Grouping Data into Tables using RStudio for Manufacturing Energy Consumption Survey (MECS) except the Petroleum Refining Industry
VerifiedAdded on 2022/11/23
|9
|1456
|141
AI Summary
This report will highlight the distribution of the Manufacturing, Energy Consumption Survey (MECS) except the Petroleum Refining Industry by the MECS region. The data used will be from the MECS 2014 which in total had 20,117 establishments which is a good representation of the US industry. The survey data are collected as a national sample survey with information about energy consumption and expenditures and energy-related characteristics.
Contribute Materials
Your contribution can guide someone’s learning journey. Share your
documents today.
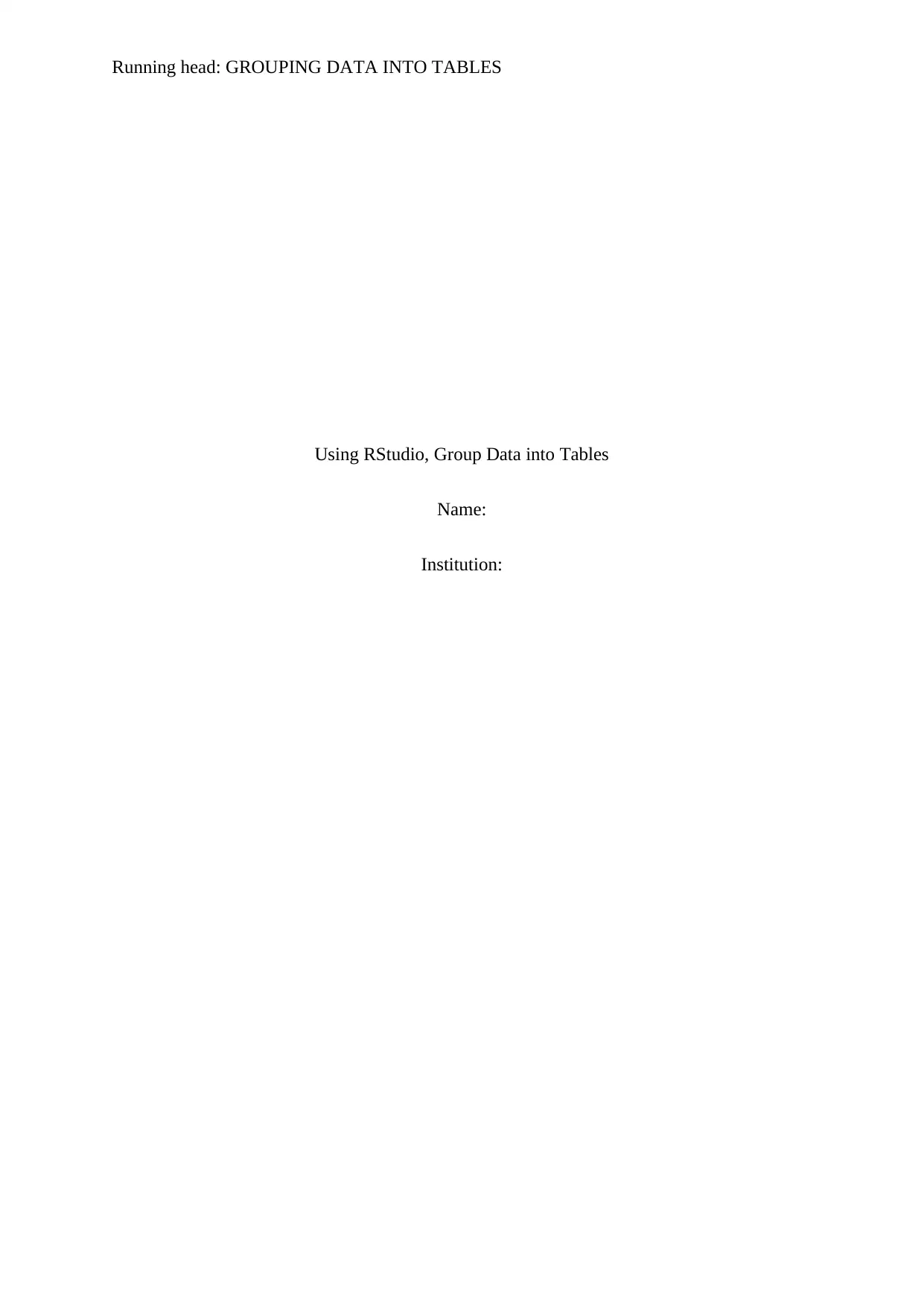
Running head: GROUPING DATA INTO TABLES
Using RStudio, Group Data into Tables
Name:
Institution:
Using RStudio, Group Data into Tables
Name:
Institution:
Secure Best Marks with AI Grader
Need help grading? Try our AI Grader for instant feedback on your assignments.
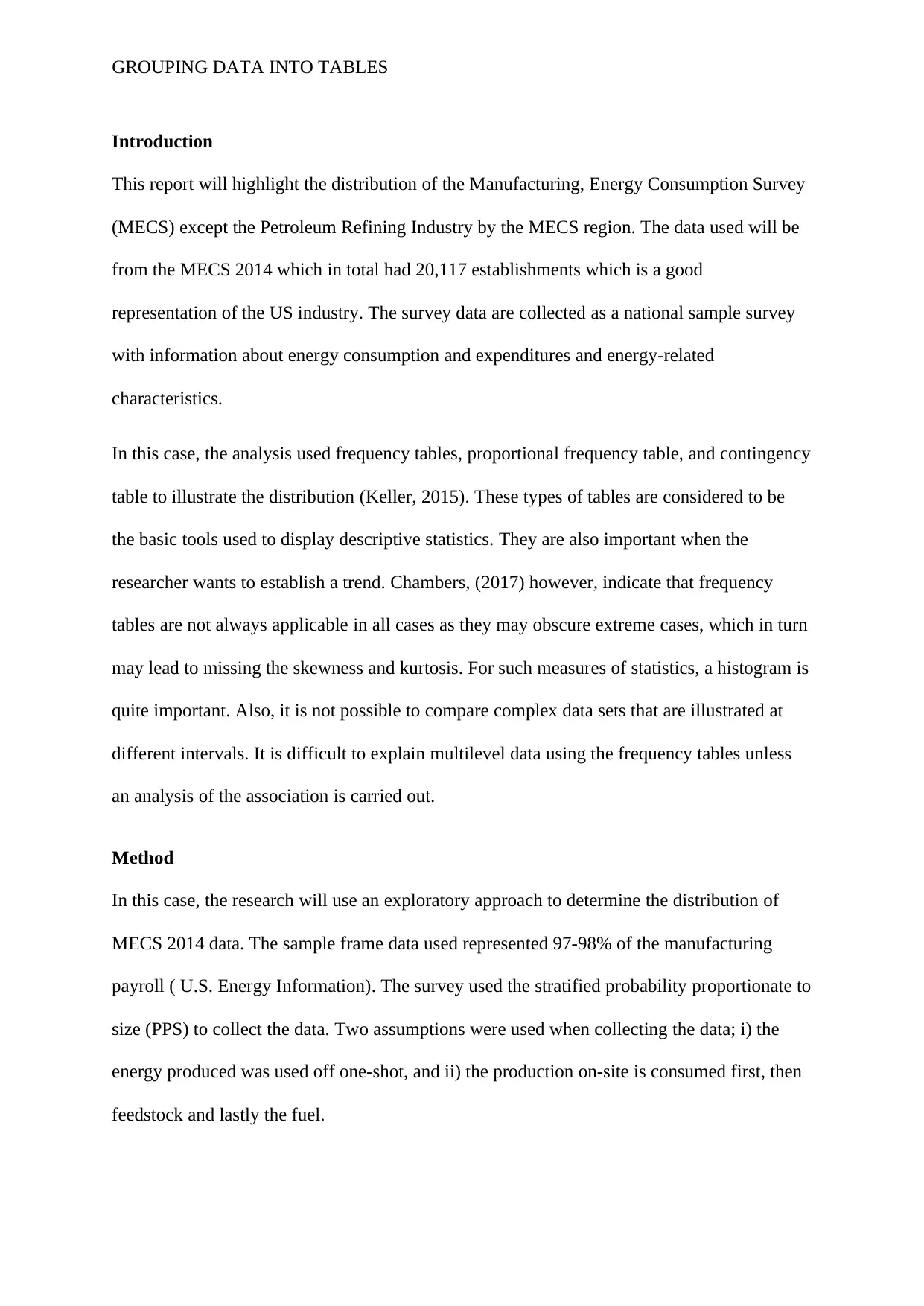
GROUPING DATA INTO TABLES
Introduction
This report will highlight the distribution of the Manufacturing, Energy Consumption Survey
(MECS) except the Petroleum Refining Industry by the MECS region. The data used will be
from the MECS 2014 which in total had 20,117 establishments which is a good
representation of the US industry. The survey data are collected as a national sample survey
with information about energy consumption and expenditures and energy-related
characteristics.
In this case, the analysis used frequency tables, proportional frequency table, and contingency
table to illustrate the distribution (Keller, 2015). These types of tables are considered to be
the basic tools used to display descriptive statistics. They are also important when the
researcher wants to establish a trend. Chambers, (2017) however, indicate that frequency
tables are not always applicable in all cases as they may obscure extreme cases, which in turn
may lead to missing the skewness and kurtosis. For such measures of statistics, a histogram is
quite important. Also, it is not possible to compare complex data sets that are illustrated at
different intervals. It is difficult to explain multilevel data using the frequency tables unless
an analysis of the association is carried out.
Method
In this case, the research will use an exploratory approach to determine the distribution of
MECS 2014 data. The sample frame data used represented 97-98% of the manufacturing
payroll ( U.S. Energy Information). The survey used the stratified probability proportionate to
size (PPS) to collect the data. Two assumptions were used when collecting the data; i) the
energy produced was used off one-shot, and ii) the production on-site is consumed first, then
feedstock and lastly the fuel.
Introduction
This report will highlight the distribution of the Manufacturing, Energy Consumption Survey
(MECS) except the Petroleum Refining Industry by the MECS region. The data used will be
from the MECS 2014 which in total had 20,117 establishments which is a good
representation of the US industry. The survey data are collected as a national sample survey
with information about energy consumption and expenditures and energy-related
characteristics.
In this case, the analysis used frequency tables, proportional frequency table, and contingency
table to illustrate the distribution (Keller, 2015). These types of tables are considered to be
the basic tools used to display descriptive statistics. They are also important when the
researcher wants to establish a trend. Chambers, (2017) however, indicate that frequency
tables are not always applicable in all cases as they may obscure extreme cases, which in turn
may lead to missing the skewness and kurtosis. For such measures of statistics, a histogram is
quite important. Also, it is not possible to compare complex data sets that are illustrated at
different intervals. It is difficult to explain multilevel data using the frequency tables unless
an analysis of the association is carried out.
Method
In this case, the research will use an exploratory approach to determine the distribution of
MECS 2014 data. The sample frame data used represented 97-98% of the manufacturing
payroll ( U.S. Energy Information). The survey used the stratified probability proportionate to
size (PPS) to collect the data. Two assumptions were used when collecting the data; i) the
energy produced was used off one-shot, and ii) the production on-site is consumed first, then
feedstock and lastly the fuel.
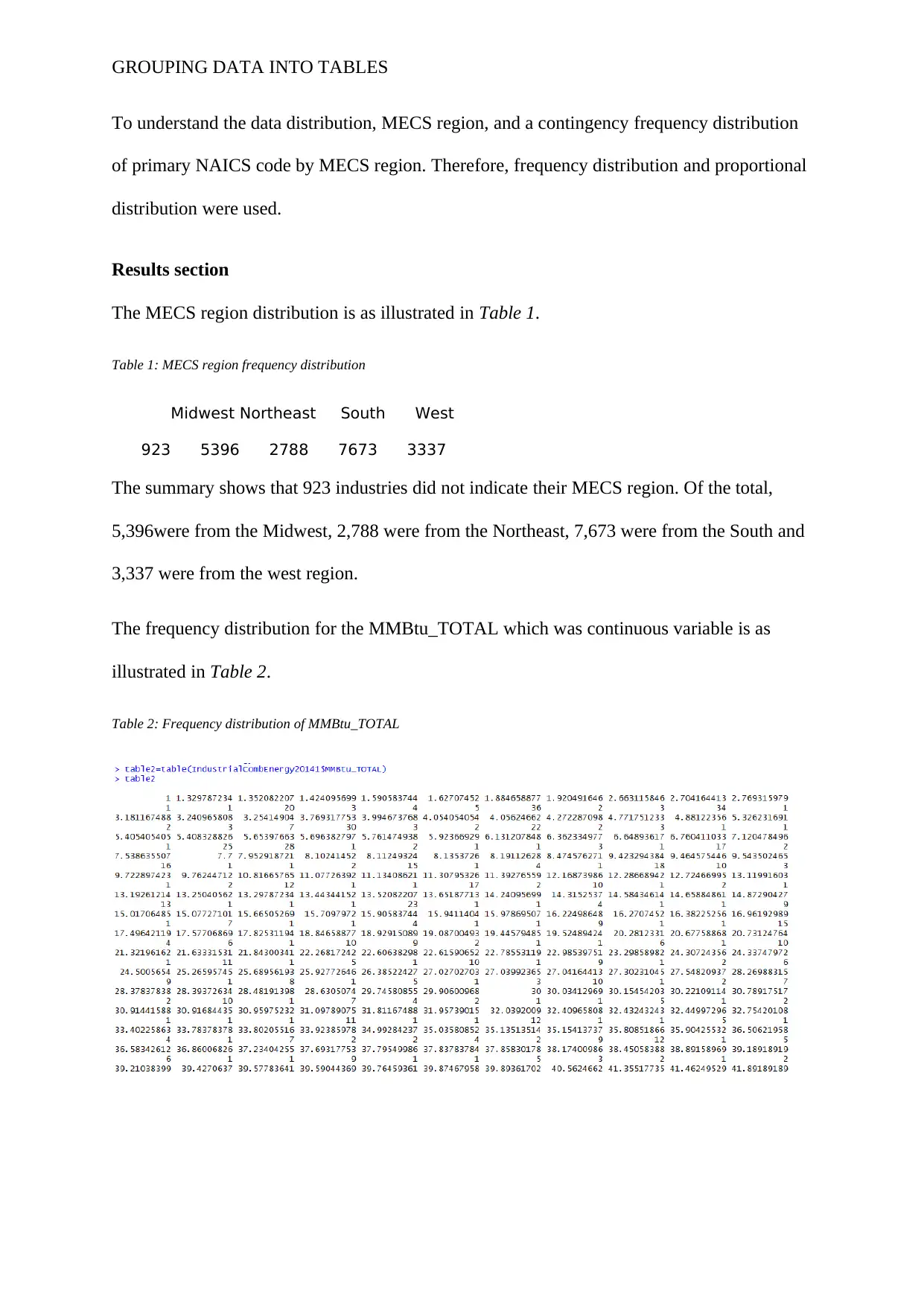
GROUPING DATA INTO TABLES
To understand the data distribution, MECS region, and a contingency frequency distribution
of primary NAICS code by MECS region. Therefore, frequency distribution and proportional
distribution were used.
Results section
The MECS region distribution is as illustrated in Table 1.
Table 1: MECS region frequency distribution
Midwest Northeast South West
923 5396 2788 7673 3337
The summary shows that 923 industries did not indicate their MECS region. Of the total,
5,396were from the Midwest, 2,788 were from the Northeast, 7,673 were from the South and
3,337 were from the west region.
The frequency distribution for the MMBtu_TOTAL which was continuous variable is as
illustrated in Table 2.
Table 2: Frequency distribution of MMBtu_TOTAL
To understand the data distribution, MECS region, and a contingency frequency distribution
of primary NAICS code by MECS region. Therefore, frequency distribution and proportional
distribution were used.
Results section
The MECS region distribution is as illustrated in Table 1.
Table 1: MECS region frequency distribution
Midwest Northeast South West
923 5396 2788 7673 3337
The summary shows that 923 industries did not indicate their MECS region. Of the total,
5,396were from the Midwest, 2,788 were from the Northeast, 7,673 were from the South and
3,337 were from the west region.
The frequency distribution for the MMBtu_TOTAL which was continuous variable is as
illustrated in Table 2.
Table 2: Frequency distribution of MMBtu_TOTAL
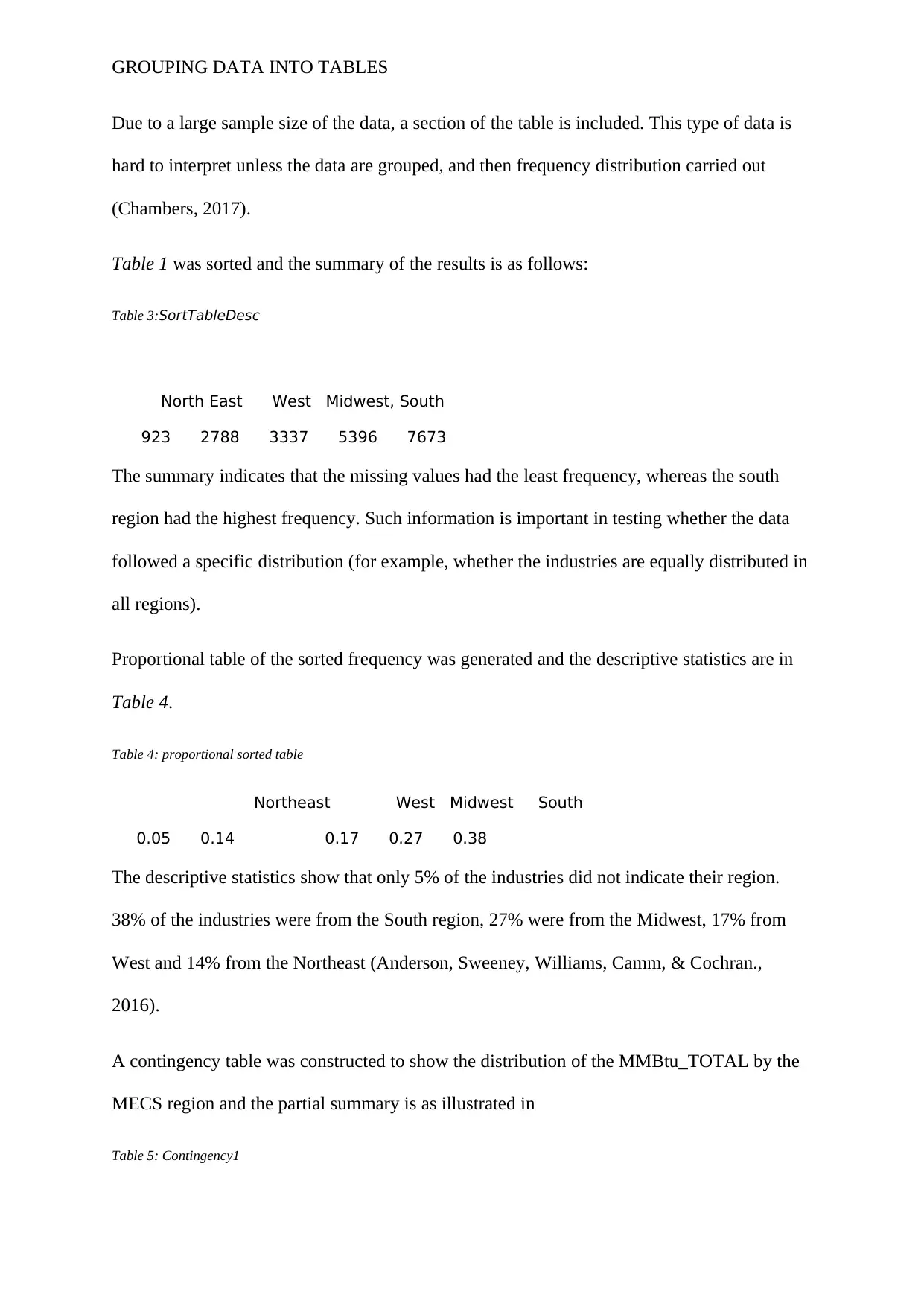
GROUPING DATA INTO TABLES
Due to a large sample size of the data, a section of the table is included. This type of data is
hard to interpret unless the data are grouped, and then frequency distribution carried out
(Chambers, 2017).
Table 1 was sorted and the summary of the results is as follows:
Table 3:SortTableDesc
North East West Midwest, South
923 2788 3337 5396 7673
The summary indicates that the missing values had the least frequency, whereas the south
region had the highest frequency. Such information is important in testing whether the data
followed a specific distribution (for example, whether the industries are equally distributed in
all regions).
Proportional table of the sorted frequency was generated and the descriptive statistics are in
Table 4.
Table 4: proportional sorted table
Northeast West Midwest South
0.05 0.14 0.17 0.27 0.38
The descriptive statistics show that only 5% of the industries did not indicate their region.
38% of the industries were from the South region, 27% were from the Midwest, 17% from
West and 14% from the Northeast (Anderson, Sweeney, Williams, Camm, & Cochran.,
2016).
A contingency table was constructed to show the distribution of the MMBtu_TOTAL by the
MECS region and the partial summary is as illustrated in
Table 5: Contingency1
Due to a large sample size of the data, a section of the table is included. This type of data is
hard to interpret unless the data are grouped, and then frequency distribution carried out
(Chambers, 2017).
Table 1 was sorted and the summary of the results is as follows:
Table 3:SortTableDesc
North East West Midwest, South
923 2788 3337 5396 7673
The summary indicates that the missing values had the least frequency, whereas the south
region had the highest frequency. Such information is important in testing whether the data
followed a specific distribution (for example, whether the industries are equally distributed in
all regions).
Proportional table of the sorted frequency was generated and the descriptive statistics are in
Table 4.
Table 4: proportional sorted table
Northeast West Midwest South
0.05 0.14 0.17 0.27 0.38
The descriptive statistics show that only 5% of the industries did not indicate their region.
38% of the industries were from the South region, 27% were from the Midwest, 17% from
West and 14% from the Northeast (Anderson, Sweeney, Williams, Camm, & Cochran.,
2016).
A contingency table was constructed to show the distribution of the MMBtu_TOTAL by the
MECS region and the partial summary is as illustrated in
Table 5: Contingency1
Secure Best Marks with AI Grader
Need help grading? Try our AI Grader for instant feedback on your assignments.
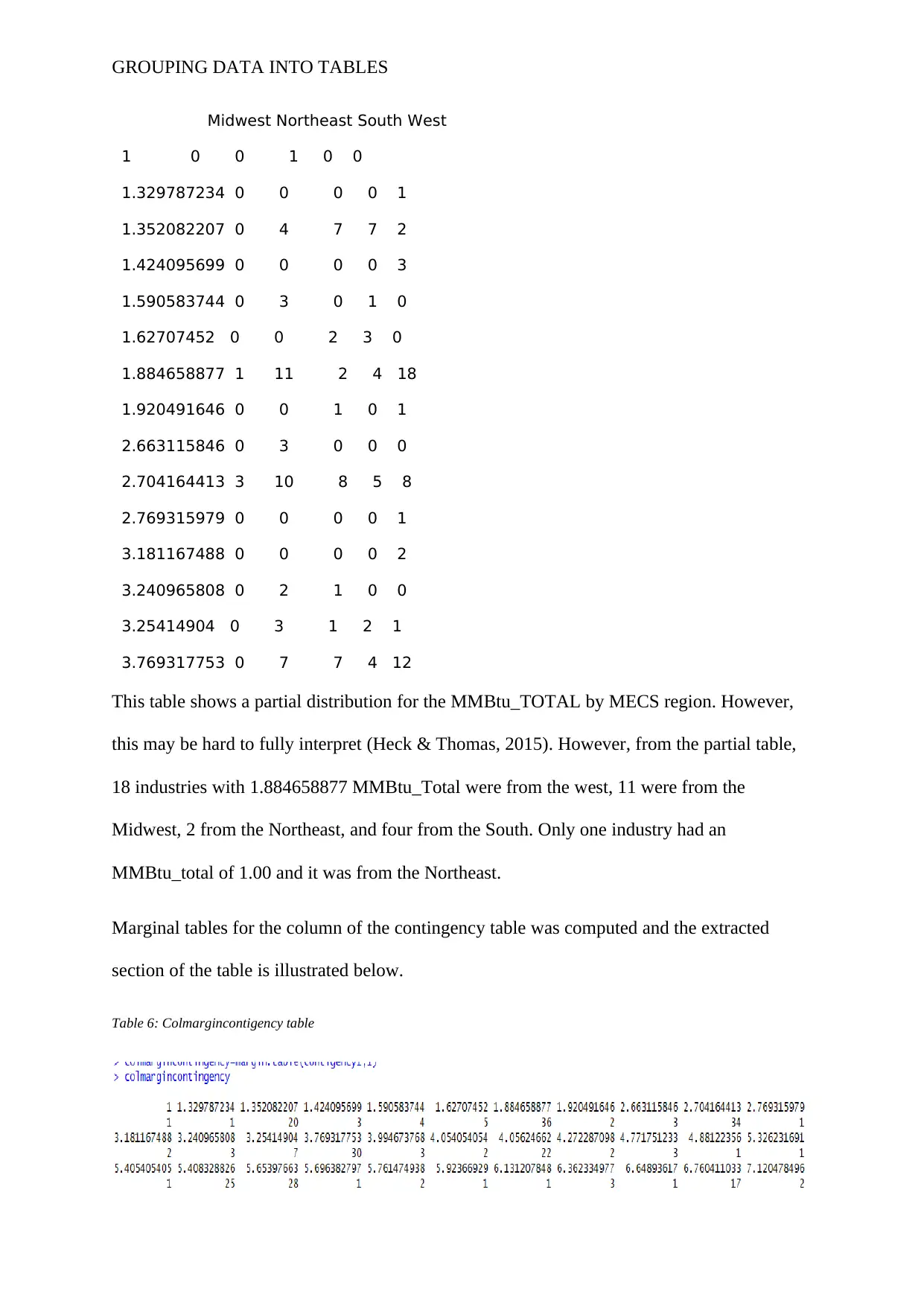
GROUPING DATA INTO TABLES
Midwest Northeast South West
1 0 0 1 0 0
1.329787234 0 0 0 0 1
1.352082207 0 4 7 7 2
1.424095699 0 0 0 0 3
1.590583744 0 3 0 1 0
1.62707452 0 0 2 3 0
1.884658877 1 11 2 4 18
1.920491646 0 0 1 0 1
2.663115846 0 3 0 0 0
2.704164413 3 10 8 5 8
2.769315979 0 0 0 0 1
3.181167488 0 0 0 0 2
3.240965808 0 2 1 0 0
3.25414904 0 3 1 2 1
3.769317753 0 7 7 4 12
This table shows a partial distribution for the MMBtu_TOTAL by MECS region. However,
this may be hard to fully interpret (Heck & Thomas, 2015). However, from the partial table,
18 industries with 1.884658877 MMBtu_Total were from the west, 11 were from the
Midwest, 2 from the Northeast, and four from the South. Only one industry had an
MMBtu_total of 1.00 and it was from the Northeast.
Marginal tables for the column of the contingency table was computed and the extracted
section of the table is illustrated below.
Table 6: Colmargincontigency table
Midwest Northeast South West
1 0 0 1 0 0
1.329787234 0 0 0 0 1
1.352082207 0 4 7 7 2
1.424095699 0 0 0 0 3
1.590583744 0 3 0 1 0
1.62707452 0 0 2 3 0
1.884658877 1 11 2 4 18
1.920491646 0 0 1 0 1
2.663115846 0 3 0 0 0
2.704164413 3 10 8 5 8
2.769315979 0 0 0 0 1
3.181167488 0 0 0 0 2
3.240965808 0 2 1 0 0
3.25414904 0 3 1 2 1
3.769317753 0 7 7 4 12
This table shows a partial distribution for the MMBtu_TOTAL by MECS region. However,
this may be hard to fully interpret (Heck & Thomas, 2015). However, from the partial table,
18 industries with 1.884658877 MMBtu_Total were from the west, 11 were from the
Midwest, 2 from the Northeast, and four from the South. Only one industry had an
MMBtu_total of 1.00 and it was from the Northeast.
Marginal tables for the column of the contingency table was computed and the extracted
section of the table is illustrated below.
Table 6: Colmargincontigency table
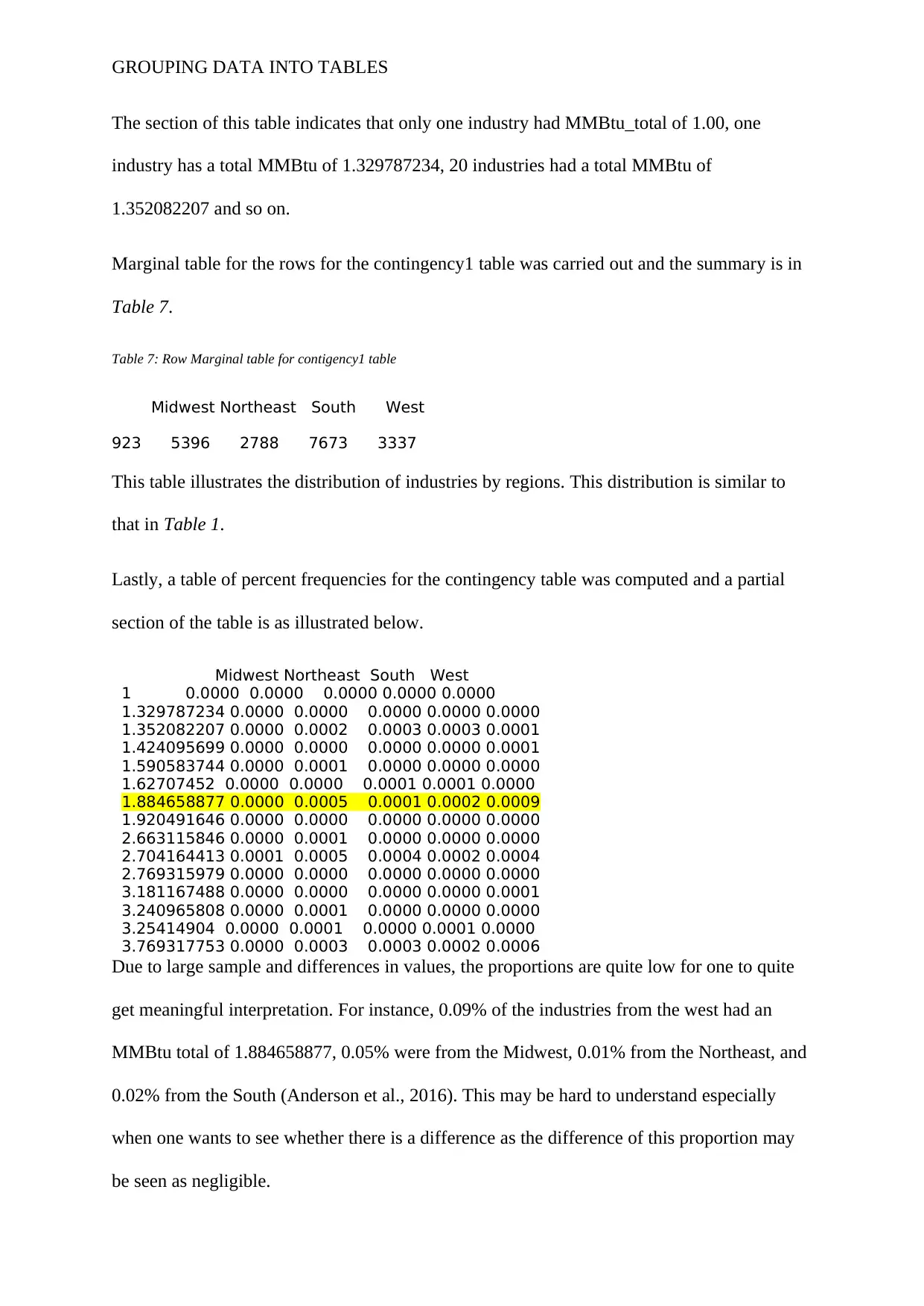
GROUPING DATA INTO TABLES
The section of this table indicates that only one industry had MMBtu_total of 1.00, one
industry has a total MMBtu of 1.329787234, 20 industries had a total MMBtu of
1.352082207 and so on.
Marginal table for the rows for the contingency1 table was carried out and the summary is in
Table 7.
Table 7: Row Marginal table for contigency1 table
Midwest Northeast South West
923 5396 2788 7673 3337
This table illustrates the distribution of industries by regions. This distribution is similar to
that in Table 1.
Lastly, a table of percent frequencies for the contingency table was computed and a partial
section of the table is as illustrated below.
Midwest Northeast South West
1 0.0000 0.0000 0.0000 0.0000 0.0000
1.329787234 0.0000 0.0000 0.0000 0.0000 0.0000
1.352082207 0.0000 0.0002 0.0003 0.0003 0.0001
1.424095699 0.0000 0.0000 0.0000 0.0000 0.0001
1.590583744 0.0000 0.0001 0.0000 0.0000 0.0000
1.62707452 0.0000 0.0000 0.0001 0.0001 0.0000
1.884658877 0.0000 0.0005 0.0001 0.0002 0.0009
1.920491646 0.0000 0.0000 0.0000 0.0000 0.0000
2.663115846 0.0000 0.0001 0.0000 0.0000 0.0000
2.704164413 0.0001 0.0005 0.0004 0.0002 0.0004
2.769315979 0.0000 0.0000 0.0000 0.0000 0.0000
3.181167488 0.0000 0.0000 0.0000 0.0000 0.0001
3.240965808 0.0000 0.0001 0.0000 0.0000 0.0000
3.25414904 0.0000 0.0001 0.0000 0.0001 0.0000
3.769317753 0.0000 0.0003 0.0003 0.0002 0.0006
Due to large sample and differences in values, the proportions are quite low for one to quite
get meaningful interpretation. For instance, 0.09% of the industries from the west had an
MMBtu total of 1.884658877, 0.05% were from the Midwest, 0.01% from the Northeast, and
0.02% from the South (Anderson et al., 2016). This may be hard to understand especially
when one wants to see whether there is a difference as the difference of this proportion may
be seen as negligible.
The section of this table indicates that only one industry had MMBtu_total of 1.00, one
industry has a total MMBtu of 1.329787234, 20 industries had a total MMBtu of
1.352082207 and so on.
Marginal table for the rows for the contingency1 table was carried out and the summary is in
Table 7.
Table 7: Row Marginal table for contigency1 table
Midwest Northeast South West
923 5396 2788 7673 3337
This table illustrates the distribution of industries by regions. This distribution is similar to
that in Table 1.
Lastly, a table of percent frequencies for the contingency table was computed and a partial
section of the table is as illustrated below.
Midwest Northeast South West
1 0.0000 0.0000 0.0000 0.0000 0.0000
1.329787234 0.0000 0.0000 0.0000 0.0000 0.0000
1.352082207 0.0000 0.0002 0.0003 0.0003 0.0001
1.424095699 0.0000 0.0000 0.0000 0.0000 0.0001
1.590583744 0.0000 0.0001 0.0000 0.0000 0.0000
1.62707452 0.0000 0.0000 0.0001 0.0001 0.0000
1.884658877 0.0000 0.0005 0.0001 0.0002 0.0009
1.920491646 0.0000 0.0000 0.0000 0.0000 0.0000
2.663115846 0.0000 0.0001 0.0000 0.0000 0.0000
2.704164413 0.0001 0.0005 0.0004 0.0002 0.0004
2.769315979 0.0000 0.0000 0.0000 0.0000 0.0000
3.181167488 0.0000 0.0000 0.0000 0.0000 0.0001
3.240965808 0.0000 0.0001 0.0000 0.0000 0.0000
3.25414904 0.0000 0.0001 0.0000 0.0001 0.0000
3.769317753 0.0000 0.0003 0.0003 0.0002 0.0006
Due to large sample and differences in values, the proportions are quite low for one to quite
get meaningful interpretation. For instance, 0.09% of the industries from the west had an
MMBtu total of 1.884658877, 0.05% were from the Midwest, 0.01% from the Northeast, and
0.02% from the South (Anderson et al., 2016). This may be hard to understand especially
when one wants to see whether there is a difference as the difference of this proportion may
be seen as negligible.
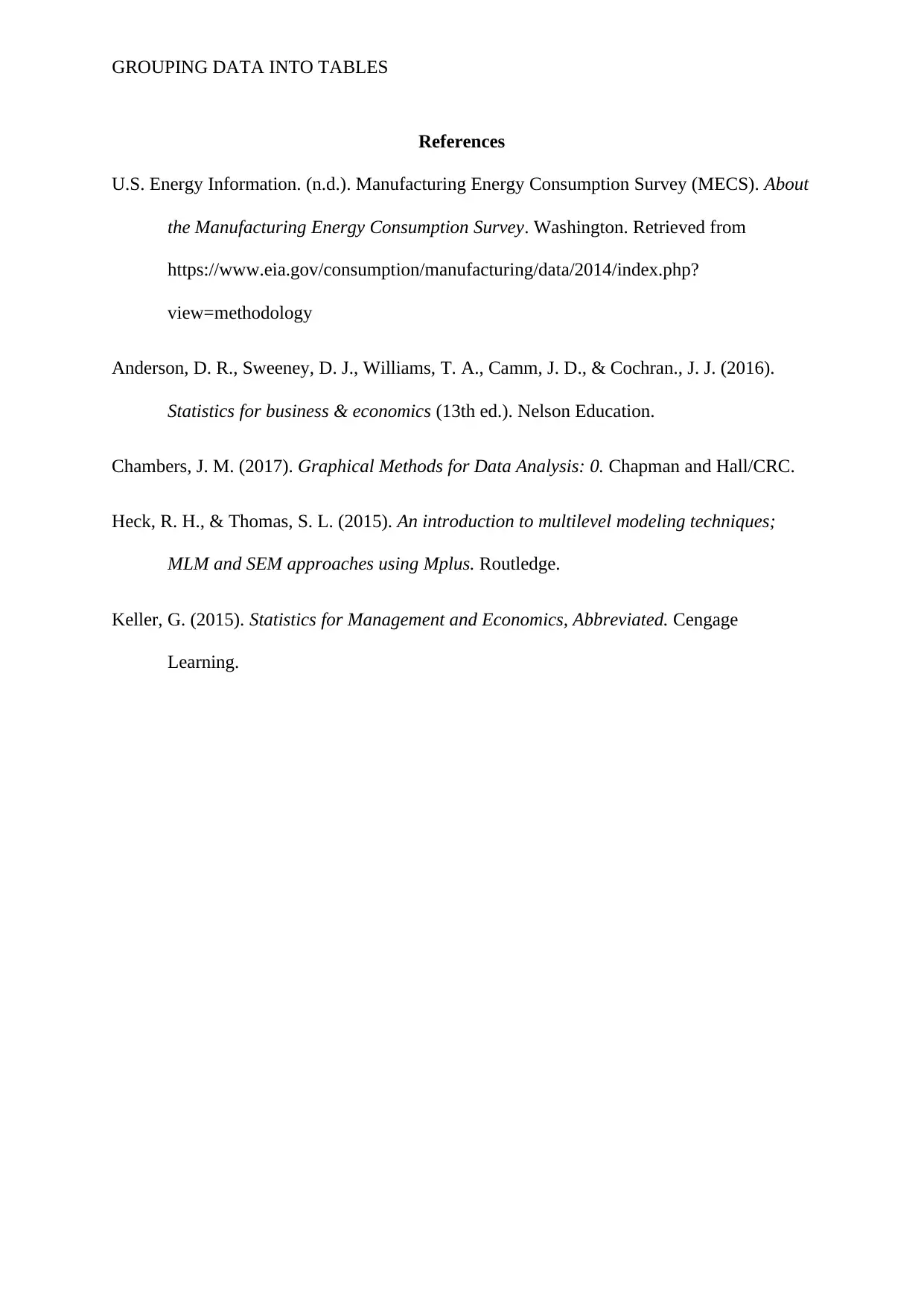
GROUPING DATA INTO TABLES
References
U.S. Energy Information. (n.d.). Manufacturing Energy Consumption Survey (MECS). About
the Manufacturing Energy Consumption Survey. Washington. Retrieved from
https://www.eia.gov/consumption/manufacturing/data/2014/index.php?
view=methodology
Anderson, D. R., Sweeney, D. J., Williams, T. A., Camm, J. D., & Cochran., J. J. (2016).
Statistics for business & economics (13th ed.). Nelson Education.
Chambers, J. M. (2017). Graphical Methods for Data Analysis: 0. Chapman and Hall/CRC.
Heck, R. H., & Thomas, S. L. (2015). An introduction to multilevel modeling techniques;
MLM and SEM approaches using Mplus. Routledge.
Keller, G. (2015). Statistics for Management and Economics, Abbreviated. Cengage
Learning.
References
U.S. Energy Information. (n.d.). Manufacturing Energy Consumption Survey (MECS). About
the Manufacturing Energy Consumption Survey. Washington. Retrieved from
https://www.eia.gov/consumption/manufacturing/data/2014/index.php?
view=methodology
Anderson, D. R., Sweeney, D. J., Williams, T. A., Camm, J. D., & Cochran., J. J. (2016).
Statistics for business & economics (13th ed.). Nelson Education.
Chambers, J. M. (2017). Graphical Methods for Data Analysis: 0. Chapman and Hall/CRC.
Heck, R. H., & Thomas, S. L. (2015). An introduction to multilevel modeling techniques;
MLM and SEM approaches using Mplus. Routledge.
Keller, G. (2015). Statistics for Management and Economics, Abbreviated. Cengage
Learning.
Paraphrase This Document
Need a fresh take? Get an instant paraphrase of this document with our AI Paraphraser
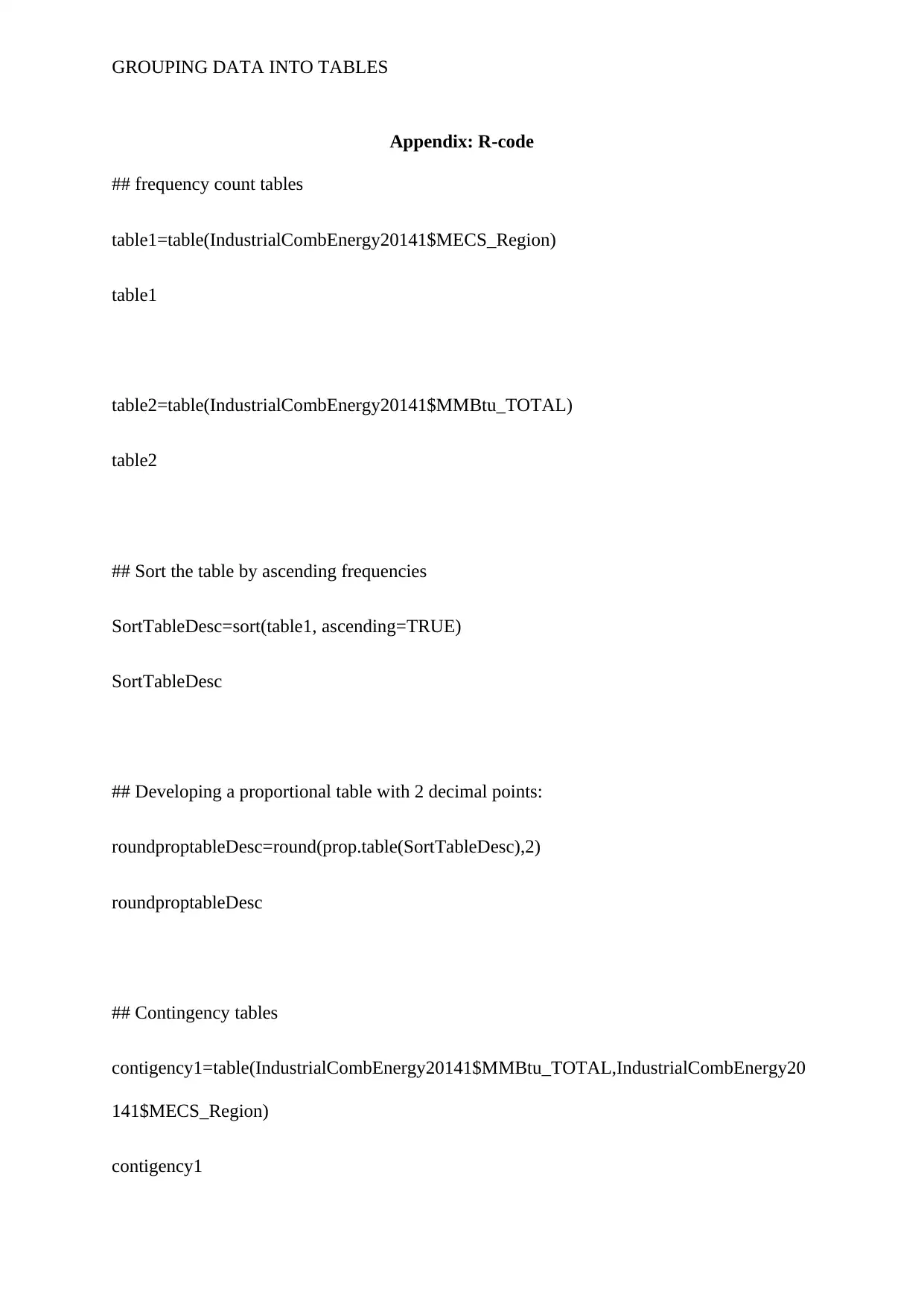
GROUPING DATA INTO TABLES
Appendix: R-code
## frequency count tables
table1=table(IndustrialCombEnergy20141$MECS_Region)
table1
table2=table(IndustrialCombEnergy20141$MMBtu_TOTAL)
table2
## Sort the table by ascending frequencies
SortTableDesc=sort(table1, ascending=TRUE)
SortTableDesc
## Developing a proportional table with 2 decimal points:
roundproptableDesc=round(prop.table(SortTableDesc),2)
roundproptableDesc
## Contingency tables
contigency1=table(IndustrialCombEnergy20141$MMBtu_TOTAL,IndustrialCombEnergy20
141$MECS_Region)
contigency1
Appendix: R-code
## frequency count tables
table1=table(IndustrialCombEnergy20141$MECS_Region)
table1
table2=table(IndustrialCombEnergy20141$MMBtu_TOTAL)
table2
## Sort the table by ascending frequencies
SortTableDesc=sort(table1, ascending=TRUE)
SortTableDesc
## Developing a proportional table with 2 decimal points:
roundproptableDesc=round(prop.table(SortTableDesc),2)
roundproptableDesc
## Contingency tables
contigency1=table(IndustrialCombEnergy20141$MMBtu_TOTAL,IndustrialCombEnergy20
141$MECS_Region)
contigency1
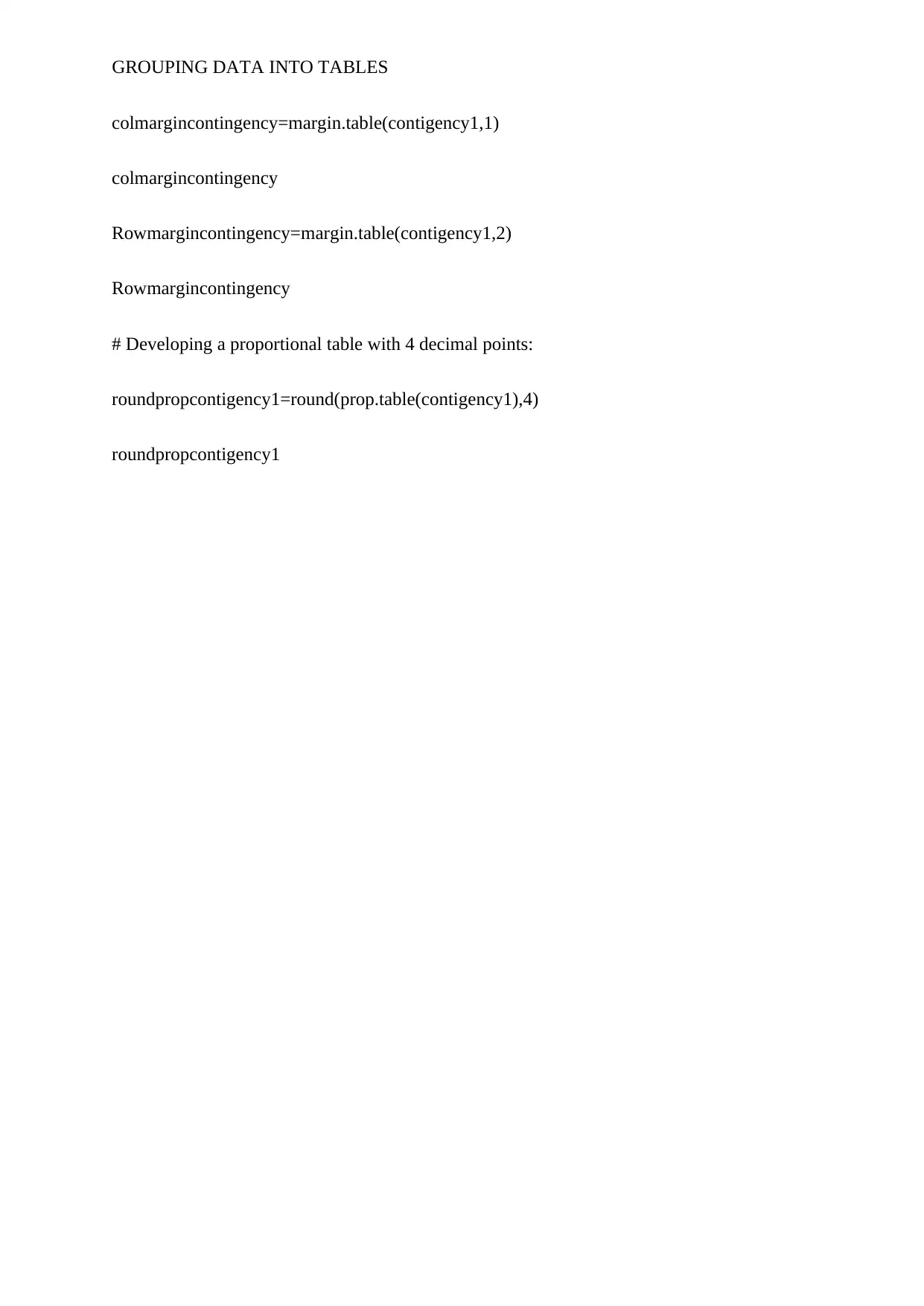
GROUPING DATA INTO TABLES
colmargincontingency=margin.table(contigency1,1)
colmargincontingency
Rowmargincontingency=margin.table(contigency1,2)
Rowmargincontingency
# Developing a proportional table with 4 decimal points:
roundpropcontigency1=round(prop.table(contigency1),4)
roundpropcontigency1
colmargincontingency=margin.table(contigency1,1)
colmargincontingency
Rowmargincontingency=margin.table(contigency1,2)
Rowmargincontingency
# Developing a proportional table with 4 decimal points:
roundpropcontigency1=round(prop.table(contigency1),4)
roundpropcontigency1
1 out of 9
Related Documents
![[object Object]](/_next/image/?url=%2F_next%2Fstatic%2Fmedia%2Flogo.6d15ce61.png&w=640&q=75)
Your All-in-One AI-Powered Toolkit for Academic Success.
+13062052269
info@desklib.com
Available 24*7 on WhatsApp / Email
Unlock your academic potential
© 2024 | Zucol Services PVT LTD | All rights reserved.