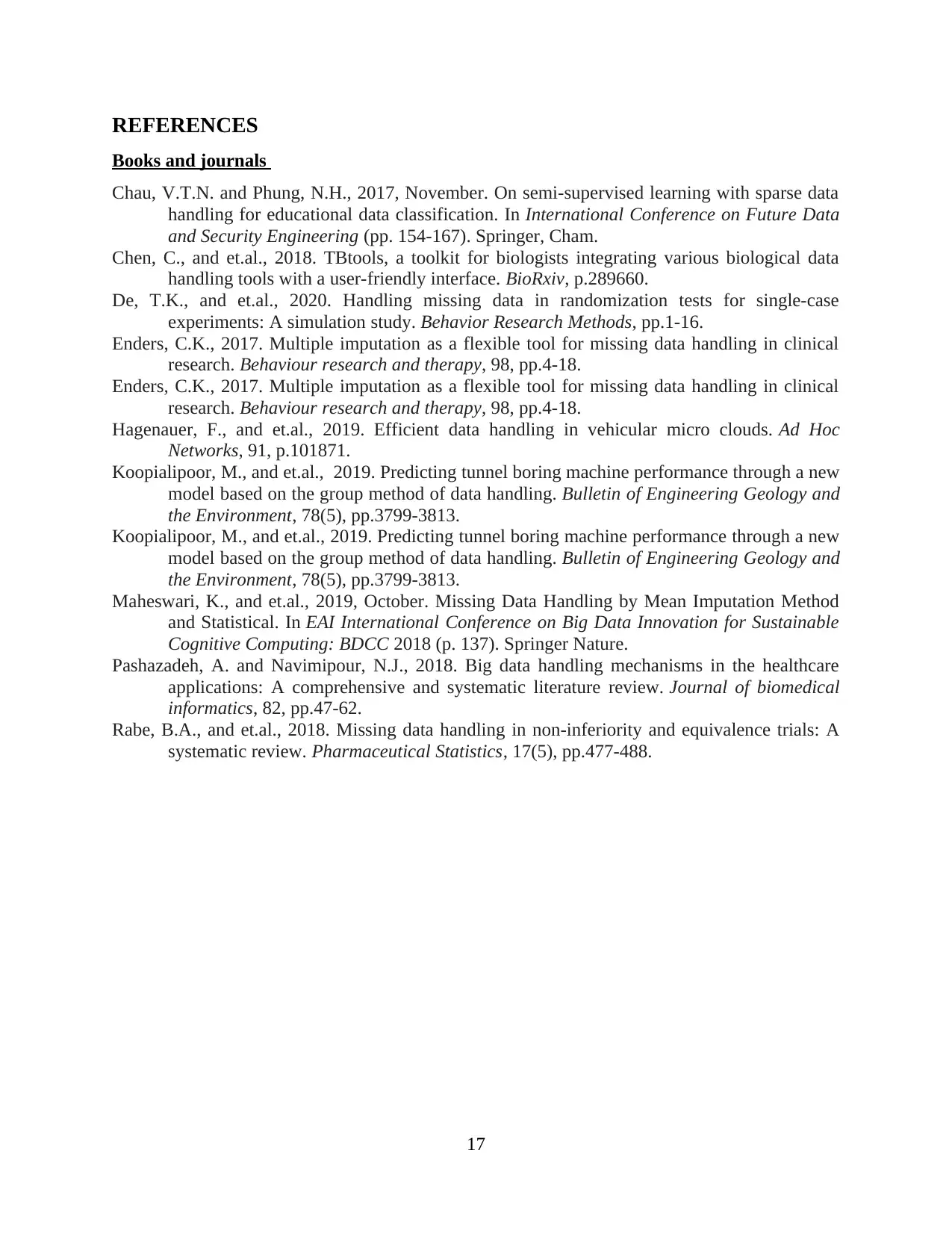
REFERENCES
Books and journals
Chau, V.T.N. and Phung, N.H., 2017, November. On semi-supervised learning with sparse data
handling for educational data classification. In International Conference on Future Data
and Security Engineering (pp. 154-167). Springer, Cham.
Chen, C., and et.al., 2018. TBtools, a toolkit for biologists integrating various biological data
handling tools with a user-friendly interface. BioRxiv, p.289660.
De, T.K., and et.al., 2020. Handling missing data in randomization tests for single-case
experiments: A simulation study. Behavior Research Methods, pp.1-16.
Enders, C.K., 2017. Multiple imputation as a flexible tool for missing data handling in clinical
research. Behaviour research and therapy, 98, pp.4-18.
Enders, C.K., 2017. Multiple imputation as a flexible tool for missing data handling in clinical
research. Behaviour research and therapy, 98, pp.4-18.
Hagenauer, F., and et.al., 2019. Efficient data handling in vehicular micro clouds. Ad Hoc
Networks, 91, p.101871.
Koopialipoor, M., and et.al., 2019. Predicting tunnel boring machine performance through a new
model based on the group method of data handling. Bulletin of Engineering Geology and
the Environment, 78(5), pp.3799-3813.
Koopialipoor, M., and et.al., 2019. Predicting tunnel boring machine performance through a new
model based on the group method of data handling. Bulletin of Engineering Geology and
the Environment, 78(5), pp.3799-3813.
Maheswari, K., and et.al., 2019, October. Missing Data Handling by Mean Imputation Method
and Statistical. In EAI International Conference on Big Data Innovation for Sustainable
Cognitive Computing: BDCC 2018 (p. 137). Springer Nature.
Pashazadeh, A. and Navimipour, N.J., 2018. Big data handling mechanisms in the healthcare
applications: A comprehensive and systematic literature review. Journal of biomedical
informatics, 82, pp.47-62.
Rabe, B.A., and et.al., 2018. Missing data handling in non‐inferiority and equivalence trials: A
systematic review. Pharmaceutical Statistics, 17(5), pp.477-488.
17
Books and journals
Chau, V.T.N. and Phung, N.H., 2017, November. On semi-supervised learning with sparse data
handling for educational data classification. In International Conference on Future Data
and Security Engineering (pp. 154-167). Springer, Cham.
Chen, C., and et.al., 2018. TBtools, a toolkit for biologists integrating various biological data
handling tools with a user-friendly interface. BioRxiv, p.289660.
De, T.K., and et.al., 2020. Handling missing data in randomization tests for single-case
experiments: A simulation study. Behavior Research Methods, pp.1-16.
Enders, C.K., 2017. Multiple imputation as a flexible tool for missing data handling in clinical
research. Behaviour research and therapy, 98, pp.4-18.
Enders, C.K., 2017. Multiple imputation as a flexible tool for missing data handling in clinical
research. Behaviour research and therapy, 98, pp.4-18.
Hagenauer, F., and et.al., 2019. Efficient data handling in vehicular micro clouds. Ad Hoc
Networks, 91, p.101871.
Koopialipoor, M., and et.al., 2019. Predicting tunnel boring machine performance through a new
model based on the group method of data handling. Bulletin of Engineering Geology and
the Environment, 78(5), pp.3799-3813.
Koopialipoor, M., and et.al., 2019. Predicting tunnel boring machine performance through a new
model based on the group method of data handling. Bulletin of Engineering Geology and
the Environment, 78(5), pp.3799-3813.
Maheswari, K., and et.al., 2019, October. Missing Data Handling by Mean Imputation Method
and Statistical. In EAI International Conference on Big Data Innovation for Sustainable
Cognitive Computing: BDCC 2018 (p. 137). Springer Nature.
Pashazadeh, A. and Navimipour, N.J., 2018. Big data handling mechanisms in the healthcare
applications: A comprehensive and systematic literature review. Journal of biomedical
informatics, 82, pp.47-62.
Rabe, B.A., and et.al., 2018. Missing data handling in non‐inferiority and equivalence trials: A
systematic review. Pharmaceutical Statistics, 17(5), pp.477-488.
17
1 out of 17
Related Documents

Your All-in-One AI-Powered Toolkit for Academic Success.
+13062052269
info@desklib.com
Available 24*7 on WhatsApp / Email
Unlock your academic potential
© 2024 | Zucol Services PVT LTD | All rights reserved.