Environmental Management Assignment
VerifiedAdded on 2020/05/01
|17
|4300
|57
AI Summary
Contribute Materials
Your contribution can guide someone’s learning journey. Share your
documents today.
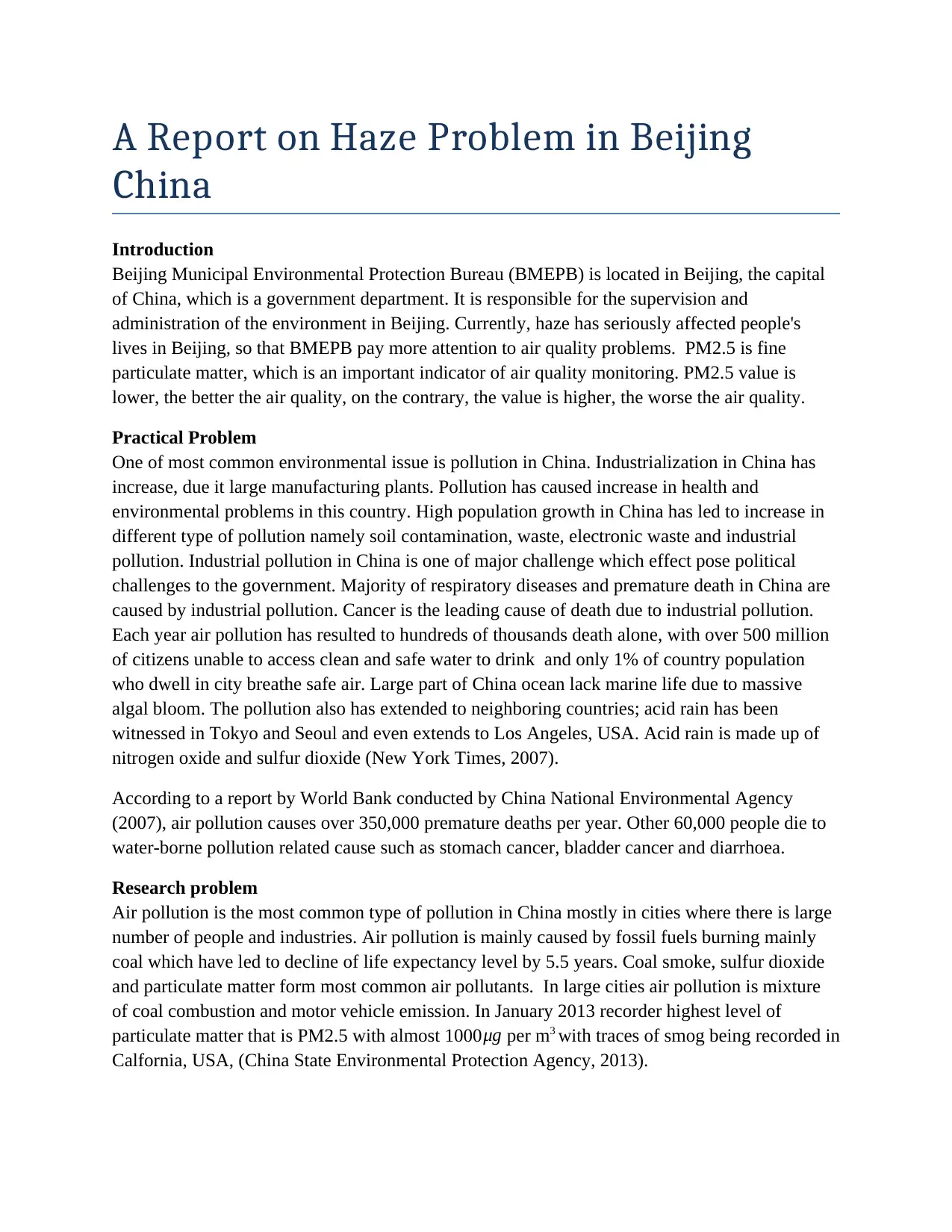
A Report on Haze Problem in Beijing
China
Introduction
Beijing Municipal Environmental Protection Bureau (BMEPB) is located in Beijing, the capital
of China, which is a government department. It is responsible for the supervision and
administration of the environment in Beijing. Currently, haze has seriously affected people's
lives in Beijing, so that BMEPB pay more attention to air quality problems. PM2.5 is fine
particulate matter, which is an important indicator of air quality monitoring. PM2.5 value is
lower, the better the air quality, on the contrary, the value is higher, the worse the air quality.
Practical Problem
One of most common environmental issue is pollution in China. Industrialization in China has
increase, due it large manufacturing plants. Pollution has caused increase in health and
environmental problems in this country. High population growth in China has led to increase in
different type of pollution namely soil contamination, waste, electronic waste and industrial
pollution. Industrial pollution in China is one of major challenge which effect pose political
challenges to the government. Majority of respiratory diseases and premature death in China are
caused by industrial pollution. Cancer is the leading cause of death due to industrial pollution.
Each year air pollution has resulted to hundreds of thousands death alone, with over 500 million
of citizens unable to access clean and safe water to drink and only 1% of country population
who dwell in city breathe safe air. Large part of China ocean lack marine life due to massive
algal bloom. The pollution also has extended to neighboring countries; acid rain has been
witnessed in Tokyo and Seoul and even extends to Los Angeles, USA. Acid rain is made up of
nitrogen oxide and sulfur dioxide (New York Times, 2007).
According to a report by World Bank conducted by China National Environmental Agency
(2007), air pollution causes over 350,000 premature deaths per year. Other 60,000 people die to
water-borne pollution related cause such as stomach cancer, bladder cancer and diarrhoea.
Research problem
Air pollution is the most common type of pollution in China mostly in cities where there is large
number of people and industries. Air pollution is mainly caused by fossil fuels burning mainly
coal which have led to decline of life expectancy level by 5.5 years. Coal smoke, sulfur dioxide
and particulate matter form most common air pollutants. In large cities air pollution is mixture
of coal combustion and motor vehicle emission. In January 2013 recorder highest level of
particulate matter that is PM2.5 with almost 1000 μg per m3 with traces of smog being recorded in
Calfornia, USA, (China State Environmental Protection Agency, 2013).
China
Introduction
Beijing Municipal Environmental Protection Bureau (BMEPB) is located in Beijing, the capital
of China, which is a government department. It is responsible for the supervision and
administration of the environment in Beijing. Currently, haze has seriously affected people's
lives in Beijing, so that BMEPB pay more attention to air quality problems. PM2.5 is fine
particulate matter, which is an important indicator of air quality monitoring. PM2.5 value is
lower, the better the air quality, on the contrary, the value is higher, the worse the air quality.
Practical Problem
One of most common environmental issue is pollution in China. Industrialization in China has
increase, due it large manufacturing plants. Pollution has caused increase in health and
environmental problems in this country. High population growth in China has led to increase in
different type of pollution namely soil contamination, waste, electronic waste and industrial
pollution. Industrial pollution in China is one of major challenge which effect pose political
challenges to the government. Majority of respiratory diseases and premature death in China are
caused by industrial pollution. Cancer is the leading cause of death due to industrial pollution.
Each year air pollution has resulted to hundreds of thousands death alone, with over 500 million
of citizens unable to access clean and safe water to drink and only 1% of country population
who dwell in city breathe safe air. Large part of China ocean lack marine life due to massive
algal bloom. The pollution also has extended to neighboring countries; acid rain has been
witnessed in Tokyo and Seoul and even extends to Los Angeles, USA. Acid rain is made up of
nitrogen oxide and sulfur dioxide (New York Times, 2007).
According to a report by World Bank conducted by China National Environmental Agency
(2007), air pollution causes over 350,000 premature deaths per year. Other 60,000 people die to
water-borne pollution related cause such as stomach cancer, bladder cancer and diarrhoea.
Research problem
Air pollution is the most common type of pollution in China mostly in cities where there is large
number of people and industries. Air pollution is mainly caused by fossil fuels burning mainly
coal which have led to decline of life expectancy level by 5.5 years. Coal smoke, sulfur dioxide
and particulate matter form most common air pollutants. In large cities air pollution is mixture
of coal combustion and motor vehicle emission. In January 2013 recorder highest level of
particulate matter that is PM2.5 with almost 1000 μg per m3 with traces of smog being recorded in
Calfornia, USA, (China State Environmental Protection Agency, 2013).
Secure Best Marks with AI Grader
Need help grading? Try our AI Grader for instant feedback on your assignments.
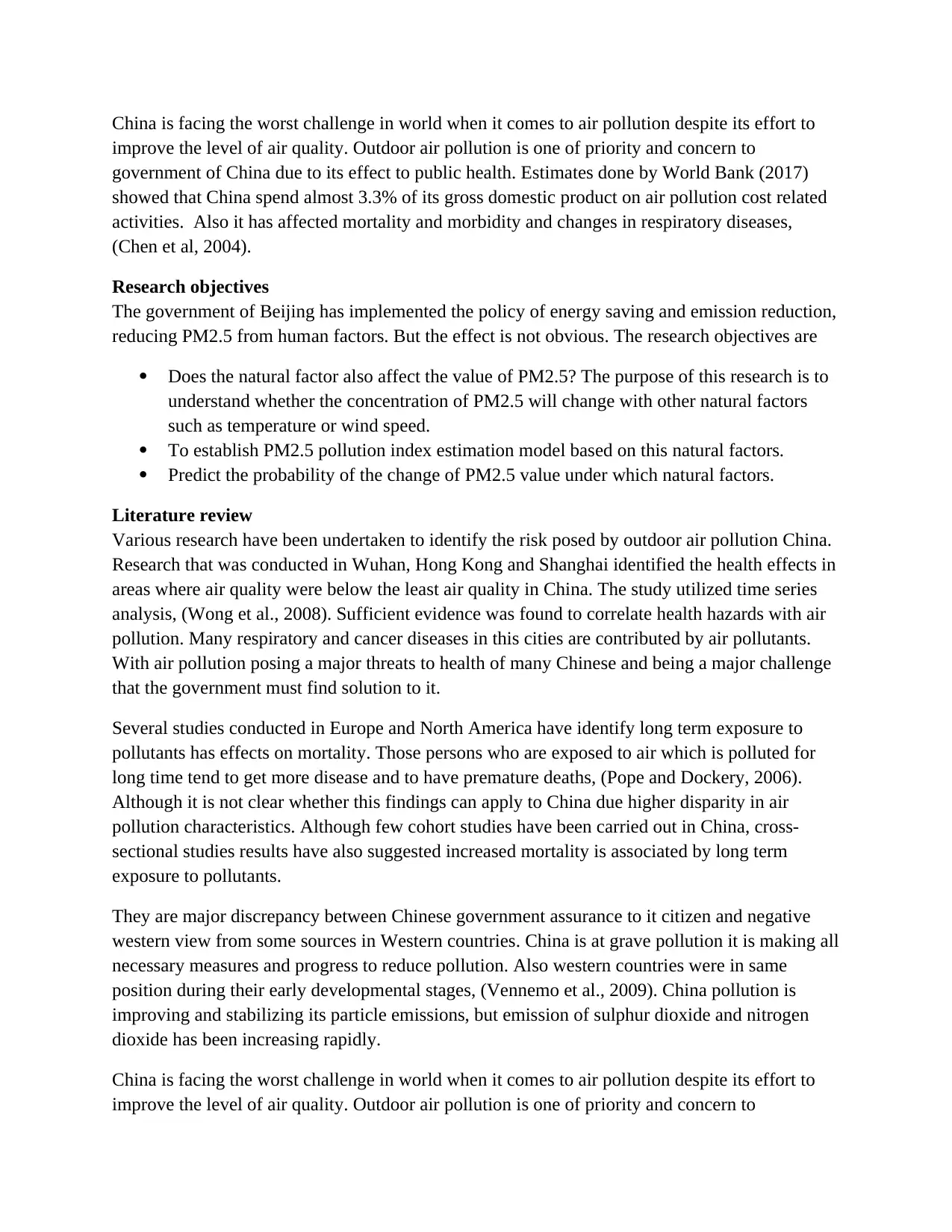
China is facing the worst challenge in world when it comes to air pollution despite its effort to
improve the level of air quality. Outdoor air pollution is one of priority and concern to
government of China due to its effect to public health. Estimates done by World Bank (2017)
showed that China spend almost 3.3% of its gross domestic product on air pollution cost related
activities. Also it has affected mortality and morbidity and changes in respiratory diseases,
(Chen et al, 2004).
Research objectives
The government of Beijing has implemented the policy of energy saving and emission reduction,
reducing PM2.5 from human factors. But the effect is not obvious. The research objectives are
Does the natural factor also affect the value of PM2.5? The purpose of this research is to
understand whether the concentration of PM2.5 will change with other natural factors
such as temperature or wind speed.
To establish PM2.5 pollution index estimation model based on this natural factors.
Predict the probability of the change of PM2.5 value under which natural factors.
Literature review
Various research have been undertaken to identify the risk posed by outdoor air pollution China.
Research that was conducted in Wuhan, Hong Kong and Shanghai identified the health effects in
areas where air quality were below the least air quality in China. The study utilized time series
analysis, (Wong et al., 2008). Sufficient evidence was found to correlate health hazards with air
pollution. Many respiratory and cancer diseases in this cities are contributed by air pollutants.
With air pollution posing a major threats to health of many Chinese and being a major challenge
that the government must find solution to it.
Several studies conducted in Europe and North America have identify long term exposure to
pollutants has effects on mortality. Those persons who are exposed to air which is polluted for
long time tend to get more disease and to have premature deaths, (Pope and Dockery, 2006).
Although it is not clear whether this findings can apply to China due higher disparity in air
pollution characteristics. Although few cohort studies have been carried out in China, cross-
sectional studies results have also suggested increased mortality is associated by long term
exposure to pollutants.
They are major discrepancy between Chinese government assurance to it citizen and negative
western view from some sources in Western countries. China is at grave pollution it is making all
necessary measures and progress to reduce pollution. Also western countries were in same
position during their early developmental stages, (Vennemo et al., 2009). China pollution is
improving and stabilizing its particle emissions, but emission of sulphur dioxide and nitrogen
dioxide has been increasing rapidly.
China is facing the worst challenge in world when it comes to air pollution despite its effort to
improve the level of air quality. Outdoor air pollution is one of priority and concern to
improve the level of air quality. Outdoor air pollution is one of priority and concern to
government of China due to its effect to public health. Estimates done by World Bank (2017)
showed that China spend almost 3.3% of its gross domestic product on air pollution cost related
activities. Also it has affected mortality and morbidity and changes in respiratory diseases,
(Chen et al, 2004).
Research objectives
The government of Beijing has implemented the policy of energy saving and emission reduction,
reducing PM2.5 from human factors. But the effect is not obvious. The research objectives are
Does the natural factor also affect the value of PM2.5? The purpose of this research is to
understand whether the concentration of PM2.5 will change with other natural factors
such as temperature or wind speed.
To establish PM2.5 pollution index estimation model based on this natural factors.
Predict the probability of the change of PM2.5 value under which natural factors.
Literature review
Various research have been undertaken to identify the risk posed by outdoor air pollution China.
Research that was conducted in Wuhan, Hong Kong and Shanghai identified the health effects in
areas where air quality were below the least air quality in China. The study utilized time series
analysis, (Wong et al., 2008). Sufficient evidence was found to correlate health hazards with air
pollution. Many respiratory and cancer diseases in this cities are contributed by air pollutants.
With air pollution posing a major threats to health of many Chinese and being a major challenge
that the government must find solution to it.
Several studies conducted in Europe and North America have identify long term exposure to
pollutants has effects on mortality. Those persons who are exposed to air which is polluted for
long time tend to get more disease and to have premature deaths, (Pope and Dockery, 2006).
Although it is not clear whether this findings can apply to China due higher disparity in air
pollution characteristics. Although few cohort studies have been carried out in China, cross-
sectional studies results have also suggested increased mortality is associated by long term
exposure to pollutants.
They are major discrepancy between Chinese government assurance to it citizen and negative
western view from some sources in Western countries. China is at grave pollution it is making all
necessary measures and progress to reduce pollution. Also western countries were in same
position during their early developmental stages, (Vennemo et al., 2009). China pollution is
improving and stabilizing its particle emissions, but emission of sulphur dioxide and nitrogen
dioxide has been increasing rapidly.
China is facing the worst challenge in world when it comes to air pollution despite its effort to
improve the level of air quality. Outdoor air pollution is one of priority and concern to
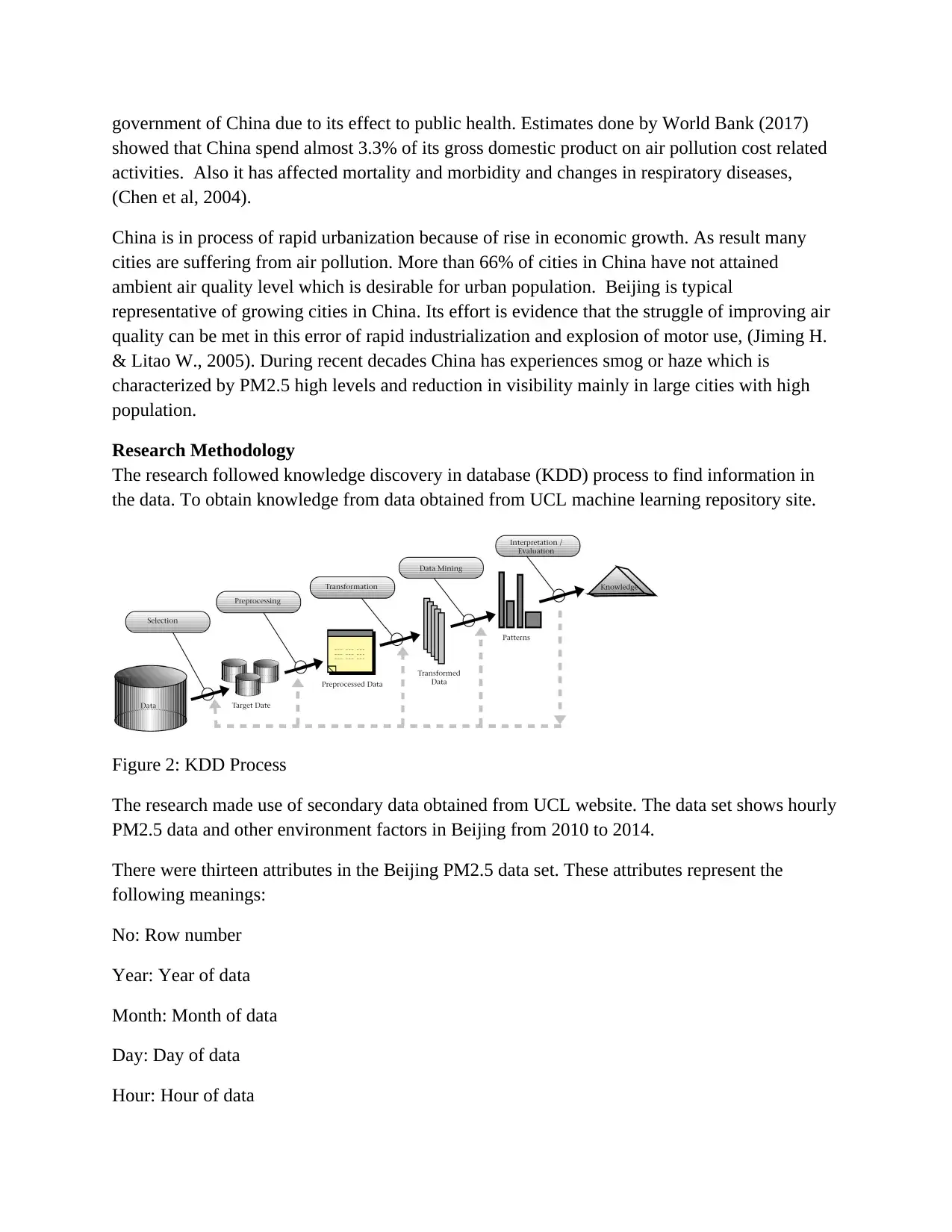
government of China due to its effect to public health. Estimates done by World Bank (2017)
showed that China spend almost 3.3% of its gross domestic product on air pollution cost related
activities. Also it has affected mortality and morbidity and changes in respiratory diseases,
(Chen et al, 2004).
China is in process of rapid urbanization because of rise in economic growth. As result many
cities are suffering from air pollution. More than 66% of cities in China have not attained
ambient air quality level which is desirable for urban population. Beijing is typical
representative of growing cities in China. Its effort is evidence that the struggle of improving air
quality can be met in this error of rapid industrialization and explosion of motor use, (Jiming H.
& Litao W., 2005). During recent decades China has experiences smog or haze which is
characterized by PM2.5 high levels and reduction in visibility mainly in large cities with high
population.
Research Methodology
The research followed knowledge discovery in database (KDD) process to find information in
the data. To obtain knowledge from data obtained from UCL machine learning repository site.
Figure 2: KDD Process
The research made use of secondary data obtained from UCL website. The data set shows hourly
PM2.5 data and other environment factors in Beijing from 2010 to 2014.
There were thirteen attributes in the Beijing PM2.5 data set. These attributes represent the
following meanings:
No: Row number
Year: Year of data
Month: Month of data
Day: Day of data
Hour: Hour of data
showed that China spend almost 3.3% of its gross domestic product on air pollution cost related
activities. Also it has affected mortality and morbidity and changes in respiratory diseases,
(Chen et al, 2004).
China is in process of rapid urbanization because of rise in economic growth. As result many
cities are suffering from air pollution. More than 66% of cities in China have not attained
ambient air quality level which is desirable for urban population. Beijing is typical
representative of growing cities in China. Its effort is evidence that the struggle of improving air
quality can be met in this error of rapid industrialization and explosion of motor use, (Jiming H.
& Litao W., 2005). During recent decades China has experiences smog or haze which is
characterized by PM2.5 high levels and reduction in visibility mainly in large cities with high
population.
Research Methodology
The research followed knowledge discovery in database (KDD) process to find information in
the data. To obtain knowledge from data obtained from UCL machine learning repository site.
Figure 2: KDD Process
The research made use of secondary data obtained from UCL website. The data set shows hourly
PM2.5 data and other environment factors in Beijing from 2010 to 2014.
There were thirteen attributes in the Beijing PM2.5 data set. These attributes represent the
following meanings:
No: Row number
Year: Year of data
Month: Month of data
Day: Day of data
Hour: Hour of data
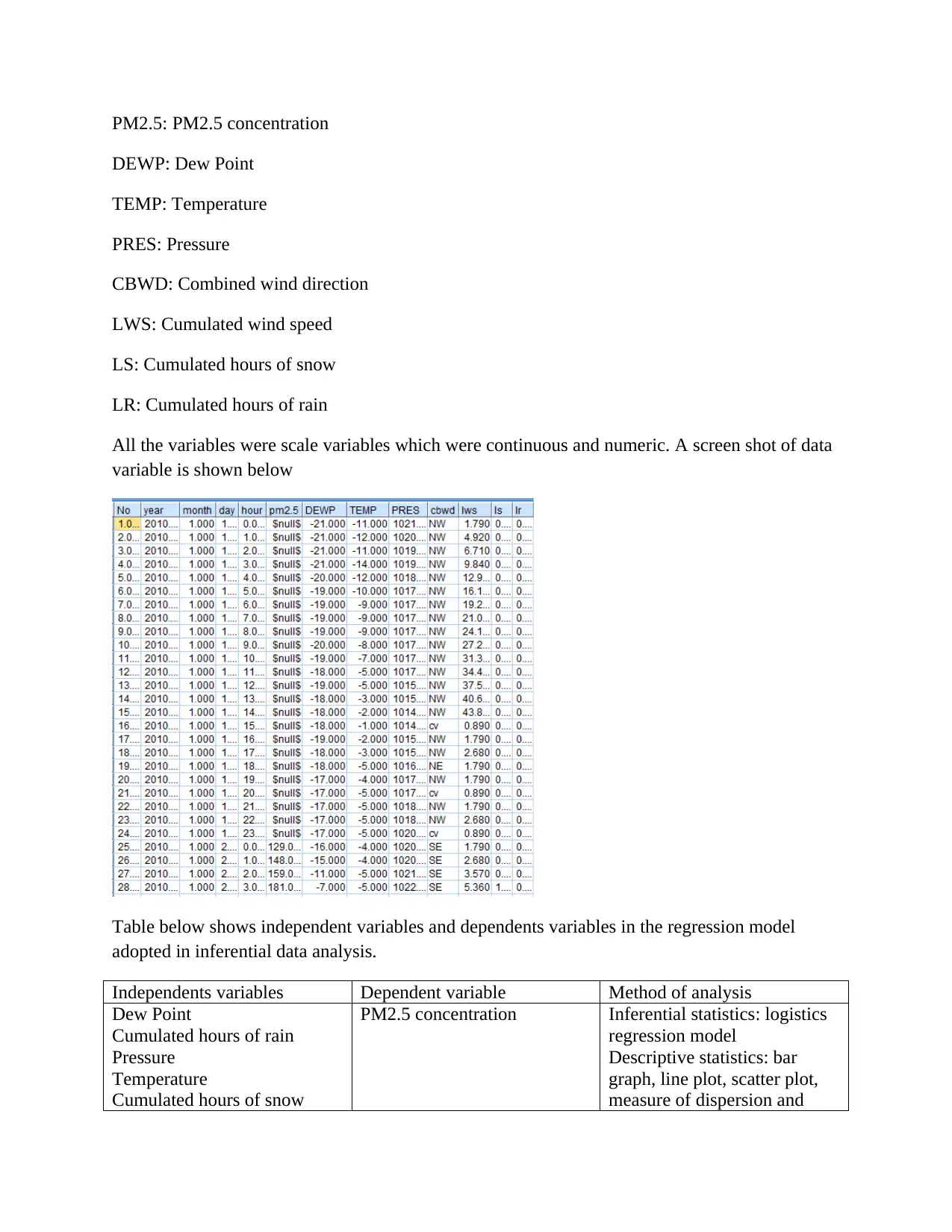
PM2.5: PM2.5 concentration
DEWP: Dew Point
TEMP: Temperature
PRES: Pressure
CBWD: Combined wind direction
LWS: Cumulated wind speed
LS: Cumulated hours of snow
LR: Cumulated hours of rain
All the variables were scale variables which were continuous and numeric. A screen shot of data
variable is shown below
Table below shows independent variables and dependents variables in the regression model
adopted in inferential data analysis.
Independents variables Dependent variable Method of analysis
Dew Point
Cumulated hours of rain
Pressure
Temperature
Cumulated hours of snow
PM2.5 concentration Inferential statistics: logistics
regression model
Descriptive statistics: bar
graph, line plot, scatter plot,
measure of dispersion and
DEWP: Dew Point
TEMP: Temperature
PRES: Pressure
CBWD: Combined wind direction
LWS: Cumulated wind speed
LS: Cumulated hours of snow
LR: Cumulated hours of rain
All the variables were scale variables which were continuous and numeric. A screen shot of data
variable is shown below
Table below shows independent variables and dependents variables in the regression model
adopted in inferential data analysis.
Independents variables Dependent variable Method of analysis
Dew Point
Cumulated hours of rain
Pressure
Temperature
Cumulated hours of snow
PM2.5 concentration Inferential statistics: logistics
regression model
Descriptive statistics: bar
graph, line plot, scatter plot,
measure of dispersion and
Paraphrase This Document
Need a fresh take? Get an instant paraphrase of this document with our AI Paraphraser
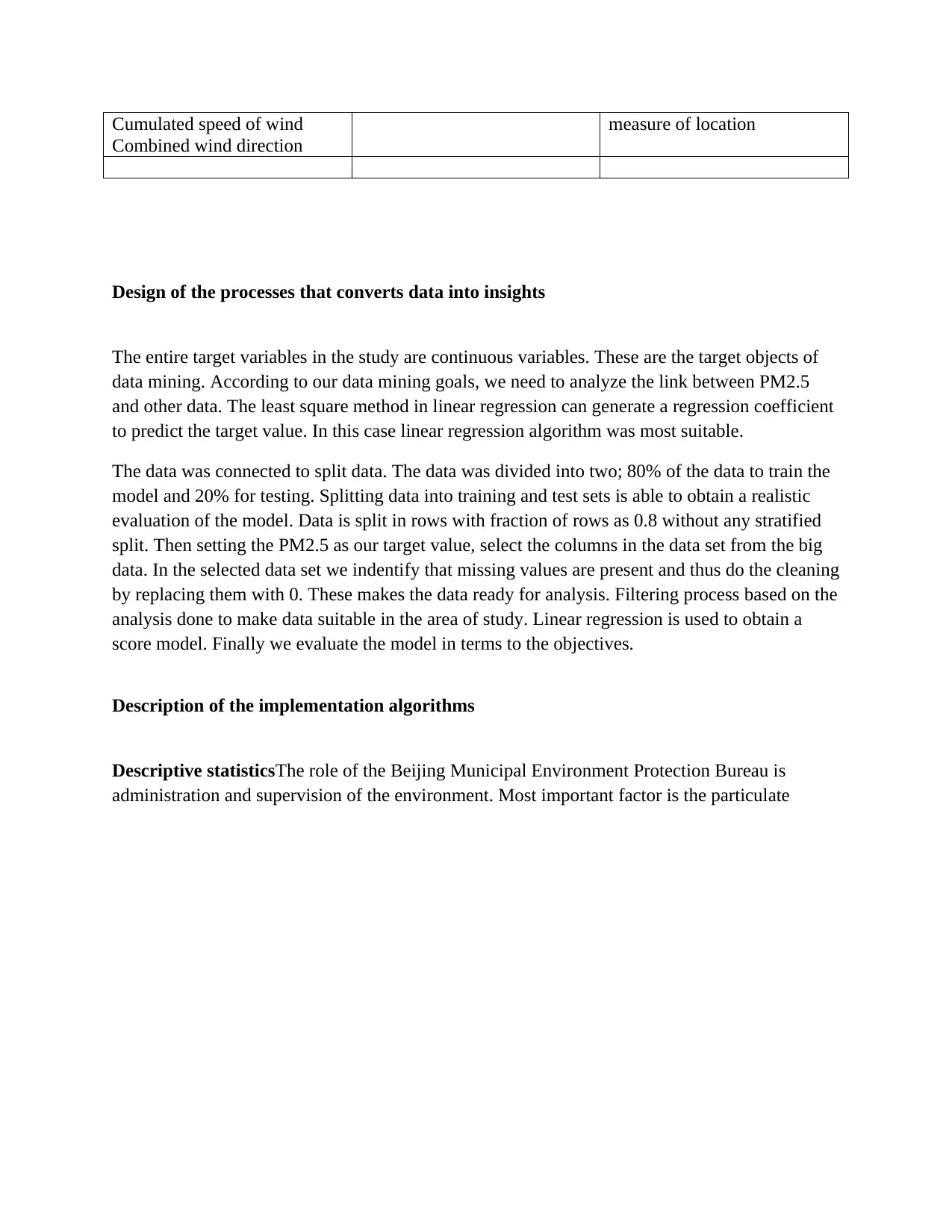
Cumulated speed of wind
Combined wind direction
measure of location
Design of the processes that converts data into insights
The entire target variables in the study are continuous variables. These are the target objects of
data mining. According to our data mining goals, we need to analyze the link between PM2.5
and other data. The least square method in linear regression can generate a regression coefficient
to predict the target value. In this case linear regression algorithm was most suitable.
The data was connected to split data. The data was divided into two; 80% of the data to train the
model and 20% for testing. Splitting data into training and test sets is able to obtain a realistic
evaluation of the model. Data is split in rows with fraction of rows as 0.8 without any stratified
split. Then setting the PM2.5 as our target value, select the columns in the data set from the big
data. In the selected data set we indentify that missing values are present and thus do the cleaning
by replacing them with 0. These makes the data ready for analysis. Filtering process based on the
analysis done to make data suitable in the area of study. Linear regression is used to obtain a
score model. Finally we evaluate the model in terms to the objectives.
Description of the implementation algorithms
Descriptive statisticsThe role of the Beijing Municipal Environment Protection Bureau is
administration and supervision of the environment. Most important factor is the particulate
Combined wind direction
measure of location
Design of the processes that converts data into insights
The entire target variables in the study are continuous variables. These are the target objects of
data mining. According to our data mining goals, we need to analyze the link between PM2.5
and other data. The least square method in linear regression can generate a regression coefficient
to predict the target value. In this case linear regression algorithm was most suitable.
The data was connected to split data. The data was divided into two; 80% of the data to train the
model and 20% for testing. Splitting data into training and test sets is able to obtain a realistic
evaluation of the model. Data is split in rows with fraction of rows as 0.8 without any stratified
split. Then setting the PM2.5 as our target value, select the columns in the data set from the big
data. In the selected data set we indentify that missing values are present and thus do the cleaning
by replacing them with 0. These makes the data ready for analysis. Filtering process based on the
analysis done to make data suitable in the area of study. Linear regression is used to obtain a
score model. Finally we evaluate the model in terms to the objectives.
Description of the implementation algorithms
Descriptive statisticsThe role of the Beijing Municipal Environment Protection Bureau is
administration and supervision of the environment. Most important factor is the particulate
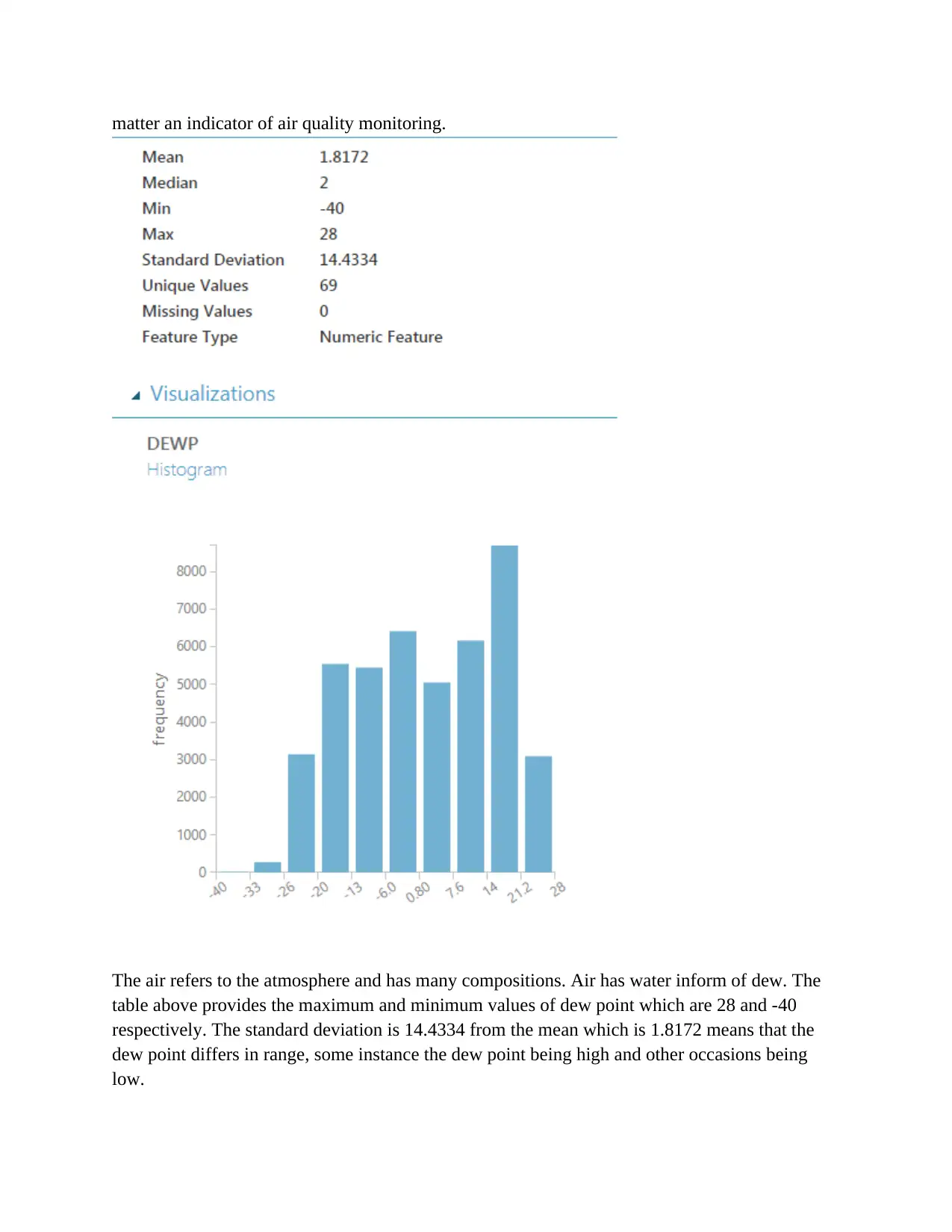
matter an indicator of air quality monitoring.
The air refers to the atmosphere and has many compositions. Air has water inform of dew. The
table above provides the maximum and minimum values of dew point which are 28 and -40
respectively. The standard deviation is 14.4334 from the mean which is 1.8172 means that the
dew point differs in range, some instance the dew point being high and other occasions being
low.
The air refers to the atmosphere and has many compositions. Air has water inform of dew. The
table above provides the maximum and minimum values of dew point which are 28 and -40
respectively. The standard deviation is 14.4334 from the mean which is 1.8172 means that the
dew point differs in range, some instance the dew point being high and other occasions being
low.
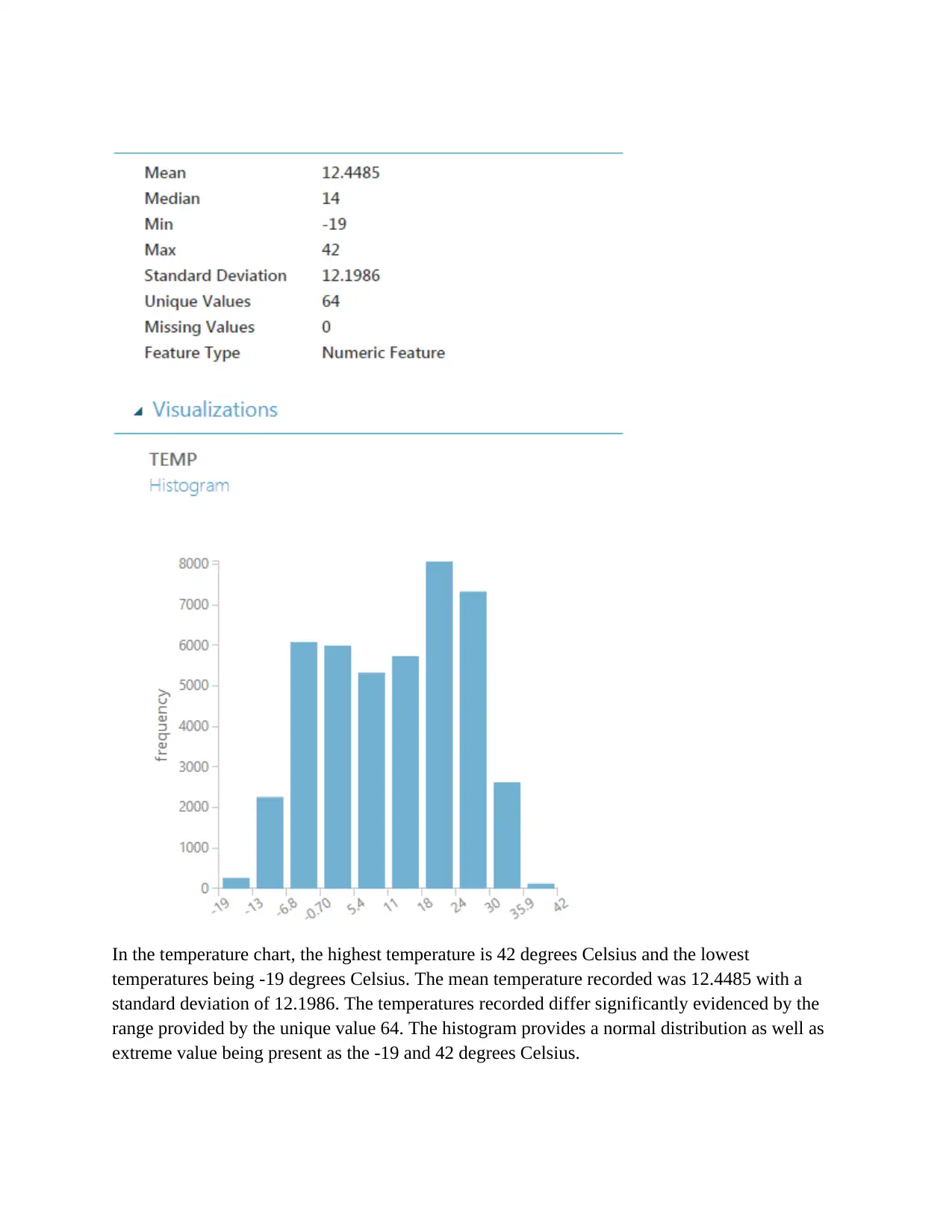
In the temperature chart, the highest temperature is 42 degrees Celsius and the lowest
temperatures being -19 degrees Celsius. The mean temperature recorded was 12.4485 with a
standard deviation of 12.1986. The temperatures recorded differ significantly evidenced by the
range provided by the unique value 64. The histogram provides a normal distribution as well as
extreme value being present as the -19 and 42 degrees Celsius.
temperatures being -19 degrees Celsius. The mean temperature recorded was 12.4485 with a
standard deviation of 12.1986. The temperatures recorded differ significantly evidenced by the
range provided by the unique value 64. The histogram provides a normal distribution as well as
extreme value being present as the -19 and 42 degrees Celsius.
Secure Best Marks with AI Grader
Need help grading? Try our AI Grader for instant feedback on your assignments.
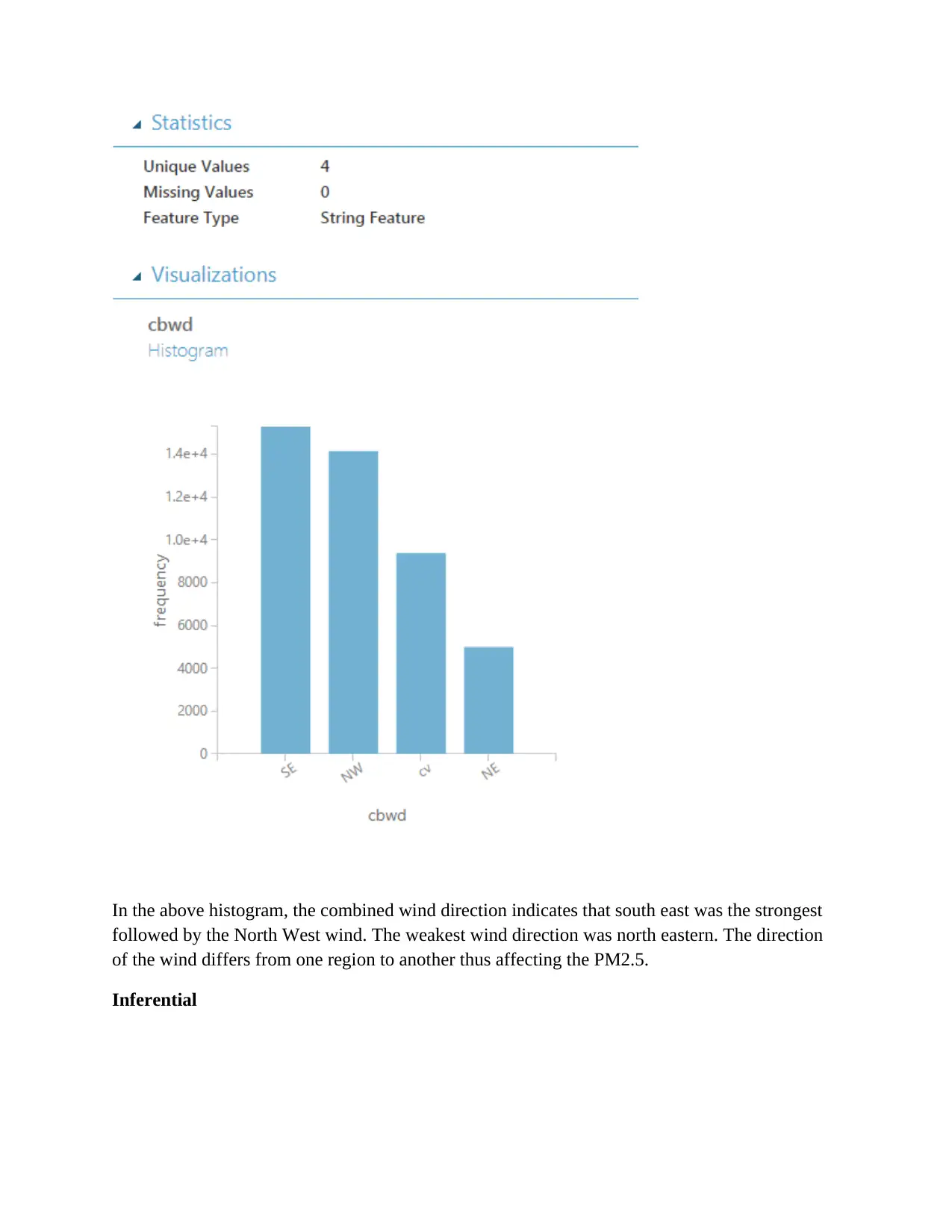
In the above histogram, the combined wind direction indicates that south east was the strongest
followed by the North West wind. The weakest wind direction was north eastern. The direction
of the wind differs from one region to another thus affecting the PM2.5.
Inferential
followed by the North West wind. The weakest wind direction was north eastern. The direction
of the wind differs from one region to another thus affecting the PM2.5.
Inferential
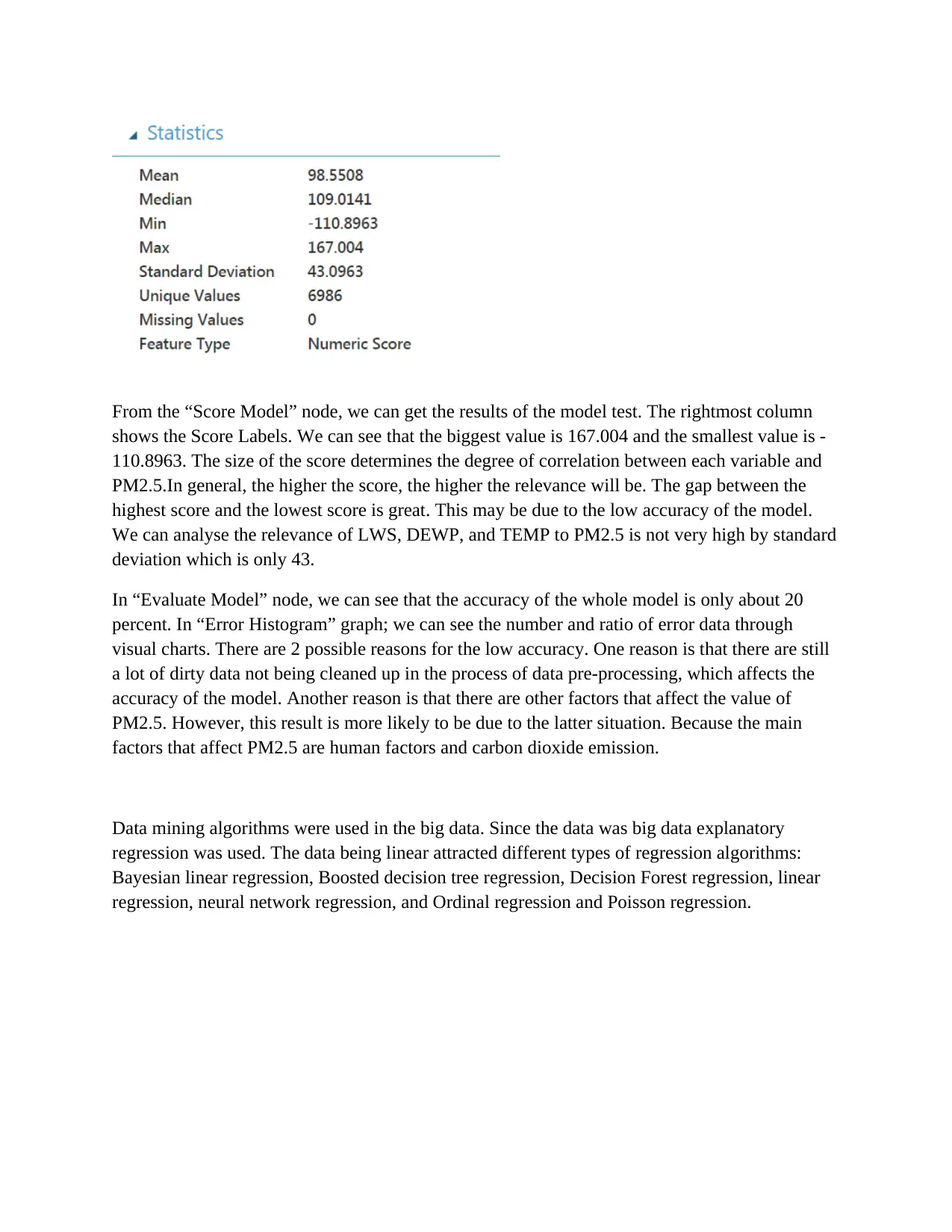
From the “Score Model” node, we can get the results of the model test. The rightmost column
shows the Score Labels. We can see that the biggest value is 167.004 and the smallest value is -
110.8963. The size of the score determines the degree of correlation between each variable and
PM2.5.In general, the higher the score, the higher the relevance will be. The gap between the
highest score and the lowest score is great. This may be due to the low accuracy of the model.
We can analyse the relevance of LWS, DEWP, and TEMP to PM2.5 is not very high by standard
deviation which is only 43.
In “Evaluate Model” node, we can see that the accuracy of the whole model is only about 20
percent. In “Error Histogram” graph; we can see the number and ratio of error data through
visual charts. There are 2 possible reasons for the low accuracy. One reason is that there are still
a lot of dirty data not being cleaned up in the process of data pre-processing, which affects the
accuracy of the model. Another reason is that there are other factors that affect the value of
PM2.5. However, this result is more likely to be due to the latter situation. Because the main
factors that affect PM2.5 are human factors and carbon dioxide emission.
Data mining algorithms were used in the big data. Since the data was big data explanatory
regression was used. The data being linear attracted different types of regression algorithms:
Bayesian linear regression, Boosted decision tree regression, Decision Forest regression, linear
regression, neural network regression, and Ordinal regression and Poisson regression.
shows the Score Labels. We can see that the biggest value is 167.004 and the smallest value is -
110.8963. The size of the score determines the degree of correlation between each variable and
PM2.5.In general, the higher the score, the higher the relevance will be. The gap between the
highest score and the lowest score is great. This may be due to the low accuracy of the model.
We can analyse the relevance of LWS, DEWP, and TEMP to PM2.5 is not very high by standard
deviation which is only 43.
In “Evaluate Model” node, we can see that the accuracy of the whole model is only about 20
percent. In “Error Histogram” graph; we can see the number and ratio of error data through
visual charts. There are 2 possible reasons for the low accuracy. One reason is that there are still
a lot of dirty data not being cleaned up in the process of data pre-processing, which affects the
accuracy of the model. Another reason is that there are other factors that affect the value of
PM2.5. However, this result is more likely to be due to the latter situation. Because the main
factors that affect PM2.5 are human factors and carbon dioxide emission.
Data mining algorithms were used in the big data. Since the data was big data explanatory
regression was used. The data being linear attracted different types of regression algorithms:
Bayesian linear regression, Boosted decision tree regression, Decision Forest regression, linear
regression, neural network regression, and Ordinal regression and Poisson regression.
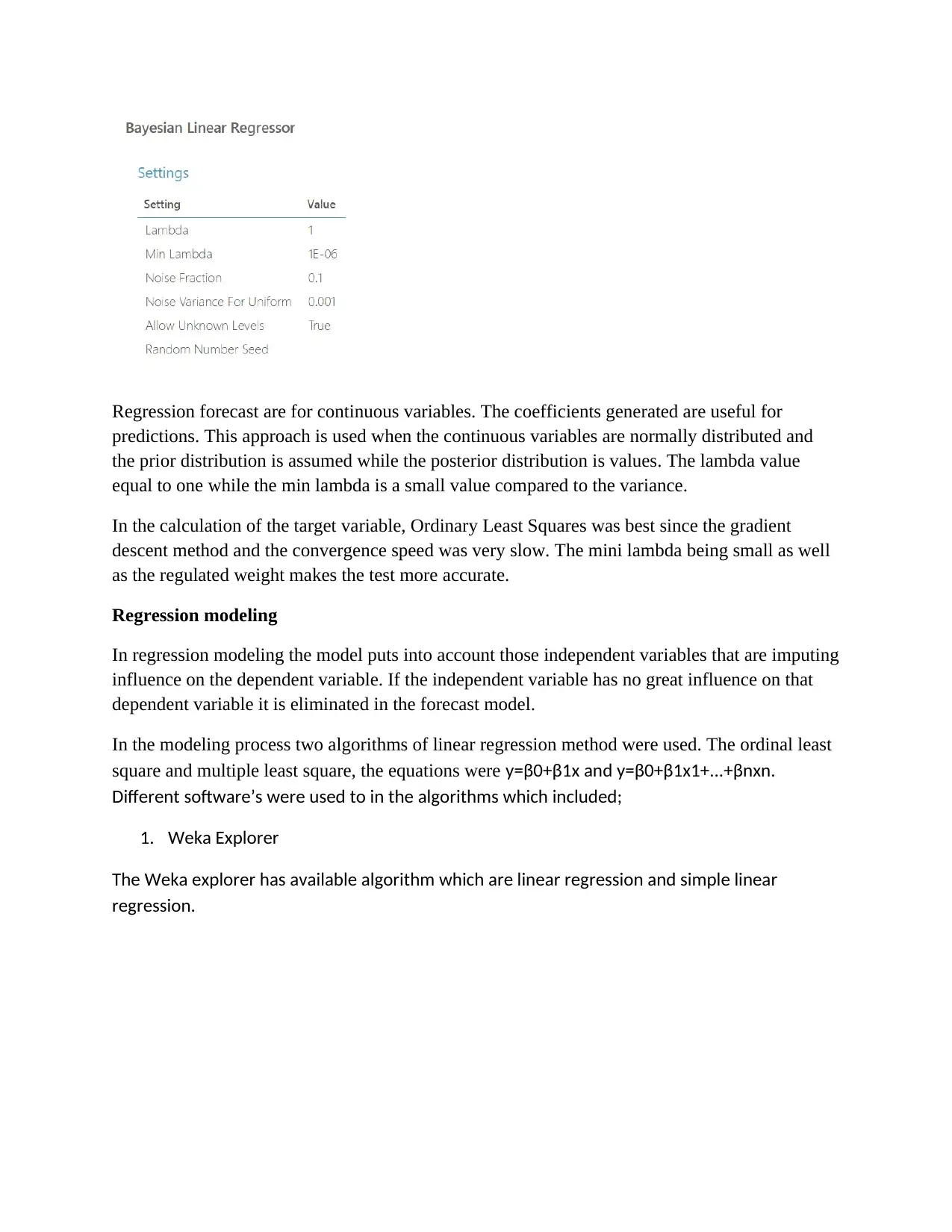
Regression forecast are for continuous variables. The coefficients generated are useful for
predictions. This approach is used when the continuous variables are normally distributed and
the prior distribution is assumed while the posterior distribution is values. The lambda value
equal to one while the min lambda is a small value compared to the variance.
In the calculation of the target variable, Ordinary Least Squares was best since the gradient
descent method and the convergence speed was very slow. The mini lambda being small as well
as the regulated weight makes the test more accurate.
Regression modeling
In regression modeling the model puts into account those independent variables that are imputing
influence on the dependent variable. If the independent variable has no great influence on that
dependent variable it is eliminated in the forecast model.
In the modeling process two algorithms of linear regression method were used. The ordinal least
square and multiple least square, the equations were y=β0+β1x and y=β0+β1x1+...+βnxn.
Different software’s were used to in the algorithms which included;
1. Weka Explorer
The Weka explorer has available algorithm which are linear regression and simple linear
regression.
predictions. This approach is used when the continuous variables are normally distributed and
the prior distribution is assumed while the posterior distribution is values. The lambda value
equal to one while the min lambda is a small value compared to the variance.
In the calculation of the target variable, Ordinary Least Squares was best since the gradient
descent method and the convergence speed was very slow. The mini lambda being small as well
as the regulated weight makes the test more accurate.
Regression modeling
In regression modeling the model puts into account those independent variables that are imputing
influence on the dependent variable. If the independent variable has no great influence on that
dependent variable it is eliminated in the forecast model.
In the modeling process two algorithms of linear regression method were used. The ordinal least
square and multiple least square, the equations were y=β0+β1x and y=β0+β1x1+...+βnxn.
Different software’s were used to in the algorithms which included;
1. Weka Explorer
The Weka explorer has available algorithm which are linear regression and simple linear
regression.
Paraphrase This Document
Need a fresh take? Get an instant paraphrase of this document with our AI Paraphraser
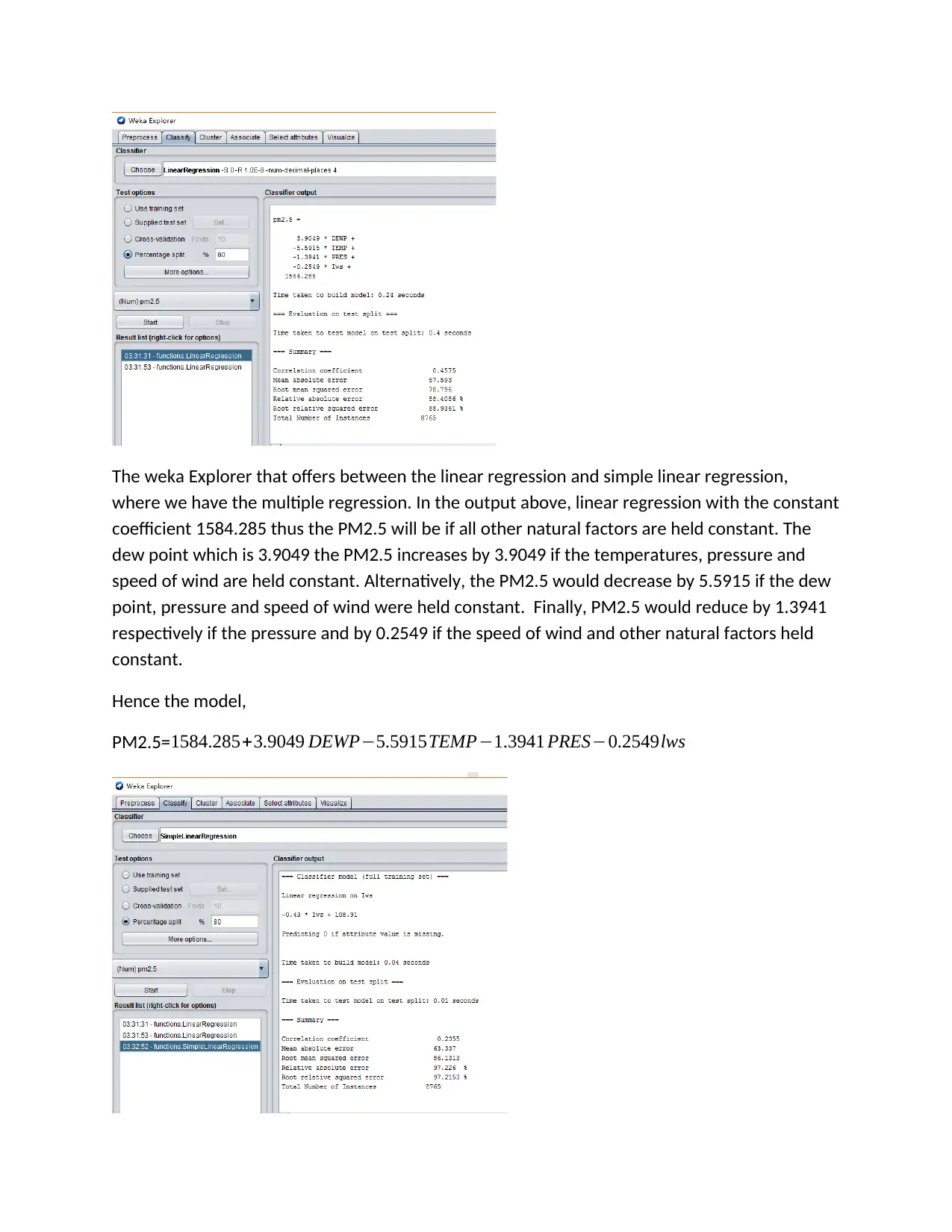
The weka Explorer that offers between the linear regression and simple linear regression,
where we have the multiple regression. In the output above, linear regression with the constant
coefficient 1584.285 thus the PM2.5 will be if all other natural factors are held constant. The
dew point which is 3.9049 the PM2.5 increases by 3.9049 if the temperatures, pressure and
speed of wind are held constant. Alternatively, the PM2.5 would decrease by 5.5915 if the dew
point, pressure and speed of wind were held constant. Finally, PM2.5 would reduce by 1.3941
respectively if the pressure and by 0.2549 if the speed of wind and other natural factors held
constant.
Hence the model,
PM2.5=1584.285+3.9049 DEWP−5.5915TEMP−1.3941 PRES−0.2549lws
where we have the multiple regression. In the output above, linear regression with the constant
coefficient 1584.285 thus the PM2.5 will be if all other natural factors are held constant. The
dew point which is 3.9049 the PM2.5 increases by 3.9049 if the temperatures, pressure and
speed of wind are held constant. Alternatively, the PM2.5 would decrease by 5.5915 if the dew
point, pressure and speed of wind were held constant. Finally, PM2.5 would reduce by 1.3941
respectively if the pressure and by 0.2549 if the speed of wind and other natural factors held
constant.
Hence the model,
PM2.5=1584.285+3.9049 DEWP−5.5915TEMP−1.3941 PRES−0.2549lws
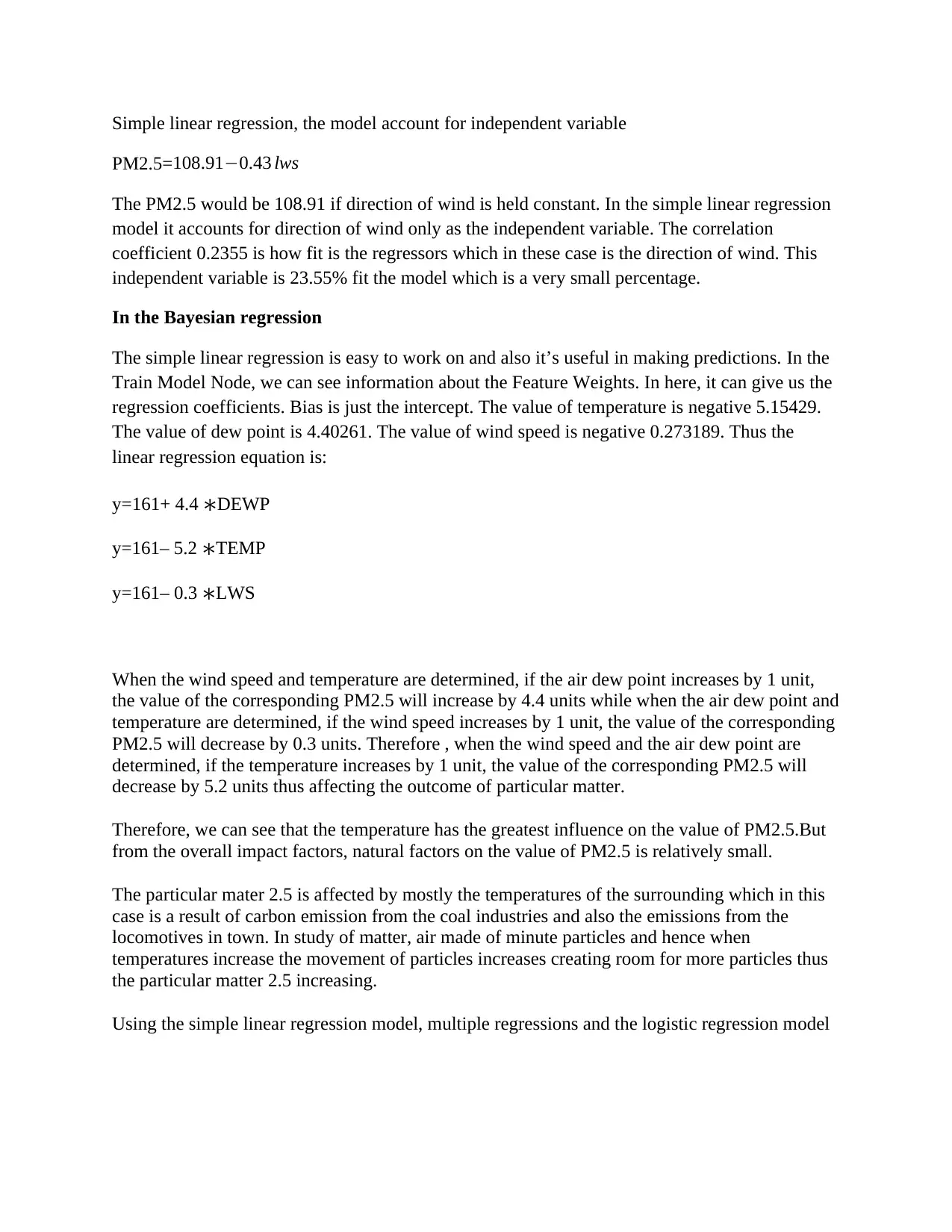
Simple linear regression, the model account for independent variable
PM2.5=108.91−0.43 lws
The PM2.5 would be 108.91 if direction of wind is held constant. In the simple linear regression
model it accounts for direction of wind only as the independent variable. The correlation
coefficient 0.2355 is how fit is the regressors which in these case is the direction of wind. This
independent variable is 23.55% fit the model which is a very small percentage.
In the Bayesian regression
The simple linear regression is easy to work on and also it’s useful in making predictions. In the
Train Model Node, we can see information about the Feature Weights. In here, it can give us the
regression coefficients. Bias is just the intercept. The value of temperature is negative 5.15429.
The value of dew point is 4.40261. The value of wind speed is negative 0.273189. Thus the
linear regression equation is:
y=161+ 4.4 ∗DEWP
y=161– 5.2 ∗TEMP
y=161– 0.3 ∗LWS
When the wind speed and temperature are determined, if the air dew point increases by 1 unit,
the value of the corresponding PM2.5 will increase by 4.4 units while when the air dew point and
temperature are determined, if the wind speed increases by 1 unit, the value of the corresponding
PM2.5 will decrease by 0.3 units. Therefore , when the wind speed and the air dew point are
determined, if the temperature increases by 1 unit, the value of the corresponding PM2.5 will
decrease by 5.2 units thus affecting the outcome of particular matter.
Therefore, we can see that the temperature has the greatest influence on the value of PM2.5.But
from the overall impact factors, natural factors on the value of PM2.5 is relatively small.
The particular mater 2.5 is affected by mostly the temperatures of the surrounding which in this
case is a result of carbon emission from the coal industries and also the emissions from the
locomotives in town. In study of matter, air made of minute particles and hence when
temperatures increase the movement of particles increases creating room for more particles thus
the particular matter 2.5 increasing.
Using the simple linear regression model, multiple regressions and the logistic regression model
PM2.5=108.91−0.43 lws
The PM2.5 would be 108.91 if direction of wind is held constant. In the simple linear regression
model it accounts for direction of wind only as the independent variable. The correlation
coefficient 0.2355 is how fit is the regressors which in these case is the direction of wind. This
independent variable is 23.55% fit the model which is a very small percentage.
In the Bayesian regression
The simple linear regression is easy to work on and also it’s useful in making predictions. In the
Train Model Node, we can see information about the Feature Weights. In here, it can give us the
regression coefficients. Bias is just the intercept. The value of temperature is negative 5.15429.
The value of dew point is 4.40261. The value of wind speed is negative 0.273189. Thus the
linear regression equation is:
y=161+ 4.4 ∗DEWP
y=161– 5.2 ∗TEMP
y=161– 0.3 ∗LWS
When the wind speed and temperature are determined, if the air dew point increases by 1 unit,
the value of the corresponding PM2.5 will increase by 4.4 units while when the air dew point and
temperature are determined, if the wind speed increases by 1 unit, the value of the corresponding
PM2.5 will decrease by 0.3 units. Therefore , when the wind speed and the air dew point are
determined, if the temperature increases by 1 unit, the value of the corresponding PM2.5 will
decrease by 5.2 units thus affecting the outcome of particular matter.
Therefore, we can see that the temperature has the greatest influence on the value of PM2.5.But
from the overall impact factors, natural factors on the value of PM2.5 is relatively small.
The particular mater 2.5 is affected by mostly the temperatures of the surrounding which in this
case is a result of carbon emission from the coal industries and also the emissions from the
locomotives in town. In study of matter, air made of minute particles and hence when
temperatures increase the movement of particles increases creating room for more particles thus
the particular matter 2.5 increasing.
Using the simple linear regression model, multiple regressions and the logistic regression model
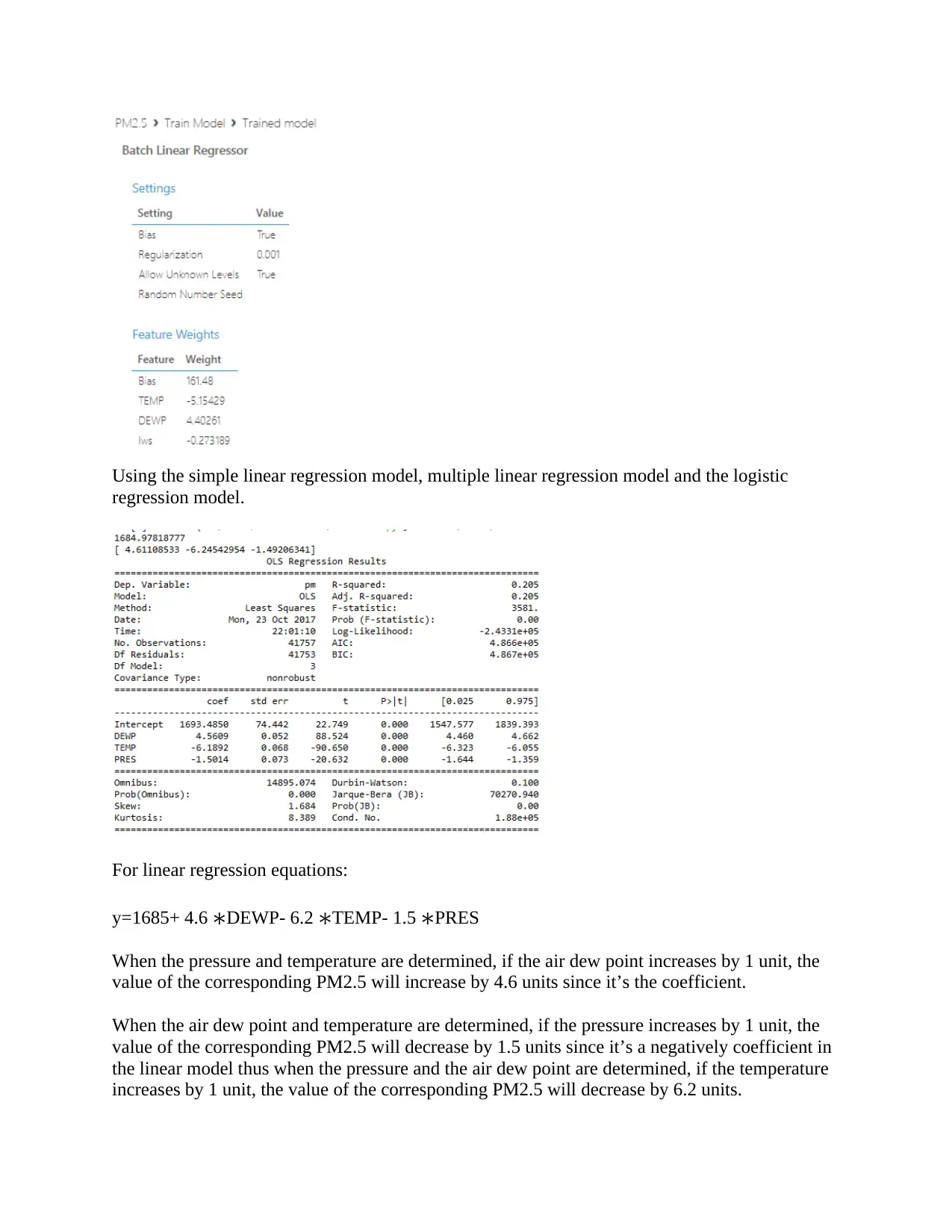
Using the simple linear regression model, multiple linear regression model and the logistic
regression model.
For linear regression equations:
y=1685+ 4.6 ∗DEWP- 6.2 ∗TEMP- 1.5 ∗PRES
When the pressure and temperature are determined, if the air dew point increases by 1 unit, the
value of the corresponding PM2.5 will increase by 4.6 units since it’s the coefficient.
When the air dew point and temperature are determined, if the pressure increases by 1 unit, the
value of the corresponding PM2.5 will decrease by 1.5 units since it’s a negatively coefficient in
the linear model thus when the pressure and the air dew point are determined, if the temperature
increases by 1 unit, the value of the corresponding PM2.5 will decrease by 6.2 units.
regression model.
For linear regression equations:
y=1685+ 4.6 ∗DEWP- 6.2 ∗TEMP- 1.5 ∗PRES
When the pressure and temperature are determined, if the air dew point increases by 1 unit, the
value of the corresponding PM2.5 will increase by 4.6 units since it’s the coefficient.
When the air dew point and temperature are determined, if the pressure increases by 1 unit, the
value of the corresponding PM2.5 will decrease by 1.5 units since it’s a negatively coefficient in
the linear model thus when the pressure and the air dew point are determined, if the temperature
increases by 1 unit, the value of the corresponding PM2.5 will decrease by 6.2 units.
Secure Best Marks with AI Grader
Need help grading? Try our AI Grader for instant feedback on your assignments.
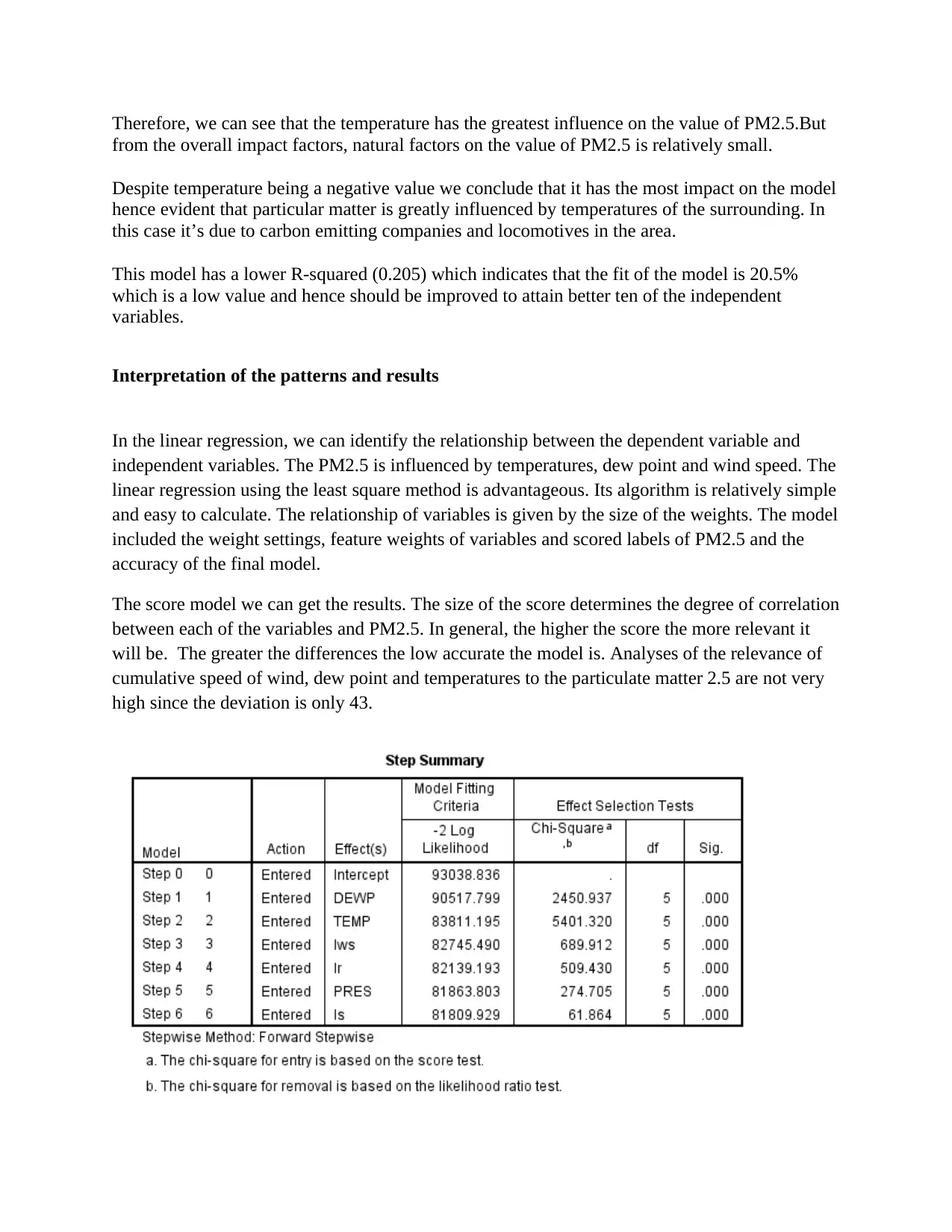
Therefore, we can see that the temperature has the greatest influence on the value of PM2.5.But
from the overall impact factors, natural factors on the value of PM2.5 is relatively small.
Despite temperature being a negative value we conclude that it has the most impact on the model
hence evident that particular matter is greatly influenced by temperatures of the surrounding. In
this case it’s due to carbon emitting companies and locomotives in the area.
This model has a lower R-squared (0.205) which indicates that the fit of the model is 20.5%
which is a low value and hence should be improved to attain better ten of the independent
variables.
Interpretation of the patterns and results
In the linear regression, we can identify the relationship between the dependent variable and
independent variables. The PM2.5 is influenced by temperatures, dew point and wind speed. The
linear regression using the least square method is advantageous. Its algorithm is relatively simple
and easy to calculate. The relationship of variables is given by the size of the weights. The model
included the weight settings, feature weights of variables and scored labels of PM2.5 and the
accuracy of the final model.
The score model we can get the results. The size of the score determines the degree of correlation
between each of the variables and PM2.5. In general, the higher the score the more relevant it
will be. The greater the differences the low accurate the model is. Analyses of the relevance of
cumulative speed of wind, dew point and temperatures to the particulate matter 2.5 are not very
high since the deviation is only 43.
from the overall impact factors, natural factors on the value of PM2.5 is relatively small.
Despite temperature being a negative value we conclude that it has the most impact on the model
hence evident that particular matter is greatly influenced by temperatures of the surrounding. In
this case it’s due to carbon emitting companies and locomotives in the area.
This model has a lower R-squared (0.205) which indicates that the fit of the model is 20.5%
which is a low value and hence should be improved to attain better ten of the independent
variables.
Interpretation of the patterns and results
In the linear regression, we can identify the relationship between the dependent variable and
independent variables. The PM2.5 is influenced by temperatures, dew point and wind speed. The
linear regression using the least square method is advantageous. Its algorithm is relatively simple
and easy to calculate. The relationship of variables is given by the size of the weights. The model
included the weight settings, feature weights of variables and scored labels of PM2.5 and the
accuracy of the final model.
The score model we can get the results. The size of the score determines the degree of correlation
between each of the variables and PM2.5. In general, the higher the score the more relevant it
will be. The greater the differences the low accurate the model is. Analyses of the relevance of
cumulative speed of wind, dew point and temperatures to the particulate matter 2.5 are not very
high since the deviation is only 43.
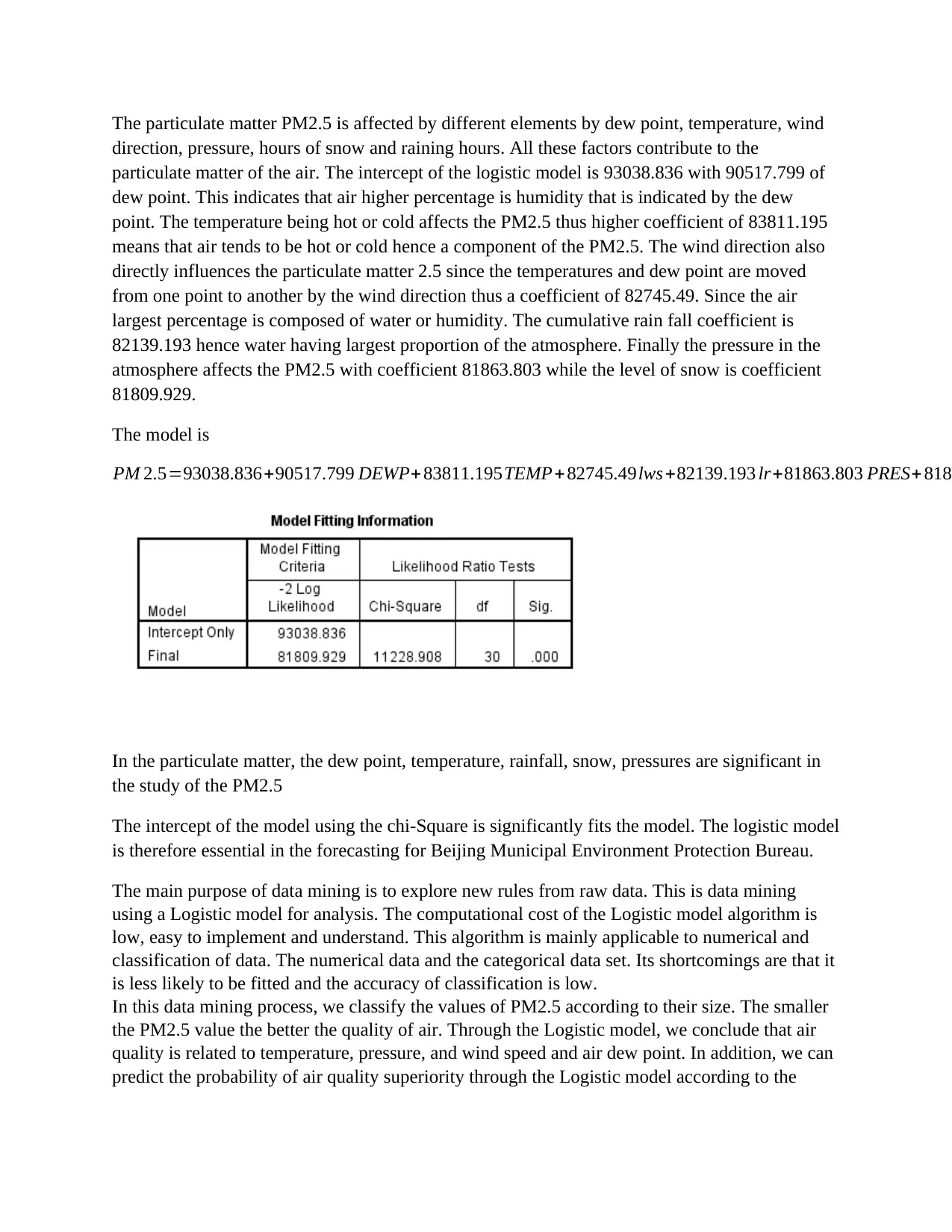
The particulate matter PM2.5 is affected by different elements by dew point, temperature, wind
direction, pressure, hours of snow and raining hours. All these factors contribute to the
particulate matter of the air. The intercept of the logistic model is 93038.836 with 90517.799 of
dew point. This indicates that air higher percentage is humidity that is indicated by the dew
point. The temperature being hot or cold affects the PM2.5 thus higher coefficient of 83811.195
means that air tends to be hot or cold hence a component of the PM2.5. The wind direction also
directly influences the particulate matter 2.5 since the temperatures and dew point are moved
from one point to another by the wind direction thus a coefficient of 82745.49. Since the air
largest percentage is composed of water or humidity. The cumulative rain fall coefficient is
82139.193 hence water having largest proportion of the atmosphere. Finally the pressure in the
atmosphere affects the PM2.5 with coefficient 81863.803 while the level of snow is coefficient
81809.929.
The model is
PM 2.5=93038.836+90517.799 DEWP+ 83811.195TEMP+ 82745.49lws+82139.193 lr+81863.803 PRES+8180
In the particulate matter, the dew point, temperature, rainfall, snow, pressures are significant in
the study of the PM2.5
The intercept of the model using the chi-Square is significantly fits the model. The logistic model
is therefore essential in the forecasting for Beijing Municipal Environment Protection Bureau.
The main purpose of data mining is to explore new rules from raw data. This is data mining
using a Logistic model for analysis. The computational cost of the Logistic model algorithm is
low, easy to implement and understand. This algorithm is mainly applicable to numerical and
classification of data. The numerical data and the categorical data set. Its shortcomings are that it
is less likely to be fitted and the accuracy of classification is low.
In this data mining process, we classify the values of PM2.5 according to their size. The smaller
the PM2.5 value the better the quality of air. Through the Logistic model, we conclude that air
quality is related to temperature, pressure, and wind speed and air dew point. In addition, we can
predict the probability of air quality superiority through the Logistic model according to the
direction, pressure, hours of snow and raining hours. All these factors contribute to the
particulate matter of the air. The intercept of the logistic model is 93038.836 with 90517.799 of
dew point. This indicates that air higher percentage is humidity that is indicated by the dew
point. The temperature being hot or cold affects the PM2.5 thus higher coefficient of 83811.195
means that air tends to be hot or cold hence a component of the PM2.5. The wind direction also
directly influences the particulate matter 2.5 since the temperatures and dew point are moved
from one point to another by the wind direction thus a coefficient of 82745.49. Since the air
largest percentage is composed of water or humidity. The cumulative rain fall coefficient is
82139.193 hence water having largest proportion of the atmosphere. Finally the pressure in the
atmosphere affects the PM2.5 with coefficient 81863.803 while the level of snow is coefficient
81809.929.
The model is
PM 2.5=93038.836+90517.799 DEWP+ 83811.195TEMP+ 82745.49lws+82139.193 lr+81863.803 PRES+8180
In the particulate matter, the dew point, temperature, rainfall, snow, pressures are significant in
the study of the PM2.5
The intercept of the model using the chi-Square is significantly fits the model. The logistic model
is therefore essential in the forecasting for Beijing Municipal Environment Protection Bureau.
The main purpose of data mining is to explore new rules from raw data. This is data mining
using a Logistic model for analysis. The computational cost of the Logistic model algorithm is
low, easy to implement and understand. This algorithm is mainly applicable to numerical and
classification of data. The numerical data and the categorical data set. Its shortcomings are that it
is less likely to be fitted and the accuracy of classification is low.
In this data mining process, we classify the values of PM2.5 according to their size. The smaller
the PM2.5 value the better the quality of air. Through the Logistic model, we conclude that air
quality is related to temperature, pressure, and wind speed and air dew point. In addition, we can
predict the probability of air quality superiority through the Logistic model according to the
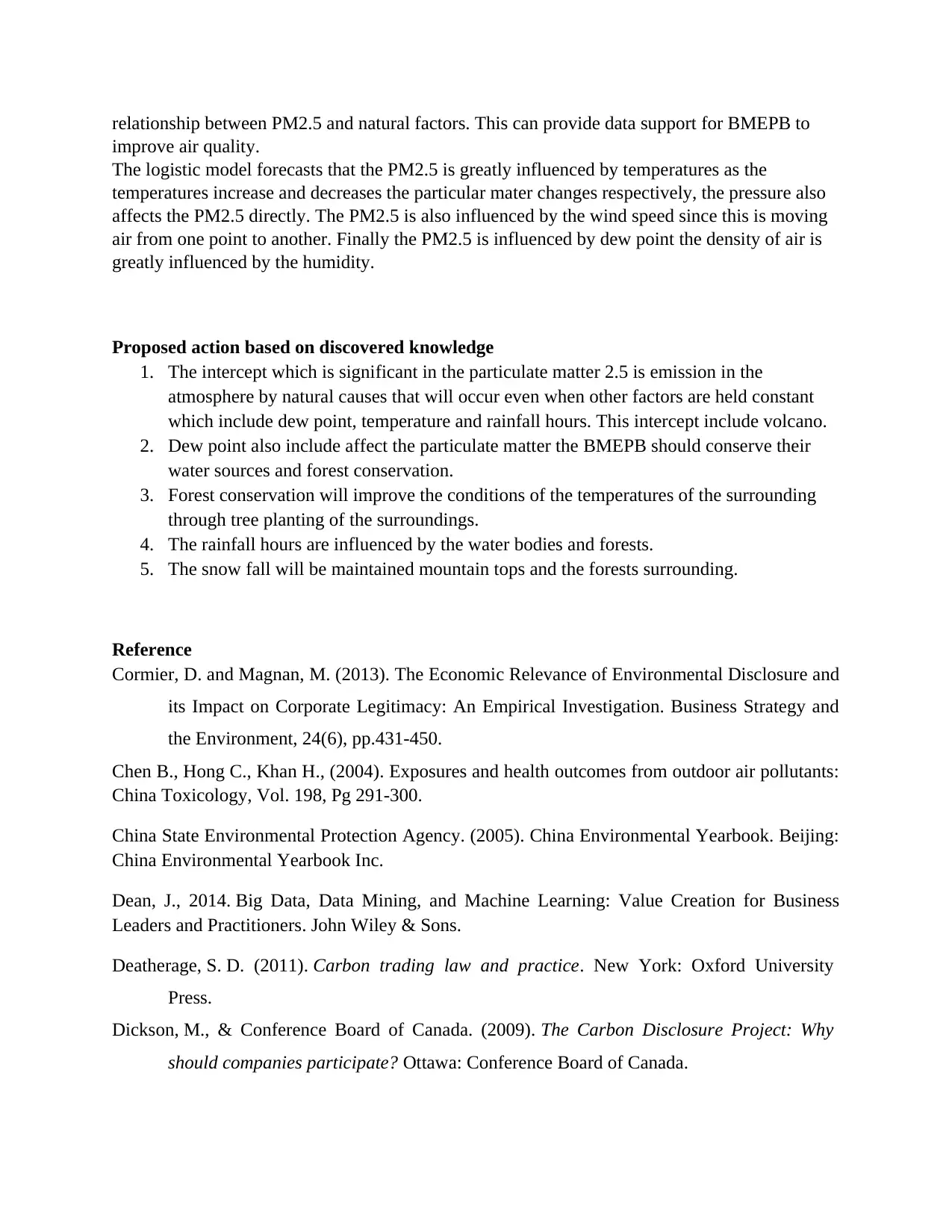
relationship between PM2.5 and natural factors. This can provide data support for BMEPB to
improve air quality.
The logistic model forecasts that the PM2.5 is greatly influenced by temperatures as the
temperatures increase and decreases the particular mater changes respectively, the pressure also
affects the PM2.5 directly. The PM2.5 is also influenced by the wind speed since this is moving
air from one point to another. Finally the PM2.5 is influenced by dew point the density of air is
greatly influenced by the humidity.
Proposed action based on discovered knowledge
1. The intercept which is significant in the particulate matter 2.5 is emission in the
atmosphere by natural causes that will occur even when other factors are held constant
which include dew point, temperature and rainfall hours. This intercept include volcano.
2. Dew point also include affect the particulate matter the BMEPB should conserve their
water sources and forest conservation.
3. Forest conservation will improve the conditions of the temperatures of the surrounding
through tree planting of the surroundings.
4. The rainfall hours are influenced by the water bodies and forests.
5. The snow fall will be maintained mountain tops and the forests surrounding.
Reference
Cormier, D. and Magnan, M. (2013). The Economic Relevance of Environmental Disclosure and
its Impact on Corporate Legitimacy: An Empirical Investigation. Business Strategy and
the Environment, 24(6), pp.431-450.
Chen B., Hong C., Khan H., (2004). Exposures and health outcomes from outdoor air pollutants:
China Toxicology, Vol. 198, Pg 291-300.
China State Environmental Protection Agency. (2005). China Environmental Yearbook. Beijing:
China Environmental Yearbook Inc.
Dean, J., 2014. Big Data, Data Mining, and Machine Learning: Value Creation for Business
Leaders and Practitioners. John Wiley & Sons.
Deatherage, S. D. (2011). Carbon trading law and practice. New York: Oxford University
Press.
Dickson, M., & Conference Board of Canada. (2009). The Carbon Disclosure Project: Why
should companies participate? Ottawa: Conference Board of Canada.
improve air quality.
The logistic model forecasts that the PM2.5 is greatly influenced by temperatures as the
temperatures increase and decreases the particular mater changes respectively, the pressure also
affects the PM2.5 directly. The PM2.5 is also influenced by the wind speed since this is moving
air from one point to another. Finally the PM2.5 is influenced by dew point the density of air is
greatly influenced by the humidity.
Proposed action based on discovered knowledge
1. The intercept which is significant in the particulate matter 2.5 is emission in the
atmosphere by natural causes that will occur even when other factors are held constant
which include dew point, temperature and rainfall hours. This intercept include volcano.
2. Dew point also include affect the particulate matter the BMEPB should conserve their
water sources and forest conservation.
3. Forest conservation will improve the conditions of the temperatures of the surrounding
through tree planting of the surroundings.
4. The rainfall hours are influenced by the water bodies and forests.
5. The snow fall will be maintained mountain tops and the forests surrounding.
Reference
Cormier, D. and Magnan, M. (2013). The Economic Relevance of Environmental Disclosure and
its Impact on Corporate Legitimacy: An Empirical Investigation. Business Strategy and
the Environment, 24(6), pp.431-450.
Chen B., Hong C., Khan H., (2004). Exposures and health outcomes from outdoor air pollutants:
China Toxicology, Vol. 198, Pg 291-300.
China State Environmental Protection Agency. (2005). China Environmental Yearbook. Beijing:
China Environmental Yearbook Inc.
Dean, J., 2014. Big Data, Data Mining, and Machine Learning: Value Creation for Business
Leaders and Practitioners. John Wiley & Sons.
Deatherage, S. D. (2011). Carbon trading law and practice. New York: Oxford University
Press.
Dickson, M., & Conference Board of Canada. (2009). The Carbon Disclosure Project: Why
should companies participate? Ottawa: Conference Board of Canada.
Paraphrase This Document
Need a fresh take? Get an instant paraphrase of this document with our AI Paraphraser
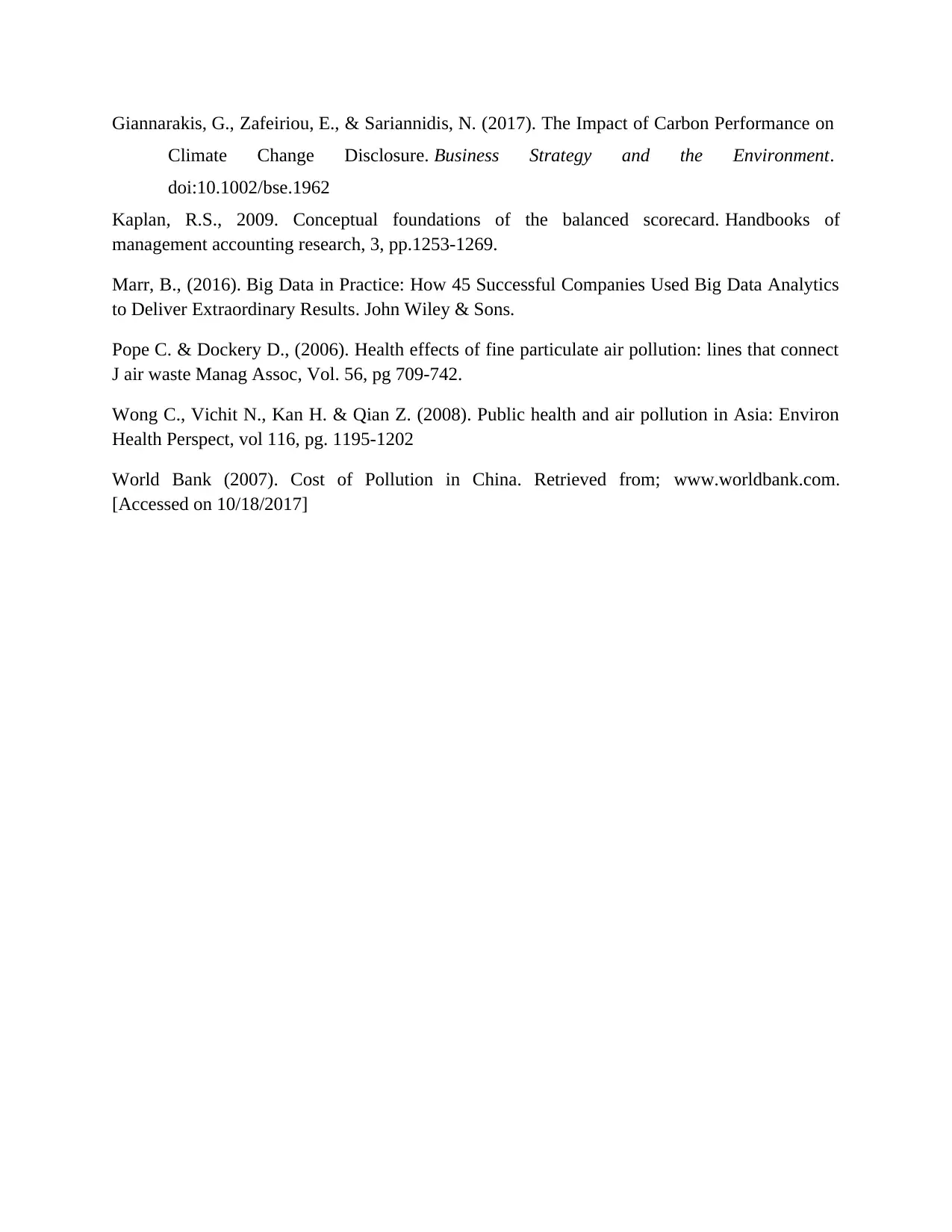
Giannarakis, G., Zafeiriou, E., & Sariannidis, N. (2017). The Impact of Carbon Performance on
Climate Change Disclosure. Business Strategy and the Environment.
doi:10.1002/bse.1962
Kaplan, R.S., 2009. Conceptual foundations of the balanced scorecard. Handbooks of
management accounting research, 3, pp.1253-1269.
Marr, B., (2016). Big Data in Practice: How 45 Successful Companies Used Big Data Analytics
to Deliver Extraordinary Results. John Wiley & Sons.
Pope C. & Dockery D., (2006). Health effects of fine particulate air pollution: lines that connect
J air waste Manag Assoc, Vol. 56, pg 709-742.
Wong C., Vichit N., Kan H. & Qian Z. (2008). Public health and air pollution in Asia: Environ
Health Perspect, vol 116, pg. 1195-1202
World Bank (2007). Cost of Pollution in China. Retrieved from; www.worldbank.com.
[Accessed on 10/18/2017]
Climate Change Disclosure. Business Strategy and the Environment.
doi:10.1002/bse.1962
Kaplan, R.S., 2009. Conceptual foundations of the balanced scorecard. Handbooks of
management accounting research, 3, pp.1253-1269.
Marr, B., (2016). Big Data in Practice: How 45 Successful Companies Used Big Data Analytics
to Deliver Extraordinary Results. John Wiley & Sons.
Pope C. & Dockery D., (2006). Health effects of fine particulate air pollution: lines that connect
J air waste Manag Assoc, Vol. 56, pg 709-742.
Wong C., Vichit N., Kan H. & Qian Z. (2008). Public health and air pollution in Asia: Environ
Health Perspect, vol 116, pg. 1195-1202
World Bank (2007). Cost of Pollution in China. Retrieved from; www.worldbank.com.
[Accessed on 10/18/2017]
1 out of 17
Related Documents
![[object Object]](/_next/image/?url=%2F_next%2Fstatic%2Fmedia%2Flogo.6d15ce61.png&w=640&q=75)
Your All-in-One AI-Powered Toolkit for Academic Success.
+13062052269
info@desklib.com
Available 24*7 on WhatsApp / Email
Unlock your academic potential
© 2024 | Zucol Services PVT LTD | All rights reserved.