Product Break-Even Analysis and Profit Targets
VerifiedAdded on 2020/03/23
|15
|2022
|181
AI Summary
This assignment analyzes the break-even point and profit targets for two products (A and B) with given contribution margins, fixed costs, and a product mix ratio. It calculates the break-even point in units and dollar value for each product, then determines the required sales volume to achieve a pre-tax profit of $5,000 and $30,000, considering the product mix ratio. The calculations involve formulas for unit and dollar break-even points, as well as adjustments for the product mix.
Contribute Materials
Your contribution can guide someone’s learning journey. Share your
documents today.
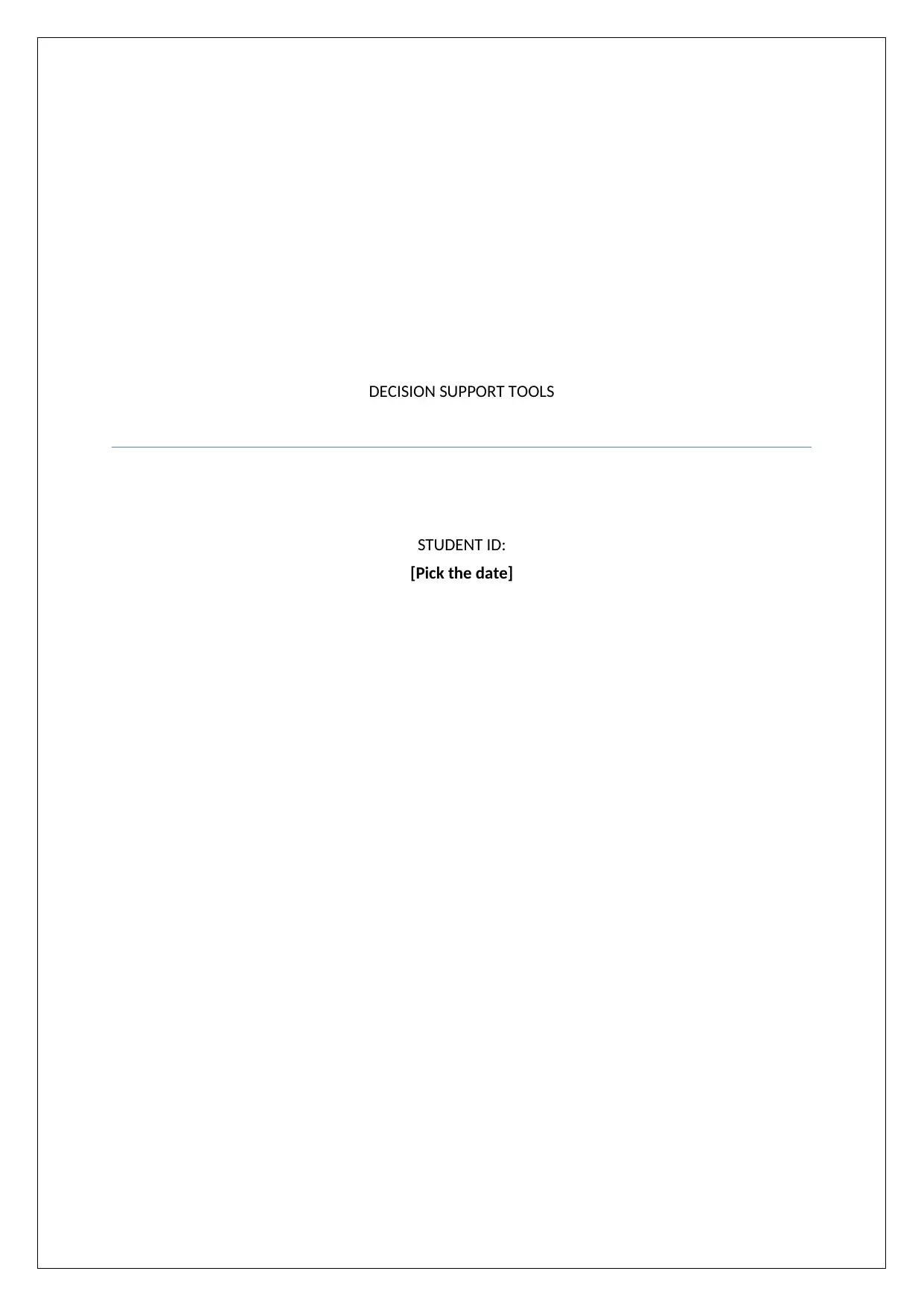
DECISION SUPPORT TOOLS
STUDENT ID:
[Pick the date]
STUDENT ID:
[Pick the date]
Secure Best Marks with AI Grader
Need help grading? Try our AI Grader for instant feedback on your assignments.
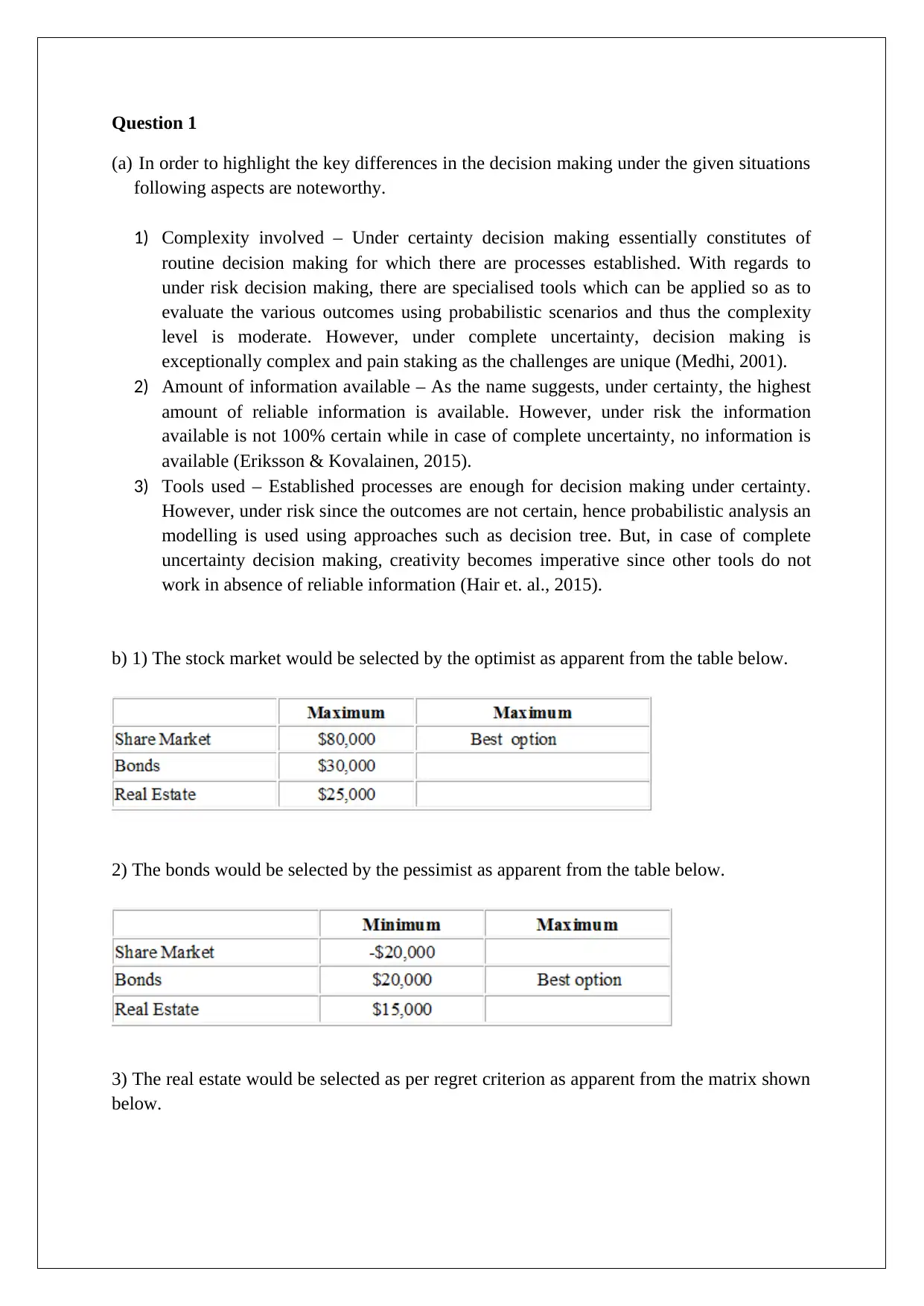
Question 1
(a) In order to highlight the key differences in the decision making under the given situations
following aspects are noteworthy.
1) Complexity involved – Under certainty decision making essentially constitutes of
routine decision making for which there are processes established. With regards to
under risk decision making, there are specialised tools which can be applied so as to
evaluate the various outcomes using probabilistic scenarios and thus the complexity
level is moderate. However, under complete uncertainty, decision making is
exceptionally complex and pain staking as the challenges are unique (Medhi, 2001).
2) Amount of information available – As the name suggests, under certainty, the highest
amount of reliable information is available. However, under risk the information
available is not 100% certain while in case of complete uncertainty, no information is
available (Eriksson & Kovalainen, 2015).
3) Tools used – Established processes are enough for decision making under certainty.
However, under risk since the outcomes are not certain, hence probabilistic analysis an
modelling is used using approaches such as decision tree. But, in case of complete
uncertainty decision making, creativity becomes imperative since other tools do not
work in absence of reliable information (Hair et. al., 2015).
b) 1) The stock market would be selected by the optimist as apparent from the table below.
2) The bonds would be selected by the pessimist as apparent from the table below.
3) The real estate would be selected as per regret criterion as apparent from the matrix shown
below.
(a) In order to highlight the key differences in the decision making under the given situations
following aspects are noteworthy.
1) Complexity involved – Under certainty decision making essentially constitutes of
routine decision making for which there are processes established. With regards to
under risk decision making, there are specialised tools which can be applied so as to
evaluate the various outcomes using probabilistic scenarios and thus the complexity
level is moderate. However, under complete uncertainty, decision making is
exceptionally complex and pain staking as the challenges are unique (Medhi, 2001).
2) Amount of information available – As the name suggests, under certainty, the highest
amount of reliable information is available. However, under risk the information
available is not 100% certain while in case of complete uncertainty, no information is
available (Eriksson & Kovalainen, 2015).
3) Tools used – Established processes are enough for decision making under certainty.
However, under risk since the outcomes are not certain, hence probabilistic analysis an
modelling is used using approaches such as decision tree. But, in case of complete
uncertainty decision making, creativity becomes imperative since other tools do not
work in absence of reliable information (Hair et. al., 2015).
b) 1) The stock market would be selected by the optimist as apparent from the table below.
2) The bonds would be selected by the pessimist as apparent from the table below.
3) The real estate would be selected as per regret criterion as apparent from the matrix shown
below.
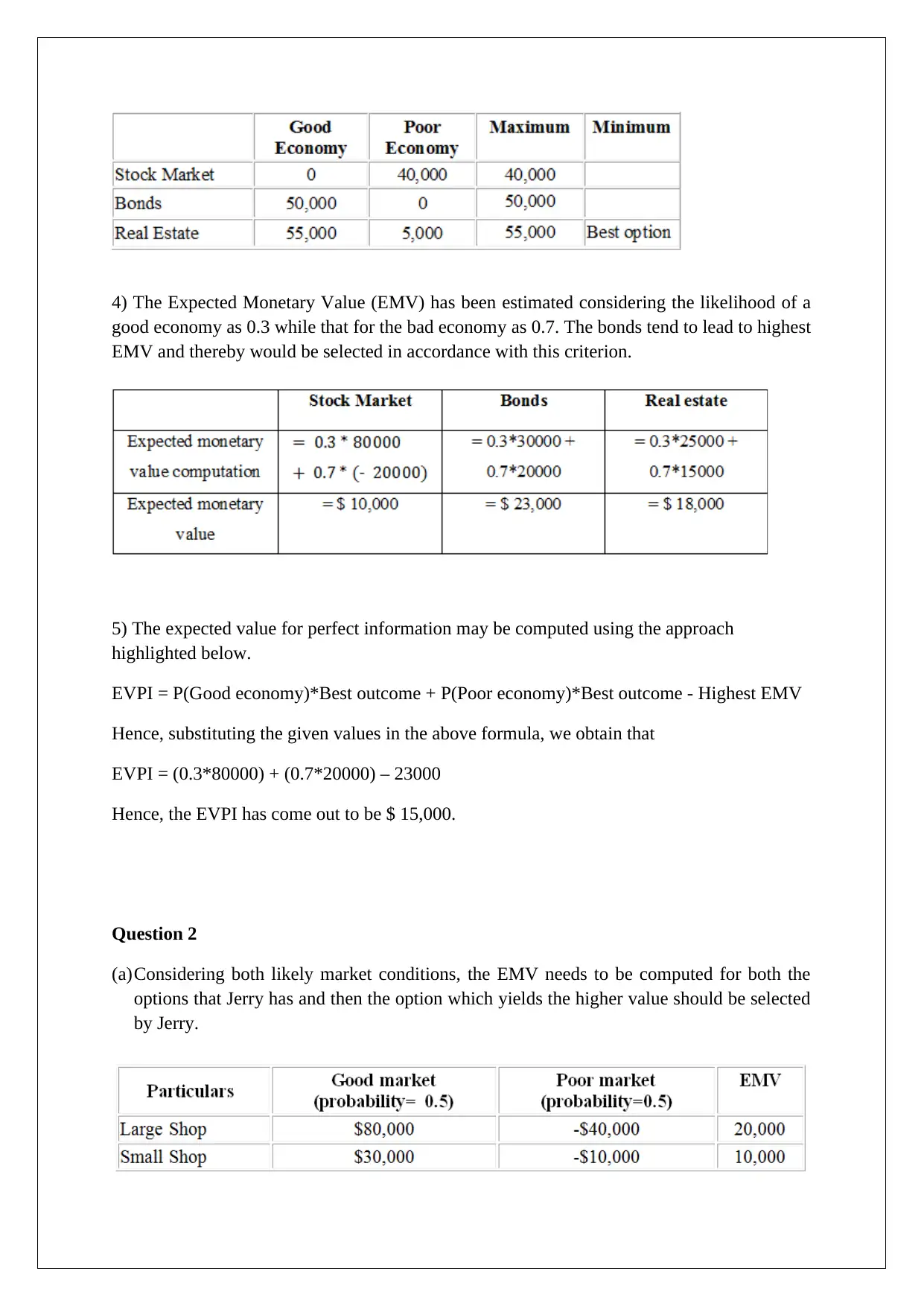
4) The Expected Monetary Value (EMV) has been estimated considering the likelihood of a
good economy as 0.3 while that for the bad economy as 0.7. The bonds tend to lead to highest
EMV and thereby would be selected in accordance with this criterion.
5) The expected value for perfect information may be computed using the approach
highlighted below.
EVPI = P(Good economy)*Best outcome + P(Poor economy)*Best outcome - Highest EMV
Hence, substituting the given values in the above formula, we obtain that
EVPI = (0.3*80000) + (0.7*20000) – 23000
Hence, the EVPI has come out to be $ 15,000.
Question 2
(a)Considering both likely market conditions, the EMV needs to be computed for both the
options that Jerry has and then the option which yields the higher value should be selected
by Jerry.
good economy as 0.3 while that for the bad economy as 0.7. The bonds tend to lead to highest
EMV and thereby would be selected in accordance with this criterion.
5) The expected value for perfect information may be computed using the approach
highlighted below.
EVPI = P(Good economy)*Best outcome + P(Poor economy)*Best outcome - Highest EMV
Hence, substituting the given values in the above formula, we obtain that
EVPI = (0.3*80000) + (0.7*20000) – 23000
Hence, the EVPI has come out to be $ 15,000.
Question 2
(a)Considering both likely market conditions, the EMV needs to be computed for both the
options that Jerry has and then the option which yields the higher value should be selected
by Jerry.
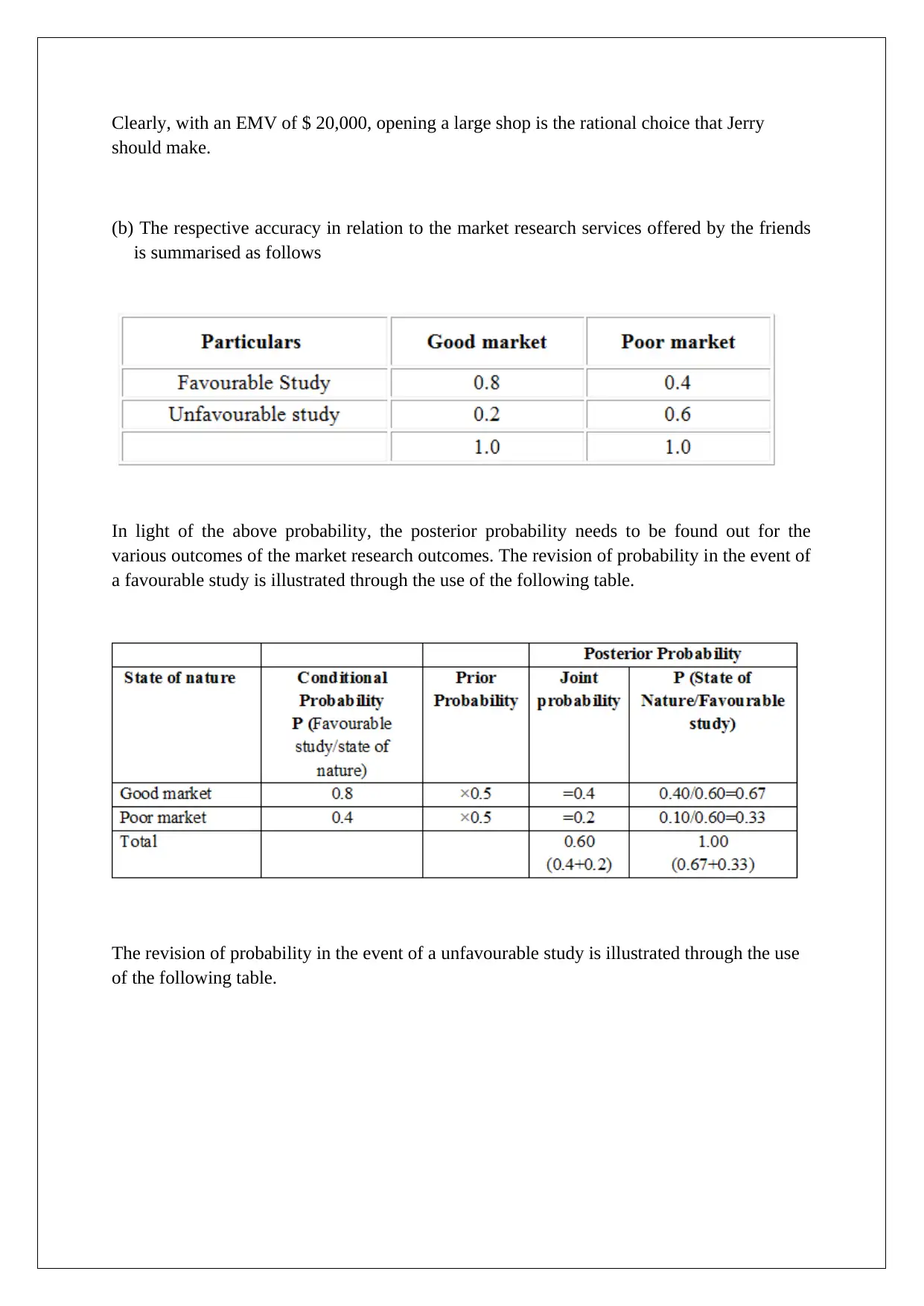
Clearly, with an EMV of $ 20,000, opening a large shop is the rational choice that Jerry
should make.
(b) The respective accuracy in relation to the market research services offered by the friends
is summarised as follows
In light of the above probability, the posterior probability needs to be found out for the
various outcomes of the market research outcomes. The revision of probability in the event of
a favourable study is illustrated through the use of the following table.
The revision of probability in the event of a unfavourable study is illustrated through the use
of the following table.
should make.
(b) The respective accuracy in relation to the market research services offered by the friends
is summarised as follows
In light of the above probability, the posterior probability needs to be found out for the
various outcomes of the market research outcomes. The revision of probability in the event of
a favourable study is illustrated through the use of the following table.
The revision of probability in the event of a unfavourable study is illustrated through the use
of the following table.
Secure Best Marks with AI Grader
Need help grading? Try our AI Grader for instant feedback on your assignments.
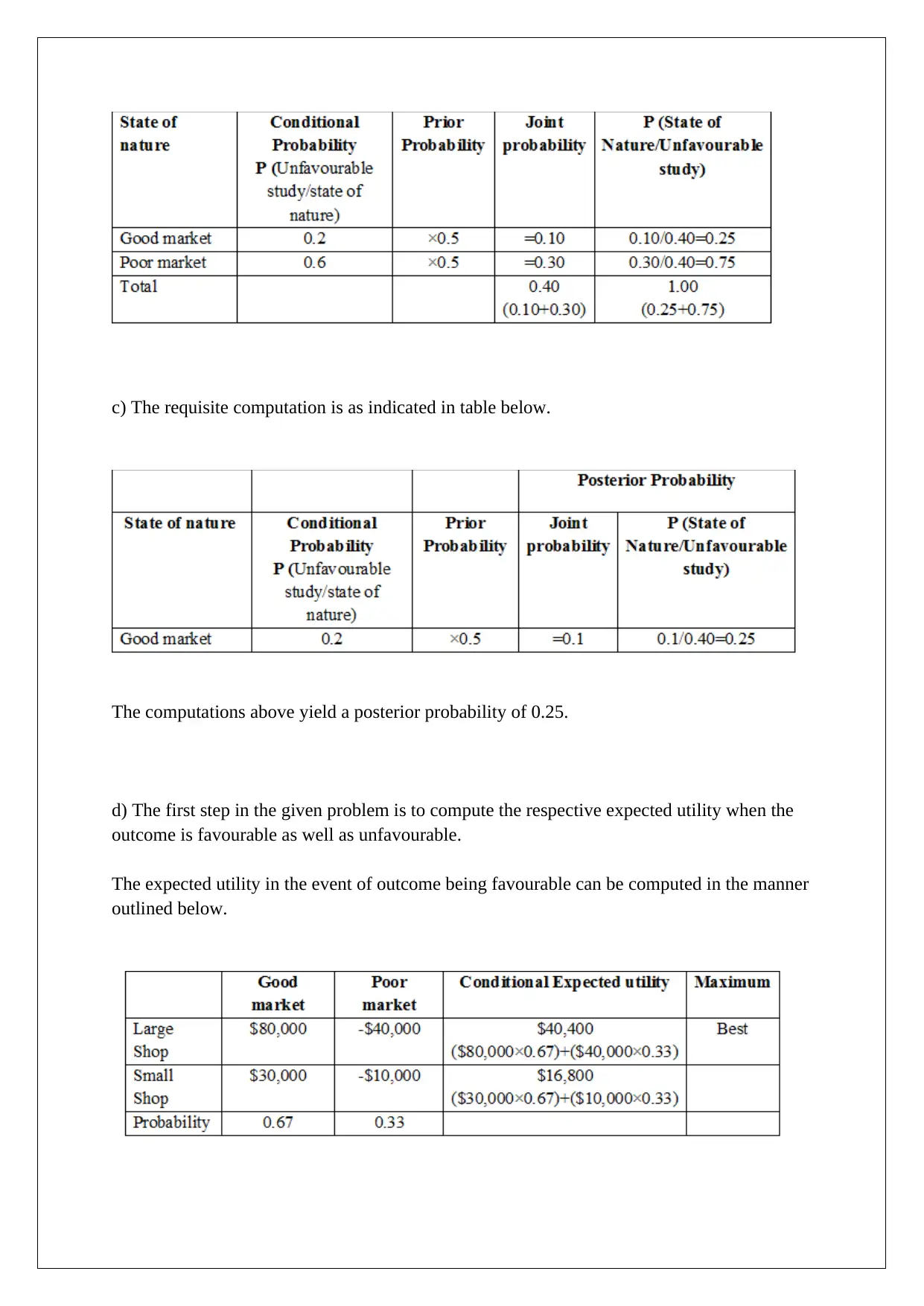
c) The requisite computation is as indicated in table below.
The computations above yield a posterior probability of 0.25.
d) The first step in the given problem is to compute the respective expected utility when the
outcome is favourable as well as unfavourable.
The expected utility in the event of outcome being favourable can be computed in the manner
outlined below.
The computations above yield a posterior probability of 0.25.
d) The first step in the given problem is to compute the respective expected utility when the
outcome is favourable as well as unfavourable.
The expected utility in the event of outcome being favourable can be computed in the manner
outlined below.
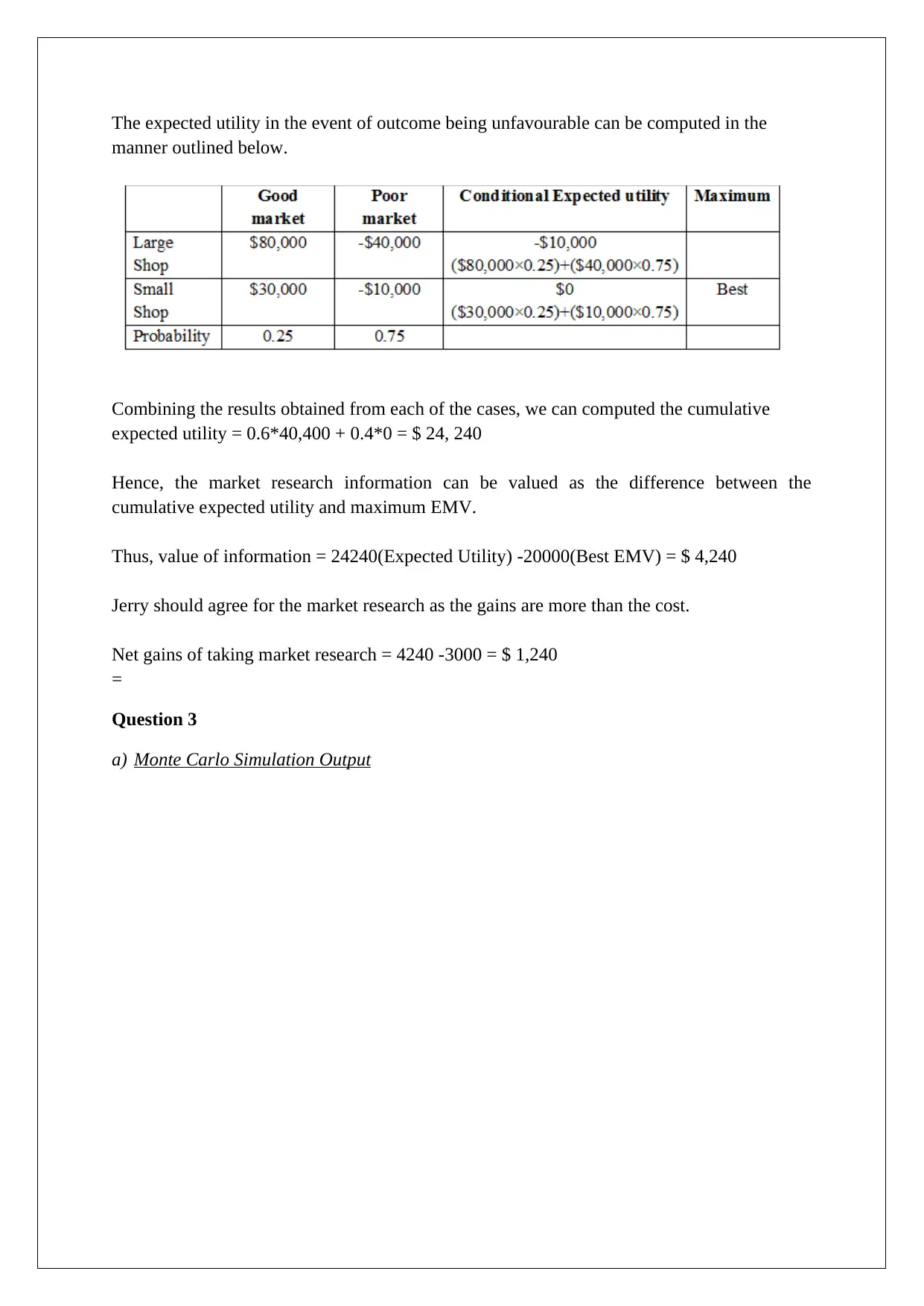
The expected utility in the event of outcome being unfavourable can be computed in the
manner outlined below.
Combining the results obtained from each of the cases, we can computed the cumulative
expected utility = 0.6*40,400 + 0.4*0 = $ 24, 240
Hence, the market research information can be valued as the difference between the
cumulative expected utility and maximum EMV.
Thus, value of information = 24240(Expected Utility) -20000(Best EMV) = $ 4,240
Jerry should agree for the market research as the gains are more than the cost.
Net gains of taking market research = 4240 -3000 = $ 1,240
=
Question 3
a) Monte Carlo Simulation Output
manner outlined below.
Combining the results obtained from each of the cases, we can computed the cumulative
expected utility = 0.6*40,400 + 0.4*0 = $ 24, 240
Hence, the market research information can be valued as the difference between the
cumulative expected utility and maximum EMV.
Thus, value of information = 24240(Expected Utility) -20000(Best EMV) = $ 4,240
Jerry should agree for the market research as the gains are more than the cost.
Net gains of taking market research = 4240 -3000 = $ 1,240
=
Question 3
a) Monte Carlo Simulation Output
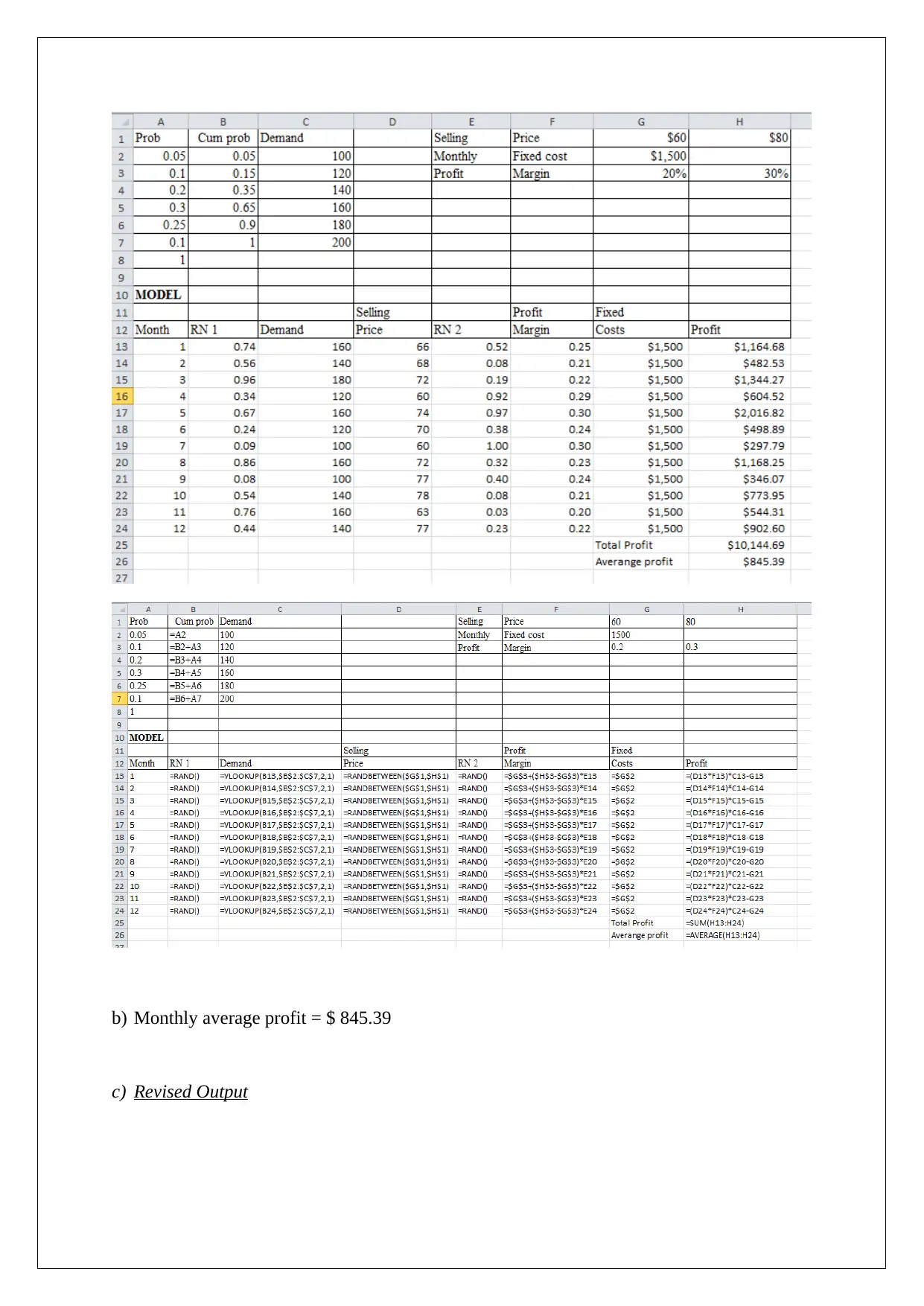
b) Monthly average profit = $ 845.39
c) Revised Output
c) Revised Output
Paraphrase This Document
Need a fresh take? Get an instant paraphrase of this document with our AI Paraphraser
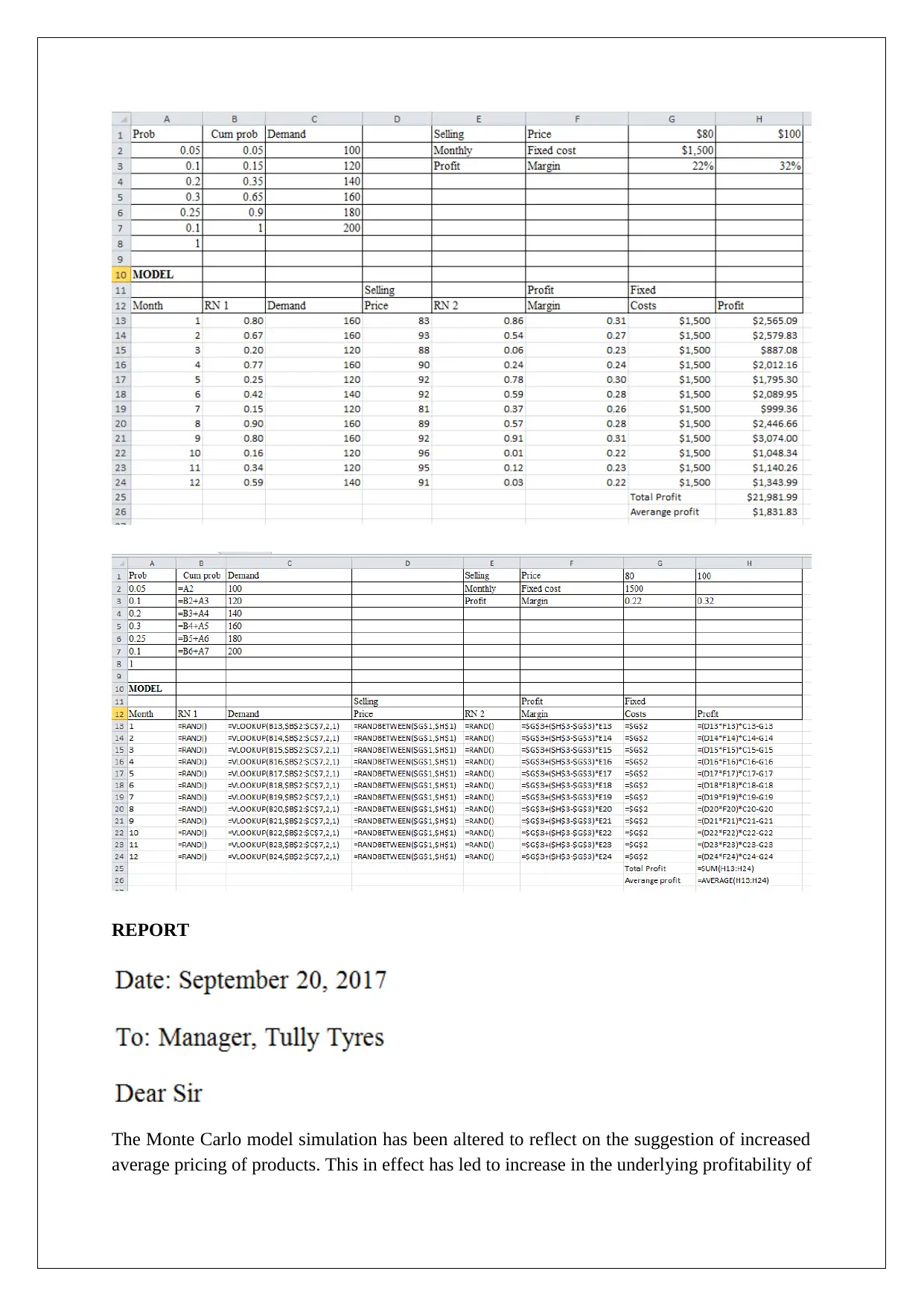
REPORT
The Monte Carlo model simulation has been altered to reflect on the suggestion of increased
average pricing of products. This in effect has led to increase in the underlying profitability of
The Monte Carlo model simulation has been altered to reflect on the suggestion of increased
average pricing of products. This in effect has led to increase in the underlying profitability of
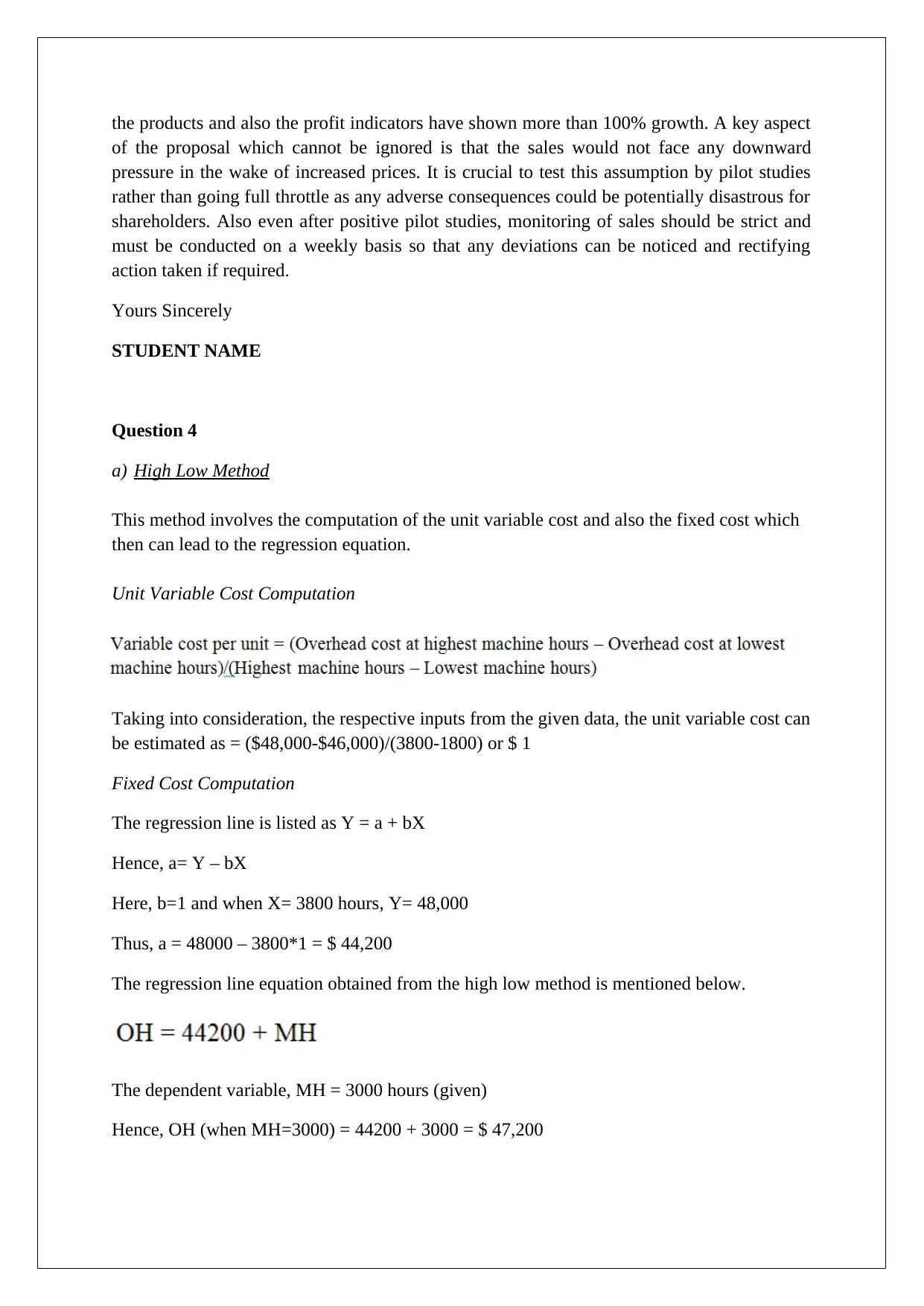
the products and also the profit indicators have shown more than 100% growth. A key aspect
of the proposal which cannot be ignored is that the sales would not face any downward
pressure in the wake of increased prices. It is crucial to test this assumption by pilot studies
rather than going full throttle as any adverse consequences could be potentially disastrous for
shareholders. Also even after positive pilot studies, monitoring of sales should be strict and
must be conducted on a weekly basis so that any deviations can be noticed and rectifying
action taken if required.
Yours Sincerely
STUDENT NAME
Question 4
a) High Low Method
This method involves the computation of the unit variable cost and also the fixed cost which
then can lead to the regression equation.
Unit Variable Cost Computation
Taking into consideration, the respective inputs from the given data, the unit variable cost can
be estimated as = ($48,000-$46,000)/(3800-1800) or $ 1
Fixed Cost Computation
The regression line is listed as Y = a + bX
Hence, a= Y – bX
Here, b=1 and when X= 3800 hours, Y= 48,000
Thus, a = 48000 – 3800*1 = $ 44,200
The regression line equation obtained from the high low method is mentioned below.
The dependent variable, MH = 3000 hours (given)
Hence, OH (when MH=3000) = 44200 + 3000 = $ 47,200
of the proposal which cannot be ignored is that the sales would not face any downward
pressure in the wake of increased prices. It is crucial to test this assumption by pilot studies
rather than going full throttle as any adverse consequences could be potentially disastrous for
shareholders. Also even after positive pilot studies, monitoring of sales should be strict and
must be conducted on a weekly basis so that any deviations can be noticed and rectifying
action taken if required.
Yours Sincerely
STUDENT NAME
Question 4
a) High Low Method
This method involves the computation of the unit variable cost and also the fixed cost which
then can lead to the regression equation.
Unit Variable Cost Computation
Taking into consideration, the respective inputs from the given data, the unit variable cost can
be estimated as = ($48,000-$46,000)/(3800-1800) or $ 1
Fixed Cost Computation
The regression line is listed as Y = a + bX
Hence, a= Y – bX
Here, b=1 and when X= 3800 hours, Y= 48,000
Thus, a = 48000 – 3800*1 = $ 44,200
The regression line equation obtained from the high low method is mentioned below.
The dependent variable, MH = 3000 hours (given)
Hence, OH (when MH=3000) = 44200 + 3000 = $ 47,200
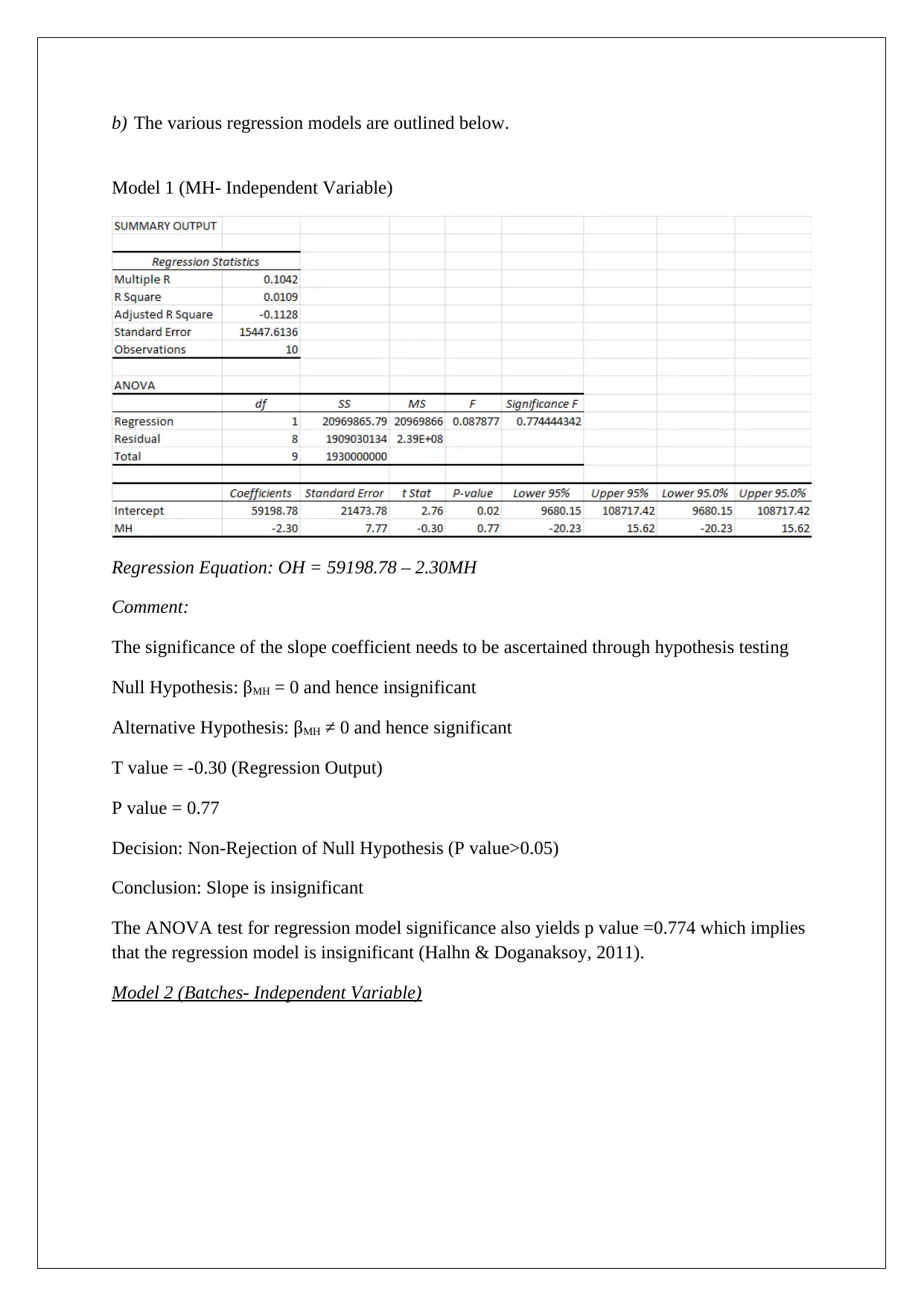
b) The various regression models are outlined below.
Model 1 (MH- Independent Variable)
Regression Equation: OH = 59198.78 – 2.30MH
Comment:
The significance of the slope coefficient needs to be ascertained through hypothesis testing
Null Hypothesis: βMH = 0 and hence insignificant
Alternative Hypothesis: βMH ≠ 0 and hence significant
T value = -0.30 (Regression Output)
P value = 0.77
Decision: Non-Rejection of Null Hypothesis (P value>0.05)
Conclusion: Slope is insignificant
The ANOVA test for regression model significance also yields p value =0.774 which implies
that the regression model is insignificant (Halhn & Doganaksoy, 2011).
Model 2 (Batches- Independent Variable)
Model 1 (MH- Independent Variable)
Regression Equation: OH = 59198.78 – 2.30MH
Comment:
The significance of the slope coefficient needs to be ascertained through hypothesis testing
Null Hypothesis: βMH = 0 and hence insignificant
Alternative Hypothesis: βMH ≠ 0 and hence significant
T value = -0.30 (Regression Output)
P value = 0.77
Decision: Non-Rejection of Null Hypothesis (P value>0.05)
Conclusion: Slope is insignificant
The ANOVA test for regression model significance also yields p value =0.774 which implies
that the regression model is insignificant (Halhn & Doganaksoy, 2011).
Model 2 (Batches- Independent Variable)
Secure Best Marks with AI Grader
Need help grading? Try our AI Grader for instant feedback on your assignments.
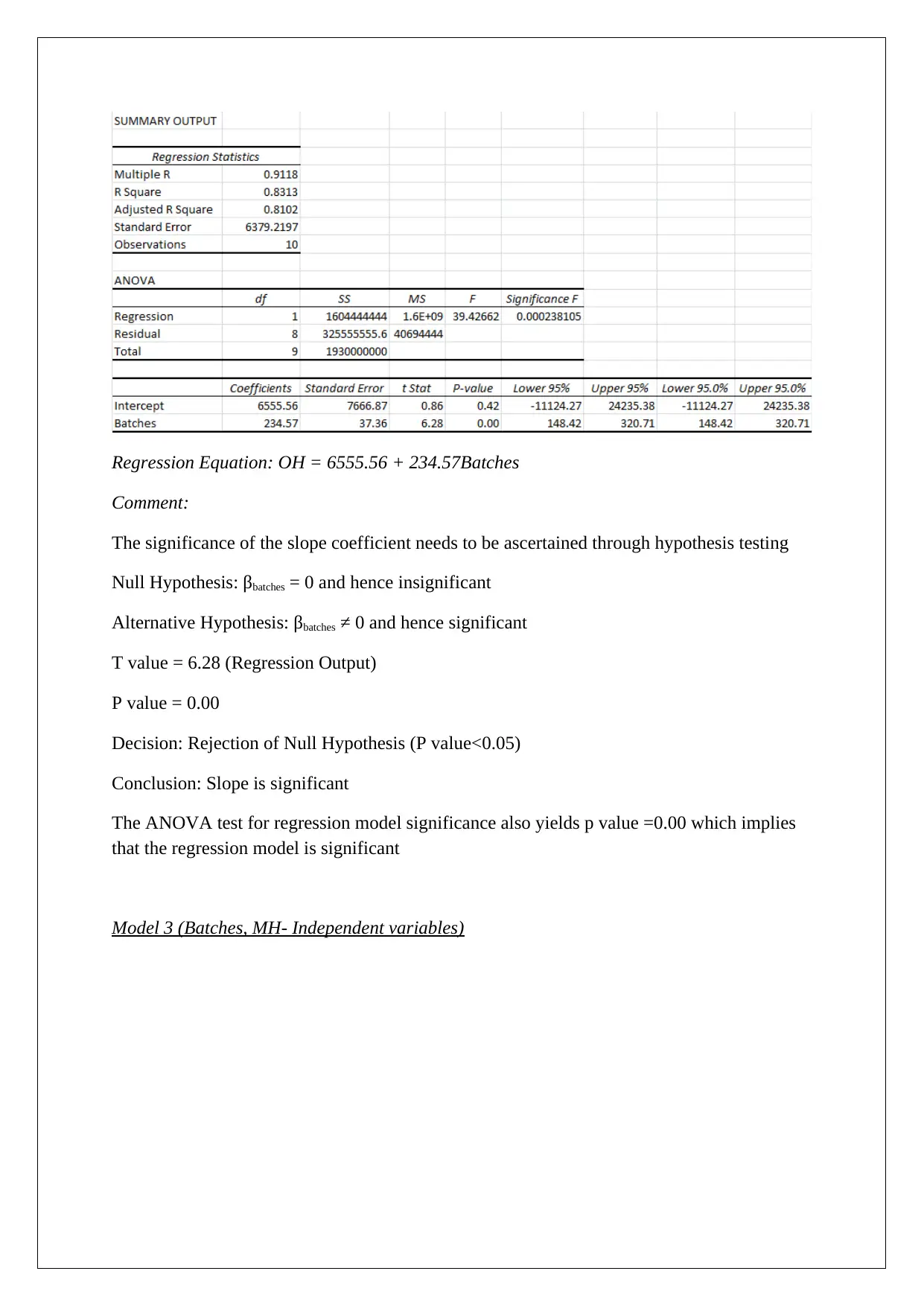
Regression Equation: OH = 6555.56 + 234.57Batches
Comment:
The significance of the slope coefficient needs to be ascertained through hypothesis testing
Null Hypothesis: βbatches = 0 and hence insignificant
Alternative Hypothesis: βbatches ≠ 0 and hence significant
T value = 6.28 (Regression Output)
P value = 0.00
Decision: Rejection of Null Hypothesis (P value<0.05)
Conclusion: Slope is significant
The ANOVA test for regression model significance also yields p value =0.00 which implies
that the regression model is significant
Model 3 (Batches, MH- Independent variables)
Comment:
The significance of the slope coefficient needs to be ascertained through hypothesis testing
Null Hypothesis: βbatches = 0 and hence insignificant
Alternative Hypothesis: βbatches ≠ 0 and hence significant
T value = 6.28 (Regression Output)
P value = 0.00
Decision: Rejection of Null Hypothesis (P value<0.05)
Conclusion: Slope is significant
The ANOVA test for regression model significance also yields p value =0.00 which implies
that the regression model is significant
Model 3 (Batches, MH- Independent variables)
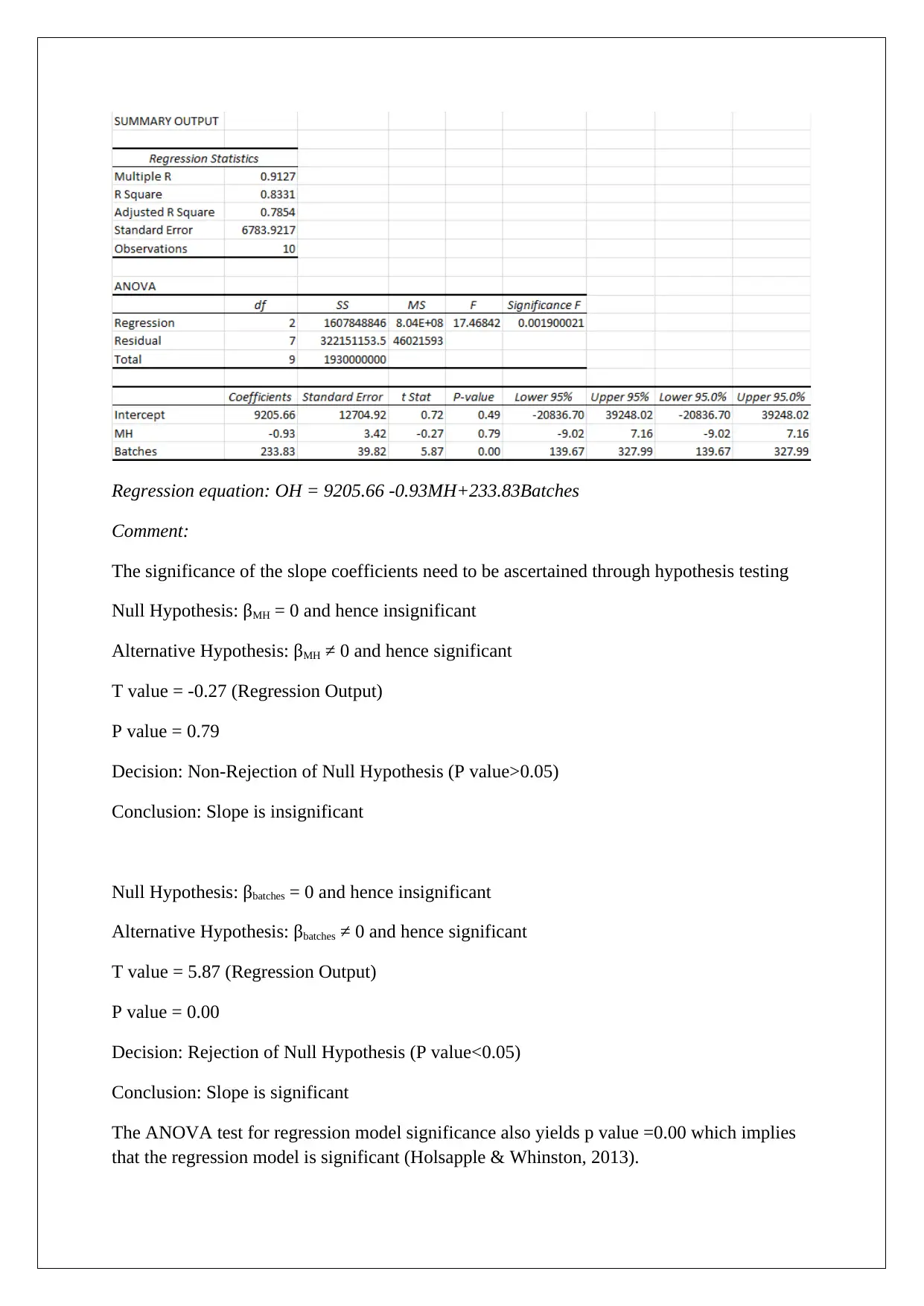
Regression equation: OH = 9205.66 -0.93MH+233.83Batches
Comment:
The significance of the slope coefficients need to be ascertained through hypothesis testing
Null Hypothesis: βMH = 0 and hence insignificant
Alternative Hypothesis: βMH ≠ 0 and hence significant
T value = -0.27 (Regression Output)
P value = 0.79
Decision: Non-Rejection of Null Hypothesis (P value>0.05)
Conclusion: Slope is insignificant
Null Hypothesis: βbatches = 0 and hence insignificant
Alternative Hypothesis: βbatches ≠ 0 and hence significant
T value = 5.87 (Regression Output)
P value = 0.00
Decision: Rejection of Null Hypothesis (P value<0.05)
Conclusion: Slope is significant
The ANOVA test for regression model significance also yields p value =0.00 which implies
that the regression model is significant (Holsapple & Whinston, 2013).
Comment:
The significance of the slope coefficients need to be ascertained through hypothesis testing
Null Hypothesis: βMH = 0 and hence insignificant
Alternative Hypothesis: βMH ≠ 0 and hence significant
T value = -0.27 (Regression Output)
P value = 0.79
Decision: Non-Rejection of Null Hypothesis (P value>0.05)
Conclusion: Slope is insignificant
Null Hypothesis: βbatches = 0 and hence insignificant
Alternative Hypothesis: βbatches ≠ 0 and hence significant
T value = 5.87 (Regression Output)
P value = 0.00
Decision: Rejection of Null Hypothesis (P value<0.05)
Conclusion: Slope is significant
The ANOVA test for regression model significance also yields p value =0.00 which implies
that the regression model is significant (Holsapple & Whinston, 2013).
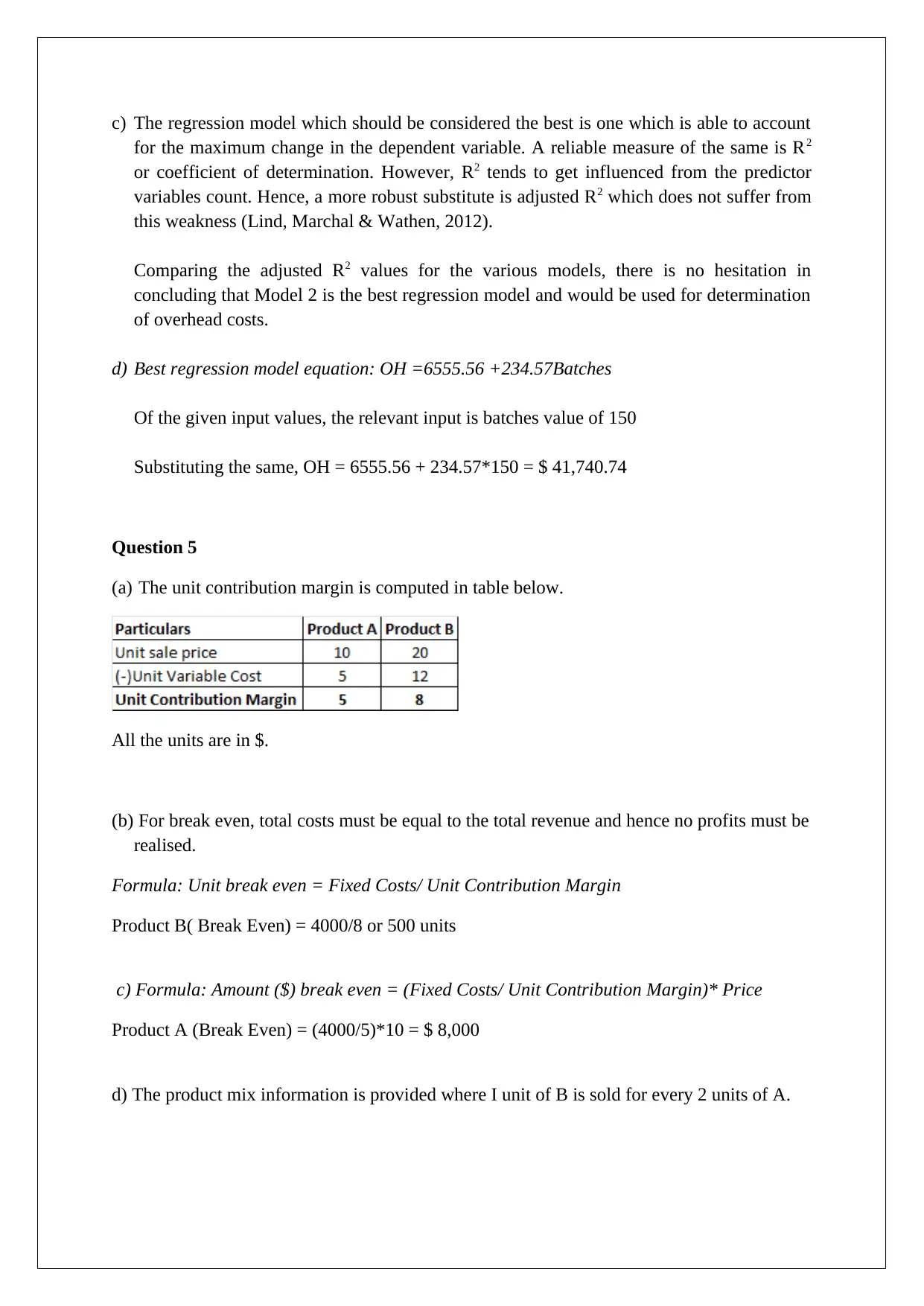
c) The regression model which should be considered the best is one which is able to account
for the maximum change in the dependent variable. A reliable measure of the same is R2
or coefficient of determination. However, R2 tends to get influenced from the predictor
variables count. Hence, a more robust substitute is adjusted R2 which does not suffer from
this weakness (Lind, Marchal & Wathen, 2012).
Comparing the adjusted R2 values for the various models, there is no hesitation in
concluding that Model 2 is the best regression model and would be used for determination
of overhead costs.
d) Best regression model equation: OH =6555.56 +234.57Batches
Of the given input values, the relevant input is batches value of 150
Substituting the same, OH = 6555.56 + 234.57*150 = $ 41,740.74
Question 5
(a) The unit contribution margin is computed in table below.
All the units are in $.
(b) For break even, total costs must be equal to the total revenue and hence no profits must be
realised.
Formula: Unit break even = Fixed Costs/ Unit Contribution Margin
Product B( Break Even) = 4000/8 or 500 units
c) Formula: Amount ($) break even = (Fixed Costs/ Unit Contribution Margin)* Price
Product A (Break Even) = (4000/5)*10 = $ 8,000
d) The product mix information is provided where I unit of B is sold for every 2 units of A.
for the maximum change in the dependent variable. A reliable measure of the same is R2
or coefficient of determination. However, R2 tends to get influenced from the predictor
variables count. Hence, a more robust substitute is adjusted R2 which does not suffer from
this weakness (Lind, Marchal & Wathen, 2012).
Comparing the adjusted R2 values for the various models, there is no hesitation in
concluding that Model 2 is the best regression model and would be used for determination
of overhead costs.
d) Best regression model equation: OH =6555.56 +234.57Batches
Of the given input values, the relevant input is batches value of 150
Substituting the same, OH = 6555.56 + 234.57*150 = $ 41,740.74
Question 5
(a) The unit contribution margin is computed in table below.
All the units are in $.
(b) For break even, total costs must be equal to the total revenue and hence no profits must be
realised.
Formula: Unit break even = Fixed Costs/ Unit Contribution Margin
Product B( Break Even) = 4000/8 or 500 units
c) Formula: Amount ($) break even = (Fixed Costs/ Unit Contribution Margin)* Price
Product A (Break Even) = (4000/5)*10 = $ 8,000
d) The product mix information is provided where I unit of B is sold for every 2 units of A.
Paraphrase This Document
Need a fresh take? Get an instant paraphrase of this document with our AI Paraphraser
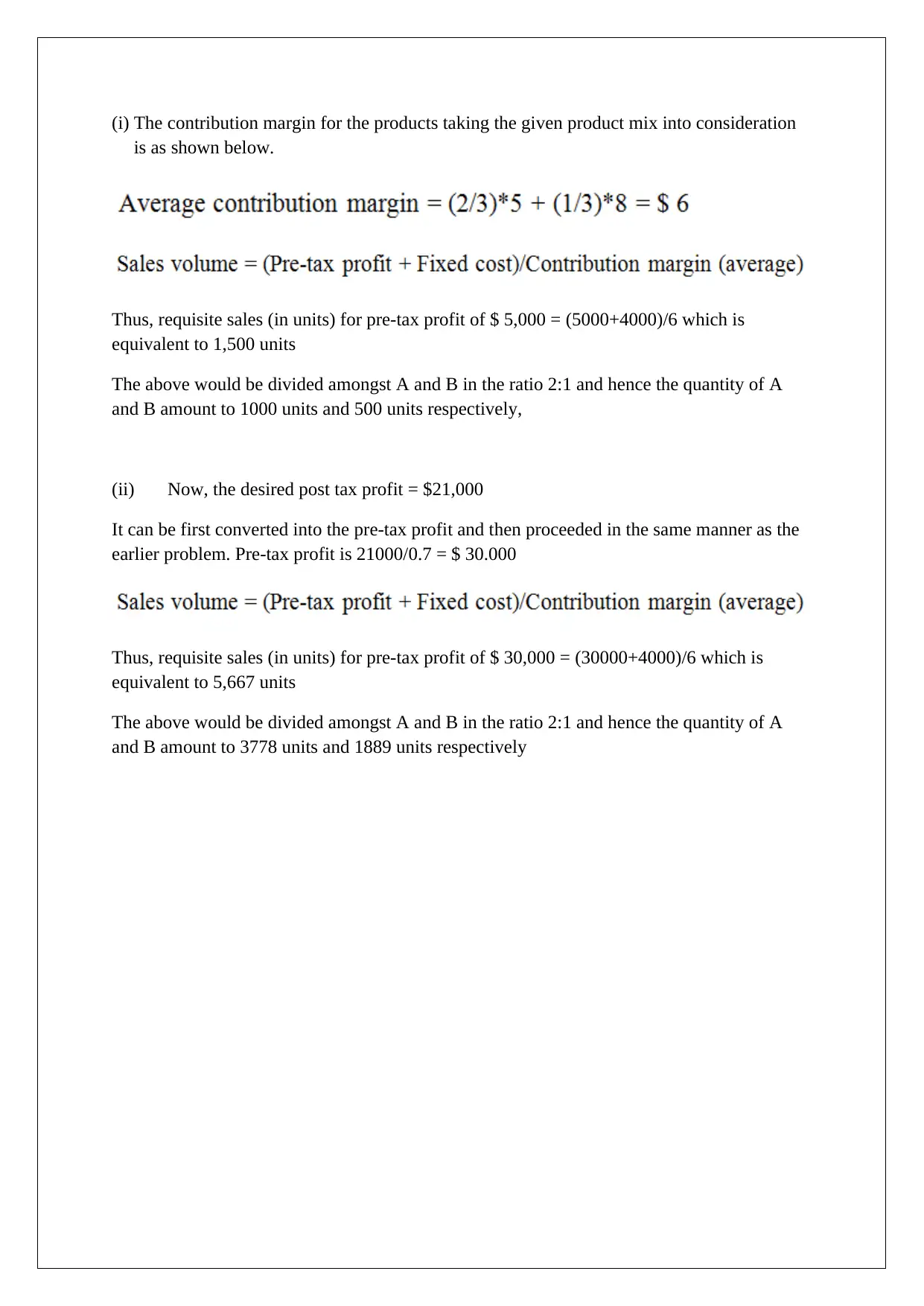
(i) The contribution margin for the products taking the given product mix into consideration
is as shown below.
Thus, requisite sales (in units) for pre-tax profit of $ 5,000 = (5000+4000)/6 which is
equivalent to 1,500 units
The above would be divided amongst A and B in the ratio 2:1 and hence the quantity of A
and B amount to 1000 units and 500 units respectively,
(ii) Now, the desired post tax profit = $21,000
It can be first converted into the pre-tax profit and then proceeded in the same manner as the
earlier problem. Pre-tax profit is 21000/0.7 = $ 30.000
Thus, requisite sales (in units) for pre-tax profit of $ 30,000 = (30000+4000)/6 which is
equivalent to 5,667 units
The above would be divided amongst A and B in the ratio 2:1 and hence the quantity of A
and B amount to 3778 units and 1889 units respectively
is as shown below.
Thus, requisite sales (in units) for pre-tax profit of $ 5,000 = (5000+4000)/6 which is
equivalent to 1,500 units
The above would be divided amongst A and B in the ratio 2:1 and hence the quantity of A
and B amount to 1000 units and 500 units respectively,
(ii) Now, the desired post tax profit = $21,000
It can be first converted into the pre-tax profit and then proceeded in the same manner as the
earlier problem. Pre-tax profit is 21000/0.7 = $ 30.000
Thus, requisite sales (in units) for pre-tax profit of $ 30,000 = (30000+4000)/6 which is
equivalent to 5,667 units
The above would be divided amongst A and B in the ratio 2:1 and hence the quantity of A
and B amount to 3778 units and 1889 units respectively
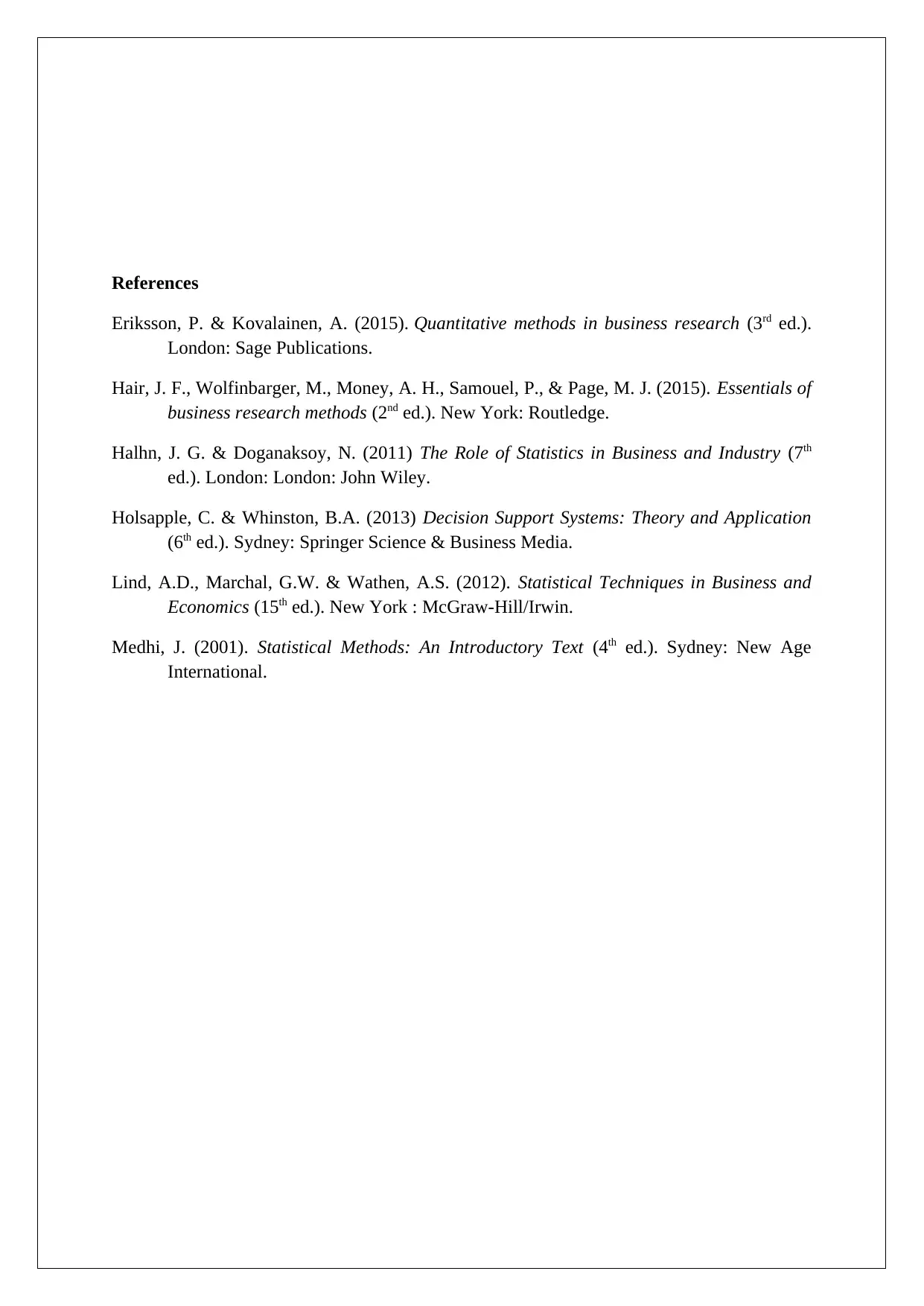
References
Eriksson, P. & Kovalainen, A. (2015). Quantitative methods in business research (3rd ed.).
London: Sage Publications.
Hair, J. F., Wolfinbarger, M., Money, A. H., Samouel, P., & Page, M. J. (2015). Essentials of
business research methods (2nd ed.). New York: Routledge.
Halhn, J. G. & Doganaksoy, N. (2011) The Role of Statistics in Business and Industry (7th
ed.). London: London: John Wiley.
Holsapple, C. & Whinston, B.A. (2013) Decision Support Systems: Theory and Application
(6th ed.). Sydney: Springer Science & Business Media.
Lind, A.D., Marchal, G.W. & Wathen, A.S. (2012). Statistical Techniques in Business and
Economics (15th ed.). New York : McGraw-Hill/Irwin.
Medhi, J. (2001). Statistical Methods: An Introductory Text (4th ed.). Sydney: New Age
International.
Eriksson, P. & Kovalainen, A. (2015). Quantitative methods in business research (3rd ed.).
London: Sage Publications.
Hair, J. F., Wolfinbarger, M., Money, A. H., Samouel, P., & Page, M. J. (2015). Essentials of
business research methods (2nd ed.). New York: Routledge.
Halhn, J. G. & Doganaksoy, N. (2011) The Role of Statistics in Business and Industry (7th
ed.). London: London: John Wiley.
Holsapple, C. & Whinston, B.A. (2013) Decision Support Systems: Theory and Application
(6th ed.). Sydney: Springer Science & Business Media.
Lind, A.D., Marchal, G.W. & Wathen, A.S. (2012). Statistical Techniques in Business and
Economics (15th ed.). New York : McGraw-Hill/Irwin.
Medhi, J. (2001). Statistical Methods: An Introductory Text (4th ed.). Sydney: New Age
International.
1 out of 15
Related Documents
![[object Object]](/_next/image/?url=%2F_next%2Fstatic%2Fmedia%2Flogo.6d15ce61.png&w=640&q=75)
Your All-in-One AI-Powered Toolkit for Academic Success.
+13062052269
info@desklib.com
Available 24*7 on WhatsApp / Email
Unlock your academic potential
© 2024 | Zucol Services PVT LTD | All rights reserved.