Face Recognition Technology Applications
VerifiedAdded on 2020/05/28
|27
|7463
|220
AI Summary
This assignment delves into the multifaceted world of face recognition technology. It examines various applications spanning fields like security, attendance management, and personalized services. The analysis highlights the benefits and challenges associated with face recognition, considering factors such as accuracy, privacy concerns, and potential biases. Moreover, it explores emerging trends and future directions in this rapidly evolving field.
Contribute Materials
Your contribution can guide someone’s learning journey. Share your
documents today.
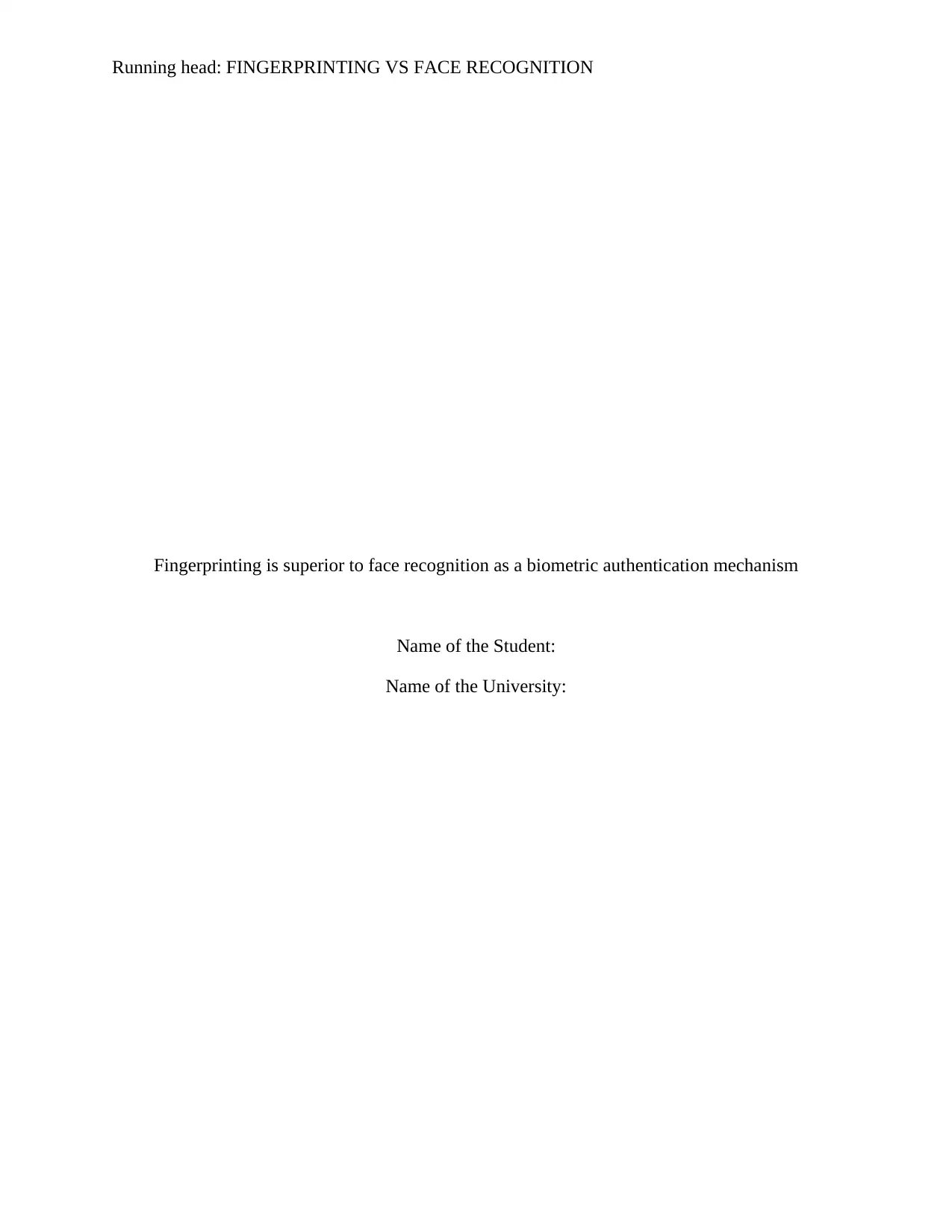
Running head: FINGERPRINTING VS FACE RECOGNITION
Fingerprinting is superior to face recognition as a biometric authentication mechanism
Name of the Student:
Name of the University:
Fingerprinting is superior to face recognition as a biometric authentication mechanism
Name of the Student:
Name of the University:
Secure Best Marks with AI Grader
Need help grading? Try our AI Grader for instant feedback on your assignments.
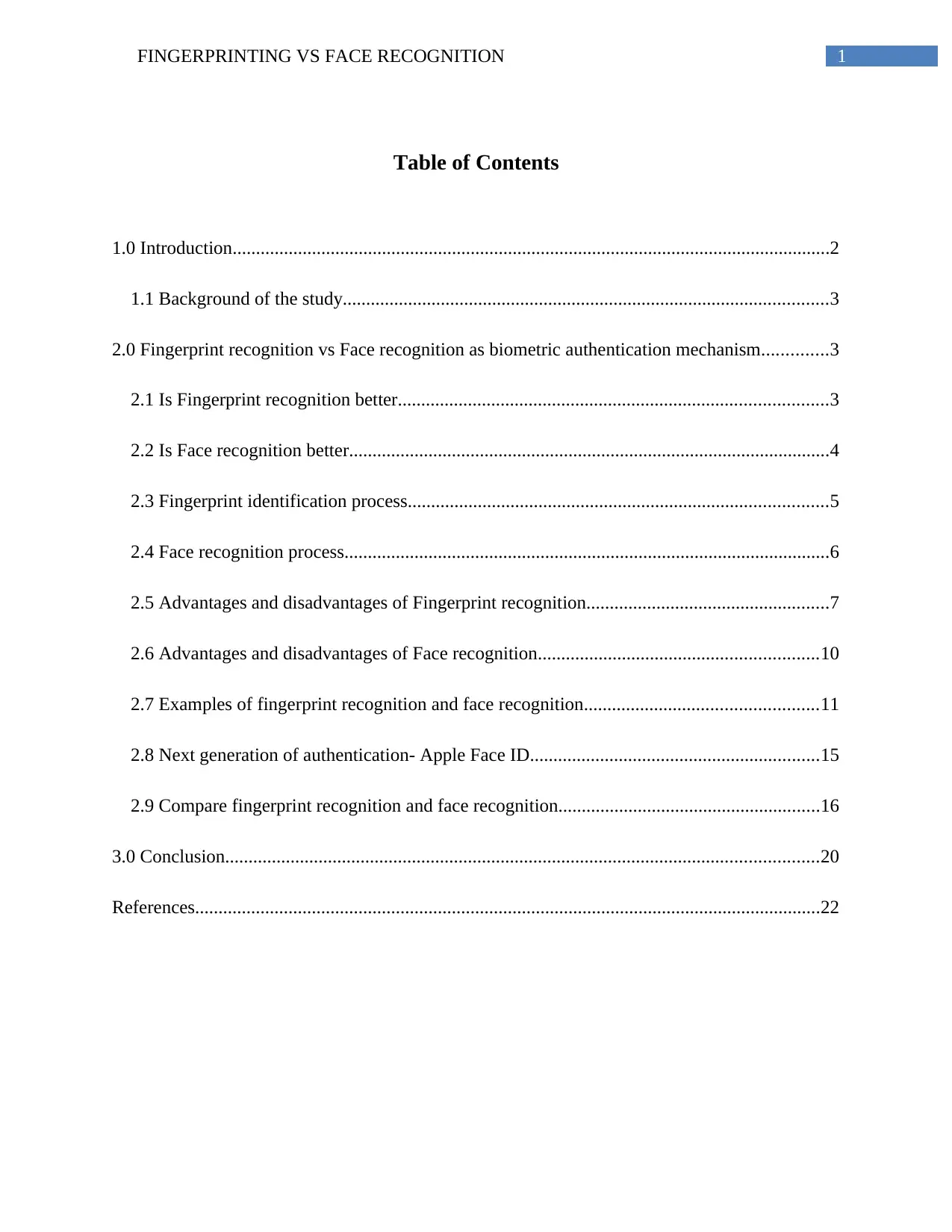
1FINGERPRINTING VS FACE RECOGNITION
Table of Contents
1.0 Introduction................................................................................................................................2
1.1 Background of the study........................................................................................................3
2.0 Fingerprint recognition vs Face recognition as biometric authentication mechanism..............3
2.1 Is Fingerprint recognition better............................................................................................3
2.2 Is Face recognition better.......................................................................................................4
2.3 Fingerprint identification process..........................................................................................5
2.4 Face recognition process........................................................................................................6
2.5 Advantages and disadvantages of Fingerprint recognition....................................................7
2.6 Advantages and disadvantages of Face recognition............................................................10
2.7 Examples of fingerprint recognition and face recognition..................................................11
2.8 Next generation of authentication- Apple Face ID..............................................................15
2.9 Compare fingerprint recognition and face recognition........................................................16
3.0 Conclusion...............................................................................................................................20
References......................................................................................................................................22
Table of Contents
1.0 Introduction................................................................................................................................2
1.1 Background of the study........................................................................................................3
2.0 Fingerprint recognition vs Face recognition as biometric authentication mechanism..............3
2.1 Is Fingerprint recognition better............................................................................................3
2.2 Is Face recognition better.......................................................................................................4
2.3 Fingerprint identification process..........................................................................................5
2.4 Face recognition process........................................................................................................6
2.5 Advantages and disadvantages of Fingerprint recognition....................................................7
2.6 Advantages and disadvantages of Face recognition............................................................10
2.7 Examples of fingerprint recognition and face recognition..................................................11
2.8 Next generation of authentication- Apple Face ID..............................................................15
2.9 Compare fingerprint recognition and face recognition........................................................16
3.0 Conclusion...............................................................................................................................20
References......................................................................................................................................22
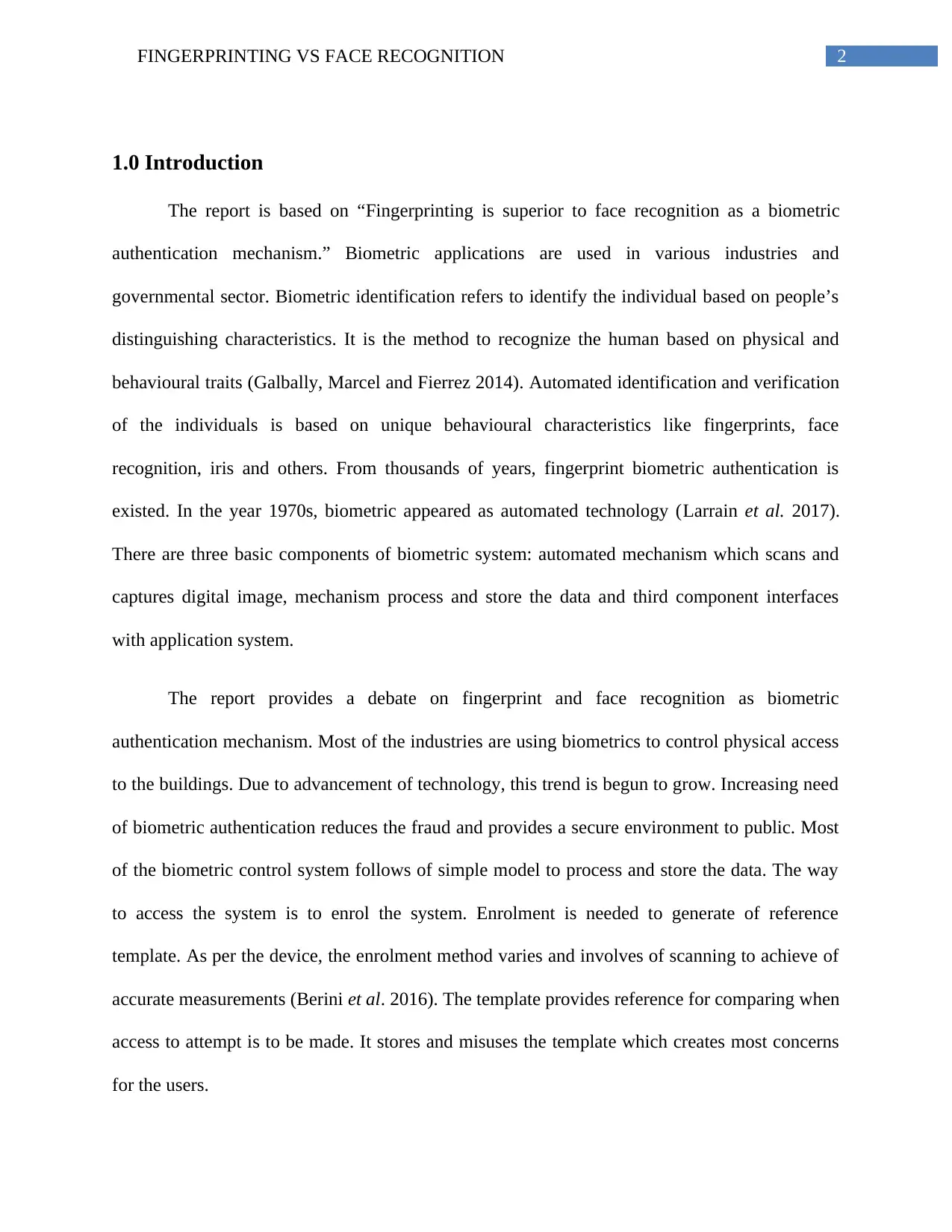
2FINGERPRINTING VS FACE RECOGNITION
1.0 Introduction
The report is based on “Fingerprinting is superior to face recognition as a biometric
authentication mechanism.” Biometric applications are used in various industries and
governmental sector. Biometric identification refers to identify the individual based on people’s
distinguishing characteristics. It is the method to recognize the human based on physical and
behavioural traits (Galbally, Marcel and Fierrez 2014). Automated identification and verification
of the individuals is based on unique behavioural characteristics like fingerprints, face
recognition, iris and others. From thousands of years, fingerprint biometric authentication is
existed. In the year 1970s, biometric appeared as automated technology (Larrain et al. 2017).
There are three basic components of biometric system: automated mechanism which scans and
captures digital image, mechanism process and store the data and third component interfaces
with application system.
The report provides a debate on fingerprint and face recognition as biometric
authentication mechanism. Most of the industries are using biometrics to control physical access
to the buildings. Due to advancement of technology, this trend is begun to grow. Increasing need
of biometric authentication reduces the fraud and provides a secure environment to public. Most
of the biometric control system follows of simple model to process and store the data. The way
to access the system is to enrol the system. Enrolment is needed to generate of reference
template. As per the device, the enrolment method varies and involves of scanning to achieve of
accurate measurements (Berini et al. 2016). The template provides reference for comparing when
access to attempt is to be made. It stores and misuses the template which creates most concerns
for the users.
1.0 Introduction
The report is based on “Fingerprinting is superior to face recognition as a biometric
authentication mechanism.” Biometric applications are used in various industries and
governmental sector. Biometric identification refers to identify the individual based on people’s
distinguishing characteristics. It is the method to recognize the human based on physical and
behavioural traits (Galbally, Marcel and Fierrez 2014). Automated identification and verification
of the individuals is based on unique behavioural characteristics like fingerprints, face
recognition, iris and others. From thousands of years, fingerprint biometric authentication is
existed. In the year 1970s, biometric appeared as automated technology (Larrain et al. 2017).
There are three basic components of biometric system: automated mechanism which scans and
captures digital image, mechanism process and store the data and third component interfaces
with application system.
The report provides a debate on fingerprint and face recognition as biometric
authentication mechanism. Most of the industries are using biometrics to control physical access
to the buildings. Due to advancement of technology, this trend is begun to grow. Increasing need
of biometric authentication reduces the fraud and provides a secure environment to public. Most
of the biometric control system follows of simple model to process and store the data. The way
to access the system is to enrol the system. Enrolment is needed to generate of reference
template. As per the device, the enrolment method varies and involves of scanning to achieve of
accurate measurements (Berini et al. 2016). The template provides reference for comparing when
access to attempt is to be made. It stores and misuses the template which creates most concerns
for the users.
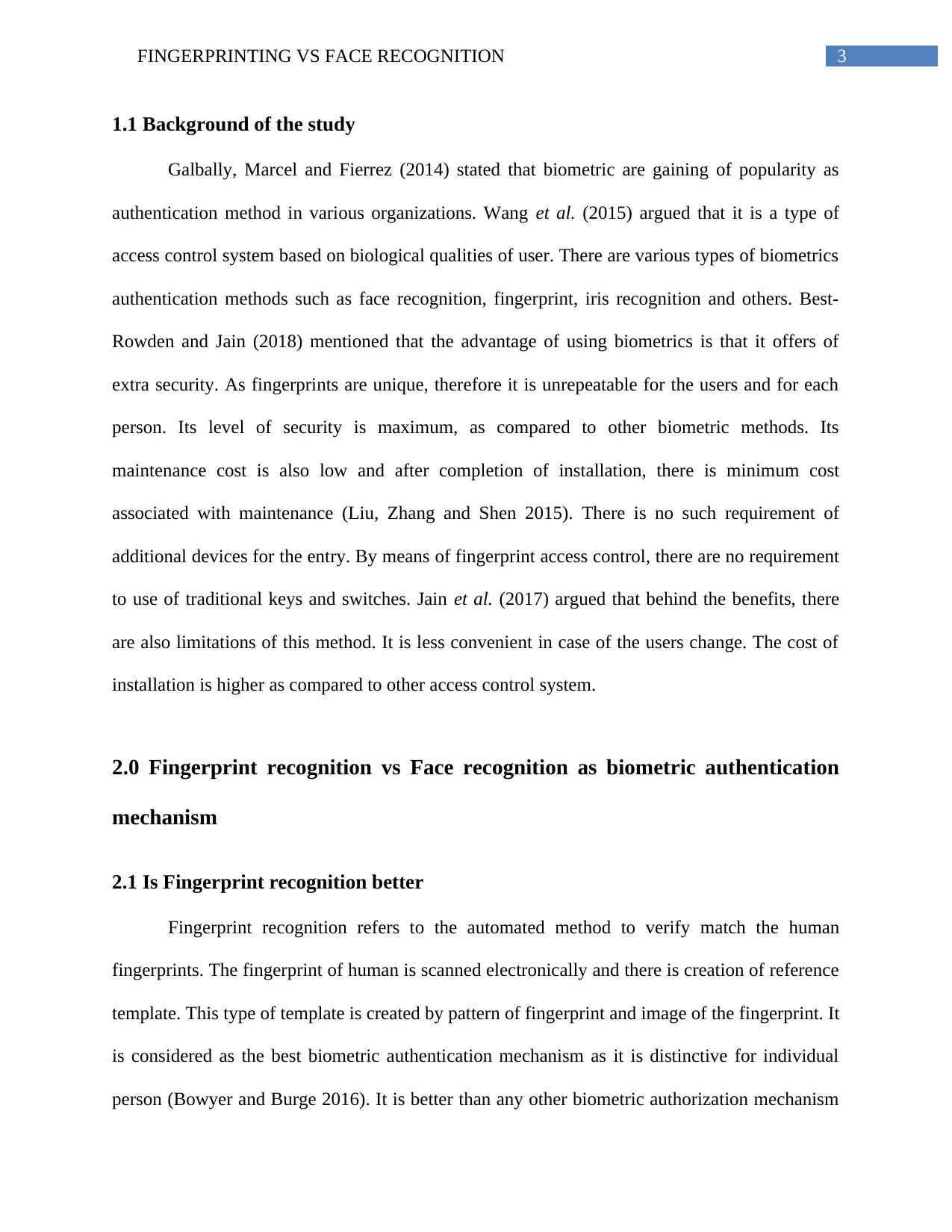
3FINGERPRINTING VS FACE RECOGNITION
1.1 Background of the study
Galbally, Marcel and Fierrez (2014) stated that biometric are gaining of popularity as
authentication method in various organizations. Wang et al. (2015) argued that it is a type of
access control system based on biological qualities of user. There are various types of biometrics
authentication methods such as face recognition, fingerprint, iris recognition and others. Best-
Rowden and Jain (2018) mentioned that the advantage of using biometrics is that it offers of
extra security. As fingerprints are unique, therefore it is unrepeatable for the users and for each
person. Its level of security is maximum, as compared to other biometric methods. Its
maintenance cost is also low and after completion of installation, there is minimum cost
associated with maintenance (Liu, Zhang and Shen 2015). There is no such requirement of
additional devices for the entry. By means of fingerprint access control, there are no requirement
to use of traditional keys and switches. Jain et al. (2017) argued that behind the benefits, there
are also limitations of this method. It is less convenient in case of the users change. The cost of
installation is higher as compared to other access control system.
2.0 Fingerprint recognition vs Face recognition as biometric authentication
mechanism
2.1 Is Fingerprint recognition better
Fingerprint recognition refers to the automated method to verify match the human
fingerprints. The fingerprint of human is scanned electronically and there is creation of reference
template. This type of template is created by pattern of fingerprint and image of the fingerprint. It
is considered as the best biometric authentication mechanism as it is distinctive for individual
person (Bowyer and Burge 2016). It is better than any other biometric authorization mechanism
1.1 Background of the study
Galbally, Marcel and Fierrez (2014) stated that biometric are gaining of popularity as
authentication method in various organizations. Wang et al. (2015) argued that it is a type of
access control system based on biological qualities of user. There are various types of biometrics
authentication methods such as face recognition, fingerprint, iris recognition and others. Best-
Rowden and Jain (2018) mentioned that the advantage of using biometrics is that it offers of
extra security. As fingerprints are unique, therefore it is unrepeatable for the users and for each
person. Its level of security is maximum, as compared to other biometric methods. Its
maintenance cost is also low and after completion of installation, there is minimum cost
associated with maintenance (Liu, Zhang and Shen 2015). There is no such requirement of
additional devices for the entry. By means of fingerprint access control, there are no requirement
to use of traditional keys and switches. Jain et al. (2017) argued that behind the benefits, there
are also limitations of this method. It is less convenient in case of the users change. The cost of
installation is higher as compared to other access control system.
2.0 Fingerprint recognition vs Face recognition as biometric authentication
mechanism
2.1 Is Fingerprint recognition better
Fingerprint recognition refers to the automated method to verify match the human
fingerprints. The fingerprint of human is scanned electronically and there is creation of reference
template. This type of template is created by pattern of fingerprint and image of the fingerprint. It
is considered as the best biometric authentication mechanism as it is distinctive for individual
person (Bowyer and Burge 2016). It is better than any other biometric authorization mechanism
Secure Best Marks with AI Grader
Need help grading? Try our AI Grader for instant feedback on your assignments.
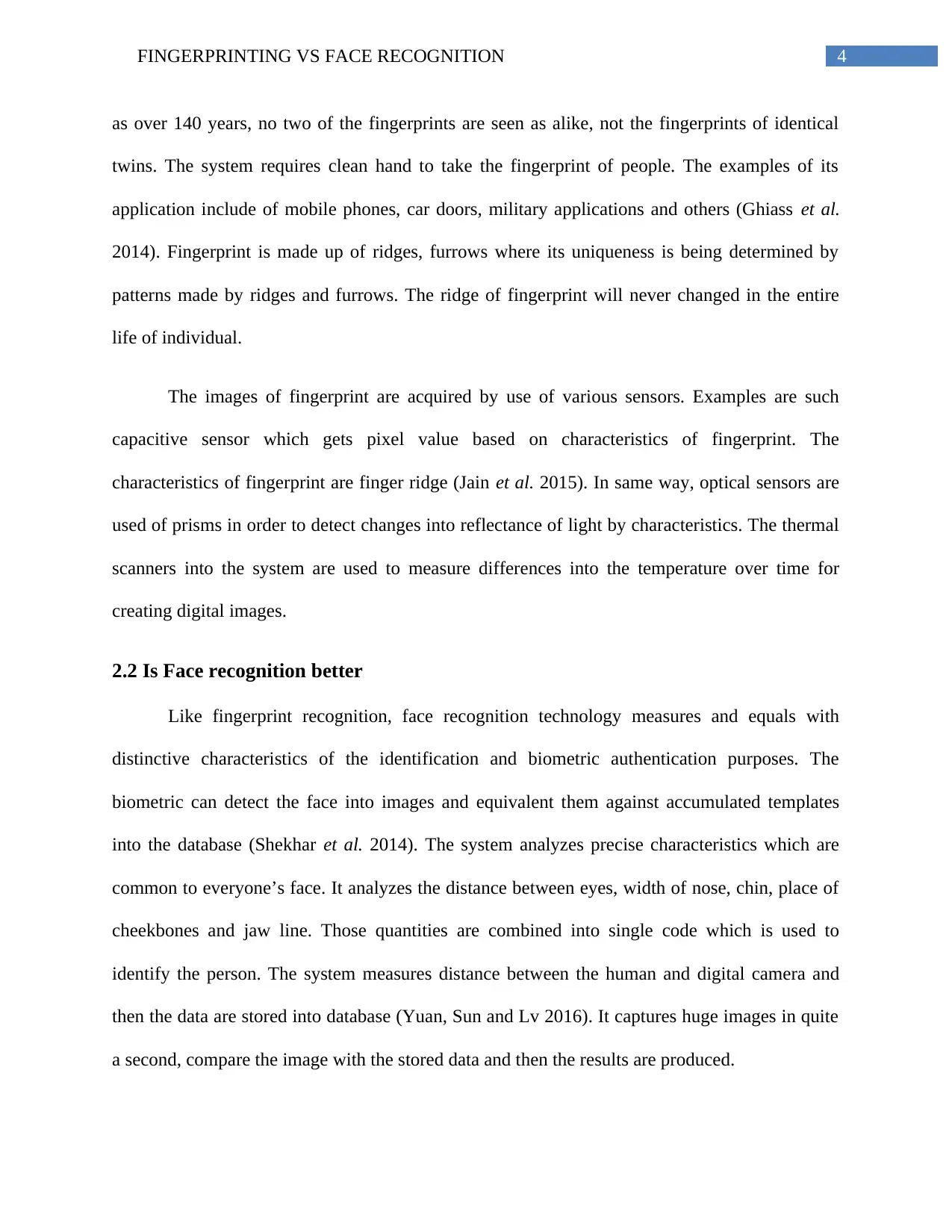
4FINGERPRINTING VS FACE RECOGNITION
as over 140 years, no two of the fingerprints are seen as alike, not the fingerprints of identical
twins. The system requires clean hand to take the fingerprint of people. The examples of its
application include of mobile phones, car doors, military applications and others (Ghiass et al.
2014). Fingerprint is made up of ridges, furrows where its uniqueness is being determined by
patterns made by ridges and furrows. The ridge of fingerprint will never changed in the entire
life of individual.
The images of fingerprint are acquired by use of various sensors. Examples are such
capacitive sensor which gets pixel value based on characteristics of fingerprint. The
characteristics of fingerprint are finger ridge (Jain et al. 2015). In same way, optical sensors are
used of prisms in order to detect changes into reflectance of light by characteristics. The thermal
scanners into the system are used to measure differences into the temperature over time for
creating digital images.
2.2 Is Face recognition better
Like fingerprint recognition, face recognition technology measures and equals with
distinctive characteristics of the identification and biometric authentication purposes. The
biometric can detect the face into images and equivalent them against accumulated templates
into the database (Shekhar et al. 2014). The system analyzes precise characteristics which are
common to everyone’s face. It analyzes the distance between eyes, width of nose, chin, place of
cheekbones and jaw line. Those quantities are combined into single code which is used to
identify the person. The system measures distance between the human and digital camera and
then the data are stored into database (Yuan, Sun and Lv 2016). It captures huge images in quite
a second, compare the image with the stored data and then the results are produced.
as over 140 years, no two of the fingerprints are seen as alike, not the fingerprints of identical
twins. The system requires clean hand to take the fingerprint of people. The examples of its
application include of mobile phones, car doors, military applications and others (Ghiass et al.
2014). Fingerprint is made up of ridges, furrows where its uniqueness is being determined by
patterns made by ridges and furrows. The ridge of fingerprint will never changed in the entire
life of individual.
The images of fingerprint are acquired by use of various sensors. Examples are such
capacitive sensor which gets pixel value based on characteristics of fingerprint. The
characteristics of fingerprint are finger ridge (Jain et al. 2015). In same way, optical sensors are
used of prisms in order to detect changes into reflectance of light by characteristics. The thermal
scanners into the system are used to measure differences into the temperature over time for
creating digital images.
2.2 Is Face recognition better
Like fingerprint recognition, face recognition technology measures and equals with
distinctive characteristics of the identification and biometric authentication purposes. The
biometric can detect the face into images and equivalent them against accumulated templates
into the database (Shekhar et al. 2014). The system analyzes precise characteristics which are
common to everyone’s face. It analyzes the distance between eyes, width of nose, chin, place of
cheekbones and jaw line. Those quantities are combined into single code which is used to
identify the person. The system measures distance between the human and digital camera and
then the data are stored into database (Yuan, Sun and Lv 2016). It captures huge images in quite
a second, compare the image with the stored data and then the results are produced.
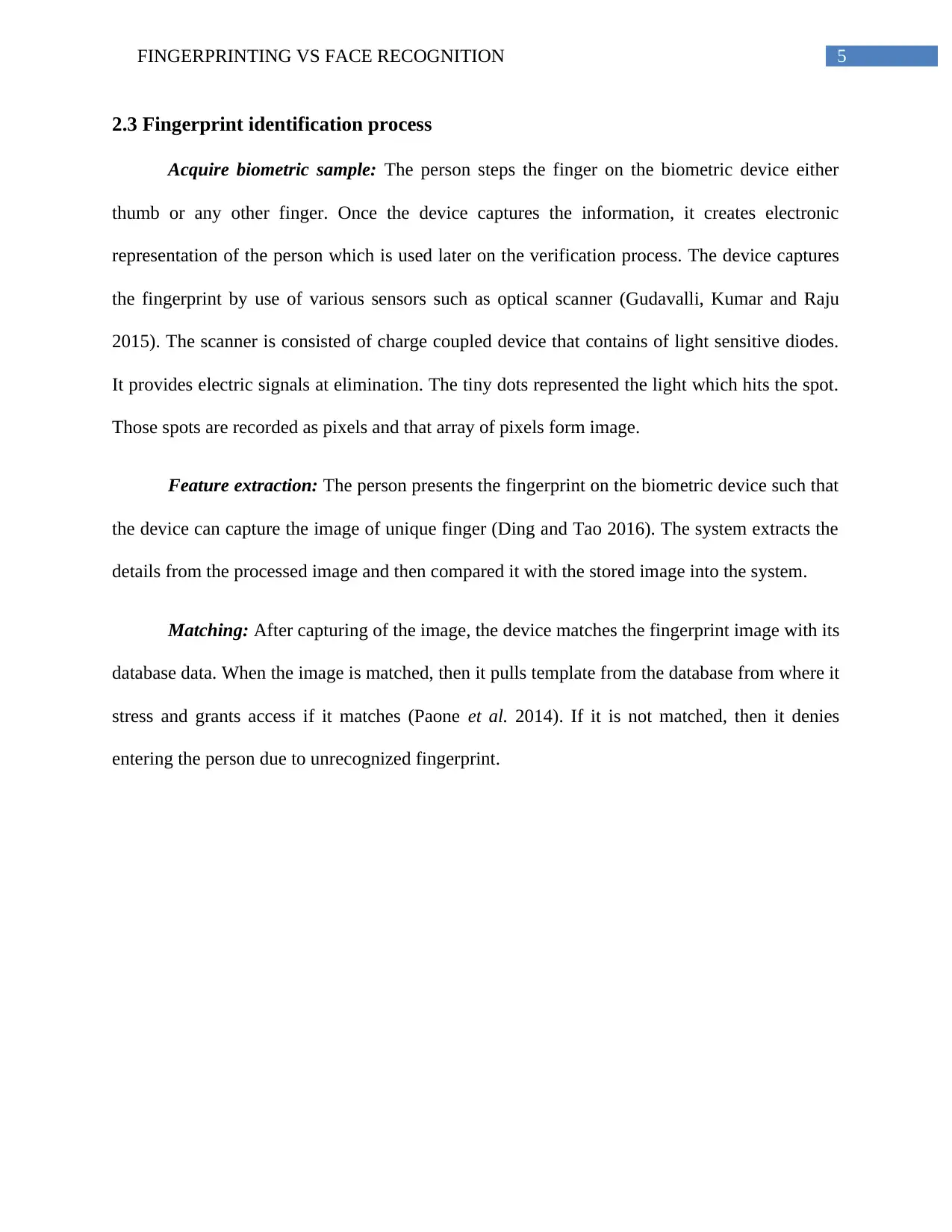
5FINGERPRINTING VS FACE RECOGNITION
2.3 Fingerprint identification process
Acquire biometric sample: The person steps the finger on the biometric device either
thumb or any other finger. Once the device captures the information, it creates electronic
representation of the person which is used later on the verification process. The device captures
the fingerprint by use of various sensors such as optical scanner (Gudavalli, Kumar and Raju
2015). The scanner is consisted of charge coupled device that contains of light sensitive diodes.
It provides electric signals at elimination. The tiny dots represented the light which hits the spot.
Those spots are recorded as pixels and that array of pixels form image.
Feature extraction: The person presents the fingerprint on the biometric device such that
the device can capture the image of unique finger (Ding and Tao 2016). The system extracts the
details from the processed image and then compared it with the stored image into the system.
Matching: After capturing of the image, the device matches the fingerprint image with its
database data. When the image is matched, then it pulls template from the database from where it
stress and grants access if it matches (Paone et al. 2014). If it is not matched, then it denies
entering the person due to unrecognized fingerprint.
2.3 Fingerprint identification process
Acquire biometric sample: The person steps the finger on the biometric device either
thumb or any other finger. Once the device captures the information, it creates electronic
representation of the person which is used later on the verification process. The device captures
the fingerprint by use of various sensors such as optical scanner (Gudavalli, Kumar and Raju
2015). The scanner is consisted of charge coupled device that contains of light sensitive diodes.
It provides electric signals at elimination. The tiny dots represented the light which hits the spot.
Those spots are recorded as pixels and that array of pixels form image.
Feature extraction: The person presents the fingerprint on the biometric device such that
the device can capture the image of unique finger (Ding and Tao 2016). The system extracts the
details from the processed image and then compared it with the stored image into the system.
Matching: After capturing of the image, the device matches the fingerprint image with its
database data. When the image is matched, then it pulls template from the database from where it
stress and grants access if it matches (Paone et al. 2014). If it is not matched, then it denies
entering the person due to unrecognized fingerprint.
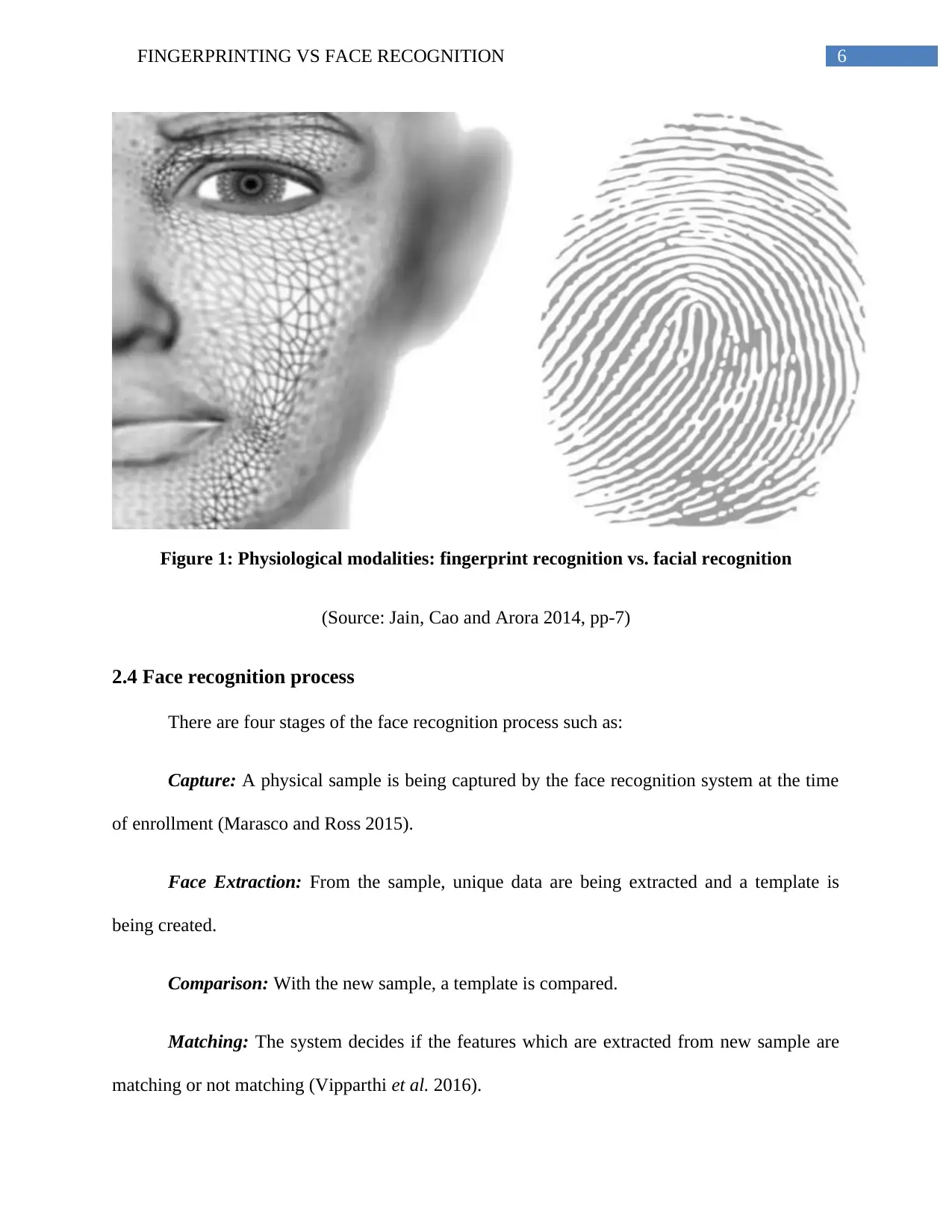
6FINGERPRINTING VS FACE RECOGNITION
Figure 1: Physiological modalities: fingerprint recognition vs. facial recognition
(Source: Jain, Cao and Arora 2014, pp-7)
2.4 Face recognition process
There are four stages of the face recognition process such as:
Capture: A physical sample is being captured by the face recognition system at the time
of enrollment (Marasco and Ross 2015).
Face Extraction: From the sample, unique data are being extracted and a template is
being created.
Comparison: With the new sample, a template is compared.
Matching: The system decides if the features which are extracted from new sample are
matching or not matching (Vipparthi et al. 2016).
Figure 1: Physiological modalities: fingerprint recognition vs. facial recognition
(Source: Jain, Cao and Arora 2014, pp-7)
2.4 Face recognition process
There are four stages of the face recognition process such as:
Capture: A physical sample is being captured by the face recognition system at the time
of enrollment (Marasco and Ross 2015).
Face Extraction: From the sample, unique data are being extracted and a template is
being created.
Comparison: With the new sample, a template is compared.
Matching: The system decides if the features which are extracted from new sample are
matching or not matching (Vipparthi et al. 2016).
Paraphrase This Document
Need a fresh take? Get an instant paraphrase of this document with our AI Paraphraser
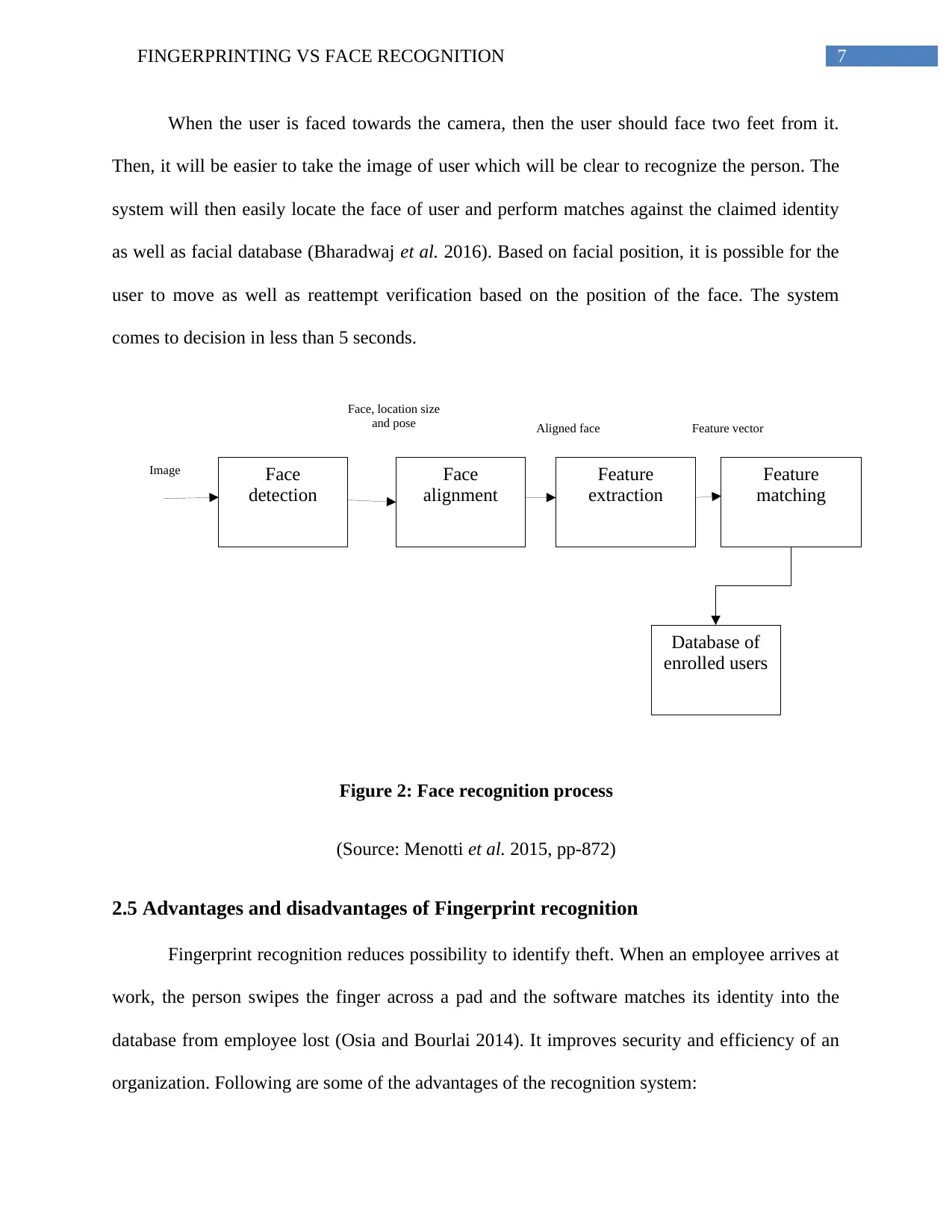
7FINGERPRINTING VS FACE RECOGNITION
Face
detection
Face
alignment
Feature
extraction
Feature
matching
Database of
enrolled users
Feature vector
Face, location size
and pose Aligned face
Image
When the user is faced towards the camera, then the user should face two feet from it.
Then, it will be easier to take the image of user which will be clear to recognize the person. The
system will then easily locate the face of user and perform matches against the claimed identity
as well as facial database (Bharadwaj et al. 2016). Based on facial position, it is possible for the
user to move as well as reattempt verification based on the position of the face. The system
comes to decision in less than 5 seconds.
Figure 2: Face recognition process
(Source: Menotti et al. 2015, pp-872)
2.5 Advantages and disadvantages of Fingerprint recognition
Fingerprint recognition reduces possibility to identify theft. When an employee arrives at
work, the person swipes the finger across a pad and the software matches its identity into the
database from employee lost (Osia and Bourlai 2014). It improves security and efficiency of an
organization. Following are some of the advantages of the recognition system:
Face
detection
Face
alignment
Feature
extraction
Feature
matching
Database of
enrolled users
Feature vector
Face, location size
and pose Aligned face
Image
When the user is faced towards the camera, then the user should face two feet from it.
Then, it will be easier to take the image of user which will be clear to recognize the person. The
system will then easily locate the face of user and perform matches against the claimed identity
as well as facial database (Bharadwaj et al. 2016). Based on facial position, it is possible for the
user to move as well as reattempt verification based on the position of the face. The system
comes to decision in less than 5 seconds.
Figure 2: Face recognition process
(Source: Menotti et al. 2015, pp-872)
2.5 Advantages and disadvantages of Fingerprint recognition
Fingerprint recognition reduces possibility to identify theft. When an employee arrives at
work, the person swipes the finger across a pad and the software matches its identity into the
database from employee lost (Osia and Bourlai 2014). It improves security and efficiency of an
organization. Following are some of the advantages of the recognition system:
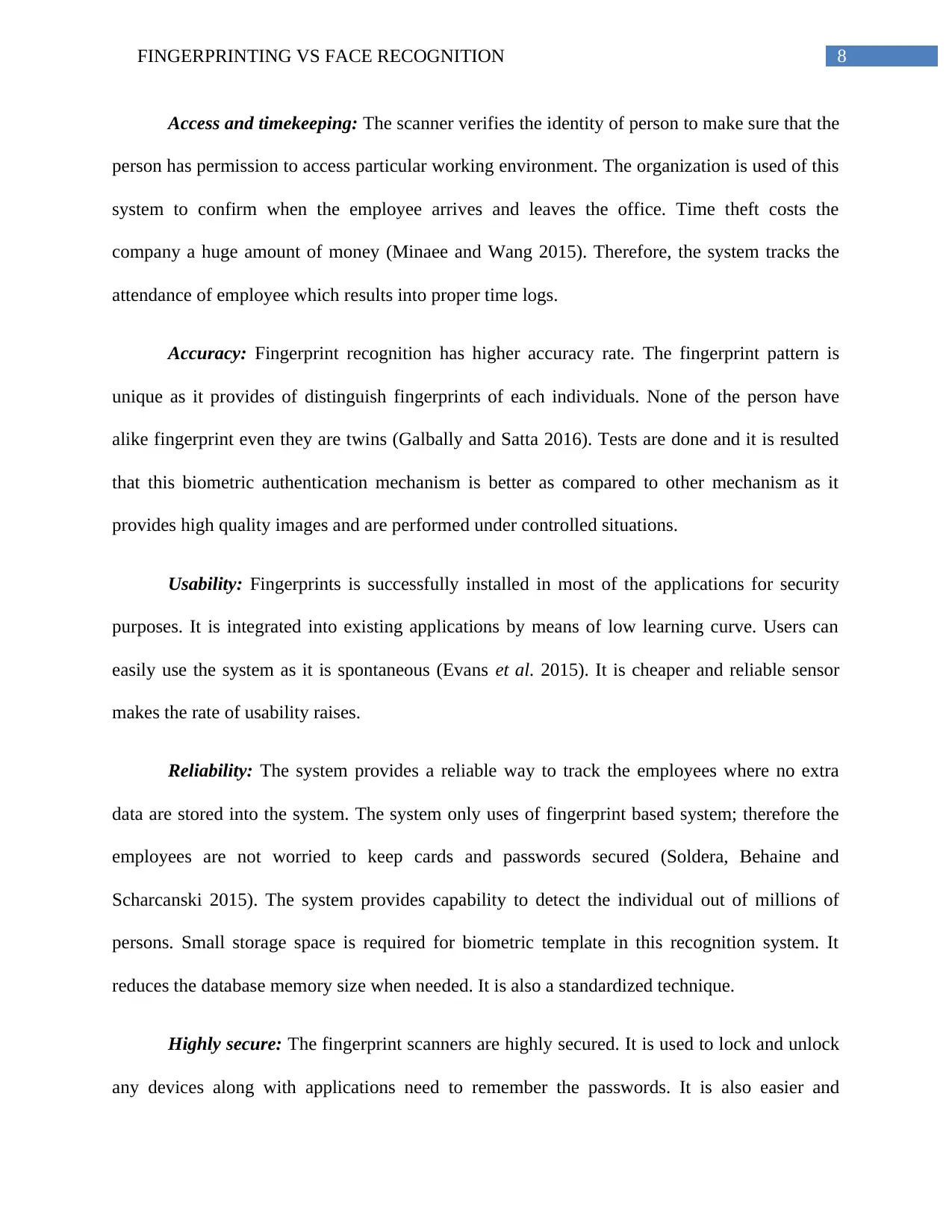
8FINGERPRINTING VS FACE RECOGNITION
Access and timekeeping: The scanner verifies the identity of person to make sure that the
person has permission to access particular working environment. The organization is used of this
system to confirm when the employee arrives and leaves the office. Time theft costs the
company a huge amount of money (Minaee and Wang 2015). Therefore, the system tracks the
attendance of employee which results into proper time logs.
Accuracy: Fingerprint recognition has higher accuracy rate. The fingerprint pattern is
unique as it provides of distinguish fingerprints of each individuals. None of the person have
alike fingerprint even they are twins (Galbally and Satta 2016). Tests are done and it is resulted
that this biometric authentication mechanism is better as compared to other mechanism as it
provides high quality images and are performed under controlled situations.
Usability: Fingerprints is successfully installed in most of the applications for security
purposes. It is integrated into existing applications by means of low learning curve. Users can
easily use the system as it is spontaneous (Evans et al. 2015). It is cheaper and reliable sensor
makes the rate of usability raises.
Reliability: The system provides a reliable way to track the employees where no extra
data are stored into the system. The system only uses of fingerprint based system; therefore the
employees are not worried to keep cards and passwords secured (Soldera, Behaine and
Scharcanski 2015). The system provides capability to detect the individual out of millions of
persons. Small storage space is required for biometric template in this recognition system. It
reduces the database memory size when needed. It is also a standardized technique.
Highly secure: The fingerprint scanners are highly secured. It is used to lock and unlock
any devices along with applications need to remember the passwords. It is also easier and
Access and timekeeping: The scanner verifies the identity of person to make sure that the
person has permission to access particular working environment. The organization is used of this
system to confirm when the employee arrives and leaves the office. Time theft costs the
company a huge amount of money (Minaee and Wang 2015). Therefore, the system tracks the
attendance of employee which results into proper time logs.
Accuracy: Fingerprint recognition has higher accuracy rate. The fingerprint pattern is
unique as it provides of distinguish fingerprints of each individuals. None of the person have
alike fingerprint even they are twins (Galbally and Satta 2016). Tests are done and it is resulted
that this biometric authentication mechanism is better as compared to other mechanism as it
provides high quality images and are performed under controlled situations.
Usability: Fingerprints is successfully installed in most of the applications for security
purposes. It is integrated into existing applications by means of low learning curve. Users can
easily use the system as it is spontaneous (Evans et al. 2015). It is cheaper and reliable sensor
makes the rate of usability raises.
Reliability: The system provides a reliable way to track the employees where no extra
data are stored into the system. The system only uses of fingerprint based system; therefore the
employees are not worried to keep cards and passwords secured (Soldera, Behaine and
Scharcanski 2015). The system provides capability to detect the individual out of millions of
persons. Small storage space is required for biometric template in this recognition system. It
reduces the database memory size when needed. It is also a standardized technique.
Highly secure: The fingerprint scanners are highly secured. It is used to lock and unlock
any devices along with applications need to remember the passwords. It is also easier and
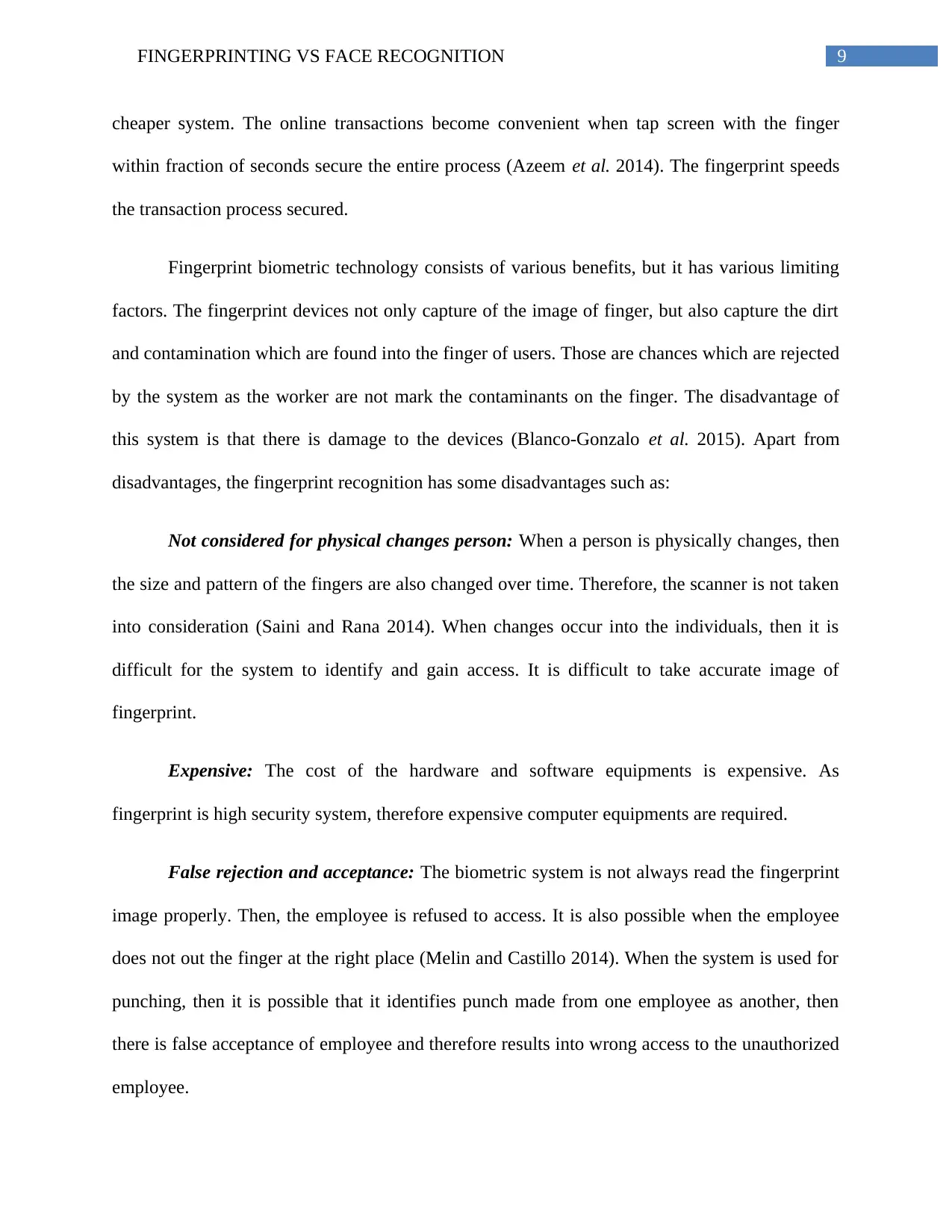
9FINGERPRINTING VS FACE RECOGNITION
cheaper system. The online transactions become convenient when tap screen with the finger
within fraction of seconds secure the entire process (Azeem et al. 2014). The fingerprint speeds
the transaction process secured.
Fingerprint biometric technology consists of various benefits, but it has various limiting
factors. The fingerprint devices not only capture of the image of finger, but also capture the dirt
and contamination which are found into the finger of users. Those are chances which are rejected
by the system as the worker are not mark the contaminants on the finger. The disadvantage of
this system is that there is damage to the devices (Blanco-Gonzalo et al. 2015). Apart from
disadvantages, the fingerprint recognition has some disadvantages such as:
Not considered for physical changes person: When a person is physically changes, then
the size and pattern of the fingers are also changed over time. Therefore, the scanner is not taken
into consideration (Saini and Rana 2014). When changes occur into the individuals, then it is
difficult for the system to identify and gain access. It is difficult to take accurate image of
fingerprint.
Expensive: The cost of the hardware and software equipments is expensive. As
fingerprint is high security system, therefore expensive computer equipments are required.
False rejection and acceptance: The biometric system is not always read the fingerprint
image properly. Then, the employee is refused to access. It is also possible when the employee
does not out the finger at the right place (Melin and Castillo 2014). When the system is used for
punching, then it is possible that it identifies punch made from one employee as another, then
there is false acceptance of employee and therefore results into wrong access to the unauthorized
employee.
cheaper system. The online transactions become convenient when tap screen with the finger
within fraction of seconds secure the entire process (Azeem et al. 2014). The fingerprint speeds
the transaction process secured.
Fingerprint biometric technology consists of various benefits, but it has various limiting
factors. The fingerprint devices not only capture of the image of finger, but also capture the dirt
and contamination which are found into the finger of users. Those are chances which are rejected
by the system as the worker are not mark the contaminants on the finger. The disadvantage of
this system is that there is damage to the devices (Blanco-Gonzalo et al. 2015). Apart from
disadvantages, the fingerprint recognition has some disadvantages such as:
Not considered for physical changes person: When a person is physically changes, then
the size and pattern of the fingers are also changed over time. Therefore, the scanner is not taken
into consideration (Saini and Rana 2014). When changes occur into the individuals, then it is
difficult for the system to identify and gain access. It is difficult to take accurate image of
fingerprint.
Expensive: The cost of the hardware and software equipments is expensive. As
fingerprint is high security system, therefore expensive computer equipments are required.
False rejection and acceptance: The biometric system is not always read the fingerprint
image properly. Then, the employee is refused to access. It is also possible when the employee
does not out the finger at the right place (Melin and Castillo 2014). When the system is used for
punching, then it is possible that it identifies punch made from one employee as another, then
there is false acceptance of employee and therefore results into wrong access to the unauthorized
employee.
Secure Best Marks with AI Grader
Need help grading? Try our AI Grader for instant feedback on your assignments.
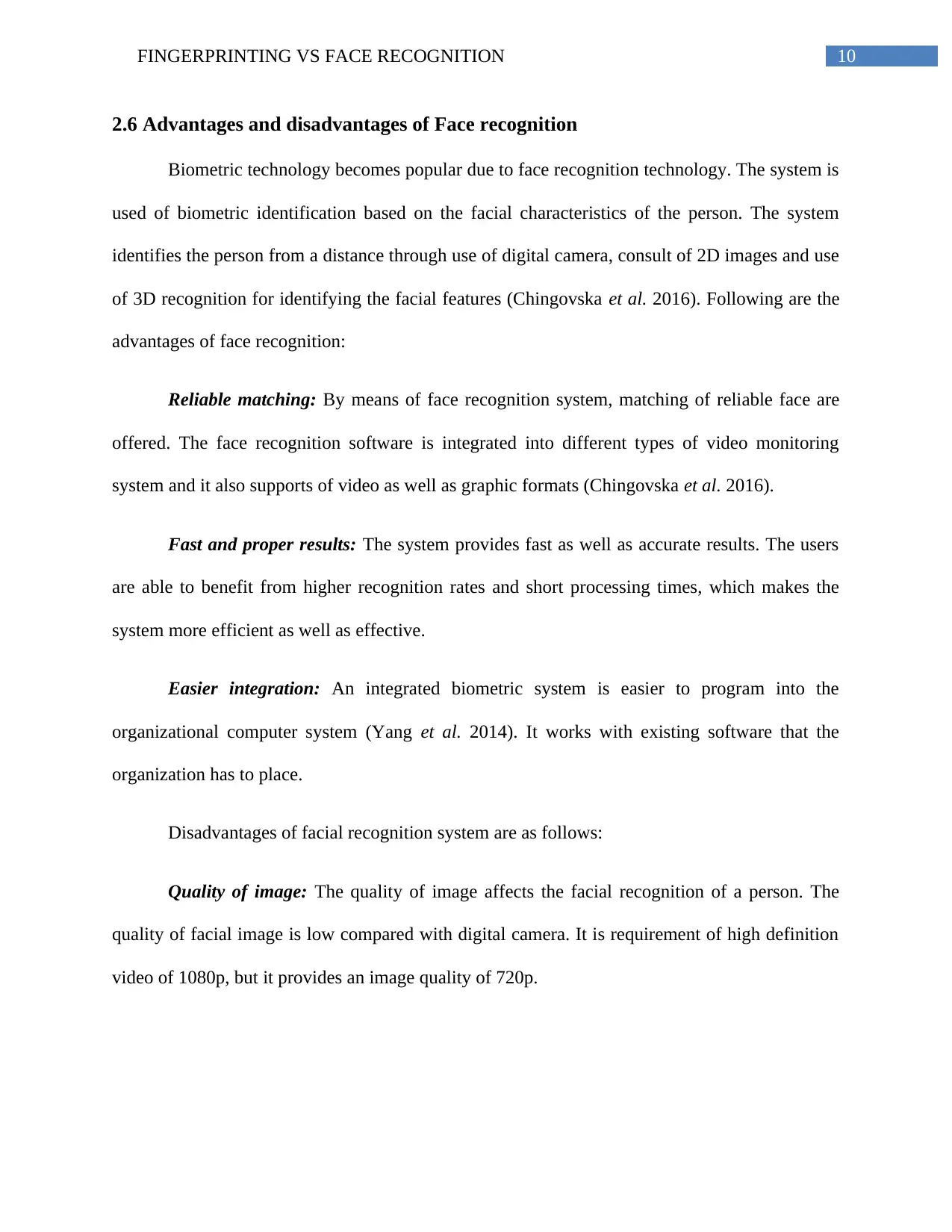
10FINGERPRINTING VS FACE RECOGNITION
2.6 Advantages and disadvantages of Face recognition
Biometric technology becomes popular due to face recognition technology. The system is
used of biometric identification based on the facial characteristics of the person. The system
identifies the person from a distance through use of digital camera, consult of 2D images and use
of 3D recognition for identifying the facial features (Chingovska et al. 2016). Following are the
advantages of face recognition:
Reliable matching: By means of face recognition system, matching of reliable face are
offered. The face recognition software is integrated into different types of video monitoring
system and it also supports of video as well as graphic formats (Chingovska et al. 2016).
Fast and proper results: The system provides fast as well as accurate results. The users
are able to benefit from higher recognition rates and short processing times, which makes the
system more efficient as well as effective.
Easier integration: An integrated biometric system is easier to program into the
organizational computer system (Yang et al. 2014). It works with existing software that the
organization has to place.
Disadvantages of facial recognition system are as follows:
Quality of image: The quality of image affects the facial recognition of a person. The
quality of facial image is low compared with digital camera. It is requirement of high definition
video of 1080p, but it provides an image quality of 720p.
2.6 Advantages and disadvantages of Face recognition
Biometric technology becomes popular due to face recognition technology. The system is
used of biometric identification based on the facial characteristics of the person. The system
identifies the person from a distance through use of digital camera, consult of 2D images and use
of 3D recognition for identifying the facial features (Chingovska et al. 2016). Following are the
advantages of face recognition:
Reliable matching: By means of face recognition system, matching of reliable face are
offered. The face recognition software is integrated into different types of video monitoring
system and it also supports of video as well as graphic formats (Chingovska et al. 2016).
Fast and proper results: The system provides fast as well as accurate results. The users
are able to benefit from higher recognition rates and short processing times, which makes the
system more efficient as well as effective.
Easier integration: An integrated biometric system is easier to program into the
organizational computer system (Yang et al. 2014). It works with existing software that the
organization has to place.
Disadvantages of facial recognition system are as follows:
Quality of image: The quality of image affects the facial recognition of a person. The
quality of facial image is low compared with digital camera. It is requirement of high definition
video of 1080p, but it provides an image quality of 720p.
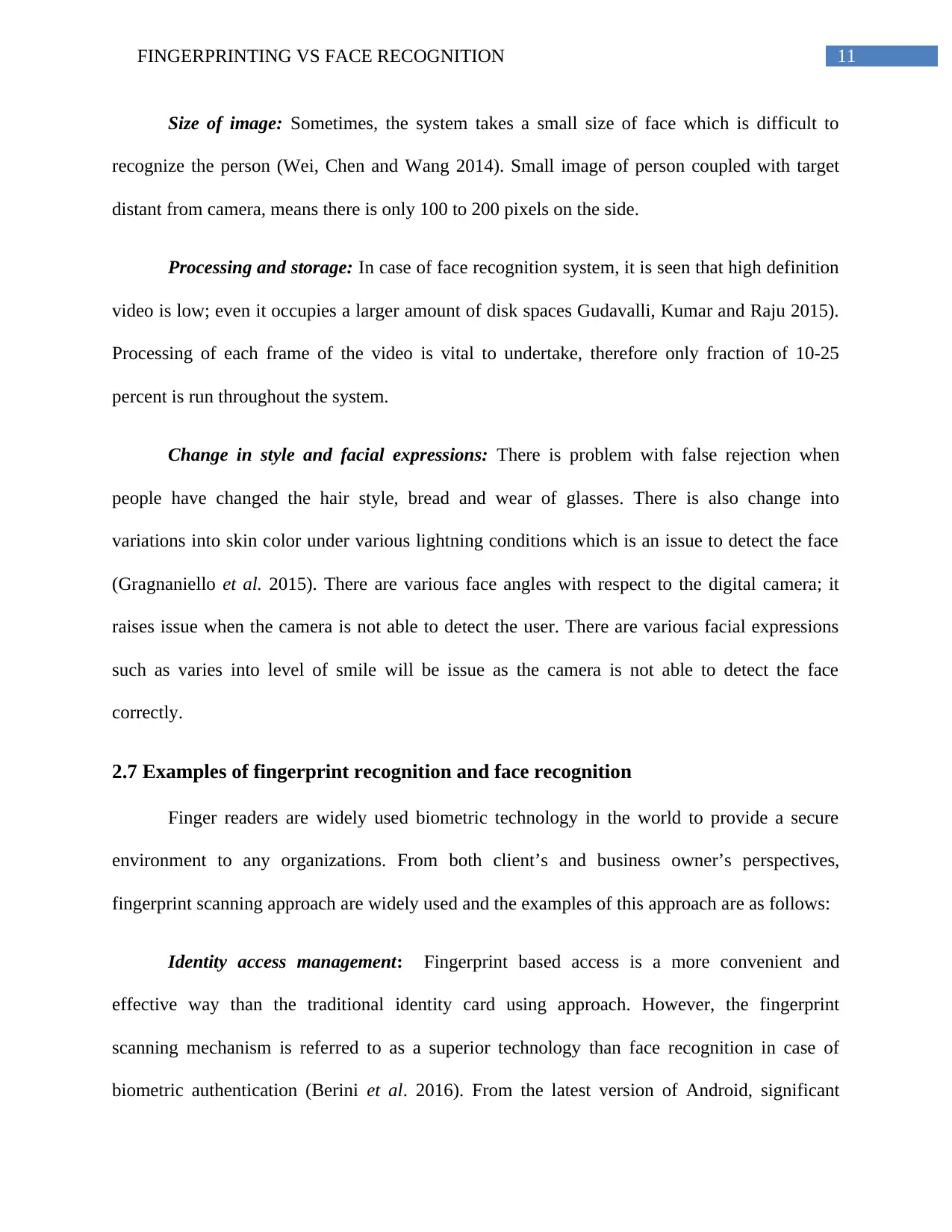
11FINGERPRINTING VS FACE RECOGNITION
Size of image: Sometimes, the system takes a small size of face which is difficult to
recognize the person (Wei, Chen and Wang 2014). Small image of person coupled with target
distant from camera, means there is only 100 to 200 pixels on the side.
Processing and storage: In case of face recognition system, it is seen that high definition
video is low; even it occupies a larger amount of disk spaces Gudavalli, Kumar and Raju 2015).
Processing of each frame of the video is vital to undertake, therefore only fraction of 10-25
percent is run throughout the system.
Change in style and facial expressions: There is problem with false rejection when
people have changed the hair style, bread and wear of glasses. There is also change into
variations into skin color under various lightning conditions which is an issue to detect the face
(Gragnaniello et al. 2015). There are various face angles with respect to the digital camera; it
raises issue when the camera is not able to detect the user. There are various facial expressions
such as varies into level of smile will be issue as the camera is not able to detect the face
correctly.
2.7 Examples of fingerprint recognition and face recognition
Finger readers are widely used biometric technology in the world to provide a secure
environment to any organizations. From both client’s and business owner’s perspectives,
fingerprint scanning approach are widely used and the examples of this approach are as follows:
Identity access management: Fingerprint based access is a more convenient and
effective way than the traditional identity card using approach. However, the fingerprint
scanning mechanism is referred to as a superior technology than face recognition in case of
biometric authentication (Berini et al. 2016). From the latest version of Android, significant
Size of image: Sometimes, the system takes a small size of face which is difficult to
recognize the person (Wei, Chen and Wang 2014). Small image of person coupled with target
distant from camera, means there is only 100 to 200 pixels on the side.
Processing and storage: In case of face recognition system, it is seen that high definition
video is low; even it occupies a larger amount of disk spaces Gudavalli, Kumar and Raju 2015).
Processing of each frame of the video is vital to undertake, therefore only fraction of 10-25
percent is run throughout the system.
Change in style and facial expressions: There is problem with false rejection when
people have changed the hair style, bread and wear of glasses. There is also change into
variations into skin color under various lightning conditions which is an issue to detect the face
(Gragnaniello et al. 2015). There are various face angles with respect to the digital camera; it
raises issue when the camera is not able to detect the user. There are various facial expressions
such as varies into level of smile will be issue as the camera is not able to detect the face
correctly.
2.7 Examples of fingerprint recognition and face recognition
Finger readers are widely used biometric technology in the world to provide a secure
environment to any organizations. From both client’s and business owner’s perspectives,
fingerprint scanning approach are widely used and the examples of this approach are as follows:
Identity access management: Fingerprint based access is a more convenient and
effective way than the traditional identity card using approach. However, the fingerprint
scanning mechanism is referred to as a superior technology than face recognition in case of
biometric authentication (Berini et al. 2016). From the latest version of Android, significant
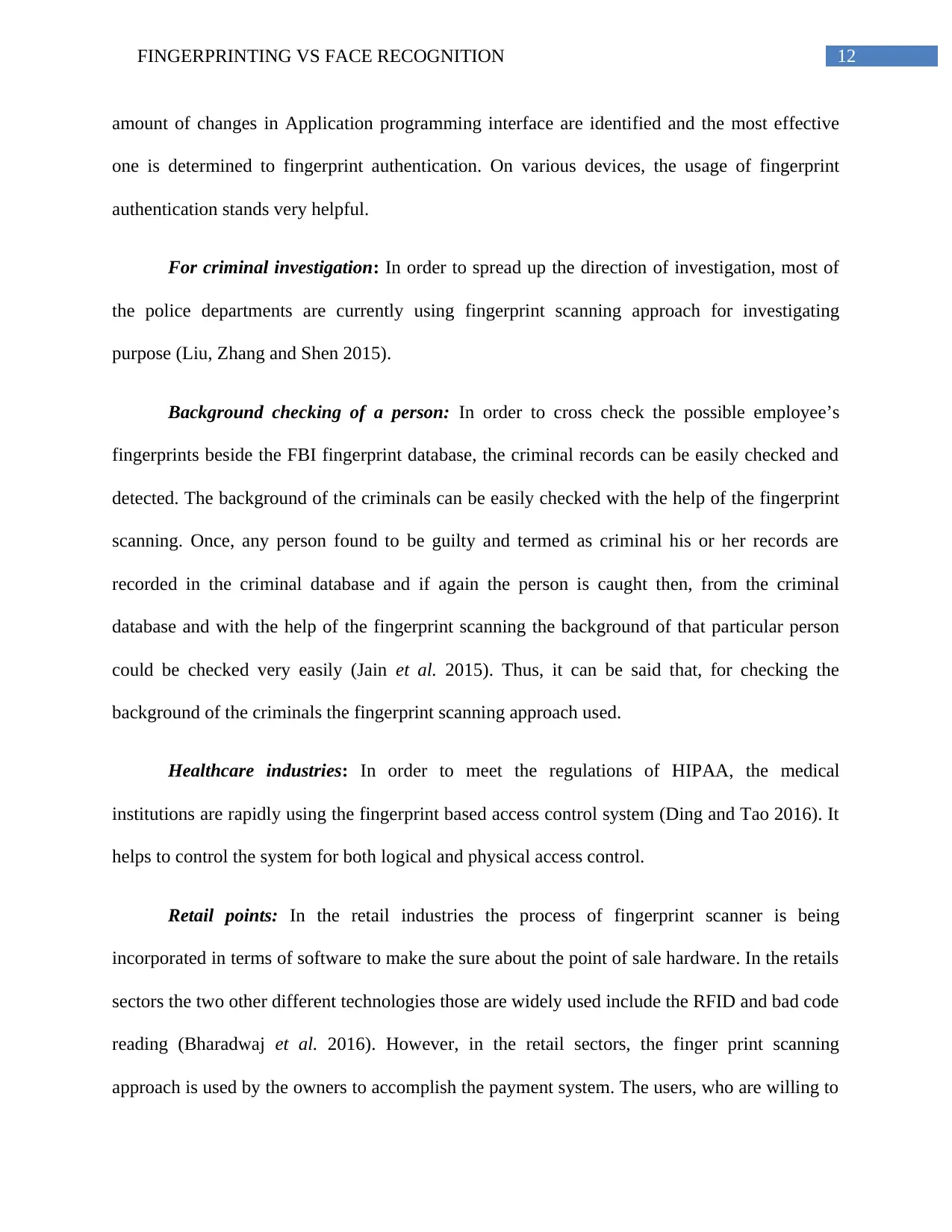
12FINGERPRINTING VS FACE RECOGNITION
amount of changes in Application programming interface are identified and the most effective
one is determined to fingerprint authentication. On various devices, the usage of fingerprint
authentication stands very helpful.
For criminal investigation: In order to spread up the direction of investigation, most of
the police departments are currently using fingerprint scanning approach for investigating
purpose (Liu, Zhang and Shen 2015).
Background checking of a person: In order to cross check the possible employee’s
fingerprints beside the FBI fingerprint database, the criminal records can be easily checked and
detected. The background of the criminals can be easily checked with the help of the fingerprint
scanning. Once, any person found to be guilty and termed as criminal his or her records are
recorded in the criminal database and if again the person is caught then, from the criminal
database and with the help of the fingerprint scanning the background of that particular person
could be checked very easily (Jain et al. 2015). Thus, it can be said that, for checking the
background of the criminals the fingerprint scanning approach used.
Healthcare industries: In order to meet the regulations of HIPAA, the medical
institutions are rapidly using the fingerprint based access control system (Ding and Tao 2016). It
helps to control the system for both logical and physical access control.
Retail points: In the retail industries the process of fingerprint scanner is being
incorporated in terms of software to make the sure about the point of sale hardware. In the retails
sectors the two other different technologies those are widely used include the RFID and bad code
reading (Bharadwaj et al. 2016). However, in the retail sectors, the finger print scanning
approach is used by the owners to accomplish the payment system. The users, who are willing to
amount of changes in Application programming interface are identified and the most effective
one is determined to fingerprint authentication. On various devices, the usage of fingerprint
authentication stands very helpful.
For criminal investigation: In order to spread up the direction of investigation, most of
the police departments are currently using fingerprint scanning approach for investigating
purpose (Liu, Zhang and Shen 2015).
Background checking of a person: In order to cross check the possible employee’s
fingerprints beside the FBI fingerprint database, the criminal records can be easily checked and
detected. The background of the criminals can be easily checked with the help of the fingerprint
scanning. Once, any person found to be guilty and termed as criminal his or her records are
recorded in the criminal database and if again the person is caught then, from the criminal
database and with the help of the fingerprint scanning the background of that particular person
could be checked very easily (Jain et al. 2015). Thus, it can be said that, for checking the
background of the criminals the fingerprint scanning approach used.
Healthcare industries: In order to meet the regulations of HIPAA, the medical
institutions are rapidly using the fingerprint based access control system (Ding and Tao 2016). It
helps to control the system for both logical and physical access control.
Retail points: In the retail industries the process of fingerprint scanner is being
incorporated in terms of software to make the sure about the point of sale hardware. In the retails
sectors the two other different technologies those are widely used include the RFID and bad code
reading (Bharadwaj et al. 2016). However, in the retail sectors, the finger print scanning
approach is used by the owners to accomplish the payment system. The users, who are willing to
Paraphrase This Document
Need a fresh take? Get an instant paraphrase of this document with our AI Paraphraser
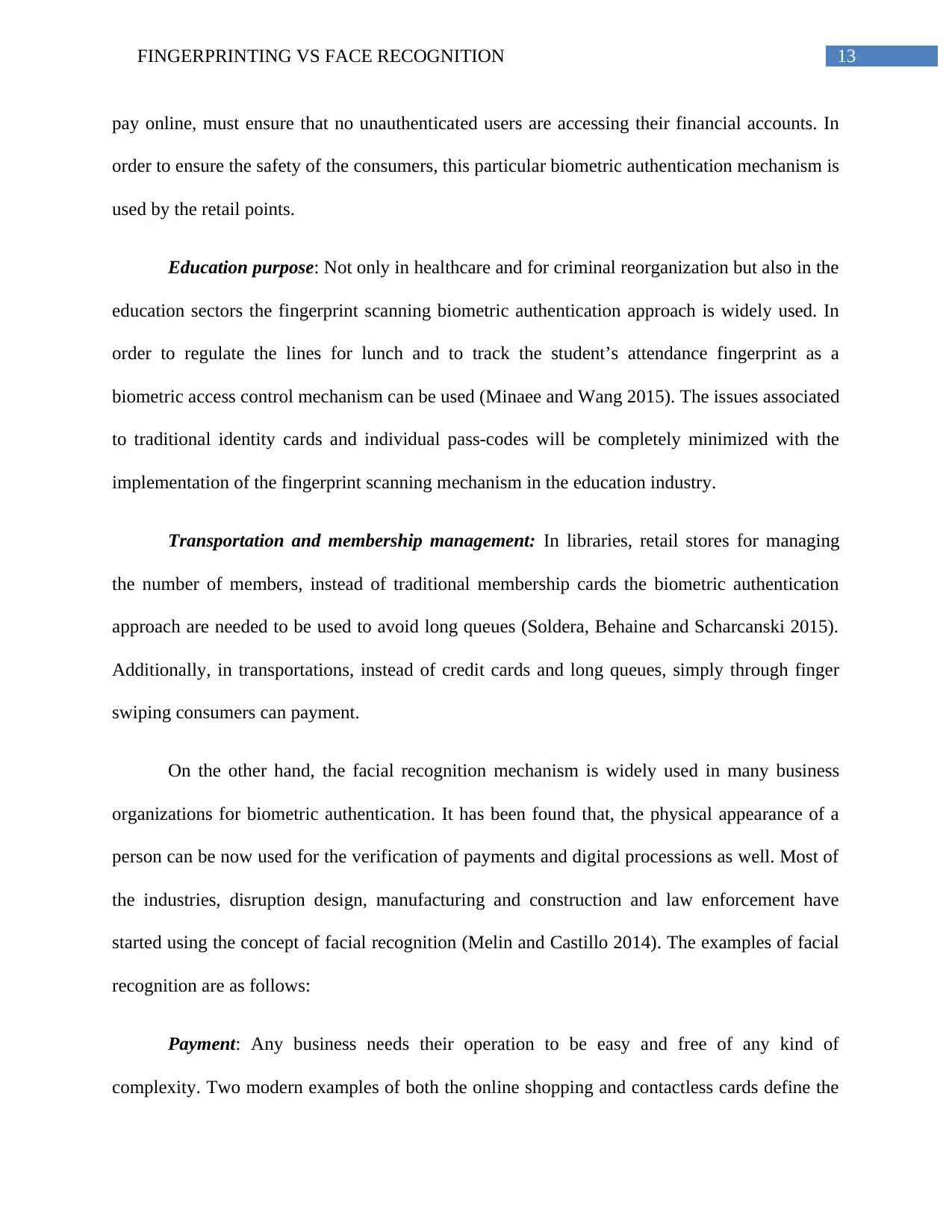
13FINGERPRINTING VS FACE RECOGNITION
pay online, must ensure that no unauthenticated users are accessing their financial accounts. In
order to ensure the safety of the consumers, this particular biometric authentication mechanism is
used by the retail points.
Education purpose: Not only in healthcare and for criminal reorganization but also in the
education sectors the fingerprint scanning biometric authentication approach is widely used. In
order to regulate the lines for lunch and to track the student’s attendance fingerprint as a
biometric access control mechanism can be used (Minaee and Wang 2015). The issues associated
to traditional identity cards and individual pass-codes will be completely minimized with the
implementation of the fingerprint scanning mechanism in the education industry.
Transportation and membership management: In libraries, retail stores for managing
the number of members, instead of traditional membership cards the biometric authentication
approach are needed to be used to avoid long queues (Soldera, Behaine and Scharcanski 2015).
Additionally, in transportations, instead of credit cards and long queues, simply through finger
swiping consumers can payment.
On the other hand, the facial recognition mechanism is widely used in many business
organizations for biometric authentication. It has been found that, the physical appearance of a
person can be now used for the verification of payments and digital processions as well. Most of
the industries, disruption design, manufacturing and construction and law enforcement have
started using the concept of facial recognition (Melin and Castillo 2014). The examples of facial
recognition are as follows:
Payment: Any business needs their operation to be easy and free of any kind of
complexity. Two modern examples of both the online shopping and contactless cards define the
pay online, must ensure that no unauthenticated users are accessing their financial accounts. In
order to ensure the safety of the consumers, this particular biometric authentication mechanism is
used by the retail points.
Education purpose: Not only in healthcare and for criminal reorganization but also in the
education sectors the fingerprint scanning biometric authentication approach is widely used. In
order to regulate the lines for lunch and to track the student’s attendance fingerprint as a
biometric access control mechanism can be used (Minaee and Wang 2015). The issues associated
to traditional identity cards and individual pass-codes will be completely minimized with the
implementation of the fingerprint scanning mechanism in the education industry.
Transportation and membership management: In libraries, retail stores for managing
the number of members, instead of traditional membership cards the biometric authentication
approach are needed to be used to avoid long queues (Soldera, Behaine and Scharcanski 2015).
Additionally, in transportations, instead of credit cards and long queues, simply through finger
swiping consumers can payment.
On the other hand, the facial recognition mechanism is widely used in many business
organizations for biometric authentication. It has been found that, the physical appearance of a
person can be now used for the verification of payments and digital processions as well. Most of
the industries, disruption design, manufacturing and construction and law enforcement have
started using the concept of facial recognition (Melin and Castillo 2014). The examples of facial
recognition are as follows:
Payment: Any business needs their operation to be easy and free of any kind of
complexity. Two modern examples of both the online shopping and contactless cards define the
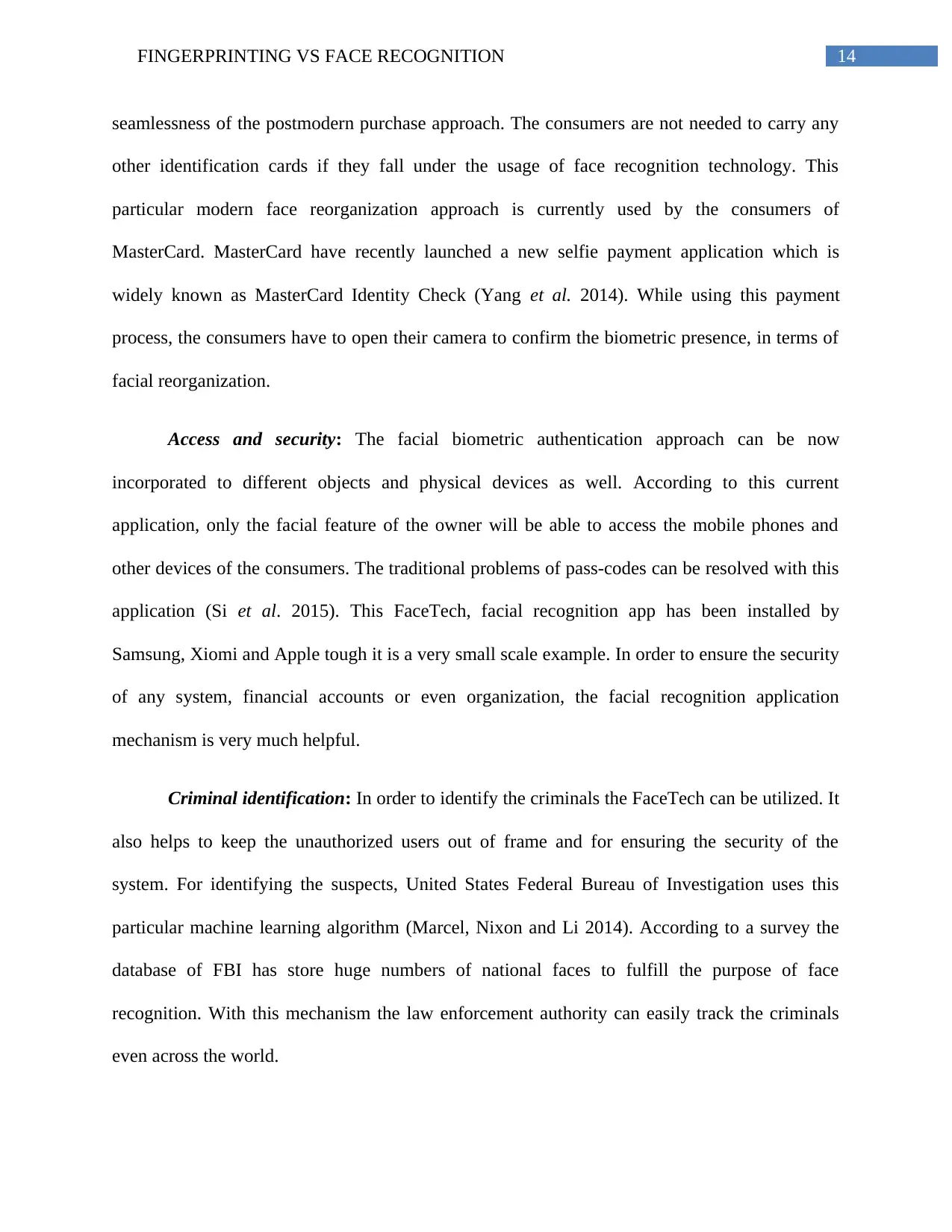
14FINGERPRINTING VS FACE RECOGNITION
seamlessness of the postmodern purchase approach. The consumers are not needed to carry any
other identification cards if they fall under the usage of face recognition technology. This
particular modern face reorganization approach is currently used by the consumers of
MasterCard. MasterCard have recently launched a new selfie payment application which is
widely known as MasterCard Identity Check (Yang et al. 2014). While using this payment
process, the consumers have to open their camera to confirm the biometric presence, in terms of
facial reorganization.
Access and security: The facial biometric authentication approach can be now
incorporated to different objects and physical devices as well. According to this current
application, only the facial feature of the owner will be able to access the mobile phones and
other devices of the consumers. The traditional problems of pass-codes can be resolved with this
application (Si et al. 2015). This FaceTech, facial recognition app has been installed by
Samsung, Xiomi and Apple tough it is a very small scale example. In order to ensure the security
of any system, financial accounts or even organization, the facial recognition application
mechanism is very much helpful.
Criminal identification: In order to identify the criminals the FaceTech can be utilized. It
also helps to keep the unauthorized users out of frame and for ensuring the security of the
system. For identifying the suspects, United States Federal Bureau of Investigation uses this
particular machine learning algorithm (Marcel, Nixon and Li 2014). According to a survey the
database of FBI has store huge numbers of national faces to fulfill the purpose of face
recognition. With this mechanism the law enforcement authority can easily track the criminals
even across the world.
seamlessness of the postmodern purchase approach. The consumers are not needed to carry any
other identification cards if they fall under the usage of face recognition technology. This
particular modern face reorganization approach is currently used by the consumers of
MasterCard. MasterCard have recently launched a new selfie payment application which is
widely known as MasterCard Identity Check (Yang et al. 2014). While using this payment
process, the consumers have to open their camera to confirm the biometric presence, in terms of
facial reorganization.
Access and security: The facial biometric authentication approach can be now
incorporated to different objects and physical devices as well. According to this current
application, only the facial feature of the owner will be able to access the mobile phones and
other devices of the consumers. The traditional problems of pass-codes can be resolved with this
application (Si et al. 2015). This FaceTech, facial recognition app has been installed by
Samsung, Xiomi and Apple tough it is a very small scale example. In order to ensure the security
of any system, financial accounts or even organization, the facial recognition application
mechanism is very much helpful.
Criminal identification: In order to identify the criminals the FaceTech can be utilized. It
also helps to keep the unauthorized users out of frame and for ensuring the security of the
system. For identifying the suspects, United States Federal Bureau of Investigation uses this
particular machine learning algorithm (Marcel, Nixon and Li 2014). According to a survey the
database of FBI has store huge numbers of national faces to fulfill the purpose of face
recognition. With this mechanism the law enforcement authority can easily track the criminals
even across the world.
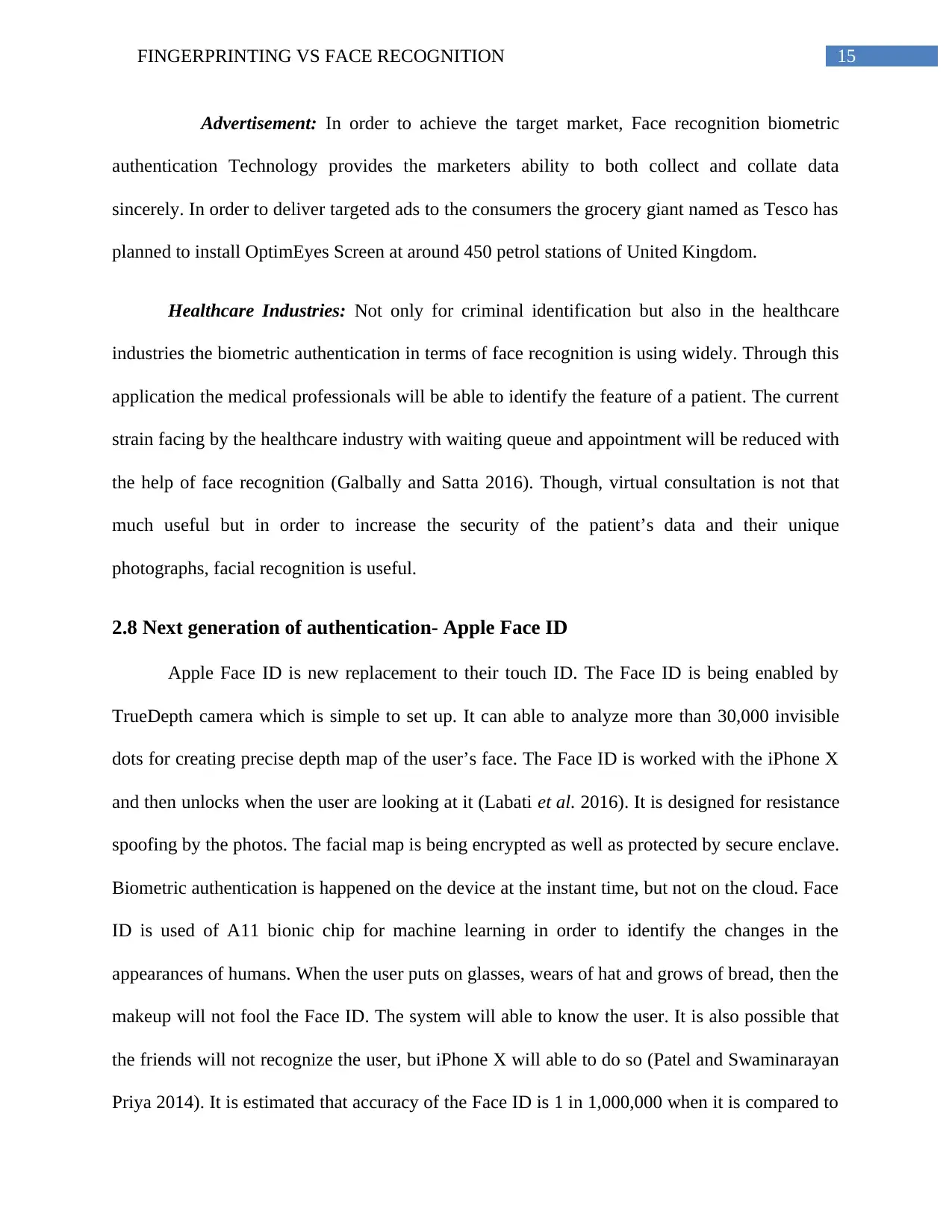
15FINGERPRINTING VS FACE RECOGNITION
Advertisement: In order to achieve the target market, Face recognition biometric
authentication Technology provides the marketers ability to both collect and collate data
sincerely. In order to deliver targeted ads to the consumers the grocery giant named as Tesco has
planned to install OptimEyes Screen at around 450 petrol stations of United Kingdom.
Healthcare Industries: Not only for criminal identification but also in the healthcare
industries the biometric authentication in terms of face recognition is using widely. Through this
application the medical professionals will be able to identify the feature of a patient. The current
strain facing by the healthcare industry with waiting queue and appointment will be reduced with
the help of face recognition (Galbally and Satta 2016). Though, virtual consultation is not that
much useful but in order to increase the security of the patient’s data and their unique
photographs, facial recognition is useful.
2.8 Next generation of authentication- Apple Face ID
Apple Face ID is new replacement to their touch ID. The Face ID is being enabled by
TrueDepth camera which is simple to set up. It can able to analyze more than 30,000 invisible
dots for creating precise depth map of the user’s face. The Face ID is worked with the iPhone X
and then unlocks when the user are looking at it (Labati et al. 2016). It is designed for resistance
spoofing by the photos. The facial map is being encrypted as well as protected by secure enclave.
Biometric authentication is happened on the device at the instant time, but not on the cloud. Face
ID is used of A11 bionic chip for machine learning in order to identify the changes in the
appearances of humans. When the user puts on glasses, wears of hat and grows of bread, then the
makeup will not fool the Face ID. The system will able to know the user. It is also possible that
the friends will not recognize the user, but iPhone X will able to do so (Patel and Swaminarayan
Priya 2014). It is estimated that accuracy of the Face ID is 1 in 1,000,000 when it is compared to
Advertisement: In order to achieve the target market, Face recognition biometric
authentication Technology provides the marketers ability to both collect and collate data
sincerely. In order to deliver targeted ads to the consumers the grocery giant named as Tesco has
planned to install OptimEyes Screen at around 450 petrol stations of United Kingdom.
Healthcare Industries: Not only for criminal identification but also in the healthcare
industries the biometric authentication in terms of face recognition is using widely. Through this
application the medical professionals will be able to identify the feature of a patient. The current
strain facing by the healthcare industry with waiting queue and appointment will be reduced with
the help of face recognition (Galbally and Satta 2016). Though, virtual consultation is not that
much useful but in order to increase the security of the patient’s data and their unique
photographs, facial recognition is useful.
2.8 Next generation of authentication- Apple Face ID
Apple Face ID is new replacement to their touch ID. The Face ID is being enabled by
TrueDepth camera which is simple to set up. It can able to analyze more than 30,000 invisible
dots for creating precise depth map of the user’s face. The Face ID is worked with the iPhone X
and then unlocks when the user are looking at it (Labati et al. 2016). It is designed for resistance
spoofing by the photos. The facial map is being encrypted as well as protected by secure enclave.
Biometric authentication is happened on the device at the instant time, but not on the cloud. Face
ID is used of A11 bionic chip for machine learning in order to identify the changes in the
appearances of humans. When the user puts on glasses, wears of hat and grows of bread, then the
makeup will not fool the Face ID. The system will able to know the user. It is also possible that
the friends will not recognize the user, but iPhone X will able to do so (Patel and Swaminarayan
Priya 2014). It is estimated that accuracy of the Face ID is 1 in 1,000,000 when it is compared to
Secure Best Marks with AI Grader
Need help grading? Try our AI Grader for instant feedback on your assignments.

16FINGERPRINTING VS FACE RECOGNITION
1 in 50,000 of the Touch ID fingerprints. The TrueDepth camera is used of 30,000 infrared dots
projected on the face for depth mapping. The infrared camera is able to read back the features as
well as below is unique characteristics of skin in order to secure the biometric image of the face.
Galaxy S8 fingerprint sensor analyzes that when the user faces the finger on sensor, then
it unlocks the phone instantaneously without pressing the power button. It is possible to register
various fingerprints and use of various fingerprints in order to verify the account of Samsung.
There is no need to enter the password (Si et al. 2015). Fingerprint is also used to pay for the
items. Face recognition into Galaxy S8 is easier to set up and it is working well under lower to
moderate lightning. The cons of face recognition is that it is less secure option as face
recognition is fooled with picture. The features are troubled into recognition of user both into
bright sunlight as well as dark (Kaur, Singh and Kumar 2014). It is not supporting Samsung Pay.
Therefore, fingerprint identification is the best biometric authentication method used.
2.9 Compare fingerprint recognition and face recognition
Fingerprint recognition Face recognition
It is intrusive as there is requirement of subject
to touch the machine to present any biometric
sample.
It is non-intrusive as the subject is not required
to present any biometric sample.
There is requirement of consent. There is no requirement of consent.
It is higher distinctiveness and unique among
people in case of twins too (Marcel, Nixon and
Li 2014).
It is low distinctiveness as it may be same in
case of twins (Gudavalli, Kumar and Raju
2015).
Highly accurate Low accuracy
The subjects are not identified from a distance. The subjects are identified from a distance.
1 in 50,000 of the Touch ID fingerprints. The TrueDepth camera is used of 30,000 infrared dots
projected on the face for depth mapping. The infrared camera is able to read back the features as
well as below is unique characteristics of skin in order to secure the biometric image of the face.
Galaxy S8 fingerprint sensor analyzes that when the user faces the finger on sensor, then
it unlocks the phone instantaneously without pressing the power button. It is possible to register
various fingerprints and use of various fingerprints in order to verify the account of Samsung.
There is no need to enter the password (Si et al. 2015). Fingerprint is also used to pay for the
items. Face recognition into Galaxy S8 is easier to set up and it is working well under lower to
moderate lightning. The cons of face recognition is that it is less secure option as face
recognition is fooled with picture. The features are troubled into recognition of user both into
bright sunlight as well as dark (Kaur, Singh and Kumar 2014). It is not supporting Samsung Pay.
Therefore, fingerprint identification is the best biometric authentication method used.
2.9 Compare fingerprint recognition and face recognition
Fingerprint recognition Face recognition
It is intrusive as there is requirement of subject
to touch the machine to present any biometric
sample.
It is non-intrusive as the subject is not required
to present any biometric sample.
There is requirement of consent. There is no requirement of consent.
It is higher distinctiveness and unique among
people in case of twins too (Marcel, Nixon and
Li 2014).
It is low distinctiveness as it may be same in
case of twins (Gudavalli, Kumar and Raju
2015).
Highly accurate Low accuracy
The subjects are not identified from a distance. The subjects are identified from a distance.
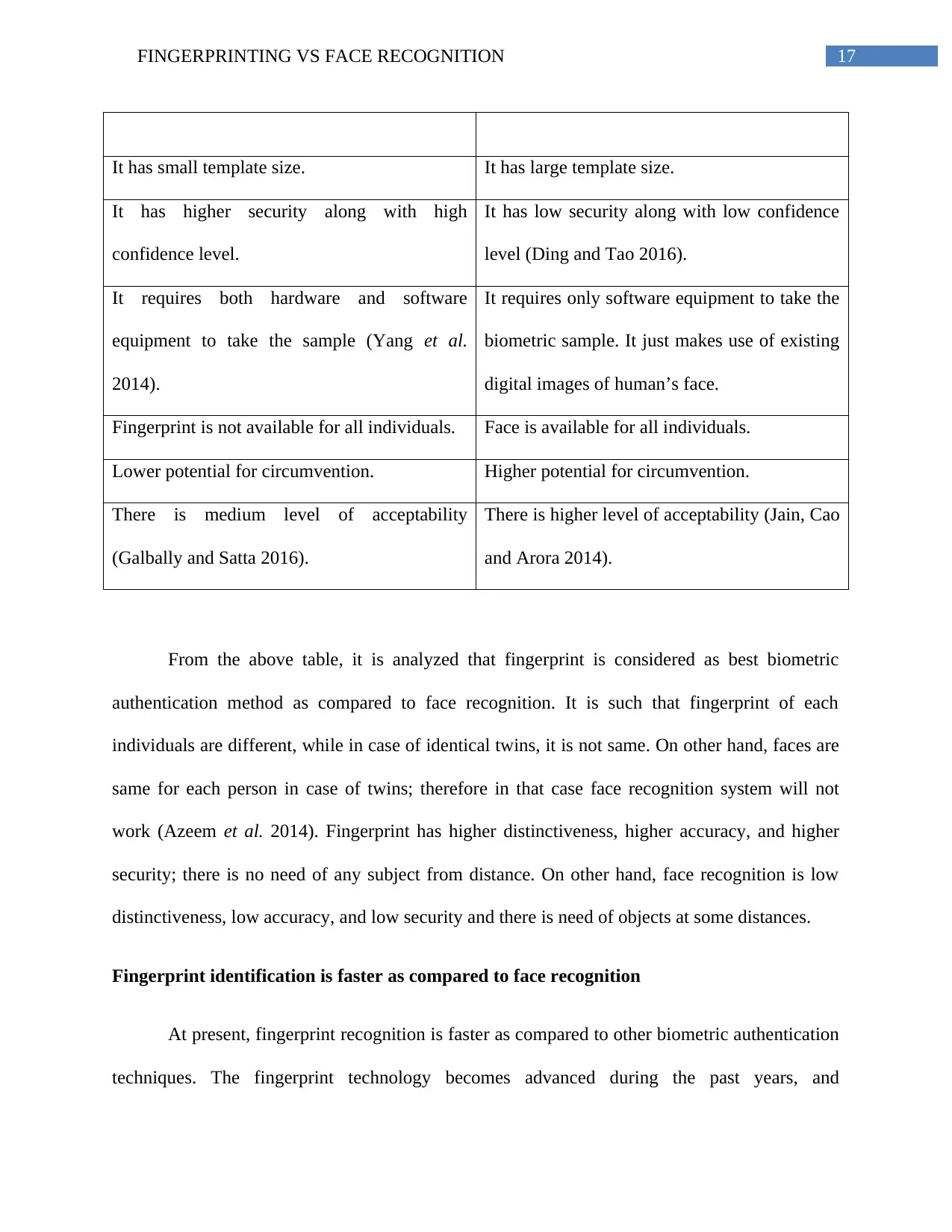
17FINGERPRINTING VS FACE RECOGNITION
It has small template size. It has large template size.
It has higher security along with high
confidence level.
It has low security along with low confidence
level (Ding and Tao 2016).
It requires both hardware and software
equipment to take the sample (Yang et al.
2014).
It requires only software equipment to take the
biometric sample. It just makes use of existing
digital images of human’s face.
Fingerprint is not available for all individuals. Face is available for all individuals.
Lower potential for circumvention. Higher potential for circumvention.
There is medium level of acceptability
(Galbally and Satta 2016).
There is higher level of acceptability (Jain, Cao
and Arora 2014).
From the above table, it is analyzed that fingerprint is considered as best biometric
authentication method as compared to face recognition. It is such that fingerprint of each
individuals are different, while in case of identical twins, it is not same. On other hand, faces are
same for each person in case of twins; therefore in that case face recognition system will not
work (Azeem et al. 2014). Fingerprint has higher distinctiveness, higher accuracy, and higher
security; there is no need of any subject from distance. On other hand, face recognition is low
distinctiveness, low accuracy, and low security and there is need of objects at some distances.
Fingerprint identification is faster as compared to face recognition
At present, fingerprint recognition is faster as compared to other biometric authentication
techniques. The fingerprint technology becomes advanced during the past years, and
It has small template size. It has large template size.
It has higher security along with high
confidence level.
It has low security along with low confidence
level (Ding and Tao 2016).
It requires both hardware and software
equipment to take the sample (Yang et al.
2014).
It requires only software equipment to take the
biometric sample. It just makes use of existing
digital images of human’s face.
Fingerprint is not available for all individuals. Face is available for all individuals.
Lower potential for circumvention. Higher potential for circumvention.
There is medium level of acceptability
(Galbally and Satta 2016).
There is higher level of acceptability (Jain, Cao
and Arora 2014).
From the above table, it is analyzed that fingerprint is considered as best biometric
authentication method as compared to face recognition. It is such that fingerprint of each
individuals are different, while in case of identical twins, it is not same. On other hand, faces are
same for each person in case of twins; therefore in that case face recognition system will not
work (Azeem et al. 2014). Fingerprint has higher distinctiveness, higher accuracy, and higher
security; there is no need of any subject from distance. On other hand, face recognition is low
distinctiveness, low accuracy, and low security and there is need of objects at some distances.
Fingerprint identification is faster as compared to face recognition
At present, fingerprint recognition is faster as compared to other biometric authentication
techniques. The fingerprint technology becomes advanced during the past years, and
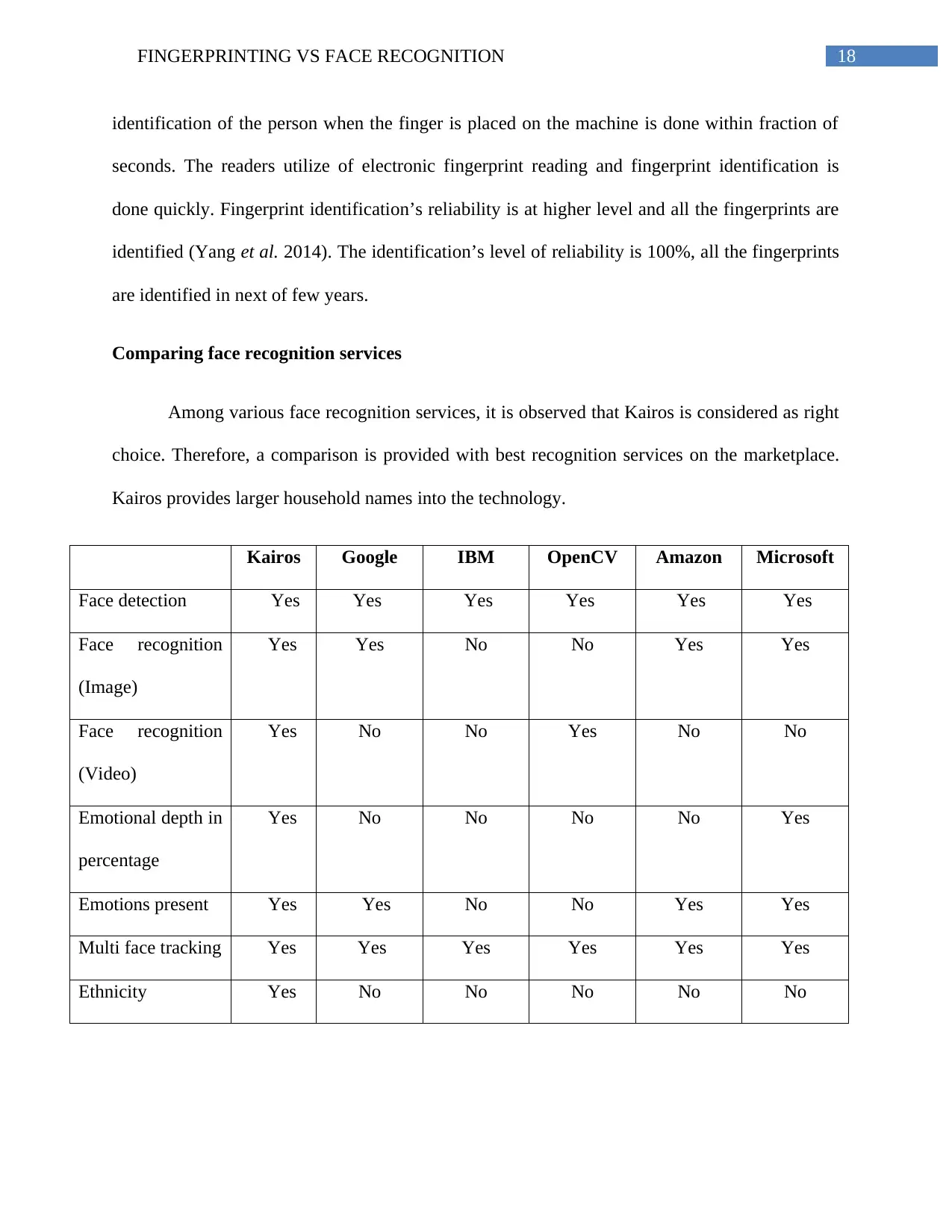
18FINGERPRINTING VS FACE RECOGNITION
identification of the person when the finger is placed on the machine is done within fraction of
seconds. The readers utilize of electronic fingerprint reading and fingerprint identification is
done quickly. Fingerprint identification’s reliability is at higher level and all the fingerprints are
identified (Yang et al. 2014). The identification’s level of reliability is 100%, all the fingerprints
are identified in next of few years.
Comparing face recognition services
Among various face recognition services, it is observed that Kairos is considered as right
choice. Therefore, a comparison is provided with best recognition services on the marketplace.
Kairos provides larger household names into the technology.
Kairos Google IBM OpenCV Amazon Microsoft
Face detection Yes Yes Yes Yes Yes Yes
Face recognition
(Image)
Yes Yes No No Yes Yes
Face recognition
(Video)
Yes No No Yes No No
Emotional depth in
percentage
Yes No No No No Yes
Emotions present Yes Yes No No Yes Yes
Multi face tracking Yes Yes Yes Yes Yes Yes
Ethnicity Yes No No No No No
identification of the person when the finger is placed on the machine is done within fraction of
seconds. The readers utilize of electronic fingerprint reading and fingerprint identification is
done quickly. Fingerprint identification’s reliability is at higher level and all the fingerprints are
identified (Yang et al. 2014). The identification’s level of reliability is 100%, all the fingerprints
are identified in next of few years.
Comparing face recognition services
Among various face recognition services, it is observed that Kairos is considered as right
choice. Therefore, a comparison is provided with best recognition services on the marketplace.
Kairos provides larger household names into the technology.
Kairos Google IBM OpenCV Amazon Microsoft
Face detection Yes Yes Yes Yes Yes Yes
Face recognition
(Image)
Yes Yes No No Yes Yes
Face recognition
(Video)
Yes No No Yes No No
Emotional depth in
percentage
Yes No No No No Yes
Emotions present Yes Yes No No Yes Yes
Multi face tracking Yes Yes Yes Yes Yes Yes
Ethnicity Yes No No No No No
Paraphrase This Document
Need a fresh take? Get an instant paraphrase of this document with our AI Paraphraser
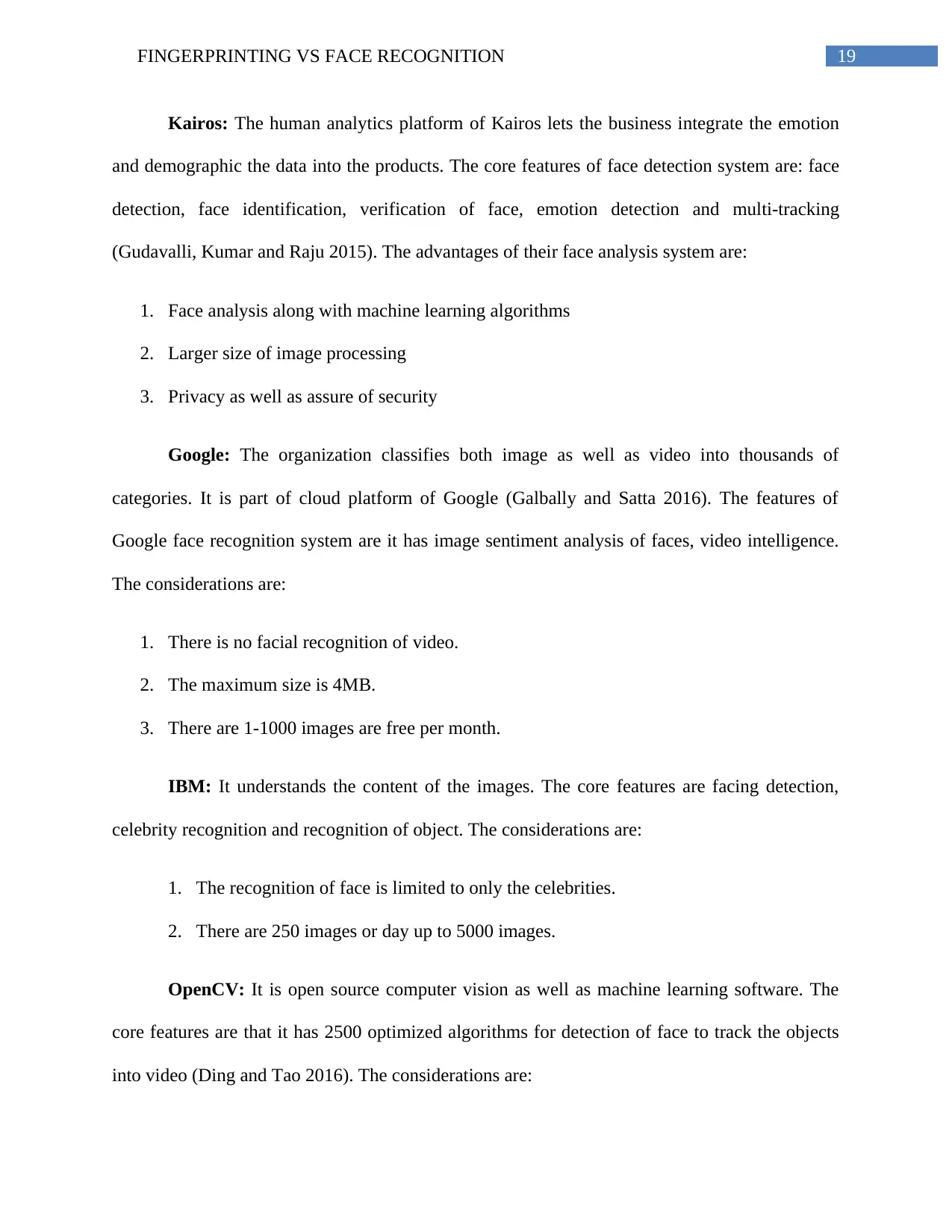
19FINGERPRINTING VS FACE RECOGNITION
Kairos: The human analytics platform of Kairos lets the business integrate the emotion
and demographic the data into the products. The core features of face detection system are: face
detection, face identification, verification of face, emotion detection and multi-tracking
(Gudavalli, Kumar and Raju 2015). The advantages of their face analysis system are:
1. Face analysis along with machine learning algorithms
2. Larger size of image processing
3. Privacy as well as assure of security
Google: The organization classifies both image as well as video into thousands of
categories. It is part of cloud platform of Google (Galbally and Satta 2016). The features of
Google face recognition system are it has image sentiment analysis of faces, video intelligence.
The considerations are:
1. There is no facial recognition of video.
2. The maximum size is 4MB.
3. There are 1-1000 images are free per month.
IBM: It understands the content of the images. The core features are facing detection,
celebrity recognition and recognition of object. The considerations are:
1. The recognition of face is limited to only the celebrities.
2. There are 250 images or day up to 5000 images.
OpenCV: It is open source computer vision as well as machine learning software. The
core features are that it has 2500 optimized algorithms for detection of face to track the objects
into video (Ding and Tao 2016). The considerations are:
Kairos: The human analytics platform of Kairos lets the business integrate the emotion
and demographic the data into the products. The core features of face detection system are: face
detection, face identification, verification of face, emotion detection and multi-tracking
(Gudavalli, Kumar and Raju 2015). The advantages of their face analysis system are:
1. Face analysis along with machine learning algorithms
2. Larger size of image processing
3. Privacy as well as assure of security
Google: The organization classifies both image as well as video into thousands of
categories. It is part of cloud platform of Google (Galbally and Satta 2016). The features of
Google face recognition system are it has image sentiment analysis of faces, video intelligence.
The considerations are:
1. There is no facial recognition of video.
2. The maximum size is 4MB.
3. There are 1-1000 images are free per month.
IBM: It understands the content of the images. The core features are facing detection,
celebrity recognition and recognition of object. The considerations are:
1. The recognition of face is limited to only the celebrities.
2. There are 250 images or day up to 5000 images.
OpenCV: It is open source computer vision as well as machine learning software. The
core features are that it has 2500 optimized algorithms for detection of face to track the objects
into video (Ding and Tao 2016). The considerations are:
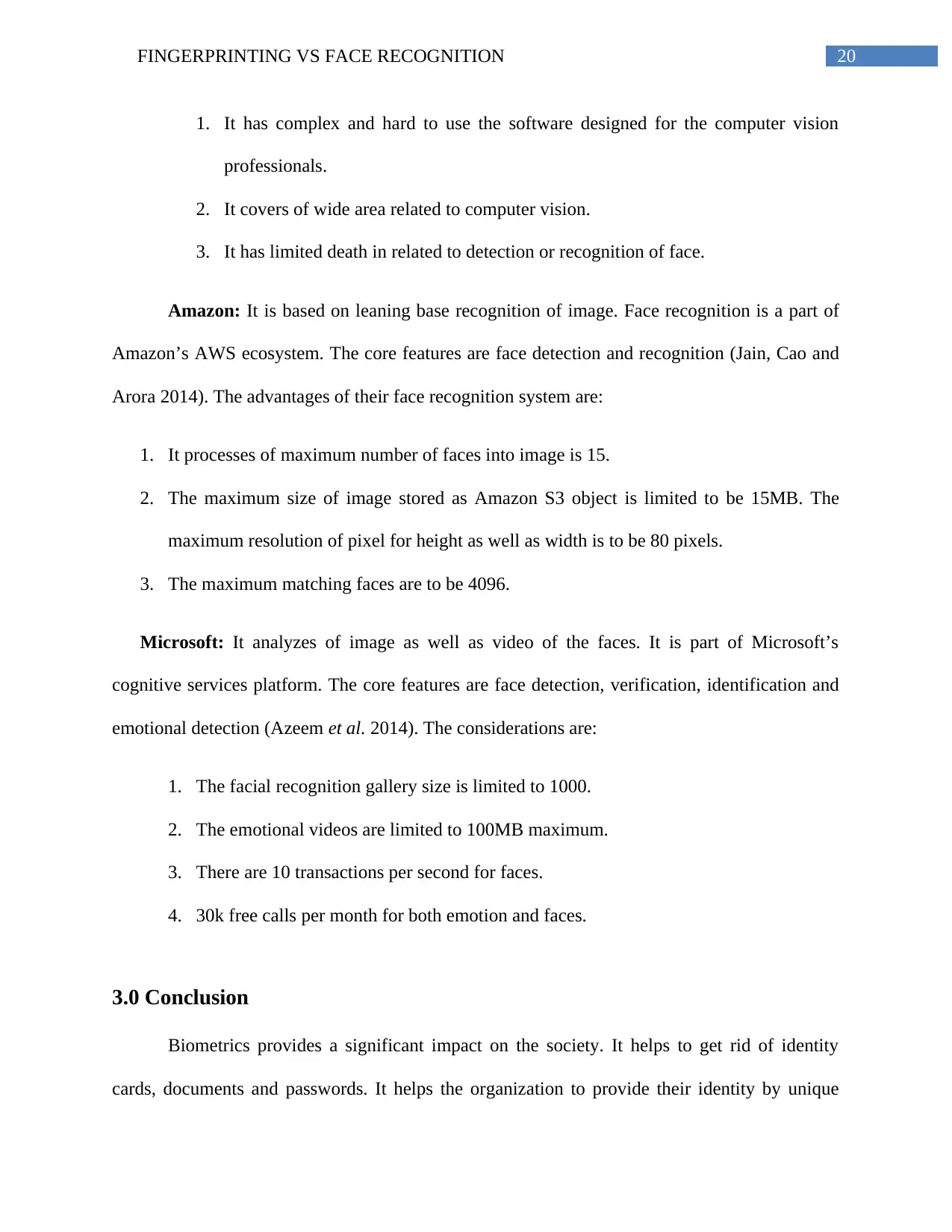
20FINGERPRINTING VS FACE RECOGNITION
1. It has complex and hard to use the software designed for the computer vision
professionals.
2. It covers of wide area related to computer vision.
3. It has limited death in related to detection or recognition of face.
Amazon: It is based on leaning base recognition of image. Face recognition is a part of
Amazon’s AWS ecosystem. The core features are face detection and recognition (Jain, Cao and
Arora 2014). The advantages of their face recognition system are:
1. It processes of maximum number of faces into image is 15.
2. The maximum size of image stored as Amazon S3 object is limited to be 15MB. The
maximum resolution of pixel for height as well as width is to be 80 pixels.
3. The maximum matching faces are to be 4096.
Microsoft: It analyzes of image as well as video of the faces. It is part of Microsoft’s
cognitive services platform. The core features are face detection, verification, identification and
emotional detection (Azeem et al. 2014). The considerations are:
1. The facial recognition gallery size is limited to 1000.
2. The emotional videos are limited to 100MB maximum.
3. There are 10 transactions per second for faces.
4. 30k free calls per month for both emotion and faces.
3.0 Conclusion
Biometrics provides a significant impact on the society. It helps to get rid of identity
cards, documents and passwords. It helps the organization to provide their identity by unique
1. It has complex and hard to use the software designed for the computer vision
professionals.
2. It covers of wide area related to computer vision.
3. It has limited death in related to detection or recognition of face.
Amazon: It is based on leaning base recognition of image. Face recognition is a part of
Amazon’s AWS ecosystem. The core features are face detection and recognition (Jain, Cao and
Arora 2014). The advantages of their face recognition system are:
1. It processes of maximum number of faces into image is 15.
2. The maximum size of image stored as Amazon S3 object is limited to be 15MB. The
maximum resolution of pixel for height as well as width is to be 80 pixels.
3. The maximum matching faces are to be 4096.
Microsoft: It analyzes of image as well as video of the faces. It is part of Microsoft’s
cognitive services platform. The core features are face detection, verification, identification and
emotional detection (Azeem et al. 2014). The considerations are:
1. The facial recognition gallery size is limited to 1000.
2. The emotional videos are limited to 100MB maximum.
3. There are 10 transactions per second for faces.
4. 30k free calls per month for both emotion and faces.
3.0 Conclusion
Biometrics provides a significant impact on the society. It helps to get rid of identity
cards, documents and passwords. It helps the organization to provide their identity by unique
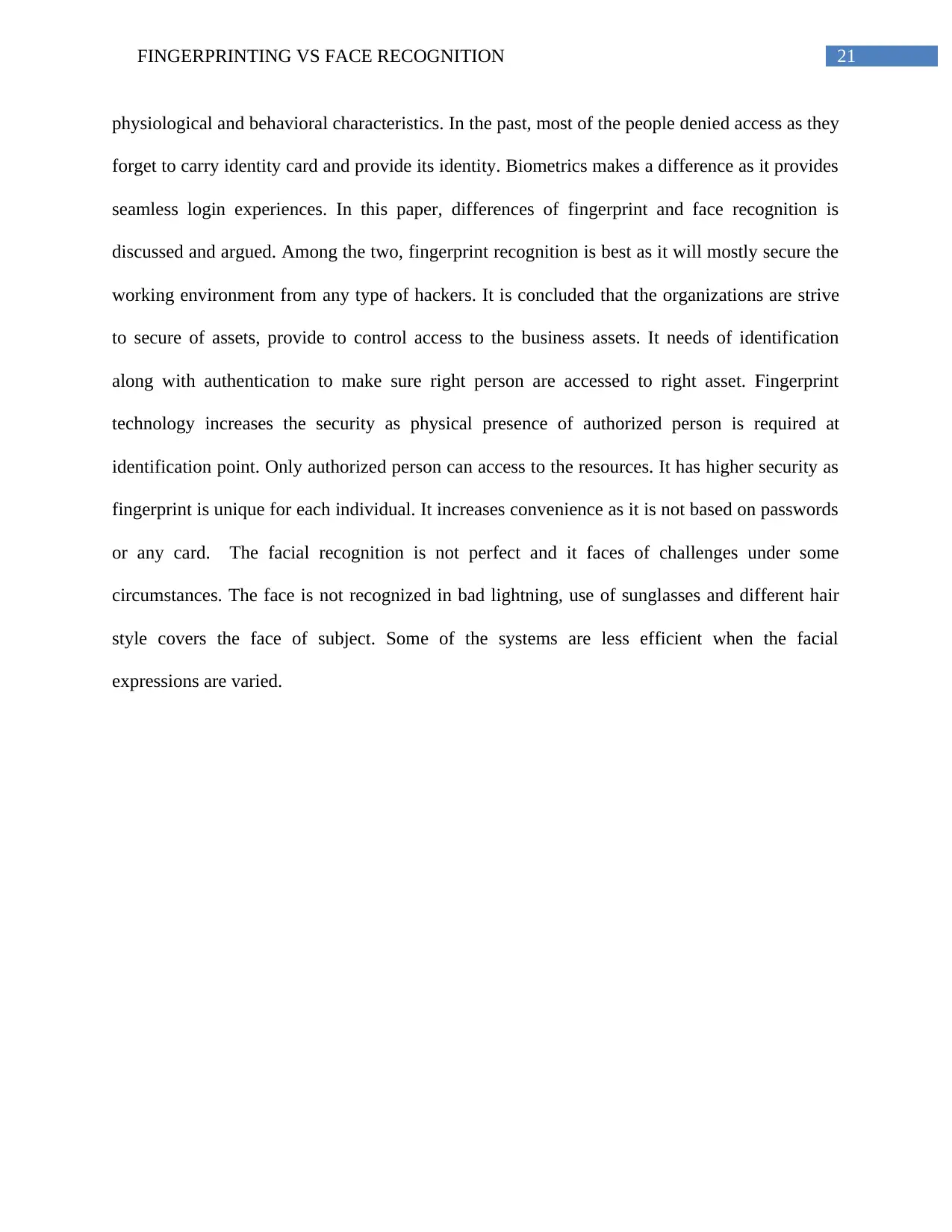
21FINGERPRINTING VS FACE RECOGNITION
physiological and behavioral characteristics. In the past, most of the people denied access as they
forget to carry identity card and provide its identity. Biometrics makes a difference as it provides
seamless login experiences. In this paper, differences of fingerprint and face recognition is
discussed and argued. Among the two, fingerprint recognition is best as it will mostly secure the
working environment from any type of hackers. It is concluded that the organizations are strive
to secure of assets, provide to control access to the business assets. It needs of identification
along with authentication to make sure right person are accessed to right asset. Fingerprint
technology increases the security as physical presence of authorized person is required at
identification point. Only authorized person can access to the resources. It has higher security as
fingerprint is unique for each individual. It increases convenience as it is not based on passwords
or any card. The facial recognition is not perfect and it faces of challenges under some
circumstances. The face is not recognized in bad lightning, use of sunglasses and different hair
style covers the face of subject. Some of the systems are less efficient when the facial
expressions are varied.
physiological and behavioral characteristics. In the past, most of the people denied access as they
forget to carry identity card and provide its identity. Biometrics makes a difference as it provides
seamless login experiences. In this paper, differences of fingerprint and face recognition is
discussed and argued. Among the two, fingerprint recognition is best as it will mostly secure the
working environment from any type of hackers. It is concluded that the organizations are strive
to secure of assets, provide to control access to the business assets. It needs of identification
along with authentication to make sure right person are accessed to right asset. Fingerprint
technology increases the security as physical presence of authorized person is required at
identification point. Only authorized person can access to the resources. It has higher security as
fingerprint is unique for each individual. It increases convenience as it is not based on passwords
or any card. The facial recognition is not perfect and it faces of challenges under some
circumstances. The face is not recognized in bad lightning, use of sunglasses and different hair
style covers the face of subject. Some of the systems are less efficient when the facial
expressions are varied.
Secure Best Marks with AI Grader
Need help grading? Try our AI Grader for instant feedback on your assignments.
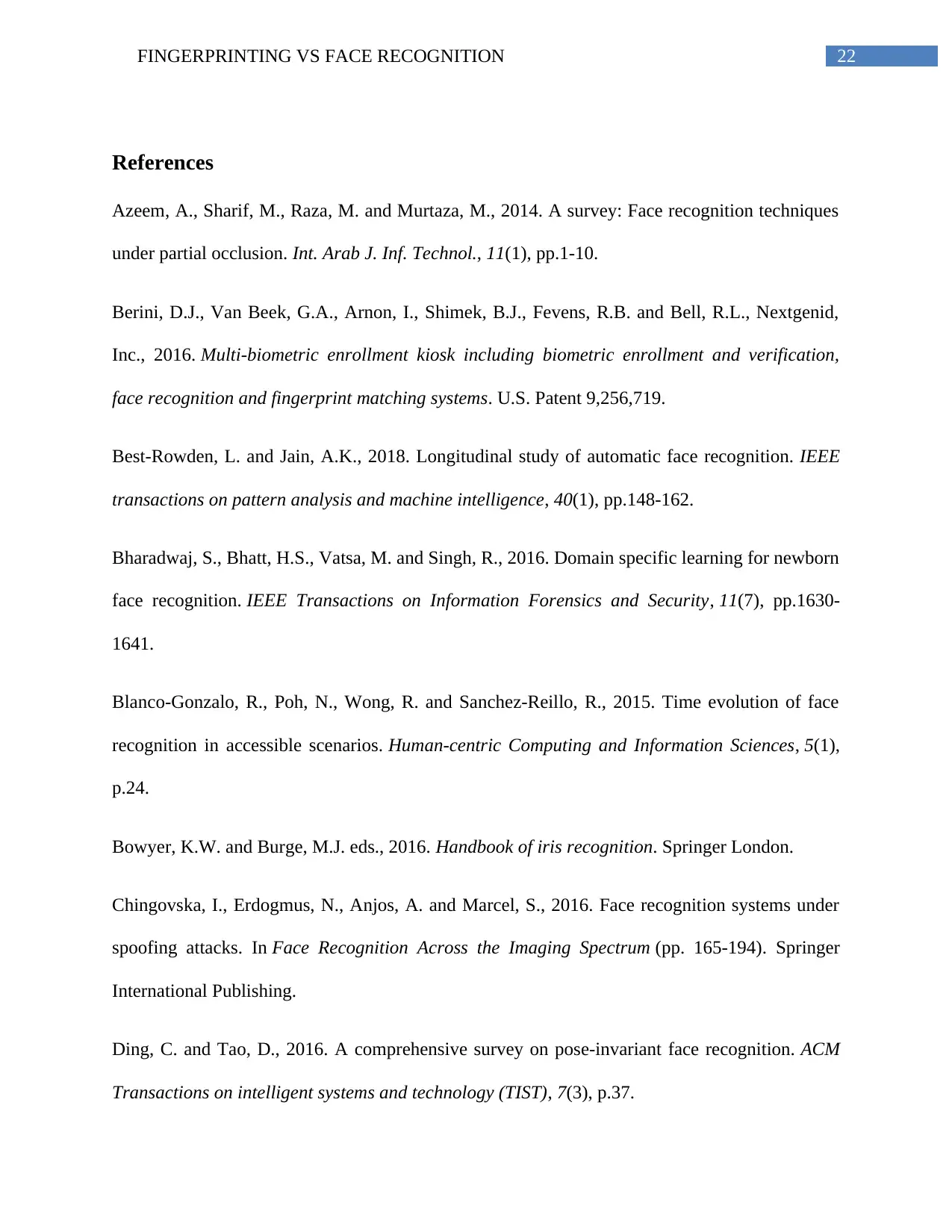
22FINGERPRINTING VS FACE RECOGNITION
References
Azeem, A., Sharif, M., Raza, M. and Murtaza, M., 2014. A survey: Face recognition techniques
under partial occlusion. Int. Arab J. Inf. Technol., 11(1), pp.1-10.
Berini, D.J., Van Beek, G.A., Arnon, I., Shimek, B.J., Fevens, R.B. and Bell, R.L., Nextgenid,
Inc., 2016. Multi-biometric enrollment kiosk including biometric enrollment and verification,
face recognition and fingerprint matching systems. U.S. Patent 9,256,719.
Best-Rowden, L. and Jain, A.K., 2018. Longitudinal study of automatic face recognition. IEEE
transactions on pattern analysis and machine intelligence, 40(1), pp.148-162.
Bharadwaj, S., Bhatt, H.S., Vatsa, M. and Singh, R., 2016. Domain specific learning for newborn
face recognition. IEEE Transactions on Information Forensics and Security, 11(7), pp.1630-
1641.
Blanco-Gonzalo, R., Poh, N., Wong, R. and Sanchez-Reillo, R., 2015. Time evolution of face
recognition in accessible scenarios. Human-centric Computing and Information Sciences, 5(1),
p.24.
Bowyer, K.W. and Burge, M.J. eds., 2016. Handbook of iris recognition. Springer London.
Chingovska, I., Erdogmus, N., Anjos, A. and Marcel, S., 2016. Face recognition systems under
spoofing attacks. In Face Recognition Across the Imaging Spectrum (pp. 165-194). Springer
International Publishing.
Ding, C. and Tao, D., 2016. A comprehensive survey on pose-invariant face recognition. ACM
Transactions on intelligent systems and technology (TIST), 7(3), p.37.
References
Azeem, A., Sharif, M., Raza, M. and Murtaza, M., 2014. A survey: Face recognition techniques
under partial occlusion. Int. Arab J. Inf. Technol., 11(1), pp.1-10.
Berini, D.J., Van Beek, G.A., Arnon, I., Shimek, B.J., Fevens, R.B. and Bell, R.L., Nextgenid,
Inc., 2016. Multi-biometric enrollment kiosk including biometric enrollment and verification,
face recognition and fingerprint matching systems. U.S. Patent 9,256,719.
Best-Rowden, L. and Jain, A.K., 2018. Longitudinal study of automatic face recognition. IEEE
transactions on pattern analysis and machine intelligence, 40(1), pp.148-162.
Bharadwaj, S., Bhatt, H.S., Vatsa, M. and Singh, R., 2016. Domain specific learning for newborn
face recognition. IEEE Transactions on Information Forensics and Security, 11(7), pp.1630-
1641.
Blanco-Gonzalo, R., Poh, N., Wong, R. and Sanchez-Reillo, R., 2015. Time evolution of face
recognition in accessible scenarios. Human-centric Computing and Information Sciences, 5(1),
p.24.
Bowyer, K.W. and Burge, M.J. eds., 2016. Handbook of iris recognition. Springer London.
Chingovska, I., Erdogmus, N., Anjos, A. and Marcel, S., 2016. Face recognition systems under
spoofing attacks. In Face Recognition Across the Imaging Spectrum (pp. 165-194). Springer
International Publishing.
Ding, C. and Tao, D., 2016. A comprehensive survey on pose-invariant face recognition. ACM
Transactions on intelligent systems and technology (TIST), 7(3), p.37.
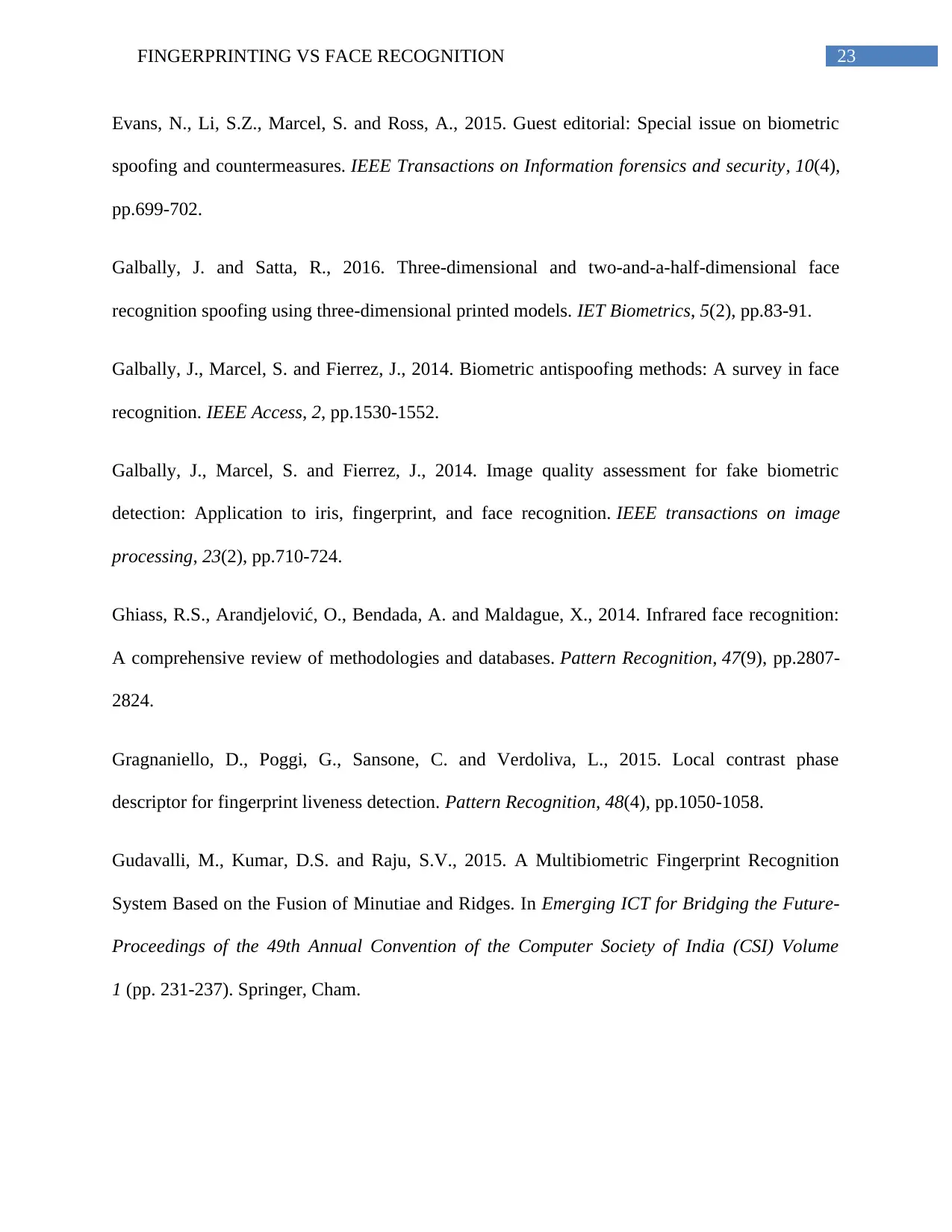
23FINGERPRINTING VS FACE RECOGNITION
Evans, N., Li, S.Z., Marcel, S. and Ross, A., 2015. Guest editorial: Special issue on biometric
spoofing and countermeasures. IEEE Transactions on Information forensics and security, 10(4),
pp.699-702.
Galbally, J. and Satta, R., 2016. Three-dimensional and two-and-a-half-dimensional face
recognition spoofing using three-dimensional printed models. IET Biometrics, 5(2), pp.83-91.
Galbally, J., Marcel, S. and Fierrez, J., 2014. Biometric antispoofing methods: A survey in face
recognition. IEEE Access, 2, pp.1530-1552.
Galbally, J., Marcel, S. and Fierrez, J., 2014. Image quality assessment for fake biometric
detection: Application to iris, fingerprint, and face recognition. IEEE transactions on image
processing, 23(2), pp.710-724.
Ghiass, R.S., Arandjelović, O., Bendada, A. and Maldague, X., 2014. Infrared face recognition:
A comprehensive review of methodologies and databases. Pattern Recognition, 47(9), pp.2807-
2824.
Gragnaniello, D., Poggi, G., Sansone, C. and Verdoliva, L., 2015. Local contrast phase
descriptor for fingerprint liveness detection. Pattern Recognition, 48(4), pp.1050-1058.
Gudavalli, M., Kumar, D.S. and Raju, S.V., 2015. A Multibiometric Fingerprint Recognition
System Based on the Fusion of Minutiae and Ridges. In Emerging ICT for Bridging the Future-
Proceedings of the 49th Annual Convention of the Computer Society of India (CSI) Volume
1 (pp. 231-237). Springer, Cham.
Evans, N., Li, S.Z., Marcel, S. and Ross, A., 2015. Guest editorial: Special issue on biometric
spoofing and countermeasures. IEEE Transactions on Information forensics and security, 10(4),
pp.699-702.
Galbally, J. and Satta, R., 2016. Three-dimensional and two-and-a-half-dimensional face
recognition spoofing using three-dimensional printed models. IET Biometrics, 5(2), pp.83-91.
Galbally, J., Marcel, S. and Fierrez, J., 2014. Biometric antispoofing methods: A survey in face
recognition. IEEE Access, 2, pp.1530-1552.
Galbally, J., Marcel, S. and Fierrez, J., 2014. Image quality assessment for fake biometric
detection: Application to iris, fingerprint, and face recognition. IEEE transactions on image
processing, 23(2), pp.710-724.
Ghiass, R.S., Arandjelović, O., Bendada, A. and Maldague, X., 2014. Infrared face recognition:
A comprehensive review of methodologies and databases. Pattern Recognition, 47(9), pp.2807-
2824.
Gragnaniello, D., Poggi, G., Sansone, C. and Verdoliva, L., 2015. Local contrast phase
descriptor for fingerprint liveness detection. Pattern Recognition, 48(4), pp.1050-1058.
Gudavalli, M., Kumar, D.S. and Raju, S.V., 2015. A Multibiometric Fingerprint Recognition
System Based on the Fusion of Minutiae and Ridges. In Emerging ICT for Bridging the Future-
Proceedings of the 49th Annual Convention of the Computer Society of India (CSI) Volume
1 (pp. 231-237). Springer, Cham.
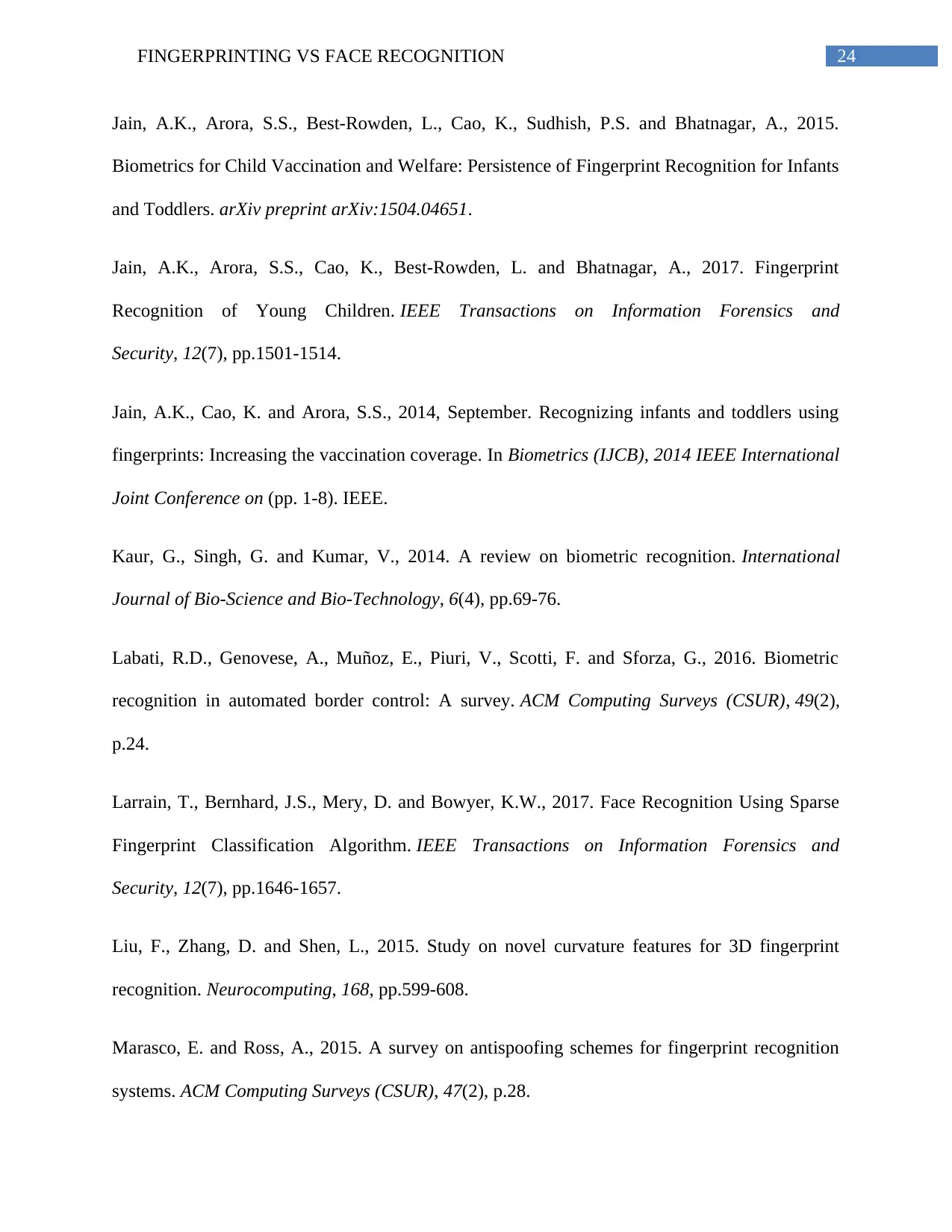
24FINGERPRINTING VS FACE RECOGNITION
Jain, A.K., Arora, S.S., Best-Rowden, L., Cao, K., Sudhish, P.S. and Bhatnagar, A., 2015.
Biometrics for Child Vaccination and Welfare: Persistence of Fingerprint Recognition for Infants
and Toddlers. arXiv preprint arXiv:1504.04651.
Jain, A.K., Arora, S.S., Cao, K., Best-Rowden, L. and Bhatnagar, A., 2017. Fingerprint
Recognition of Young Children. IEEE Transactions on Information Forensics and
Security, 12(7), pp.1501-1514.
Jain, A.K., Cao, K. and Arora, S.S., 2014, September. Recognizing infants and toddlers using
fingerprints: Increasing the vaccination coverage. In Biometrics (IJCB), 2014 IEEE International
Joint Conference on (pp. 1-8). IEEE.
Kaur, G., Singh, G. and Kumar, V., 2014. A review on biometric recognition. International
Journal of Bio-Science and Bio-Technology, 6(4), pp.69-76.
Labati, R.D., Genovese, A., Muñoz, E., Piuri, V., Scotti, F. and Sforza, G., 2016. Biometric
recognition in automated border control: A survey. ACM Computing Surveys (CSUR), 49(2),
p.24.
Larrain, T., Bernhard, J.S., Mery, D. and Bowyer, K.W., 2017. Face Recognition Using Sparse
Fingerprint Classification Algorithm. IEEE Transactions on Information Forensics and
Security, 12(7), pp.1646-1657.
Liu, F., Zhang, D. and Shen, L., 2015. Study on novel curvature features for 3D fingerprint
recognition. Neurocomputing, 168, pp.599-608.
Marasco, E. and Ross, A., 2015. A survey on antispoofing schemes for fingerprint recognition
systems. ACM Computing Surveys (CSUR), 47(2), p.28.
Jain, A.K., Arora, S.S., Best-Rowden, L., Cao, K., Sudhish, P.S. and Bhatnagar, A., 2015.
Biometrics for Child Vaccination and Welfare: Persistence of Fingerprint Recognition for Infants
and Toddlers. arXiv preprint arXiv:1504.04651.
Jain, A.K., Arora, S.S., Cao, K., Best-Rowden, L. and Bhatnagar, A., 2017. Fingerprint
Recognition of Young Children. IEEE Transactions on Information Forensics and
Security, 12(7), pp.1501-1514.
Jain, A.K., Cao, K. and Arora, S.S., 2014, September. Recognizing infants and toddlers using
fingerprints: Increasing the vaccination coverage. In Biometrics (IJCB), 2014 IEEE International
Joint Conference on (pp. 1-8). IEEE.
Kaur, G., Singh, G. and Kumar, V., 2014. A review on biometric recognition. International
Journal of Bio-Science and Bio-Technology, 6(4), pp.69-76.
Labati, R.D., Genovese, A., Muñoz, E., Piuri, V., Scotti, F. and Sforza, G., 2016. Biometric
recognition in automated border control: A survey. ACM Computing Surveys (CSUR), 49(2),
p.24.
Larrain, T., Bernhard, J.S., Mery, D. and Bowyer, K.W., 2017. Face Recognition Using Sparse
Fingerprint Classification Algorithm. IEEE Transactions on Information Forensics and
Security, 12(7), pp.1646-1657.
Liu, F., Zhang, D. and Shen, L., 2015. Study on novel curvature features for 3D fingerprint
recognition. Neurocomputing, 168, pp.599-608.
Marasco, E. and Ross, A., 2015. A survey on antispoofing schemes for fingerprint recognition
systems. ACM Computing Surveys (CSUR), 47(2), p.28.
Paraphrase This Document
Need a fresh take? Get an instant paraphrase of this document with our AI Paraphraser
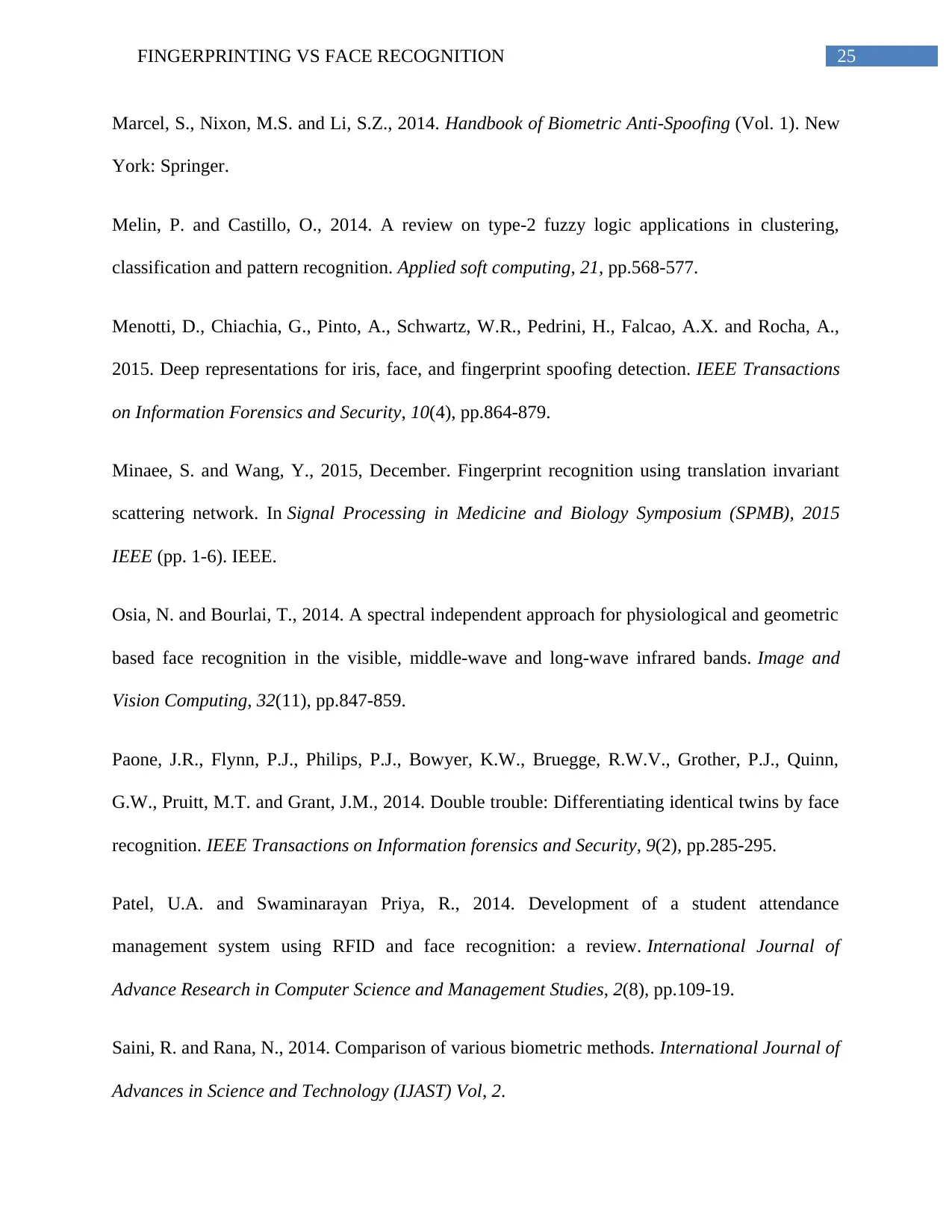
25FINGERPRINTING VS FACE RECOGNITION
Marcel, S., Nixon, M.S. and Li, S.Z., 2014. Handbook of Biometric Anti-Spoofing (Vol. 1). New
York: Springer.
Melin, P. and Castillo, O., 2014. A review on type-2 fuzzy logic applications in clustering,
classification and pattern recognition. Applied soft computing, 21, pp.568-577.
Menotti, D., Chiachia, G., Pinto, A., Schwartz, W.R., Pedrini, H., Falcao, A.X. and Rocha, A.,
2015. Deep representations for iris, face, and fingerprint spoofing detection. IEEE Transactions
on Information Forensics and Security, 10(4), pp.864-879.
Minaee, S. and Wang, Y., 2015, December. Fingerprint recognition using translation invariant
scattering network. In Signal Processing in Medicine and Biology Symposium (SPMB), 2015
IEEE (pp. 1-6). IEEE.
Osia, N. and Bourlai, T., 2014. A spectral independent approach for physiological and geometric
based face recognition in the visible, middle-wave and long-wave infrared bands. Image and
Vision Computing, 32(11), pp.847-859.
Paone, J.R., Flynn, P.J., Philips, P.J., Bowyer, K.W., Bruegge, R.W.V., Grother, P.J., Quinn,
G.W., Pruitt, M.T. and Grant, J.M., 2014. Double trouble: Differentiating identical twins by face
recognition. IEEE Transactions on Information forensics and Security, 9(2), pp.285-295.
Patel, U.A. and Swaminarayan Priya, R., 2014. Development of a student attendance
management system using RFID and face recognition: a review. International Journal of
Advance Research in Computer Science and Management Studies, 2(8), pp.109-19.
Saini, R. and Rana, N., 2014. Comparison of various biometric methods. International Journal of
Advances in Science and Technology (IJAST) Vol, 2.
Marcel, S., Nixon, M.S. and Li, S.Z., 2014. Handbook of Biometric Anti-Spoofing (Vol. 1). New
York: Springer.
Melin, P. and Castillo, O., 2014. A review on type-2 fuzzy logic applications in clustering,
classification and pattern recognition. Applied soft computing, 21, pp.568-577.
Menotti, D., Chiachia, G., Pinto, A., Schwartz, W.R., Pedrini, H., Falcao, A.X. and Rocha, A.,
2015. Deep representations for iris, face, and fingerprint spoofing detection. IEEE Transactions
on Information Forensics and Security, 10(4), pp.864-879.
Minaee, S. and Wang, Y., 2015, December. Fingerprint recognition using translation invariant
scattering network. In Signal Processing in Medicine and Biology Symposium (SPMB), 2015
IEEE (pp. 1-6). IEEE.
Osia, N. and Bourlai, T., 2014. A spectral independent approach for physiological and geometric
based face recognition in the visible, middle-wave and long-wave infrared bands. Image and
Vision Computing, 32(11), pp.847-859.
Paone, J.R., Flynn, P.J., Philips, P.J., Bowyer, K.W., Bruegge, R.W.V., Grother, P.J., Quinn,
G.W., Pruitt, M.T. and Grant, J.M., 2014. Double trouble: Differentiating identical twins by face
recognition. IEEE Transactions on Information forensics and Security, 9(2), pp.285-295.
Patel, U.A. and Swaminarayan Priya, R., 2014. Development of a student attendance
management system using RFID and face recognition: a review. International Journal of
Advance Research in Computer Science and Management Studies, 2(8), pp.109-19.
Saini, R. and Rana, N., 2014. Comparison of various biometric methods. International Journal of
Advances in Science and Technology (IJAST) Vol, 2.
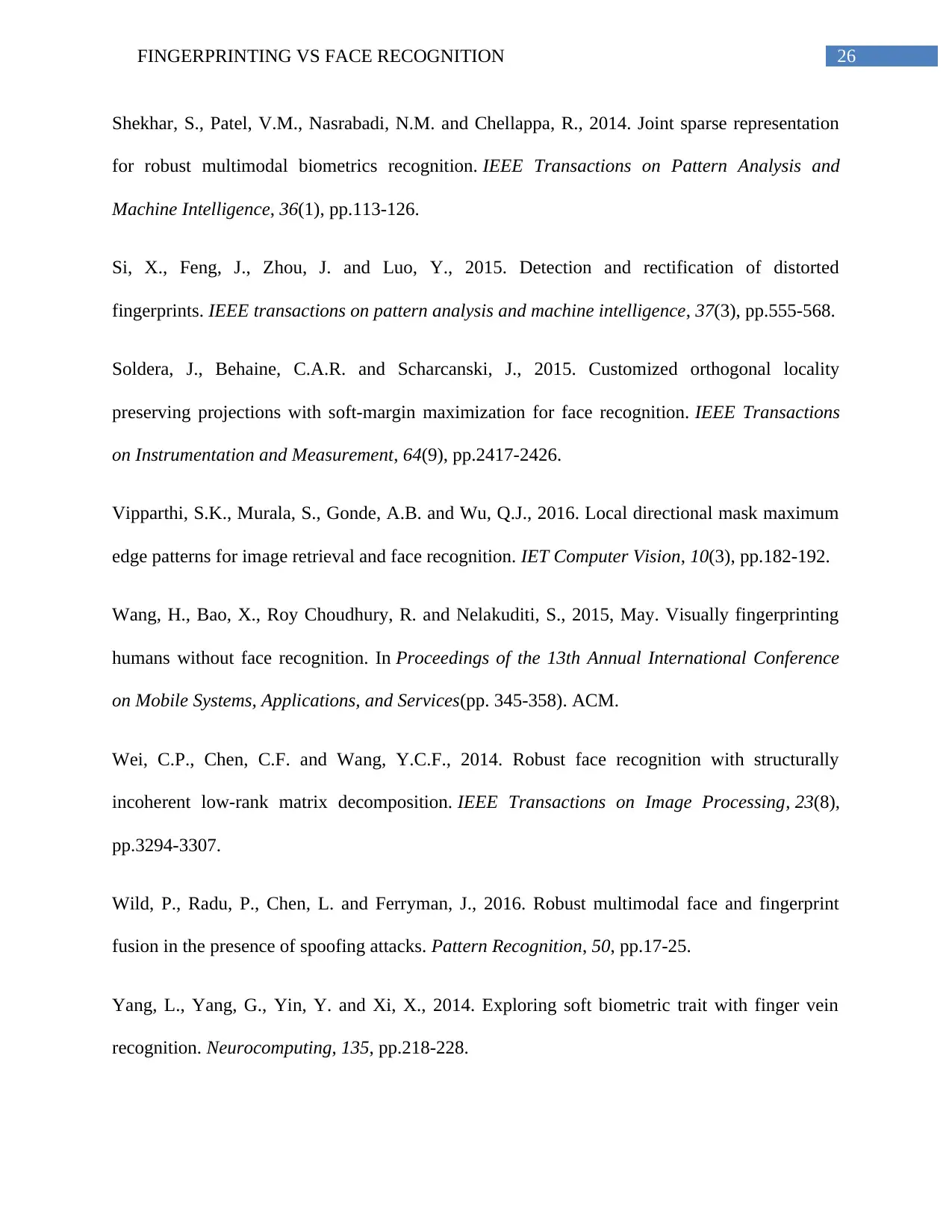
26FINGERPRINTING VS FACE RECOGNITION
Shekhar, S., Patel, V.M., Nasrabadi, N.M. and Chellappa, R., 2014. Joint sparse representation
for robust multimodal biometrics recognition. IEEE Transactions on Pattern Analysis and
Machine Intelligence, 36(1), pp.113-126.
Si, X., Feng, J., Zhou, J. and Luo, Y., 2015. Detection and rectification of distorted
fingerprints. IEEE transactions on pattern analysis and machine intelligence, 37(3), pp.555-568.
Soldera, J., Behaine, C.A.R. and Scharcanski, J., 2015. Customized orthogonal locality
preserving projections with soft-margin maximization for face recognition. IEEE Transactions
on Instrumentation and Measurement, 64(9), pp.2417-2426.
Vipparthi, S.K., Murala, S., Gonde, A.B. and Wu, Q.J., 2016. Local directional mask maximum
edge patterns for image retrieval and face recognition. IET Computer Vision, 10(3), pp.182-192.
Wang, H., Bao, X., Roy Choudhury, R. and Nelakuditi, S., 2015, May. Visually fingerprinting
humans without face recognition. In Proceedings of the 13th Annual International Conference
on Mobile Systems, Applications, and Services(pp. 345-358). ACM.
Wei, C.P., Chen, C.F. and Wang, Y.C.F., 2014. Robust face recognition with structurally
incoherent low-rank matrix decomposition. IEEE Transactions on Image Processing, 23(8),
pp.3294-3307.
Wild, P., Radu, P., Chen, L. and Ferryman, J., 2016. Robust multimodal face and fingerprint
fusion in the presence of spoofing attacks. Pattern Recognition, 50, pp.17-25.
Yang, L., Yang, G., Yin, Y. and Xi, X., 2014. Exploring soft biometric trait with finger vein
recognition. Neurocomputing, 135, pp.218-228.
Shekhar, S., Patel, V.M., Nasrabadi, N.M. and Chellappa, R., 2014. Joint sparse representation
for robust multimodal biometrics recognition. IEEE Transactions on Pattern Analysis and
Machine Intelligence, 36(1), pp.113-126.
Si, X., Feng, J., Zhou, J. and Luo, Y., 2015. Detection and rectification of distorted
fingerprints. IEEE transactions on pattern analysis and machine intelligence, 37(3), pp.555-568.
Soldera, J., Behaine, C.A.R. and Scharcanski, J., 2015. Customized orthogonal locality
preserving projections with soft-margin maximization for face recognition. IEEE Transactions
on Instrumentation and Measurement, 64(9), pp.2417-2426.
Vipparthi, S.K., Murala, S., Gonde, A.B. and Wu, Q.J., 2016. Local directional mask maximum
edge patterns for image retrieval and face recognition. IET Computer Vision, 10(3), pp.182-192.
Wang, H., Bao, X., Roy Choudhury, R. and Nelakuditi, S., 2015, May. Visually fingerprinting
humans without face recognition. In Proceedings of the 13th Annual International Conference
on Mobile Systems, Applications, and Services(pp. 345-358). ACM.
Wei, C.P., Chen, C.F. and Wang, Y.C.F., 2014. Robust face recognition with structurally
incoherent low-rank matrix decomposition. IEEE Transactions on Image Processing, 23(8),
pp.3294-3307.
Wild, P., Radu, P., Chen, L. and Ferryman, J., 2016. Robust multimodal face and fingerprint
fusion in the presence of spoofing attacks. Pattern Recognition, 50, pp.17-25.
Yang, L., Yang, G., Yin, Y. and Xi, X., 2014. Exploring soft biometric trait with finger vein
recognition. Neurocomputing, 135, pp.218-228.
1 out of 27
Related Documents
![[object Object]](/_next/image/?url=%2F_next%2Fstatic%2Fmedia%2Flogo.6d15ce61.png&w=640&q=75)
Your All-in-One AI-Powered Toolkit for Academic Success.
+13062052269
info@desklib.com
Available 24*7 on WhatsApp / Email
Unlock your academic potential
© 2024 | Zucol Services PVT LTD | All rights reserved.