Enhancing Healthcare System with Artificial Intelligence-based EHR
VerifiedAdded on 2023/04/25
|8
|1756
|352
AI Summary
This study focuses on providing an enhancing software technology that will be improving the existing healthcare system. The artificial intelligence-based EHR will have the usual dashboard like interface and will be able to learn and adapt to the different scenarios and will also be able to capitalize on the commonalities in the behavior of the doctor across all the setting.
Contribute Materials
Your contribution can guide someone’s learning journey. Share your
documents today.
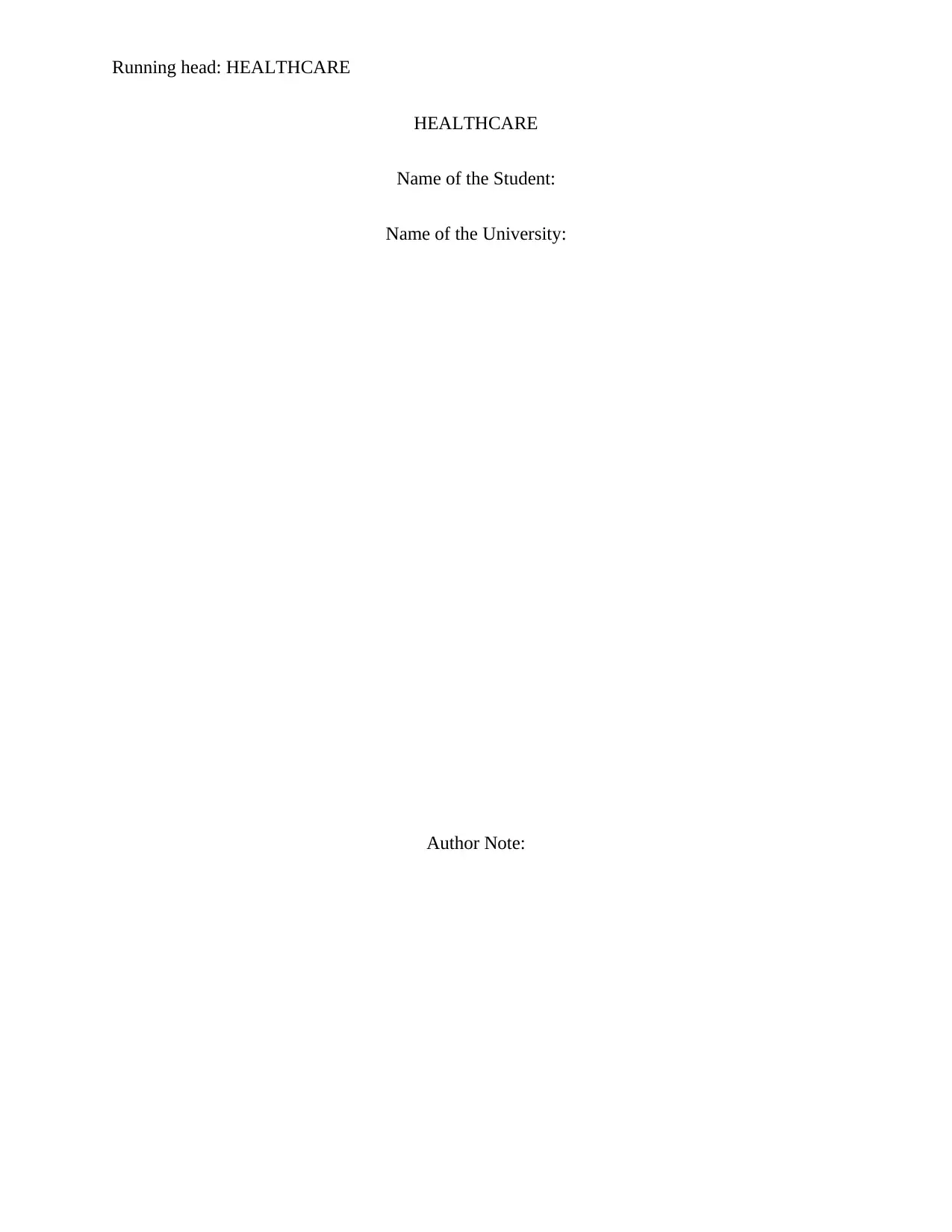
Running head: HEALTHCARE
HEALTHCARE
Name of the Student:
Name of the University:
Author Note:
HEALTHCARE
Name of the Student:
Name of the University:
Author Note:
Secure Best Marks with AI Grader
Need help grading? Try our AI Grader for instant feedback on your assignments.
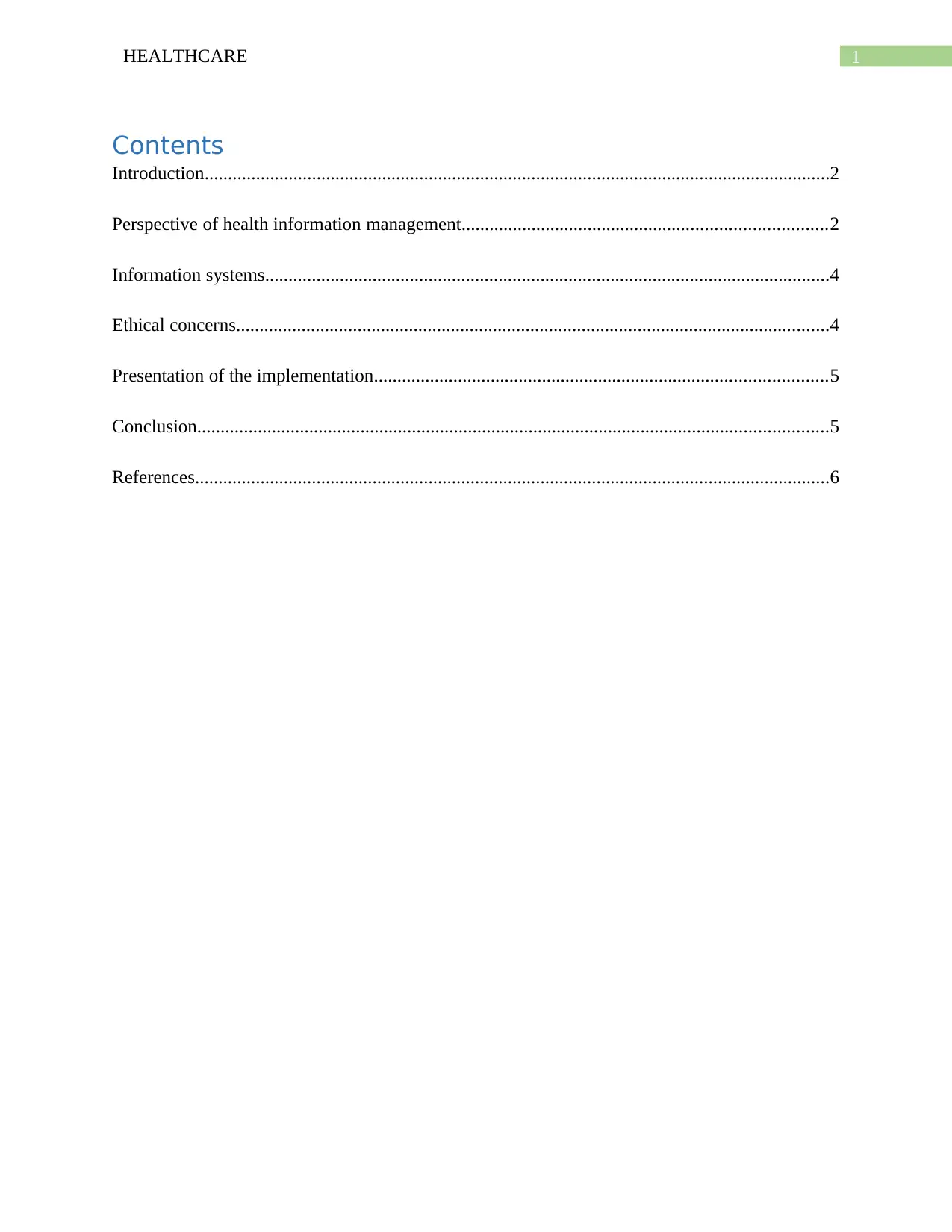
1HEALTHCARE
Contents
Introduction......................................................................................................................................2
Perspective of health information management..............................................................................2
Information systems.........................................................................................................................4
Ethical concerns...............................................................................................................................4
Presentation of the implementation.................................................................................................5
Conclusion.......................................................................................................................................5
References........................................................................................................................................6
Contents
Introduction......................................................................................................................................2
Perspective of health information management..............................................................................2
Information systems.........................................................................................................................4
Ethical concerns...............................................................................................................................4
Presentation of the implementation.................................................................................................5
Conclusion.......................................................................................................................................5
References........................................................................................................................................6
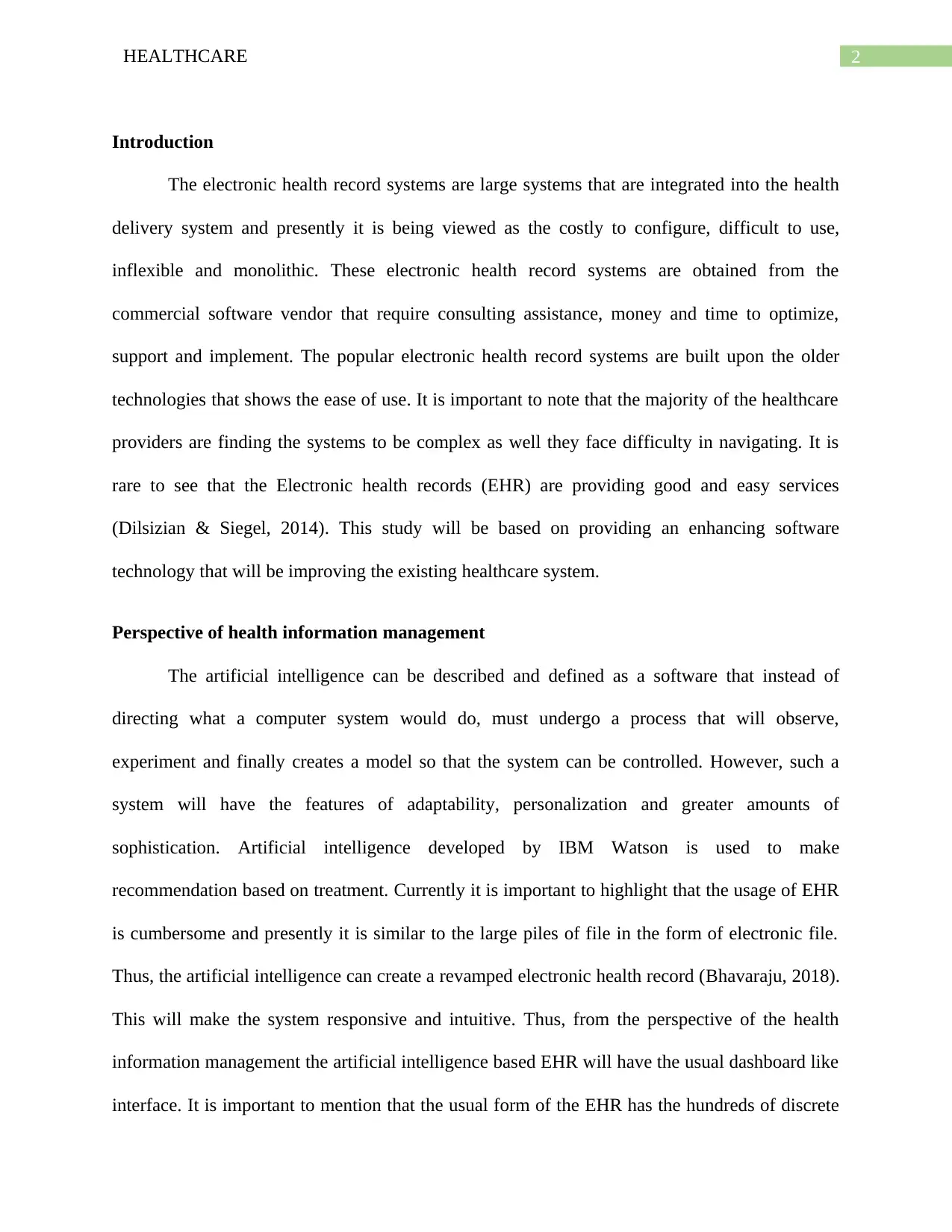
2HEALTHCARE
Introduction
The electronic health record systems are large systems that are integrated into the health
delivery system and presently it is being viewed as the costly to configure, difficult to use,
inflexible and monolithic. These electronic health record systems are obtained from the
commercial software vendor that require consulting assistance, money and time to optimize,
support and implement. The popular electronic health record systems are built upon the older
technologies that shows the ease of use. It is important to note that the majority of the healthcare
providers are finding the systems to be complex as well they face difficulty in navigating. It is
rare to see that the Electronic health records (EHR) are providing good and easy services
(Dilsizian & Siegel, 2014). This study will be based on providing an enhancing software
technology that will be improving the existing healthcare system.
Perspective of health information management
The artificial intelligence can be described and defined as a software that instead of
directing what a computer system would do, must undergo a process that will observe,
experiment and finally creates a model so that the system can be controlled. However, such a
system will have the features of adaptability, personalization and greater amounts of
sophistication. Artificial intelligence developed by IBM Watson is used to make
recommendation based on treatment. Currently it is important to highlight that the usage of EHR
is cumbersome and presently it is similar to the large piles of file in the form of electronic file.
Thus, the artificial intelligence can create a revamped electronic health record (Bhavaraju, 2018).
This will make the system responsive and intuitive. Thus, from the perspective of the health
information management the artificial intelligence based EHR will have the usual dashboard like
interface. It is important to mention that the usual form of the EHR has the hundreds of discrete
Introduction
The electronic health record systems are large systems that are integrated into the health
delivery system and presently it is being viewed as the costly to configure, difficult to use,
inflexible and monolithic. These electronic health record systems are obtained from the
commercial software vendor that require consulting assistance, money and time to optimize,
support and implement. The popular electronic health record systems are built upon the older
technologies that shows the ease of use. It is important to note that the majority of the healthcare
providers are finding the systems to be complex as well they face difficulty in navigating. It is
rare to see that the Electronic health records (EHR) are providing good and easy services
(Dilsizian & Siegel, 2014). This study will be based on providing an enhancing software
technology that will be improving the existing healthcare system.
Perspective of health information management
The artificial intelligence can be described and defined as a software that instead of
directing what a computer system would do, must undergo a process that will observe,
experiment and finally creates a model so that the system can be controlled. However, such a
system will have the features of adaptability, personalization and greater amounts of
sophistication. Artificial intelligence developed by IBM Watson is used to make
recommendation based on treatment. Currently it is important to highlight that the usage of EHR
is cumbersome and presently it is similar to the large piles of file in the form of electronic file.
Thus, the artificial intelligence can create a revamped electronic health record (Bhavaraju, 2018).
This will make the system responsive and intuitive. Thus, from the perspective of the health
information management the artificial intelligence based EHR will have the usual dashboard like
interface. It is important to mention that the usual form of the EHR has the hundreds of discrete
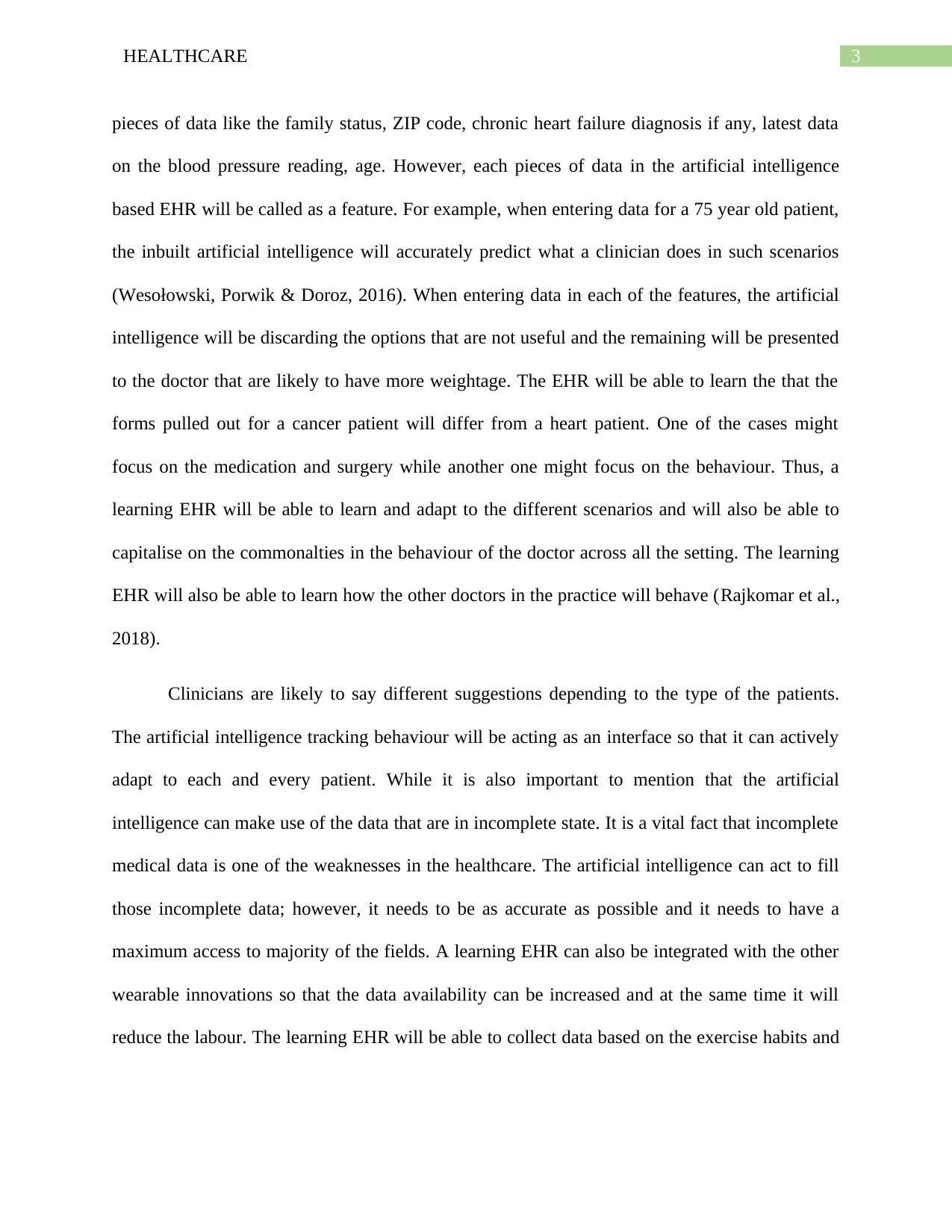
3HEALTHCARE
pieces of data like the family status, ZIP code, chronic heart failure diagnosis if any, latest data
on the blood pressure reading, age. However, each pieces of data in the artificial intelligence
based EHR will be called as a feature. For example, when entering data for a 75 year old patient,
the inbuilt artificial intelligence will accurately predict what a clinician does in such scenarios
(Wesołowski, Porwik & Doroz, 2016). When entering data in each of the features, the artificial
intelligence will be discarding the options that are not useful and the remaining will be presented
to the doctor that are likely to have more weightage. The EHR will be able to learn the that the
forms pulled out for a cancer patient will differ from a heart patient. One of the cases might
focus on the medication and surgery while another one might focus on the behaviour. Thus, a
learning EHR will be able to learn and adapt to the different scenarios and will also be able to
capitalise on the commonalties in the behaviour of the doctor across all the setting. The learning
EHR will also be able to learn how the other doctors in the practice will behave (Rajkomar et al.,
2018).
Clinicians are likely to say different suggestions depending to the type of the patients.
The artificial intelligence tracking behaviour will be acting as an interface so that it can actively
adapt to each and every patient. While it is also important to mention that the artificial
intelligence can make use of the data that are in incomplete state. It is a vital fact that incomplete
medical data is one of the weaknesses in the healthcare. The artificial intelligence can act to fill
those incomplete data; however, it needs to be as accurate as possible and it needs to have a
maximum access to majority of the fields. A learning EHR can also be integrated with the other
wearable innovations so that the data availability can be increased and at the same time it will
reduce the labour. The learning EHR will be able to collect data based on the exercise habits and
pieces of data like the family status, ZIP code, chronic heart failure diagnosis if any, latest data
on the blood pressure reading, age. However, each pieces of data in the artificial intelligence
based EHR will be called as a feature. For example, when entering data for a 75 year old patient,
the inbuilt artificial intelligence will accurately predict what a clinician does in such scenarios
(Wesołowski, Porwik & Doroz, 2016). When entering data in each of the features, the artificial
intelligence will be discarding the options that are not useful and the remaining will be presented
to the doctor that are likely to have more weightage. The EHR will be able to learn the that the
forms pulled out for a cancer patient will differ from a heart patient. One of the cases might
focus on the medication and surgery while another one might focus on the behaviour. Thus, a
learning EHR will be able to learn and adapt to the different scenarios and will also be able to
capitalise on the commonalties in the behaviour of the doctor across all the setting. The learning
EHR will also be able to learn how the other doctors in the practice will behave (Rajkomar et al.,
2018).
Clinicians are likely to say different suggestions depending to the type of the patients.
The artificial intelligence tracking behaviour will be acting as an interface so that it can actively
adapt to each and every patient. While it is also important to mention that the artificial
intelligence can make use of the data that are in incomplete state. It is a vital fact that incomplete
medical data is one of the weaknesses in the healthcare. The artificial intelligence can act to fill
those incomplete data; however, it needs to be as accurate as possible and it needs to have a
maximum access to majority of the fields. A learning EHR can also be integrated with the other
wearable innovations so that the data availability can be increased and at the same time it will
reduce the labour. The learning EHR will be able to collect data based on the exercise habits and
Secure Best Marks with AI Grader
Need help grading? Try our AI Grader for instant feedback on your assignments.
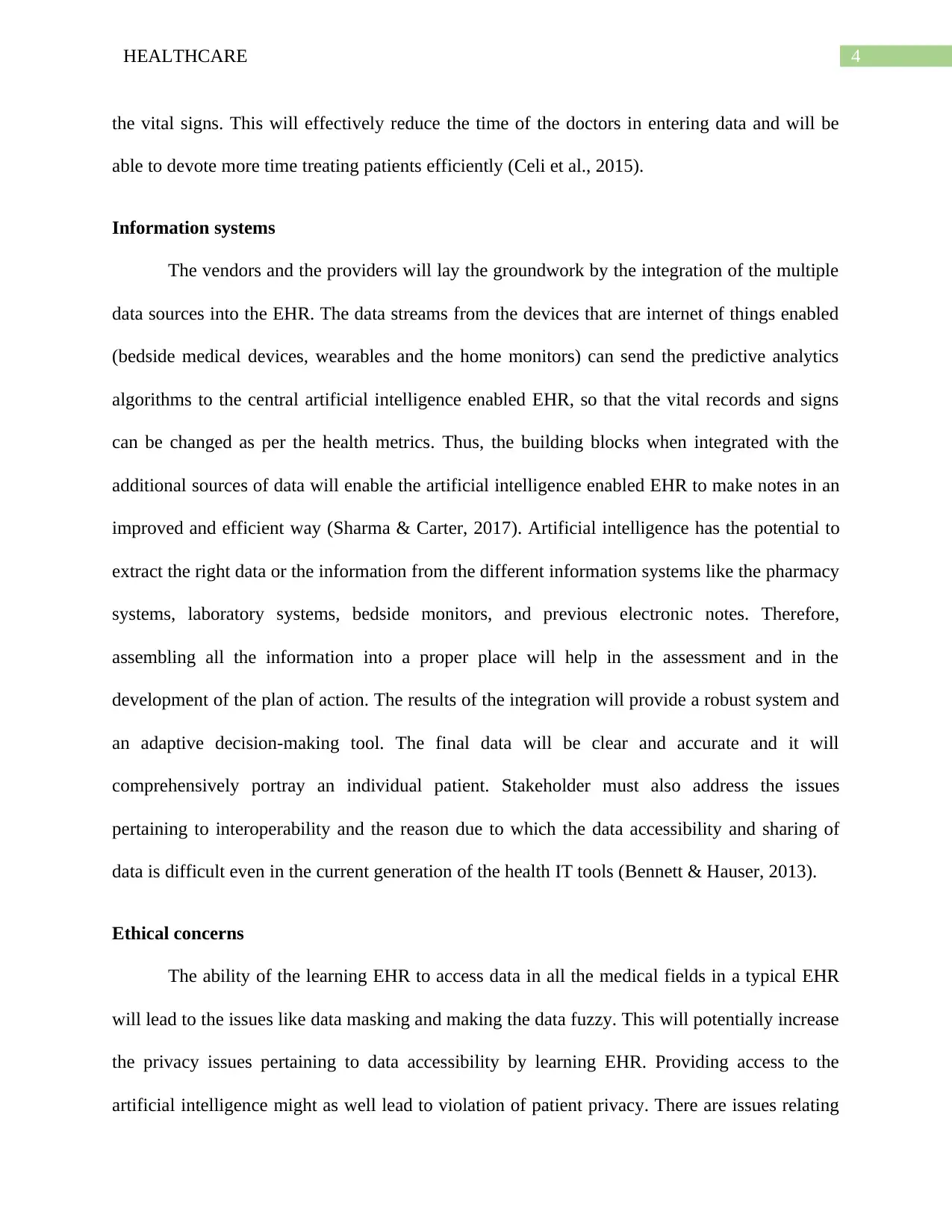
4HEALTHCARE
the vital signs. This will effectively reduce the time of the doctors in entering data and will be
able to devote more time treating patients efficiently (Celi et al., 2015).
Information systems
The vendors and the providers will lay the groundwork by the integration of the multiple
data sources into the EHR. The data streams from the devices that are internet of things enabled
(bedside medical devices, wearables and the home monitors) can send the predictive analytics
algorithms to the central artificial intelligence enabled EHR, so that the vital records and signs
can be changed as per the health metrics. Thus, the building blocks when integrated with the
additional sources of data will enable the artificial intelligence enabled EHR to make notes in an
improved and efficient way (Sharma & Carter, 2017). Artificial intelligence has the potential to
extract the right data or the information from the different information systems like the pharmacy
systems, laboratory systems, bedside monitors, and previous electronic notes. Therefore,
assembling all the information into a proper place will help in the assessment and in the
development of the plan of action. The results of the integration will provide a robust system and
an adaptive decision-making tool. The final data will be clear and accurate and it will
comprehensively portray an individual patient. Stakeholder must also address the issues
pertaining to interoperability and the reason due to which the data accessibility and sharing of
data is difficult even in the current generation of the health IT tools (Bennett & Hauser, 2013).
Ethical concerns
The ability of the learning EHR to access data in all the medical fields in a typical EHR
will lead to the issues like data masking and making the data fuzzy. This will potentially increase
the privacy issues pertaining to data accessibility by learning EHR. Providing access to the
artificial intelligence might as well lead to violation of patient privacy. There are issues relating
the vital signs. This will effectively reduce the time of the doctors in entering data and will be
able to devote more time treating patients efficiently (Celi et al., 2015).
Information systems
The vendors and the providers will lay the groundwork by the integration of the multiple
data sources into the EHR. The data streams from the devices that are internet of things enabled
(bedside medical devices, wearables and the home monitors) can send the predictive analytics
algorithms to the central artificial intelligence enabled EHR, so that the vital records and signs
can be changed as per the health metrics. Thus, the building blocks when integrated with the
additional sources of data will enable the artificial intelligence enabled EHR to make notes in an
improved and efficient way (Sharma & Carter, 2017). Artificial intelligence has the potential to
extract the right data or the information from the different information systems like the pharmacy
systems, laboratory systems, bedside monitors, and previous electronic notes. Therefore,
assembling all the information into a proper place will help in the assessment and in the
development of the plan of action. The results of the integration will provide a robust system and
an adaptive decision-making tool. The final data will be clear and accurate and it will
comprehensively portray an individual patient. Stakeholder must also address the issues
pertaining to interoperability and the reason due to which the data accessibility and sharing of
data is difficult even in the current generation of the health IT tools (Bennett & Hauser, 2013).
Ethical concerns
The ability of the learning EHR to access data in all the medical fields in a typical EHR
will lead to the issues like data masking and making the data fuzzy. This will potentially increase
the privacy issues pertaining to data accessibility by learning EHR. Providing access to the
artificial intelligence might as well lead to violation of patient privacy. There are issues relating
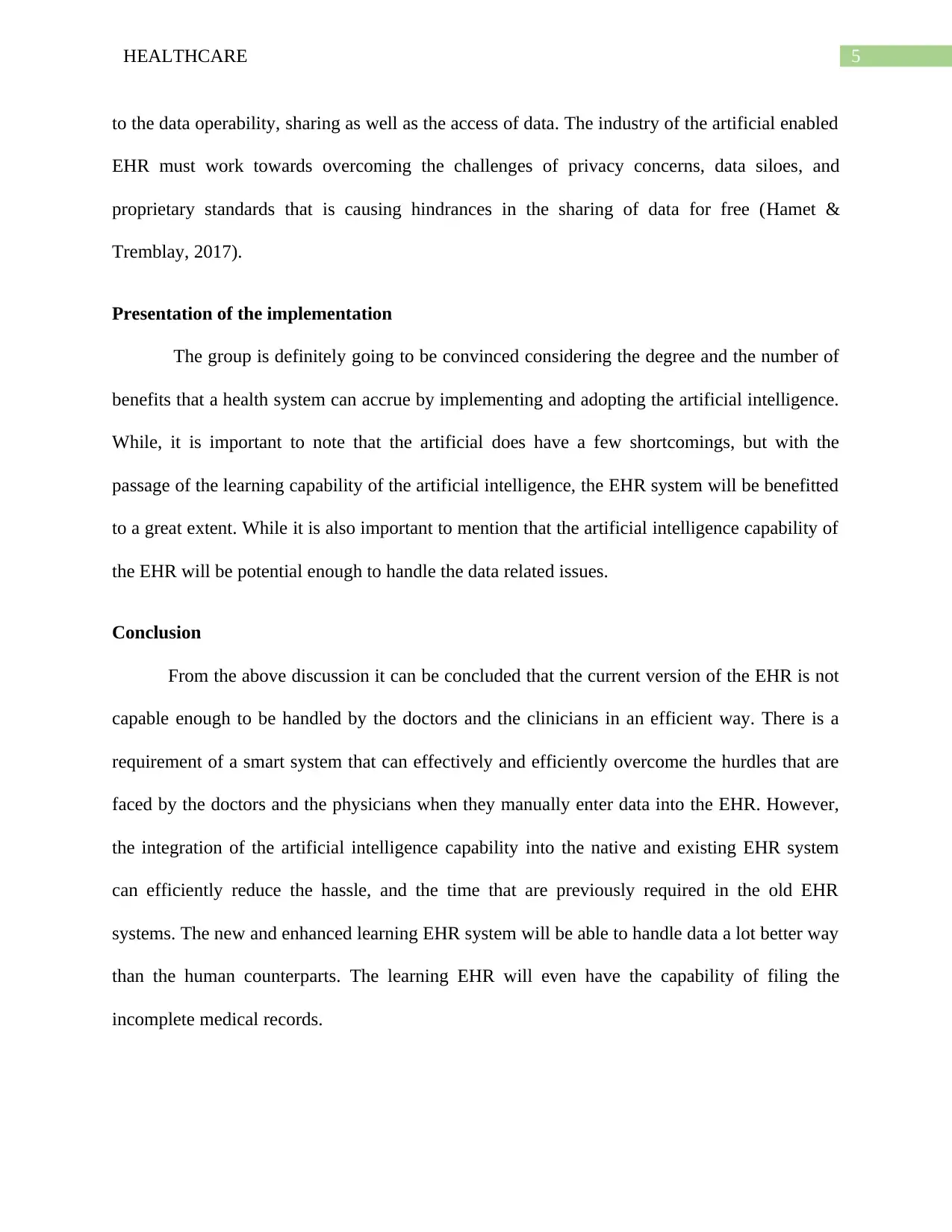
5HEALTHCARE
to the data operability, sharing as well as the access of data. The industry of the artificial enabled
EHR must work towards overcoming the challenges of privacy concerns, data siloes, and
proprietary standards that is causing hindrances in the sharing of data for free (Hamet &
Tremblay, 2017).
Presentation of the implementation
The group is definitely going to be convinced considering the degree and the number of
benefits that a health system can accrue by implementing and adopting the artificial intelligence.
While, it is important to note that the artificial does have a few shortcomings, but with the
passage of the learning capability of the artificial intelligence, the EHR system will be benefitted
to a great extent. While it is also important to mention that the artificial intelligence capability of
the EHR will be potential enough to handle the data related issues.
Conclusion
From the above discussion it can be concluded that the current version of the EHR is not
capable enough to be handled by the doctors and the clinicians in an efficient way. There is a
requirement of a smart system that can effectively and efficiently overcome the hurdles that are
faced by the doctors and the physicians when they manually enter data into the EHR. However,
the integration of the artificial intelligence capability into the native and existing EHR system
can efficiently reduce the hassle, and the time that are previously required in the old EHR
systems. The new and enhanced learning EHR system will be able to handle data a lot better way
than the human counterparts. The learning EHR will even have the capability of filing the
incomplete medical records.
to the data operability, sharing as well as the access of data. The industry of the artificial enabled
EHR must work towards overcoming the challenges of privacy concerns, data siloes, and
proprietary standards that is causing hindrances in the sharing of data for free (Hamet &
Tremblay, 2017).
Presentation of the implementation
The group is definitely going to be convinced considering the degree and the number of
benefits that a health system can accrue by implementing and adopting the artificial intelligence.
While, it is important to note that the artificial does have a few shortcomings, but with the
passage of the learning capability of the artificial intelligence, the EHR system will be benefitted
to a great extent. While it is also important to mention that the artificial intelligence capability of
the EHR will be potential enough to handle the data related issues.
Conclusion
From the above discussion it can be concluded that the current version of the EHR is not
capable enough to be handled by the doctors and the clinicians in an efficient way. There is a
requirement of a smart system that can effectively and efficiently overcome the hurdles that are
faced by the doctors and the physicians when they manually enter data into the EHR. However,
the integration of the artificial intelligence capability into the native and existing EHR system
can efficiently reduce the hassle, and the time that are previously required in the old EHR
systems. The new and enhanced learning EHR system will be able to handle data a lot better way
than the human counterparts. The learning EHR will even have the capability of filing the
incomplete medical records.
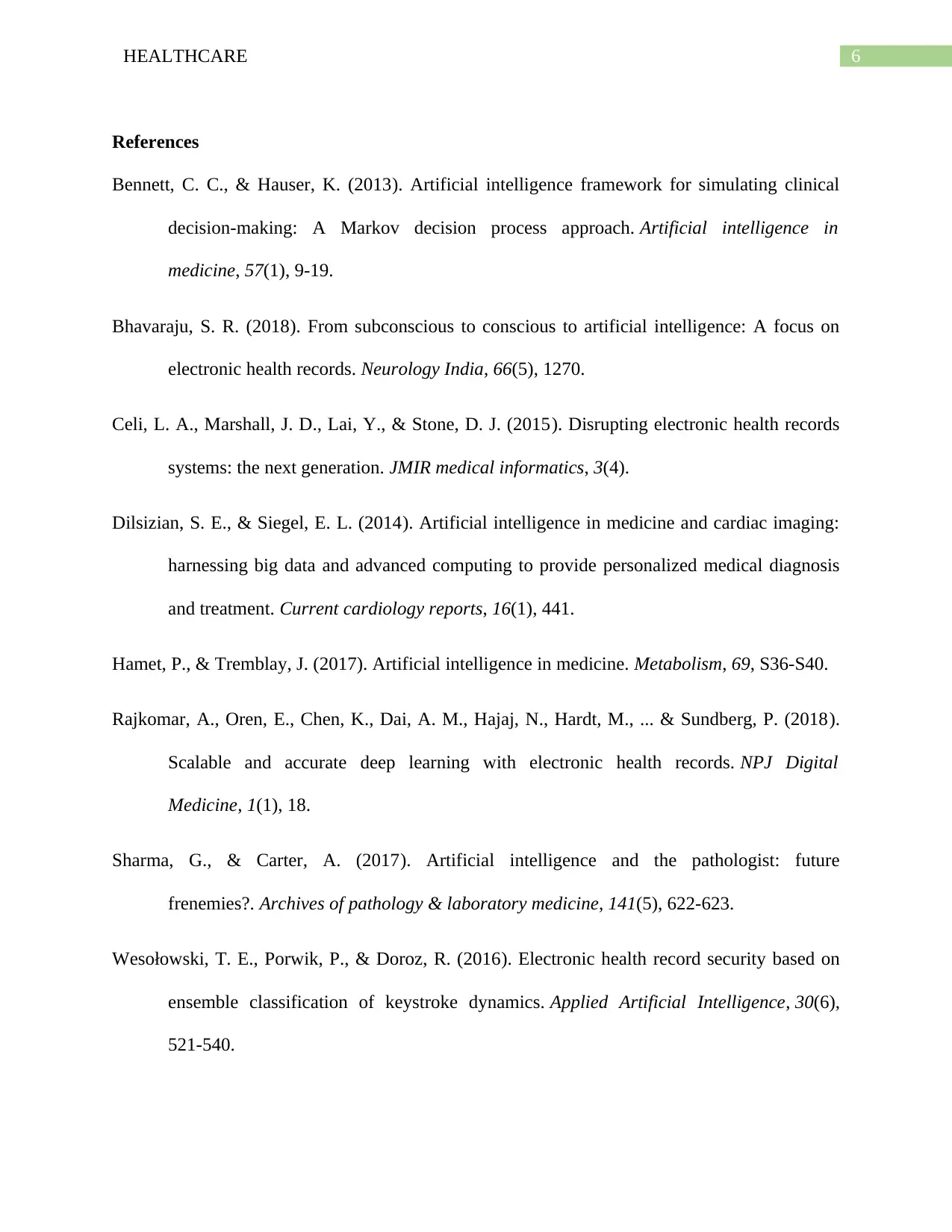
6HEALTHCARE
References
Bennett, C. C., & Hauser, K. (2013). Artificial intelligence framework for simulating clinical
decision-making: A Markov decision process approach. Artificial intelligence in
medicine, 57(1), 9-19.
Bhavaraju, S. R. (2018). From subconscious to conscious to artificial intelligence: A focus on
electronic health records. Neurology India, 66(5), 1270.
Celi, L. A., Marshall, J. D., Lai, Y., & Stone, D. J. (2015). Disrupting electronic health records
systems: the next generation. JMIR medical informatics, 3(4).
Dilsizian, S. E., & Siegel, E. L. (2014). Artificial intelligence in medicine and cardiac imaging:
harnessing big data and advanced computing to provide personalized medical diagnosis
and treatment. Current cardiology reports, 16(1), 441.
Hamet, P., & Tremblay, J. (2017). Artificial intelligence in medicine. Metabolism, 69, S36-S40.
Rajkomar, A., Oren, E., Chen, K., Dai, A. M., Hajaj, N., Hardt, M., ... & Sundberg, P. (2018).
Scalable and accurate deep learning with electronic health records. NPJ Digital
Medicine, 1(1), 18.
Sharma, G., & Carter, A. (2017). Artificial intelligence and the pathologist: future
frenemies?. Archives of pathology & laboratory medicine, 141(5), 622-623.
Wesołowski, T. E., Porwik, P., & Doroz, R. (2016). Electronic health record security based on
ensemble classification of keystroke dynamics. Applied Artificial Intelligence, 30(6),
521-540.
References
Bennett, C. C., & Hauser, K. (2013). Artificial intelligence framework for simulating clinical
decision-making: A Markov decision process approach. Artificial intelligence in
medicine, 57(1), 9-19.
Bhavaraju, S. R. (2018). From subconscious to conscious to artificial intelligence: A focus on
electronic health records. Neurology India, 66(5), 1270.
Celi, L. A., Marshall, J. D., Lai, Y., & Stone, D. J. (2015). Disrupting electronic health records
systems: the next generation. JMIR medical informatics, 3(4).
Dilsizian, S. E., & Siegel, E. L. (2014). Artificial intelligence in medicine and cardiac imaging:
harnessing big data and advanced computing to provide personalized medical diagnosis
and treatment. Current cardiology reports, 16(1), 441.
Hamet, P., & Tremblay, J. (2017). Artificial intelligence in medicine. Metabolism, 69, S36-S40.
Rajkomar, A., Oren, E., Chen, K., Dai, A. M., Hajaj, N., Hardt, M., ... & Sundberg, P. (2018).
Scalable and accurate deep learning with electronic health records. NPJ Digital
Medicine, 1(1), 18.
Sharma, G., & Carter, A. (2017). Artificial intelligence and the pathologist: future
frenemies?. Archives of pathology & laboratory medicine, 141(5), 622-623.
Wesołowski, T. E., Porwik, P., & Doroz, R. (2016). Electronic health record security based on
ensemble classification of keystroke dynamics. Applied Artificial Intelligence, 30(6),
521-540.
Paraphrase This Document
Need a fresh take? Get an instant paraphrase of this document with our AI Paraphraser
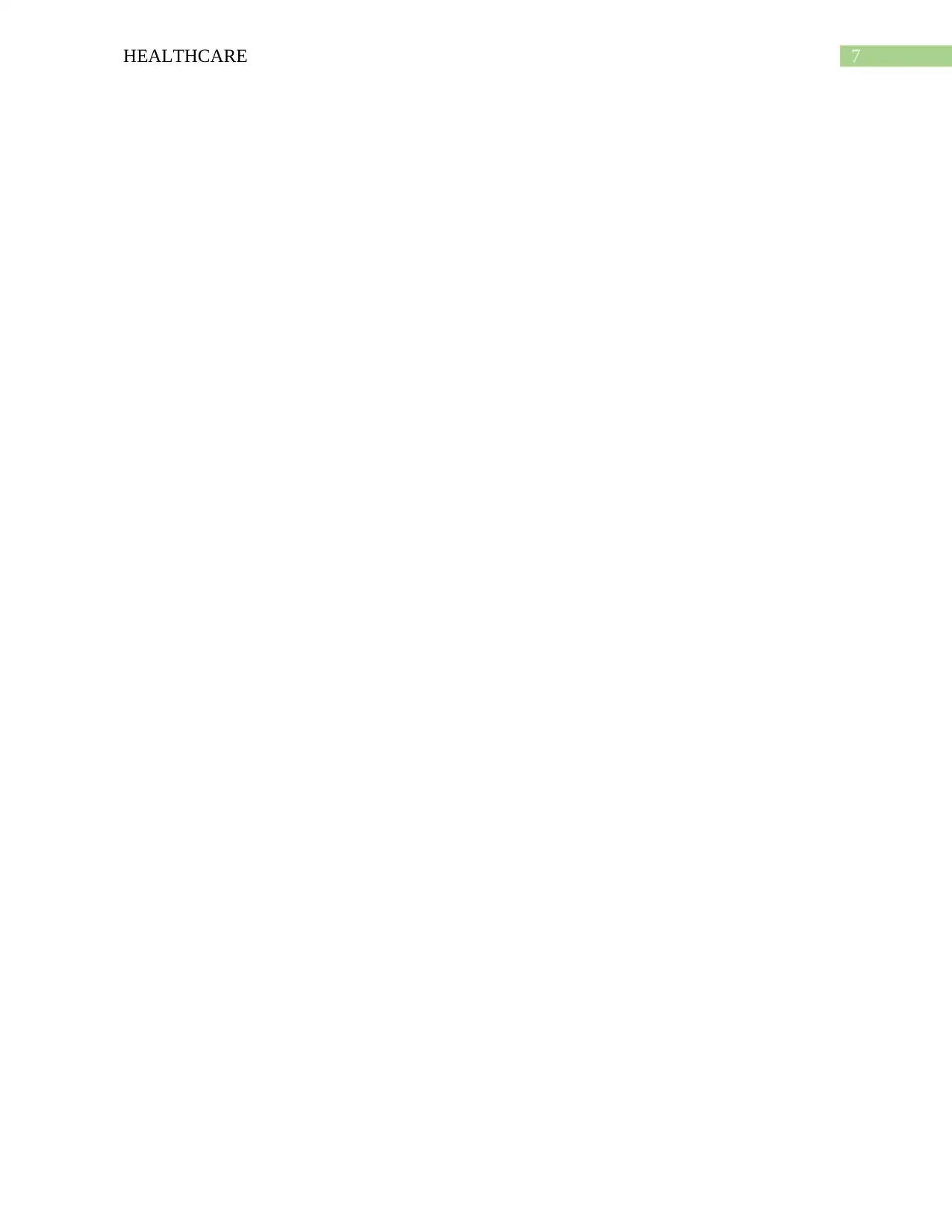
7HEALTHCARE
1 out of 8
Related Documents
![[object Object]](/_next/image/?url=%2F_next%2Fstatic%2Fmedia%2Flogo.6d15ce61.png&w=640&q=75)
Your All-in-One AI-Powered Toolkit for Academic Success.
+13062052269
info@desklib.com
Available 24*7 on WhatsApp / Email
Unlock your academic potential
© 2024 | Zucol Services PVT LTD | All rights reserved.