Human Factors in System Design: Usability Testing of a Complex CDS Tool in an Emergency Department
VerifiedAdded on 2023/04/07
|14
|3285
|152
AI Summary
This paper outlines the usability phase of a study, which will test the impact of integration of the Wells CDSS for pulmonary embolism (PE) diagnosis into a large urban emergency department, where workflow is often chaotic and high stakes decisions are frequently made.
Contribute Materials
Your contribution can guide someone’s learning journey. Share your
documents today.
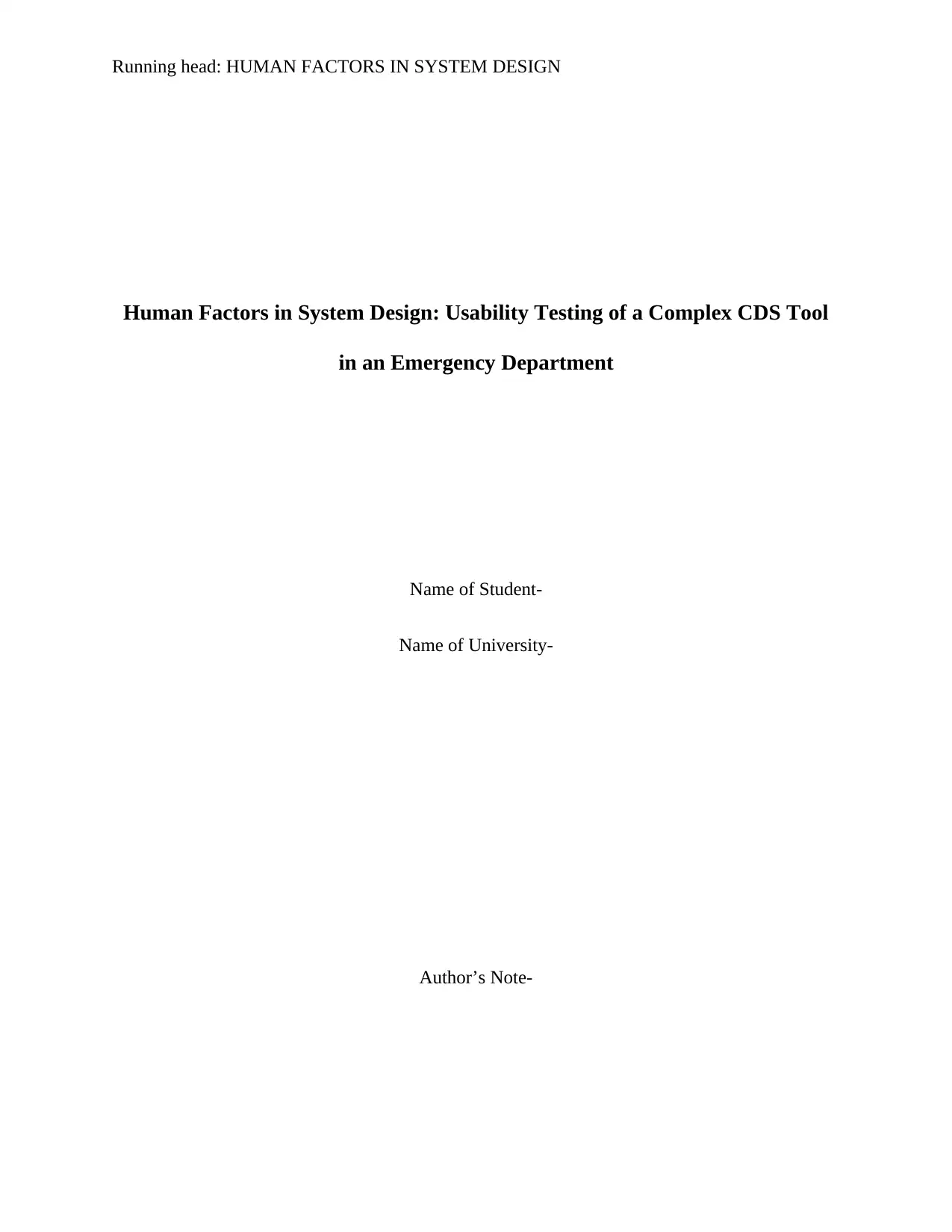
Running head: HUMAN FACTORS IN SYSTEM DESIGN
Human Factors in System Design: Usability Testing of a Complex CDS Tool
in an Emergency Department
Name of Student-
Name of University-
Author’s Note-
Human Factors in System Design: Usability Testing of a Complex CDS Tool
in an Emergency Department
Name of Student-
Name of University-
Author’s Note-
Secure Best Marks with AI Grader
Need help grading? Try our AI Grader for instant feedback on your assignments.
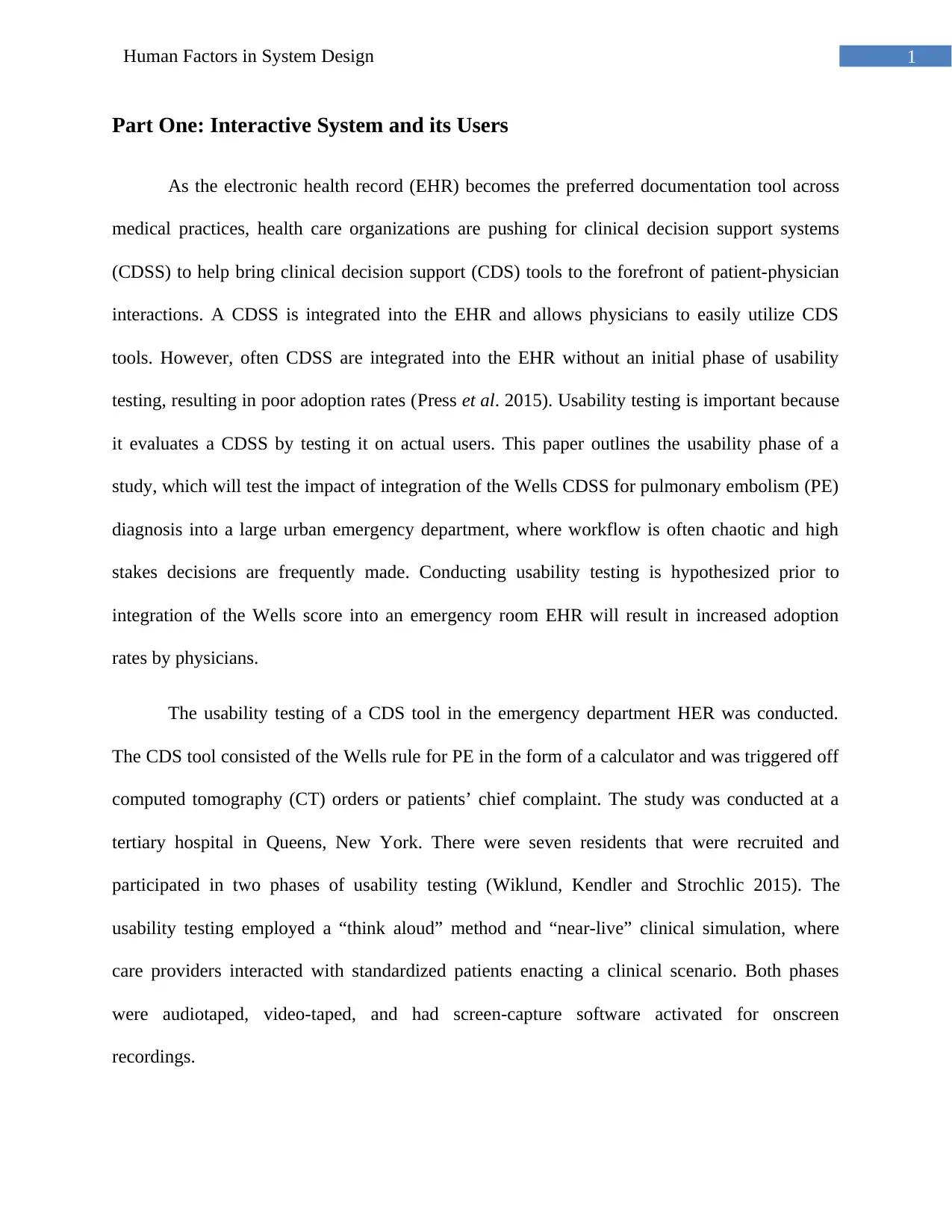
1Human Factors in System Design
Part One: Interactive System and its Users
As the electronic health record (EHR) becomes the preferred documentation tool across
medical practices, health care organizations are pushing for clinical decision support systems
(CDSS) to help bring clinical decision support (CDS) tools to the forefront of patient-physician
interactions. A CDSS is integrated into the EHR and allows physicians to easily utilize CDS
tools. However, often CDSS are integrated into the EHR without an initial phase of usability
testing, resulting in poor adoption rates (Press et al. 2015). Usability testing is important because
it evaluates a CDSS by testing it on actual users. This paper outlines the usability phase of a
study, which will test the impact of integration of the Wells CDSS for pulmonary embolism (PE)
diagnosis into a large urban emergency department, where workflow is often chaotic and high
stakes decisions are frequently made. Conducting usability testing is hypothesized prior to
integration of the Wells score into an emergency room EHR will result in increased adoption
rates by physicians.
The usability testing of a CDS tool in the emergency department HER was conducted.
The CDS tool consisted of the Wells rule for PE in the form of a calculator and was triggered off
computed tomography (CT) orders or patients’ chief complaint. The study was conducted at a
tertiary hospital in Queens, New York. There were seven residents that were recruited and
participated in two phases of usability testing (Wiklund, Kendler and Strochlic 2015). The
usability testing employed a “think aloud” method and “near-live” clinical simulation, where
care providers interacted with standardized patients enacting a clinical scenario. Both phases
were audiotaped, video-taped, and had screen-capture software activated for onscreen
recordings.
Part One: Interactive System and its Users
As the electronic health record (EHR) becomes the preferred documentation tool across
medical practices, health care organizations are pushing for clinical decision support systems
(CDSS) to help bring clinical decision support (CDS) tools to the forefront of patient-physician
interactions. A CDSS is integrated into the EHR and allows physicians to easily utilize CDS
tools. However, often CDSS are integrated into the EHR without an initial phase of usability
testing, resulting in poor adoption rates (Press et al. 2015). Usability testing is important because
it evaluates a CDSS by testing it on actual users. This paper outlines the usability phase of a
study, which will test the impact of integration of the Wells CDSS for pulmonary embolism (PE)
diagnosis into a large urban emergency department, where workflow is often chaotic and high
stakes decisions are frequently made. Conducting usability testing is hypothesized prior to
integration of the Wells score into an emergency room EHR will result in increased adoption
rates by physicians.
The usability testing of a CDS tool in the emergency department HER was conducted.
The CDS tool consisted of the Wells rule for PE in the form of a calculator and was triggered off
computed tomography (CT) orders or patients’ chief complaint. The study was conducted at a
tertiary hospital in Queens, New York. There were seven residents that were recruited and
participated in two phases of usability testing (Wiklund, Kendler and Strochlic 2015). The
usability testing employed a “think aloud” method and “near-live” clinical simulation, where
care providers interacted with standardized patients enacting a clinical scenario. Both phases
were audiotaped, video-taped, and had screen-capture software activated for onscreen
recordings.
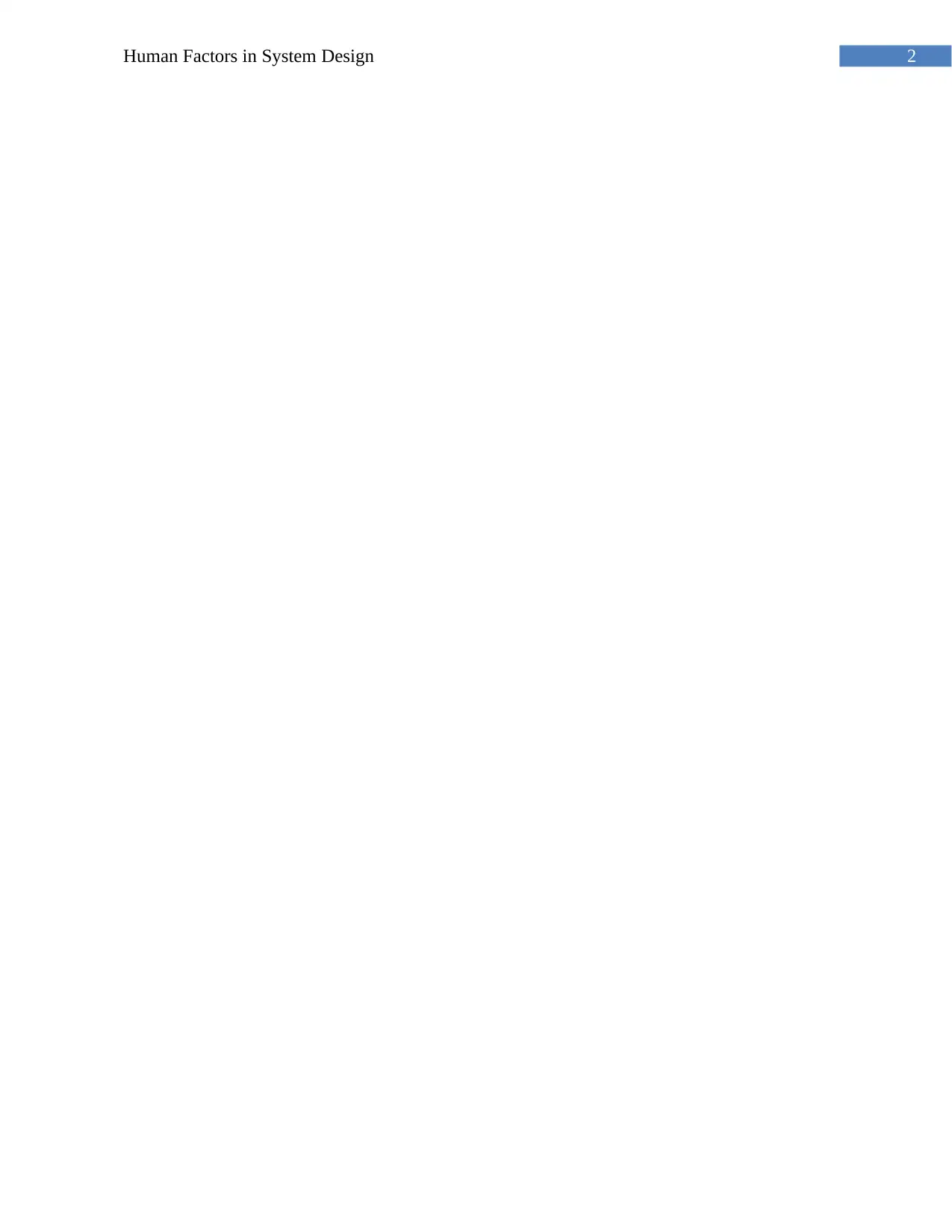
2Human Factors in System Design
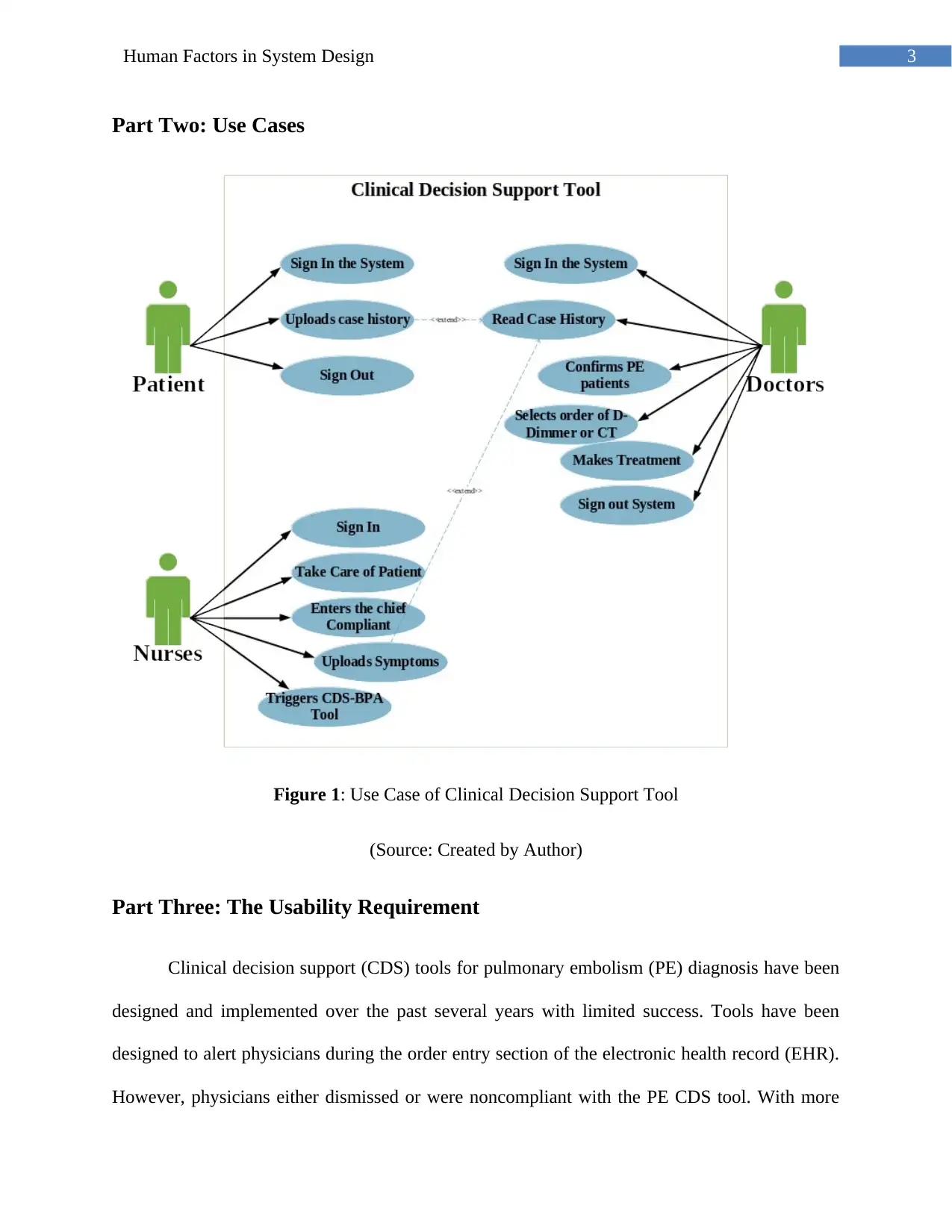
3Human Factors in System Design
Part Two: Use Cases
Figure 1: Use Case of Clinical Decision Support Tool
(Source: Created by Author)
Part Three: The Usability Requirement
Clinical decision support (CDS) tools for pulmonary embolism (PE) diagnosis have been
designed and implemented over the past several years with limited success. Tools have been
designed to alert physicians during the order entry section of the electronic health record (EHR).
However, physicians either dismissed or were noncompliant with the PE CDS tool. With more
Part Two: Use Cases
Figure 1: Use Case of Clinical Decision Support Tool
(Source: Created by Author)
Part Three: The Usability Requirement
Clinical decision support (CDS) tools for pulmonary embolism (PE) diagnosis have been
designed and implemented over the past several years with limited success. Tools have been
designed to alert physicians during the order entry section of the electronic health record (EHR).
However, physicians either dismissed or were noncompliant with the PE CDS tool. With more
Secure Best Marks with AI Grader
Need help grading? Try our AI Grader for instant feedback on your assignments.
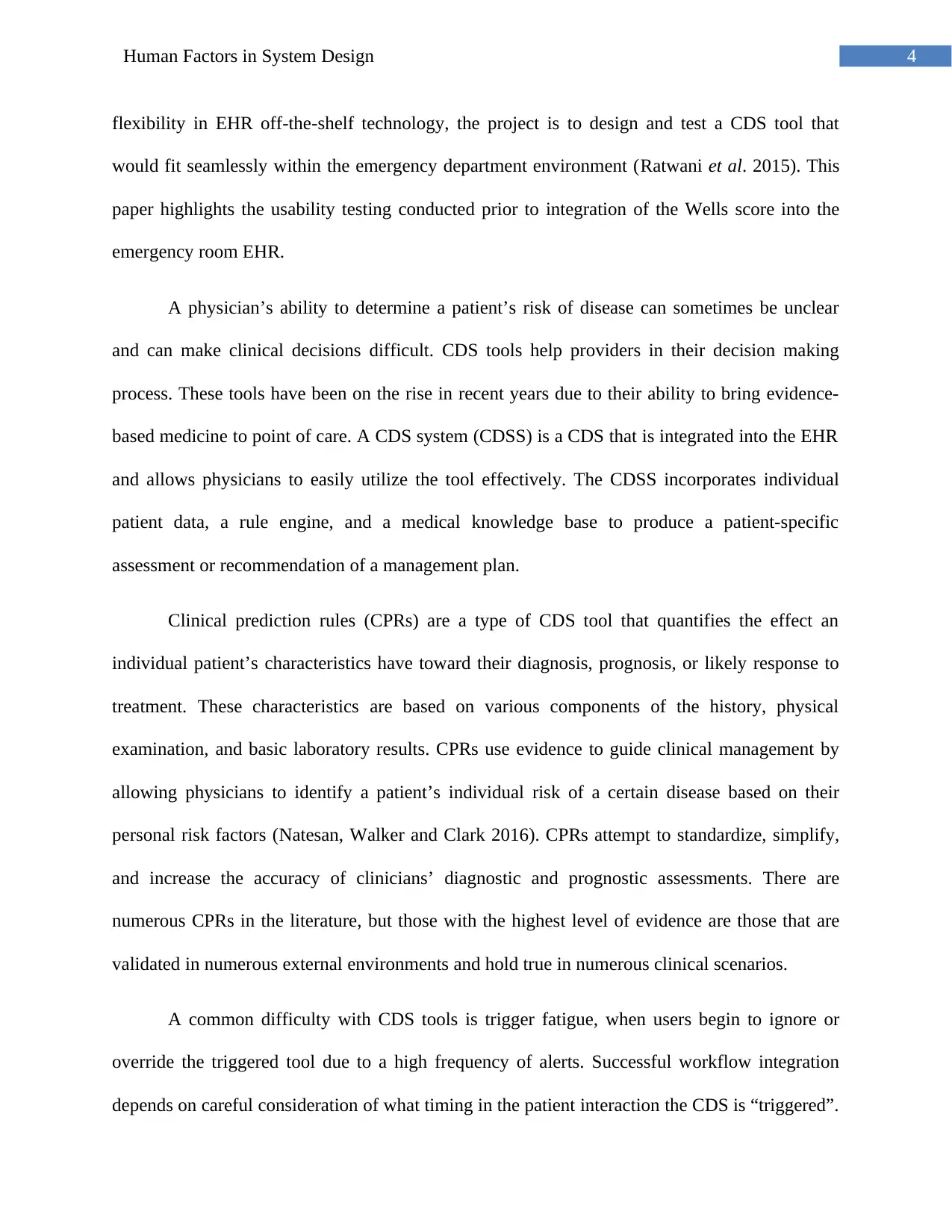
4Human Factors in System Design
flexibility in EHR off-the-shelf technology, the project is to design and test a CDS tool that
would fit seamlessly within the emergency department environment (Ratwani et al. 2015). This
paper highlights the usability testing conducted prior to integration of the Wells score into the
emergency room EHR.
A physician’s ability to determine a patient’s risk of disease can sometimes be unclear
and can make clinical decisions difficult. CDS tools help providers in their decision making
process. These tools have been on the rise in recent years due to their ability to bring evidence-
based medicine to point of care. A CDS system (CDSS) is a CDS that is integrated into the EHR
and allows physicians to easily utilize the tool effectively. The CDSS incorporates individual
patient data, a rule engine, and a medical knowledge base to produce a patient-specific
assessment or recommendation of a management plan.
Clinical prediction rules (CPRs) are a type of CDS tool that quantifies the effect an
individual patient’s characteristics have toward their diagnosis, prognosis, or likely response to
treatment. These characteristics are based on various components of the history, physical
examination, and basic laboratory results. CPRs use evidence to guide clinical management by
allowing physicians to identify a patient’s individual risk of a certain disease based on their
personal risk factors (Natesan, Walker and Clark 2016). CPRs attempt to standardize, simplify,
and increase the accuracy of clinicians’ diagnostic and prognostic assessments. There are
numerous CPRs in the literature, but those with the highest level of evidence are those that are
validated in numerous external environments and hold true in numerous clinical scenarios.
A common difficulty with CDS tools is trigger fatigue, when users begin to ignore or
override the triggered tool due to a high frequency of alerts. Successful workflow integration
depends on careful consideration of what timing in the patient interaction the CDS is “triggered”.
flexibility in EHR off-the-shelf technology, the project is to design and test a CDS tool that
would fit seamlessly within the emergency department environment (Ratwani et al. 2015). This
paper highlights the usability testing conducted prior to integration of the Wells score into the
emergency room EHR.
A physician’s ability to determine a patient’s risk of disease can sometimes be unclear
and can make clinical decisions difficult. CDS tools help providers in their decision making
process. These tools have been on the rise in recent years due to their ability to bring evidence-
based medicine to point of care. A CDS system (CDSS) is a CDS that is integrated into the EHR
and allows physicians to easily utilize the tool effectively. The CDSS incorporates individual
patient data, a rule engine, and a medical knowledge base to produce a patient-specific
assessment or recommendation of a management plan.
Clinical prediction rules (CPRs) are a type of CDS tool that quantifies the effect an
individual patient’s characteristics have toward their diagnosis, prognosis, or likely response to
treatment. These characteristics are based on various components of the history, physical
examination, and basic laboratory results. CPRs use evidence to guide clinical management by
allowing physicians to identify a patient’s individual risk of a certain disease based on their
personal risk factors (Natesan, Walker and Clark 2016). CPRs attempt to standardize, simplify,
and increase the accuracy of clinicians’ diagnostic and prognostic assessments. There are
numerous CPRs in the literature, but those with the highest level of evidence are those that are
validated in numerous external environments and hold true in numerous clinical scenarios.
A common difficulty with CDS tools is trigger fatigue, when users begin to ignore or
override the triggered tool due to a high frequency of alerts. Successful workflow integration
depends on careful consideration of what timing in the patient interaction the CDS is “triggered”.
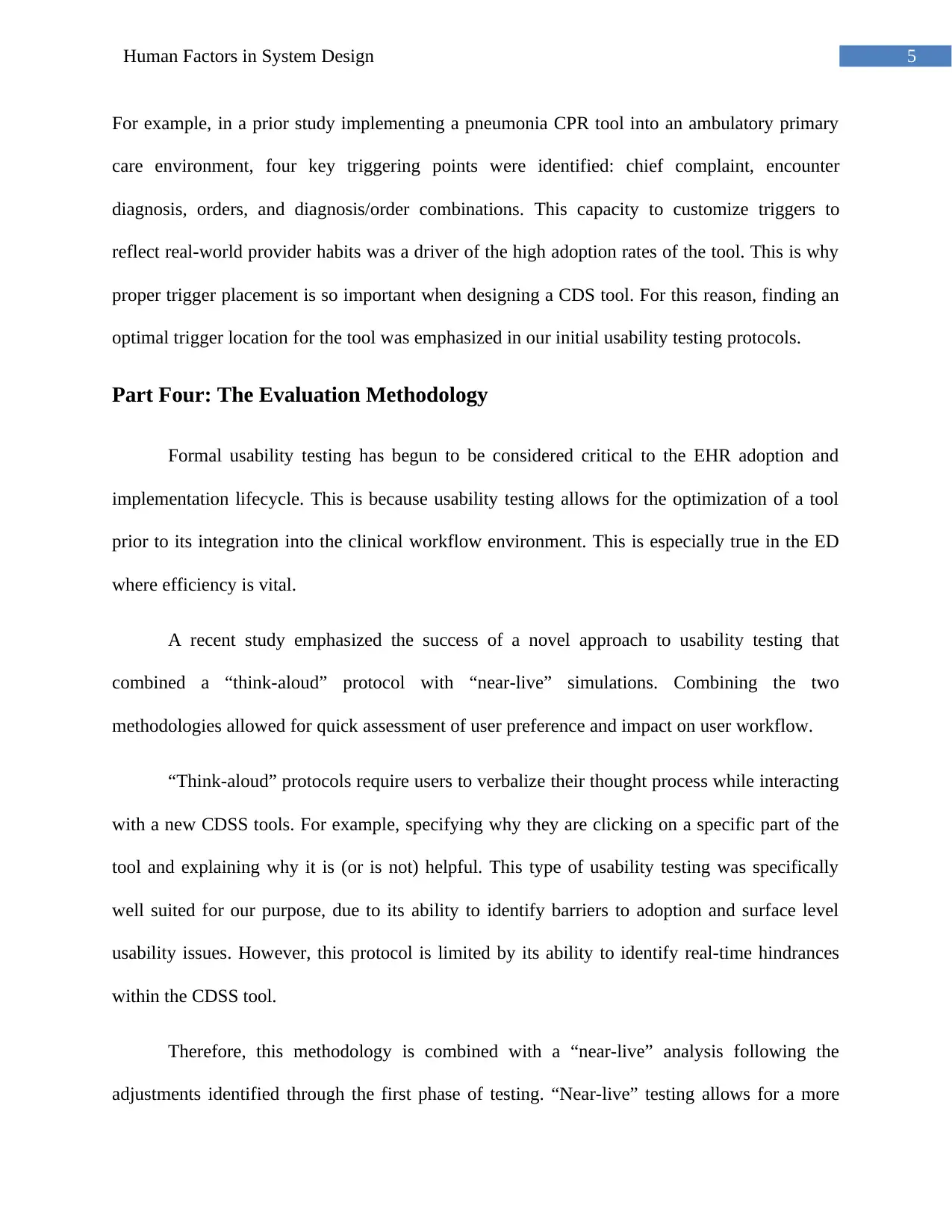
5Human Factors in System Design
For example, in a prior study implementing a pneumonia CPR tool into an ambulatory primary
care environment, four key triggering points were identified: chief complaint, encounter
diagnosis, orders, and diagnosis/order combinations. This capacity to customize triggers to
reflect real-world provider habits was a driver of the high adoption rates of the tool. This is why
proper trigger placement is so important when designing a CDS tool. For this reason, finding an
optimal trigger location for the tool was emphasized in our initial usability testing protocols.
Part Four: The Evaluation Methodology
Formal usability testing has begun to be considered critical to the EHR adoption and
implementation lifecycle. This is because usability testing allows for the optimization of a tool
prior to its integration into the clinical workflow environment. This is especially true in the ED
where efficiency is vital.
A recent study emphasized the success of a novel approach to usability testing that
combined a “think-aloud” protocol with “near-live” simulations. Combining the two
methodologies allowed for quick assessment of user preference and impact on user workflow.
“Think-aloud” protocols require users to verbalize their thought process while interacting
with a new CDSS tools. For example, specifying why they are clicking on a specific part of the
tool and explaining why it is (or is not) helpful. This type of usability testing was specifically
well suited for our purpose, due to its ability to identify barriers to adoption and surface level
usability issues. However, this protocol is limited by its ability to identify real-time hindrances
within the CDSS tool.
Therefore, this methodology is combined with a “near-live” analysis following the
adjustments identified through the first phase of testing. “Near-live” testing allows for a more
For example, in a prior study implementing a pneumonia CPR tool into an ambulatory primary
care environment, four key triggering points were identified: chief complaint, encounter
diagnosis, orders, and diagnosis/order combinations. This capacity to customize triggers to
reflect real-world provider habits was a driver of the high adoption rates of the tool. This is why
proper trigger placement is so important when designing a CDS tool. For this reason, finding an
optimal trigger location for the tool was emphasized in our initial usability testing protocols.
Part Four: The Evaluation Methodology
Formal usability testing has begun to be considered critical to the EHR adoption and
implementation lifecycle. This is because usability testing allows for the optimization of a tool
prior to its integration into the clinical workflow environment. This is especially true in the ED
where efficiency is vital.
A recent study emphasized the success of a novel approach to usability testing that
combined a “think-aloud” protocol with “near-live” simulations. Combining the two
methodologies allowed for quick assessment of user preference and impact on user workflow.
“Think-aloud” protocols require users to verbalize their thought process while interacting
with a new CDSS tools. For example, specifying why they are clicking on a specific part of the
tool and explaining why it is (or is not) helpful. This type of usability testing was specifically
well suited for our purpose, due to its ability to identify barriers to adoption and surface level
usability issues. However, this protocol is limited by its ability to identify real-time hindrances
within the CDSS tool.
Therefore, this methodology is combined with a “near-live” analysis following the
adjustments identified through the first phase of testing. “Near-live” testing allows for a more
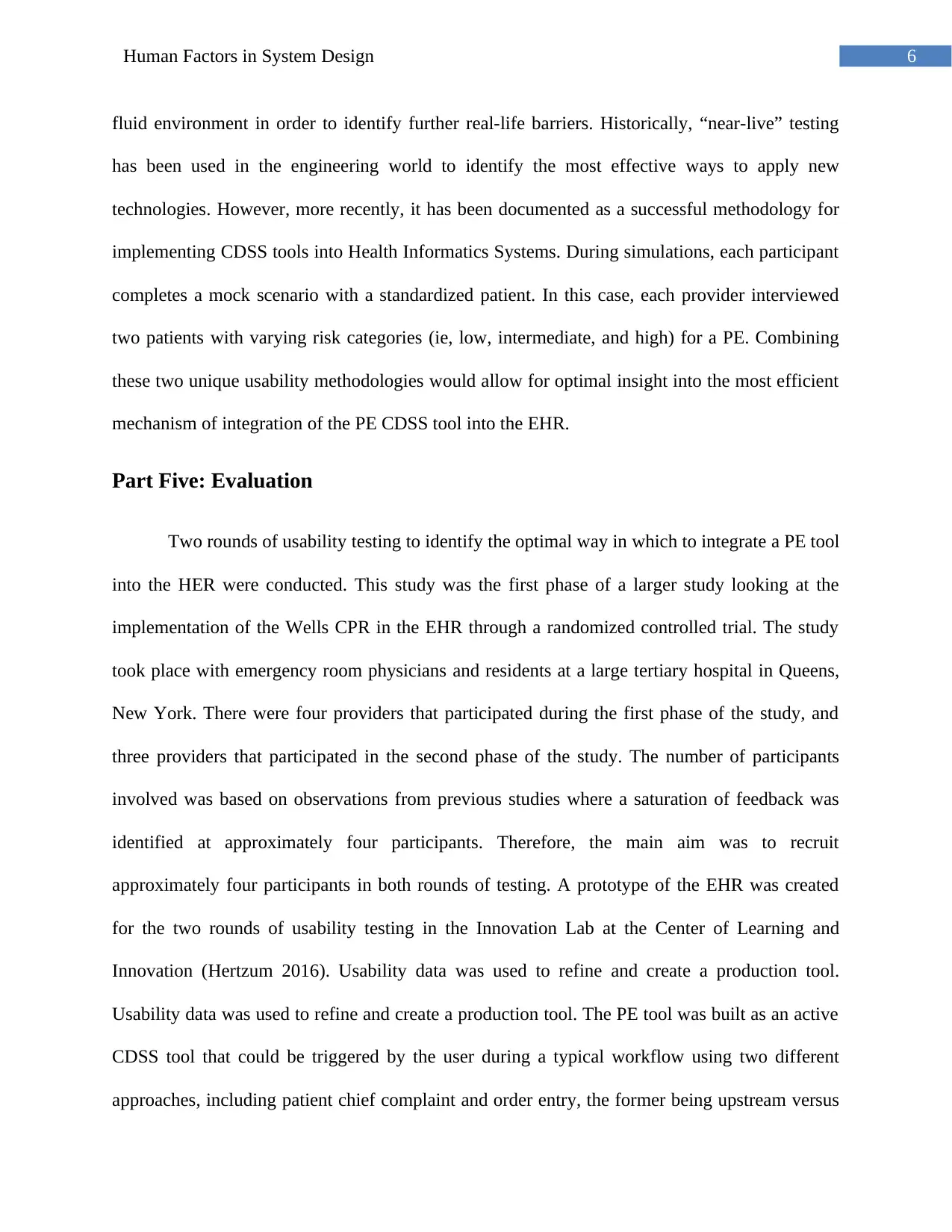
6Human Factors in System Design
fluid environment in order to identify further real-life barriers. Historically, “near-live” testing
has been used in the engineering world to identify the most effective ways to apply new
technologies. However, more recently, it has been documented as a successful methodology for
implementing CDSS tools into Health Informatics Systems. During simulations, each participant
completes a mock scenario with a standardized patient. In this case, each provider interviewed
two patients with varying risk categories (ie, low, intermediate, and high) for a PE. Combining
these two unique usability methodologies would allow for optimal insight into the most efficient
mechanism of integration of the PE CDSS tool into the EHR.
Part Five: Evaluation
Two rounds of usability testing to identify the optimal way in which to integrate a PE tool
into the HER were conducted. This study was the first phase of a larger study looking at the
implementation of the Wells CPR in the EHR through a randomized controlled trial. The study
took place with emergency room physicians and residents at a large tertiary hospital in Queens,
New York. There were four providers that participated during the first phase of the study, and
three providers that participated in the second phase of the study. The number of participants
involved was based on observations from previous studies where a saturation of feedback was
identified at approximately four participants. Therefore, the main aim was to recruit
approximately four participants in both rounds of testing. A prototype of the EHR was created
for the two rounds of usability testing in the Innovation Lab at the Center of Learning and
Innovation (Hertzum 2016). Usability data was used to refine and create a production tool.
Usability data was used to refine and create a production tool. The PE tool was built as an active
CDSS tool that could be triggered by the user during a typical workflow using two different
approaches, including patient chief complaint and order entry, the former being upstream versus
fluid environment in order to identify further real-life barriers. Historically, “near-live” testing
has been used in the engineering world to identify the most effective ways to apply new
technologies. However, more recently, it has been documented as a successful methodology for
implementing CDSS tools into Health Informatics Systems. During simulations, each participant
completes a mock scenario with a standardized patient. In this case, each provider interviewed
two patients with varying risk categories (ie, low, intermediate, and high) for a PE. Combining
these two unique usability methodologies would allow for optimal insight into the most efficient
mechanism of integration of the PE CDSS tool into the EHR.
Part Five: Evaluation
Two rounds of usability testing to identify the optimal way in which to integrate a PE tool
into the HER were conducted. This study was the first phase of a larger study looking at the
implementation of the Wells CPR in the EHR through a randomized controlled trial. The study
took place with emergency room physicians and residents at a large tertiary hospital in Queens,
New York. There were four providers that participated during the first phase of the study, and
three providers that participated in the second phase of the study. The number of participants
involved was based on observations from previous studies where a saturation of feedback was
identified at approximately four participants. Therefore, the main aim was to recruit
approximately four participants in both rounds of testing. A prototype of the EHR was created
for the two rounds of usability testing in the Innovation Lab at the Center of Learning and
Innovation (Hertzum 2016). Usability data was used to refine and create a production tool.
Usability data was used to refine and create a production tool. The PE tool was built as an active
CDSS tool that could be triggered by the user during a typical workflow using two different
approaches, including patient chief complaint and order entry, the former being upstream versus
Paraphrase This Document
Need a fresh take? Get an instant paraphrase of this document with our AI Paraphraser
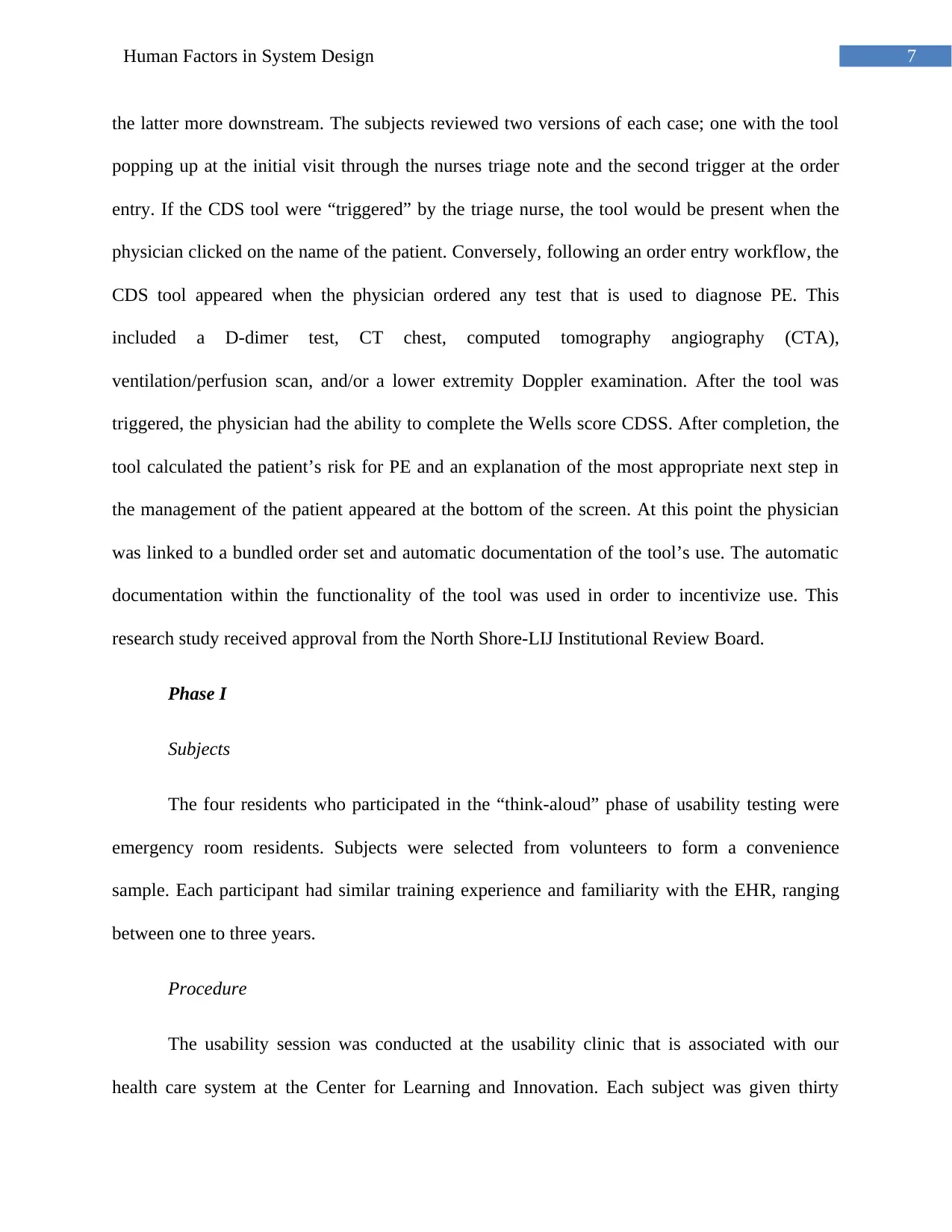
7Human Factors in System Design
the latter more downstream. The subjects reviewed two versions of each case; one with the tool
popping up at the initial visit through the nurses triage note and the second trigger at the order
entry. If the CDS tool were “triggered” by the triage nurse, the tool would be present when the
physician clicked on the name of the patient. Conversely, following an order entry workflow, the
CDS tool appeared when the physician ordered any test that is used to diagnose PE. This
included a D-dimer test, CT chest, computed tomography angiography (CTA),
ventilation/perfusion scan, and/or a lower extremity Doppler examination. After the tool was
triggered, the physician had the ability to complete the Wells score CDSS. After completion, the
tool calculated the patient’s risk for PE and an explanation of the most appropriate next step in
the management of the patient appeared at the bottom of the screen. At this point the physician
was linked to a bundled order set and automatic documentation of the tool’s use. The automatic
documentation within the functionality of the tool was used in order to incentivize use. This
research study received approval from the North Shore-LIJ Institutional Review Board.
Phase I
Subjects
The four residents who participated in the “think-aloud” phase of usability testing were
emergency room residents. Subjects were selected from volunteers to form a convenience
sample. Each participant had similar training experience and familiarity with the EHR, ranging
between one to three years.
Procedure
The usability session was conducted at the usability clinic that is associated with our
health care system at the Center for Learning and Innovation. Each subject was given thirty
the latter more downstream. The subjects reviewed two versions of each case; one with the tool
popping up at the initial visit through the nurses triage note and the second trigger at the order
entry. If the CDS tool were “triggered” by the triage nurse, the tool would be present when the
physician clicked on the name of the patient. Conversely, following an order entry workflow, the
CDS tool appeared when the physician ordered any test that is used to diagnose PE. This
included a D-dimer test, CT chest, computed tomography angiography (CTA),
ventilation/perfusion scan, and/or a lower extremity Doppler examination. After the tool was
triggered, the physician had the ability to complete the Wells score CDSS. After completion, the
tool calculated the patient’s risk for PE and an explanation of the most appropriate next step in
the management of the patient appeared at the bottom of the screen. At this point the physician
was linked to a bundled order set and automatic documentation of the tool’s use. The automatic
documentation within the functionality of the tool was used in order to incentivize use. This
research study received approval from the North Shore-LIJ Institutional Review Board.
Phase I
Subjects
The four residents who participated in the “think-aloud” phase of usability testing were
emergency room residents. Subjects were selected from volunteers to form a convenience
sample. Each participant had similar training experience and familiarity with the EHR, ranging
between one to three years.
Procedure
The usability session was conducted at the usability clinic that is associated with our
health care system at the Center for Learning and Innovation. Each subject was given thirty
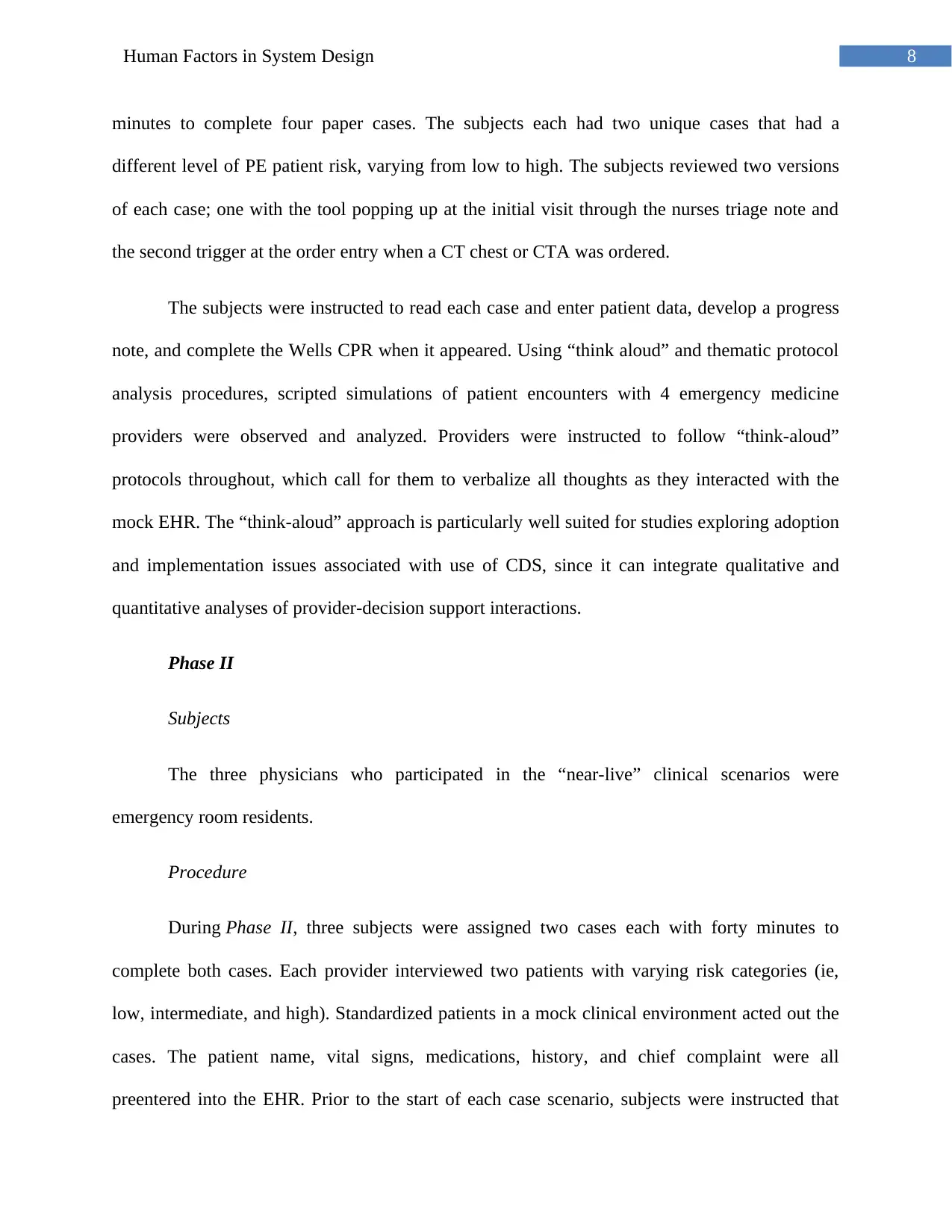
8Human Factors in System Design
minutes to complete four paper cases. The subjects each had two unique cases that had a
different level of PE patient risk, varying from low to high. The subjects reviewed two versions
of each case; one with the tool popping up at the initial visit through the nurses triage note and
the second trigger at the order entry when a CT chest or CTA was ordered.
The subjects were instructed to read each case and enter patient data, develop a progress
note, and complete the Wells CPR when it appeared. Using “think aloud” and thematic protocol
analysis procedures, scripted simulations of patient encounters with 4 emergency medicine
providers were observed and analyzed. Providers were instructed to follow “think-aloud”
protocols throughout, which call for them to verbalize all thoughts as they interacted with the
mock EHR. The “think-aloud” approach is particularly well suited for studies exploring adoption
and implementation issues associated with use of CDS, since it can integrate qualitative and
quantitative analyses of provider-decision support interactions.
Phase II
Subjects
The three physicians who participated in the “near-live” clinical scenarios were
emergency room residents.
Procedure
During Phase II, three subjects were assigned two cases each with forty minutes to
complete both cases. Each provider interviewed two patients with varying risk categories (ie,
low, intermediate, and high). Standardized patients in a mock clinical environment acted out the
cases. The patient name, vital signs, medications, history, and chief complaint were all
preentered into the EHR. Prior to the start of each case scenario, subjects were instructed that
minutes to complete four paper cases. The subjects each had two unique cases that had a
different level of PE patient risk, varying from low to high. The subjects reviewed two versions
of each case; one with the tool popping up at the initial visit through the nurses triage note and
the second trigger at the order entry when a CT chest or CTA was ordered.
The subjects were instructed to read each case and enter patient data, develop a progress
note, and complete the Wells CPR when it appeared. Using “think aloud” and thematic protocol
analysis procedures, scripted simulations of patient encounters with 4 emergency medicine
providers were observed and analyzed. Providers were instructed to follow “think-aloud”
protocols throughout, which call for them to verbalize all thoughts as they interacted with the
mock EHR. The “think-aloud” approach is particularly well suited for studies exploring adoption
and implementation issues associated with use of CDS, since it can integrate qualitative and
quantitative analyses of provider-decision support interactions.
Phase II
Subjects
The three physicians who participated in the “near-live” clinical scenarios were
emergency room residents.
Procedure
During Phase II, three subjects were assigned two cases each with forty minutes to
complete both cases. Each provider interviewed two patients with varying risk categories (ie,
low, intermediate, and high). Standardized patients in a mock clinical environment acted out the
cases. The patient name, vital signs, medications, history, and chief complaint were all
preentered into the EHR. Prior to the start of each case scenario, subjects were instructed that
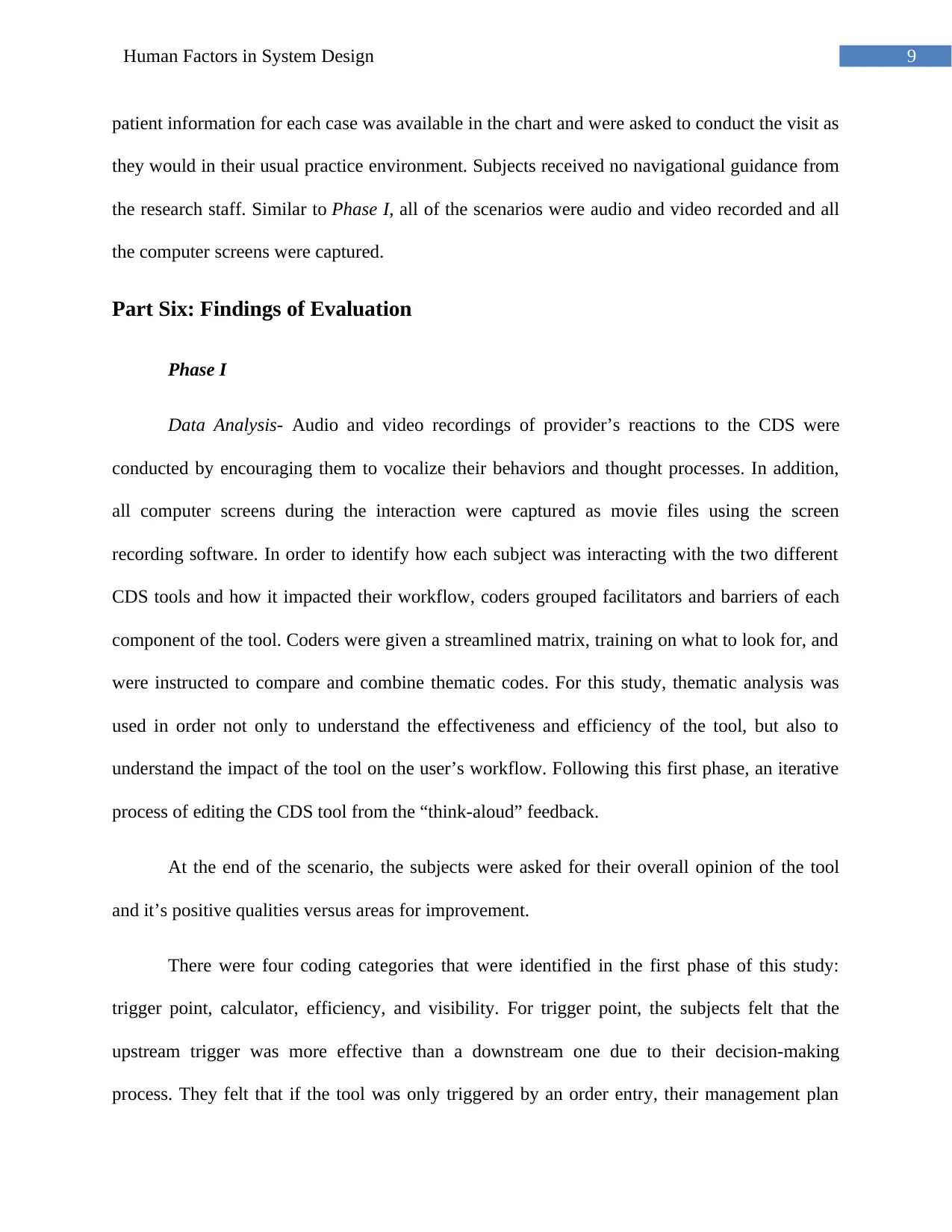
9Human Factors in System Design
patient information for each case was available in the chart and were asked to conduct the visit as
they would in their usual practice environment. Subjects received no navigational guidance from
the research staff. Similar to Phase I, all of the scenarios were audio and video recorded and all
the computer screens were captured.
Part Six: Findings of Evaluation
Phase I
Data Analysis- Audio and video recordings of provider’s reactions to the CDS were
conducted by encouraging them to vocalize their behaviors and thought processes. In addition,
all computer screens during the interaction were captured as movie files using the screen
recording software. In order to identify how each subject was interacting with the two different
CDS tools and how it impacted their workflow, coders grouped facilitators and barriers of each
component of the tool. Coders were given a streamlined matrix, training on what to look for, and
were instructed to compare and combine thematic codes. For this study, thematic analysis was
used in order not only to understand the effectiveness and efficiency of the tool, but also to
understand the impact of the tool on the user’s workflow. Following this first phase, an iterative
process of editing the CDS tool from the “think-aloud” feedback.
At the end of the scenario, the subjects were asked for their overall opinion of the tool
and it’s positive qualities versus areas for improvement.
There were four coding categories that were identified in the first phase of this study:
trigger point, calculator, efficiency, and visibility. For trigger point, the subjects felt that the
upstream trigger was more effective than a downstream one due to their decision-making
process. They felt that if the tool was only triggered by an order entry, their management plan
patient information for each case was available in the chart and were asked to conduct the visit as
they would in their usual practice environment. Subjects received no navigational guidance from
the research staff. Similar to Phase I, all of the scenarios were audio and video recorded and all
the computer screens were captured.
Part Six: Findings of Evaluation
Phase I
Data Analysis- Audio and video recordings of provider’s reactions to the CDS were
conducted by encouraging them to vocalize their behaviors and thought processes. In addition,
all computer screens during the interaction were captured as movie files using the screen
recording software. In order to identify how each subject was interacting with the two different
CDS tools and how it impacted their workflow, coders grouped facilitators and barriers of each
component of the tool. Coders were given a streamlined matrix, training on what to look for, and
were instructed to compare and combine thematic codes. For this study, thematic analysis was
used in order not only to understand the effectiveness and efficiency of the tool, but also to
understand the impact of the tool on the user’s workflow. Following this first phase, an iterative
process of editing the CDS tool from the “think-aloud” feedback.
At the end of the scenario, the subjects were asked for their overall opinion of the tool
and it’s positive qualities versus areas for improvement.
There were four coding categories that were identified in the first phase of this study:
trigger point, calculator, efficiency, and visibility. For trigger point, the subjects felt that the
upstream trigger was more effective than a downstream one due to their decision-making
process. They felt that if the tool was only triggered by an order entry, their management plan
Secure Best Marks with AI Grader
Need help grading? Try our AI Grader for instant feedback on your assignments.
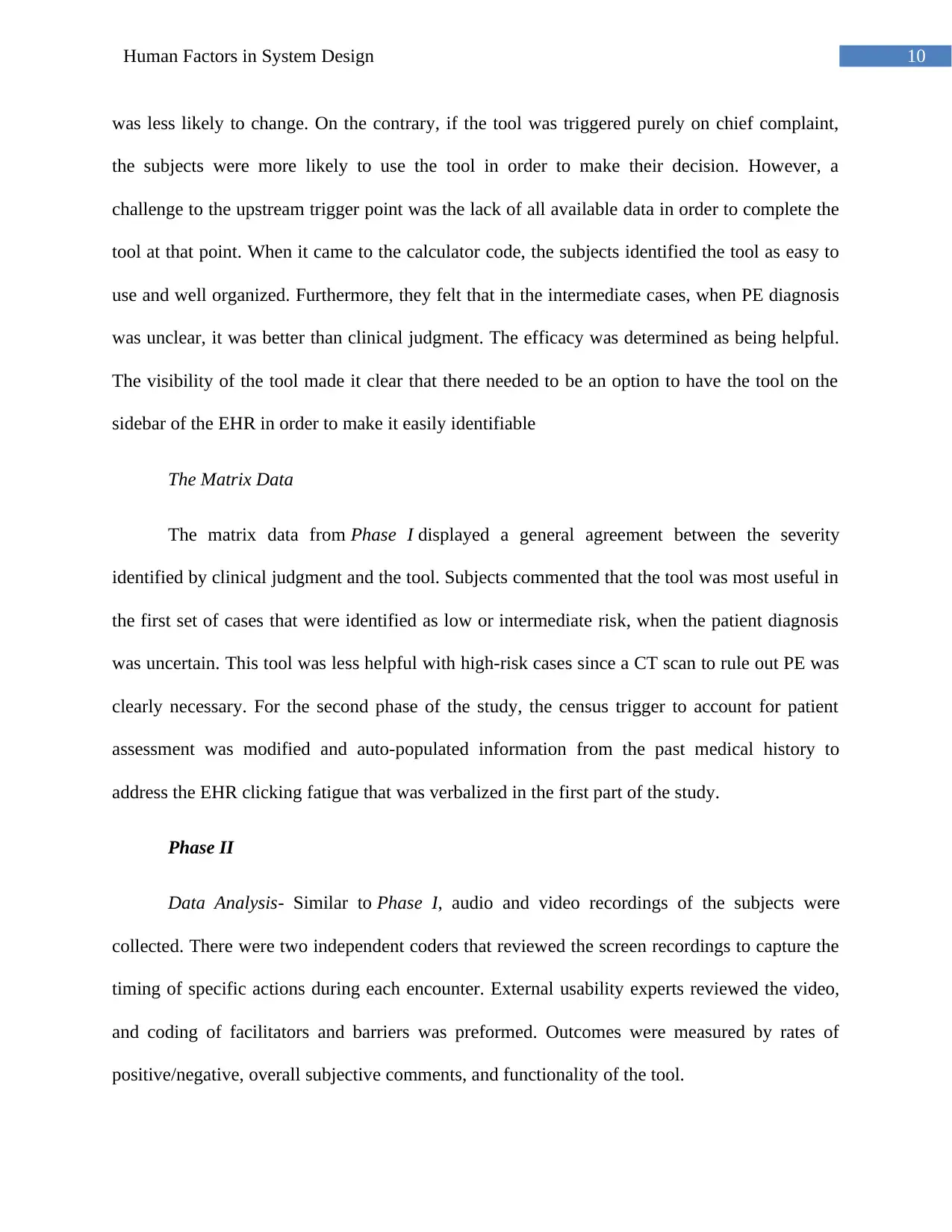
10Human Factors in System Design
was less likely to change. On the contrary, if the tool was triggered purely on chief complaint,
the subjects were more likely to use the tool in order to make their decision. However, a
challenge to the upstream trigger point was the lack of all available data in order to complete the
tool at that point. When it came to the calculator code, the subjects identified the tool as easy to
use and well organized. Furthermore, they felt that in the intermediate cases, when PE diagnosis
was unclear, it was better than clinical judgment. The efficacy was determined as being helpful.
The visibility of the tool made it clear that there needed to be an option to have the tool on the
sidebar of the EHR in order to make it easily identifiable
The Matrix Data
The matrix data from Phase I displayed a general agreement between the severity
identified by clinical judgment and the tool. Subjects commented that the tool was most useful in
the first set of cases that were identified as low or intermediate risk, when the patient diagnosis
was uncertain. This tool was less helpful with high-risk cases since a CT scan to rule out PE was
clearly necessary. For the second phase of the study, the census trigger to account for patient
assessment was modified and auto-populated information from the past medical history to
address the EHR clicking fatigue that was verbalized in the first part of the study.
Phase II
Data Analysis- Similar to Phase I, audio and video recordings of the subjects were
collected. There were two independent coders that reviewed the screen recordings to capture the
timing of specific actions during each encounter. External usability experts reviewed the video,
and coding of facilitators and barriers was preformed. Outcomes were measured by rates of
positive/negative, overall subjective comments, and functionality of the tool.
was less likely to change. On the contrary, if the tool was triggered purely on chief complaint,
the subjects were more likely to use the tool in order to make their decision. However, a
challenge to the upstream trigger point was the lack of all available data in order to complete the
tool at that point. When it came to the calculator code, the subjects identified the tool as easy to
use and well organized. Furthermore, they felt that in the intermediate cases, when PE diagnosis
was unclear, it was better than clinical judgment. The efficacy was determined as being helpful.
The visibility of the tool made it clear that there needed to be an option to have the tool on the
sidebar of the EHR in order to make it easily identifiable
The Matrix Data
The matrix data from Phase I displayed a general agreement between the severity
identified by clinical judgment and the tool. Subjects commented that the tool was most useful in
the first set of cases that were identified as low or intermediate risk, when the patient diagnosis
was uncertain. This tool was less helpful with high-risk cases since a CT scan to rule out PE was
clearly necessary. For the second phase of the study, the census trigger to account for patient
assessment was modified and auto-populated information from the past medical history to
address the EHR clicking fatigue that was verbalized in the first part of the study.
Phase II
Data Analysis- Similar to Phase I, audio and video recordings of the subjects were
collected. There were two independent coders that reviewed the screen recordings to capture the
timing of specific actions during each encounter. External usability experts reviewed the video,
and coding of facilitators and barriers was preformed. Outcomes were measured by rates of
positive/negative, overall subjective comments, and functionality of the tool.
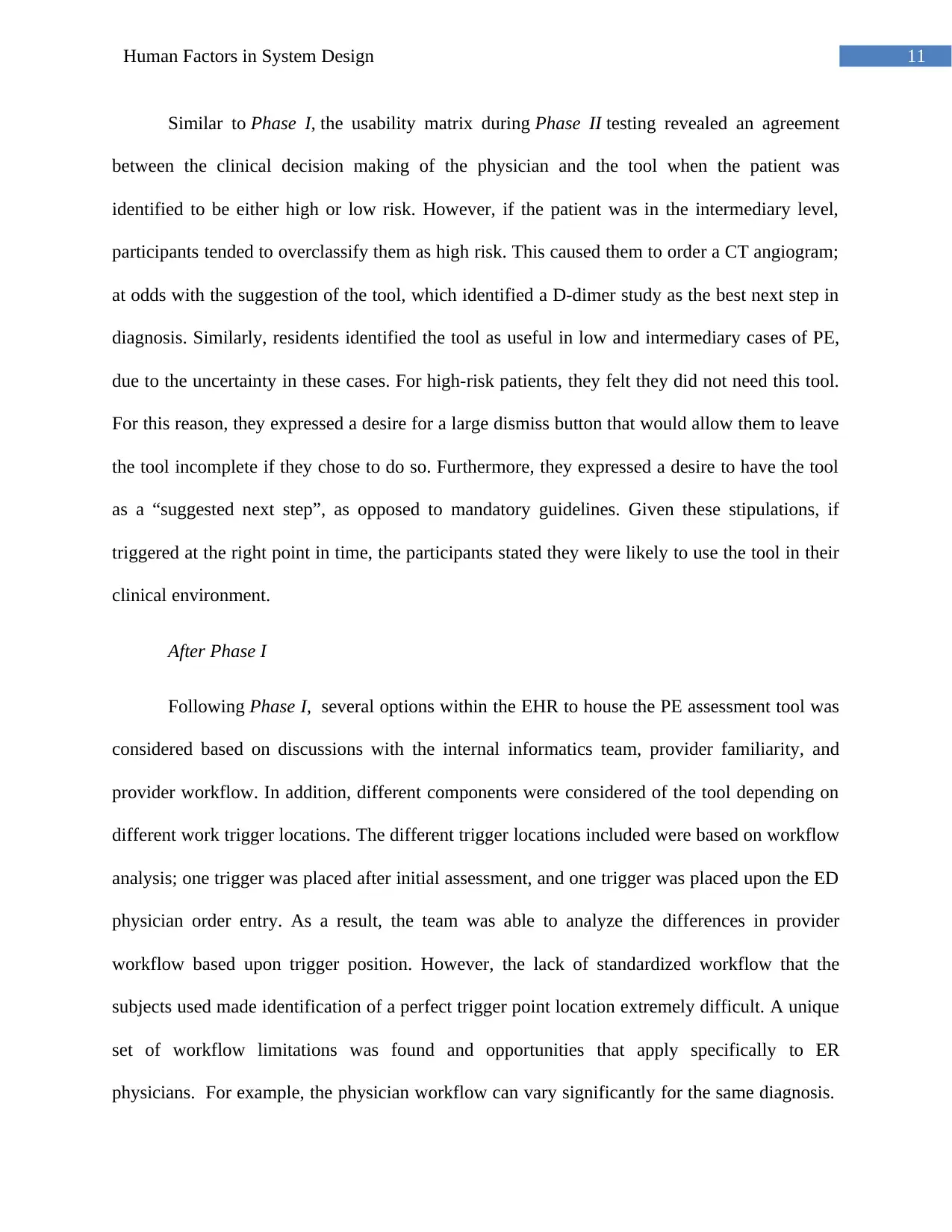
11Human Factors in System Design
Similar to Phase I, the usability matrix during Phase II testing revealed an agreement
between the clinical decision making of the physician and the tool when the patient was
identified to be either high or low risk. However, if the patient was in the intermediary level,
participants tended to overclassify them as high risk. This caused them to order a CT angiogram;
at odds with the suggestion of the tool, which identified a D-dimer study as the best next step in
diagnosis. Similarly, residents identified the tool as useful in low and intermediary cases of PE,
due to the uncertainty in these cases. For high-risk patients, they felt they did not need this tool.
For this reason, they expressed a desire for a large dismiss button that would allow them to leave
the tool incomplete if they chose to do so. Furthermore, they expressed a desire to have the tool
as a “suggested next step”, as opposed to mandatory guidelines. Given these stipulations, if
triggered at the right point in time, the participants stated they were likely to use the tool in their
clinical environment.
After Phase I
Following Phase I, several options within the EHR to house the PE assessment tool was
considered based on discussions with the internal informatics team, provider familiarity, and
provider workflow. In addition, different components were considered of the tool depending on
different work trigger locations. The different trigger locations included were based on workflow
analysis; one trigger was placed after initial assessment, and one trigger was placed upon the ED
physician order entry. As a result, the team was able to analyze the differences in provider
workflow based upon trigger position. However, the lack of standardized workflow that the
subjects used made identification of a perfect trigger point location extremely difficult. A unique
set of workflow limitations was found and opportunities that apply specifically to ER
physicians. For example, the physician workflow can vary significantly for the same diagnosis.
Similar to Phase I, the usability matrix during Phase II testing revealed an agreement
between the clinical decision making of the physician and the tool when the patient was
identified to be either high or low risk. However, if the patient was in the intermediary level,
participants tended to overclassify them as high risk. This caused them to order a CT angiogram;
at odds with the suggestion of the tool, which identified a D-dimer study as the best next step in
diagnosis. Similarly, residents identified the tool as useful in low and intermediary cases of PE,
due to the uncertainty in these cases. For high-risk patients, they felt they did not need this tool.
For this reason, they expressed a desire for a large dismiss button that would allow them to leave
the tool incomplete if they chose to do so. Furthermore, they expressed a desire to have the tool
as a “suggested next step”, as opposed to mandatory guidelines. Given these stipulations, if
triggered at the right point in time, the participants stated they were likely to use the tool in their
clinical environment.
After Phase I
Following Phase I, several options within the EHR to house the PE assessment tool was
considered based on discussions with the internal informatics team, provider familiarity, and
provider workflow. In addition, different components were considered of the tool depending on
different work trigger locations. The different trigger locations included were based on workflow
analysis; one trigger was placed after initial assessment, and one trigger was placed upon the ED
physician order entry. As a result, the team was able to analyze the differences in provider
workflow based upon trigger position. However, the lack of standardized workflow that the
subjects used made identification of a perfect trigger point location extremely difficult. A unique
set of workflow limitations was found and opportunities that apply specifically to ER
physicians. For example, the physician workflow can vary significantly for the same diagnosis.
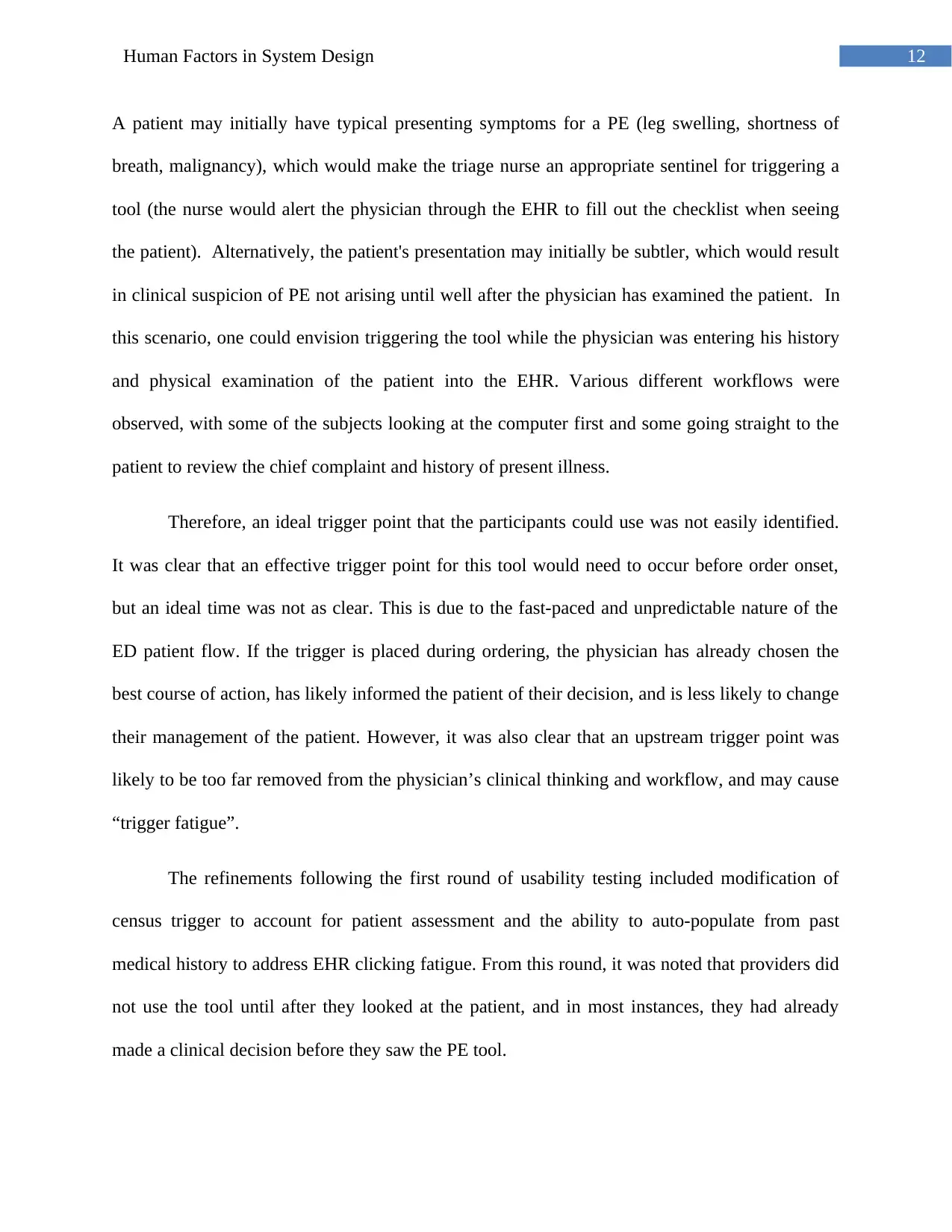
12Human Factors in System Design
A patient may initially have typical presenting symptoms for a PE (leg swelling, shortness of
breath, malignancy), which would make the triage nurse an appropriate sentinel for triggering a
tool (the nurse would alert the physician through the EHR to fill out the checklist when seeing
the patient). Alternatively, the patient's presentation may initially be subtler, which would result
in clinical suspicion of PE not arising until well after the physician has examined the patient. In
this scenario, one could envision triggering the tool while the physician was entering his history
and physical examination of the patient into the EHR. Various different workflows were
observed, with some of the subjects looking at the computer first and some going straight to the
patient to review the chief complaint and history of present illness.
Therefore, an ideal trigger point that the participants could use was not easily identified.
It was clear that an effective trigger point for this tool would need to occur before order onset,
but an ideal time was not as clear. This is due to the fast-paced and unpredictable nature of the
ED patient flow. If the trigger is placed during ordering, the physician has already chosen the
best course of action, has likely informed the patient of their decision, and is less likely to change
their management of the patient. However, it was also clear that an upstream trigger point was
likely to be too far removed from the physician’s clinical thinking and workflow, and may cause
“trigger fatigue”.
The refinements following the first round of usability testing included modification of
census trigger to account for patient assessment and the ability to auto-populate from past
medical history to address EHR clicking fatigue. From this round, it was noted that providers did
not use the tool until after they looked at the patient, and in most instances, they had already
made a clinical decision before they saw the PE tool.
A patient may initially have typical presenting symptoms for a PE (leg swelling, shortness of
breath, malignancy), which would make the triage nurse an appropriate sentinel for triggering a
tool (the nurse would alert the physician through the EHR to fill out the checklist when seeing
the patient). Alternatively, the patient's presentation may initially be subtler, which would result
in clinical suspicion of PE not arising until well after the physician has examined the patient. In
this scenario, one could envision triggering the tool while the physician was entering his history
and physical examination of the patient into the EHR. Various different workflows were
observed, with some of the subjects looking at the computer first and some going straight to the
patient to review the chief complaint and history of present illness.
Therefore, an ideal trigger point that the participants could use was not easily identified.
It was clear that an effective trigger point for this tool would need to occur before order onset,
but an ideal time was not as clear. This is due to the fast-paced and unpredictable nature of the
ED patient flow. If the trigger is placed during ordering, the physician has already chosen the
best course of action, has likely informed the patient of their decision, and is less likely to change
their management of the patient. However, it was also clear that an upstream trigger point was
likely to be too far removed from the physician’s clinical thinking and workflow, and may cause
“trigger fatigue”.
The refinements following the first round of usability testing included modification of
census trigger to account for patient assessment and the ability to auto-populate from past
medical history to address EHR clicking fatigue. From this round, it was noted that providers did
not use the tool until after they looked at the patient, and in most instances, they had already
made a clinical decision before they saw the PE tool.
Paraphrase This Document
Need a fresh take? Get an instant paraphrase of this document with our AI Paraphraser
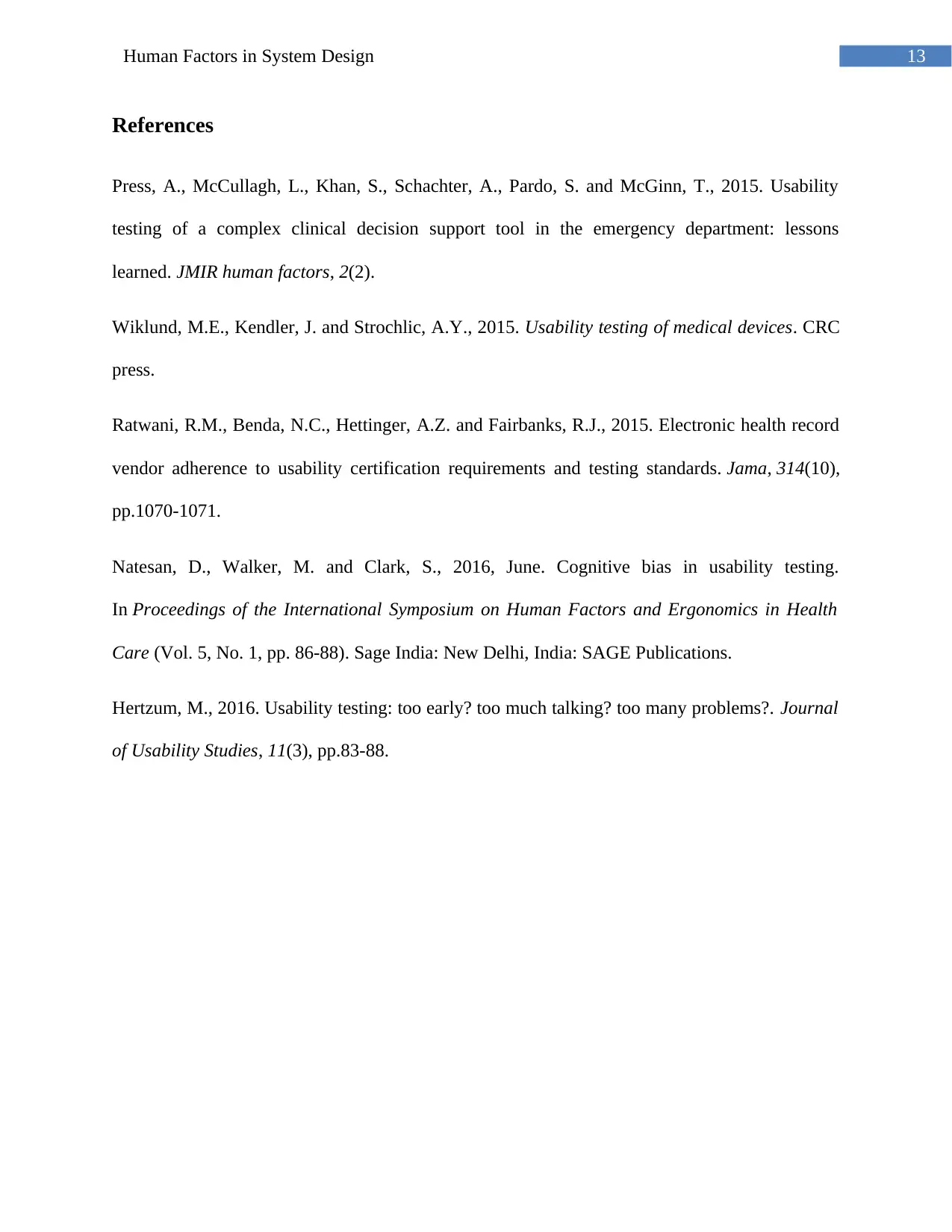
13Human Factors in System Design
References
Press, A., McCullagh, L., Khan, S., Schachter, A., Pardo, S. and McGinn, T., 2015. Usability
testing of a complex clinical decision support tool in the emergency department: lessons
learned. JMIR human factors, 2(2).
Wiklund, M.E., Kendler, J. and Strochlic, A.Y., 2015. Usability testing of medical devices. CRC
press.
Ratwani, R.M., Benda, N.C., Hettinger, A.Z. and Fairbanks, R.J., 2015. Electronic health record
vendor adherence to usability certification requirements and testing standards. Jama, 314(10),
pp.1070-1071.
Natesan, D., Walker, M. and Clark, S., 2016, June. Cognitive bias in usability testing.
In Proceedings of the International Symposium on Human Factors and Ergonomics in Health
Care (Vol. 5, No. 1, pp. 86-88). Sage India: New Delhi, India: SAGE Publications.
Hertzum, M., 2016. Usability testing: too early? too much talking? too many problems?. Journal
of Usability Studies, 11(3), pp.83-88.
References
Press, A., McCullagh, L., Khan, S., Schachter, A., Pardo, S. and McGinn, T., 2015. Usability
testing of a complex clinical decision support tool in the emergency department: lessons
learned. JMIR human factors, 2(2).
Wiklund, M.E., Kendler, J. and Strochlic, A.Y., 2015. Usability testing of medical devices. CRC
press.
Ratwani, R.M., Benda, N.C., Hettinger, A.Z. and Fairbanks, R.J., 2015. Electronic health record
vendor adherence to usability certification requirements and testing standards. Jama, 314(10),
pp.1070-1071.
Natesan, D., Walker, M. and Clark, S., 2016, June. Cognitive bias in usability testing.
In Proceedings of the International Symposium on Human Factors and Ergonomics in Health
Care (Vol. 5, No. 1, pp. 86-88). Sage India: New Delhi, India: SAGE Publications.
Hertzum, M., 2016. Usability testing: too early? too much talking? too many problems?. Journal
of Usability Studies, 11(3), pp.83-88.
1 out of 14
Related Documents
![[object Object]](/_next/image/?url=%2F_next%2Fstatic%2Fmedia%2Flogo.6d15ce61.png&w=640&q=75)
Your All-in-One AI-Powered Toolkit for Academic Success.
+13062052269
info@desklib.com
Available 24*7 on WhatsApp / Email
Unlock your academic potential
© 2024 | Zucol Services PVT LTD | All rights reserved.