HI6007: Statistical Analysis of Frequency, Regression, and ANOVA
VerifiedAdded on 2023/06/12
|13
|2207
|492
Homework Assignment
AI Summary
This assignment solution covers several statistical concepts. It begins with constructing a frequency distribution, relative frequency distribution, and percent frequency distribution for furniture order data, followed by creating a percent frequency histogram and discussing the data's skewness. The solution then delves into simple linear regression, testing the relationship between demand and unit price using ANOVA, calculating the coefficient of determination, and determining the correlation coefficient. Furthermore, the assignment addresses hypothesis testing in the context of treatment means using ANOVA, including completing the ANOVA table and comparing calculated and tabulated F-values. Finally, it tackles multiple linear regression, establishing a regression equation for mobile phone sales based on price and advertising spots, testing the significance of the relationship, interpreting slopes, and predicting sales based on given values for the predictor variables. The document concludes with a list of references. Desklib provides a platform to access this and many other solved assignments.
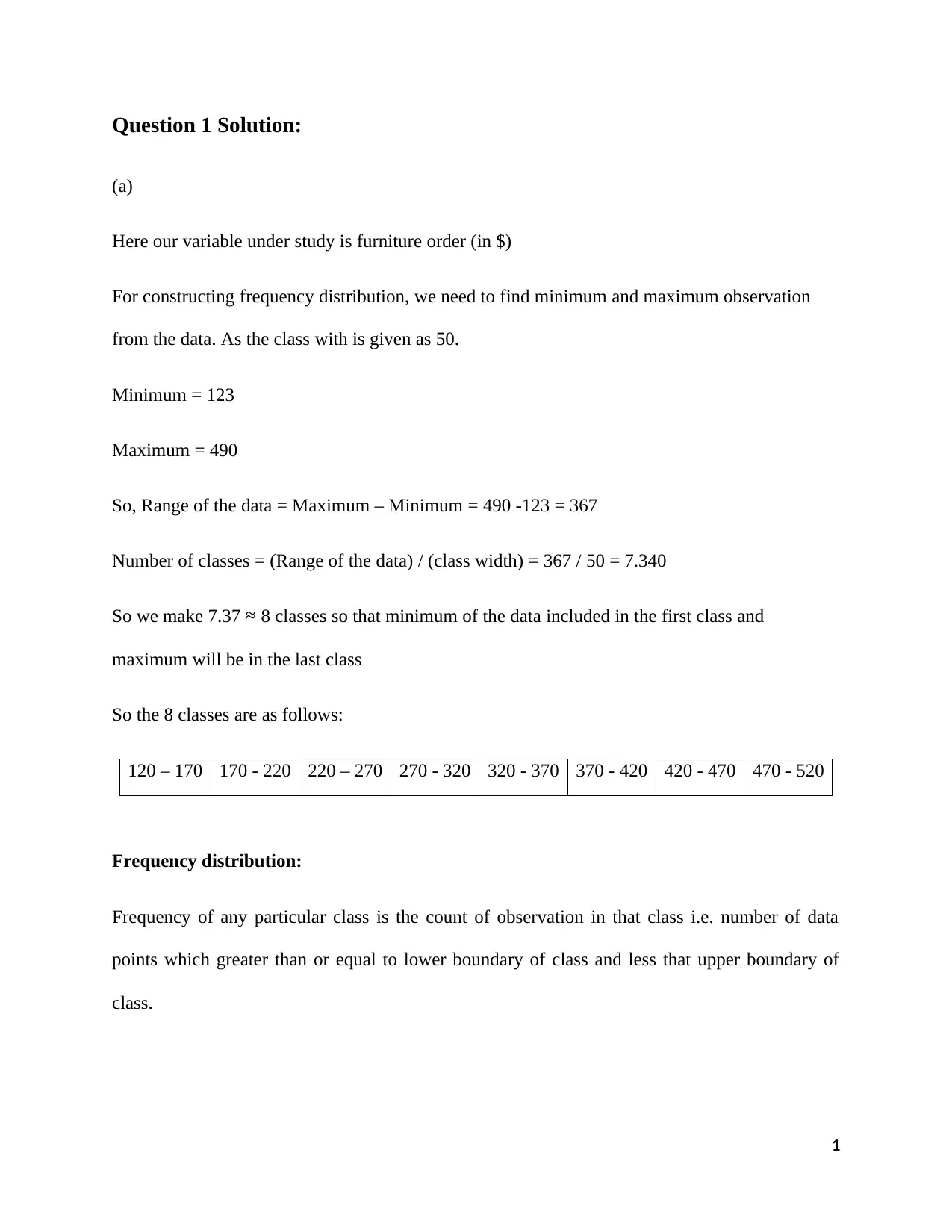
Question 1 Solution:
(a)
Here our variable under study is furniture order (in $)
For constructing frequency distribution, we need to find minimum and maximum observation
from the data. As the class with is given as 50.
Minimum = 123
Maximum = 490
So, Range of the data = Maximum – Minimum = 490 -123 = 367
Number of classes = (Range of the data) / (class width) = 367 / 50 = 7.340
So we make 7.37 ≈ 8 classes so that minimum of the data included in the first class and
maximum will be in the last class
So the 8 classes are as follows:
120 – 170 170 - 220 220 – 270 270 - 320 320 - 370 370 - 420 420 - 470 470 - 520
Frequency distribution:
Frequency of any particular class is the count of observation in that class i.e. number of data
points which greater than or equal to lower boundary of class and less that upper boundary of
class.
1
(a)
Here our variable under study is furniture order (in $)
For constructing frequency distribution, we need to find minimum and maximum observation
from the data. As the class with is given as 50.
Minimum = 123
Maximum = 490
So, Range of the data = Maximum – Minimum = 490 -123 = 367
Number of classes = (Range of the data) / (class width) = 367 / 50 = 7.340
So we make 7.37 ≈ 8 classes so that minimum of the data included in the first class and
maximum will be in the last class
So the 8 classes are as follows:
120 – 170 170 - 220 220 – 270 270 - 320 320 - 370 370 - 420 420 - 470 470 - 520
Frequency distribution:
Frequency of any particular class is the count of observation in that class i.e. number of data
points which greater than or equal to lower boundary of class and less that upper boundary of
class.
1
Paraphrase This Document
Need a fresh take? Get an instant paraphrase of this document with our AI Paraphraser
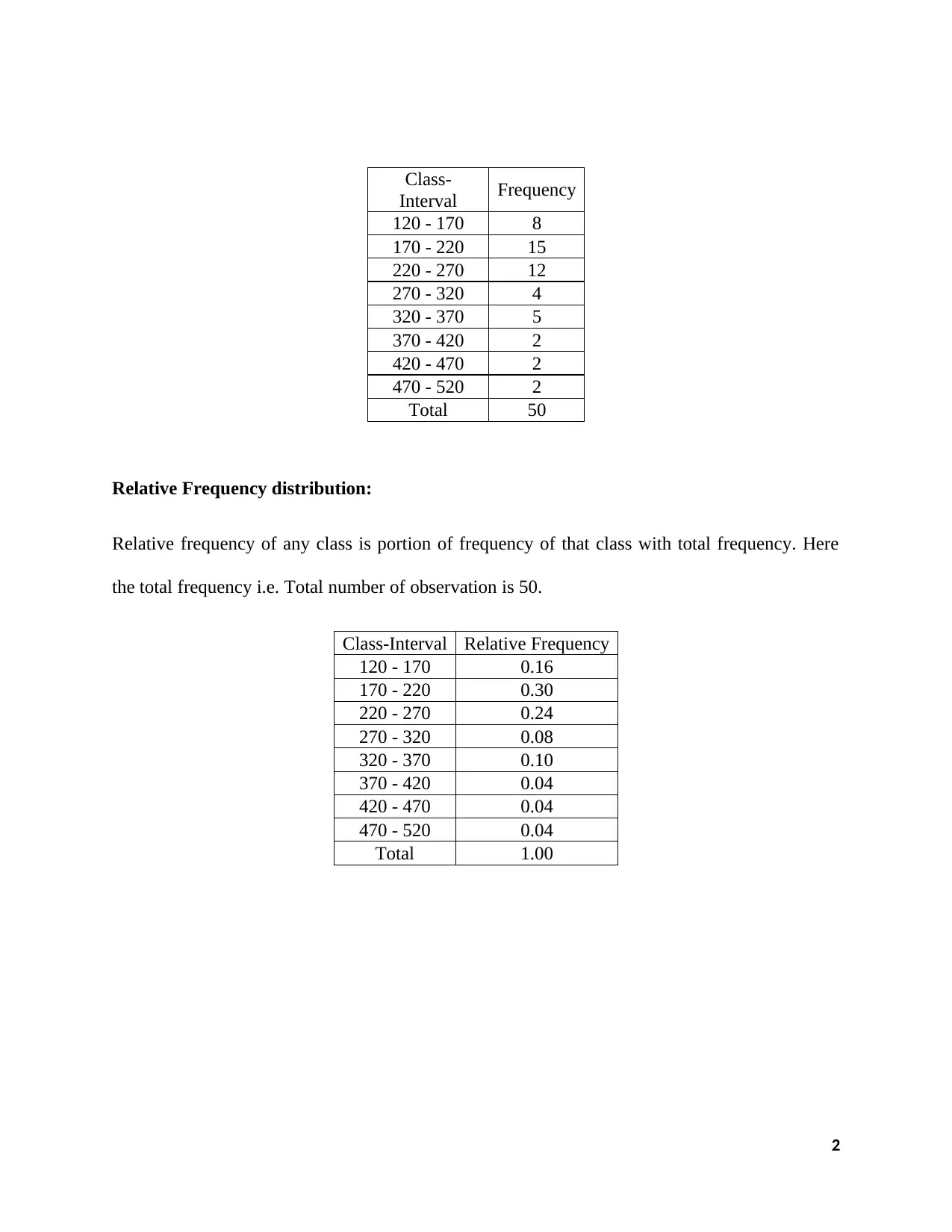
Class-
Interval Frequency
120 - 170 8
170 - 220 15
220 - 270 12
270 - 320 4
320 - 370 5
370 - 420 2
420 - 470 2
470 - 520 2
Total 50
Relative Frequency distribution:
Relative frequency of any class is portion of frequency of that class with total frequency. Here
the total frequency i.e. Total number of observation is 50.
Class-Interval Relative Frequency
120 - 170 0.16
170 - 220 0.30
220 - 270 0.24
270 - 320 0.08
320 - 370 0.10
370 - 420 0.04
420 - 470 0.04
470 - 520 0.04
Total 1.00
2
Interval Frequency
120 - 170 8
170 - 220 15
220 - 270 12
270 - 320 4
320 - 370 5
370 - 420 2
420 - 470 2
470 - 520 2
Total 50
Relative Frequency distribution:
Relative frequency of any class is portion of frequency of that class with total frequency. Here
the total frequency i.e. Total number of observation is 50.
Class-Interval Relative Frequency
120 - 170 0.16
170 - 220 0.30
220 - 270 0.24
270 - 320 0.08
320 - 370 0.10
370 - 420 0.04
420 - 470 0.04
470 - 520 0.04
Total 1.00
2
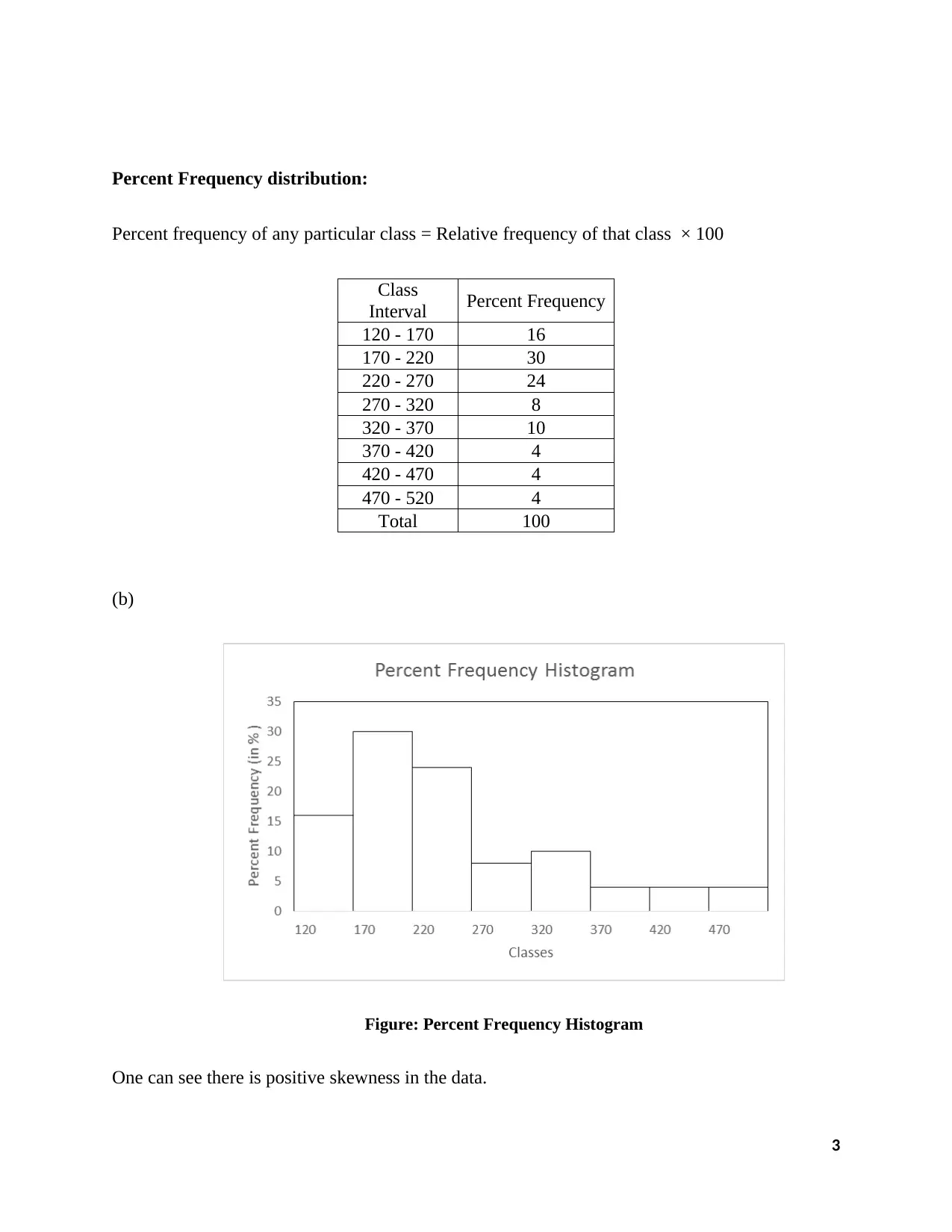
Percent Frequency distribution:
Percent frequency of any particular class = Relative frequency of that class × 100
Class
Interval Percent Frequency
120 - 170 16
170 - 220 30
220 - 270 24
270 - 320 8
320 - 370 10
370 - 420 4
420 - 470 4
470 - 520 4
Total 100
(b)
Figure: Percent Frequency Histogram
One can see there is positive skewness in the data.
3
Percent frequency of any particular class = Relative frequency of that class × 100
Class
Interval Percent Frequency
120 - 170 16
170 - 220 30
220 - 270 24
270 - 320 8
320 - 370 10
370 - 420 4
420 - 470 4
470 - 520 4
Total 100
(b)
Figure: Percent Frequency Histogram
One can see there is positive skewness in the data.
3
You're viewing a preview
Unlock full access by subscribing today!
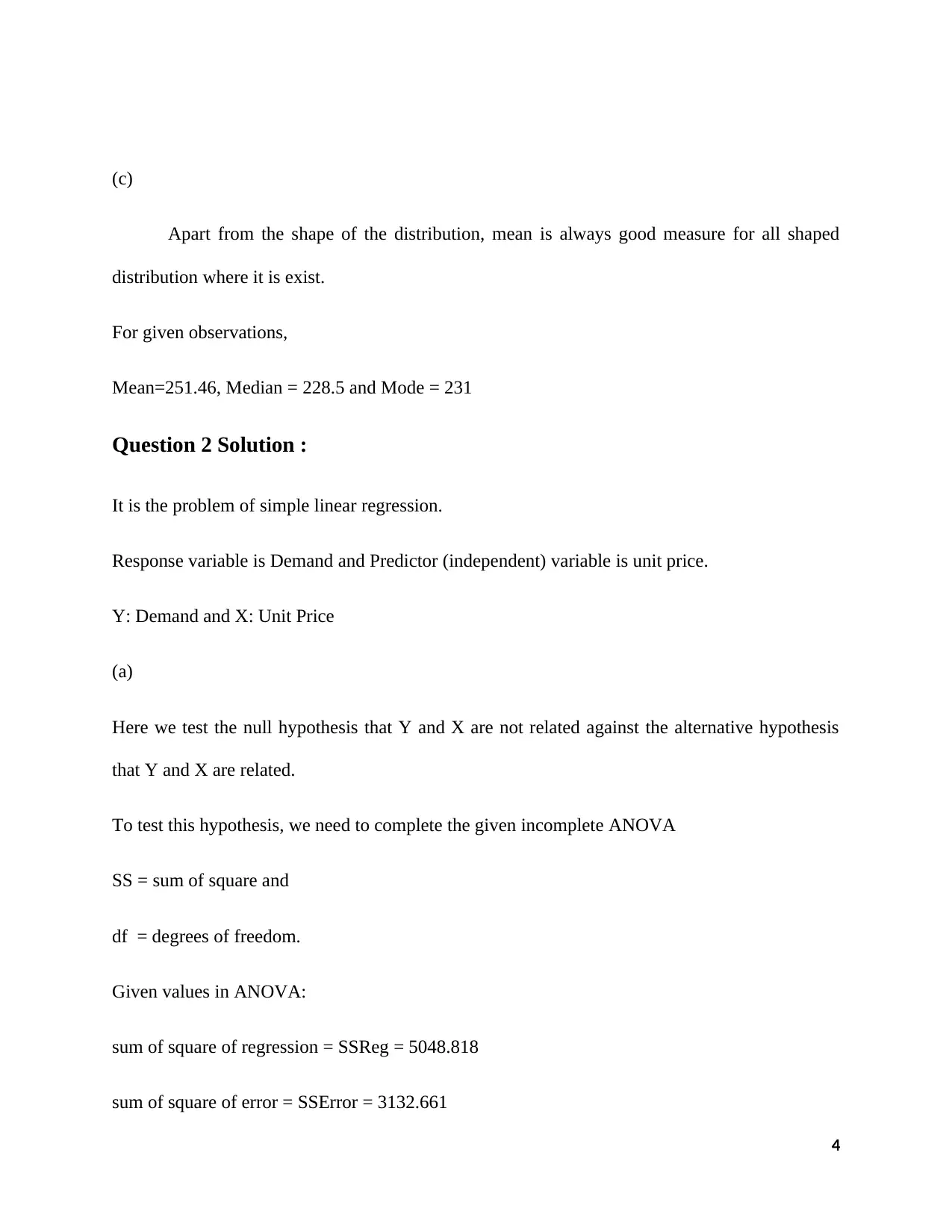
(c)
Apart from the shape of the distribution, mean is always good measure for all shaped
distribution where it is exist.
For given observations,
Mean=251.46, Median = 228.5 and Mode = 231
Question 2 Solution :
It is the problem of simple linear regression.
Response variable is Demand and Predictor (independent) variable is unit price.
Y: Demand and X: Unit Price
(a)
Here we test the null hypothesis that Y and X are not related against the alternative hypothesis
that Y and X are related.
To test this hypothesis, we need to complete the given incomplete ANOVA
SS = sum of square and
df = degrees of freedom.
Given values in ANOVA:
sum of square of regression = SSReg = 5048.818
sum of square of error = SSError = 3132.661
4
Apart from the shape of the distribution, mean is always good measure for all shaped
distribution where it is exist.
For given observations,
Mean=251.46, Median = 228.5 and Mode = 231
Question 2 Solution :
It is the problem of simple linear regression.
Response variable is Demand and Predictor (independent) variable is unit price.
Y: Demand and X: Unit Price
(a)
Here we test the null hypothesis that Y and X are not related against the alternative hypothesis
that Y and X are related.
To test this hypothesis, we need to complete the given incomplete ANOVA
SS = sum of square and
df = degrees of freedom.
Given values in ANOVA:
sum of square of regression = SSReg = 5048.818
sum of square of error = SSError = 3132.661
4
Paraphrase This Document
Need a fresh take? Get an instant paraphrase of this document with our AI Paraphraser
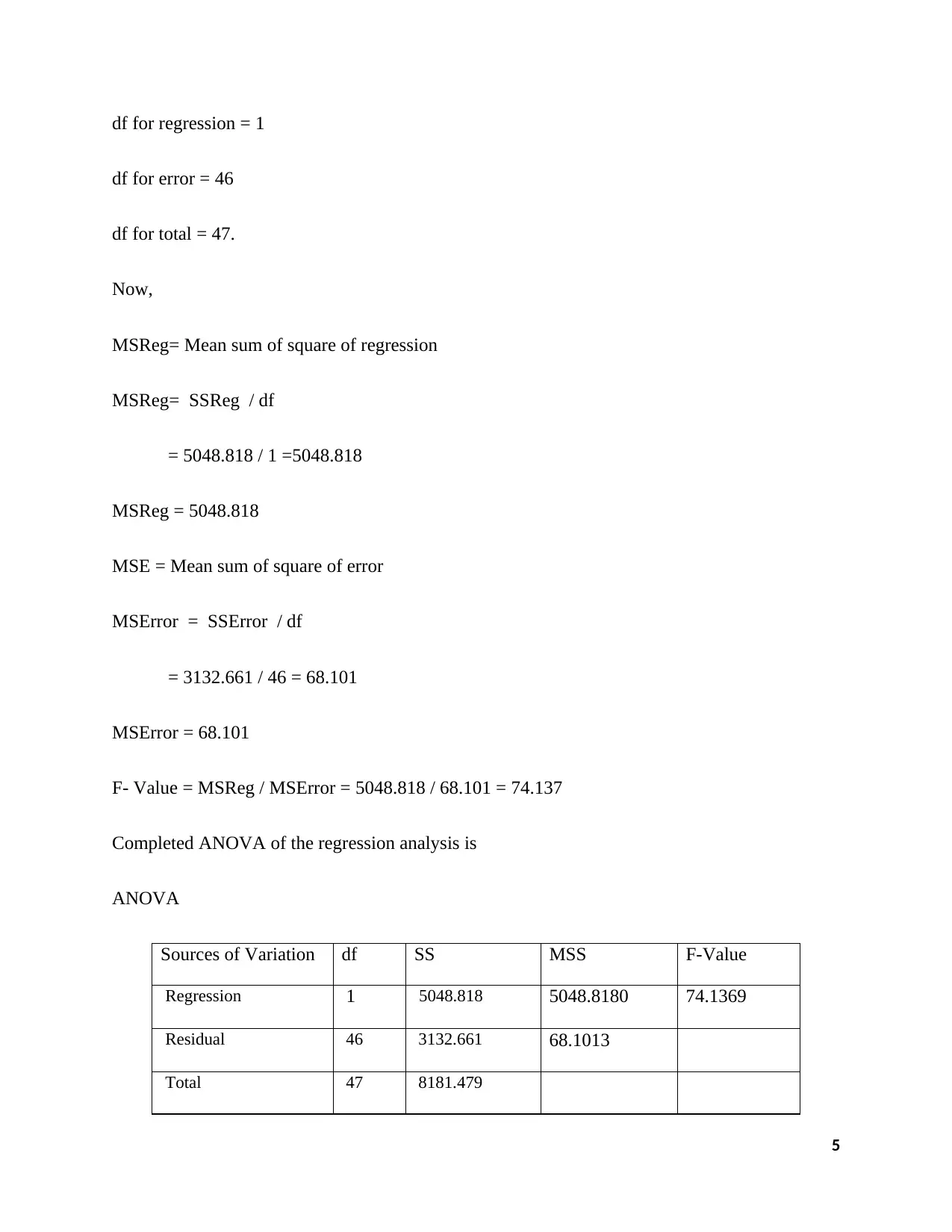
df for regression = 1
df for error = 46
df for total = 47.
Now,
MSReg= Mean sum of square of regression
MSReg= SSReg / df
= 5048.818 / 1 =5048.818
MSReg = 5048.818
MSE = Mean sum of square of error
MSError = SSError / df
= 3132.661 / 46 = 68.101
MSError = 68.101
F- Value = MSReg / MSError = 5048.818 / 68.101 = 74.137
Completed ANOVA of the regression analysis is
ANOVA
Sources of Variation df SS MSS F-Value
Regression 1 5048.818 5048.8180 74.1369
Residual 46 3132.661 68.1013
Total 47 8181.479
5
df for error = 46
df for total = 47.
Now,
MSReg= Mean sum of square of regression
MSReg= SSReg / df
= 5048.818 / 1 =5048.818
MSReg = 5048.818
MSE = Mean sum of square of error
MSError = SSError / df
= 3132.661 / 46 = 68.101
MSError = 68.101
F- Value = MSReg / MSError = 5048.818 / 68.101 = 74.137
Completed ANOVA of the regression analysis is
ANOVA
Sources of Variation df SS MSS F-Value
Regression 1 5048.818 5048.8180 74.1369
Residual 46 3132.661 68.1013
Total 47 8181.479
5
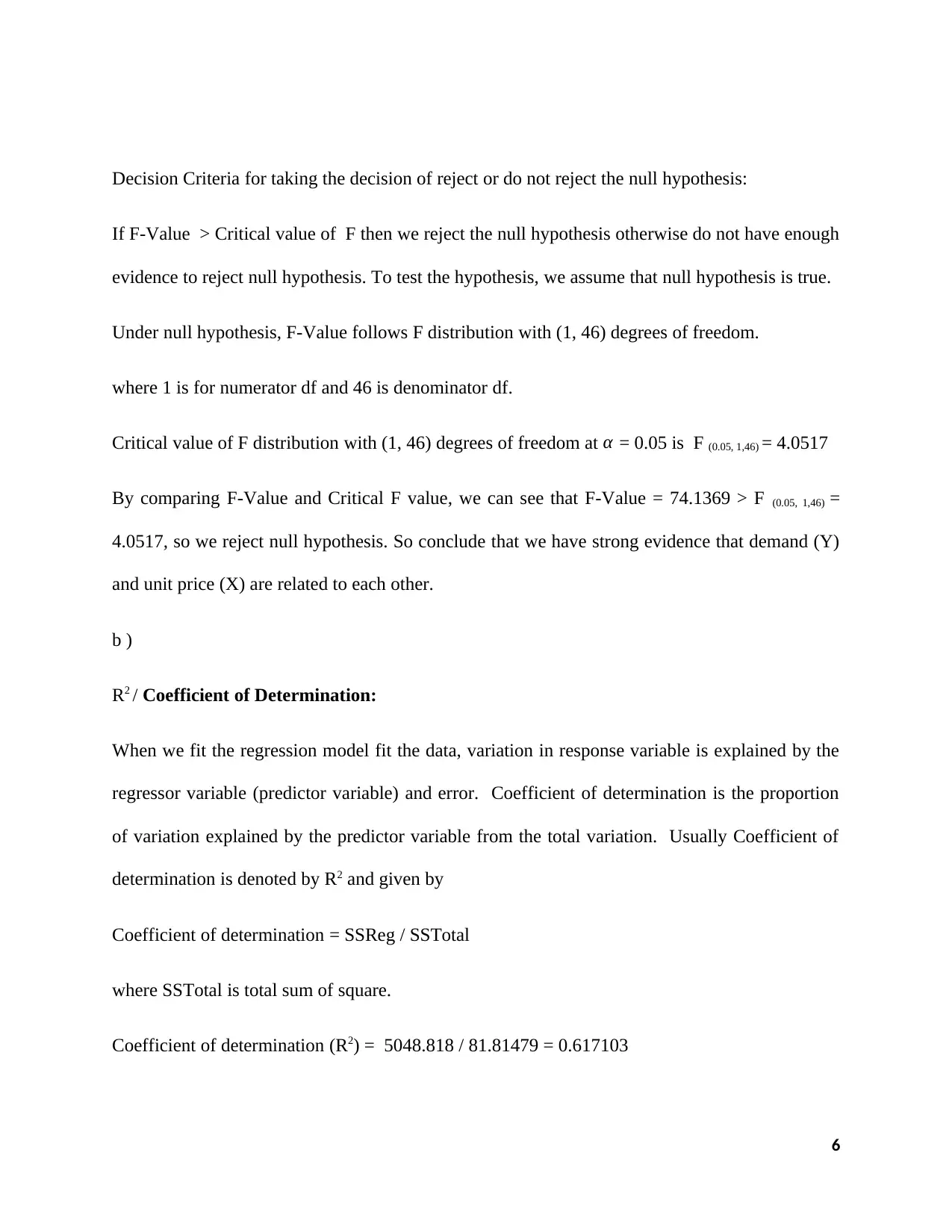
Decision Criteria for taking the decision of reject or do not reject the null hypothesis:
If F-Value > Critical value of F then we reject the null hypothesis otherwise do not have enough
evidence to reject null hypothesis. To test the hypothesis, we assume that null hypothesis is true.
Under null hypothesis, F-Value follows F distribution with (1, 46) degrees of freedom.
where 1 is for numerator df and 46 is denominator df.
Critical value of F distribution with (1, 46) degrees of freedom at α = 0.05 is F (0.05, 1,46) = 4.0517
By comparing F-Value and Critical F value, we can see that F-Value = 74.1369 > F (0.05, 1,46) =
4.0517, so we reject null hypothesis. So conclude that we have strong evidence that demand (Y)
and unit price (X) are related to each other.
b )
R2 / Coefficient of Determination:
When we fit the regression model fit the data, variation in response variable is explained by the
regressor variable (predictor variable) and error. Coefficient of determination is the proportion
of variation explained by the predictor variable from the total variation. Usually Coefficient of
determination is denoted by R2 and given by
Coefficient of determination = SSReg / SSTotal
where SSTotal is total sum of square.
Coefficient of determination (R2) = 5048.818 / 81.81479 = 0.617103
6
If F-Value > Critical value of F then we reject the null hypothesis otherwise do not have enough
evidence to reject null hypothesis. To test the hypothesis, we assume that null hypothesis is true.
Under null hypothesis, F-Value follows F distribution with (1, 46) degrees of freedom.
where 1 is for numerator df and 46 is denominator df.
Critical value of F distribution with (1, 46) degrees of freedom at α = 0.05 is F (0.05, 1,46) = 4.0517
By comparing F-Value and Critical F value, we can see that F-Value = 74.1369 > F (0.05, 1,46) =
4.0517, so we reject null hypothesis. So conclude that we have strong evidence that demand (Y)
and unit price (X) are related to each other.
b )
R2 / Coefficient of Determination:
When we fit the regression model fit the data, variation in response variable is explained by the
regressor variable (predictor variable) and error. Coefficient of determination is the proportion
of variation explained by the predictor variable from the total variation. Usually Coefficient of
determination is denoted by R2 and given by
Coefficient of determination = SSReg / SSTotal
where SSTotal is total sum of square.
Coefficient of determination (R2) = 5048.818 / 81.81479 = 0.617103
6
You're viewing a preview
Unlock full access by subscribing today!
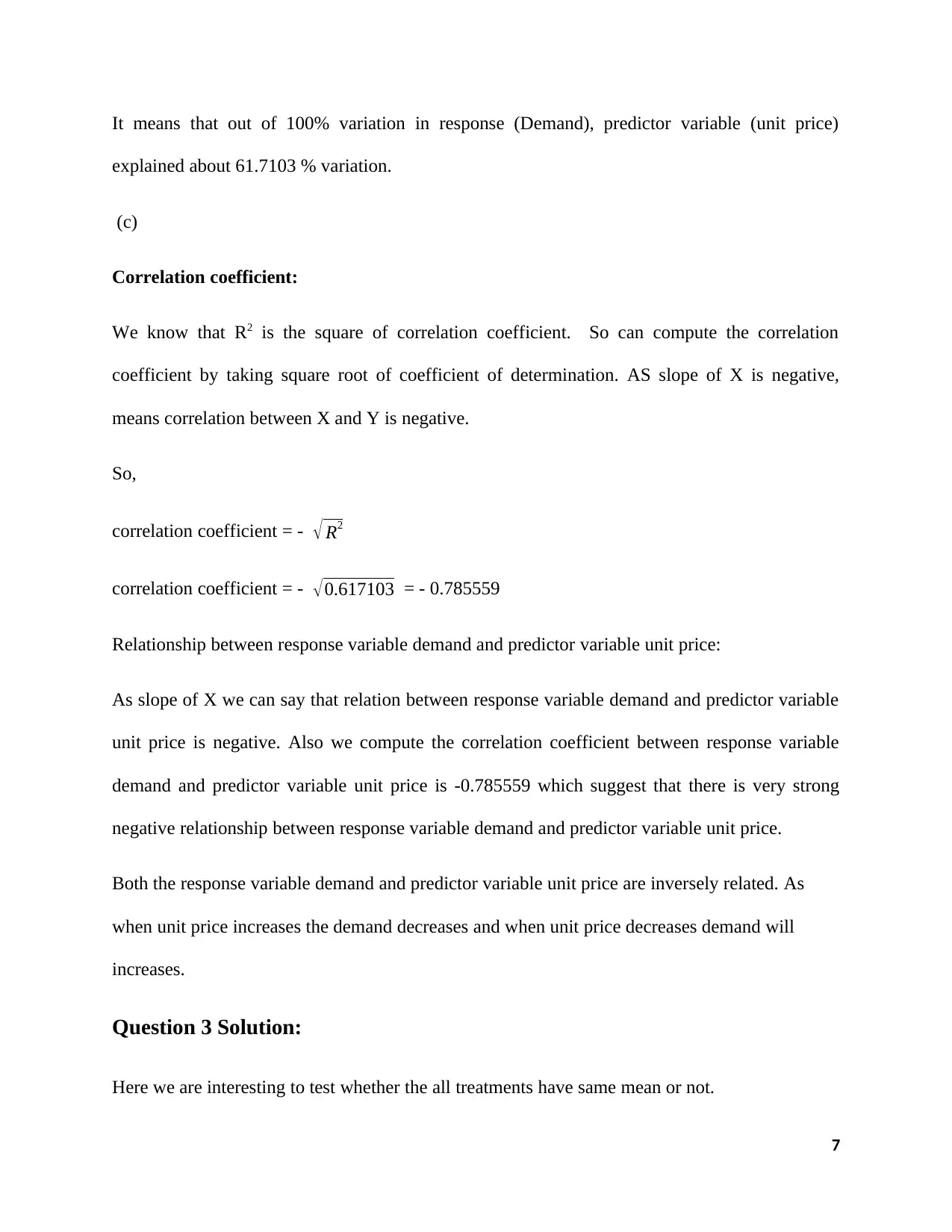
It means that out of 100% variation in response (Demand), predictor variable (unit price)
explained about 61.7103 % variation.
(c)
Correlation coefficient:
We know that R2 is the square of correlation coefficient. So can compute the correlation
coefficient by taking square root of coefficient of determination. AS slope of X is negative,
means correlation between X and Y is negative.
So,
correlation coefficient = - √ R2
correlation coefficient = - √0.617103 = - 0.785559
Relationship between response variable demand and predictor variable unit price:
As slope of X we can say that relation between response variable demand and predictor variable
unit price is negative. Also we compute the correlation coefficient between response variable
demand and predictor variable unit price is -0.785559 which suggest that there is very strong
negative relationship between response variable demand and predictor variable unit price.
Both the response variable demand and predictor variable unit price are inversely related. As
when unit price increases the demand decreases and when unit price decreases demand will
increases.
Question 3 Solution:
Here we are interesting to test whether the all treatments have same mean or not.
7
explained about 61.7103 % variation.
(c)
Correlation coefficient:
We know that R2 is the square of correlation coefficient. So can compute the correlation
coefficient by taking square root of coefficient of determination. AS slope of X is negative,
means correlation between X and Y is negative.
So,
correlation coefficient = - √ R2
correlation coefficient = - √0.617103 = - 0.785559
Relationship between response variable demand and predictor variable unit price:
As slope of X we can say that relation between response variable demand and predictor variable
unit price is negative. Also we compute the correlation coefficient between response variable
demand and predictor variable unit price is -0.785559 which suggest that there is very strong
negative relationship between response variable demand and predictor variable unit price.
Both the response variable demand and predictor variable unit price are inversely related. As
when unit price increases the demand decreases and when unit price decreases demand will
increases.
Question 3 Solution:
Here we are interesting to test whether the all treatments have same mean or not.
7
Paraphrase This Document
Need a fresh take? Get an instant paraphrase of this document with our AI Paraphraser
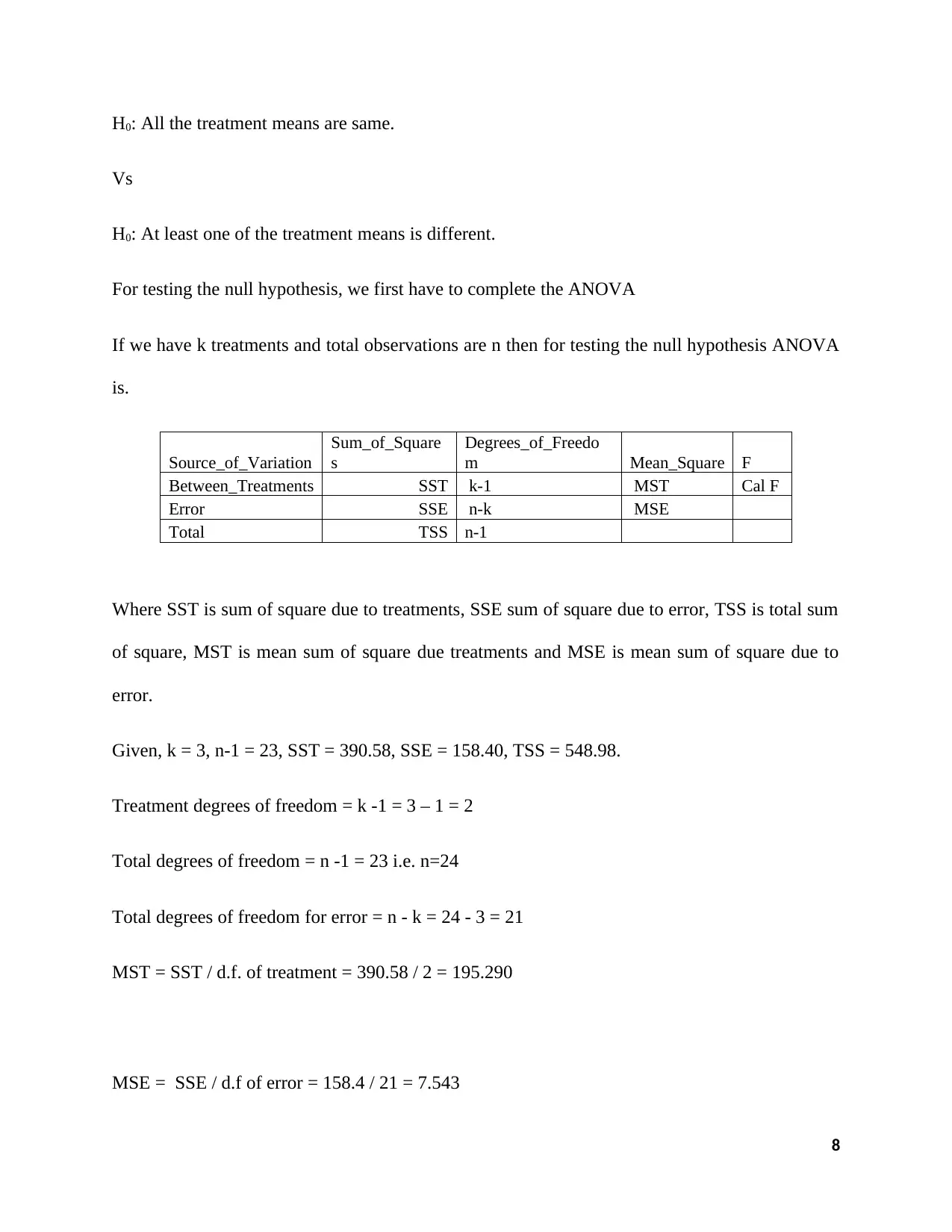
H0: All the treatment means are same.
Vs
H0: At least one of the treatment means is different.
For testing the null hypothesis, we first have to complete the ANOVA
If we have k treatments and total observations are n then for testing the null hypothesis ANOVA
is.
Source_of_Variation
Sum_of_Square
s
Degrees_of_Freedo
m Mean_Square F
Between_Treatments SST k-1 MST Cal F
Error SSE n-k MSE
Total TSS n-1
Where SST is sum of square due to treatments, SSE sum of square due to error, TSS is total sum
of square, MST is mean sum of square due treatments and MSE is mean sum of square due to
error.
Given, k = 3, n-1 = 23, SST = 390.58, SSE = 158.40, TSS = 548.98.
Treatment degrees of freedom = k -1 = 3 – 1 = 2
Total degrees of freedom = n -1 = 23 i.e. n=24
Total degrees of freedom for error = n - k = 24 - 3 = 21
MST = SST / d.f. of treatment = 390.58 / 2 = 195.290
MSE = SSE / d.f of error = 158.4 / 21 = 7.543
8
Vs
H0: At least one of the treatment means is different.
For testing the null hypothesis, we first have to complete the ANOVA
If we have k treatments and total observations are n then for testing the null hypothesis ANOVA
is.
Source_of_Variation
Sum_of_Square
s
Degrees_of_Freedo
m Mean_Square F
Between_Treatments SST k-1 MST Cal F
Error SSE n-k MSE
Total TSS n-1
Where SST is sum of square due to treatments, SSE sum of square due to error, TSS is total sum
of square, MST is mean sum of square due treatments and MSE is mean sum of square due to
error.
Given, k = 3, n-1 = 23, SST = 390.58, SSE = 158.40, TSS = 548.98.
Treatment degrees of freedom = k -1 = 3 – 1 = 2
Total degrees of freedom = n -1 = 23 i.e. n=24
Total degrees of freedom for error = n - k = 24 - 3 = 21
MST = SST / d.f. of treatment = 390.58 / 2 = 195.290
MSE = SSE / d.f of error = 158.4 / 21 = 7.543
8
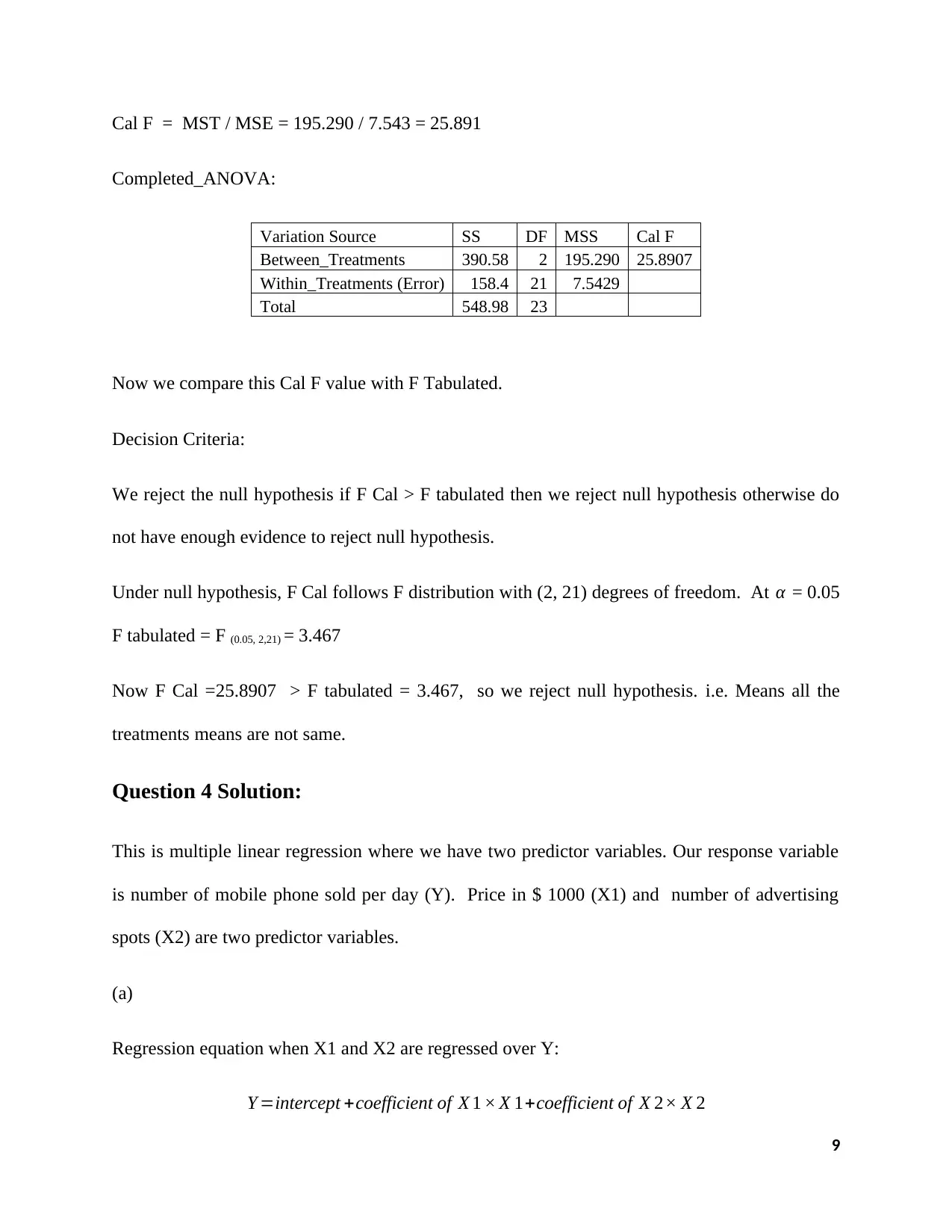
Cal F = MST / MSE = 195.290 / 7.543 = 25.891
Completed_ANOVA:
Variation Source SS DF MSS Cal F
Between_Treatments 390.58 2 195.290 25.8907
Within_Treatments (Error) 158.4 21 7.5429
Total 548.98 23
Now we compare this Cal F value with F Tabulated.
Decision Criteria:
We reject the null hypothesis if F Cal > F tabulated then we reject null hypothesis otherwise do
not have enough evidence to reject null hypothesis.
Under null hypothesis, F Cal follows F distribution with (2, 21) degrees of freedom. At α = 0.05
F tabulated = F (0.05, 2,21) = 3.467
Now F Cal =25.8907 > F tabulated = 3.467, so we reject null hypothesis. i.e. Means all the
treatments means are not same.
Question 4 Solution:
This is multiple linear regression where we have two predictor variables. Our response variable
is number of mobile phone sold per day (Y). Price in $ 1000 (X1) and number of advertising
spots (X2) are two predictor variables.
(a)
Regression equation when X1 and X2 are regressed over Y:
Y =intercept +coefficient of X 1 × X 1+coefficient of X 2× X 2
9
Completed_ANOVA:
Variation Source SS DF MSS Cal F
Between_Treatments 390.58 2 195.290 25.8907
Within_Treatments (Error) 158.4 21 7.5429
Total 548.98 23
Now we compare this Cal F value with F Tabulated.
Decision Criteria:
We reject the null hypothesis if F Cal > F tabulated then we reject null hypothesis otherwise do
not have enough evidence to reject null hypothesis.
Under null hypothesis, F Cal follows F distribution with (2, 21) degrees of freedom. At α = 0.05
F tabulated = F (0.05, 2,21) = 3.467
Now F Cal =25.8907 > F tabulated = 3.467, so we reject null hypothesis. i.e. Means all the
treatments means are not same.
Question 4 Solution:
This is multiple linear regression where we have two predictor variables. Our response variable
is number of mobile phone sold per day (Y). Price in $ 1000 (X1) and number of advertising
spots (X2) are two predictor variables.
(a)
Regression equation when X1 and X2 are regressed over Y:
Y =intercept +coefficient of X 1 × X 1+coefficient of X 2× X 2
9
You're viewing a preview
Unlock full access by subscribing today!
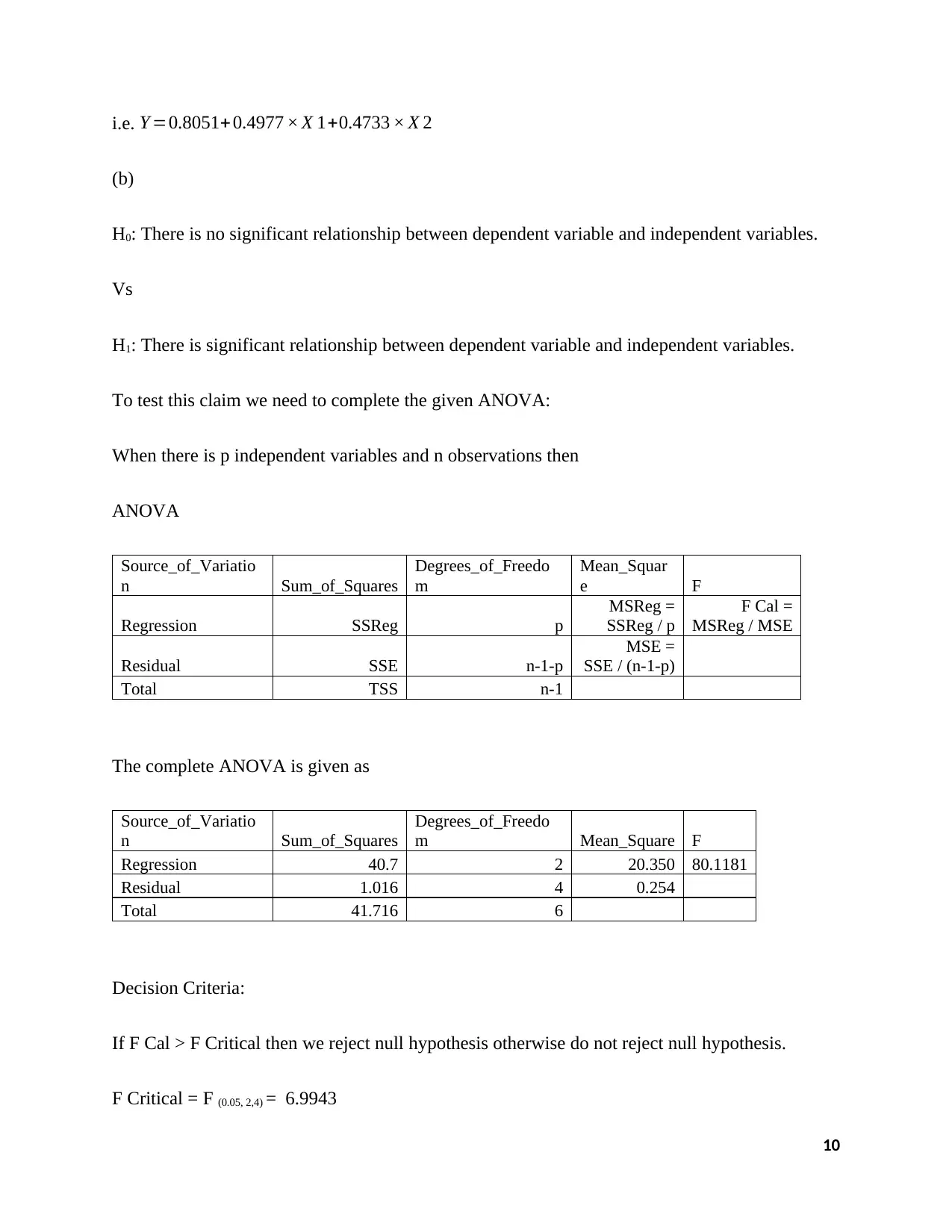
i.e. Y =0.8051+ 0.4977 × X 1+0.4733 × X 2
(b)
H0: There is no significant relationship between dependent variable and independent variables.
Vs
H1: There is significant relationship between dependent variable and independent variables.
To test this claim we need to complete the given ANOVA:
When there is p independent variables and n observations then
ANOVA
Source_of_Variatio
n Sum_of_Squares
Degrees_of_Freedo
m
Mean_Squar
e F
Regression SSReg p
MSReg =
SSReg / p
F Cal =
MSReg / MSE
Residual SSE n-1-p
MSE =
SSE / (n-1-p)
Total TSS n-1
The complete ANOVA is given as
Source_of_Variatio
n Sum_of_Squares
Degrees_of_Freedo
m Mean_Square F
Regression 40.7 2 20.350 80.1181
Residual 1.016 4 0.254
Total 41.716 6
Decision Criteria:
If F Cal > F Critical then we reject null hypothesis otherwise do not reject null hypothesis.
F Critical = F (0.05, 2,4) = 6.9943
10
(b)
H0: There is no significant relationship between dependent variable and independent variables.
Vs
H1: There is significant relationship between dependent variable and independent variables.
To test this claim we need to complete the given ANOVA:
When there is p independent variables and n observations then
ANOVA
Source_of_Variatio
n Sum_of_Squares
Degrees_of_Freedo
m
Mean_Squar
e F
Regression SSReg p
MSReg =
SSReg / p
F Cal =
MSReg / MSE
Residual SSE n-1-p
MSE =
SSE / (n-1-p)
Total TSS n-1
The complete ANOVA is given as
Source_of_Variatio
n Sum_of_Squares
Degrees_of_Freedo
m Mean_Square F
Regression 40.7 2 20.350 80.1181
Residual 1.016 4 0.254
Total 41.716 6
Decision Criteria:
If F Cal > F Critical then we reject null hypothesis otherwise do not reject null hypothesis.
F Critical = F (0.05, 2,4) = 6.9943
10
Paraphrase This Document
Need a fresh take? Get an instant paraphrase of this document with our AI Paraphraser
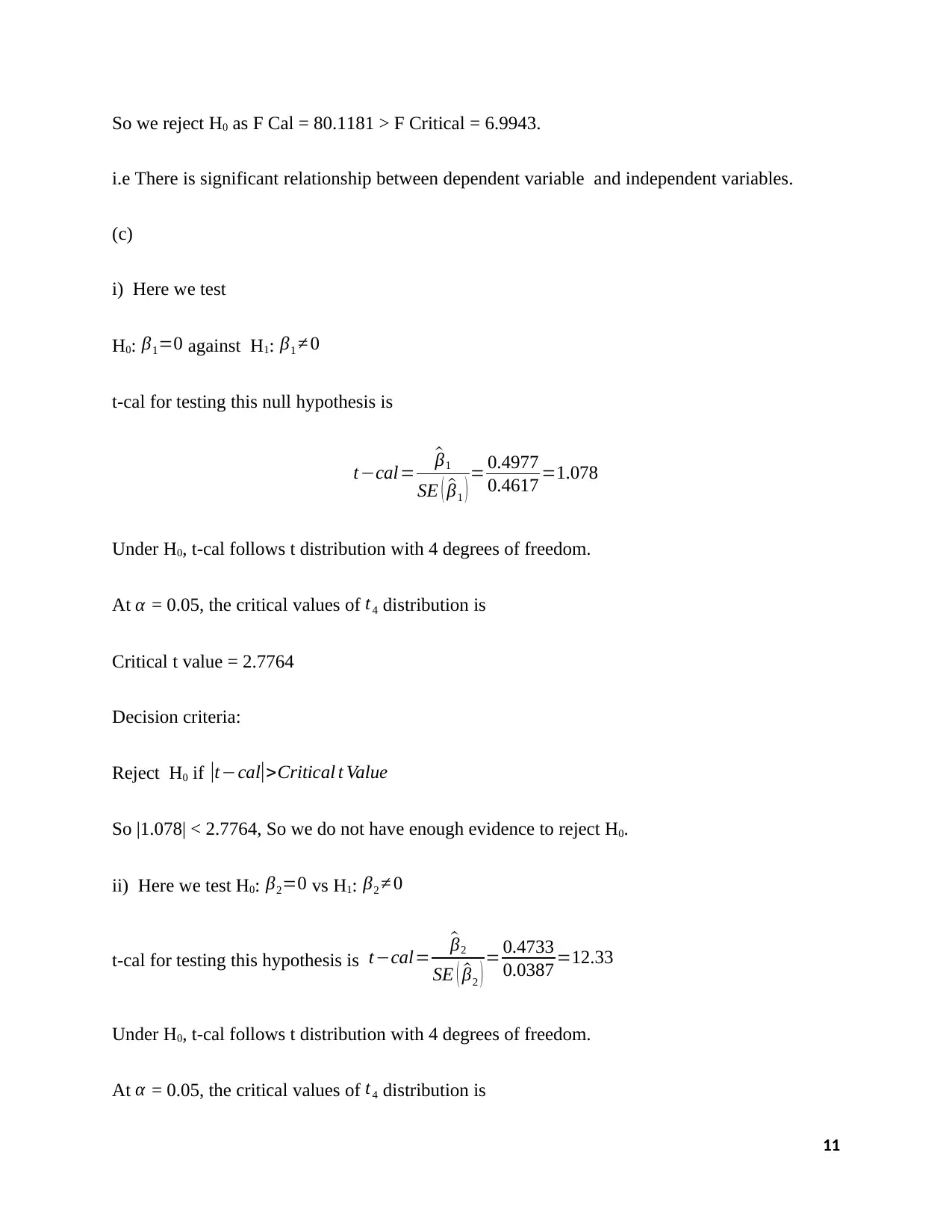
So we reject H0 as F Cal = 80.1181 > F Critical = 6.9943.
i.e There is significant relationship between dependent variable and independent variables.
(c)
i) Here we test
H0: β1=0 against H1: β1 ≠ 0
t-cal for testing this null hypothesis is
t−cal= ^β1
SE ( ^β1 ) = 0.4977
0.4617 =1.078
Under H0, t-cal follows t distribution with 4 degrees of freedom.
At α = 0.05, the critical values of t4 distribution is
Critical t value = 2.7764
Decision criteria:
Reject H0 if |t−cal|>Critical t Value
So |1.078| < 2.7764, So we do not have enough evidence to reject H0.
ii) Here we test H0: β2=0 vs H1: β2 ≠ 0
t-cal for testing this hypothesis is t−cal=
^β2
SE ( ^β2 ) = 0.4733
0.0387 =12.33
Under H0, t-cal follows t distribution with 4 degrees of freedom.
At α = 0.05, the critical values of t4 distribution is
11
i.e There is significant relationship between dependent variable and independent variables.
(c)
i) Here we test
H0: β1=0 against H1: β1 ≠ 0
t-cal for testing this null hypothesis is
t−cal= ^β1
SE ( ^β1 ) = 0.4977
0.4617 =1.078
Under H0, t-cal follows t distribution with 4 degrees of freedom.
At α = 0.05, the critical values of t4 distribution is
Critical t value = 2.7764
Decision criteria:
Reject H0 if |t−cal|>Critical t Value
So |1.078| < 2.7764, So we do not have enough evidence to reject H0.
ii) Here we test H0: β2=0 vs H1: β2 ≠ 0
t-cal for testing this hypothesis is t−cal=
^β2
SE ( ^β2 ) = 0.4733
0.0387 =12.33
Under H0, t-cal follows t distribution with 4 degrees of freedom.
At α = 0.05, the critical values of t4 distribution is
11
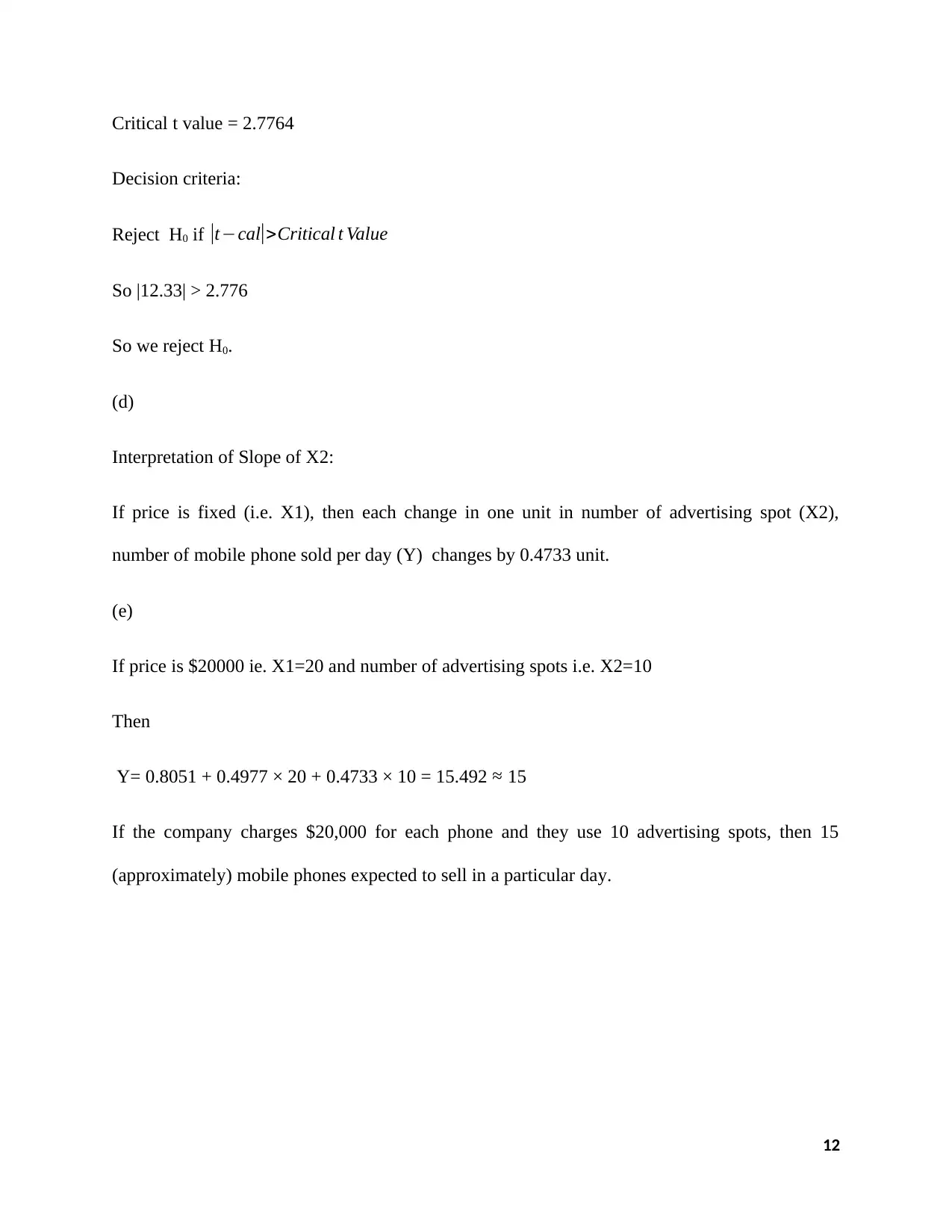
Critical t value = 2.7764
Decision criteria:
Reject H0 if |t−cal|>Critical t Value
So |12.33| > 2.776
So we reject H0.
(d)
Interpretation of Slope of X2:
If price is fixed (i.e. X1), then each change in one unit in number of advertising spot (X2),
number of mobile phone sold per day (Y) changes by 0.4733 unit.
(e)
If price is $20000 ie. X1=20 and number of advertising spots i.e. X2=10
Then
Y= 0.8051 + 0.4977 × 20 + 0.4733 × 10 = 15.492 ≈ 15
If the company charges $20,000 for each phone and they use 10 advertising spots, then 15
(approximately) mobile phones expected to sell in a particular day.
12
Decision criteria:
Reject H0 if |t−cal|>Critical t Value
So |12.33| > 2.776
So we reject H0.
(d)
Interpretation of Slope of X2:
If price is fixed (i.e. X1), then each change in one unit in number of advertising spot (X2),
number of mobile phone sold per day (Y) changes by 0.4733 unit.
(e)
If price is $20000 ie. X1=20 and number of advertising spots i.e. X2=10
Then
Y= 0.8051 + 0.4977 × 20 + 0.4733 × 10 = 15.492 ≈ 15
If the company charges $20,000 for each phone and they use 10 advertising spots, then 15
(approximately) mobile phones expected to sell in a particular day.
12
You're viewing a preview
Unlock full access by subscribing today!
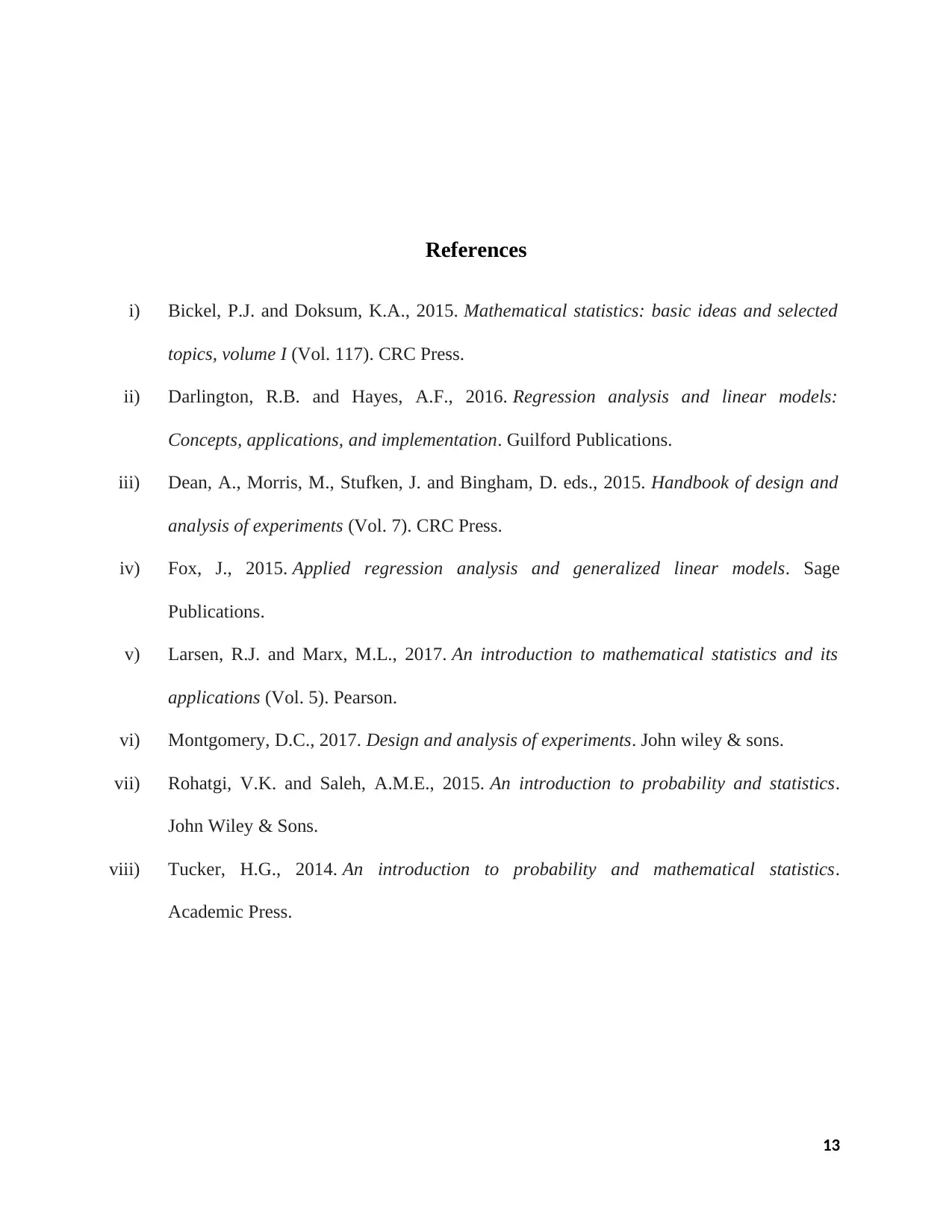
References
i) Bickel, P.J. and Doksum, K.A., 2015. Mathematical statistics: basic ideas and selected
topics, volume I (Vol. 117). CRC Press.
ii) Darlington, R.B. and Hayes, A.F., 2016. Regression analysis and linear models:
Concepts, applications, and implementation. Guilford Publications.
iii) Dean, A., Morris, M., Stufken, J. and Bingham, D. eds., 2015. Handbook of design and
analysis of experiments (Vol. 7). CRC Press.
iv) Fox, J., 2015. Applied regression analysis and generalized linear models. Sage
Publications.
v) Larsen, R.J. and Marx, M.L., 2017. An introduction to mathematical statistics and its
applications (Vol. 5). Pearson.
vi) Montgomery, D.C., 2017. Design and analysis of experiments. John wiley & sons.
vii) Rohatgi, V.K. and Saleh, A.M.E., 2015. An introduction to probability and statistics.
John Wiley & Sons.
viii) Tucker, H.G., 2014. An introduction to probability and mathematical statistics.
Academic Press.
13
i) Bickel, P.J. and Doksum, K.A., 2015. Mathematical statistics: basic ideas and selected
topics, volume I (Vol. 117). CRC Press.
ii) Darlington, R.B. and Hayes, A.F., 2016. Regression analysis and linear models:
Concepts, applications, and implementation. Guilford Publications.
iii) Dean, A., Morris, M., Stufken, J. and Bingham, D. eds., 2015. Handbook of design and
analysis of experiments (Vol. 7). CRC Press.
iv) Fox, J., 2015. Applied regression analysis and generalized linear models. Sage
Publications.
v) Larsen, R.J. and Marx, M.L., 2017. An introduction to mathematical statistics and its
applications (Vol. 5). Pearson.
vi) Montgomery, D.C., 2017. Design and analysis of experiments. John wiley & sons.
vii) Rohatgi, V.K. and Saleh, A.M.E., 2015. An introduction to probability and statistics.
John Wiley & Sons.
viii) Tucker, H.G., 2014. An introduction to probability and mathematical statistics.
Academic Press.
13
1 out of 13
Related Documents

Your All-in-One AI-Powered Toolkit for Academic Success.
+13062052269
info@desklib.com
Available 24*7 on WhatsApp / Email
Unlock your academic potential
© 2024 | Zucol Services PVT LTD | All rights reserved.