Factors Affecting Plasma Beta Carotene
VerifiedAdded on  2023/04/21
|23
|5275
|429
AI Summary
This paper investigates the factors that affect plasma beta-carotene using regression techniques of LASSO and LAR. It explores correlation analysis, regression analysis, and discusses the results. The study examines the impact of variables such as age, sex, alcohol consumption, fiber consumption, and more on plasma beta-carotene levels.
Contribute Materials
Your contribution can guide someone’s learning journey. Share your
documents today.
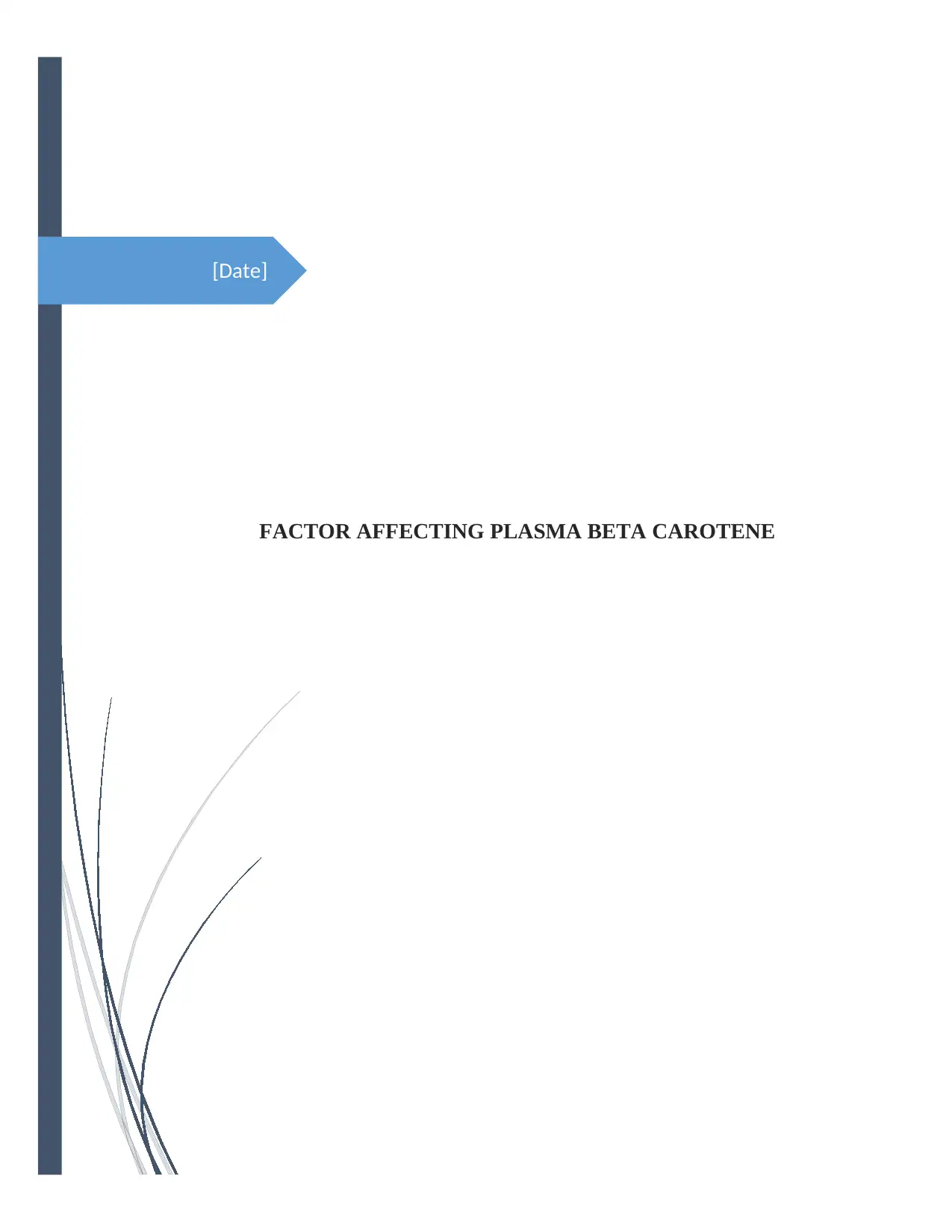
[Date]
FACTOR AFFECTING PLASMA BETA CAROTENE
FACTOR AFFECTING PLASMA BETA CAROTENE
Secure Best Marks with AI Grader
Need help grading? Try our AI Grader for instant feedback on your assignments.
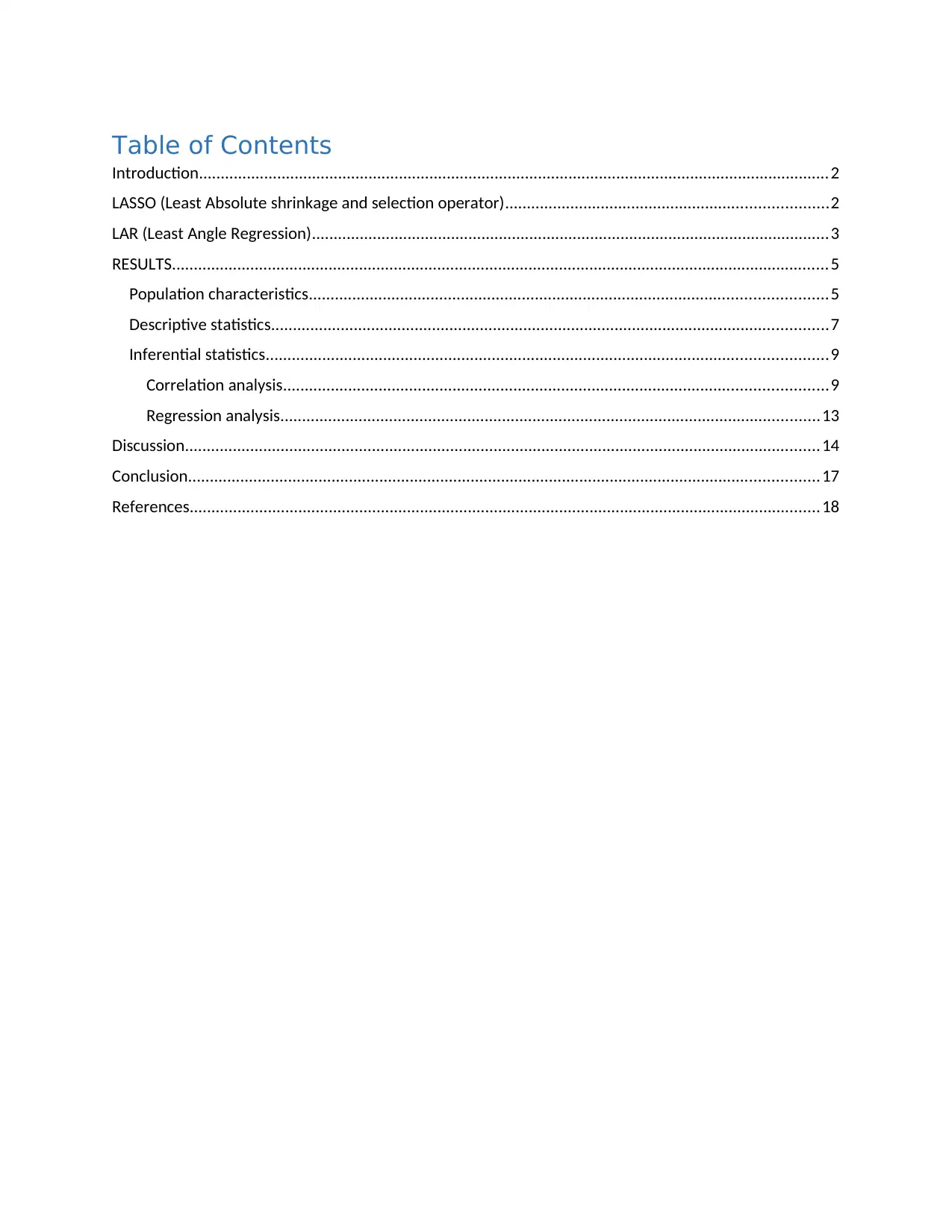
Table of Contents
Introduction.................................................................................................................................................2
LASSO (Least Absolute shrinkage and selection operator)..........................................................................2
LAR (Least Angle Regression).......................................................................................................................3
RESULTS.......................................................................................................................................................5
Population characteristics.......................................................................................................................5
Descriptive statistics................................................................................................................................7
Inferential statistics.................................................................................................................................9
Correlation analysis.............................................................................................................................9
Regression analysis............................................................................................................................13
Discussion..................................................................................................................................................14
Conclusion.................................................................................................................................................17
References.................................................................................................................................................18
Introduction.................................................................................................................................................2
LASSO (Least Absolute shrinkage and selection operator)..........................................................................2
LAR (Least Angle Regression).......................................................................................................................3
RESULTS.......................................................................................................................................................5
Population characteristics.......................................................................................................................5
Descriptive statistics................................................................................................................................7
Inferential statistics.................................................................................................................................9
Correlation analysis.............................................................................................................................9
Regression analysis............................................................................................................................13
Discussion..................................................................................................................................................14
Conclusion.................................................................................................................................................17
References.................................................................................................................................................18
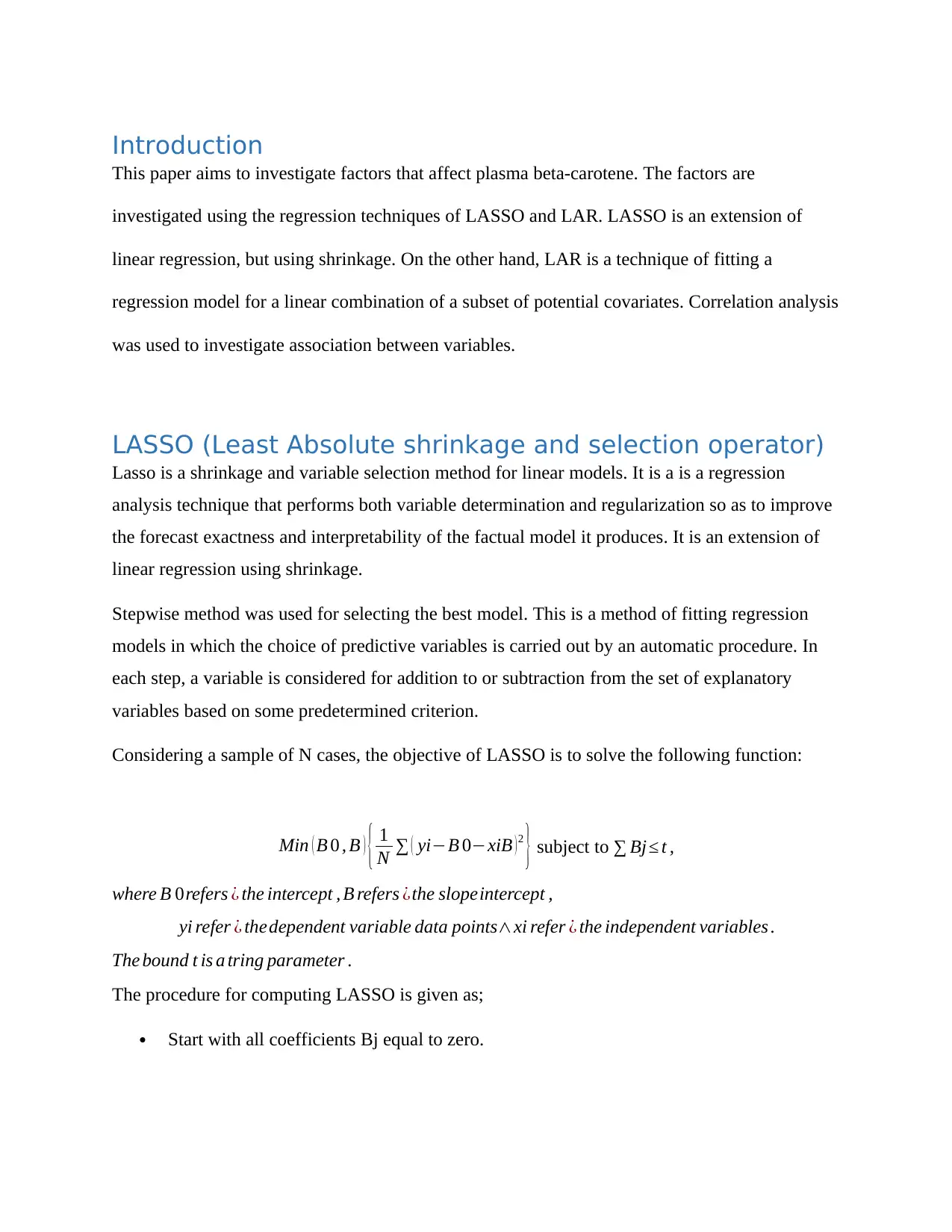
Introduction
This paper aims to investigate factors that affect plasma beta-carotene. The factors are
investigated using the regression techniques of LASSO and LAR. LASSO is an extension of
linear regression, but using shrinkage. On the other hand, LAR is a technique of fitting a
regression model for a linear combination of a subset of potential covariates. Correlation analysis
was used to investigate association between variables.
LASSO (Least Absolute shrinkage and selection operator)
Lasso is a shrinkage and variable selection method for linear models. It is a is a regression
analysis technique that performs both variable determination and regularization so as to improve
the forecast exactness and interpretability of the factual model it produces. It is an extension of
linear regression using shrinkage.
Stepwise method was used for selecting the best model. This is a method of fitting regression
models in which the choice of predictive variables is carried out by an automatic procedure. In
each step, a variable is considered for addition to or subtraction from the set of explanatory
variables based on some predetermined criterion.
Considering a sample of N cases, the objective of LASSO is to solve the following function:
Min ( B 0 , B ) { 1
N ∑ ( yi−B 0−xiB ) 2
} subject to ∑ Bj ≤ t ,
where B 0refers ¿ the intercept , B refers ¿the slopeintercept ,
yi refer ¿ thedependent variable data points∧xi refer ¿ the independent variables .
The bound t is a tring parameter .
The procedure for computing LASSO is given as;
ï‚· Start with all coefficients Bj equal to zero.
This paper aims to investigate factors that affect plasma beta-carotene. The factors are
investigated using the regression techniques of LASSO and LAR. LASSO is an extension of
linear regression, but using shrinkage. On the other hand, LAR is a technique of fitting a
regression model for a linear combination of a subset of potential covariates. Correlation analysis
was used to investigate association between variables.
LASSO (Least Absolute shrinkage and selection operator)
Lasso is a shrinkage and variable selection method for linear models. It is a is a regression
analysis technique that performs both variable determination and regularization so as to improve
the forecast exactness and interpretability of the factual model it produces. It is an extension of
linear regression using shrinkage.
Stepwise method was used for selecting the best model. This is a method of fitting regression
models in which the choice of predictive variables is carried out by an automatic procedure. In
each step, a variable is considered for addition to or subtraction from the set of explanatory
variables based on some predetermined criterion.
Considering a sample of N cases, the objective of LASSO is to solve the following function:
Min ( B 0 , B ) { 1
N ∑ ( yi−B 0−xiB ) 2
} subject to ∑ Bj ≤ t ,
where B 0refers ¿ the intercept , B refers ¿the slopeintercept ,
yi refer ¿ thedependent variable data points∧xi refer ¿ the independent variables .
The bound t is a tring parameter .
The procedure for computing LASSO is given as;
ï‚· Start with all coefficients Bj equal to zero.
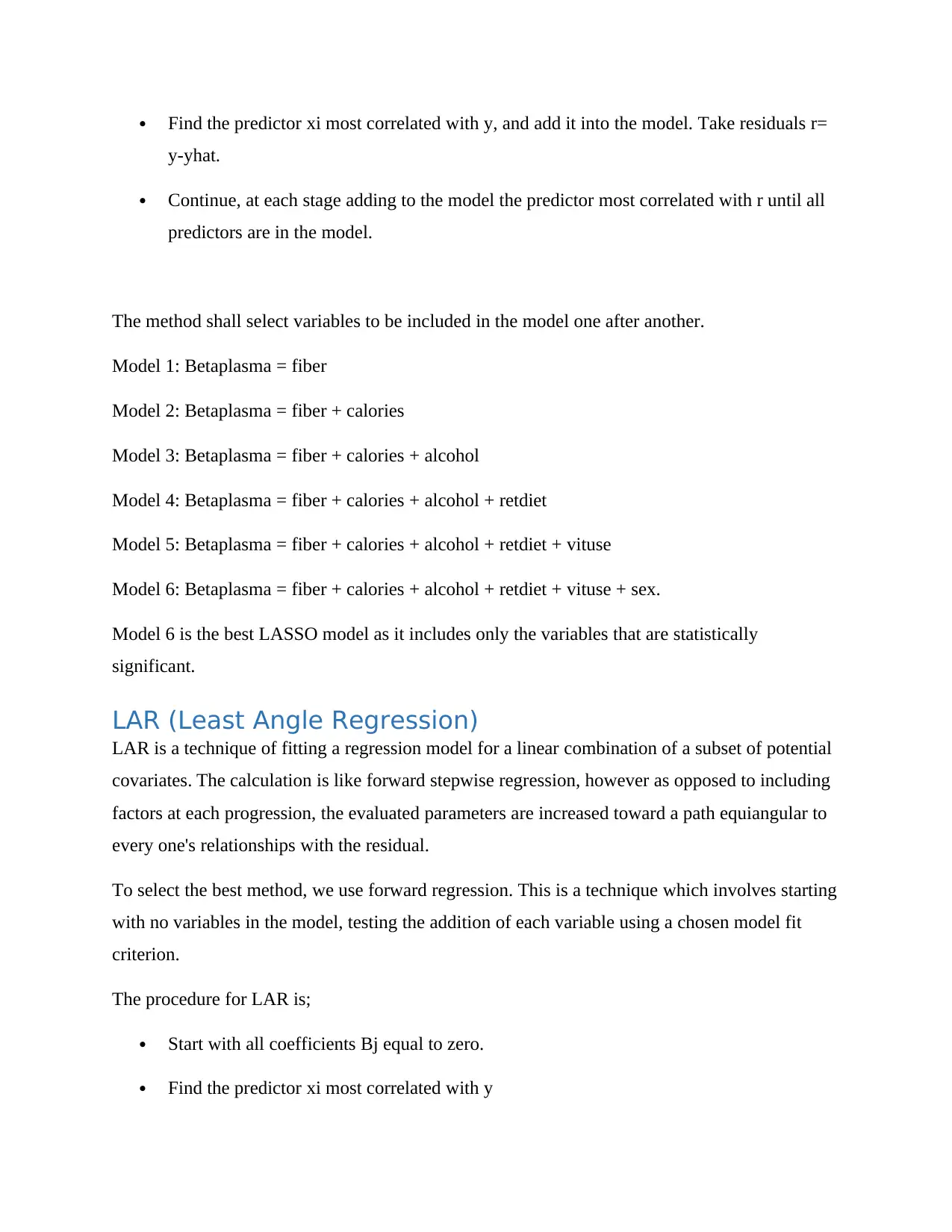
ï‚· Find the predictor xi most correlated with y, and add it into the model. Take residuals r=
y-yhat.
ï‚· Continue, at each stage adding to the model the predictor most correlated with r until all
predictors are in the model.
The method shall select variables to be included in the model one after another.
Model 1: Betaplasma = fiber
Model 2: Betaplasma = fiber + calories
Model 3: Betaplasma = fiber + calories + alcohol
Model 4: Betaplasma = fiber + calories + alcohol + retdiet
Model 5: Betaplasma = fiber + calories + alcohol + retdiet + vituse
Model 6: Betaplasma = fiber + calories + alcohol + retdiet + vituse + sex.
Model 6 is the best LASSO model as it includes only the variables that are statistically
significant.
LAR (Least Angle Regression)
LAR is a technique of fitting a regression model for a linear combination of a subset of potential
covariates. The calculation is like forward stepwise regression, however as opposed to including
factors at each progression, the evaluated parameters are increased toward a path equiangular to
every one's relationships with the residual.
To select the best method, we use forward regression. This is a technique which involves starting
with no variables in the model, testing the addition of each variable using a chosen model fit
criterion.
The procedure for LAR is;
ï‚· Start with all coefficients Bj equal to zero.
ï‚· Find the predictor xi most correlated with y
y-yhat.
ï‚· Continue, at each stage adding to the model the predictor most correlated with r until all
predictors are in the model.
The method shall select variables to be included in the model one after another.
Model 1: Betaplasma = fiber
Model 2: Betaplasma = fiber + calories
Model 3: Betaplasma = fiber + calories + alcohol
Model 4: Betaplasma = fiber + calories + alcohol + retdiet
Model 5: Betaplasma = fiber + calories + alcohol + retdiet + vituse
Model 6: Betaplasma = fiber + calories + alcohol + retdiet + vituse + sex.
Model 6 is the best LASSO model as it includes only the variables that are statistically
significant.
LAR (Least Angle Regression)
LAR is a technique of fitting a regression model for a linear combination of a subset of potential
covariates. The calculation is like forward stepwise regression, however as opposed to including
factors at each progression, the evaluated parameters are increased toward a path equiangular to
every one's relationships with the residual.
To select the best method, we use forward regression. This is a technique which involves starting
with no variables in the model, testing the addition of each variable using a chosen model fit
criterion.
The procedure for LAR is;
ï‚· Start with all coefficients Bj equal to zero.
ï‚· Find the predictor xi most correlated with y
Secure Best Marks with AI Grader
Need help grading? Try our AI Grader for instant feedback on your assignments.
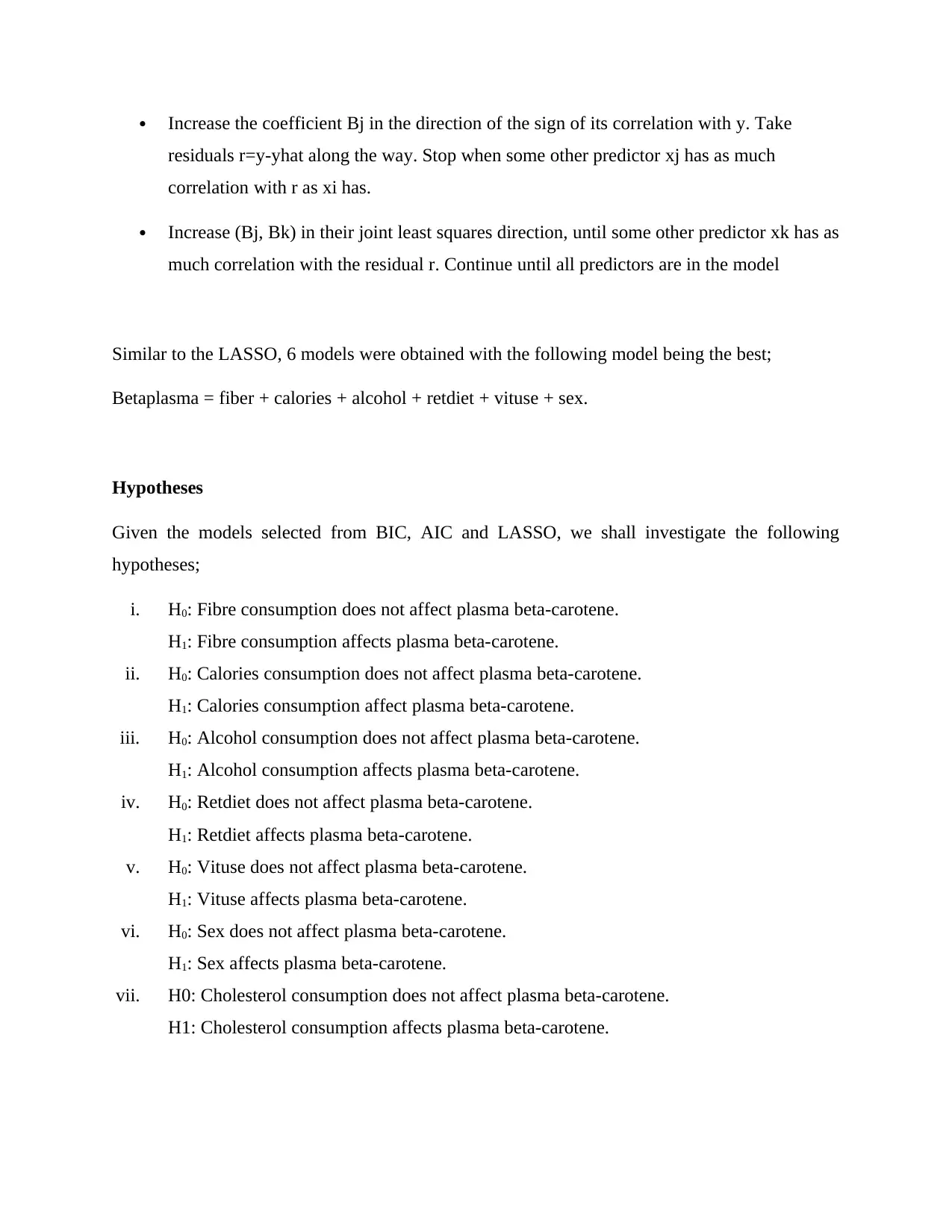
ï‚· Increase the coefficient Bj in the direction of the sign of its correlation with y. Take
residuals r=y-yhat along the way. Stop when some other predictor xj has as much
correlation with r as xi has.
ï‚· Increase (Bj, Bk) in their joint least squares direction, until some other predictor xk has as
much correlation with the residual r. Continue until all predictors are in the model
Similar to the LASSO, 6 models were obtained with the following model being the best;
Betaplasma = fiber + calories + alcohol + retdiet + vituse + sex.
Hypotheses
Given the models selected from BIC, AIC and LASSO, we shall investigate the following
hypotheses;
i. H0: Fibre consumption does not affect plasma beta-carotene.
H1: Fibre consumption affects plasma beta-carotene.
ii. H0: Calories consumption does not affect plasma beta-carotene.
H1: Calories consumption affect plasma beta-carotene.
iii. H0: Alcohol consumption does not affect plasma beta-carotene.
H1: Alcohol consumption affects plasma beta-carotene.
iv. H0: Retdiet does not affect plasma beta-carotene.
H1: Retdiet affects plasma beta-carotene.
v. H0: Vituse does not affect plasma beta-carotene.
H1: Vituse affects plasma beta-carotene.
vi. H0: Sex does not affect plasma beta-carotene.
H1: Sex affects plasma beta-carotene.
vii. H0: Cholesterol consumption does not affect plasma beta-carotene.
H1: Cholesterol consumption affects plasma beta-carotene.
residuals r=y-yhat along the way. Stop when some other predictor xj has as much
correlation with r as xi has.
ï‚· Increase (Bj, Bk) in their joint least squares direction, until some other predictor xk has as
much correlation with the residual r. Continue until all predictors are in the model
Similar to the LASSO, 6 models were obtained with the following model being the best;
Betaplasma = fiber + calories + alcohol + retdiet + vituse + sex.
Hypotheses
Given the models selected from BIC, AIC and LASSO, we shall investigate the following
hypotheses;
i. H0: Fibre consumption does not affect plasma beta-carotene.
H1: Fibre consumption affects plasma beta-carotene.
ii. H0: Calories consumption does not affect plasma beta-carotene.
H1: Calories consumption affect plasma beta-carotene.
iii. H0: Alcohol consumption does not affect plasma beta-carotene.
H1: Alcohol consumption affects plasma beta-carotene.
iv. H0: Retdiet does not affect plasma beta-carotene.
H1: Retdiet affects plasma beta-carotene.
v. H0: Vituse does not affect plasma beta-carotene.
H1: Vituse affects plasma beta-carotene.
vi. H0: Sex does not affect plasma beta-carotene.
H1: Sex affects plasma beta-carotene.
vii. H0: Cholesterol consumption does not affect plasma beta-carotene.
H1: Cholesterol consumption affects plasma beta-carotene.
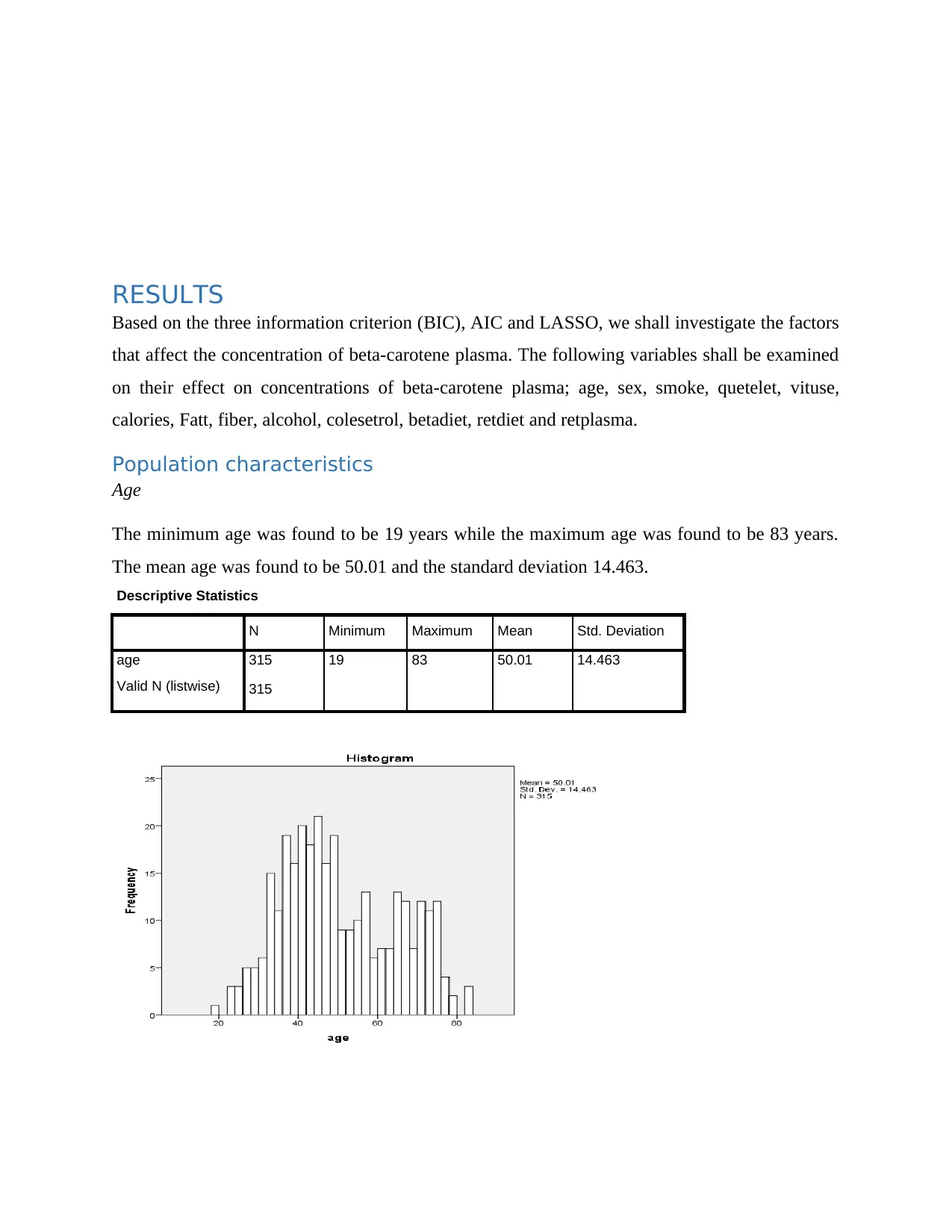
RESULTS
Based on the three information criterion (BIC), AIC and LASSO, we shall investigate the factors
that affect the concentration of beta-carotene plasma. The following variables shall be examined
on their effect on concentrations of beta-carotene plasma; age, sex, smoke, quetelet, vituse,
calories, Fatt, fiber, alcohol, colesetrol, betadiet, retdiet and retplasma.
Population characteristics
Age
The minimum age was found to be 19 years while the maximum age was found to be 83 years.
The mean age was found to be 50.01 and the standard deviation 14.463.
Descriptive Statistics
N Minimum Maximum Mean Std. Deviation
age 315 19 83 50.01 14.463
Valid N (listwise) 315
Based on the three information criterion (BIC), AIC and LASSO, we shall investigate the factors
that affect the concentration of beta-carotene plasma. The following variables shall be examined
on their effect on concentrations of beta-carotene plasma; age, sex, smoke, quetelet, vituse,
calories, Fatt, fiber, alcohol, colesetrol, betadiet, retdiet and retplasma.
Population characteristics
Age
The minimum age was found to be 19 years while the maximum age was found to be 83 years.
The mean age was found to be 50.01 and the standard deviation 14.463.
Descriptive Statistics
N Minimum Maximum Mean Std. Deviation
age 315 19 83 50.01 14.463
Valid N (listwise) 315
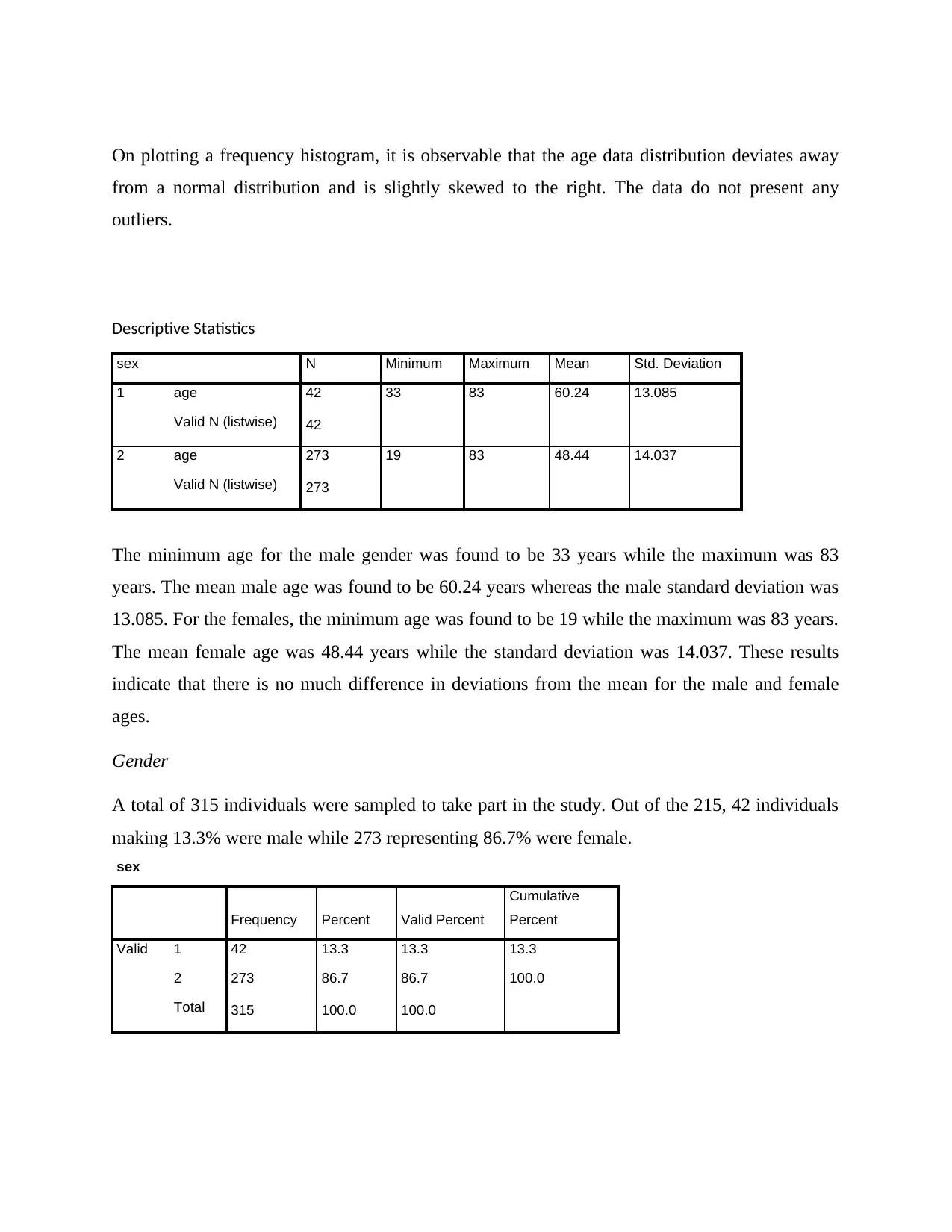
On plotting a frequency histogram, it is observable that the age data distribution deviates away
from a normal distribution and is slightly skewed to the right. The data do not present any
outliers.
Descriptive Statistics
sex N Minimum Maximum Mean Std. Deviation
1 age 42 33 83 60.24 13.085
Valid N (listwise) 42
2 age 273 19 83 48.44 14.037
Valid N (listwise) 273
The minimum age for the male gender was found to be 33 years while the maximum was 83
years. The mean male age was found to be 60.24 years whereas the male standard deviation was
13.085. For the females, the minimum age was found to be 19 while the maximum was 83 years.
The mean female age was 48.44 years while the standard deviation was 14.037. These results
indicate that there is no much difference in deviations from the mean for the male and female
ages.
Gender
A total of 315 individuals were sampled to take part in the study. Out of the 215, 42 individuals
making 13.3% were male while 273 representing 86.7% were female.
sex
Frequency Percent Valid Percent
Cumulative
Percent
Valid 1 42 13.3 13.3 13.3
2 273 86.7 86.7 100.0
Total 315 100.0 100.0
from a normal distribution and is slightly skewed to the right. The data do not present any
outliers.
Descriptive Statistics
sex N Minimum Maximum Mean Std. Deviation
1 age 42 33 83 60.24 13.085
Valid N (listwise) 42
2 age 273 19 83 48.44 14.037
Valid N (listwise) 273
The minimum age for the male gender was found to be 33 years while the maximum was 83
years. The mean male age was found to be 60.24 years whereas the male standard deviation was
13.085. For the females, the minimum age was found to be 19 while the maximum was 83 years.
The mean female age was 48.44 years while the standard deviation was 14.037. These results
indicate that there is no much difference in deviations from the mean for the male and female
ages.
Gender
A total of 315 individuals were sampled to take part in the study. Out of the 215, 42 individuals
making 13.3% were male while 273 representing 86.7% were female.
sex
Frequency Percent Valid Percent
Cumulative
Percent
Valid 1 42 13.3 13.3 13.3
2 273 86.7 86.7 100.0
Total 315 100.0 100.0
Paraphrase This Document
Need a fresh take? Get an instant paraphrase of this document with our AI Paraphraser
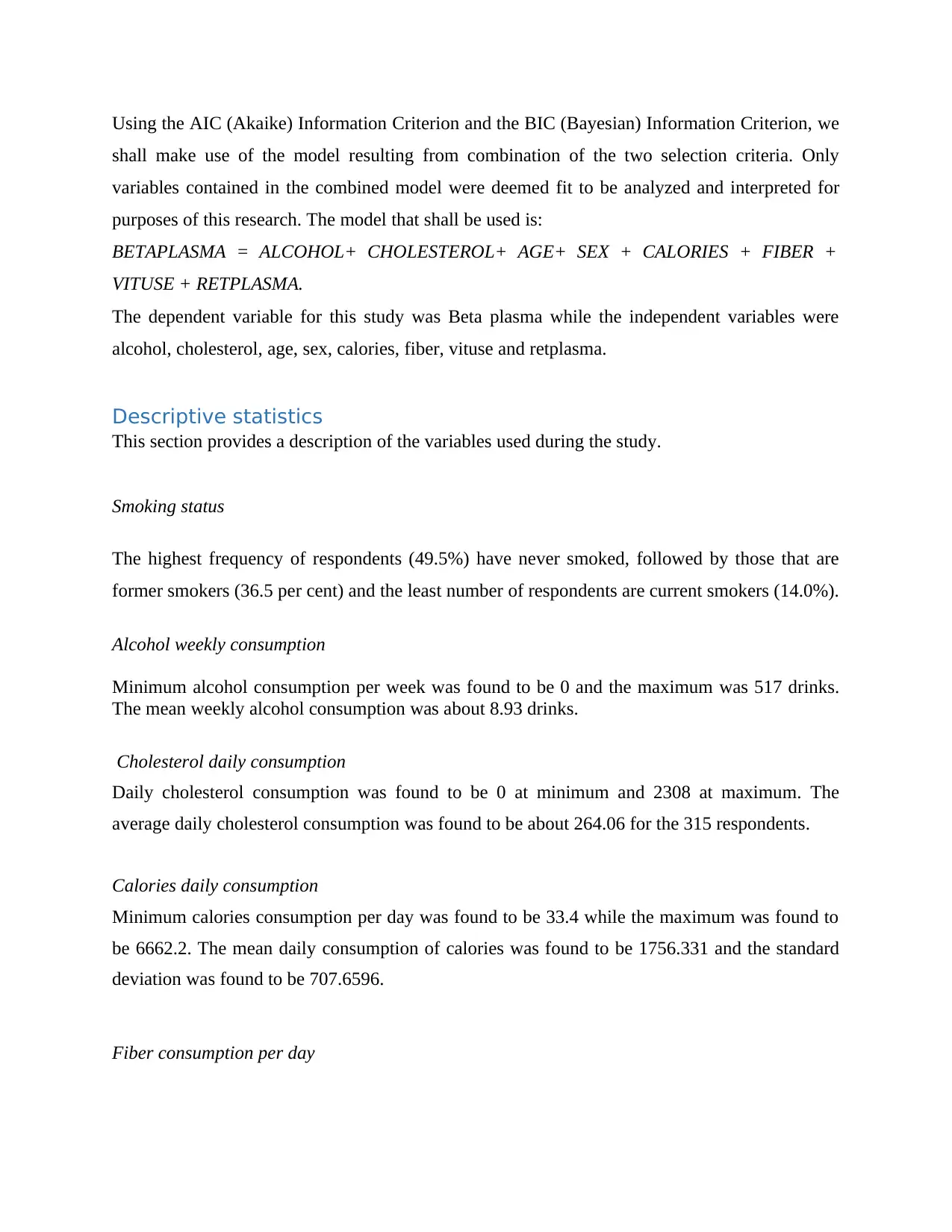
Using the AIC (Akaike) Information Criterion and the BIC (Bayesian) Information Criterion, we
shall make use of the model resulting from combination of the two selection criteria. Only
variables contained in the combined model were deemed fit to be analyzed and interpreted for
purposes of this research. The model that shall be used is:
BETAPLASMA = ALCOHOL+ CHOLESTEROL+ AGE+ SEX + CALORIES + FIBER +
VITUSE + RETPLASMA.
The dependent variable for this study was Beta plasma while the independent variables were
alcohol, cholesterol, age, sex, calories, fiber, vituse and retplasma.
Descriptive statistics
This section provides a description of the variables used during the study.
Smoking status
The highest frequency of respondents (49.5%) have never smoked, followed by those that are
former smokers (36.5 per cent) and the least number of respondents are current smokers (14.0%).
Alcohol weekly consumption
Minimum alcohol consumption per week was found to be 0 and the maximum was 517 drinks.
The mean weekly alcohol consumption was about 8.93 drinks.
Cholesterol daily consumption
Daily cholesterol consumption was found to be 0 at minimum and 2308 at maximum. The
average daily cholesterol consumption was found to be about 264.06 for the 315 respondents.
Calories daily consumption
Minimum calories consumption per day was found to be 33.4 while the maximum was found to
be 6662.2. The mean daily consumption of calories was found to be 1756.331 and the standard
deviation was found to be 707.6596.
Fiber consumption per day
shall make use of the model resulting from combination of the two selection criteria. Only
variables contained in the combined model were deemed fit to be analyzed and interpreted for
purposes of this research. The model that shall be used is:
BETAPLASMA = ALCOHOL+ CHOLESTEROL+ AGE+ SEX + CALORIES + FIBER +
VITUSE + RETPLASMA.
The dependent variable for this study was Beta plasma while the independent variables were
alcohol, cholesterol, age, sex, calories, fiber, vituse and retplasma.
Descriptive statistics
This section provides a description of the variables used during the study.
Smoking status
The highest frequency of respondents (49.5%) have never smoked, followed by those that are
former smokers (36.5 per cent) and the least number of respondents are current smokers (14.0%).
Alcohol weekly consumption
Minimum alcohol consumption per week was found to be 0 and the maximum was 517 drinks.
The mean weekly alcohol consumption was about 8.93 drinks.
Cholesterol daily consumption
Daily cholesterol consumption was found to be 0 at minimum and 2308 at maximum. The
average daily cholesterol consumption was found to be about 264.06 for the 315 respondents.
Calories daily consumption
Minimum calories consumption per day was found to be 33.4 while the maximum was found to
be 6662.2. The mean daily consumption of calories was found to be 1756.331 and the standard
deviation was found to be 707.6596.
Fiber consumption per day
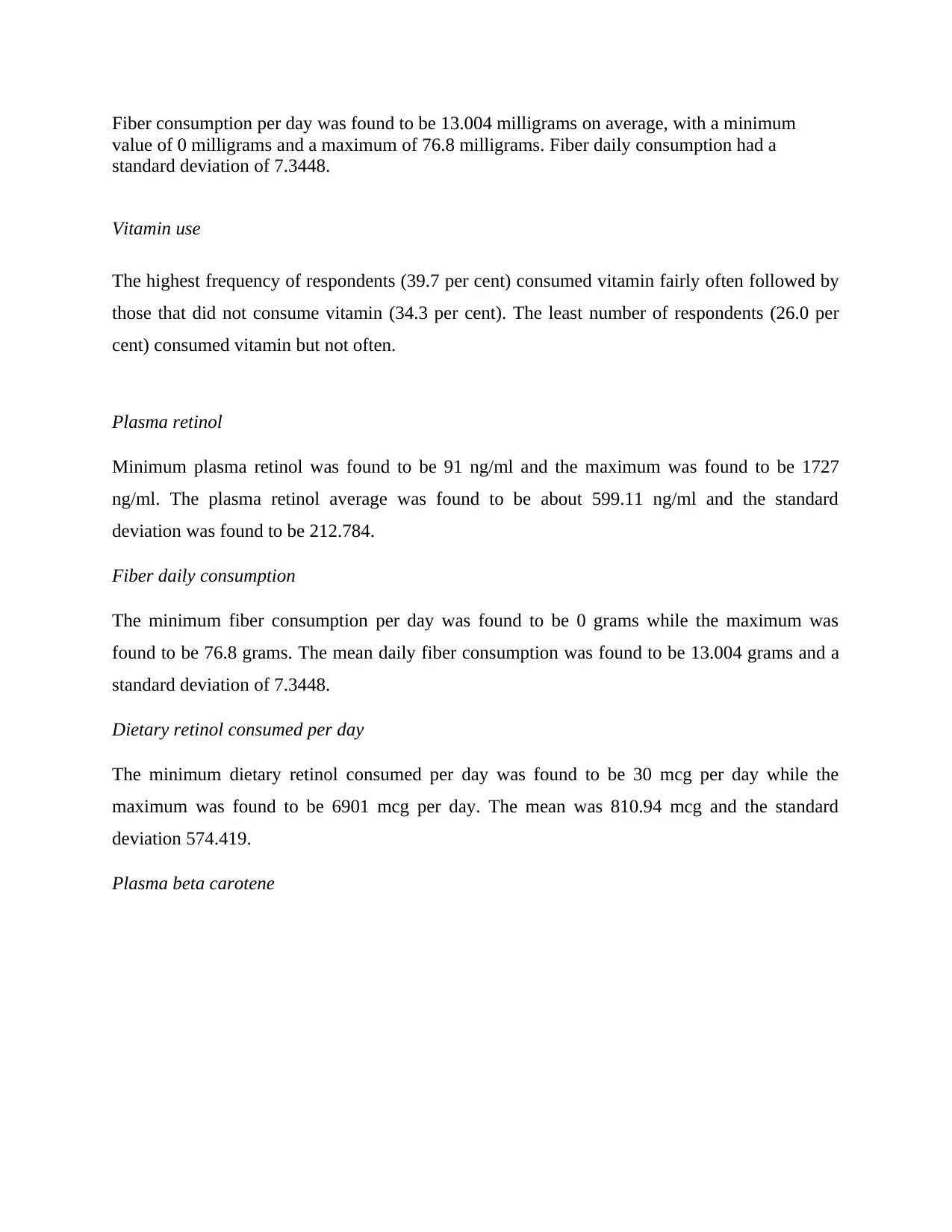
Fiber consumption per day was found to be 13.004 milligrams on average, with a minimum
value of 0 milligrams and a maximum of 76.8 milligrams. Fiber daily consumption had a
standard deviation of 7.3448.
Vitamin use
The highest frequency of respondents (39.7 per cent) consumed vitamin fairly often followed by
those that did not consume vitamin (34.3 per cent). The least number of respondents (26.0 per
cent) consumed vitamin but not often.
Plasma retinol
Minimum plasma retinol was found to be 91 ng/ml and the maximum was found to be 1727
ng/ml. The plasma retinol average was found to be about 599.11 ng/ml and the standard
deviation was found to be 212.784.
Fiber daily consumption
The minimum fiber consumption per day was found to be 0 grams while the maximum was
found to be 76.8 grams. The mean daily fiber consumption was found to be 13.004 grams and a
standard deviation of 7.3448.
Dietary retinol consumed per day
The minimum dietary retinol consumed per day was found to be 30 mcg per day while the
maximum was found to be 6901 mcg per day. The mean was 810.94 mcg and the standard
deviation 574.419.
Plasma beta carotene
value of 0 milligrams and a maximum of 76.8 milligrams. Fiber daily consumption had a
standard deviation of 7.3448.
Vitamin use
The highest frequency of respondents (39.7 per cent) consumed vitamin fairly often followed by
those that did not consume vitamin (34.3 per cent). The least number of respondents (26.0 per
cent) consumed vitamin but not often.
Plasma retinol
Minimum plasma retinol was found to be 91 ng/ml and the maximum was found to be 1727
ng/ml. The plasma retinol average was found to be about 599.11 ng/ml and the standard
deviation was found to be 212.784.
Fiber daily consumption
The minimum fiber consumption per day was found to be 0 grams while the maximum was
found to be 76.8 grams. The mean daily fiber consumption was found to be 13.004 grams and a
standard deviation of 7.3448.
Dietary retinol consumed per day
The minimum dietary retinol consumed per day was found to be 30 mcg per day while the
maximum was found to be 6901 mcg per day. The mean was 810.94 mcg and the standard
deviation 574.419.
Plasma beta carotene
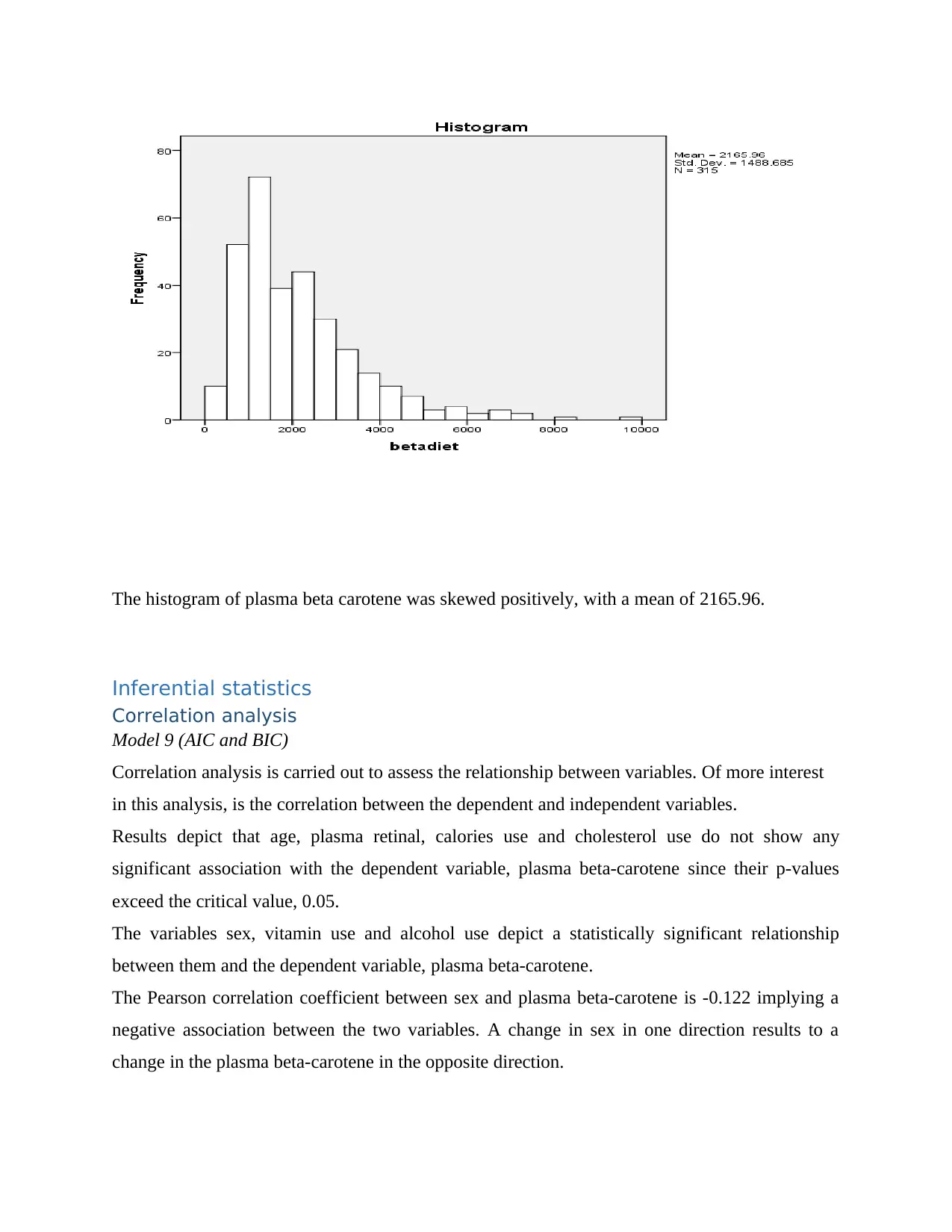
The histogram of plasma beta carotene was skewed positively, with a mean of 2165.96.
Inferential statistics
Correlation analysis
Model 9 (AIC and BIC)
Correlation analysis is carried out to assess the relationship between variables. Of more interest
in this analysis, is the correlation between the dependent and independent variables.
Results depict that age, plasma retinal, calories use and cholesterol use do not show any
significant association with the dependent variable, plasma beta-carotene since their p-values
exceed the critical value, 0.05.
The variables sex, vitamin use and alcohol use depict a statistically significant relationship
between them and the dependent variable, plasma beta-carotene.
The Pearson correlation coefficient between sex and plasma beta-carotene is -0.122 implying a
negative association between the two variables. A change in sex in one direction results to a
change in the plasma beta-carotene in the opposite direction.
Inferential statistics
Correlation analysis
Model 9 (AIC and BIC)
Correlation analysis is carried out to assess the relationship between variables. Of more interest
in this analysis, is the correlation between the dependent and independent variables.
Results depict that age, plasma retinal, calories use and cholesterol use do not show any
significant association with the dependent variable, plasma beta-carotene since their p-values
exceed the critical value, 0.05.
The variables sex, vitamin use and alcohol use depict a statistically significant relationship
between them and the dependent variable, plasma beta-carotene.
The Pearson correlation coefficient between sex and plasma beta-carotene is -0.122 implying a
negative association between the two variables. A change in sex in one direction results to a
change in the plasma beta-carotene in the opposite direction.
Secure Best Marks with AI Grader
Need help grading? Try our AI Grader for instant feedback on your assignments.
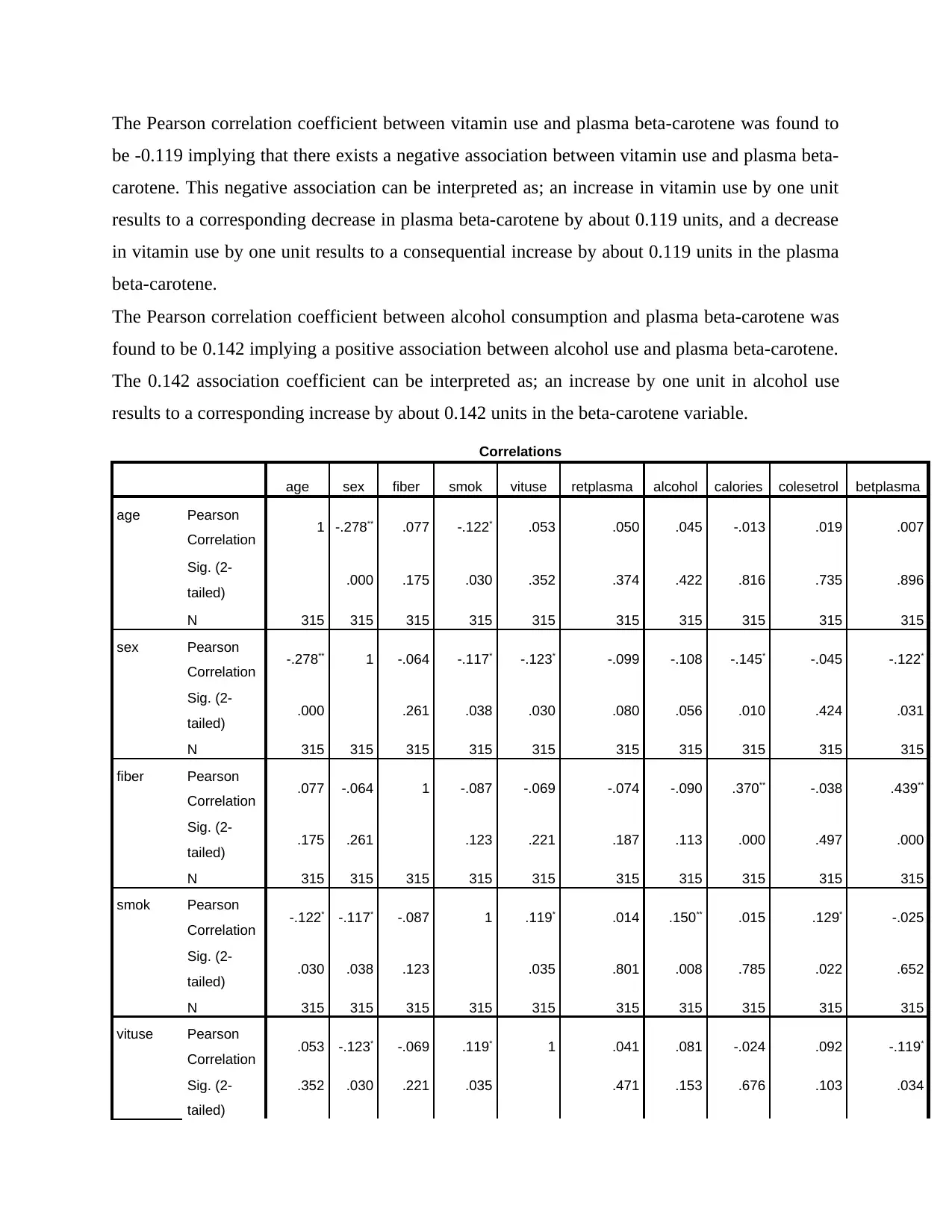
The Pearson correlation coefficient between vitamin use and plasma beta-carotene was found to
be -0.119 implying that there exists a negative association between vitamin use and plasma beta-
carotene. This negative association can be interpreted as; an increase in vitamin use by one unit
results to a corresponding decrease in plasma beta-carotene by about 0.119 units, and a decrease
in vitamin use by one unit results to a consequential increase by about 0.119 units in the plasma
beta-carotene.
The Pearson correlation coefficient between alcohol consumption and plasma beta-carotene was
found to be 0.142 implying a positive association between alcohol use and plasma beta-carotene.
The 0.142 association coefficient can be interpreted as; an increase by one unit in alcohol use
results to a corresponding increase by about 0.142 units in the beta-carotene variable.
Correlations
age sex fiber smok vituse retplasma alcohol calories colesetrol betplasma
age Pearson
Correlation 1 -.278** .077 -.122* .053 .050 .045 -.013 .019 .007
Sig. (2-
tailed) .000 .175 .030 .352 .374 .422 .816 .735 .896
N 315 315 315 315 315 315 315 315 315 315
sex Pearson
Correlation -.278** 1 -.064 -.117* -.123* -.099 -.108 -.145* -.045 -.122*
Sig. (2-
tailed) .000 .261 .038 .030 .080 .056 .010 .424 .031
N 315 315 315 315 315 315 315 315 315 315
fiber Pearson
Correlation .077 -.064 1 -.087 -.069 -.074 -.090 .370** -.038 .439**
Sig. (2-
tailed) .175 .261 .123 .221 .187 .113 .000 .497 .000
N 315 315 315 315 315 315 315 315 315 315
smok Pearson
Correlation -.122* -.117* -.087 1 .119* .014 .150** .015 .129* -.025
Sig. (2-
tailed) .030 .038 .123 .035 .801 .008 .785 .022 .652
N 315 315 315 315 315 315 315 315 315 315
vituse Pearson
Correlation .053 -.123* -.069 .119* 1 .041 .081 -.024 .092 -.119*
Sig. (2-
tailed)
.352 .030 .221 .035 .471 .153 .676 .103 .034
be -0.119 implying that there exists a negative association between vitamin use and plasma beta-
carotene. This negative association can be interpreted as; an increase in vitamin use by one unit
results to a corresponding decrease in plasma beta-carotene by about 0.119 units, and a decrease
in vitamin use by one unit results to a consequential increase by about 0.119 units in the plasma
beta-carotene.
The Pearson correlation coefficient between alcohol consumption and plasma beta-carotene was
found to be 0.142 implying a positive association between alcohol use and plasma beta-carotene.
The 0.142 association coefficient can be interpreted as; an increase by one unit in alcohol use
results to a corresponding increase by about 0.142 units in the beta-carotene variable.
Correlations
age sex fiber smok vituse retplasma alcohol calories colesetrol betplasma
age Pearson
Correlation 1 -.278** .077 -.122* .053 .050 .045 -.013 .019 .007
Sig. (2-
tailed) .000 .175 .030 .352 .374 .422 .816 .735 .896
N 315 315 315 315 315 315 315 315 315 315
sex Pearson
Correlation -.278** 1 -.064 -.117* -.123* -.099 -.108 -.145* -.045 -.122*
Sig. (2-
tailed) .000 .261 .038 .030 .080 .056 .010 .424 .031
N 315 315 315 315 315 315 315 315 315 315
fiber Pearson
Correlation .077 -.064 1 -.087 -.069 -.074 -.090 .370** -.038 .439**
Sig. (2-
tailed) .175 .261 .123 .221 .187 .113 .000 .497 .000
N 315 315 315 315 315 315 315 315 315 315
smok Pearson
Correlation -.122* -.117* -.087 1 .119* .014 .150** .015 .129* -.025
Sig. (2-
tailed) .030 .038 .123 .035 .801 .008 .785 .022 .652
N 315 315 315 315 315 315 315 315 315 315
vituse Pearson
Correlation .053 -.123* -.069 .119* 1 .041 .081 -.024 .092 -.119*
Sig. (2-
tailed)
.352 .030 .221 .035 .471 .153 .676 .103 .034
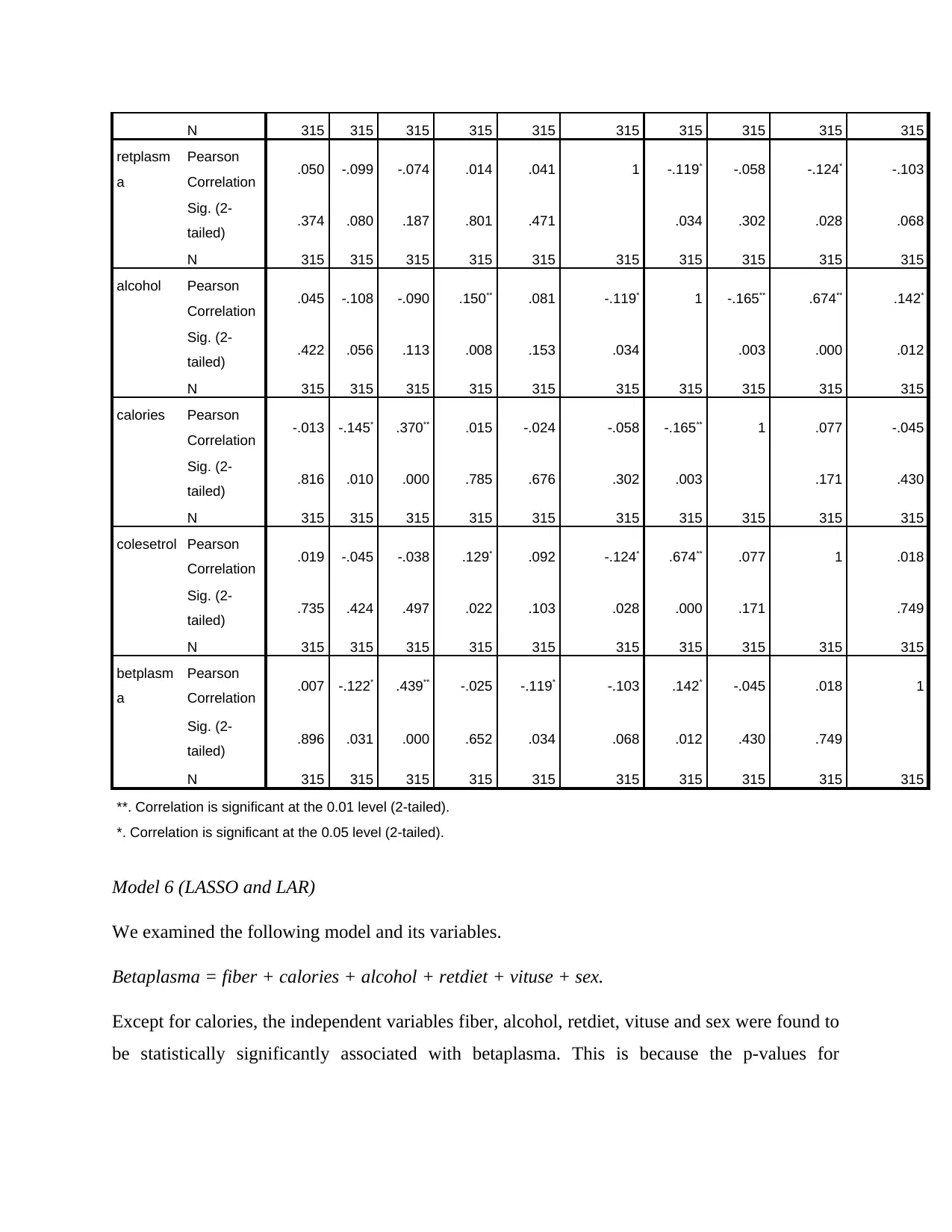
N 315 315 315 315 315 315 315 315 315 315
retplasm
a
Pearson
Correlation .050 -.099 -.074 .014 .041 1 -.119* -.058 -.124* -.103
Sig. (2-
tailed) .374 .080 .187 .801 .471 .034 .302 .028 .068
N 315 315 315 315 315 315 315 315 315 315
alcohol Pearson
Correlation .045 -.108 -.090 .150** .081 -.119* 1 -.165** .674** .142*
Sig. (2-
tailed) .422 .056 .113 .008 .153 .034 .003 .000 .012
N 315 315 315 315 315 315 315 315 315 315
calories Pearson
Correlation -.013 -.145* .370** .015 -.024 -.058 -.165** 1 .077 -.045
Sig. (2-
tailed) .816 .010 .000 .785 .676 .302 .003 .171 .430
N 315 315 315 315 315 315 315 315 315 315
colesetrol Pearson
Correlation .019 -.045 -.038 .129* .092 -.124* .674** .077 1 .018
Sig. (2-
tailed) .735 .424 .497 .022 .103 .028 .000 .171 .749
N 315 315 315 315 315 315 315 315 315 315
betplasm
a
Pearson
Correlation .007 -.122* .439** -.025 -.119* -.103 .142* -.045 .018 1
Sig. (2-
tailed) .896 .031 .000 .652 .034 .068 .012 .430 .749
N 315 315 315 315 315 315 315 315 315 315
**. Correlation is significant at the 0.01 level (2-tailed).
*. Correlation is significant at the 0.05 level (2-tailed).
Model 6 (LASSO and LAR)
We examined the following model and its variables.
Betaplasma = fiber + calories + alcohol + retdiet + vituse + sex.
Except for calories, the independent variables fiber, alcohol, retdiet, vituse and sex were found to
be statistically significantly associated with betaplasma. This is because the p-values for
retplasm
a
Pearson
Correlation .050 -.099 -.074 .014 .041 1 -.119* -.058 -.124* -.103
Sig. (2-
tailed) .374 .080 .187 .801 .471 .034 .302 .028 .068
N 315 315 315 315 315 315 315 315 315 315
alcohol Pearson
Correlation .045 -.108 -.090 .150** .081 -.119* 1 -.165** .674** .142*
Sig. (2-
tailed) .422 .056 .113 .008 .153 .034 .003 .000 .012
N 315 315 315 315 315 315 315 315 315 315
calories Pearson
Correlation -.013 -.145* .370** .015 -.024 -.058 -.165** 1 .077 -.045
Sig. (2-
tailed) .816 .010 .000 .785 .676 .302 .003 .171 .430
N 315 315 315 315 315 315 315 315 315 315
colesetrol Pearson
Correlation .019 -.045 -.038 .129* .092 -.124* .674** .077 1 .018
Sig. (2-
tailed) .735 .424 .497 .022 .103 .028 .000 .171 .749
N 315 315 315 315 315 315 315 315 315 315
betplasm
a
Pearson
Correlation .007 -.122* .439** -.025 -.119* -.103 .142* -.045 .018 1
Sig. (2-
tailed) .896 .031 .000 .652 .034 .068 .012 .430 .749
N 315 315 315 315 315 315 315 315 315 315
**. Correlation is significant at the 0.01 level (2-tailed).
*. Correlation is significant at the 0.05 level (2-tailed).
Model 6 (LASSO and LAR)
We examined the following model and its variables.
Betaplasma = fiber + calories + alcohol + retdiet + vituse + sex.
Except for calories, the independent variables fiber, alcohol, retdiet, vituse and sex were found to
be statistically significantly associated with betaplasma. This is because the p-values for
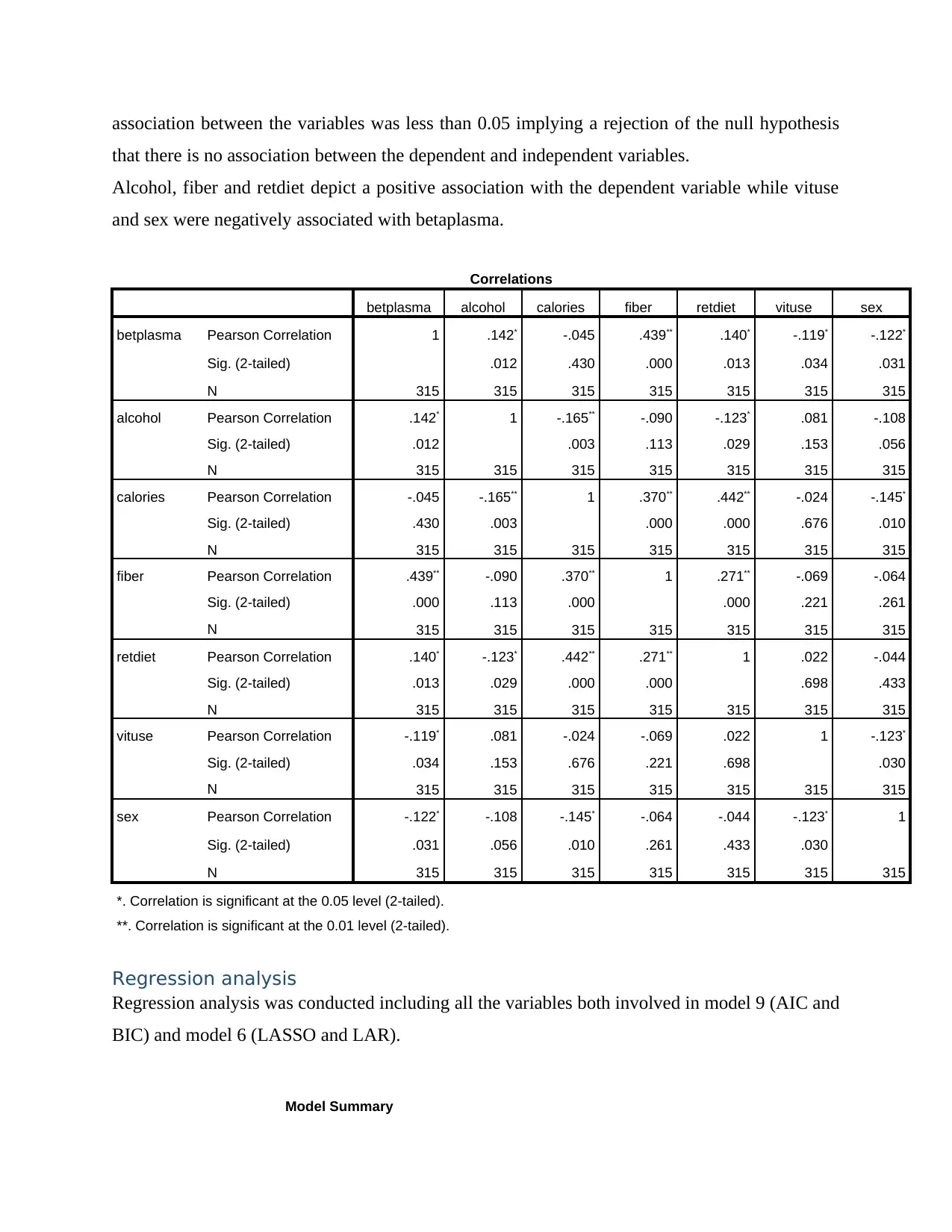
association between the variables was less than 0.05 implying a rejection of the null hypothesis
that there is no association between the dependent and independent variables.
Alcohol, fiber and retdiet depict a positive association with the dependent variable while vituse
and sex were negatively associated with betaplasma.
Correlations
betplasma alcohol calories fiber retdiet vituse sex
betplasma Pearson Correlation 1 .142* -.045 .439** .140* -.119* -.122*
Sig. (2-tailed) .012 .430 .000 .013 .034 .031
N 315 315 315 315 315 315 315
alcohol Pearson Correlation .142* 1 -.165** -.090 -.123* .081 -.108
Sig. (2-tailed) .012 .003 .113 .029 .153 .056
N 315 315 315 315 315 315 315
calories Pearson Correlation -.045 -.165** 1 .370** .442** -.024 -.145*
Sig. (2-tailed) .430 .003 .000 .000 .676 .010
N 315 315 315 315 315 315 315
fiber Pearson Correlation .439** -.090 .370** 1 .271** -.069 -.064
Sig. (2-tailed) .000 .113 .000 .000 .221 .261
N 315 315 315 315 315 315 315
retdiet Pearson Correlation .140* -.123* .442** .271** 1 .022 -.044
Sig. (2-tailed) .013 .029 .000 .000 .698 .433
N 315 315 315 315 315 315 315
vituse Pearson Correlation -.119* .081 -.024 -.069 .022 1 -.123*
Sig. (2-tailed) .034 .153 .676 .221 .698 .030
N 315 315 315 315 315 315 315
sex Pearson Correlation -.122* -.108 -.145* -.064 -.044 -.123* 1
Sig. (2-tailed) .031 .056 .010 .261 .433 .030
N 315 315 315 315 315 315 315
*. Correlation is significant at the 0.05 level (2-tailed).
**. Correlation is significant at the 0.01 level (2-tailed).
Regression analysis
Regression analysis was conducted including all the variables both involved in model 9 (AIC and
BIC) and model 6 (LASSO and LAR).
Model Summary
that there is no association between the dependent and independent variables.
Alcohol, fiber and retdiet depict a positive association with the dependent variable while vituse
and sex were negatively associated with betaplasma.
Correlations
betplasma alcohol calories fiber retdiet vituse sex
betplasma Pearson Correlation 1 .142* -.045 .439** .140* -.119* -.122*
Sig. (2-tailed) .012 .430 .000 .013 .034 .031
N 315 315 315 315 315 315 315
alcohol Pearson Correlation .142* 1 -.165** -.090 -.123* .081 -.108
Sig. (2-tailed) .012 .003 .113 .029 .153 .056
N 315 315 315 315 315 315 315
calories Pearson Correlation -.045 -.165** 1 .370** .442** -.024 -.145*
Sig. (2-tailed) .430 .003 .000 .000 .676 .010
N 315 315 315 315 315 315 315
fiber Pearson Correlation .439** -.090 .370** 1 .271** -.069 -.064
Sig. (2-tailed) .000 .113 .000 .000 .221 .261
N 315 315 315 315 315 315 315
retdiet Pearson Correlation .140* -.123* .442** .271** 1 .022 -.044
Sig. (2-tailed) .013 .029 .000 .000 .698 .433
N 315 315 315 315 315 315 315
vituse Pearson Correlation -.119* .081 -.024 -.069 .022 1 -.123*
Sig. (2-tailed) .034 .153 .676 .221 .698 .030
N 315 315 315 315 315 315 315
sex Pearson Correlation -.122* -.108 -.145* -.064 -.044 -.123* 1
Sig. (2-tailed) .031 .056 .010 .261 .433 .030
N 315 315 315 315 315 315 315
*. Correlation is significant at the 0.05 level (2-tailed).
**. Correlation is significant at the 0.01 level (2-tailed).
Regression analysis
Regression analysis was conducted including all the variables both involved in model 9 (AIC and
BIC) and model 6 (LASSO and LAR).
Model Summary
Paraphrase This Document
Need a fresh take? Get an instant paraphrase of this document with our AI Paraphraser
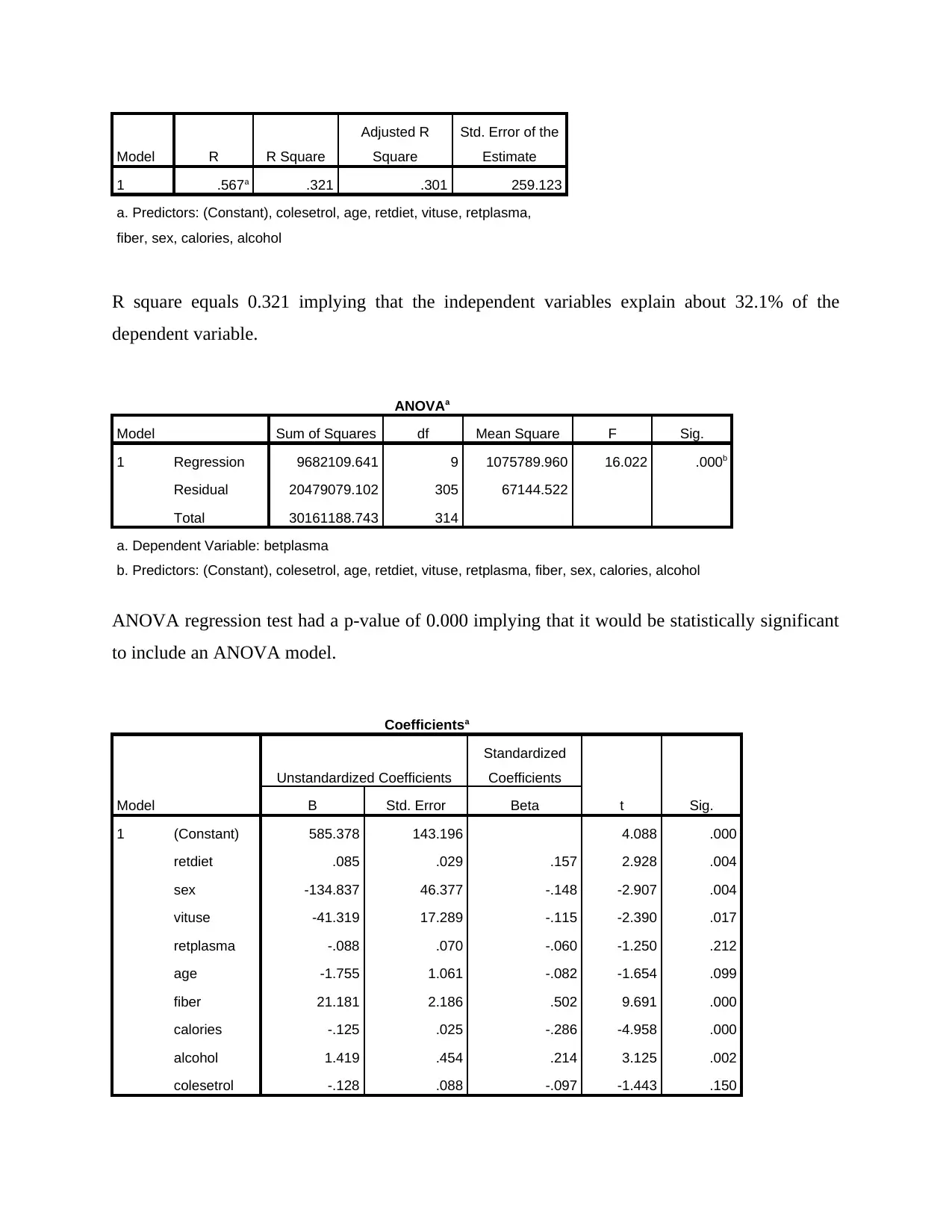
Model R R Square
Adjusted R
Square
Std. Error of the
Estimate
1 .567a .321 .301 259.123
a. Predictors: (Constant), colesetrol, age, retdiet, vituse, retplasma,
fiber, sex, calories, alcohol
R square equals 0.321 implying that the independent variables explain about 32.1% of the
dependent variable.
ANOVAa
Model Sum of Squares df Mean Square F Sig.
1 Regression 9682109.641 9 1075789.960 16.022 .000b
Residual 20479079.102 305 67144.522
Total 30161188.743 314
a. Dependent Variable: betplasma
b. Predictors: (Constant), colesetrol, age, retdiet, vituse, retplasma, fiber, sex, calories, alcohol
ANOVA regression test had a p-value of 0.000 implying that it would be statistically significant
to include an ANOVA model.
Coefficientsa
Model
Unstandardized Coefficients
Standardized
Coefficients
t Sig.B Std. Error Beta
1 (Constant) 585.378 143.196 4.088 .000
retdiet .085 .029 .157 2.928 .004
sex -134.837 46.377 -.148 -2.907 .004
vituse -41.319 17.289 -.115 -2.390 .017
retplasma -.088 .070 -.060 -1.250 .212
age -1.755 1.061 -.082 -1.654 .099
fiber 21.181 2.186 .502 9.691 .000
calories -.125 .025 -.286 -4.958 .000
alcohol 1.419 .454 .214 3.125 .002
colesetrol -.128 .088 -.097 -1.443 .150
Adjusted R
Square
Std. Error of the
Estimate
1 .567a .321 .301 259.123
a. Predictors: (Constant), colesetrol, age, retdiet, vituse, retplasma,
fiber, sex, calories, alcohol
R square equals 0.321 implying that the independent variables explain about 32.1% of the
dependent variable.
ANOVAa
Model Sum of Squares df Mean Square F Sig.
1 Regression 9682109.641 9 1075789.960 16.022 .000b
Residual 20479079.102 305 67144.522
Total 30161188.743 314
a. Dependent Variable: betplasma
b. Predictors: (Constant), colesetrol, age, retdiet, vituse, retplasma, fiber, sex, calories, alcohol
ANOVA regression test had a p-value of 0.000 implying that it would be statistically significant
to include an ANOVA model.
Coefficientsa
Model
Unstandardized Coefficients
Standardized
Coefficients
t Sig.B Std. Error Beta
1 (Constant) 585.378 143.196 4.088 .000
retdiet .085 .029 .157 2.928 .004
sex -134.837 46.377 -.148 -2.907 .004
vituse -41.319 17.289 -.115 -2.390 .017
retplasma -.088 .070 -.060 -1.250 .212
age -1.755 1.061 -.082 -1.654 .099
fiber 21.181 2.186 .502 9.691 .000
calories -.125 .025 -.286 -4.958 .000
alcohol 1.419 .454 .214 3.125 .002
colesetrol -.128 .088 -.097 -1.443 .150
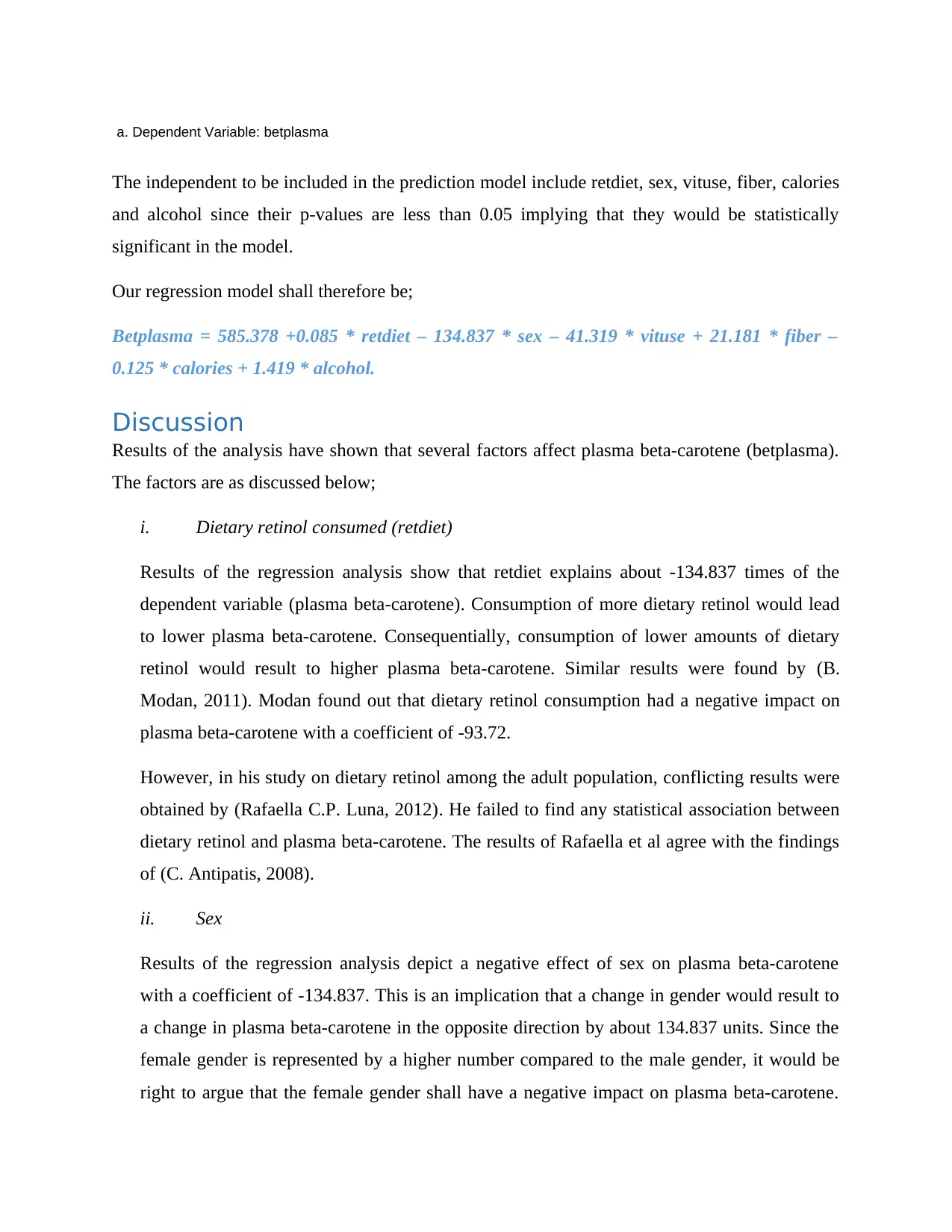
a. Dependent Variable: betplasma
The independent to be included in the prediction model include retdiet, sex, vituse, fiber, calories
and alcohol since their p-values are less than 0.05 implying that they would be statistically
significant in the model.
Our regression model shall therefore be;
Betplasma = 585.378 +0.085 * retdiet – 134.837 * sex – 41.319 * vituse + 21.181 * fiber –
0.125 * calories + 1.419 * alcohol.
Discussion
Results of the analysis have shown that several factors affect plasma beta-carotene (betplasma).
The factors are as discussed below;
i. Dietary retinol consumed (retdiet)
Results of the regression analysis show that retdiet explains about -134.837 times of the
dependent variable (plasma beta-carotene). Consumption of more dietary retinol would lead
to lower plasma beta-carotene. Consequentially, consumption of lower amounts of dietary
retinol would result to higher plasma beta-carotene. Similar results were found by (B.
Modan, 2011). Modan found out that dietary retinol consumption had a negative impact on
plasma beta-carotene with a coefficient of -93.72.
However, in his study on dietary retinol among the adult population, conflicting results were
obtained by (Rafaella C.P. Luna, 2012). He failed to find any statistical association between
dietary retinol and plasma beta-carotene. The results of Rafaella et al agree with the findings
of (C. Antipatis, 2008).
ii. Sex
Results of the regression analysis depict a negative effect of sex on plasma beta-carotene
with a coefficient of -134.837. This is an implication that a change in gender would result to
a change in plasma beta-carotene in the opposite direction by about 134.837 units. Since the
female gender is represented by a higher number compared to the male gender, it would be
right to argue that the female gender shall have a negative impact on plasma beta-carotene.
The independent to be included in the prediction model include retdiet, sex, vituse, fiber, calories
and alcohol since their p-values are less than 0.05 implying that they would be statistically
significant in the model.
Our regression model shall therefore be;
Betplasma = 585.378 +0.085 * retdiet – 134.837 * sex – 41.319 * vituse + 21.181 * fiber –
0.125 * calories + 1.419 * alcohol.
Discussion
Results of the analysis have shown that several factors affect plasma beta-carotene (betplasma).
The factors are as discussed below;
i. Dietary retinol consumed (retdiet)
Results of the regression analysis show that retdiet explains about -134.837 times of the
dependent variable (plasma beta-carotene). Consumption of more dietary retinol would lead
to lower plasma beta-carotene. Consequentially, consumption of lower amounts of dietary
retinol would result to higher plasma beta-carotene. Similar results were found by (B.
Modan, 2011). Modan found out that dietary retinol consumption had a negative impact on
plasma beta-carotene with a coefficient of -93.72.
However, in his study on dietary retinol among the adult population, conflicting results were
obtained by (Rafaella C.P. Luna, 2012). He failed to find any statistical association between
dietary retinol and plasma beta-carotene. The results of Rafaella et al agree with the findings
of (C. Antipatis, 2008).
ii. Sex
Results of the regression analysis depict a negative effect of sex on plasma beta-carotene
with a coefficient of -134.837. This is an implication that a change in gender would result to
a change in plasma beta-carotene in the opposite direction by about 134.837 units. Since the
female gender is represented by a higher number compared to the male gender, it would be
right to argue that the female gender shall have a negative impact on plasma beta-carotene.
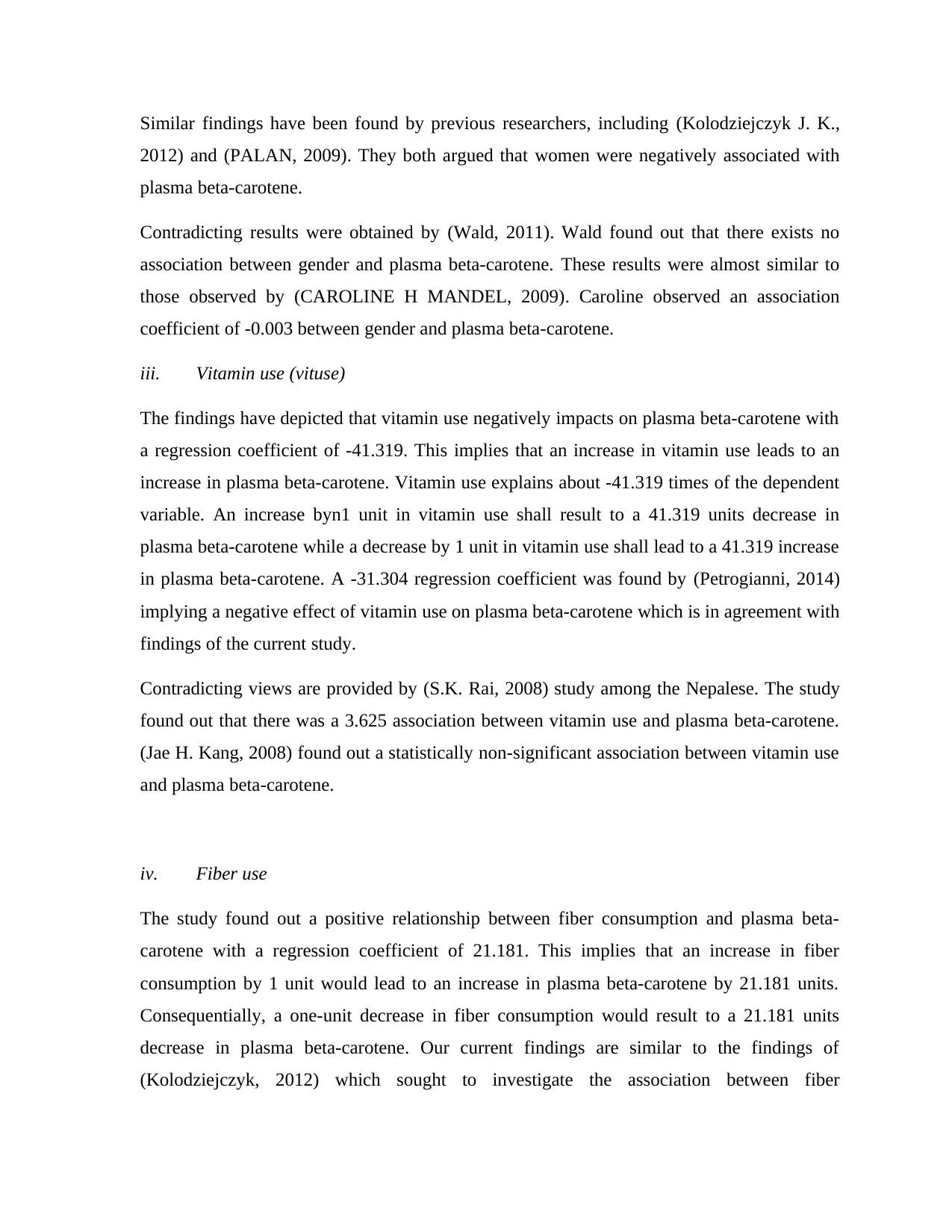
Similar findings have been found by previous researchers, including (Kolodziejczyk J. K.,
2012) and (PALAN, 2009). They both argued that women were negatively associated with
plasma beta-carotene.
Contradicting results were obtained by (Wald, 2011). Wald found out that there exists no
association between gender and plasma beta-carotene. These results were almost similar to
those observed by (CAROLINE H MANDEL, 2009). Caroline observed an association
coefficient of -0.003 between gender and plasma beta-carotene.
iii. Vitamin use (vituse)
The findings have depicted that vitamin use negatively impacts on plasma beta-carotene with
a regression coefficient of -41.319. This implies that an increase in vitamin use leads to an
increase in plasma beta-carotene. Vitamin use explains about -41.319 times of the dependent
variable. An increase byn1 unit in vitamin use shall result to a 41.319 units decrease in
plasma beta-carotene while a decrease by 1 unit in vitamin use shall lead to a 41.319 increase
in plasma beta-carotene. A -31.304 regression coefficient was found by (Petrogianni, 2014)
implying a negative effect of vitamin use on plasma beta-carotene which is in agreement with
findings of the current study.
Contradicting views are provided by (S.K. Rai, 2008) study among the Nepalese. The study
found out that there was a 3.625 association between vitamin use and plasma beta-carotene.
(Jae H. Kang, 2008) found out a statistically non-significant association between vitamin use
and plasma beta-carotene.
iv. Fiber use
The study found out a positive relationship between fiber consumption and plasma beta-
carotene with a regression coefficient of 21.181. This implies that an increase in fiber
consumption by 1 unit would lead to an increase in plasma beta-carotene by 21.181 units.
Consequentially, a one-unit decrease in fiber consumption would result to a 21.181 units
decrease in plasma beta-carotene. Our current findings are similar to the findings of
(Kolodziejczyk, 2012) which sought to investigate the association between fiber
2012) and (PALAN, 2009). They both argued that women were negatively associated with
plasma beta-carotene.
Contradicting results were obtained by (Wald, 2011). Wald found out that there exists no
association between gender and plasma beta-carotene. These results were almost similar to
those observed by (CAROLINE H MANDEL, 2009). Caroline observed an association
coefficient of -0.003 between gender and plasma beta-carotene.
iii. Vitamin use (vituse)
The findings have depicted that vitamin use negatively impacts on plasma beta-carotene with
a regression coefficient of -41.319. This implies that an increase in vitamin use leads to an
increase in plasma beta-carotene. Vitamin use explains about -41.319 times of the dependent
variable. An increase byn1 unit in vitamin use shall result to a 41.319 units decrease in
plasma beta-carotene while a decrease by 1 unit in vitamin use shall lead to a 41.319 increase
in plasma beta-carotene. A -31.304 regression coefficient was found by (Petrogianni, 2014)
implying a negative effect of vitamin use on plasma beta-carotene which is in agreement with
findings of the current study.
Contradicting views are provided by (S.K. Rai, 2008) study among the Nepalese. The study
found out that there was a 3.625 association between vitamin use and plasma beta-carotene.
(Jae H. Kang, 2008) found out a statistically non-significant association between vitamin use
and plasma beta-carotene.
iv. Fiber use
The study found out a positive relationship between fiber consumption and plasma beta-
carotene with a regression coefficient of 21.181. This implies that an increase in fiber
consumption by 1 unit would lead to an increase in plasma beta-carotene by 21.181 units.
Consequentially, a one-unit decrease in fiber consumption would result to a 21.181 units
decrease in plasma beta-carotene. Our current findings are similar to the findings of
(Kolodziejczyk, 2012) which sought to investigate the association between fiber
Secure Best Marks with AI Grader
Need help grading? Try our AI Grader for instant feedback on your assignments.
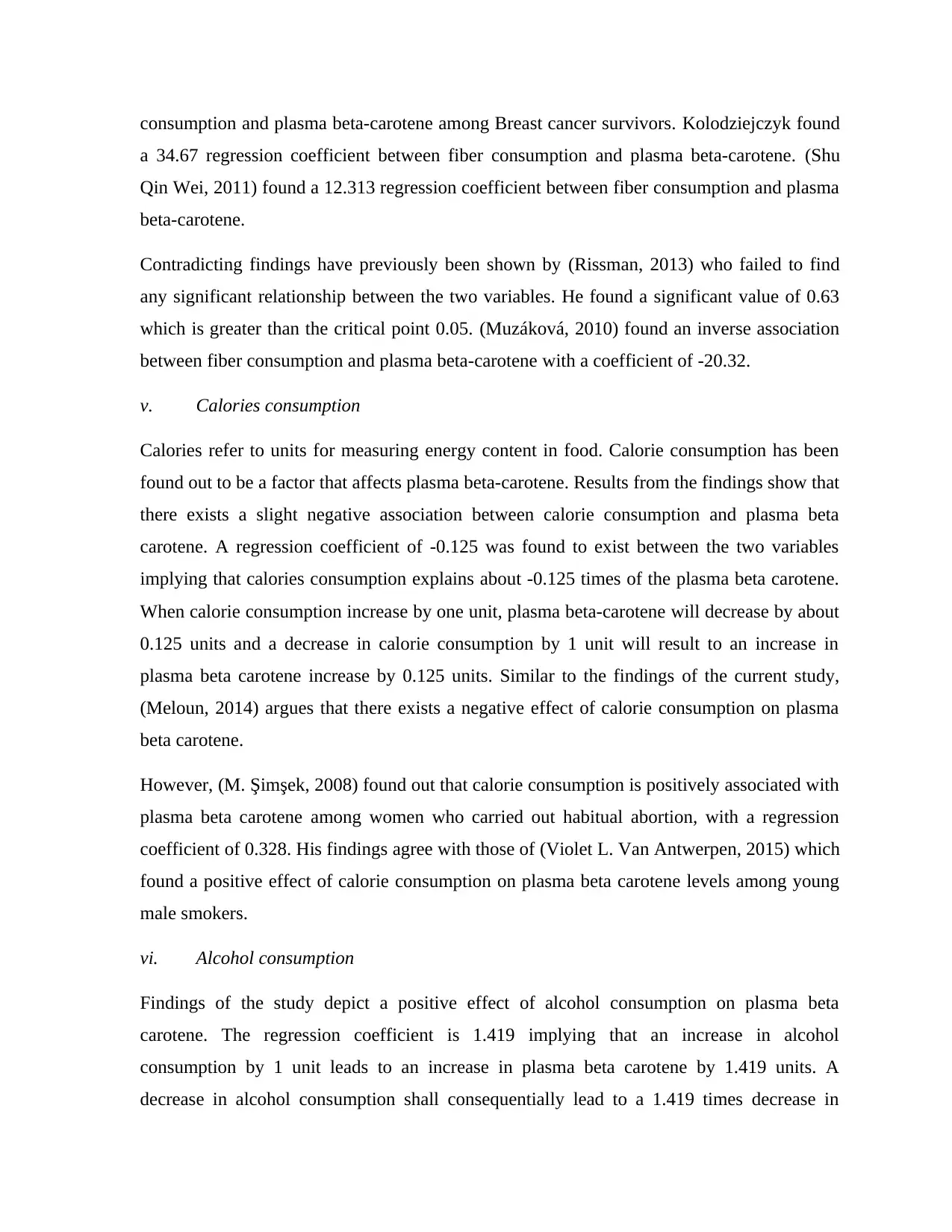
consumption and plasma beta-carotene among Breast cancer survivors. Kolodziejczyk found
a 34.67 regression coefficient between fiber consumption and plasma beta-carotene. (Shu
Qin Wei, 2011) found a 12.313 regression coefficient between fiber consumption and plasma
beta-carotene.
Contradicting findings have previously been shown by (Rissman, 2013) who failed to find
any significant relationship between the two variables. He found a significant value of 0.63
which is greater than the critical point 0.05. (Muzáková, 2010) found an inverse association
between fiber consumption and plasma beta-carotene with a coefficient of -20.32.
v. Calories consumption
Calories refer to units for measuring energy content in food. Calorie consumption has been
found out to be a factor that affects plasma beta-carotene. Results from the findings show that
there exists a slight negative association between calorie consumption and plasma beta
carotene. A regression coefficient of -0.125 was found to exist between the two variables
implying that calories consumption explains about -0.125 times of the plasma beta carotene.
When calorie consumption increase by one unit, plasma beta-carotene will decrease by about
0.125 units and a decrease in calorie consumption by 1 unit will result to an increase in
plasma beta carotene increase by 0.125 units. Similar to the findings of the current study,
(Meloun, 2014) argues that there exists a negative effect of calorie consumption on plasma
beta carotene.
However, (M. ÅžimÅŸek, 2008) found out that calorie consumption is positively associated with
plasma beta carotene among women who carried out habitual abortion, with a regression
coefficient of 0.328. His findings agree with those of (Violet L. Van Antwerpen, 2015) which
found a positive effect of calorie consumption on plasma beta carotene levels among young
male smokers.
vi. Alcohol consumption
Findings of the study depict a positive effect of alcohol consumption on plasma beta
carotene. The regression coefficient is 1.419 implying that an increase in alcohol
consumption by 1 unit leads to an increase in plasma beta carotene by 1.419 units. A
decrease in alcohol consumption shall consequentially lead to a 1.419 times decrease in
a 34.67 regression coefficient between fiber consumption and plasma beta-carotene. (Shu
Qin Wei, 2011) found a 12.313 regression coefficient between fiber consumption and plasma
beta-carotene.
Contradicting findings have previously been shown by (Rissman, 2013) who failed to find
any significant relationship between the two variables. He found a significant value of 0.63
which is greater than the critical point 0.05. (Muzáková, 2010) found an inverse association
between fiber consumption and plasma beta-carotene with a coefficient of -20.32.
v. Calories consumption
Calories refer to units for measuring energy content in food. Calorie consumption has been
found out to be a factor that affects plasma beta-carotene. Results from the findings show that
there exists a slight negative association between calorie consumption and plasma beta
carotene. A regression coefficient of -0.125 was found to exist between the two variables
implying that calories consumption explains about -0.125 times of the plasma beta carotene.
When calorie consumption increase by one unit, plasma beta-carotene will decrease by about
0.125 units and a decrease in calorie consumption by 1 unit will result to an increase in
plasma beta carotene increase by 0.125 units. Similar to the findings of the current study,
(Meloun, 2014) argues that there exists a negative effect of calorie consumption on plasma
beta carotene.
However, (M. ÅžimÅŸek, 2008) found out that calorie consumption is positively associated with
plasma beta carotene among women who carried out habitual abortion, with a regression
coefficient of 0.328. His findings agree with those of (Violet L. Van Antwerpen, 2015) which
found a positive effect of calorie consumption on plasma beta carotene levels among young
male smokers.
vi. Alcohol consumption
Findings of the study depict a positive effect of alcohol consumption on plasma beta
carotene. The regression coefficient is 1.419 implying that an increase in alcohol
consumption by 1 unit leads to an increase in plasma beta carotene by 1.419 units. A
decrease in alcohol consumption shall consequentially lead to a 1.419 times decrease in
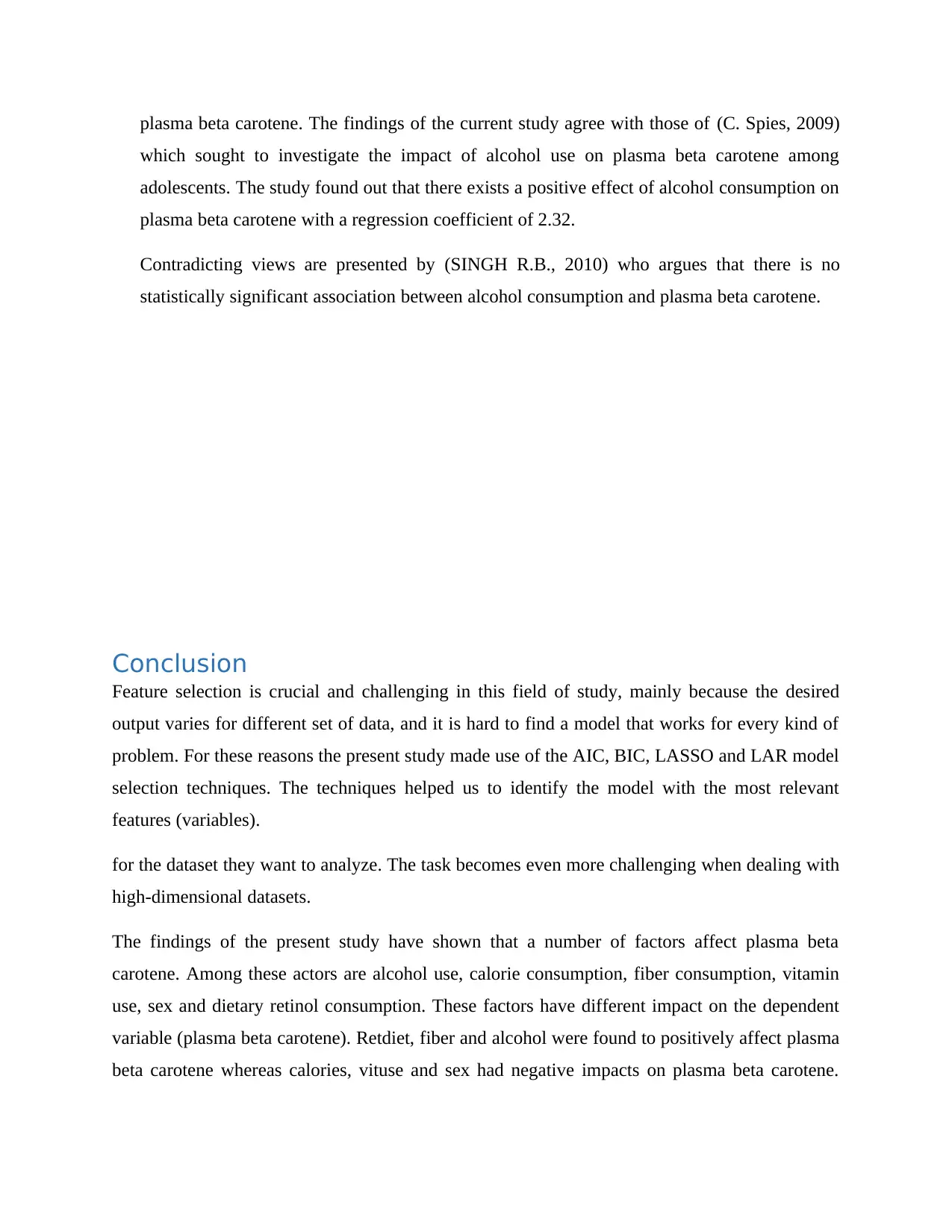
plasma beta carotene. The findings of the current study agree with those of (C. Spies, 2009)
which sought to investigate the impact of alcohol use on plasma beta carotene among
adolescents. The study found out that there exists a positive effect of alcohol consumption on
plasma beta carotene with a regression coefficient of 2.32.
Contradicting views are presented by (SINGH R.B., 2010) who argues that there is no
statistically significant association between alcohol consumption and plasma beta carotene.
Conclusion
Feature selection is crucial and challenging in this field of study, mainly because the desired
output varies for different set of data, and it is hard to find a model that works for every kind of
problem. For these reasons the present study made use of the AIC, BIC, LASSO and LAR model
selection techniques. The techniques helped us to identify the model with the most relevant
features (variables).
for the dataset they want to analyze. The task becomes even more challenging when dealing with
high-dimensional datasets.
The findings of the present study have shown that a number of factors affect plasma beta
carotene. Among these actors are alcohol use, calorie consumption, fiber consumption, vitamin
use, sex and dietary retinol consumption. These factors have different impact on the dependent
variable (plasma beta carotene). Retdiet, fiber and alcohol were found to positively affect plasma
beta carotene whereas calories, vituse and sex had negative impacts on plasma beta carotene.
which sought to investigate the impact of alcohol use on plasma beta carotene among
adolescents. The study found out that there exists a positive effect of alcohol consumption on
plasma beta carotene with a regression coefficient of 2.32.
Contradicting views are presented by (SINGH R.B., 2010) who argues that there is no
statistically significant association between alcohol consumption and plasma beta carotene.
Conclusion
Feature selection is crucial and challenging in this field of study, mainly because the desired
output varies for different set of data, and it is hard to find a model that works for every kind of
problem. For these reasons the present study made use of the AIC, BIC, LASSO and LAR model
selection techniques. The techniques helped us to identify the model with the most relevant
features (variables).
for the dataset they want to analyze. The task becomes even more challenging when dealing with
high-dimensional datasets.
The findings of the present study have shown that a number of factors affect plasma beta
carotene. Among these actors are alcohol use, calorie consumption, fiber consumption, vitamin
use, sex and dietary retinol consumption. These factors have different impact on the dependent
variable (plasma beta carotene). Retdiet, fiber and alcohol were found to positively affect plasma
beta carotene whereas calories, vituse and sex had negative impacts on plasma beta carotene.
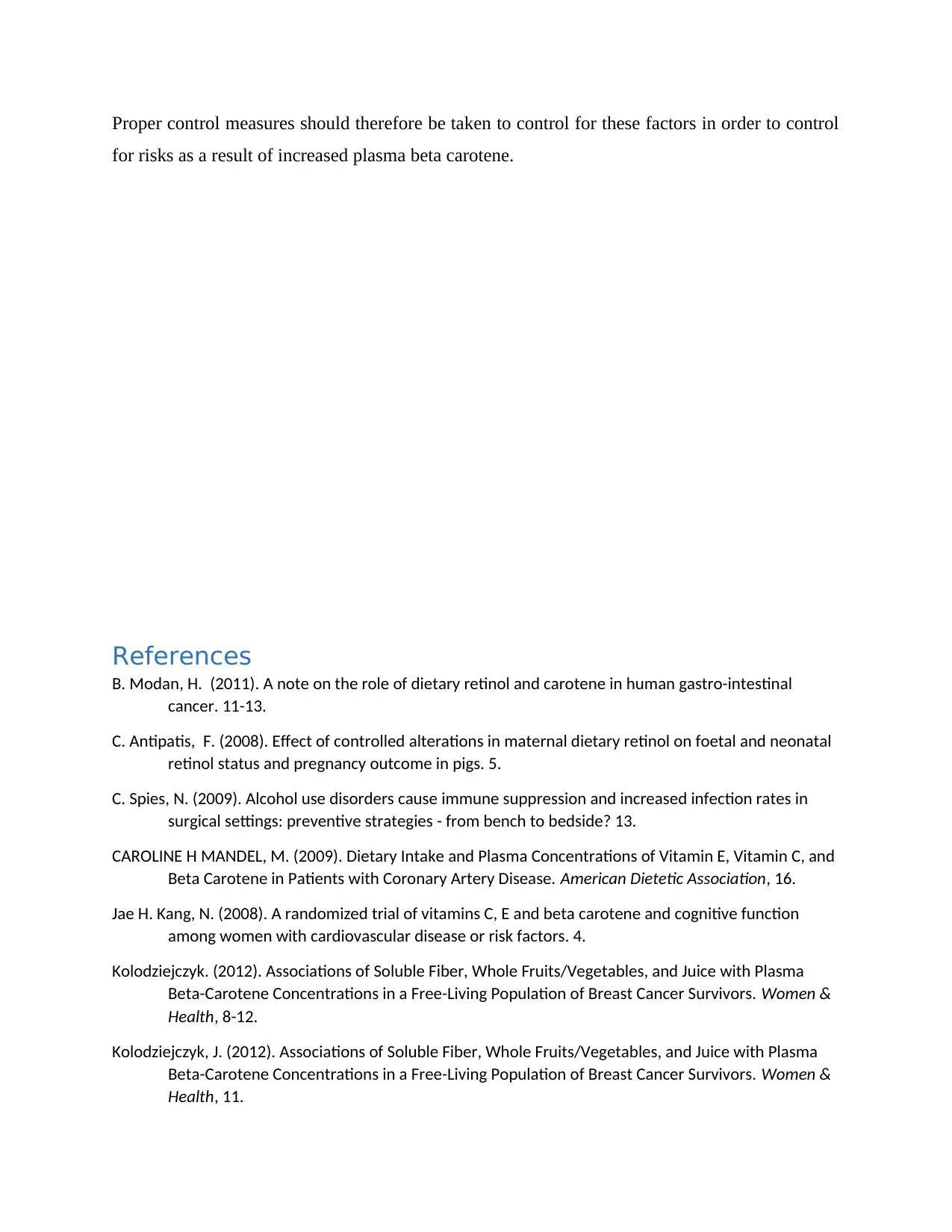
Proper control measures should therefore be taken to control for these factors in order to control
for risks as a result of increased plasma beta carotene.
References
B. Modan, H. (2011). A note on the role of dietary retinol and carotene in human gastro-intestinal
cancer. 11-13.
C. Antipatis, F. (2008). Effect of controlled alterations in maternal dietary retinol on foetal and neonatal
retinol status and pregnancy outcome in pigs. 5.
C. Spies, N. (2009). Alcohol use disorders cause immune suppression and increased infection rates in
surgical settings: preventive strategies - from bench to bedside? 13.
CAROLINE H MANDEL, M. (2009). Dietary Intake and Plasma Concentrations of Vitamin E, Vitamin C, and
Beta Carotene in Patients with Coronary Artery Disease. American Dietetic Association, 16.
Jae H. Kang, N. (2008). A randomized trial of vitamins C, E and beta carotene and cognitive function
among women with cardiovascular disease or risk factors. 4.
Kolodziejczyk. (2012). Associations of Soluble Fiber, Whole Fruits/Vegetables, and Juice with Plasma
Beta-Carotene Concentrations in a Free-Living Population of Breast Cancer Survivors. Women &
Health, 8-12.
Kolodziejczyk, J. (2012). Associations of Soluble Fiber, Whole Fruits/Vegetables, and Juice with Plasma
Beta-Carotene Concentrations in a Free-Living Population of Breast Cancer Survivors. Women &
Health, 11.
for risks as a result of increased plasma beta carotene.
References
B. Modan, H. (2011). A note on the role of dietary retinol and carotene in human gastro-intestinal
cancer. 11-13.
C. Antipatis, F. (2008). Effect of controlled alterations in maternal dietary retinol on foetal and neonatal
retinol status and pregnancy outcome in pigs. 5.
C. Spies, N. (2009). Alcohol use disorders cause immune suppression and increased infection rates in
surgical settings: preventive strategies - from bench to bedside? 13.
CAROLINE H MANDEL, M. (2009). Dietary Intake and Plasma Concentrations of Vitamin E, Vitamin C, and
Beta Carotene in Patients with Coronary Artery Disease. American Dietetic Association, 16.
Jae H. Kang, N. (2008). A randomized trial of vitamins C, E and beta carotene and cognitive function
among women with cardiovascular disease or risk factors. 4.
Kolodziejczyk. (2012). Associations of Soluble Fiber, Whole Fruits/Vegetables, and Juice with Plasma
Beta-Carotene Concentrations in a Free-Living Population of Breast Cancer Survivors. Women &
Health, 8-12.
Kolodziejczyk, J. (2012). Associations of Soluble Fiber, Whole Fruits/Vegetables, and Juice with Plasma
Beta-Carotene Concentrations in a Free-Living Population of Breast Cancer Survivors. Women &
Health, 11.
Paraphrase This Document
Need a fresh take? Get an instant paraphrase of this document with our AI Paraphraser
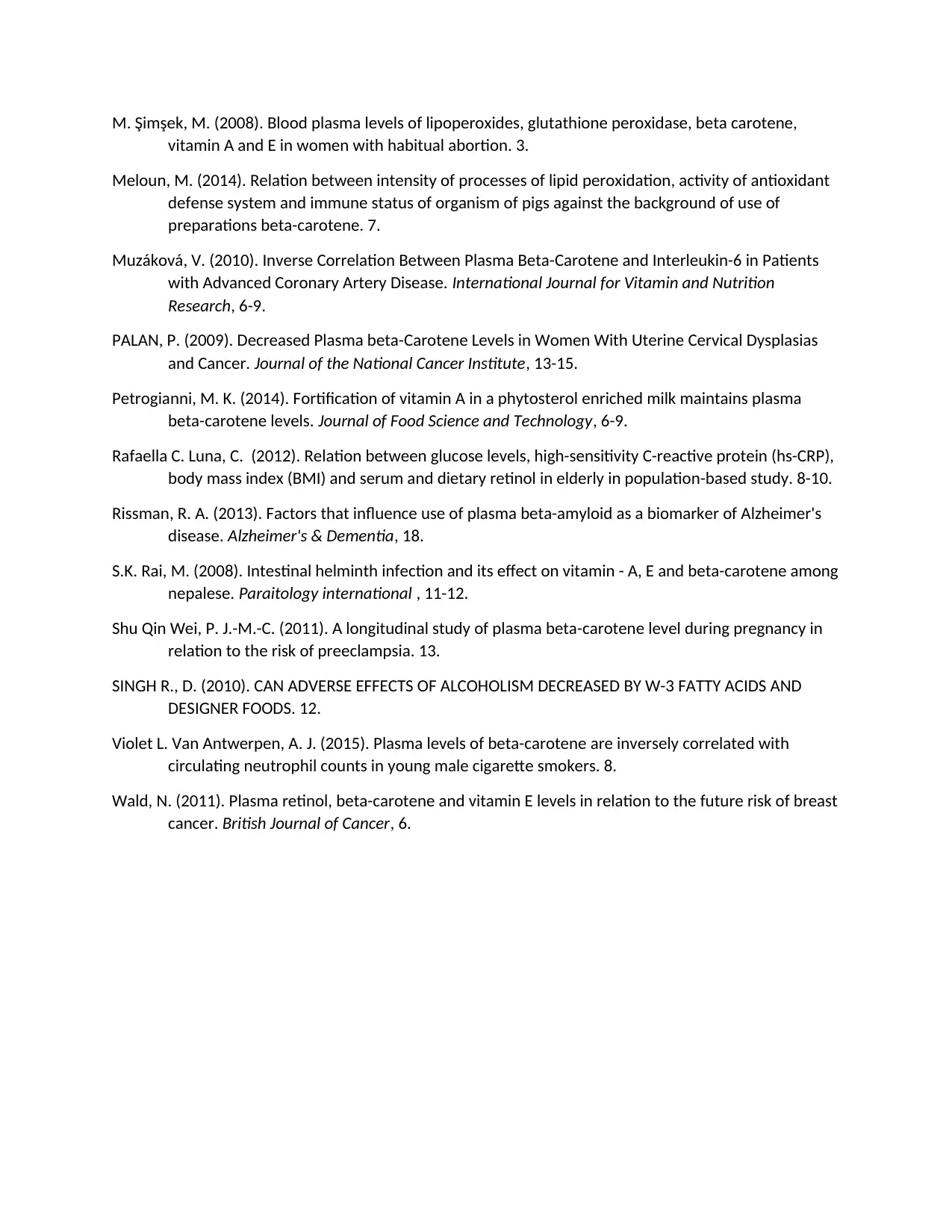
M. ÅžimÅŸek, M. (2008). Blood plasma levels of lipoperoxides, glutathione peroxidase, beta carotene,
vitamin A and E in women with habitual abortion. 3.
Meloun, M. (2014). Relation between intensity of processes of lipid peroxidation, activity of antioxidant
defense system and immune status of organism of pigs against the background of use of
preparations beta-carotene. 7.
Muzáková, V. (2010). Inverse Correlation Between Plasma Beta-Carotene and Interleukin-6 in Patients
with Advanced Coronary Artery Disease. International Journal for Vitamin and Nutrition
Research, 6-9.
PALAN, P. (2009). Decreased Plasma beta-Carotene Levels in Women With Uterine Cervical Dysplasias
and Cancer. Journal of the National Cancer Institute, 13-15.
Petrogianni, M. K. (2014). Fortification of vitamin A in a phytosterol enriched milk maintains plasma
beta-carotene levels. Journal of Food Science and Technology, 6-9.
Rafaella C. Luna, C. (2012). Relation between glucose levels, high-sensitivity C-reactive protein (hs-CRP),
body mass index (BMI) and serum and dietary retinol in elderly in population-based study. 8-10.
Rissman, R. A. (2013). Factors that influence use of plasma beta-amyloid as a biomarker of Alzheimer's
disease. Alzheimer's & Dementia, 18.
S.K. Rai, M. (2008). Intestinal helminth infection and its effect on vitamin - A, E and beta-carotene among
nepalese. Paraitology international , 11-12.
Shu Qin Wei, P. J.-M.-C. (2011). A longitudinal study of plasma beta-carotene level during pregnancy in
relation to the risk of preeclampsia. 13.
SINGH R., D. (2010). CAN ADVERSE EFFECTS OF ALCOHOLISM DECREASED BY W-3 FATTY ACIDS AND
DESIGNER FOODS. 12.
Violet L. Van Antwerpen, A. J. (2015). Plasma levels of beta-carotene are inversely correlated with
circulating neutrophil counts in young male cigarette smokers. 8.
Wald, N. (2011). Plasma retinol, beta-carotene and vitamin E levels in relation to the future risk of breast
cancer. British Journal of Cancer, 6.
vitamin A and E in women with habitual abortion. 3.
Meloun, M. (2014). Relation between intensity of processes of lipid peroxidation, activity of antioxidant
defense system and immune status of organism of pigs against the background of use of
preparations beta-carotene. 7.
Muzáková, V. (2010). Inverse Correlation Between Plasma Beta-Carotene and Interleukin-6 in Patients
with Advanced Coronary Artery Disease. International Journal for Vitamin and Nutrition
Research, 6-9.
PALAN, P. (2009). Decreased Plasma beta-Carotene Levels in Women With Uterine Cervical Dysplasias
and Cancer. Journal of the National Cancer Institute, 13-15.
Petrogianni, M. K. (2014). Fortification of vitamin A in a phytosterol enriched milk maintains plasma
beta-carotene levels. Journal of Food Science and Technology, 6-9.
Rafaella C. Luna, C. (2012). Relation between glucose levels, high-sensitivity C-reactive protein (hs-CRP),
body mass index (BMI) and serum and dietary retinol in elderly in population-based study. 8-10.
Rissman, R. A. (2013). Factors that influence use of plasma beta-amyloid as a biomarker of Alzheimer's
disease. Alzheimer's & Dementia, 18.
S.K. Rai, M. (2008). Intestinal helminth infection and its effect on vitamin - A, E and beta-carotene among
nepalese. Paraitology international , 11-12.
Shu Qin Wei, P. J.-M.-C. (2011). A longitudinal study of plasma beta-carotene level during pregnancy in
relation to the risk of preeclampsia. 13.
SINGH R., D. (2010). CAN ADVERSE EFFECTS OF ALCOHOLISM DECREASED BY W-3 FATTY ACIDS AND
DESIGNER FOODS. 12.
Violet L. Van Antwerpen, A. J. (2015). Plasma levels of beta-carotene are inversely correlated with
circulating neutrophil counts in young male cigarette smokers. 8.
Wald, N. (2011). Plasma retinol, beta-carotene and vitamin E levels in relation to the future risk of breast
cancer. British Journal of Cancer, 6.
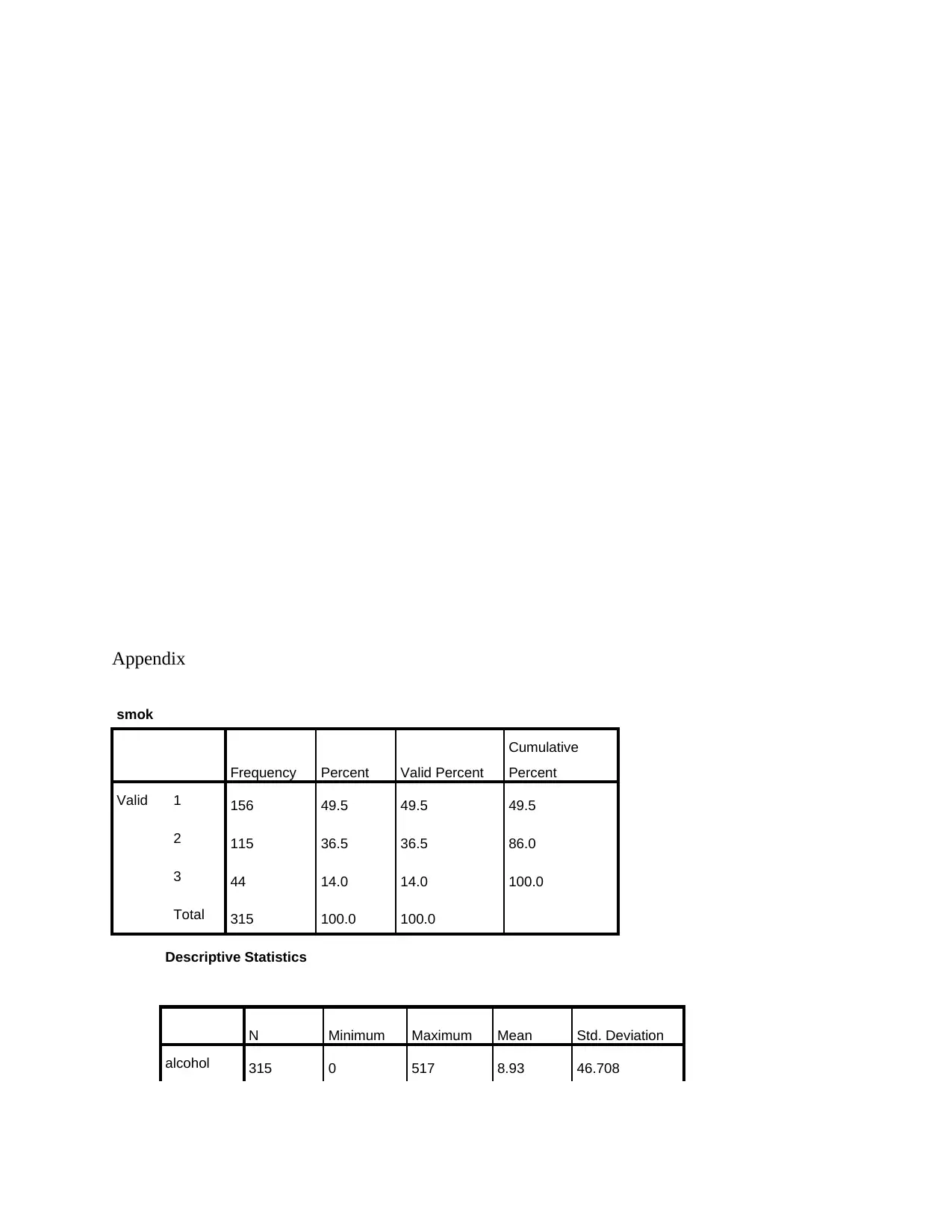
Appendix
smok
Frequency Percent Valid Percent
Cumulative
Percent
Valid 1 156 49.5 49.5 49.5
2 115 36.5 36.5 86.0
3 44 14.0 14.0 100.0
Total 315 100.0 100.0
Descriptive Statistics
N Minimum Maximum Mean Std. Deviation
alcohol 315 0 517 8.93 46.708
smok
Frequency Percent Valid Percent
Cumulative
Percent
Valid 1 156 49.5 49.5 49.5
2 115 36.5 36.5 86.0
3 44 14.0 14.0 100.0
Total 315 100.0 100.0
Descriptive Statistics
N Minimum Maximum Mean Std. Deviation
alcohol 315 0 517 8.93 46.708
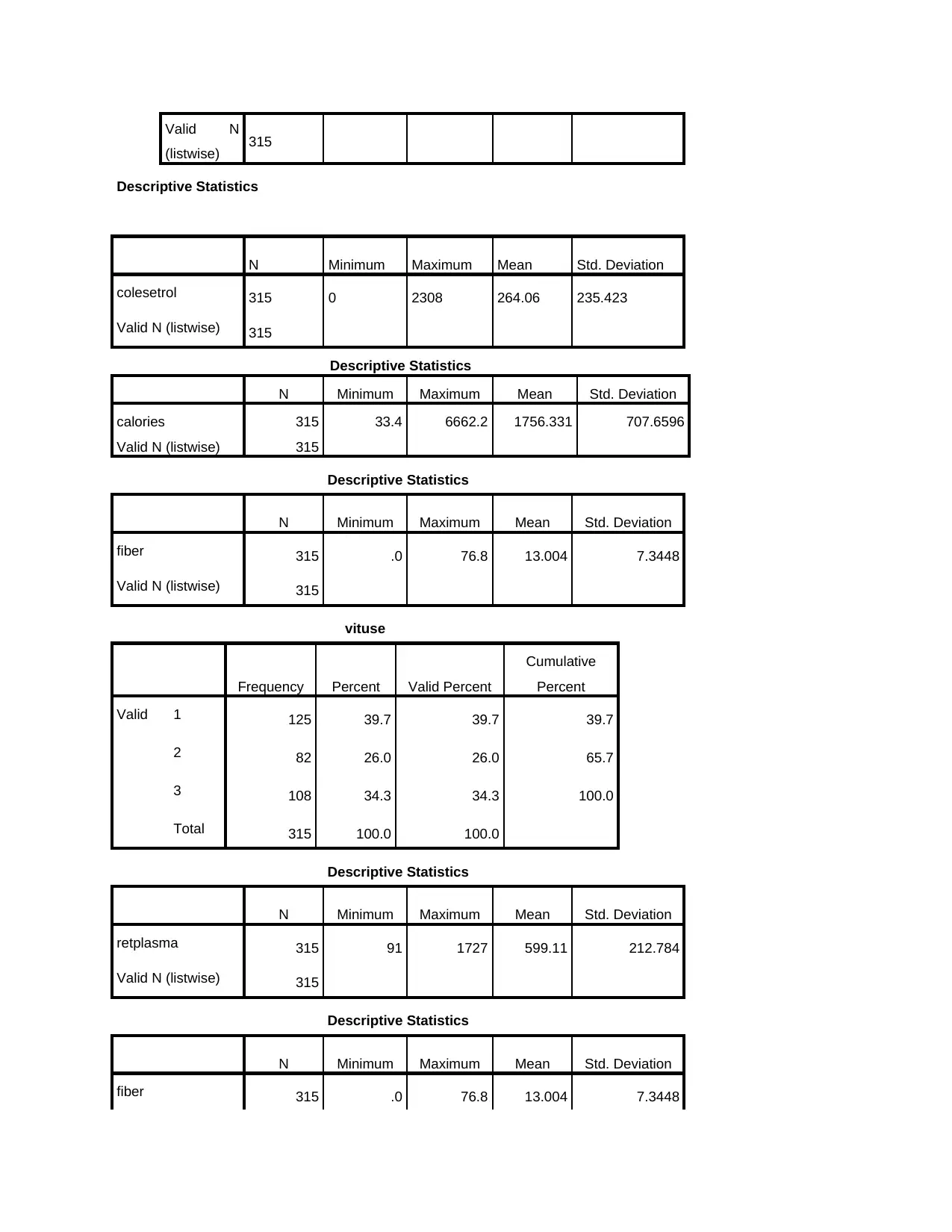
Valid N
(listwise) 315
Descriptive Statistics
N Minimum Maximum Mean Std. Deviation
colesetrol 315 0 2308 264.06 235.423
Valid N (listwise) 315
Descriptive Statistics
N Minimum Maximum Mean Std. Deviation
calories 315 33.4 6662.2 1756.331 707.6596
Valid N (listwise) 315
Descriptive Statistics
N Minimum Maximum Mean Std. Deviation
fiber 315 .0 76.8 13.004 7.3448
Valid N (listwise) 315
vituse
Frequency Percent Valid Percent
Cumulative
Percent
Valid 1 125 39.7 39.7 39.7
2 82 26.0 26.0 65.7
3 108 34.3 34.3 100.0
Total 315 100.0 100.0
Descriptive Statistics
N Minimum Maximum Mean Std. Deviation
retplasma 315 91 1727 599.11 212.784
Valid N (listwise) 315
Descriptive Statistics
N Minimum Maximum Mean Std. Deviation
fiber 315 .0 76.8 13.004 7.3448
(listwise) 315
Descriptive Statistics
N Minimum Maximum Mean Std. Deviation
colesetrol 315 0 2308 264.06 235.423
Valid N (listwise) 315
Descriptive Statistics
N Minimum Maximum Mean Std. Deviation
calories 315 33.4 6662.2 1756.331 707.6596
Valid N (listwise) 315
Descriptive Statistics
N Minimum Maximum Mean Std. Deviation
fiber 315 .0 76.8 13.004 7.3448
Valid N (listwise) 315
vituse
Frequency Percent Valid Percent
Cumulative
Percent
Valid 1 125 39.7 39.7 39.7
2 82 26.0 26.0 65.7
3 108 34.3 34.3 100.0
Total 315 100.0 100.0
Descriptive Statistics
N Minimum Maximum Mean Std. Deviation
retplasma 315 91 1727 599.11 212.784
Valid N (listwise) 315
Descriptive Statistics
N Minimum Maximum Mean Std. Deviation
fiber 315 .0 76.8 13.004 7.3448
Secure Best Marks with AI Grader
Need help grading? Try our AI Grader for instant feedback on your assignments.
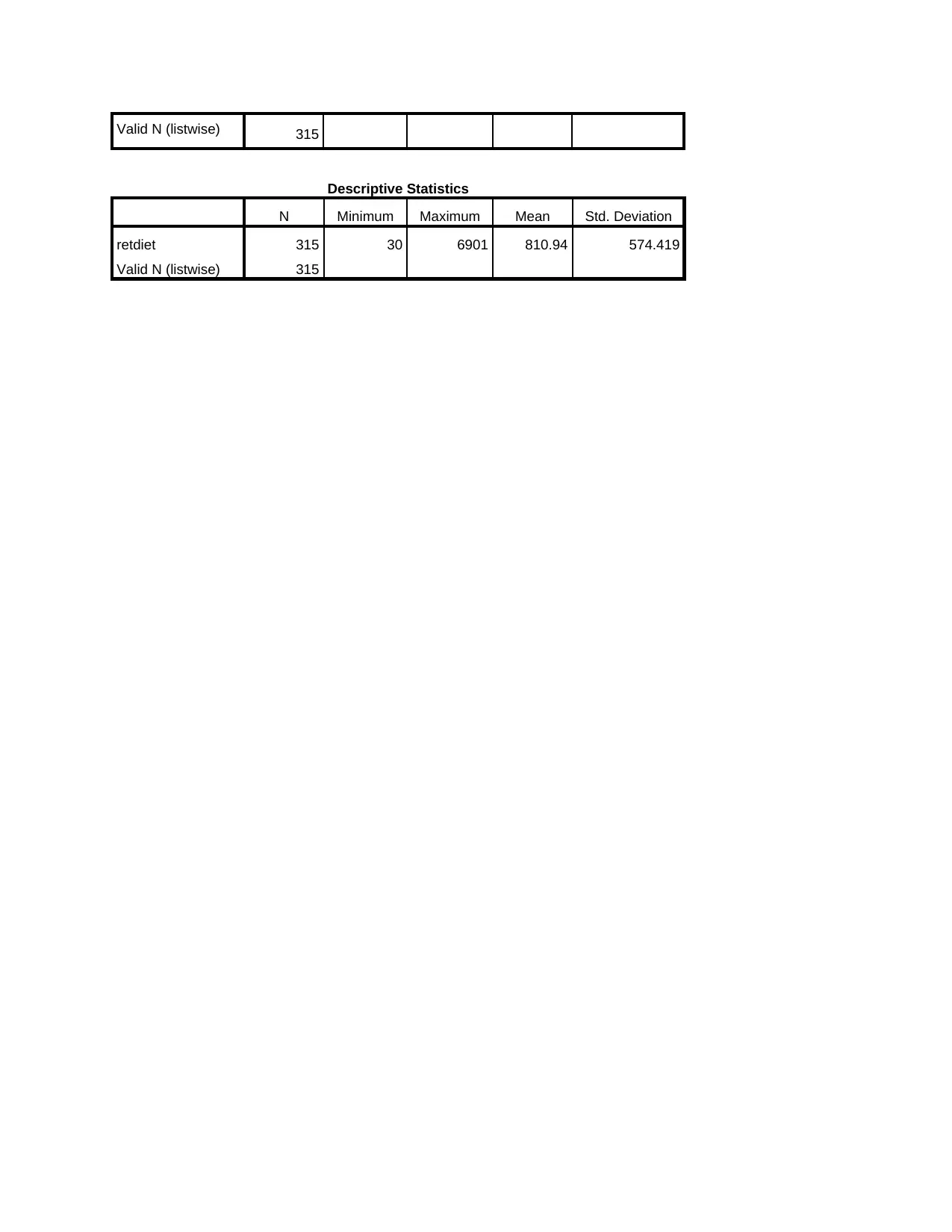
Valid N (listwise) 315
Descriptive Statistics
N Minimum Maximum Mean Std. Deviation
retdiet 315 30 6901 810.94 574.419
Valid N (listwise) 315
Descriptive Statistics
N Minimum Maximum Mean Std. Deviation
retdiet 315 30 6901 810.94 574.419
Valid N (listwise) 315
1 out of 23
![[object Object]](/_next/image/?url=%2F_next%2Fstatic%2Fmedia%2Flogo.6d15ce61.png&w=640&q=75)
Your All-in-One AI-Powered Toolkit for Academic Success.
 +13062052269
info@desklib.com
Available 24*7 on WhatsApp / Email
Unlock your academic potential
© 2024  |  Zucol Services PVT LTD  |  All rights reserved.