Health Response Tweets Analysis for Business Discussion 2022
VerifiedAdded on 2022/10/17
|16
|1560
|14
Presentation
AI Summary
Contribute Materials
Your contribution can guide someone’s learning journey. Share your
documents today.
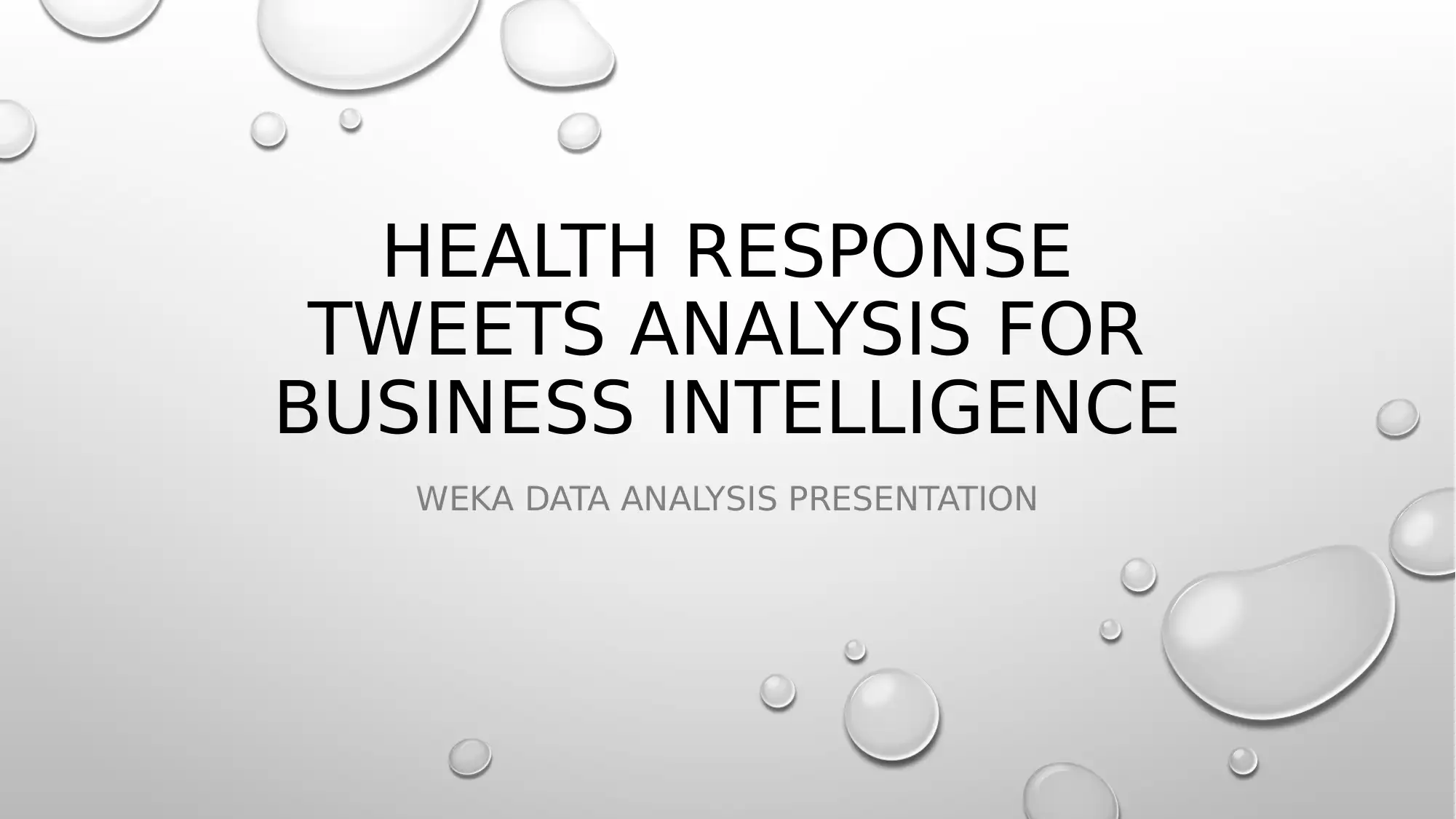
HEALTH RESPONSE
TWEETS ANALYSIS FOR
BUSINESS INTELLIGENCE
WEKA DATA ANALYSIS PRESENTATION
TWEETS ANALYSIS FOR
BUSINESS INTELLIGENCE
WEKA DATA ANALYSIS PRESENTATION
Secure Best Marks with AI Grader
Need help grading? Try our AI Grader for instant feedback on your assignments.
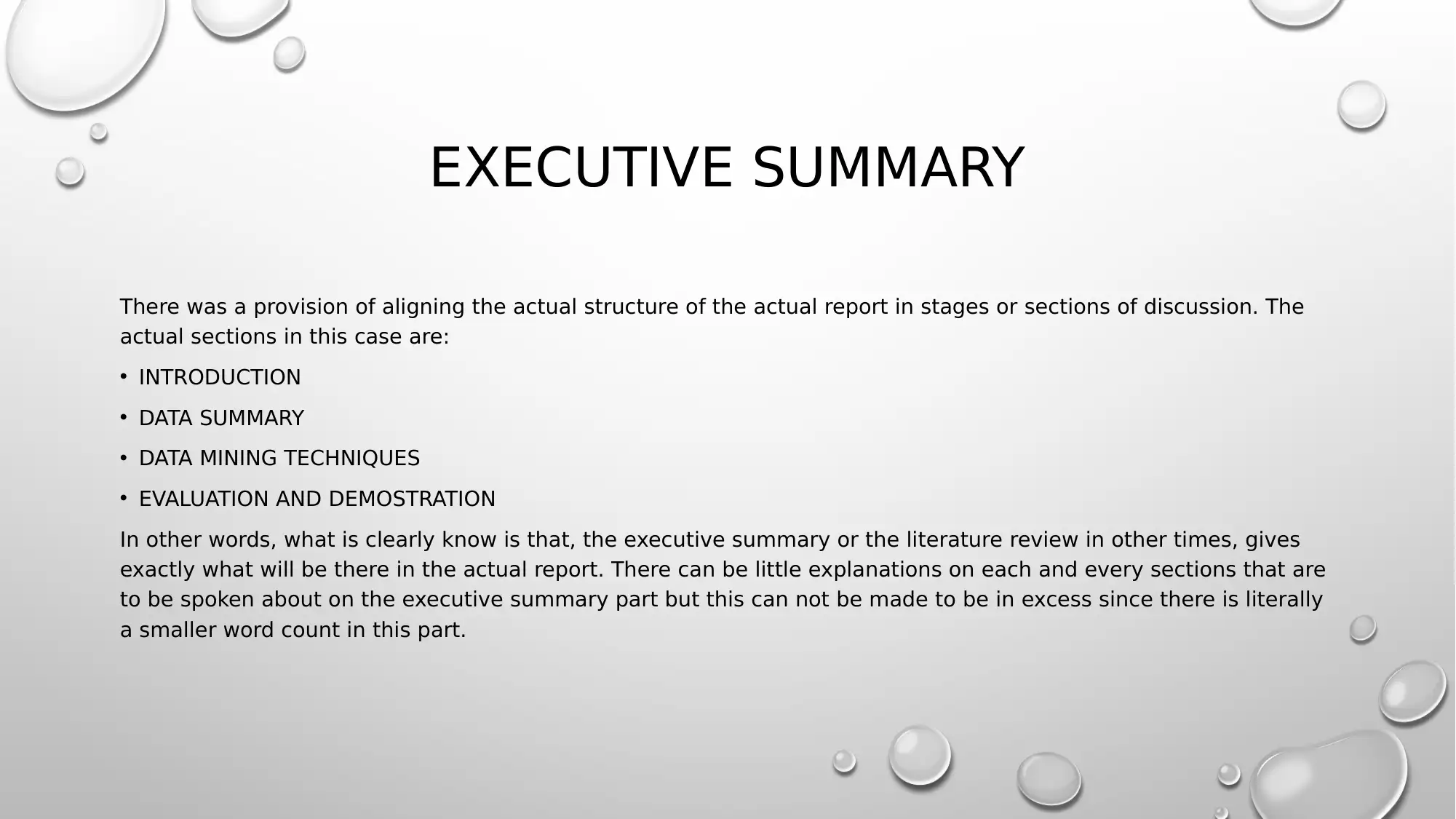
EXECUTIVE SUMMARY
There was a provision of aligning the actual structure of the actual report in stages or sections of discussion. The
actual sections in this case are:
• INTRODUCTION
• DATA SUMMARY
• DATA MINING TECHNIQUES
• EVALUATION AND DEMOSTRATION
In other words, what is clearly know is that, the executive summary or the literature review in other times, gives
exactly what will be there in the actual report. There can be little explanations on each and every sections that are
to be spoken about on the executive summary part but this can not be made to be in excess since there is literally
a smaller word count in this part.
There was a provision of aligning the actual structure of the actual report in stages or sections of discussion. The
actual sections in this case are:
• INTRODUCTION
• DATA SUMMARY
• DATA MINING TECHNIQUES
• EVALUATION AND DEMOSTRATION
In other words, what is clearly know is that, the executive summary or the literature review in other times, gives
exactly what will be there in the actual report. There can be little explanations on each and every sections that are
to be spoken about on the executive summary part but this can not be made to be in excess since there is literally
a smaller word count in this part.
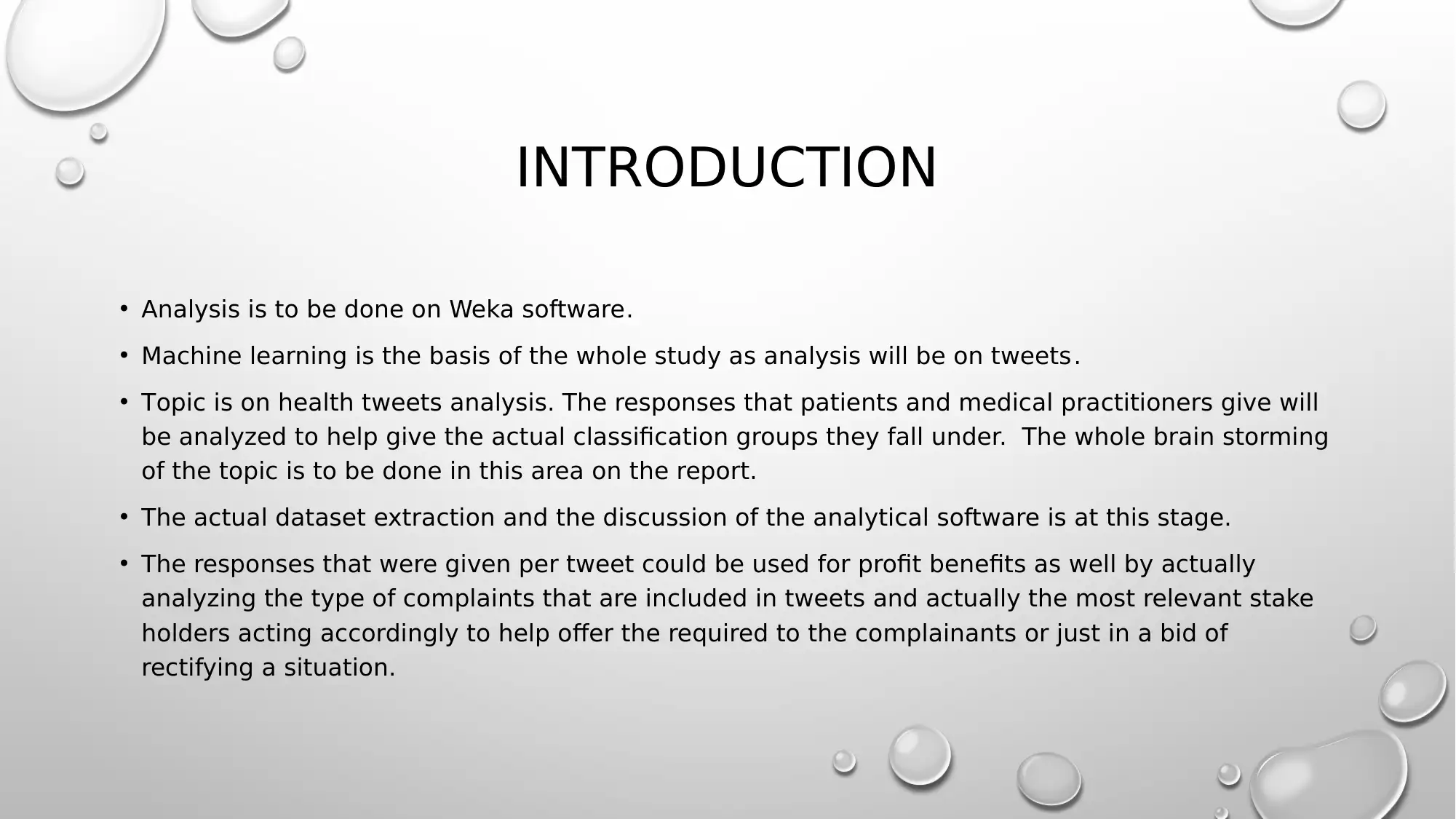
INTRODUCTION
• Analysis is to be done on Weka software.
• Machine learning is the basis of the whole study as analysis will be on tweets.
• Topic is on health tweets analysis. The responses that patients and medical practitioners give will
be analyzed to help give the actual classification groups they fall under. The whole brain storming
of the topic is to be done in this area on the report.
• The actual dataset extraction and the discussion of the analytical software is at this stage.
• The responses that were given per tweet could be used for profit benefits as well by actually
analyzing the type of complaints that are included in tweets and actually the most relevant stake
holders acting accordingly to help offer the required to the complainants or just in a bid of
rectifying a situation.
• Analysis is to be done on Weka software.
• Machine learning is the basis of the whole study as analysis will be on tweets.
• Topic is on health tweets analysis. The responses that patients and medical practitioners give will
be analyzed to help give the actual classification groups they fall under. The whole brain storming
of the topic is to be done in this area on the report.
• The actual dataset extraction and the discussion of the analytical software is at this stage.
• The responses that were given per tweet could be used for profit benefits as well by actually
analyzing the type of complaints that are included in tweets and actually the most relevant stake
holders acting accordingly to help offer the required to the complainants or just in a bid of
rectifying a situation.
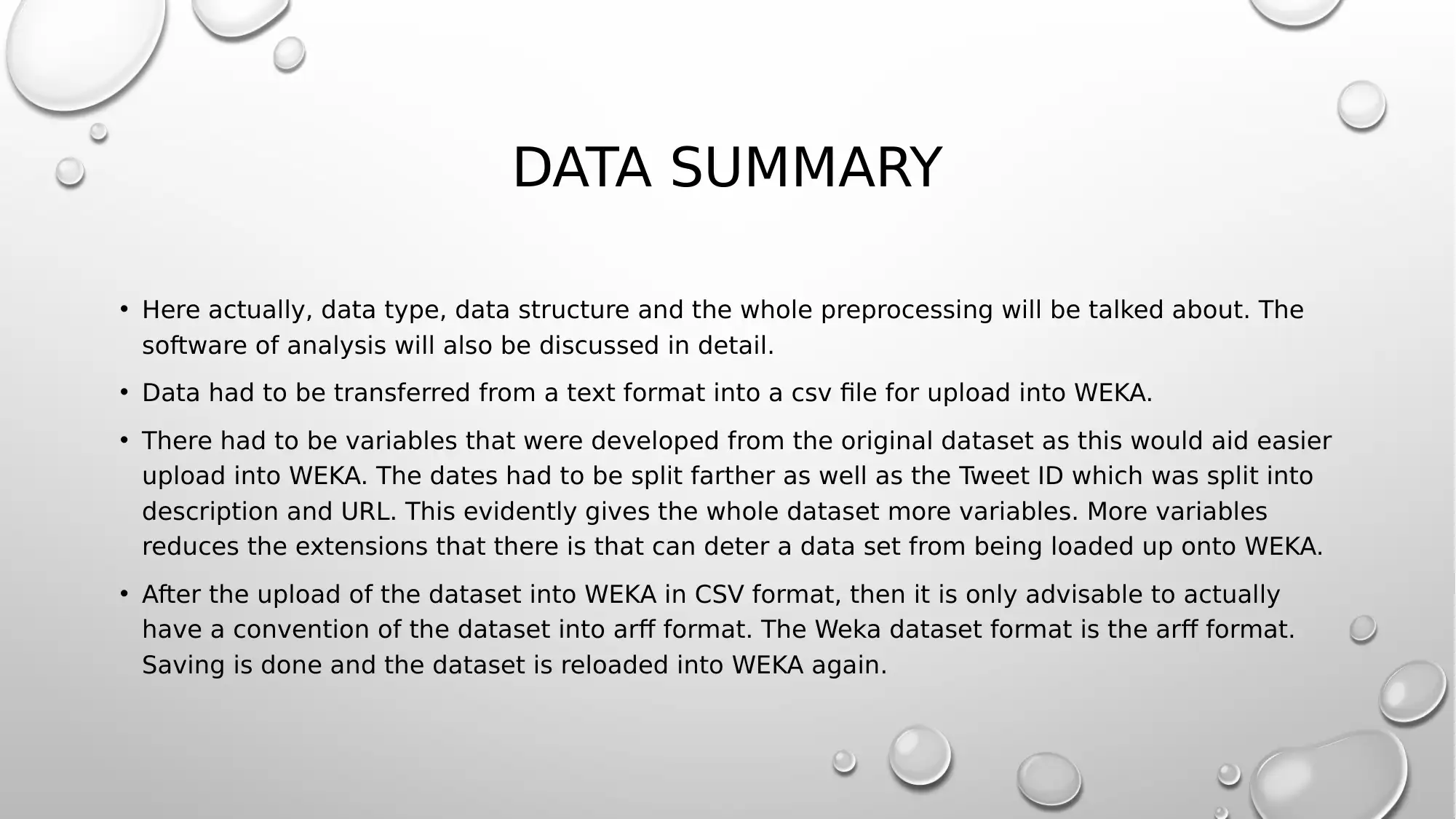
DATA SUMMARY
• Here actually, data type, data structure and the whole preprocessing will be talked about. The
software of analysis will also be discussed in detail.
• Data had to be transferred from a text format into a csv file for upload into WEKA.
• There had to be variables that were developed from the original dataset as this would aid easier
upload into WEKA. The dates had to be split farther as well as the Tweet ID which was split into
description and URL. This evidently gives the whole dataset more variables. More variables
reduces the extensions that there is that can deter a data set from being loaded up onto WEKA.
• After the upload of the dataset into WEKA in CSV format, then it is only advisable to actually
have a convention of the dataset into arff format. The Weka dataset format is the arff format.
Saving is done and the dataset is reloaded into WEKA again.
• Here actually, data type, data structure and the whole preprocessing will be talked about. The
software of analysis will also be discussed in detail.
• Data had to be transferred from a text format into a csv file for upload into WEKA.
• There had to be variables that were developed from the original dataset as this would aid easier
upload into WEKA. The dates had to be split farther as well as the Tweet ID which was split into
description and URL. This evidently gives the whole dataset more variables. More variables
reduces the extensions that there is that can deter a data set from being loaded up onto WEKA.
• After the upload of the dataset into WEKA in CSV format, then it is only advisable to actually
have a convention of the dataset into arff format. The Weka dataset format is the arff format.
Saving is done and the dataset is reloaded into WEKA again.
Secure Best Marks with AI Grader
Need help grading? Try our AI Grader for instant feedback on your assignments.
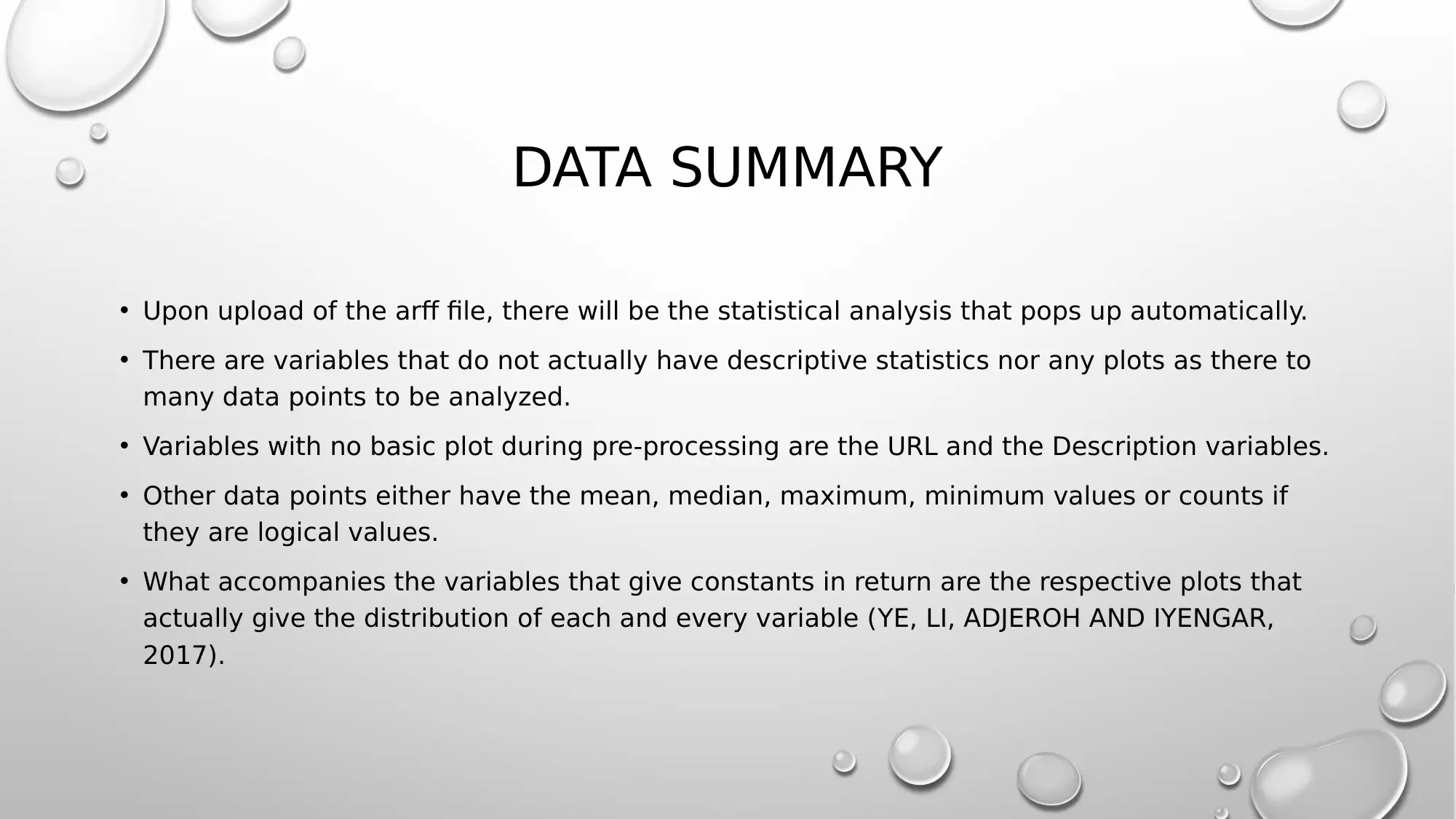
DATA SUMMARY
• Upon upload of the arff file, there will be the statistical analysis that pops up automatically.
• There are variables that do not actually have descriptive statistics nor any plots as there to
many data points to be analyzed.
• Variables with no basic plot during pre-processing are the URL and the Description variables.
• Other data points either have the mean, median, maximum, minimum values or counts if
they are logical values.
• What accompanies the variables that give constants in return are the respective plots that
actually give the distribution of each and every variable (YE, LI, ADJEROH AND IYENGAR,
2017).
• Upon upload of the arff file, there will be the statistical analysis that pops up automatically.
• There are variables that do not actually have descriptive statistics nor any plots as there to
many data points to be analyzed.
• Variables with no basic plot during pre-processing are the URL and the Description variables.
• Other data points either have the mean, median, maximum, minimum values or counts if
they are logical values.
• What accompanies the variables that give constants in return are the respective plots that
actually give the distribution of each and every variable (YE, LI, ADJEROH AND IYENGAR,
2017).
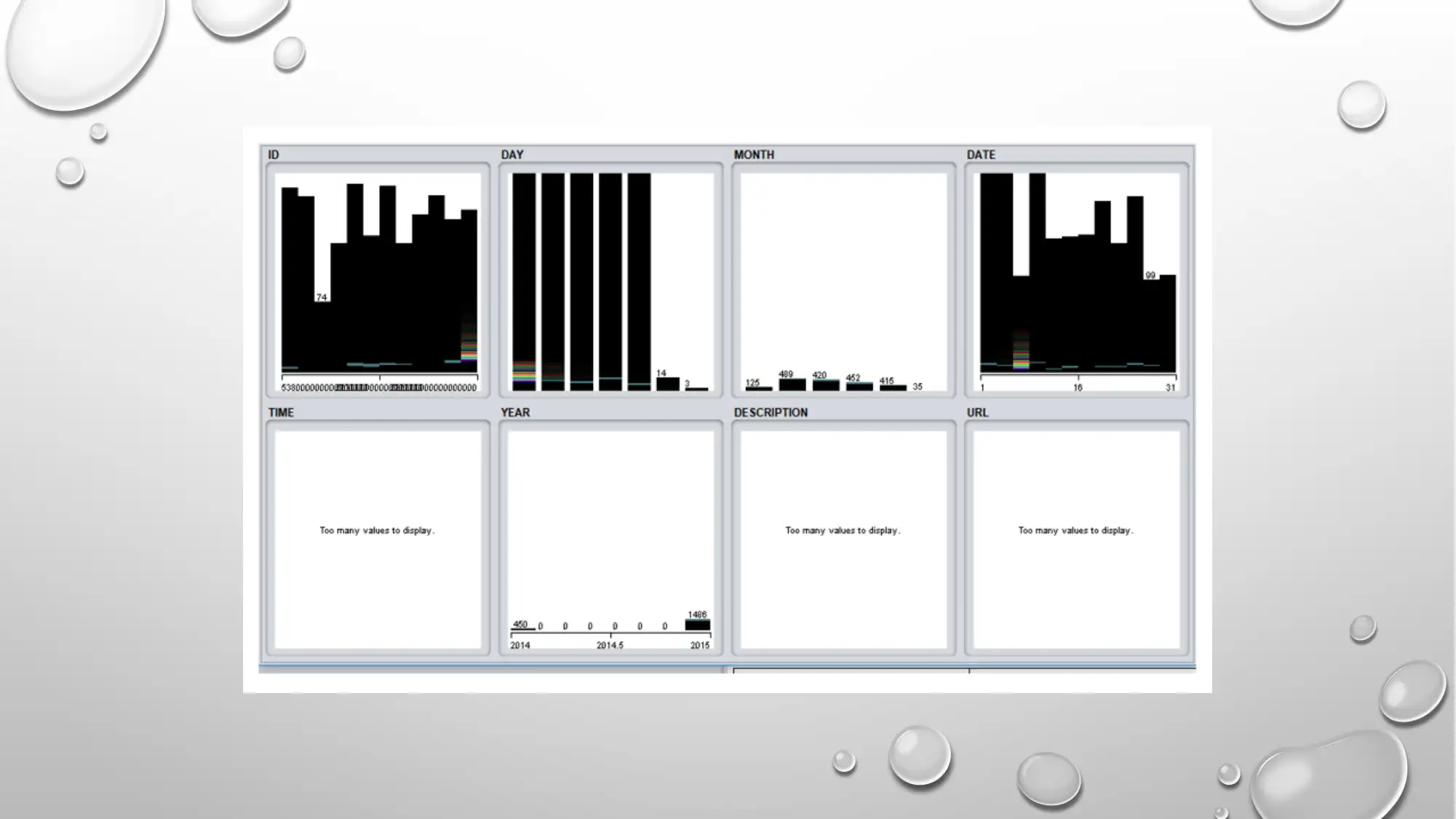
DATA SUMMARY

DATA SUMMARY
• The plot on the previous slide shows how the distributions have been presented.
moving on it is clear that URL, time and descriptions to not have plots cause of very
many data points to be included in analyzing the type of plots to be presented.
• Variables such as ID, Date, Month actually indicate the real distribution of the number
of times an ID, a date, a Month, is considered for tweets data collection.
• The dates, months and days that give higher frequencies actually indicate the fact that
there actually exists those times that individuals tweet a lot.
• The less frequent days have less frequent tweet activities people might be off work or
on holiday duing those days and therefore rarely touch their phones.
• The plot on the previous slide shows how the distributions have been presented.
moving on it is clear that URL, time and descriptions to not have plots cause of very
many data points to be included in analyzing the type of plots to be presented.
• Variables such as ID, Date, Month actually indicate the real distribution of the number
of times an ID, a date, a Month, is considered for tweets data collection.
• The dates, months and days that give higher frequencies actually indicate the fact that
there actually exists those times that individuals tweet a lot.
• The less frequent days have less frequent tweet activities people might be off work or
on holiday duing those days and therefore rarely touch their phones.
Paraphrase This Document
Need a fresh take? Get an instant paraphrase of this document with our AI Paraphraser
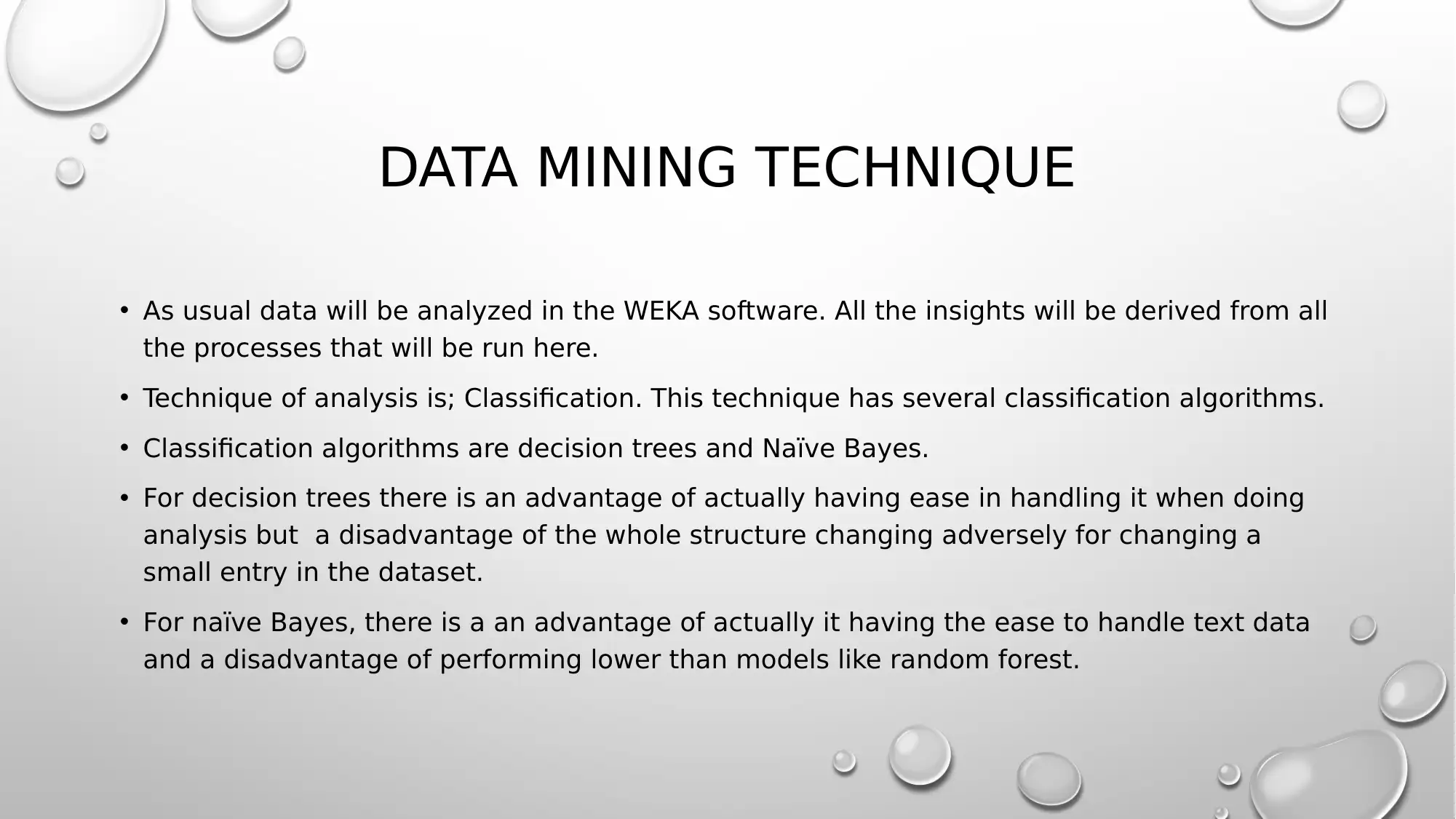
DATA MINING TECHNIQUE
• As usual data will be analyzed in the WEKA software. All the insights will be derived from all
the processes that will be run here.
• Technique of analysis is; Classification. This technique has several classification algorithms.
• Classification algorithms are decision trees and Naïve Bayes.
• For decision trees there is an advantage of actually having ease in handling it when doing
analysis but a disadvantage of the whole structure changing adversely for changing a
small entry in the dataset.
• For naïve Bayes, there is a an advantage of actually it having the ease to handle text data
and a disadvantage of performing lower than models like random forest.
• As usual data will be analyzed in the WEKA software. All the insights will be derived from all
the processes that will be run here.
• Technique of analysis is; Classification. This technique has several classification algorithms.
• Classification algorithms are decision trees and Naïve Bayes.
• For decision trees there is an advantage of actually having ease in handling it when doing
analysis but a disadvantage of the whole structure changing adversely for changing a
small entry in the dataset.
• For naïve Bayes, there is a an advantage of actually it having the ease to handle text data
and a disadvantage of performing lower than models like random forest.
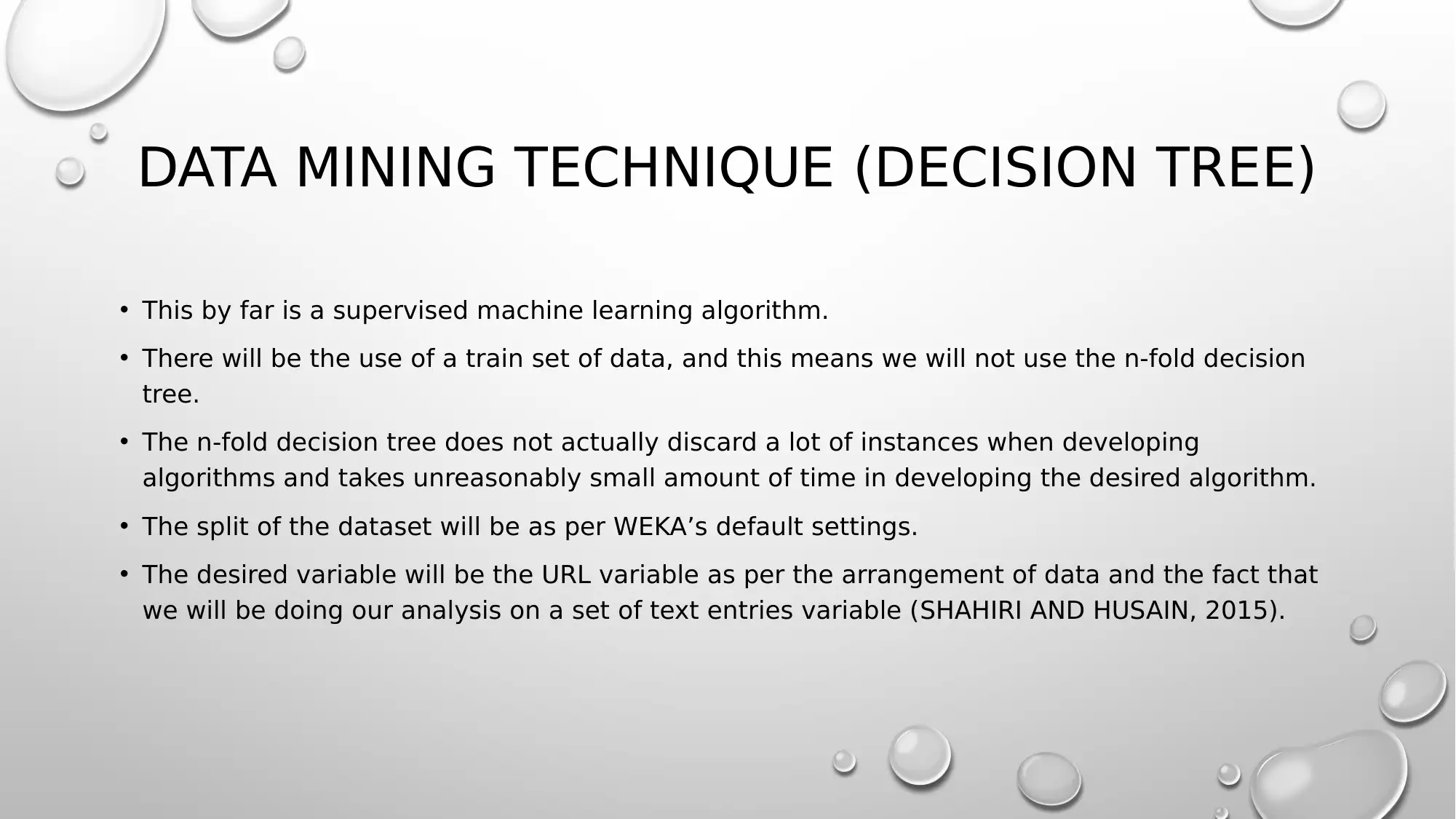
DATA MINING TECHNIQUE (DECISION TREE)
• This by far is a supervised machine learning algorithm.
• There will be the use of a train set of data, and this means we will not use the n-fold decision
tree.
• The n-fold decision tree does not actually discard a lot of instances when developing
algorithms and takes unreasonably small amount of time in developing the desired algorithm.
• The split of the dataset will be as per WEKA’s default settings.
• The desired variable will be the URL variable as per the arrangement of data and the fact that
we will be doing our analysis on a set of text entries variable (SHAHIRI AND HUSAIN, 2015).
• This by far is a supervised machine learning algorithm.
• There will be the use of a train set of data, and this means we will not use the n-fold decision
tree.
• The n-fold decision tree does not actually discard a lot of instances when developing
algorithms and takes unreasonably small amount of time in developing the desired algorithm.
• The split of the dataset will be as per WEKA’s default settings.
• The desired variable will be the URL variable as per the arrangement of data and the fact that
we will be doing our analysis on a set of text entries variable (SHAHIRI AND HUSAIN, 2015).
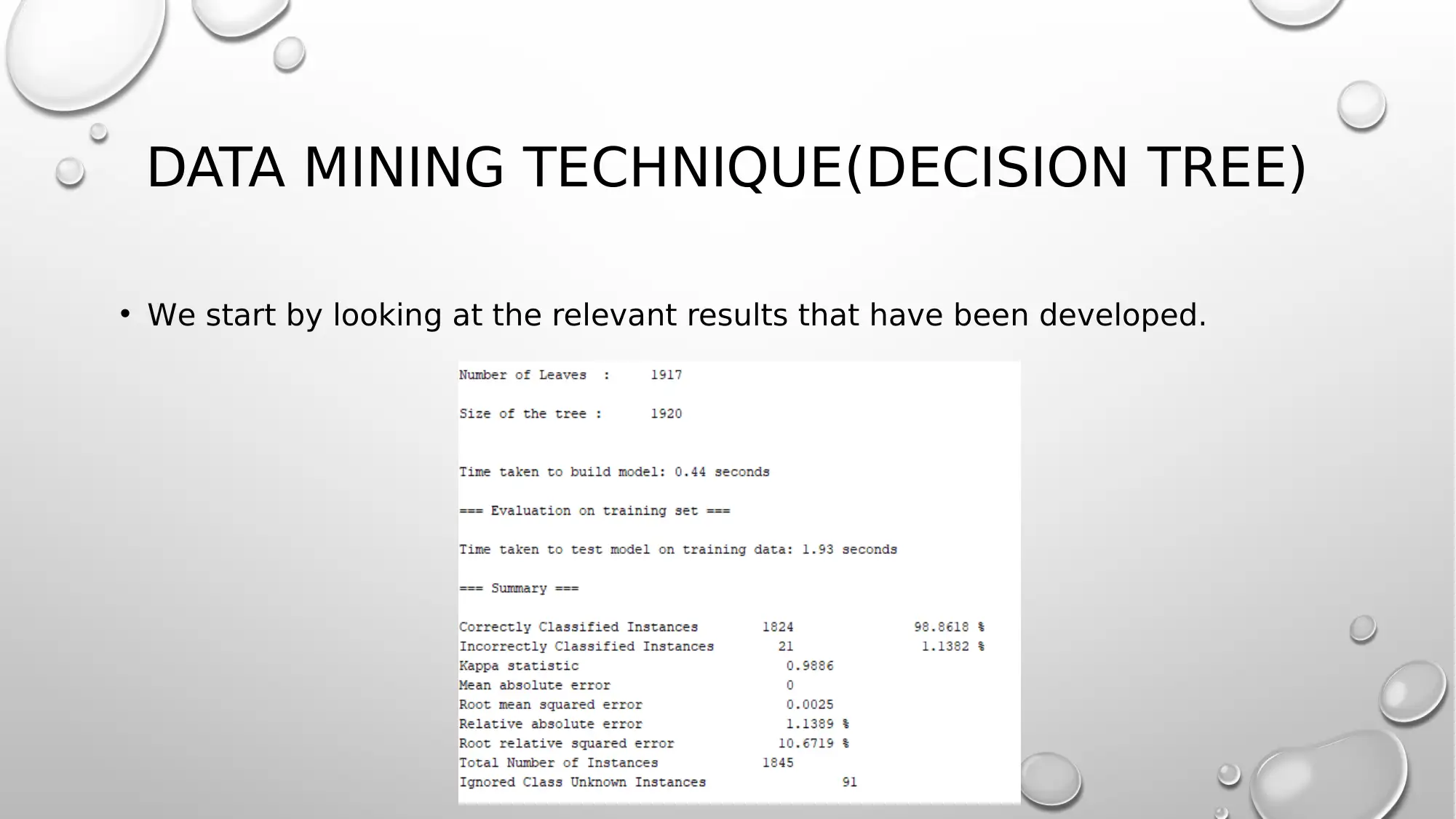
DATA MINING TECHNIQUE(DECISION TREE)
• We start by looking at the relevant results that have been developed.
• We start by looking at the relevant results that have been developed.
Secure Best Marks with AI Grader
Need help grading? Try our AI Grader for instant feedback on your assignments.
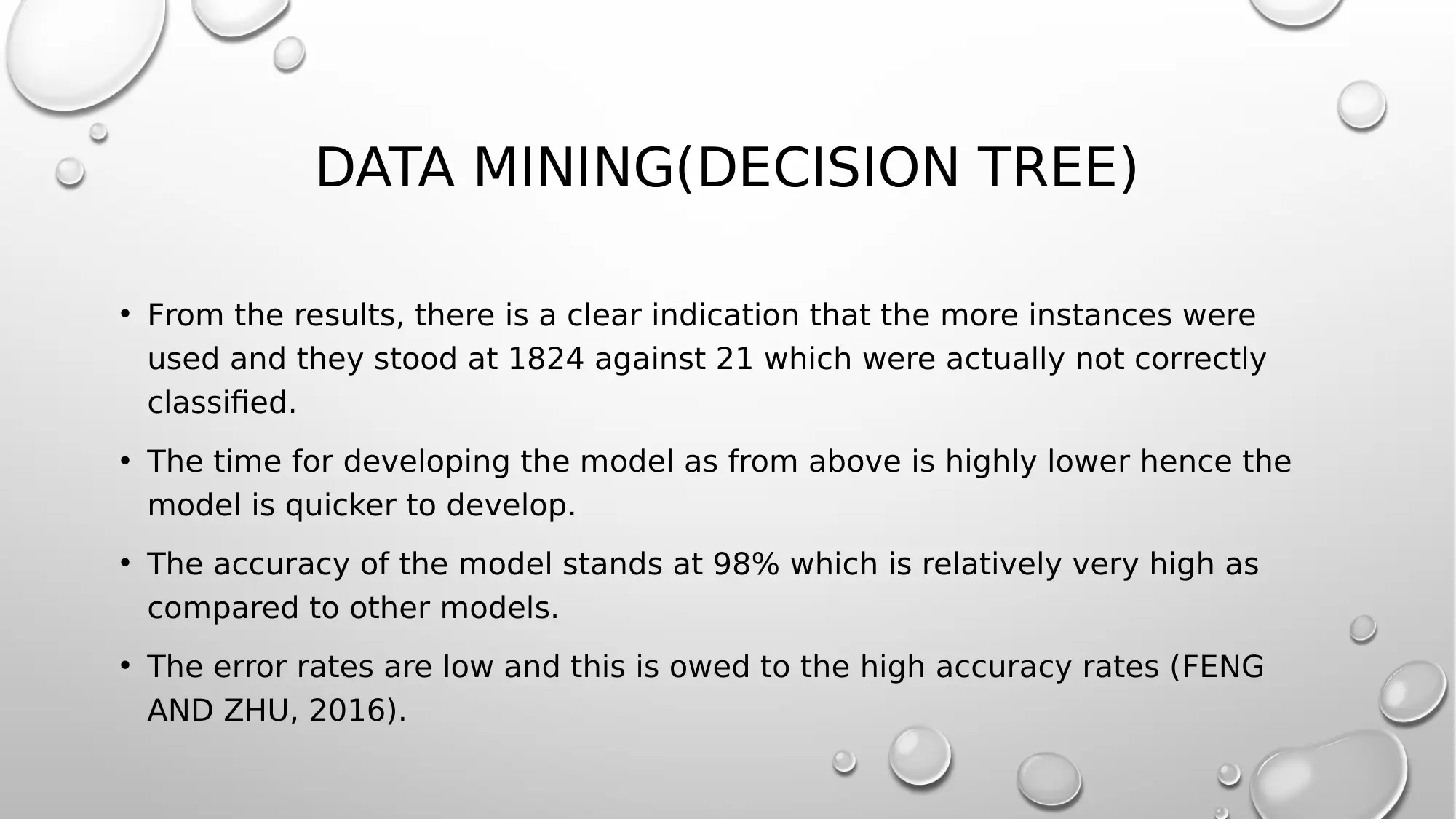
DATA MINING(DECISION TREE)
• From the results, there is a clear indication that the more instances were
used and they stood at 1824 against 21 which were actually not correctly
classified.
• The time for developing the model as from above is highly lower hence the
model is quicker to develop.
• The accuracy of the model stands at 98% which is relatively very high as
compared to other models.
• The error rates are low and this is owed to the high accuracy rates (FENG
AND ZHU, 2016).
• From the results, there is a clear indication that the more instances were
used and they stood at 1824 against 21 which were actually not correctly
classified.
• The time for developing the model as from above is highly lower hence the
model is quicker to develop.
• The accuracy of the model stands at 98% which is relatively very high as
compared to other models.
• The error rates are low and this is owed to the high accuracy rates (FENG
AND ZHU, 2016).

DATA MINING TECHNIQUE(NAÏVE BAYES)
• This will, serve as the counter model in all of the algorithms development.
there will be a need to check the difference between its performance and the
performance of the decision tree algorithm that has been developed so far.
• This will, serve as the counter model in all of the algorithms development.
there will be a need to check the difference between its performance and the
performance of the decision tree algorithm that has been developed so far.

DATA MINING TECHNIQUE(NAÏVE BAYES)
• The timing taken to build the model is actually sorter than the time taken to test the test data using
the same model. This is different because the testing time should be shorter because the fact of test
data being smaller than train data.
• The number of instances that have been discarded during classification are actually is are very high.
• Even if this was to be chosen as a counter model. It still performance poorer than the original model.
• The error values are higher than those of the decision tree model. This is attributed by the high
number of wrongly classified instances.
• This model performs poorly and does not serve as a better counter model and therefore has to be
discarded instade of being chosen (AGRAWAL AND AGRAWAL, 2015).
• The timing taken to build the model is actually sorter than the time taken to test the test data using
the same model. This is different because the testing time should be shorter because the fact of test
data being smaller than train data.
• The number of instances that have been discarded during classification are actually is are very high.
• Even if this was to be chosen as a counter model. It still performance poorer than the original model.
• The error values are higher than those of the decision tree model. This is attributed by the high
number of wrongly classified instances.
• This model performs poorly and does not serve as a better counter model and therefore has to be
discarded instade of being chosen (AGRAWAL AND AGRAWAL, 2015).
Paraphrase This Document
Need a fresh take? Get an instant paraphrase of this document with our AI Paraphraser
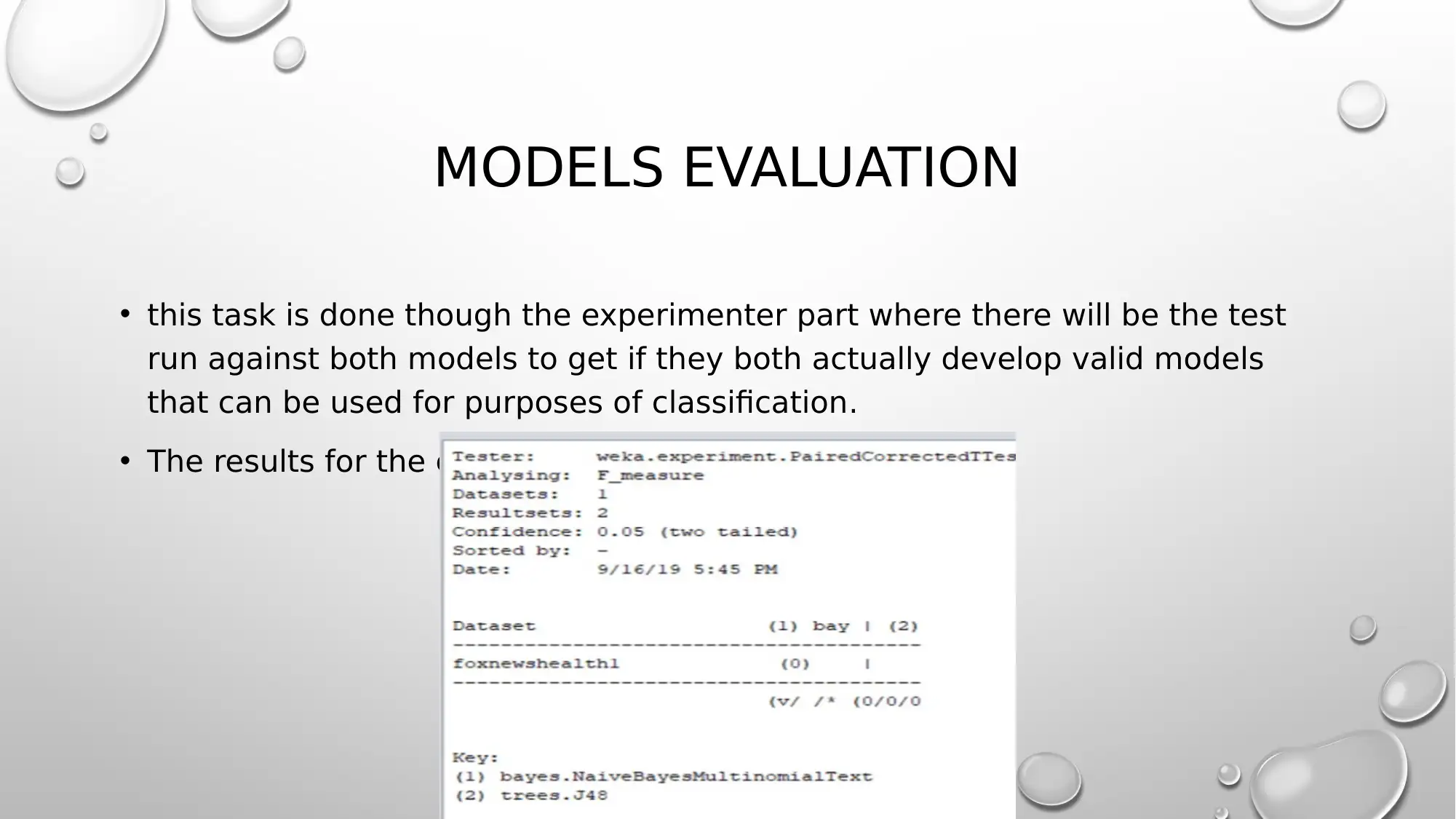
MODELS EVALUATION
• this task is done though the experimenter part where there will be the test
run against both models to get if they both actually develop valid models
that can be used for purposes of classification.
• The results for the evaluation will be as below.;
• this task is done though the experimenter part where there will be the test
run against both models to get if they both actually develop valid models
that can be used for purposes of classification.
• The results for the evaluation will be as below.;
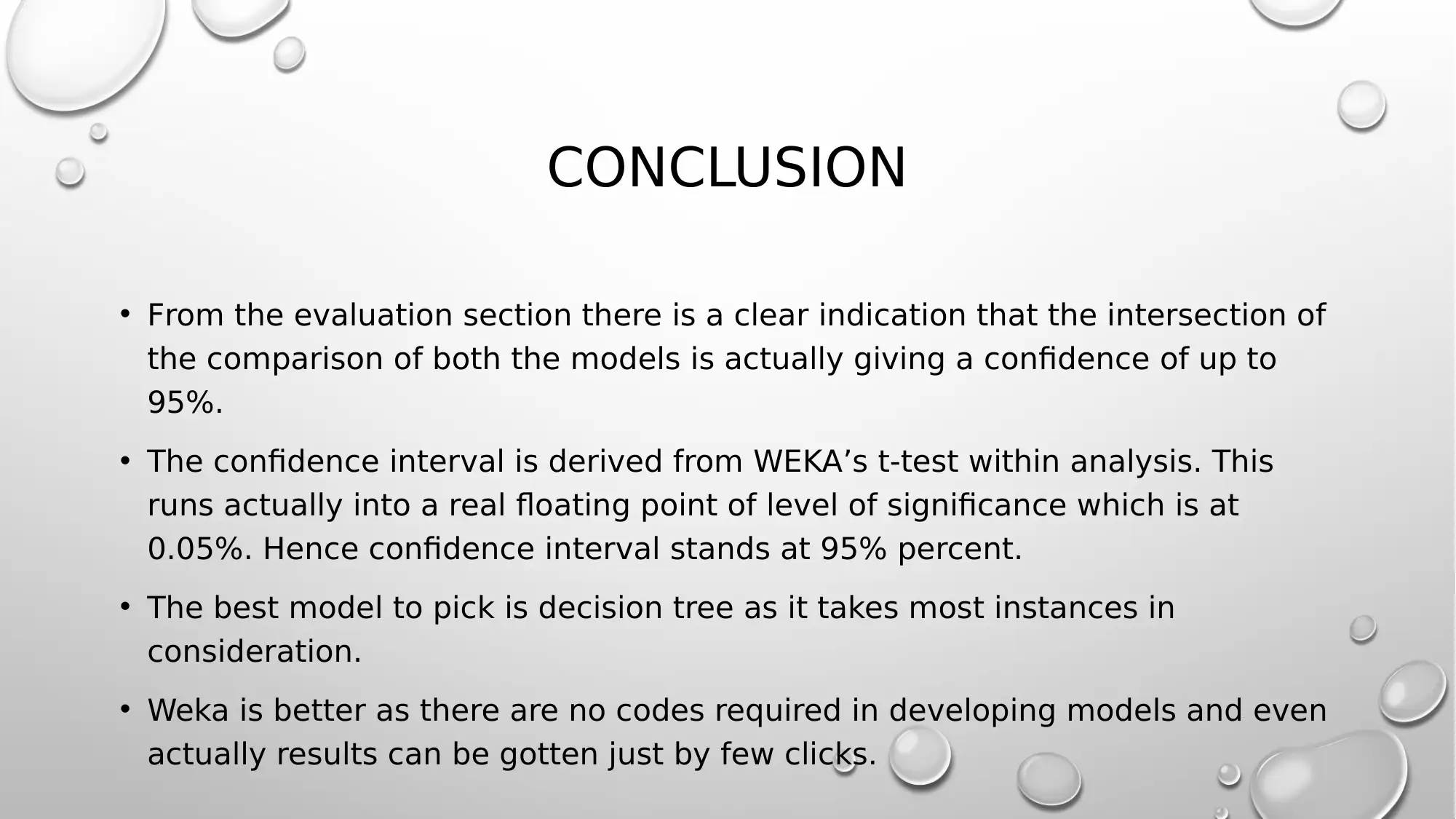
CONCLUSION
• From the evaluation section there is a clear indication that the intersection of
the comparison of both the models is actually giving a confidence of up to
95%.
• The confidence interval is derived from WEKA’s t-test within analysis. This
runs actually into a real floating point of level of significance which is at
0.05%. Hence confidence interval stands at 95% percent.
• The best model to pick is decision tree as it takes most instances in
consideration.
• Weka is better as there are no codes required in developing models and even
actually results can be gotten just by few clicks.
• From the evaluation section there is a clear indication that the intersection of
the comparison of both the models is actually giving a confidence of up to
95%.
• The confidence interval is derived from WEKA’s t-test within analysis. This
runs actually into a real floating point of level of significance which is at
0.05%. Hence confidence interval stands at 95% percent.
• The best model to pick is decision tree as it takes most instances in
consideration.
• Weka is better as there are no codes required in developing models and even
actually results can be gotten just by few clicks.
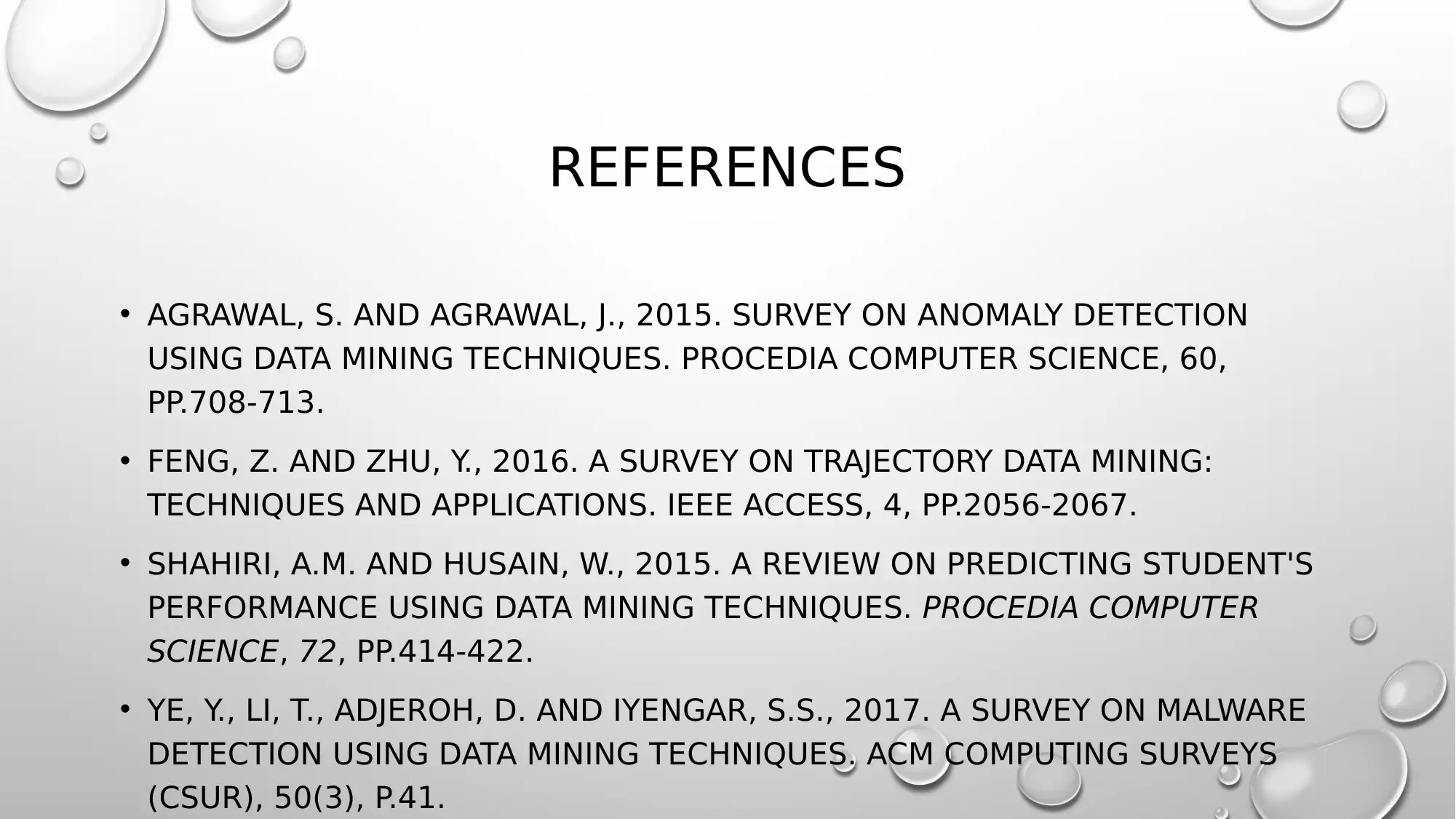
REFERENCES
• AGRAWAL, S. AND AGRAWAL, J., 2015. SURVEY ON ANOMALY DETECTION
USING DATA MINING TECHNIQUES. PROCEDIA COMPUTER SCIENCE, 60,
PP.708-713.
• FENG, Z. AND ZHU, Y., 2016. A SURVEY ON TRAJECTORY DATA MINING:
TECHNIQUES AND APPLICATIONS. IEEE ACCESS, 4, PP.2056-2067.
• SHAHIRI, A.M. AND HUSAIN, W., 2015. A REVIEW ON PREDICTING STUDENT'S
PERFORMANCE USING DATA MINING TECHNIQUES. PROCEDIA COMPUTER
SCIENCE, 72, PP.414-422.
• YE, Y., LI, T., ADJEROH, D. AND IYENGAR, S.S., 2017. A SURVEY ON MALWARE
DETECTION USING DATA MINING TECHNIQUES. ACM COMPUTING SURVEYS
(CSUR), 50(3), P.41.
• AGRAWAL, S. AND AGRAWAL, J., 2015. SURVEY ON ANOMALY DETECTION
USING DATA MINING TECHNIQUES. PROCEDIA COMPUTER SCIENCE, 60,
PP.708-713.
• FENG, Z. AND ZHU, Y., 2016. A SURVEY ON TRAJECTORY DATA MINING:
TECHNIQUES AND APPLICATIONS. IEEE ACCESS, 4, PP.2056-2067.
• SHAHIRI, A.M. AND HUSAIN, W., 2015. A REVIEW ON PREDICTING STUDENT'S
PERFORMANCE USING DATA MINING TECHNIQUES. PROCEDIA COMPUTER
SCIENCE, 72, PP.414-422.
• YE, Y., LI, T., ADJEROH, D. AND IYENGAR, S.S., 2017. A SURVEY ON MALWARE
DETECTION USING DATA MINING TECHNIQUES. ACM COMPUTING SURVEYS
(CSUR), 50(3), P.41.
1 out of 16
![[object Object]](/_next/image/?url=%2F_next%2Fstatic%2Fmedia%2Flogo.6d15ce61.png&w=640&q=75)
Your All-in-One AI-Powered Toolkit for Academic Success.
+13062052269
info@desklib.com
Available 24*7 on WhatsApp / Email
Unlock your academic potential
© 2024 | Zucol Services PVT LTD | All rights reserved.