Epidemiology: Control of Confounding Variables and Outcomes Measurement
VerifiedAdded on  2023/06/11
|4
|586
|498
AI Summary
This paper discusses the control of confounding variables in epidemiology and the measurement of outcomes. It identifies the confounding factors and strategies for controlling them. The paper also highlights the limitations of the study, such as unreliable and invalid outcomes measurement and a long exposure period.
Contribute Materials
Your contribution can guide someone’s learning journey. Share your
documents today.
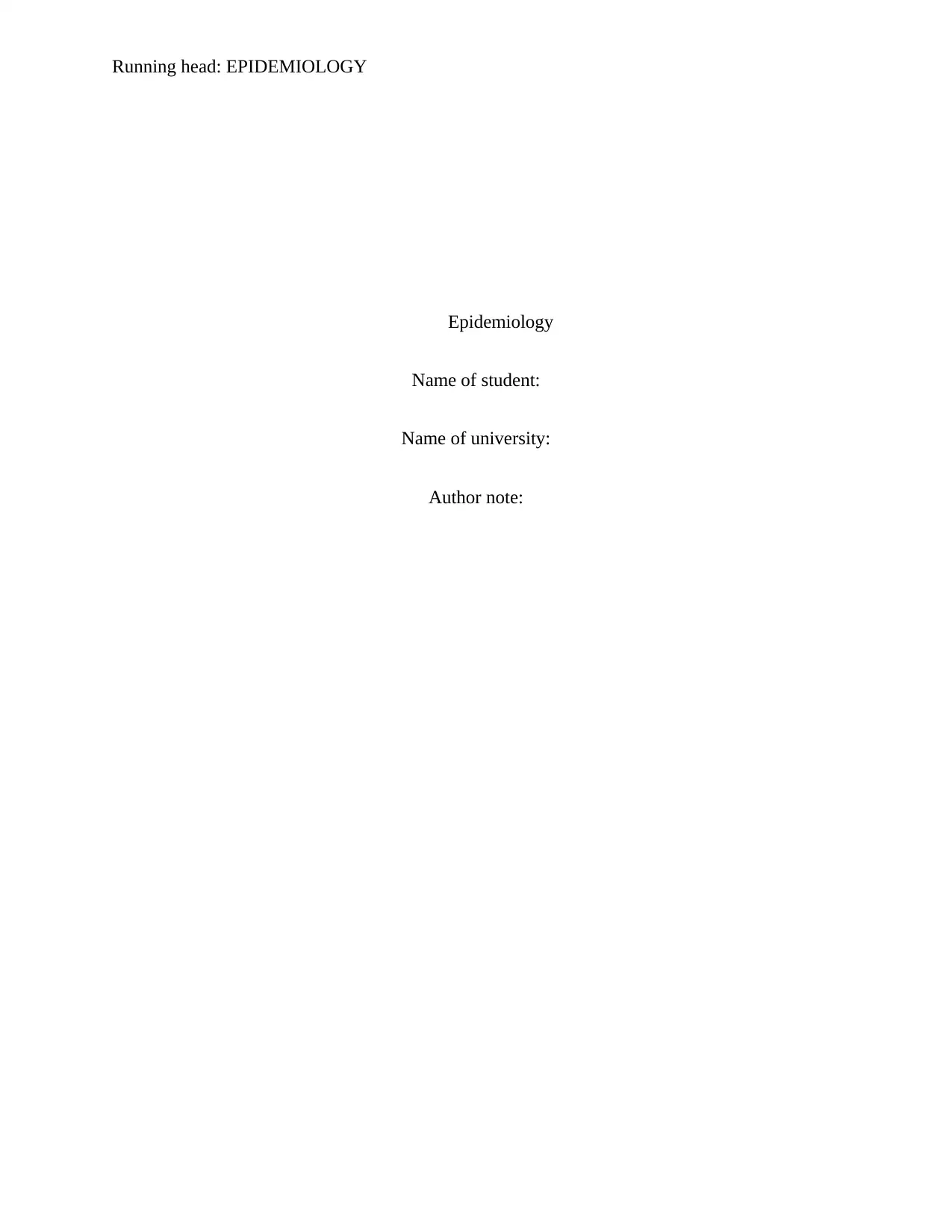
Running head: EPIDEMIOLOGY
Epidemiology
Name of student:
Name of university:
Author note:
Epidemiology
Name of student:
Name of university:
Author note:
Secure Best Marks with AI Grader
Need help grading? Try our AI Grader for instant feedback on your assignments.
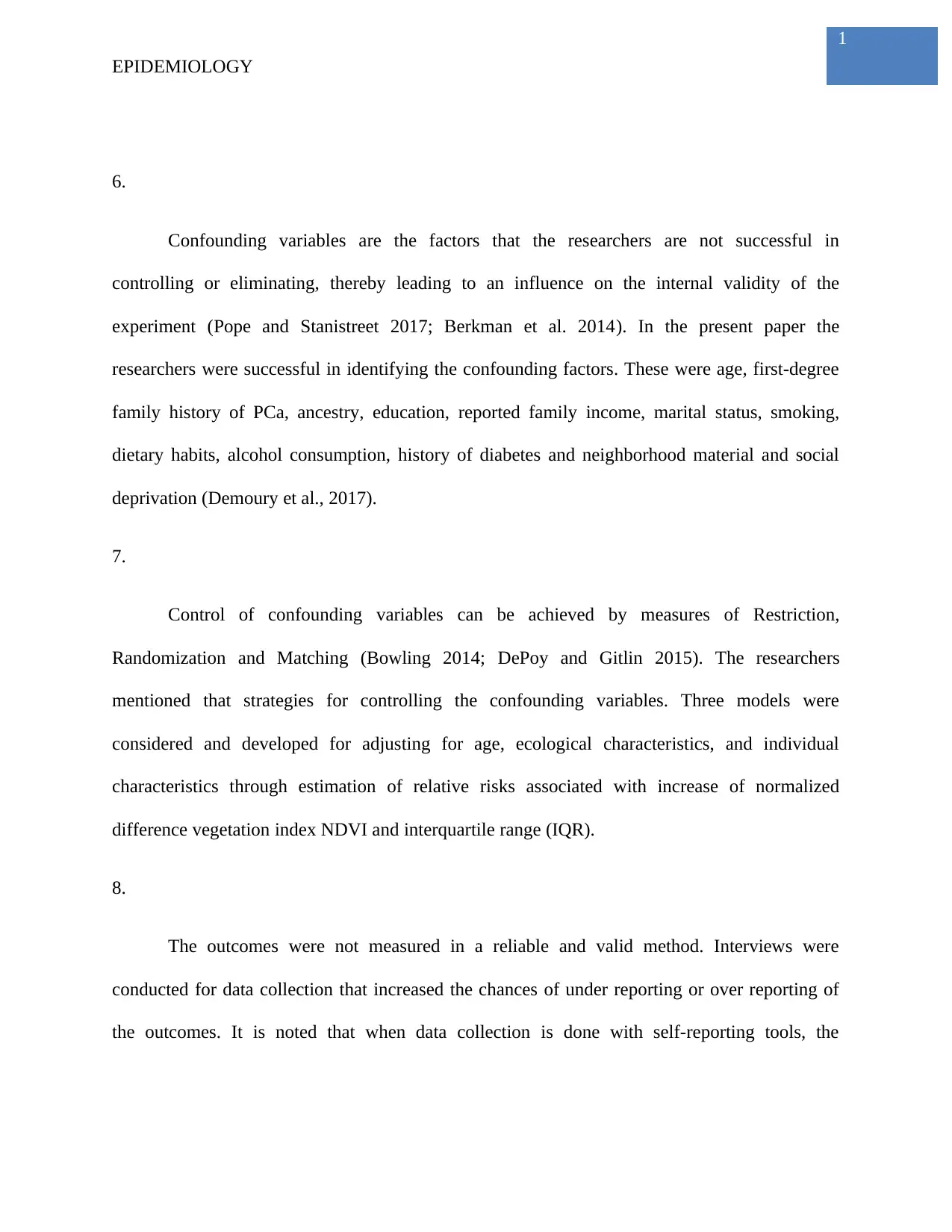
1
EPIDEMIOLOGY
6.
Confounding variables are the factors that the researchers are not successful in
controlling or eliminating, thereby leading to an influence on the internal validity of the
experiment (Pope and Stanistreet 2017; Berkman et al. 2014). In the present paper the
researchers were successful in identifying the confounding factors. These were age, first-degree
family history of PCa, ancestry, education, reported family income, marital status, smoking,
dietary habits, alcohol consumption, history of diabetes and neighborhood material and social
deprivation (Demoury et al., 2017).
7.
Control of confounding variables can be achieved by measures of Restriction,
Randomization and Matching (Bowling 2014; DePoy and Gitlin 2015). The researchers
mentioned that strategies for controlling the confounding variables. Three models were
considered and developed for adjusting for age, ecological characteristics, and individual
characteristics through estimation of relative risks associated with increase of normalized
difference vegetation index NDVI and interquartile range (IQR).
8.
The outcomes were not measured in a reliable and valid method. Interviews were
conducted for data collection that increased the chances of under reporting or over reporting of
the outcomes. It is noted that when data collection is done with self-reporting tools, the
EPIDEMIOLOGY
6.
Confounding variables are the factors that the researchers are not successful in
controlling or eliminating, thereby leading to an influence on the internal validity of the
experiment (Pope and Stanistreet 2017; Berkman et al. 2014). In the present paper the
researchers were successful in identifying the confounding factors. These were age, first-degree
family history of PCa, ancestry, education, reported family income, marital status, smoking,
dietary habits, alcohol consumption, history of diabetes and neighborhood material and social
deprivation (Demoury et al., 2017).
7.
Control of confounding variables can be achieved by measures of Restriction,
Randomization and Matching (Bowling 2014; DePoy and Gitlin 2015). The researchers
mentioned that strategies for controlling the confounding variables. Three models were
considered and developed for adjusting for age, ecological characteristics, and individual
characteristics through estimation of relative risks associated with increase of normalized
difference vegetation index NDVI and interquartile range (IQR).
8.
The outcomes were not measured in a reliable and valid method. Interviews were
conducted for data collection that increased the chances of under reporting or over reporting of
the outcomes. It is noted that when data collection is done with self-reporting tools, the
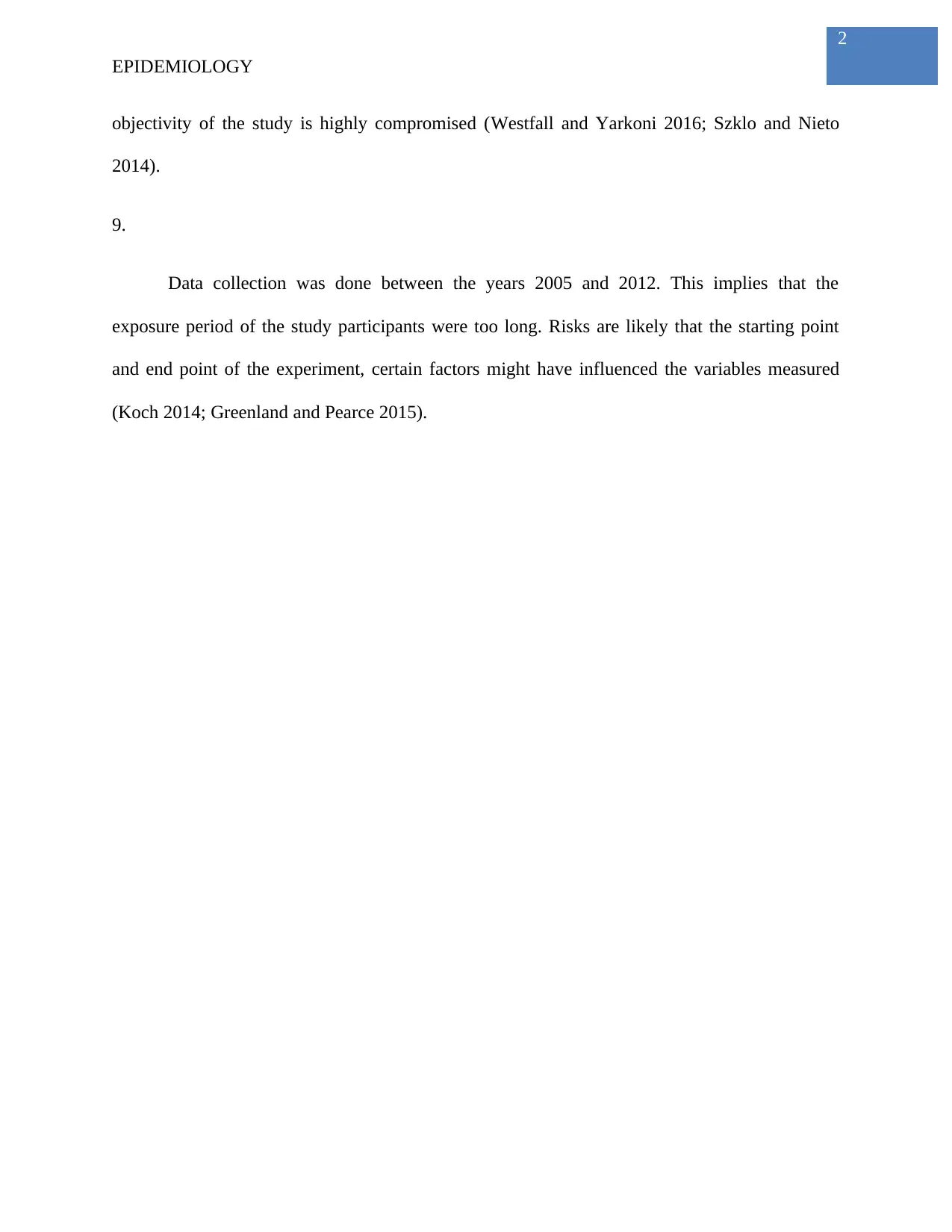
2
EPIDEMIOLOGY
objectivity of the study is highly compromised (Westfall and Yarkoni 2016; Szklo and Nieto
2014).
9.
Data collection was done between the years 2005 and 2012. This implies that the
exposure period of the study participants were too long. Risks are likely that the starting point
and end point of the experiment, certain factors might have influenced the variables measured
(Koch 2014; Greenland and Pearce 2015).
EPIDEMIOLOGY
objectivity of the study is highly compromised (Westfall and Yarkoni 2016; Szklo and Nieto
2014).
9.
Data collection was done between the years 2005 and 2012. This implies that the
exposure period of the study participants were too long. Risks are likely that the starting point
and end point of the experiment, certain factors might have influenced the variables measured
(Koch 2014; Greenland and Pearce 2015).
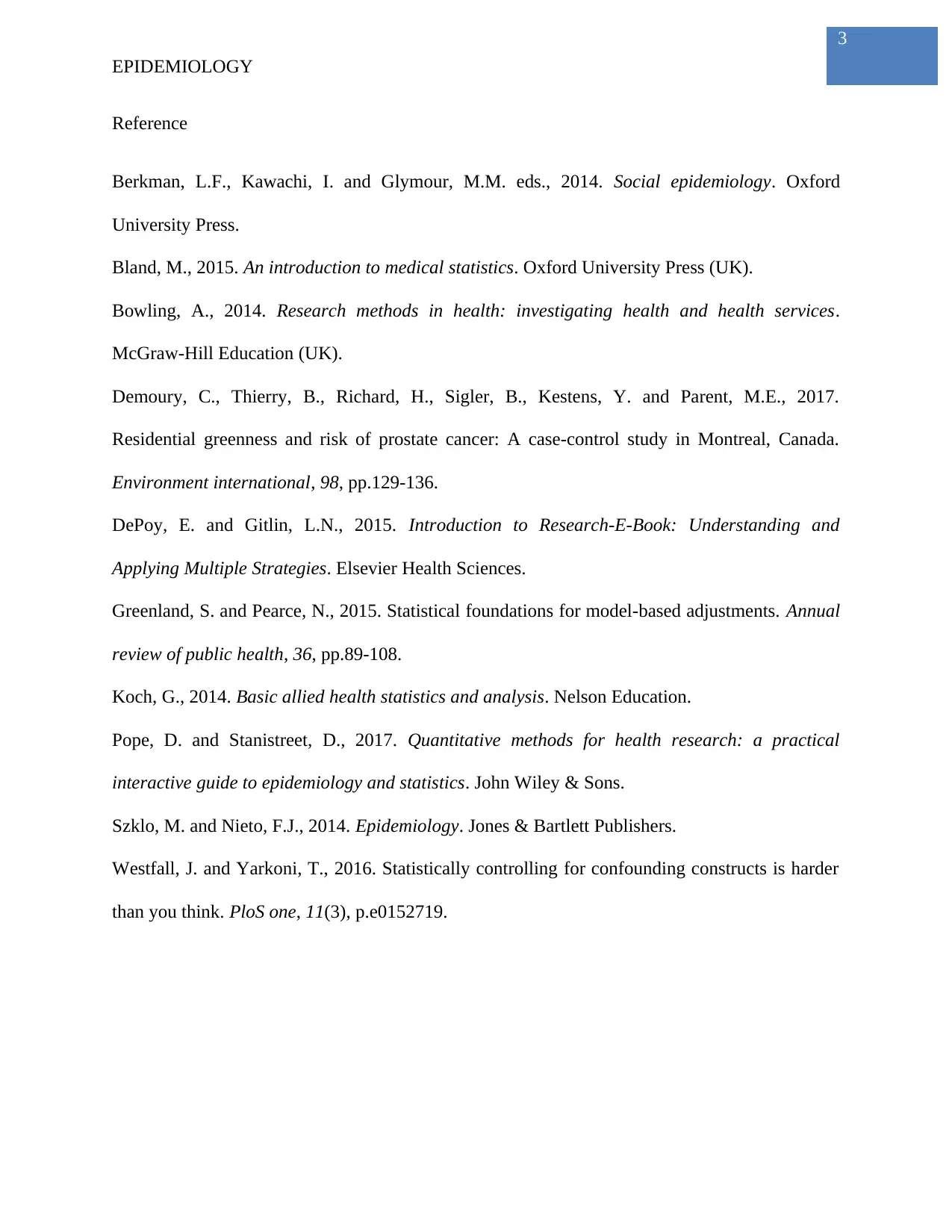
3
EPIDEMIOLOGY
Reference
Berkman, L.F., Kawachi, I. and Glymour, M.M. eds., 2014. Social epidemiology. Oxford
University Press.
Bland, M., 2015. An introduction to medical statistics. Oxford University Press (UK).
Bowling, A., 2014. Research methods in health: investigating health and health services.
McGraw-Hill Education (UK).
Demoury, C., Thierry, B., Richard, H., Sigler, B., Kestens, Y. and Parent, M.E., 2017.
Residential greenness and risk of prostate cancer: A case-control study in Montreal, Canada.
Environment international, 98, pp.129-136.
DePoy, E. and Gitlin, L.N., 2015. Introduction to Research-E-Book: Understanding and
Applying Multiple Strategies. Elsevier Health Sciences.
Greenland, S. and Pearce, N., 2015. Statistical foundations for model-based adjustments. Annual
review of public health, 36, pp.89-108.
Koch, G., 2014. Basic allied health statistics and analysis. Nelson Education.
Pope, D. and Stanistreet, D., 2017. Quantitative methods for health research: a practical
interactive guide to epidemiology and statistics. John Wiley & Sons.
Szklo, M. and Nieto, F.J., 2014. Epidemiology. Jones & Bartlett Publishers.
Westfall, J. and Yarkoni, T., 2016. Statistically controlling for confounding constructs is harder
than you think. PloS one, 11(3), p.e0152719.
EPIDEMIOLOGY
Reference
Berkman, L.F., Kawachi, I. and Glymour, M.M. eds., 2014. Social epidemiology. Oxford
University Press.
Bland, M., 2015. An introduction to medical statistics. Oxford University Press (UK).
Bowling, A., 2014. Research methods in health: investigating health and health services.
McGraw-Hill Education (UK).
Demoury, C., Thierry, B., Richard, H., Sigler, B., Kestens, Y. and Parent, M.E., 2017.
Residential greenness and risk of prostate cancer: A case-control study in Montreal, Canada.
Environment international, 98, pp.129-136.
DePoy, E. and Gitlin, L.N., 2015. Introduction to Research-E-Book: Understanding and
Applying Multiple Strategies. Elsevier Health Sciences.
Greenland, S. and Pearce, N., 2015. Statistical foundations for model-based adjustments. Annual
review of public health, 36, pp.89-108.
Koch, G., 2014. Basic allied health statistics and analysis. Nelson Education.
Pope, D. and Stanistreet, D., 2017. Quantitative methods for health research: a practical
interactive guide to epidemiology and statistics. John Wiley & Sons.
Szklo, M. and Nieto, F.J., 2014. Epidemiology. Jones & Bartlett Publishers.
Westfall, J. and Yarkoni, T., 2016. Statistically controlling for confounding constructs is harder
than you think. PloS one, 11(3), p.e0152719.
1 out of 4
![[object Object]](/_next/image/?url=%2F_next%2Fstatic%2Fmedia%2Flogo.6d15ce61.png&w=640&q=75)
Your All-in-One AI-Powered Toolkit for Academic Success.
 +13062052269
info@desklib.com
Available 24*7 on WhatsApp / Email
Unlock your academic potential
© 2024  |  Zucol Services PVT LTD  |  All rights reserved.