FIN28 Finance Report: Predicting Forked Cryptocurrency Prices
VerifiedAdded on 2022/11/13
|33
|8225
|301
Report
AI Summary
This report presents an analysis of forked cryptocurrency price prediction, focusing on Bitcoin Cash and Ethereum Classic. The study employs machine learning methods, including ARIMA, LSTM, and Facebook Prophet, to forecast price movements. The research investigates whether investing in forked cryptocurrencies can significantly alter digital currency investments and analyzes the correlation between closing prices and trade volume increases in cryptocurrency indexes. The report concludes that ARIMA demonstrates significant accuracy in predicting forked cryptocurrencies due to its ability to show correlation and stationarity. Additionally, the report found a strong relationship between USD and Bitcoin Cash and Ethereum Classic. The study also finds that the data about variables in the report were normally distributed. Recommendations and areas for future research are provided.
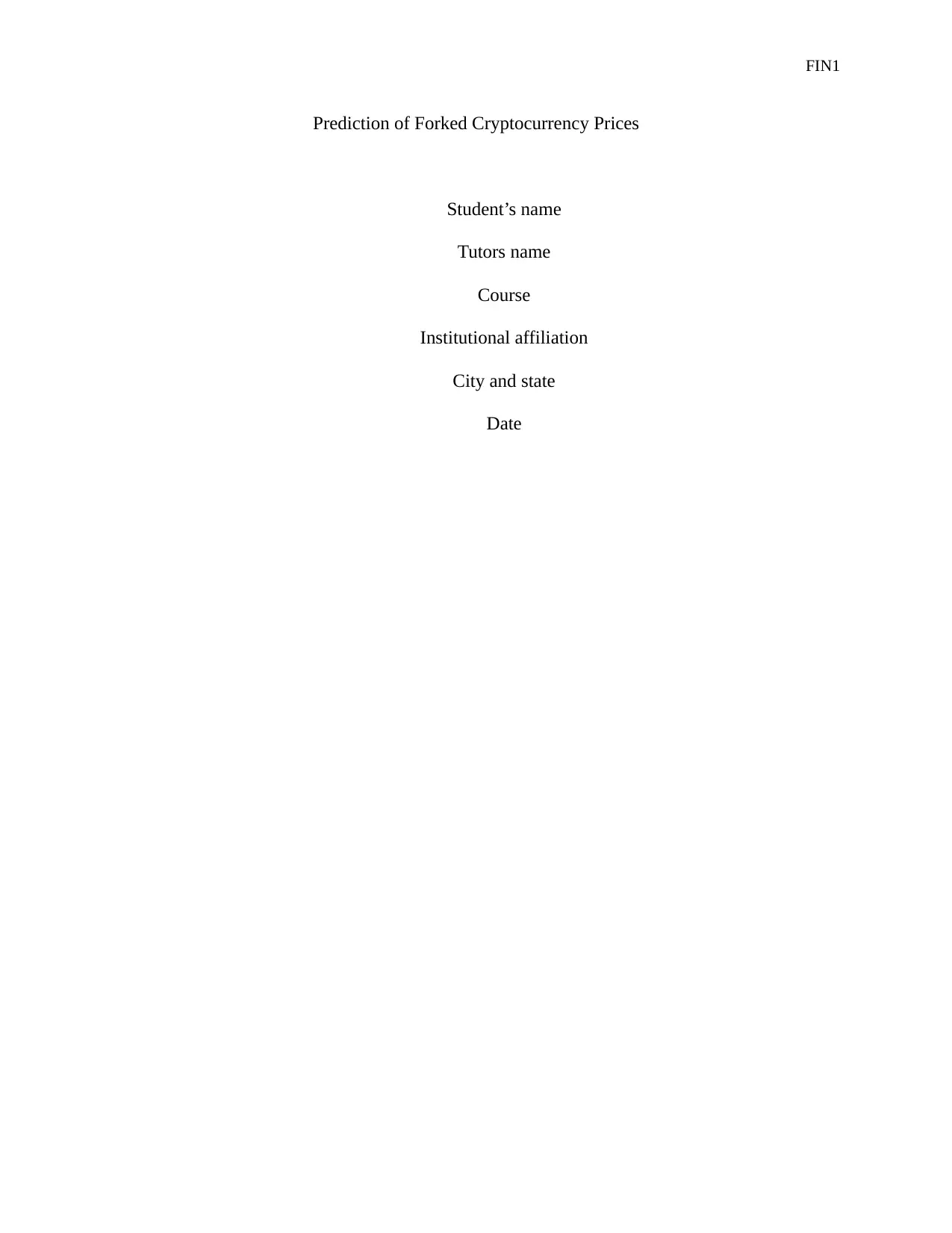
FIN1
Prediction of Forked Cryptocurrency Prices
Student’s name
Tutors name
Course
Institutional affiliation
City and state
Date
Prediction of Forked Cryptocurrency Prices
Student’s name
Tutors name
Course
Institutional affiliation
City and state
Date
Paraphrase This Document
Need a fresh take? Get an instant paraphrase of this document with our AI Paraphraser
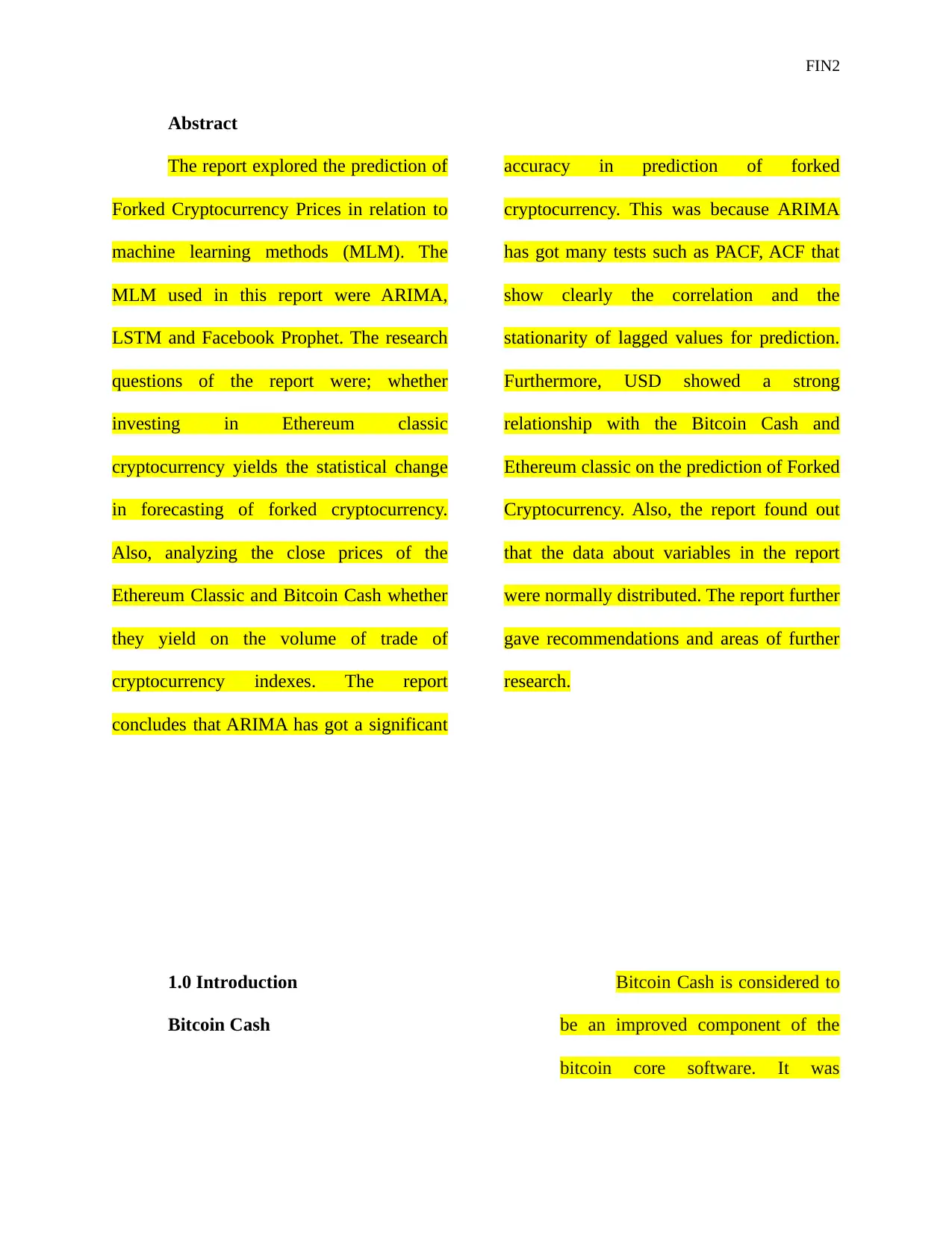
FIN2
Abstract
The report explored the prediction of
Forked Cryptocurrency Prices in relation to
machine learning methods (MLM). The
MLM used in this report were ARIMA,
LSTM and Facebook Prophet. The research
questions of the report were; whether
investing in Ethereum classic
cryptocurrency yields the statistical change
in forecasting of forked cryptocurrency.
Also, analyzing the close prices of the
Ethereum Classic and Bitcoin Cash whether
they yield on the volume of trade of
cryptocurrency indexes. The report
concludes that ARIMA has got a significant
accuracy in prediction of forked
cryptocurrency. This was because ARIMA
has got many tests such as PACF, ACF that
show clearly the correlation and the
stationarity of lagged values for prediction.
Furthermore, USD showed a strong
relationship with the Bitcoin Cash and
Ethereum classic on the prediction of Forked
Cryptocurrency. Also, the report found out
that the data about variables in the report
were normally distributed. The report further
gave recommendations and areas of further
research.
1.0 Introduction
Bitcoin Cash
Bitcoin Cash is considered to
be an improved component of the
bitcoin core software. It was
Abstract
The report explored the prediction of
Forked Cryptocurrency Prices in relation to
machine learning methods (MLM). The
MLM used in this report were ARIMA,
LSTM and Facebook Prophet. The research
questions of the report were; whether
investing in Ethereum classic
cryptocurrency yields the statistical change
in forecasting of forked cryptocurrency.
Also, analyzing the close prices of the
Ethereum Classic and Bitcoin Cash whether
they yield on the volume of trade of
cryptocurrency indexes. The report
concludes that ARIMA has got a significant
accuracy in prediction of forked
cryptocurrency. This was because ARIMA
has got many tests such as PACF, ACF that
show clearly the correlation and the
stationarity of lagged values for prediction.
Furthermore, USD showed a strong
relationship with the Bitcoin Cash and
Ethereum classic on the prediction of Forked
Cryptocurrency. Also, the report found out
that the data about variables in the report
were normally distributed. The report further
gave recommendations and areas of further
research.
1.0 Introduction
Bitcoin Cash
Bitcoin Cash is considered to
be an improved component of the
bitcoin core software. It was
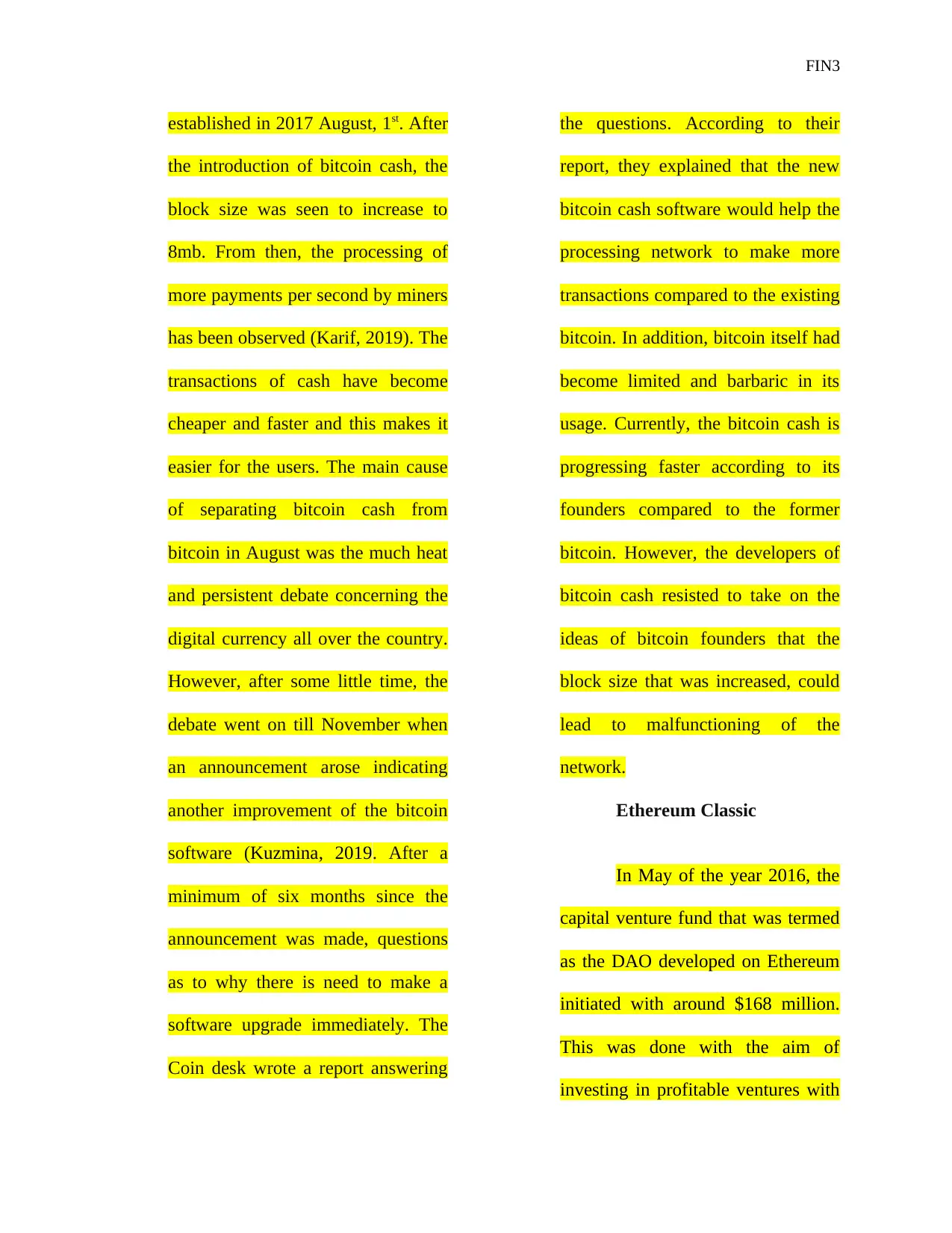
FIN3
established in 2017 August, 1st. After
the introduction of bitcoin cash, the
block size was seen to increase to
8mb. From then, the processing of
more payments per second by miners
has been observed (Karif, 2019). The
transactions of cash have become
cheaper and faster and this makes it
easier for the users. The main cause
of separating bitcoin cash from
bitcoin in August was the much heat
and persistent debate concerning the
digital currency all over the country.
However, after some little time, the
debate went on till November when
an announcement arose indicating
another improvement of the bitcoin
software (Kuzmina, 2019. After a
minimum of six months since the
announcement was made, questions
as to why there is need to make a
software upgrade immediately. The
Coin desk wrote a report answering
the questions. According to their
report, they explained that the new
bitcoin cash software would help the
processing network to make more
transactions compared to the existing
bitcoin. In addition, bitcoin itself had
become limited and barbaric in its
usage. Currently, the bitcoin cash is
progressing faster according to its
founders compared to the former
bitcoin. However, the developers of
bitcoin cash resisted to take on the
ideas of bitcoin founders that the
block size that was increased, could
lead to malfunctioning of the
network.
Ethereum Classic
In May of the year 2016, the
capital venture fund that was termed
as the DAO developed on Ethereum
initiated with around $168 million.
This was done with the aim of
investing in profitable ventures with
established in 2017 August, 1st. After
the introduction of bitcoin cash, the
block size was seen to increase to
8mb. From then, the processing of
more payments per second by miners
has been observed (Karif, 2019). The
transactions of cash have become
cheaper and faster and this makes it
easier for the users. The main cause
of separating bitcoin cash from
bitcoin in August was the much heat
and persistent debate concerning the
digital currency all over the country.
However, after some little time, the
debate went on till November when
an announcement arose indicating
another improvement of the bitcoin
software (Kuzmina, 2019. After a
minimum of six months since the
announcement was made, questions
as to why there is need to make a
software upgrade immediately. The
Coin desk wrote a report answering
the questions. According to their
report, they explained that the new
bitcoin cash software would help the
processing network to make more
transactions compared to the existing
bitcoin. In addition, bitcoin itself had
become limited and barbaric in its
usage. Currently, the bitcoin cash is
progressing faster according to its
founders compared to the former
bitcoin. However, the developers of
bitcoin cash resisted to take on the
ideas of bitcoin founders that the
block size that was increased, could
lead to malfunctioning of the
network.
Ethereum Classic
In May of the year 2016, the
capital venture fund that was termed
as the DAO developed on Ethereum
initiated with around $168 million.
This was done with the aim of
investing in profitable ventures with
⊘ This is a preview!⊘
Do you want full access?
Subscribe today to unlock all pages.

Trusted by 1+ million students worldwide
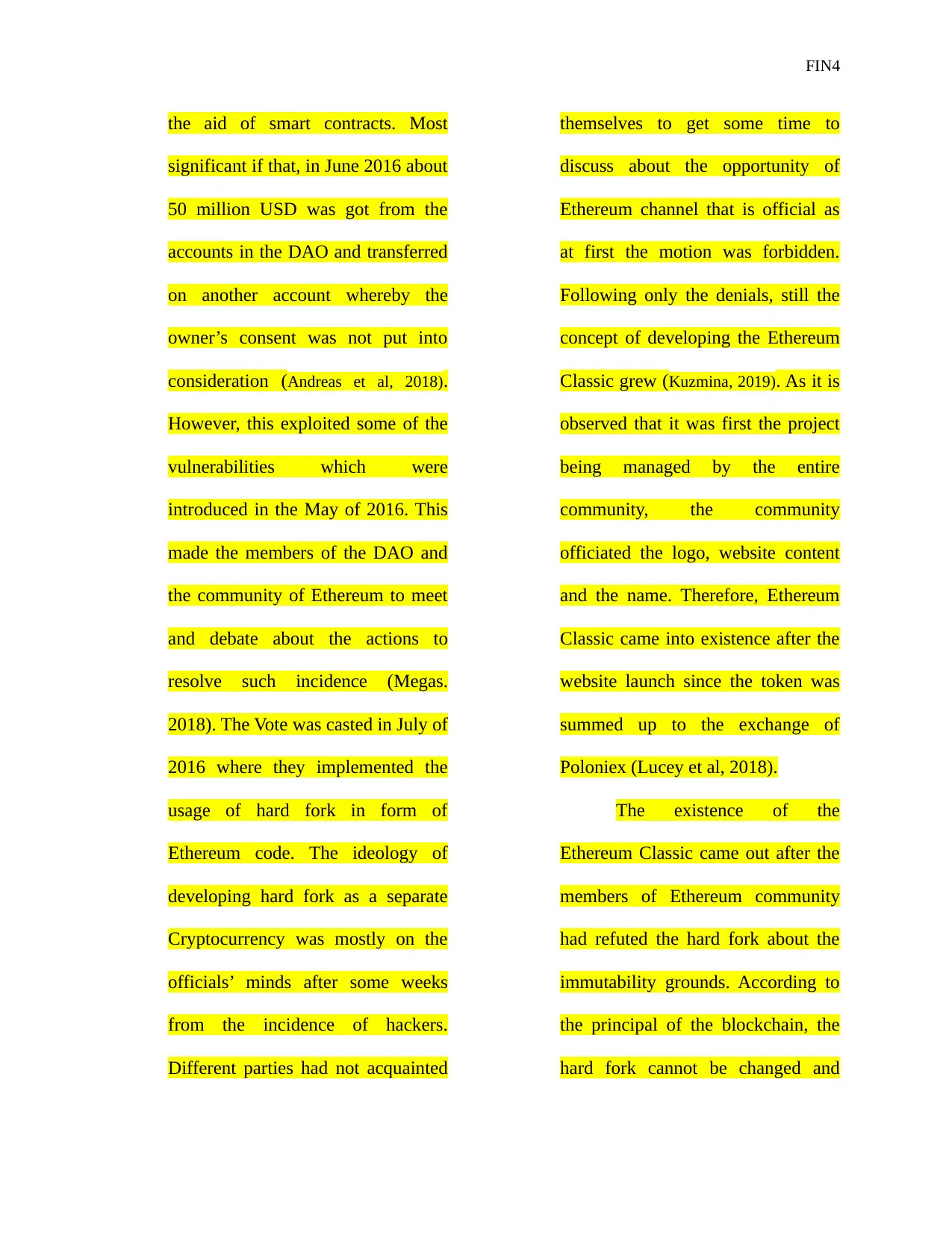
FIN4
the aid of smart contracts. Most
significant if that, in June 2016 about
50 million USD was got from the
accounts in the DAO and transferred
on another account whereby the
owner’s consent was not put into
consideration (Andreas et al, 2018).
However, this exploited some of the
vulnerabilities which were
introduced in the May of 2016. This
made the members of the DAO and
the community of Ethereum to meet
and debate about the actions to
resolve such incidence (Megas.
2018). The Vote was casted in July of
2016 where they implemented the
usage of hard fork in form of
Ethereum code. The ideology of
developing hard fork as a separate
Cryptocurrency was mostly on the
officials’ minds after some weeks
from the incidence of hackers.
Different parties had not acquainted
themselves to get some time to
discuss about the opportunity of
Ethereum channel that is official as
at first the motion was forbidden.
Following only the denials, still the
concept of developing the Ethereum
Classic grew (Kuzmina, 2019). As it is
observed that it was first the project
being managed by the entire
community, the community
officiated the logo, website content
and the name. Therefore, Ethereum
Classic came into existence after the
website launch since the token was
summed up to the exchange of
Poloniex (Lucey et al, 2018).
The existence of the
Ethereum Classic came out after the
members of Ethereum community
had refuted the hard fork about the
immutability grounds. According to
the principal of the blockchain, the
hard fork cannot be changed and
the aid of smart contracts. Most
significant if that, in June 2016 about
50 million USD was got from the
accounts in the DAO and transferred
on another account whereby the
owner’s consent was not put into
consideration (Andreas et al, 2018).
However, this exploited some of the
vulnerabilities which were
introduced in the May of 2016. This
made the members of the DAO and
the community of Ethereum to meet
and debate about the actions to
resolve such incidence (Megas.
2018). The Vote was casted in July of
2016 where they implemented the
usage of hard fork in form of
Ethereum code. The ideology of
developing hard fork as a separate
Cryptocurrency was mostly on the
officials’ minds after some weeks
from the incidence of hackers.
Different parties had not acquainted
themselves to get some time to
discuss about the opportunity of
Ethereum channel that is official as
at first the motion was forbidden.
Following only the denials, still the
concept of developing the Ethereum
Classic grew (Kuzmina, 2019). As it is
observed that it was first the project
being managed by the entire
community, the community
officiated the logo, website content
and the name. Therefore, Ethereum
Classic came into existence after the
website launch since the token was
summed up to the exchange of
Poloniex (Lucey et al, 2018).
The existence of the
Ethereum Classic came out after the
members of Ethereum community
had refuted the hard fork about the
immutability grounds. According to
the principal of the blockchain, the
hard fork cannot be changed and
Paraphrase This Document
Need a fresh take? Get an instant paraphrase of this document with our AI Paraphraser
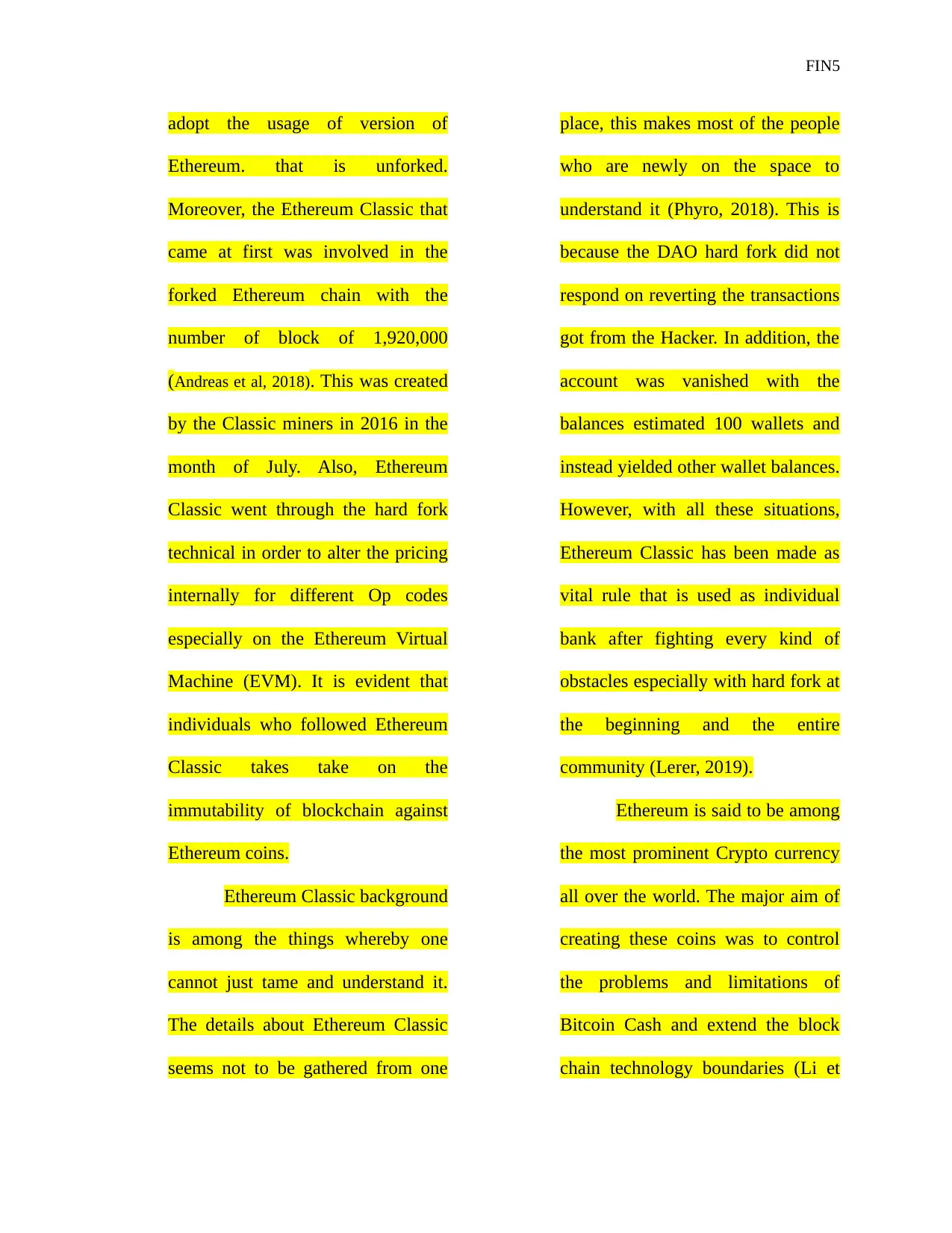
FIN5
adopt the usage of version of
Ethereum. that is unforked.
Moreover, the Ethereum Classic that
came at first was involved in the
forked Ethereum chain with the
number of block of 1,920,000
(Andreas et al, 2018). This was created
by the Classic miners in 2016 in the
month of July. Also, Ethereum
Classic went through the hard fork
technical in order to alter the pricing
internally for different Op codes
especially on the Ethereum Virtual
Machine (EVM). It is evident that
individuals who followed Ethereum
Classic takes take on the
immutability of blockchain against
Ethereum coins.
Ethereum Classic background
is among the things whereby one
cannot just tame and understand it.
The details about Ethereum Classic
seems not to be gathered from one
place, this makes most of the people
who are newly on the space to
understand it (Phyro, 2018). This is
because the DAO hard fork did not
respond on reverting the transactions
got from the Hacker. In addition, the
account was vanished with the
balances estimated 100 wallets and
instead yielded other wallet balances.
However, with all these situations,
Ethereum Classic has been made as
vital rule that is used as individual
bank after fighting every kind of
obstacles especially with hard fork at
the beginning and the entire
community (Lerer, 2019).
Ethereum is said to be among
the most prominent Crypto currency
all over the world. The major aim of
creating these coins was to control
the problems and limitations of
Bitcoin Cash and extend the block
chain technology boundaries (Li et
adopt the usage of version of
Ethereum. that is unforked.
Moreover, the Ethereum Classic that
came at first was involved in the
forked Ethereum chain with the
number of block of 1,920,000
(Andreas et al, 2018). This was created
by the Classic miners in 2016 in the
month of July. Also, Ethereum
Classic went through the hard fork
technical in order to alter the pricing
internally for different Op codes
especially on the Ethereum Virtual
Machine (EVM). It is evident that
individuals who followed Ethereum
Classic takes take on the
immutability of blockchain against
Ethereum coins.
Ethereum Classic background
is among the things whereby one
cannot just tame and understand it.
The details about Ethereum Classic
seems not to be gathered from one
place, this makes most of the people
who are newly on the space to
understand it (Phyro, 2018). This is
because the DAO hard fork did not
respond on reverting the transactions
got from the Hacker. In addition, the
account was vanished with the
balances estimated 100 wallets and
instead yielded other wallet balances.
However, with all these situations,
Ethereum Classic has been made as
vital rule that is used as individual
bank after fighting every kind of
obstacles especially with hard fork at
the beginning and the entire
community (Lerer, 2019).
Ethereum is said to be among
the most prominent Crypto currency
all over the world. The major aim of
creating these coins was to control
the problems and limitations of
Bitcoin Cash and extend the block
chain technology boundaries (Li et
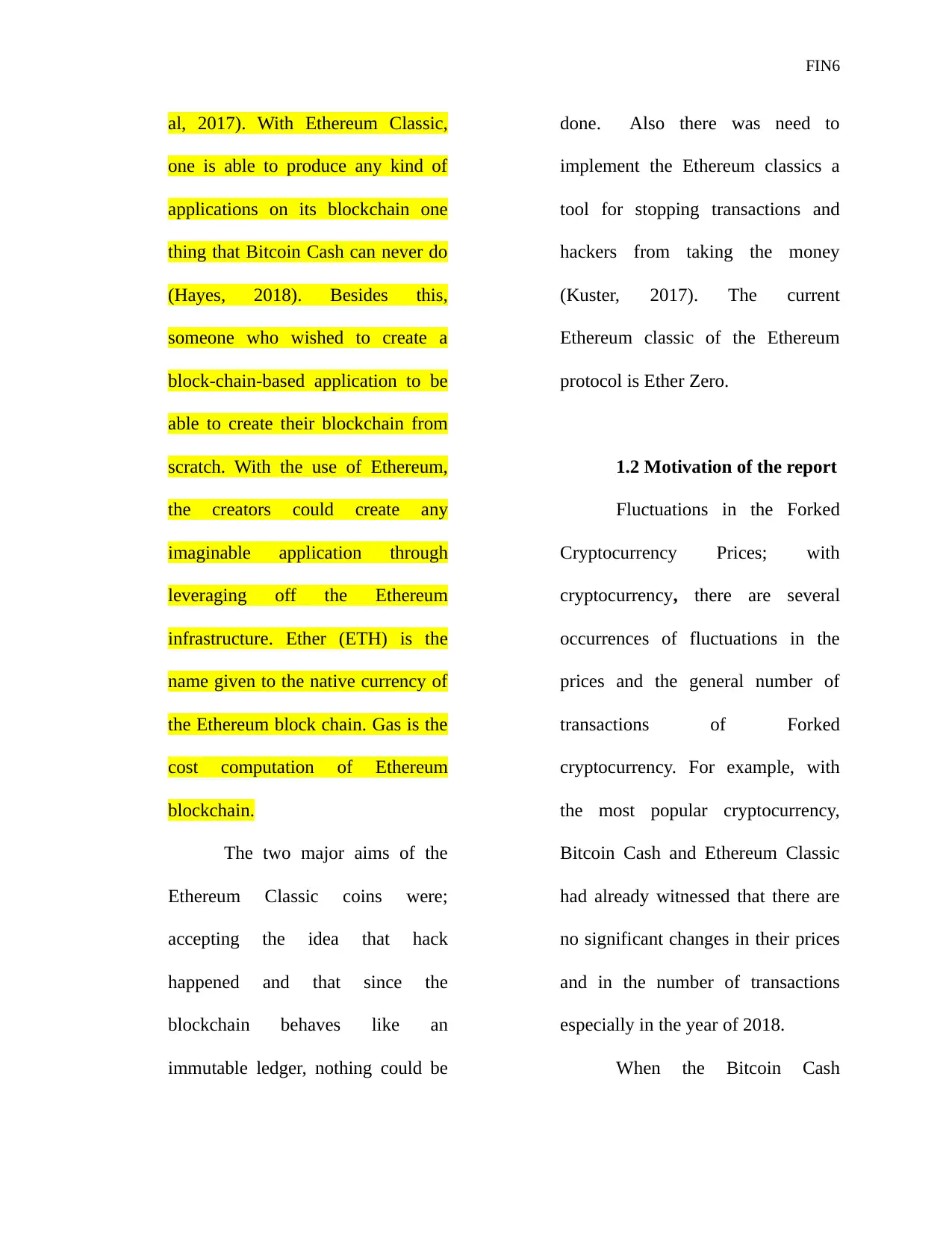
FIN6
al, 2017). With Ethereum Classic,
one is able to produce any kind of
applications on its blockchain one
thing that Bitcoin Cash can never do
(Hayes, 2018). Besides this,
someone who wished to create a
block-chain-based application to be
able to create their blockchain from
scratch. With the use of Ethereum,
the creators could create any
imaginable application through
leveraging off the Ethereum
infrastructure. Ether (ETH) is the
name given to the native currency of
the Ethereum block chain. Gas is the
cost computation of Ethereum
blockchain.
The two major aims of the
Ethereum Classic coins were;
accepting the idea that hack
happened and that since the
blockchain behaves like an
immutable ledger, nothing could be
done. Also there was need to
implement the Ethereum classics a
tool for stopping transactions and
hackers from taking the money
(Kuster, 2017). The current
Ethereum classic of the Ethereum
protocol is Ether Zero.
1.2 Motivation of the report
Fluctuations in the Forked
Cryptocurrency Prices; with
cryptocurrency, there are several
occurrences of fluctuations in the
prices and the general number of
transactions of Forked
cryptocurrency. For example, with
the most popular cryptocurrency,
Bitcoin Cash and Ethereum Classic
had already witnessed that there are
no significant changes in their prices
and in the number of transactions
especially in the year of 2018.
When the Bitcoin Cash
al, 2017). With Ethereum Classic,
one is able to produce any kind of
applications on its blockchain one
thing that Bitcoin Cash can never do
(Hayes, 2018). Besides this,
someone who wished to create a
block-chain-based application to be
able to create their blockchain from
scratch. With the use of Ethereum,
the creators could create any
imaginable application through
leveraging off the Ethereum
infrastructure. Ether (ETH) is the
name given to the native currency of
the Ethereum block chain. Gas is the
cost computation of Ethereum
blockchain.
The two major aims of the
Ethereum Classic coins were;
accepting the idea that hack
happened and that since the
blockchain behaves like an
immutable ledger, nothing could be
done. Also there was need to
implement the Ethereum classics a
tool for stopping transactions and
hackers from taking the money
(Kuster, 2017). The current
Ethereum classic of the Ethereum
protocol is Ether Zero.
1.2 Motivation of the report
Fluctuations in the Forked
Cryptocurrency Prices; with
cryptocurrency, there are several
occurrences of fluctuations in the
prices and the general number of
transactions of Forked
cryptocurrency. For example, with
the most popular cryptocurrency,
Bitcoin Cash and Ethereum Classic
had already witnessed that there are
no significant changes in their prices
and in the number of transactions
especially in the year of 2018.
When the Bitcoin Cash
⊘ This is a preview!⊘
Do you want full access?
Subscribe today to unlock all pages.

Trusted by 1+ million students worldwide
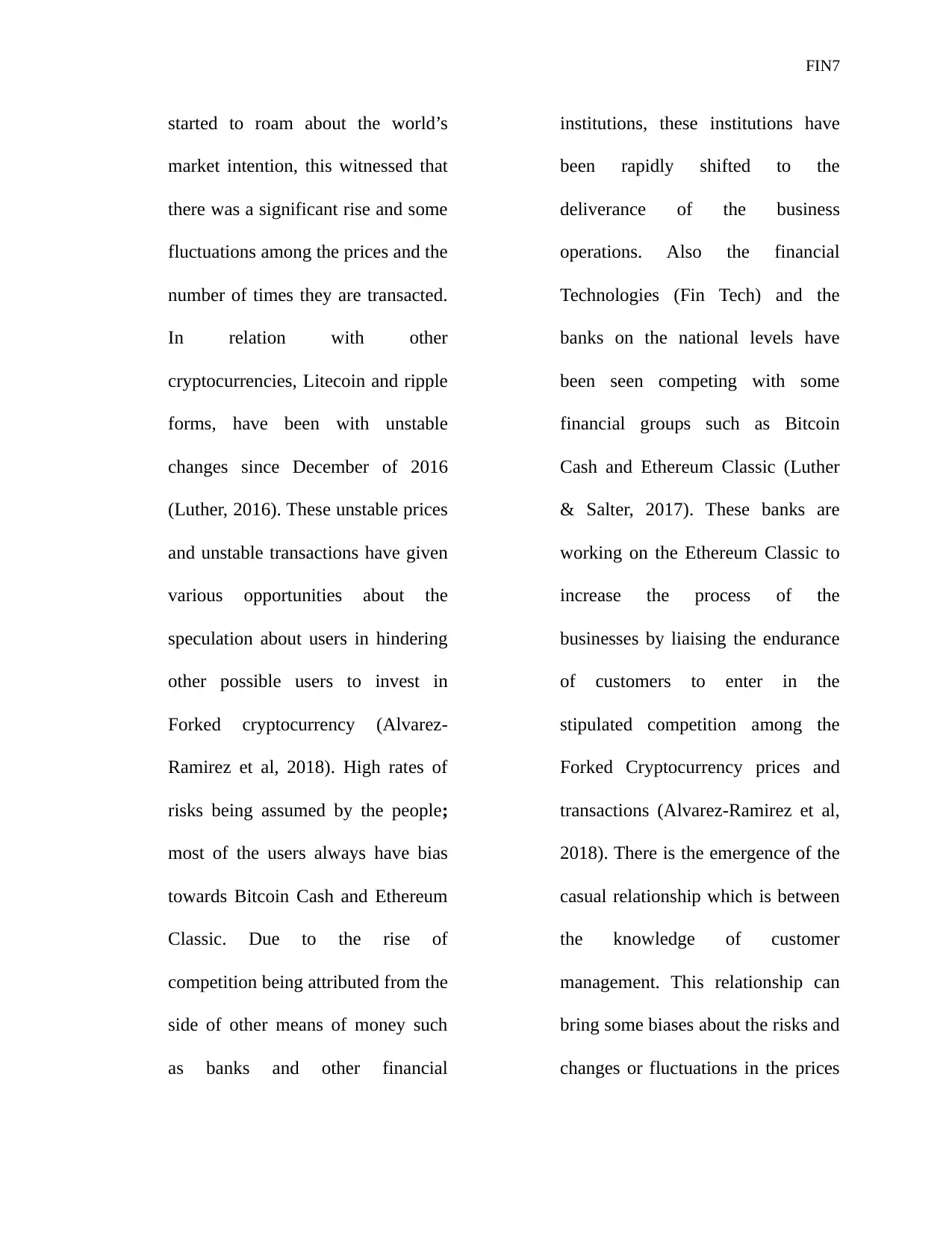
FIN7
started to roam about the world’s
market intention, this witnessed that
there was a significant rise and some
fluctuations among the prices and the
number of times they are transacted.
In relation with other
cryptocurrencies, Litecoin and ripple
forms, have been with unstable
changes since December of 2016
(Luther, 2016). These unstable prices
and unstable transactions have given
various opportunities about the
speculation about users in hindering
other possible users to invest in
Forked cryptocurrency (Alvarez-
Ramirez et al, 2018). High rates of
risks being assumed by the people;
most of the users always have bias
towards Bitcoin Cash and Ethereum
Classic. Due to the rise of
competition being attributed from the
side of other means of money such
as banks and other financial
institutions, these institutions have
been rapidly shifted to the
deliverance of the business
operations. Also the financial
Technologies (Fin Tech) and the
banks on the national levels have
been seen competing with some
financial groups such as Bitcoin
Cash and Ethereum Classic (Luther
& Salter, 2017). These banks are
working on the Ethereum Classic to
increase the process of the
businesses by liaising the endurance
of customers to enter in the
stipulated competition among the
Forked Cryptocurrency prices and
transactions (Alvarez-Ramirez et al,
2018). There is the emergence of the
casual relationship which is between
the knowledge of customer
management. This relationship can
bring some biases about the risks and
changes or fluctuations in the prices
started to roam about the world’s
market intention, this witnessed that
there was a significant rise and some
fluctuations among the prices and the
number of times they are transacted.
In relation with other
cryptocurrencies, Litecoin and ripple
forms, have been with unstable
changes since December of 2016
(Luther, 2016). These unstable prices
and unstable transactions have given
various opportunities about the
speculation about users in hindering
other possible users to invest in
Forked cryptocurrency (Alvarez-
Ramirez et al, 2018). High rates of
risks being assumed by the people;
most of the users always have bias
towards Bitcoin Cash and Ethereum
Classic. Due to the rise of
competition being attributed from the
side of other means of money such
as banks and other financial
institutions, these institutions have
been rapidly shifted to the
deliverance of the business
operations. Also the financial
Technologies (Fin Tech) and the
banks on the national levels have
been seen competing with some
financial groups such as Bitcoin
Cash and Ethereum Classic (Luther
& Salter, 2017). These banks are
working on the Ethereum Classic to
increase the process of the
businesses by liaising the endurance
of customers to enter in the
stipulated competition among the
Forked Cryptocurrency prices and
transactions (Alvarez-Ramirez et al,
2018). There is the emergence of the
casual relationship which is between
the knowledge of customer
management. This relationship can
bring some biases about the risks and
changes or fluctuations in the prices
Paraphrase This Document
Need a fresh take? Get an instant paraphrase of this document with our AI Paraphraser
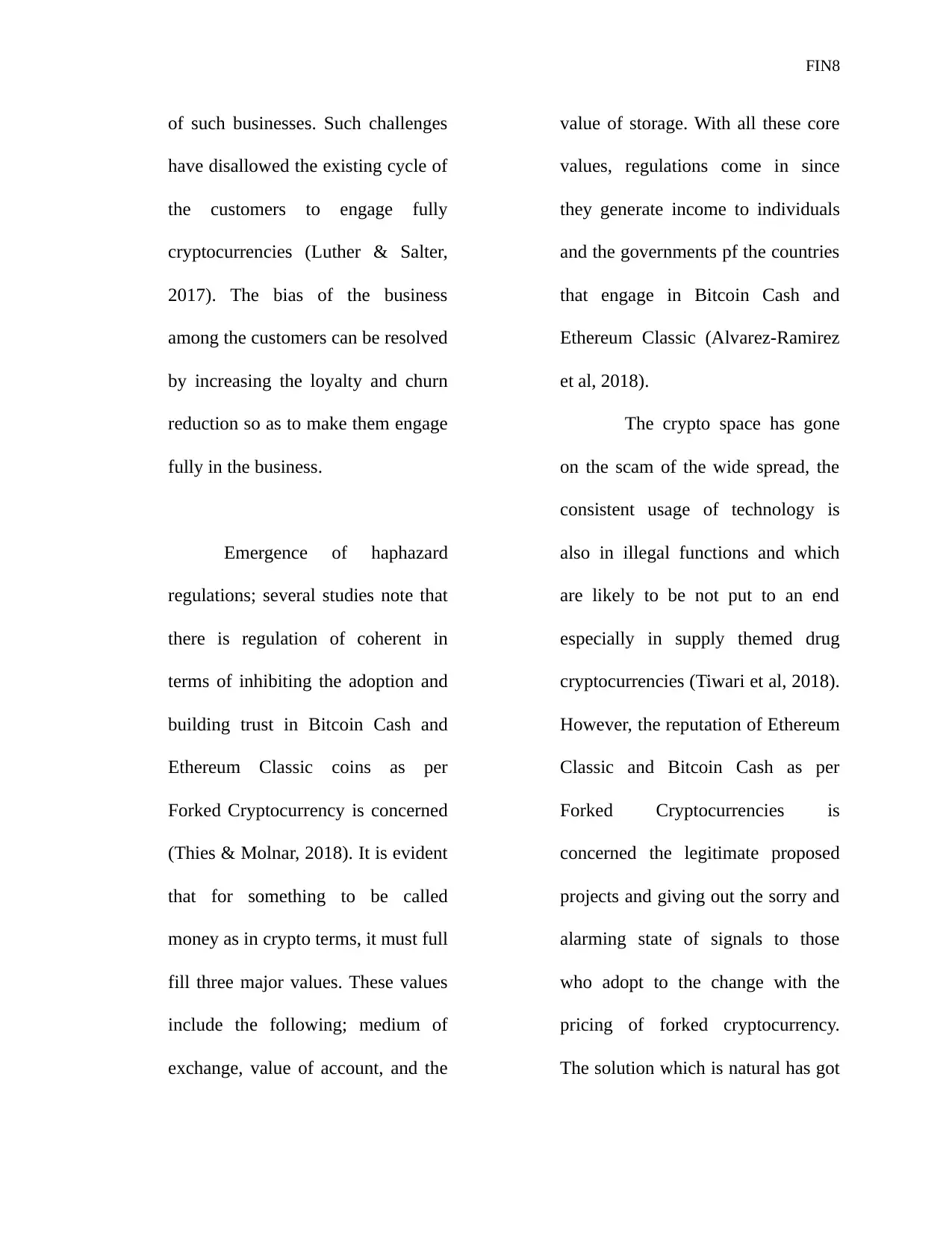
FIN8
of such businesses. Such challenges
have disallowed the existing cycle of
the customers to engage fully
cryptocurrencies (Luther & Salter,
2017). The bias of the business
among the customers can be resolved
by increasing the loyalty and churn
reduction so as to make them engage
fully in the business.
Emergence of haphazard
regulations; several studies note that
there is regulation of coherent in
terms of inhibiting the adoption and
building trust in Bitcoin Cash and
Ethereum Classic coins as per
Forked Cryptocurrency is concerned
(Thies & Molnar, 2018). It is evident
that for something to be called
money as in crypto terms, it must full
fill three major values. These values
include the following; medium of
exchange, value of account, and the
value of storage. With all these core
values, regulations come in since
they generate income to individuals
and the governments pf the countries
that engage in Bitcoin Cash and
Ethereum Classic (Alvarez-Ramirez
et al, 2018).
The crypto space has gone
on the scam of the wide spread, the
consistent usage of technology is
also in illegal functions and which
are likely to be not put to an end
especially in supply themed drug
cryptocurrencies (Tiwari et al, 2018).
However, the reputation of Ethereum
Classic and Bitcoin Cash as per
Forked Cryptocurrencies is
concerned the legitimate proposed
projects and giving out the sorry and
alarming state of signals to those
who adopt to the change with the
pricing of forked cryptocurrency.
The solution which is natural has got
of such businesses. Such challenges
have disallowed the existing cycle of
the customers to engage fully
cryptocurrencies (Luther & Salter,
2017). The bias of the business
among the customers can be resolved
by increasing the loyalty and churn
reduction so as to make them engage
fully in the business.
Emergence of haphazard
regulations; several studies note that
there is regulation of coherent in
terms of inhibiting the adoption and
building trust in Bitcoin Cash and
Ethereum Classic coins as per
Forked Cryptocurrency is concerned
(Thies & Molnar, 2018). It is evident
that for something to be called
money as in crypto terms, it must full
fill three major values. These values
include the following; medium of
exchange, value of account, and the
value of storage. With all these core
values, regulations come in since
they generate income to individuals
and the governments pf the countries
that engage in Bitcoin Cash and
Ethereum Classic (Alvarez-Ramirez
et al, 2018).
The crypto space has gone
on the scam of the wide spread, the
consistent usage of technology is
also in illegal functions and which
are likely to be not put to an end
especially in supply themed drug
cryptocurrencies (Tiwari et al, 2018).
However, the reputation of Ethereum
Classic and Bitcoin Cash as per
Forked Cryptocurrencies is
concerned the legitimate proposed
projects and giving out the sorry and
alarming state of signals to those
who adopt to the change with the
pricing of forked cryptocurrency.
The solution which is natural has got
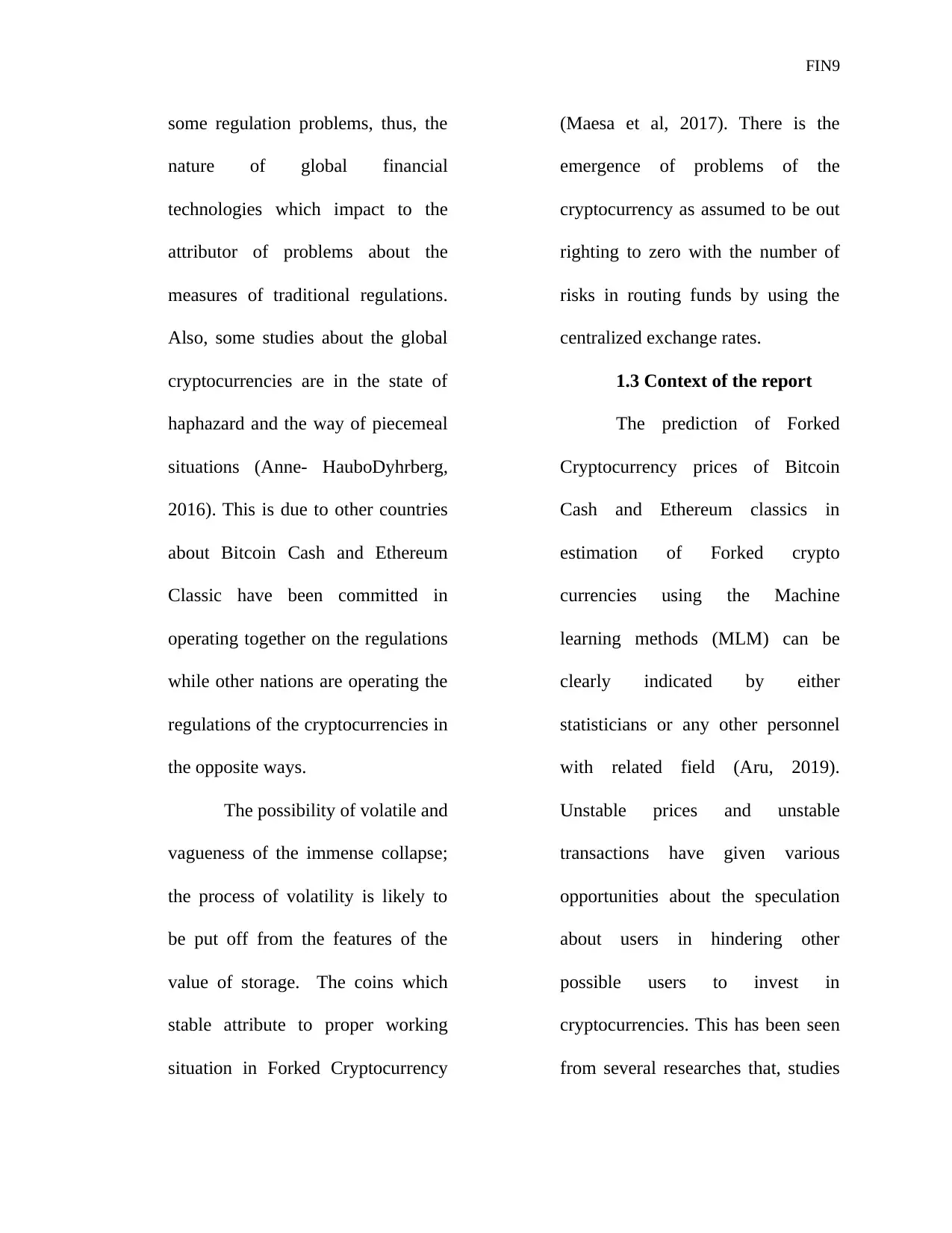
FIN9
some regulation problems, thus, the
nature of global financial
technologies which impact to the
attributor of problems about the
measures of traditional regulations.
Also, some studies about the global
cryptocurrencies are in the state of
haphazard and the way of piecemeal
situations (Anne- HauboDyhrberg,
2016). This is due to other countries
about Bitcoin Cash and Ethereum
Classic have been committed in
operating together on the regulations
while other nations are operating the
regulations of the cryptocurrencies in
the opposite ways.
The possibility of volatile and
vagueness of the immense collapse;
the process of volatility is likely to
be put off from the features of the
value of storage. The coins which
stable attribute to proper working
situation in Forked Cryptocurrency
(Maesa et al, 2017). There is the
emergence of problems of the
cryptocurrency as assumed to be out
righting to zero with the number of
risks in routing funds by using the
centralized exchange rates.
1.3 Context of the report
The prediction of Forked
Cryptocurrency prices of Bitcoin
Cash and Ethereum classics in
estimation of Forked crypto
currencies using the Machine
learning methods (MLM) can be
clearly indicated by either
statisticians or any other personnel
with related field (Aru, 2019).
Unstable prices and unstable
transactions have given various
opportunities about the speculation
about users in hindering other
possible users to invest in
cryptocurrencies. This has been seen
from several researches that, studies
some regulation problems, thus, the
nature of global financial
technologies which impact to the
attributor of problems about the
measures of traditional regulations.
Also, some studies about the global
cryptocurrencies are in the state of
haphazard and the way of piecemeal
situations (Anne- HauboDyhrberg,
2016). This is due to other countries
about Bitcoin Cash and Ethereum
Classic have been committed in
operating together on the regulations
while other nations are operating the
regulations of the cryptocurrencies in
the opposite ways.
The possibility of volatile and
vagueness of the immense collapse;
the process of volatility is likely to
be put off from the features of the
value of storage. The coins which
stable attribute to proper working
situation in Forked Cryptocurrency
(Maesa et al, 2017). There is the
emergence of problems of the
cryptocurrency as assumed to be out
righting to zero with the number of
risks in routing funds by using the
centralized exchange rates.
1.3 Context of the report
The prediction of Forked
Cryptocurrency prices of Bitcoin
Cash and Ethereum classics in
estimation of Forked crypto
currencies using the Machine
learning methods (MLM) can be
clearly indicated by either
statisticians or any other personnel
with related field (Aru, 2019).
Unstable prices and unstable
transactions have given various
opportunities about the speculation
about users in hindering other
possible users to invest in
cryptocurrencies. This has been seen
from several researches that, studies
⊘ This is a preview!⊘
Do you want full access?
Subscribe today to unlock all pages.

Trusted by 1+ million students worldwide
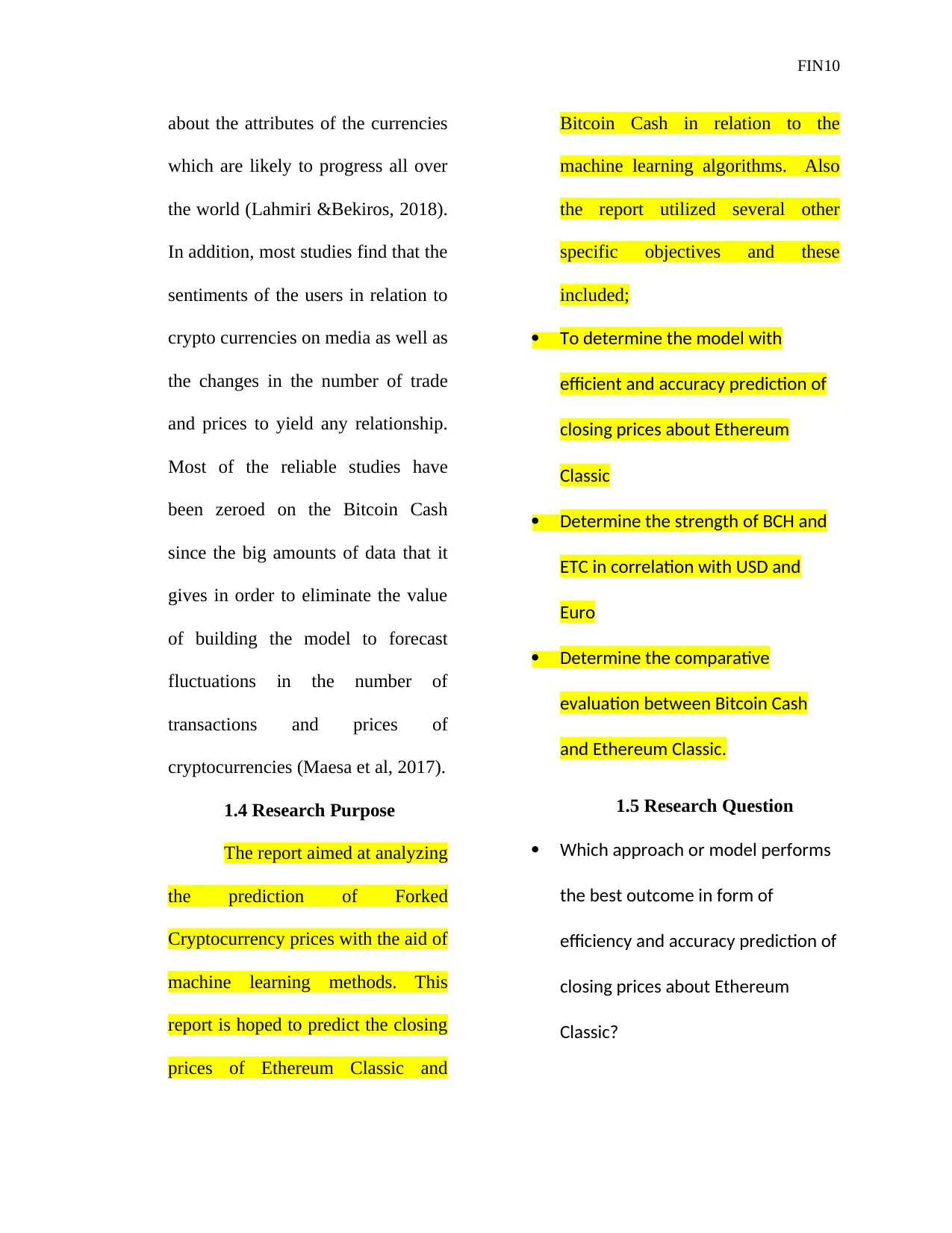
FIN10
about the attributes of the currencies
which are likely to progress all over
the world (Lahmiri &Bekiros, 2018).
In addition, most studies find that the
sentiments of the users in relation to
crypto currencies on media as well as
the changes in the number of trade
and prices to yield any relationship.
Most of the reliable studies have
been zeroed on the Bitcoin Cash
since the big amounts of data that it
gives in order to eliminate the value
of building the model to forecast
fluctuations in the number of
transactions and prices of
cryptocurrencies (Maesa et al, 2017).
1.4 Research Purpose
The report aimed at analyzing
the prediction of Forked
Cryptocurrency prices with the aid of
machine learning methods. This
report is hoped to predict the closing
prices of Ethereum Classic and
Bitcoin Cash in relation to the
machine learning algorithms. Also
the report utilized several other
specific objectives and these
included;
ï‚· To determine the model with
efficient and accuracy prediction of
closing prices about Ethereum
Classic
ï‚· Determine the strength of BCH and
ETC in correlation with USD and
Euro
ï‚· Determine the comparative
evaluation between Bitcoin Cash
and Ethereum Classic.
1.5 Research Question
ï‚· Which approach or model performs
the best outcome in form of
efficiency and accuracy prediction of
closing prices about Ethereum
Classic?
about the attributes of the currencies
which are likely to progress all over
the world (Lahmiri &Bekiros, 2018).
In addition, most studies find that the
sentiments of the users in relation to
crypto currencies on media as well as
the changes in the number of trade
and prices to yield any relationship.
Most of the reliable studies have
been zeroed on the Bitcoin Cash
since the big amounts of data that it
gives in order to eliminate the value
of building the model to forecast
fluctuations in the number of
transactions and prices of
cryptocurrencies (Maesa et al, 2017).
1.4 Research Purpose
The report aimed at analyzing
the prediction of Forked
Cryptocurrency prices with the aid of
machine learning methods. This
report is hoped to predict the closing
prices of Ethereum Classic and
Bitcoin Cash in relation to the
machine learning algorithms. Also
the report utilized several other
specific objectives and these
included;
ï‚· To determine the model with
efficient and accuracy prediction of
closing prices about Ethereum
Classic
ï‚· Determine the strength of BCH and
ETC in correlation with USD and
Euro
ï‚· Determine the comparative
evaluation between Bitcoin Cash
and Ethereum Classic.
1.5 Research Question
ï‚· Which approach or model performs
the best outcome in form of
efficiency and accuracy prediction of
closing prices about Ethereum
Classic?
Paraphrase This Document
Need a fresh take? Get an instant paraphrase of this document with our AI Paraphraser
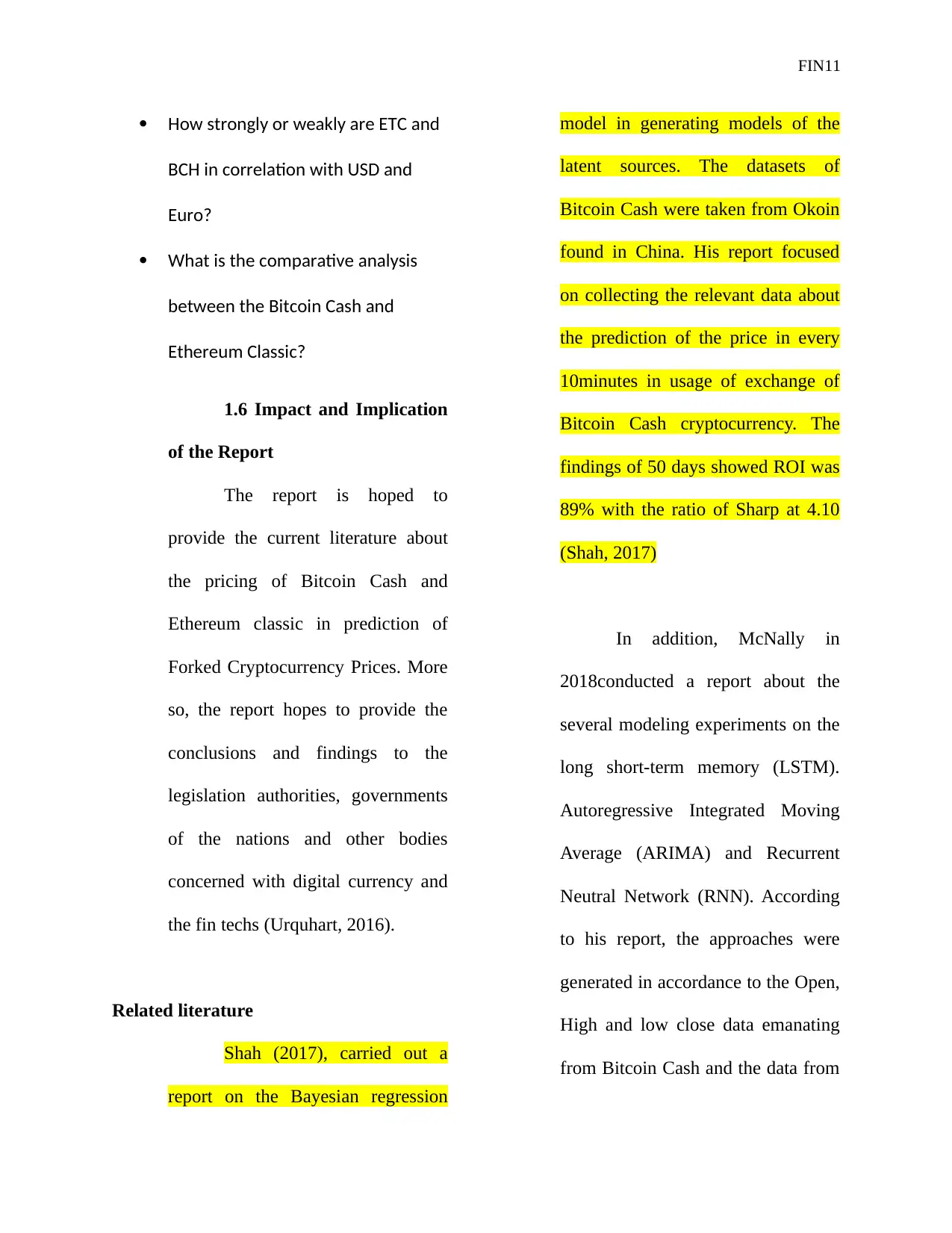
FIN11
ï‚· How strongly or weakly are ETC and
BCH in correlation with USD and
Euro?
ï‚· What is the comparative analysis
between the Bitcoin Cash and
Ethereum Classic?
1.6 Impact and Implication
of the Report
The report is hoped to
provide the current literature about
the pricing of Bitcoin Cash and
Ethereum classic in prediction of
Forked Cryptocurrency Prices. More
so, the report hopes to provide the
conclusions and findings to the
legislation authorities, governments
of the nations and other bodies
concerned with digital currency and
the fin techs (Urquhart, 2016).
Related literature
Shah (2017), carried out a
report on the Bayesian regression
model in generating models of the
latent sources. The datasets of
Bitcoin Cash were taken from Okoin
found in China. His report focused
on collecting the relevant data about
the prediction of the price in every
10minutes in usage of exchange of
Bitcoin Cash cryptocurrency. The
findings of 50 days showed ROI was
89% with the ratio of Sharp at 4.10
(Shah, 2017)
In addition, McNally in
2018conducted a report about the
several modeling experiments on the
long short-term memory (LSTM).
Autoregressive Integrated Moving
Average (ARIMA) and Recurrent
Neutral Network (RNN). According
to his report, the approaches were
generated in accordance to the Open,
High and low close data emanating
from Bitcoin Cash and the data from
ï‚· How strongly or weakly are ETC and
BCH in correlation with USD and
Euro?
ï‚· What is the comparative analysis
between the Bitcoin Cash and
Ethereum Classic?
1.6 Impact and Implication
of the Report
The report is hoped to
provide the current literature about
the pricing of Bitcoin Cash and
Ethereum classic in prediction of
Forked Cryptocurrency Prices. More
so, the report hopes to provide the
conclusions and findings to the
legislation authorities, governments
of the nations and other bodies
concerned with digital currency and
the fin techs (Urquhart, 2016).
Related literature
Shah (2017), carried out a
report on the Bayesian regression
model in generating models of the
latent sources. The datasets of
Bitcoin Cash were taken from Okoin
found in China. His report focused
on collecting the relevant data about
the prediction of the price in every
10minutes in usage of exchange of
Bitcoin Cash cryptocurrency. The
findings of 50 days showed ROI was
89% with the ratio of Sharp at 4.10
(Shah, 2017)
In addition, McNally in
2018conducted a report about the
several modeling experiments on the
long short-term memory (LSTM).
Autoregressive Integrated Moving
Average (ARIMA) and Recurrent
Neutral Network (RNN). According
to his report, the approaches were
generated in accordance to the Open,
High and low close data emanating
from Bitcoin Cash and the data from
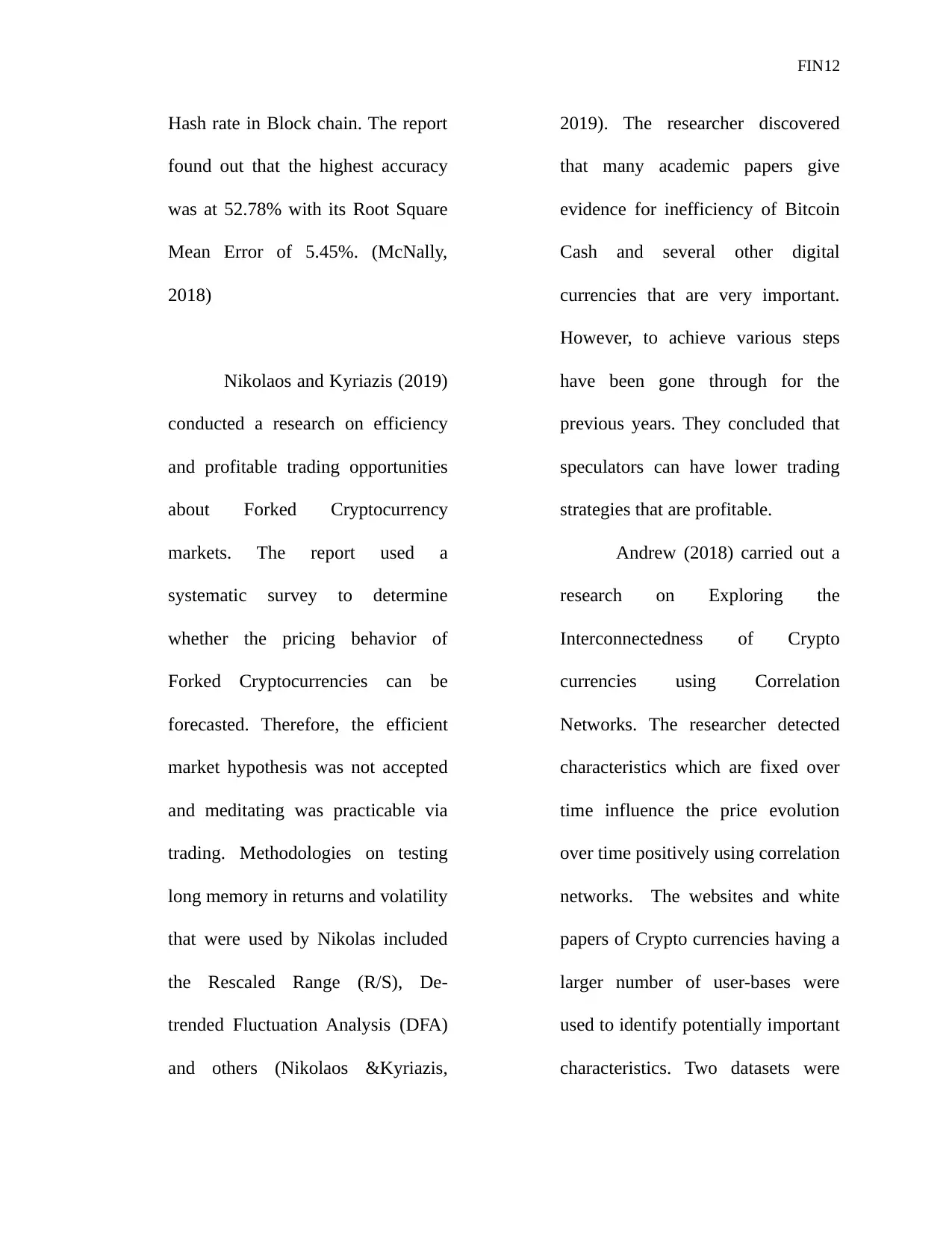
FIN12
Hash rate in Block chain. The report
found out that the highest accuracy
was at 52.78% with its Root Square
Mean Error of 5.45%. (McNally,
2018)
Nikolaos and Kyriazis (2019)
conducted a research on efficiency
and profitable trading opportunities
about Forked Cryptocurrency
markets. The report used a
systematic survey to determine
whether the pricing behavior of
Forked Cryptocurrencies can be
forecasted. Therefore, the efficient
market hypothesis was not accepted
and meditating was practicable via
trading. Methodologies on testing
long memory in returns and volatility
that were used by Nikolas included
the Rescaled Range (R/S), De-
trended Fluctuation Analysis (DFA)
and others (Nikolaos &Kyriazis,
2019). The researcher discovered
that many academic papers give
evidence for inefficiency of Bitcoin
Cash and several other digital
currencies that are very important.
However, to achieve various steps
have been gone through for the
previous years. They concluded that
speculators can have lower trading
strategies that are profitable.
Andrew (2018) carried out a
research on Exploring the
Interconnectedness of Crypto
currencies using Correlation
Networks. The researcher detected
characteristics which are fixed over
time influence the price evolution
over time positively using correlation
networks. The websites and white
papers of Crypto currencies having a
larger number of user-bases were
used to identify potentially important
characteristics. Two datasets were
Hash rate in Block chain. The report
found out that the highest accuracy
was at 52.78% with its Root Square
Mean Error of 5.45%. (McNally,
2018)
Nikolaos and Kyriazis (2019)
conducted a research on efficiency
and profitable trading opportunities
about Forked Cryptocurrency
markets. The report used a
systematic survey to determine
whether the pricing behavior of
Forked Cryptocurrencies can be
forecasted. Therefore, the efficient
market hypothesis was not accepted
and meditating was practicable via
trading. Methodologies on testing
long memory in returns and volatility
that were used by Nikolas included
the Rescaled Range (R/S), De-
trended Fluctuation Analysis (DFA)
and others (Nikolaos &Kyriazis,
2019). The researcher discovered
that many academic papers give
evidence for inefficiency of Bitcoin
Cash and several other digital
currencies that are very important.
However, to achieve various steps
have been gone through for the
previous years. They concluded that
speculators can have lower trading
strategies that are profitable.
Andrew (2018) carried out a
research on Exploring the
Interconnectedness of Crypto
currencies using Correlation
Networks. The researcher detected
characteristics which are fixed over
time influence the price evolution
over time positively using correlation
networks. The websites and white
papers of Crypto currencies having a
larger number of user-bases were
used to identify potentially important
characteristics. Two datasets were
⊘ This is a preview!⊘
Do you want full access?
Subscribe today to unlock all pages.

Trusted by 1+ million students worldwide
1 out of 33