Importance of Electronic Health Records in Healthcare Sector
VerifiedAdded on 2023/06/07
|28
|4428
|345
AI Summary
This article discusses the importance of electronic health records in the healthcare sector, including the significance of privacy, changes in the electronic health record system, and the top 5 variables for predicting loan delinquency. It also includes an exploratory data analysis of the loan.delinq.csv dataset.
Contribute Materials
Your contribution can guide someone’s learning journey. Share your
documents today.
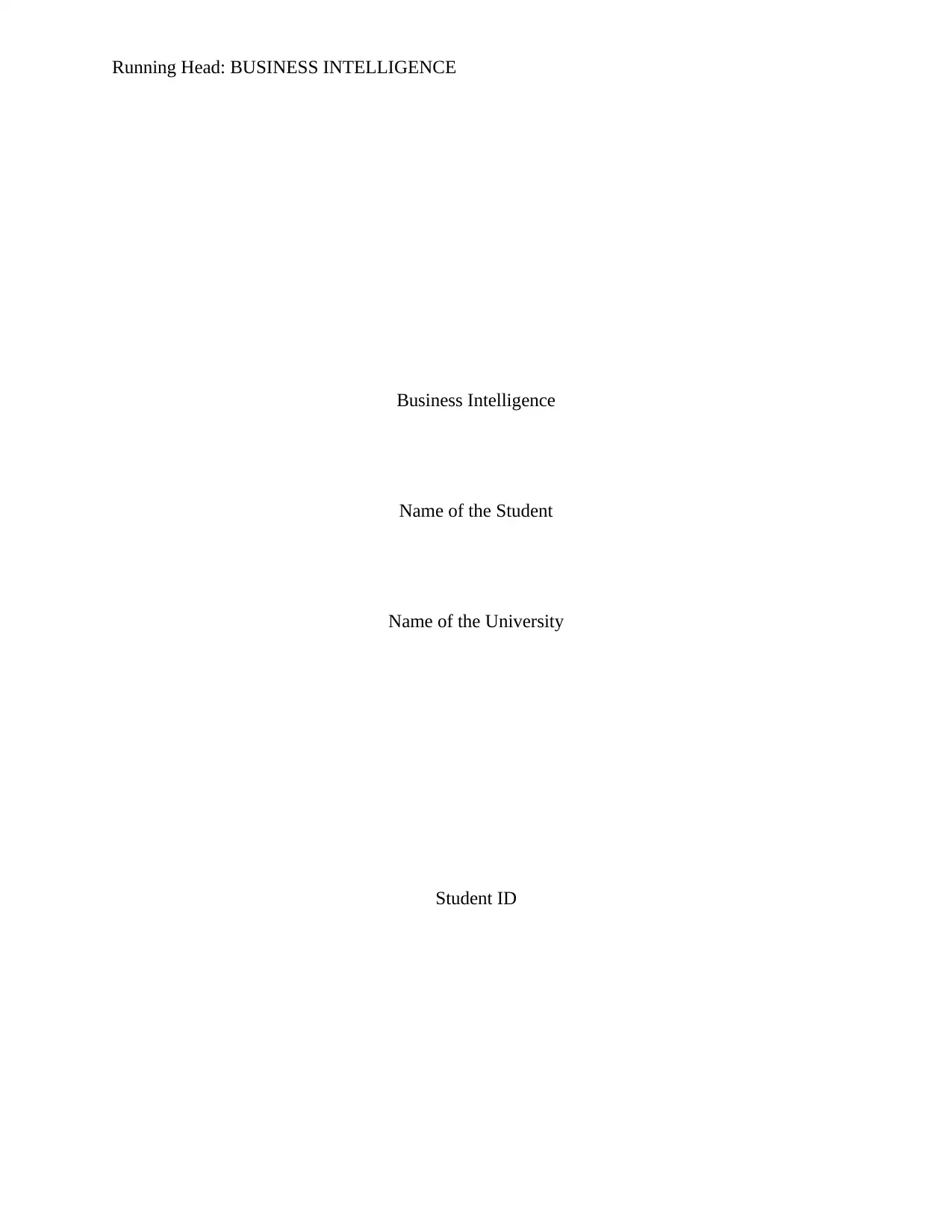
Running Head: BUSINESS INTELLIGENCE
Business Intelligence
Name of the Student
Name of the University
Student ID
Business Intelligence
Name of the Student
Name of the University
Student ID
Secure Best Marks with AI Grader
Need help grading? Try our AI Grader for instant feedback on your assignments.
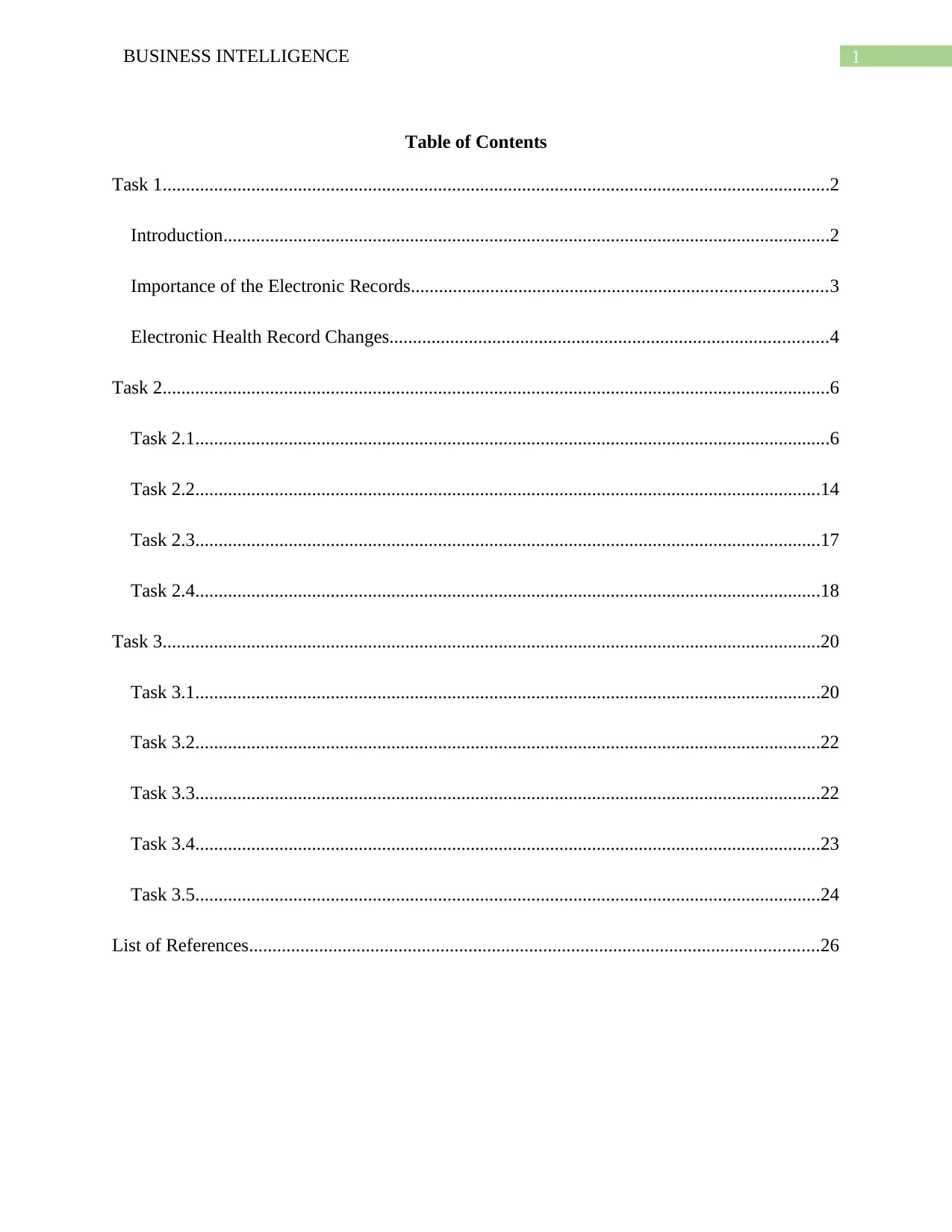
1BUSINESS INTELLIGENCE
Table of Contents
Task 1...............................................................................................................................................2
Introduction..................................................................................................................................2
Importance of the Electronic Records.........................................................................................3
Electronic Health Record Changes..............................................................................................4
Task 2...............................................................................................................................................6
Task 2.1........................................................................................................................................6
Task 2.2......................................................................................................................................14
Task 2.3......................................................................................................................................17
Task 2.4......................................................................................................................................18
Task 3.............................................................................................................................................20
Task 3.1......................................................................................................................................20
Task 3.2......................................................................................................................................22
Task 3.3......................................................................................................................................22
Task 3.4......................................................................................................................................23
Task 3.5......................................................................................................................................24
List of References..........................................................................................................................26
Table of Contents
Task 1...............................................................................................................................................2
Introduction..................................................................................................................................2
Importance of the Electronic Records.........................................................................................3
Electronic Health Record Changes..............................................................................................4
Task 2...............................................................................................................................................6
Task 2.1........................................................................................................................................6
Task 2.2......................................................................................................................................14
Task 2.3......................................................................................................................................17
Task 2.4......................................................................................................................................18
Task 3.............................................................................................................................................20
Task 3.1......................................................................................................................................20
Task 3.2......................................................................................................................................22
Task 3.3......................................................................................................................................22
Task 3.4......................................................................................................................................23
Task 3.5......................................................................................................................................24
List of References..........................................................................................................................26
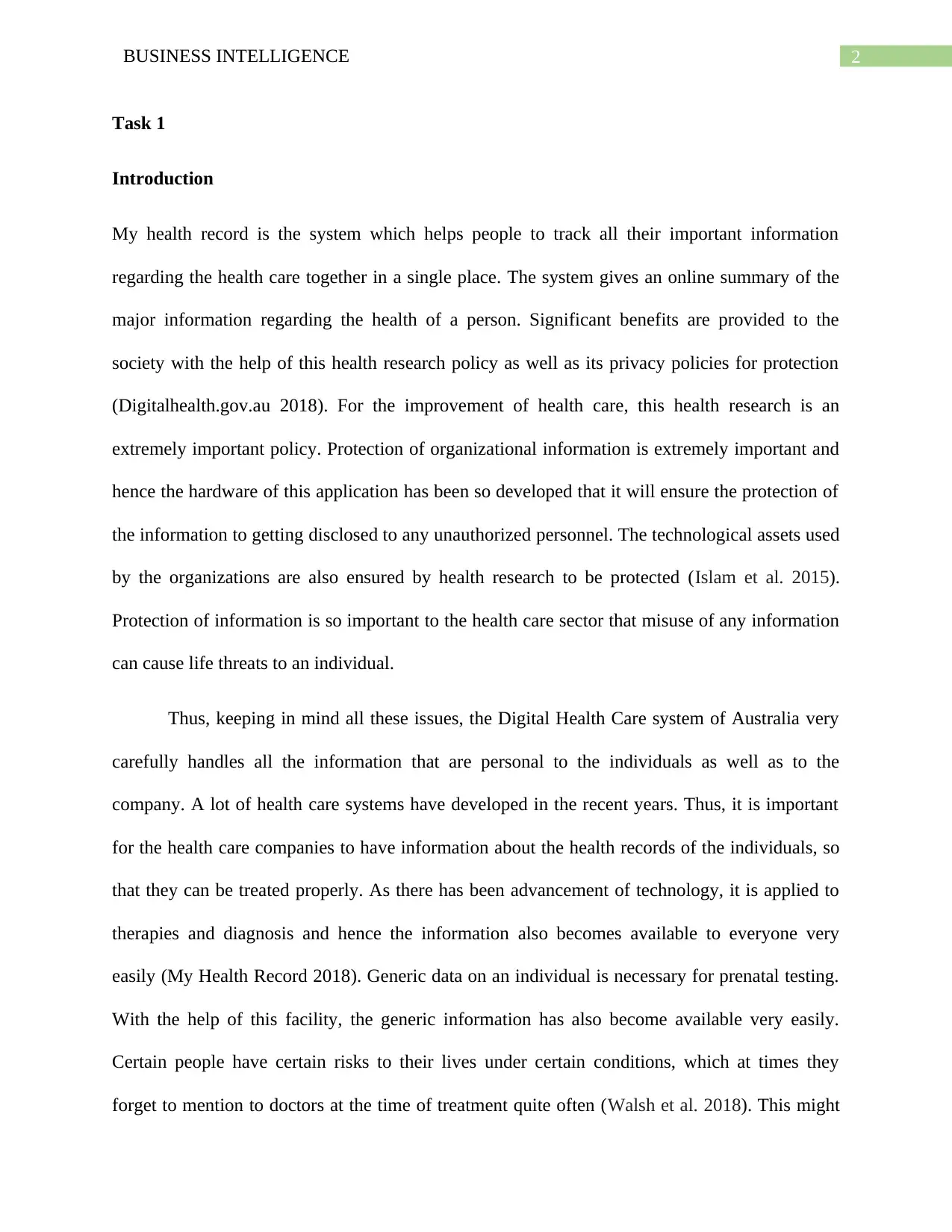
2BUSINESS INTELLIGENCE
Task 1
Introduction
My health record is the system which helps people to track all their important information
regarding the health care together in a single place. The system gives an online summary of the
major information regarding the health of a person. Significant benefits are provided to the
society with the help of this health research policy as well as its privacy policies for protection
(Digitalhealth.gov.au 2018). For the improvement of health care, this health research is an
extremely important policy. Protection of organizational information is extremely important and
hence the hardware of this application has been so developed that it will ensure the protection of
the information to getting disclosed to any unauthorized personnel. The technological assets used
by the organizations are also ensured by health research to be protected (Islam et al. 2015).
Protection of information is so important to the health care sector that misuse of any information
can cause life threats to an individual.
Thus, keeping in mind all these issues, the Digital Health Care system of Australia very
carefully handles all the information that are personal to the individuals as well as to the
company. A lot of health care systems have developed in the recent years. Thus, it is important
for the health care companies to have information about the health records of the individuals, so
that they can be treated properly. As there has been advancement of technology, it is applied to
therapies and diagnosis and hence the information also becomes available to everyone very
easily (My Health Record 2018). Generic data on an individual is necessary for prenatal testing.
With the help of this facility, the generic information has also become available very easily.
Certain people have certain risks to their lives under certain conditions, which at times they
forget to mention to doctors at the time of treatment quite often (Walsh et al. 2018). This might
Task 1
Introduction
My health record is the system which helps people to track all their important information
regarding the health care together in a single place. The system gives an online summary of the
major information regarding the health of a person. Significant benefits are provided to the
society with the help of this health research policy as well as its privacy policies for protection
(Digitalhealth.gov.au 2018). For the improvement of health care, this health research is an
extremely important policy. Protection of organizational information is extremely important and
hence the hardware of this application has been so developed that it will ensure the protection of
the information to getting disclosed to any unauthorized personnel. The technological assets used
by the organizations are also ensured by health research to be protected (Islam et al. 2015).
Protection of information is so important to the health care sector that misuse of any information
can cause life threats to an individual.
Thus, keeping in mind all these issues, the Digital Health Care system of Australia very
carefully handles all the information that are personal to the individuals as well as to the
company. A lot of health care systems have developed in the recent years. Thus, it is important
for the health care companies to have information about the health records of the individuals, so
that they can be treated properly. As there has been advancement of technology, it is applied to
therapies and diagnosis and hence the information also becomes available to everyone very
easily (My Health Record 2018). Generic data on an individual is necessary for prenatal testing.
With the help of this facility, the generic information has also become available very easily.
Certain people have certain risks to their lives under certain conditions, which at times they
forget to mention to doctors at the time of treatment quite often (Walsh et al. 2018). This might
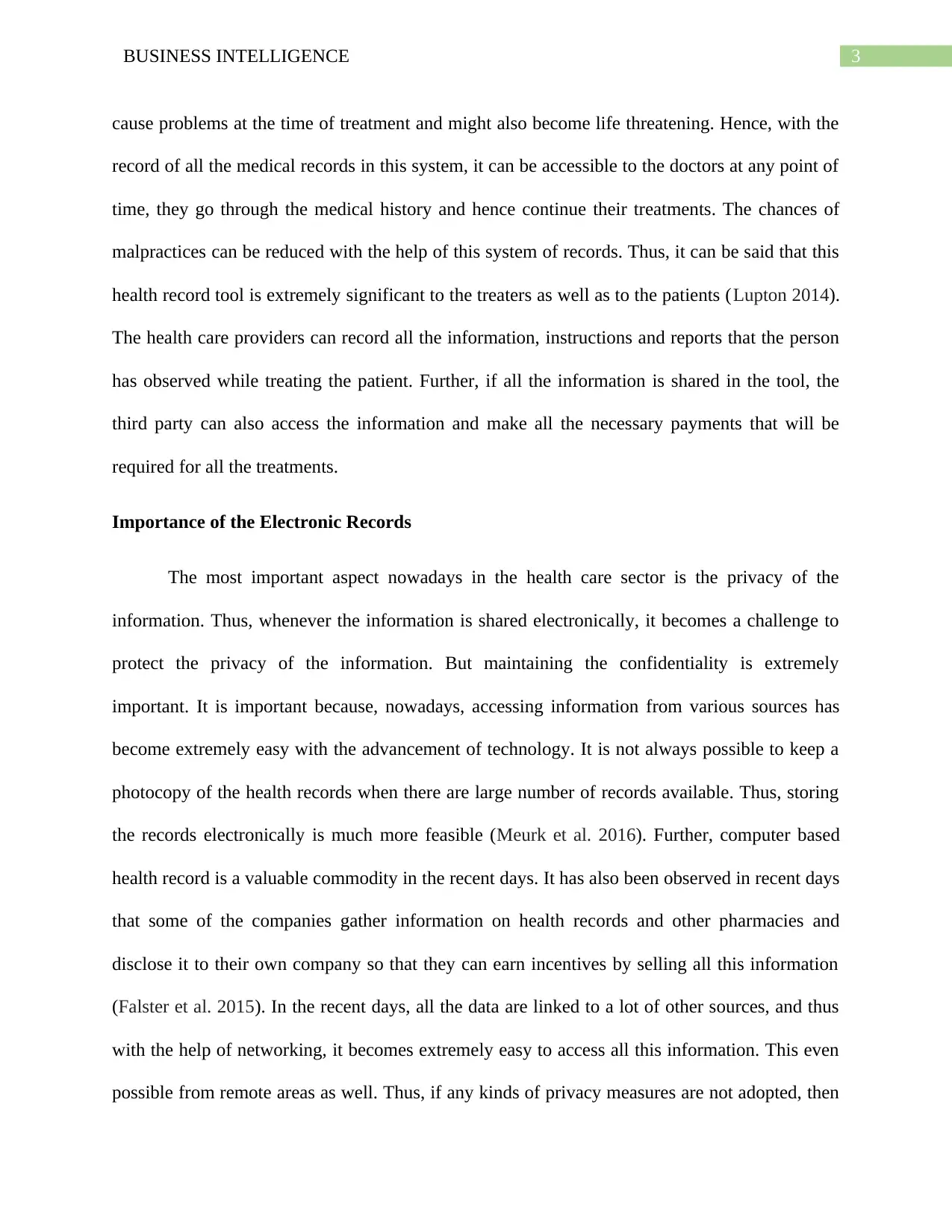
3BUSINESS INTELLIGENCE
cause problems at the time of treatment and might also become life threatening. Hence, with the
record of all the medical records in this system, it can be accessible to the doctors at any point of
time, they go through the medical history and hence continue their treatments. The chances of
malpractices can be reduced with the help of this system of records. Thus, it can be said that this
health record tool is extremely significant to the treaters as well as to the patients (Lupton 2014).
The health care providers can record all the information, instructions and reports that the person
has observed while treating the patient. Further, if all the information is shared in the tool, the
third party can also access the information and make all the necessary payments that will be
required for all the treatments.
Importance of the Electronic Records
The most important aspect nowadays in the health care sector is the privacy of the
information. Thus, whenever the information is shared electronically, it becomes a challenge to
protect the privacy of the information. But maintaining the confidentiality is extremely
important. It is important because, nowadays, accessing information from various sources has
become extremely easy with the advancement of technology. It is not always possible to keep a
photocopy of the health records when there are large number of records available. Thus, storing
the records electronically is much more feasible (Meurk et al. 2016). Further, computer based
health record is a valuable commodity in the recent days. It has also been observed in recent days
that some of the companies gather information on health records and other pharmacies and
disclose it to their own company so that they can earn incentives by selling all this information
(Falster et al. 2015). In the recent days, all the data are linked to a lot of other sources, and thus
with the help of networking, it becomes extremely easy to access all this information. This even
possible from remote areas as well. Thus, if any kinds of privacy measures are not adopted, then
cause problems at the time of treatment and might also become life threatening. Hence, with the
record of all the medical records in this system, it can be accessible to the doctors at any point of
time, they go through the medical history and hence continue their treatments. The chances of
malpractices can be reduced with the help of this system of records. Thus, it can be said that this
health record tool is extremely significant to the treaters as well as to the patients (Lupton 2014).
The health care providers can record all the information, instructions and reports that the person
has observed while treating the patient. Further, if all the information is shared in the tool, the
third party can also access the information and make all the necessary payments that will be
required for all the treatments.
Importance of the Electronic Records
The most important aspect nowadays in the health care sector is the privacy of the
information. Thus, whenever the information is shared electronically, it becomes a challenge to
protect the privacy of the information. But maintaining the confidentiality is extremely
important. It is important because, nowadays, accessing information from various sources has
become extremely easy with the advancement of technology. It is not always possible to keep a
photocopy of the health records when there are large number of records available. Thus, storing
the records electronically is much more feasible (Meurk et al. 2016). Further, computer based
health record is a valuable commodity in the recent days. It has also been observed in recent days
that some of the companies gather information on health records and other pharmacies and
disclose it to their own company so that they can earn incentives by selling all this information
(Falster et al. 2015). In the recent days, all the data are linked to a lot of other sources, and thus
with the help of networking, it becomes extremely easy to access all this information. This even
possible from remote areas as well. Thus, if any kinds of privacy measures are not adopted, then
Secure Best Marks with AI Grader
Need help grading? Try our AI Grader for instant feedback on your assignments.
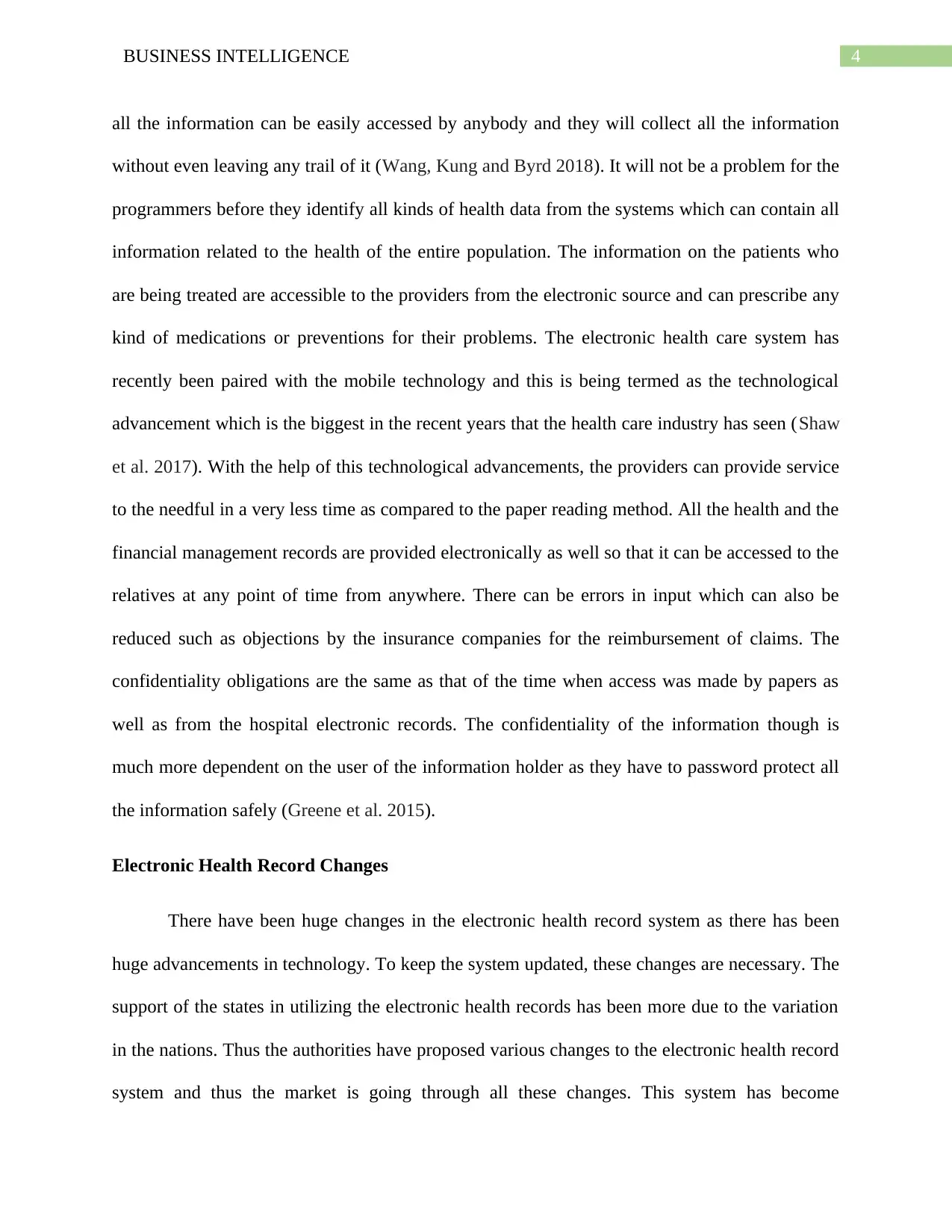
4BUSINESS INTELLIGENCE
all the information can be easily accessed by anybody and they will collect all the information
without even leaving any trail of it (Wang, Kung and Byrd 2018). It will not be a problem for the
programmers before they identify all kinds of health data from the systems which can contain all
information related to the health of the entire population. The information on the patients who
are being treated are accessible to the providers from the electronic source and can prescribe any
kind of medications or preventions for their problems. The electronic health care system has
recently been paired with the mobile technology and this is being termed as the technological
advancement which is the biggest in the recent years that the health care industry has seen (Shaw
et al. 2017). With the help of this technological advancements, the providers can provide service
to the needful in a very less time as compared to the paper reading method. All the health and the
financial management records are provided electronically as well so that it can be accessed to the
relatives at any point of time from anywhere. There can be errors in input which can also be
reduced such as objections by the insurance companies for the reimbursement of claims. The
confidentiality obligations are the same as that of the time when access was made by papers as
well as from the hospital electronic records. The confidentiality of the information though is
much more dependent on the user of the information holder as they have to password protect all
the information safely (Greene et al. 2015).
Electronic Health Record Changes
There have been huge changes in the electronic health record system as there has been
huge advancements in technology. To keep the system updated, these changes are necessary. The
support of the states in utilizing the electronic health records has been more due to the variation
in the nations. Thus the authorities have proposed various changes to the electronic health record
system and thus the market is going through all these changes. This system has become
all the information can be easily accessed by anybody and they will collect all the information
without even leaving any trail of it (Wang, Kung and Byrd 2018). It will not be a problem for the
programmers before they identify all kinds of health data from the systems which can contain all
information related to the health of the entire population. The information on the patients who
are being treated are accessible to the providers from the electronic source and can prescribe any
kind of medications or preventions for their problems. The electronic health care system has
recently been paired with the mobile technology and this is being termed as the technological
advancement which is the biggest in the recent years that the health care industry has seen (Shaw
et al. 2017). With the help of this technological advancements, the providers can provide service
to the needful in a very less time as compared to the paper reading method. All the health and the
financial management records are provided electronically as well so that it can be accessed to the
relatives at any point of time from anywhere. There can be errors in input which can also be
reduced such as objections by the insurance companies for the reimbursement of claims. The
confidentiality obligations are the same as that of the time when access was made by papers as
well as from the hospital electronic records. The confidentiality of the information though is
much more dependent on the user of the information holder as they have to password protect all
the information safely (Greene et al. 2015).
Electronic Health Record Changes
There have been huge changes in the electronic health record system as there has been
huge advancements in technology. To keep the system updated, these changes are necessary. The
support of the states in utilizing the electronic health records has been more due to the variation
in the nations. Thus the authorities have proposed various changes to the electronic health record
system and thus the market is going through all these changes. This system has become
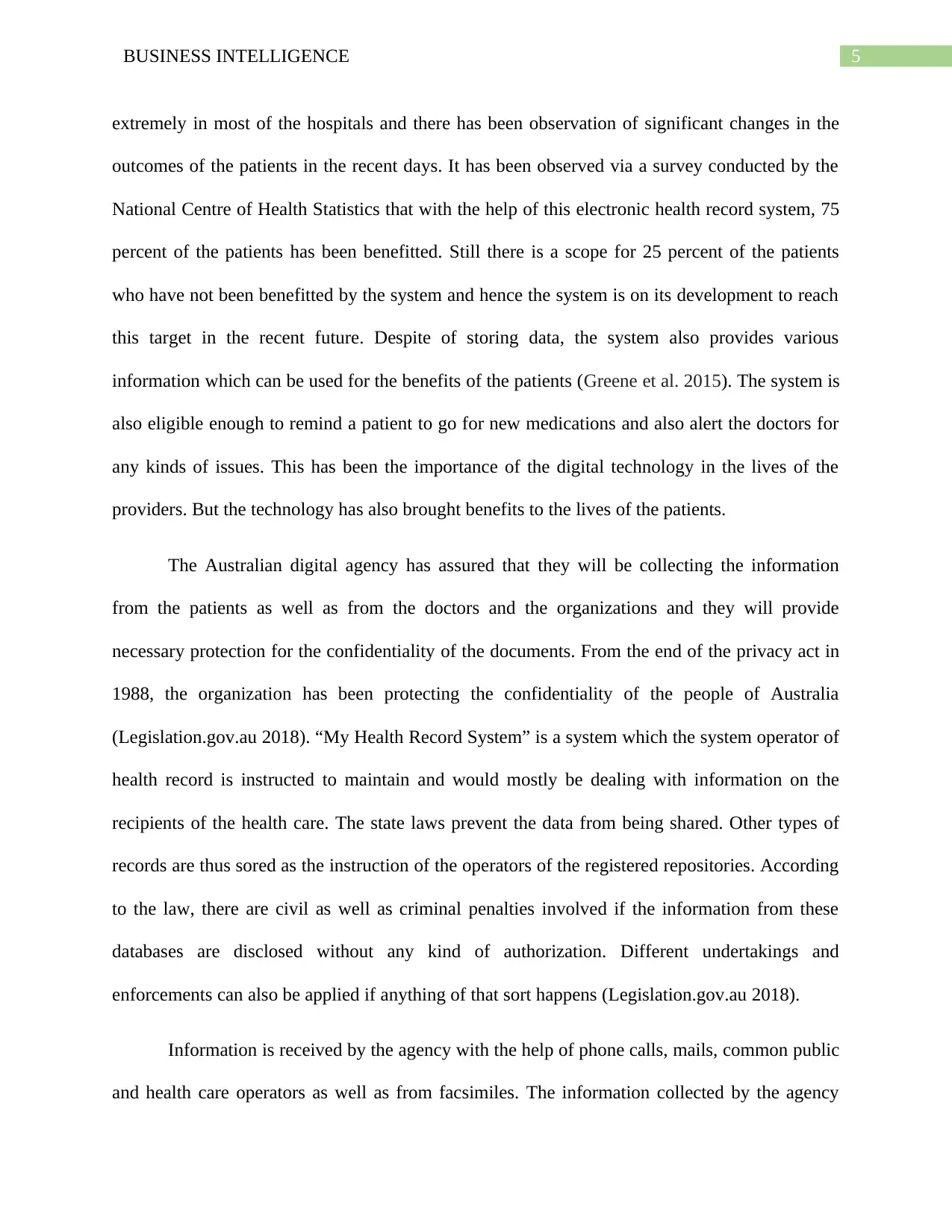
5BUSINESS INTELLIGENCE
extremely in most of the hospitals and there has been observation of significant changes in the
outcomes of the patients in the recent days. It has been observed via a survey conducted by the
National Centre of Health Statistics that with the help of this electronic health record system, 75
percent of the patients has been benefitted. Still there is a scope for 25 percent of the patients
who have not been benefitted by the system and hence the system is on its development to reach
this target in the recent future. Despite of storing data, the system also provides various
information which can be used for the benefits of the patients (Greene et al. 2015). The system is
also eligible enough to remind a patient to go for new medications and also alert the doctors for
any kinds of issues. This has been the importance of the digital technology in the lives of the
providers. But the technology has also brought benefits to the lives of the patients.
The Australian digital agency has assured that they will be collecting the information
from the patients as well as from the doctors and the organizations and they will provide
necessary protection for the confidentiality of the documents. From the end of the privacy act in
1988, the organization has been protecting the confidentiality of the people of Australia
(Legislation.gov.au 2018). “My Health Record System” is a system which the system operator of
health record is instructed to maintain and would mostly be dealing with information on the
recipients of the health care. The state laws prevent the data from being shared. Other types of
records are thus sored as the instruction of the operators of the registered repositories. According
to the law, there are civil as well as criminal penalties involved if the information from these
databases are disclosed without any kind of authorization. Different undertakings and
enforcements can also be applied if anything of that sort happens (Legislation.gov.au 2018).
Information is received by the agency with the help of phone calls, mails, common public
and health care operators as well as from facsimiles. The information collected by the agency
extremely in most of the hospitals and there has been observation of significant changes in the
outcomes of the patients in the recent days. It has been observed via a survey conducted by the
National Centre of Health Statistics that with the help of this electronic health record system, 75
percent of the patients has been benefitted. Still there is a scope for 25 percent of the patients
who have not been benefitted by the system and hence the system is on its development to reach
this target in the recent future. Despite of storing data, the system also provides various
information which can be used for the benefits of the patients (Greene et al. 2015). The system is
also eligible enough to remind a patient to go for new medications and also alert the doctors for
any kinds of issues. This has been the importance of the digital technology in the lives of the
providers. But the technology has also brought benefits to the lives of the patients.
The Australian digital agency has assured that they will be collecting the information
from the patients as well as from the doctors and the organizations and they will provide
necessary protection for the confidentiality of the documents. From the end of the privacy act in
1988, the organization has been protecting the confidentiality of the people of Australia
(Legislation.gov.au 2018). “My Health Record System” is a system which the system operator of
health record is instructed to maintain and would mostly be dealing with information on the
recipients of the health care. The state laws prevent the data from being shared. Other types of
records are thus sored as the instruction of the operators of the registered repositories. According
to the law, there are civil as well as criminal penalties involved if the information from these
databases are disclosed without any kind of authorization. Different undertakings and
enforcements can also be applied if anything of that sort happens (Legislation.gov.au 2018).
Information is received by the agency with the help of phone calls, mails, common public
and health care operators as well as from facsimiles. The information collected by the agency
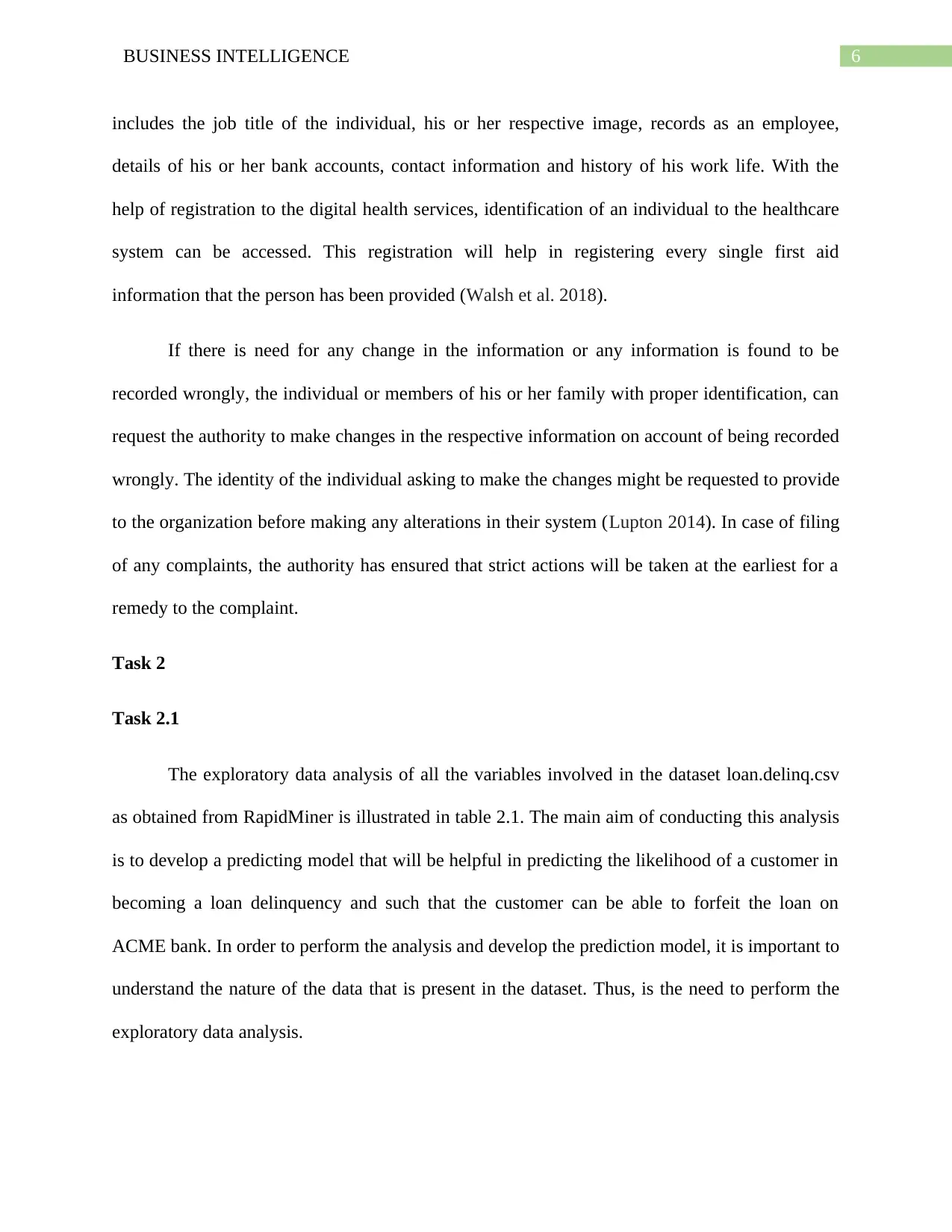
6BUSINESS INTELLIGENCE
includes the job title of the individual, his or her respective image, records as an employee,
details of his or her bank accounts, contact information and history of his work life. With the
help of registration to the digital health services, identification of an individual to the healthcare
system can be accessed. This registration will help in registering every single first aid
information that the person has been provided (Walsh et al. 2018).
If there is need for any change in the information or any information is found to be
recorded wrongly, the individual or members of his or her family with proper identification, can
request the authority to make changes in the respective information on account of being recorded
wrongly. The identity of the individual asking to make the changes might be requested to provide
to the organization before making any alterations in their system (Lupton 2014). In case of filing
of any complaints, the authority has ensured that strict actions will be taken at the earliest for a
remedy to the complaint.
Task 2
Task 2.1
The exploratory data analysis of all the variables involved in the dataset loan.delinq.csv
as obtained from RapidMiner is illustrated in table 2.1. The main aim of conducting this analysis
is to develop a predicting model that will be helpful in predicting the likelihood of a customer in
becoming a loan delinquency and such that the customer can be able to forfeit the loan on
ACME bank. In order to perform the analysis and develop the prediction model, it is important to
understand the nature of the data that is present in the dataset. Thus, is the need to perform the
exploratory data analysis.
includes the job title of the individual, his or her respective image, records as an employee,
details of his or her bank accounts, contact information and history of his work life. With the
help of registration to the digital health services, identification of an individual to the healthcare
system can be accessed. This registration will help in registering every single first aid
information that the person has been provided (Walsh et al. 2018).
If there is need for any change in the information or any information is found to be
recorded wrongly, the individual or members of his or her family with proper identification, can
request the authority to make changes in the respective information on account of being recorded
wrongly. The identity of the individual asking to make the changes might be requested to provide
to the organization before making any alterations in their system (Lupton 2014). In case of filing
of any complaints, the authority has ensured that strict actions will be taken at the earliest for a
remedy to the complaint.
Task 2
Task 2.1
The exploratory data analysis of all the variables involved in the dataset loan.delinq.csv
as obtained from RapidMiner is illustrated in table 2.1. The main aim of conducting this analysis
is to develop a predicting model that will be helpful in predicting the likelihood of a customer in
becoming a loan delinquency and such that the customer can be able to forfeit the loan on
ACME bank. In order to perform the analysis and develop the prediction model, it is important to
understand the nature of the data that is present in the dataset. Thus, is the need to perform the
exploratory data analysis.
Paraphrase This Document
Need a fresh take? Get an instant paraphrase of this document with our AI Paraphraser
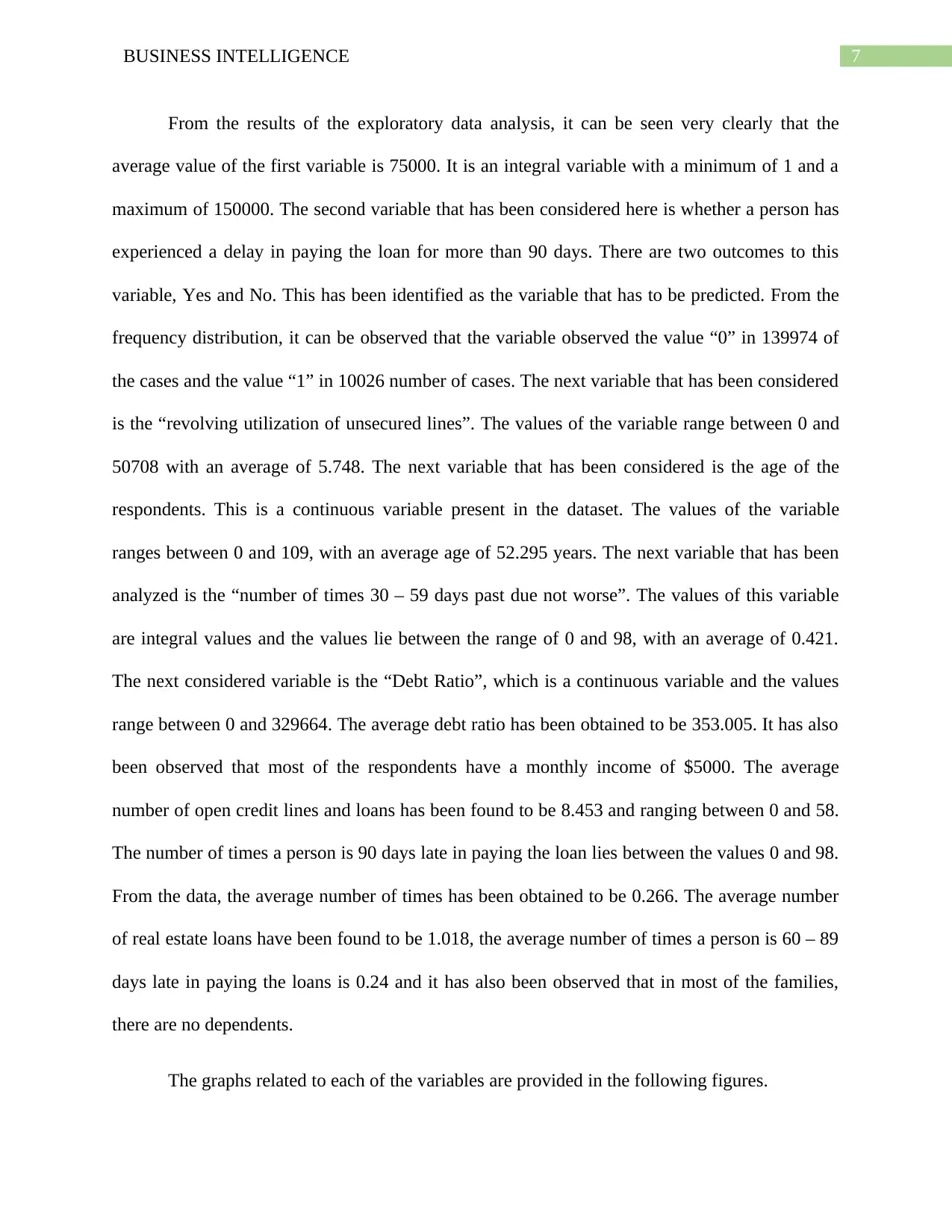
7BUSINESS INTELLIGENCE
From the results of the exploratory data analysis, it can be seen very clearly that the
average value of the first variable is 75000. It is an integral variable with a minimum of 1 and a
maximum of 150000. The second variable that has been considered here is whether a person has
experienced a delay in paying the loan for more than 90 days. There are two outcomes to this
variable, Yes and No. This has been identified as the variable that has to be predicted. From the
frequency distribution, it can be observed that the variable observed the value “0” in 139974 of
the cases and the value “1” in 10026 number of cases. The next variable that has been considered
is the “revolving utilization of unsecured lines”. The values of the variable range between 0 and
50708 with an average of 5.748. The next variable that has been considered is the age of the
respondents. This is a continuous variable present in the dataset. The values of the variable
ranges between 0 and 109, with an average age of 52.295 years. The next variable that has been
analyzed is the “number of times 30 – 59 days past due not worse”. The values of this variable
are integral values and the values lie between the range of 0 and 98, with an average of 0.421.
The next considered variable is the “Debt Ratio”, which is a continuous variable and the values
range between 0 and 329664. The average debt ratio has been obtained to be 353.005. It has also
been observed that most of the respondents have a monthly income of $5000. The average
number of open credit lines and loans has been found to be 8.453 and ranging between 0 and 58.
The number of times a person is 90 days late in paying the loan lies between the values 0 and 98.
From the data, the average number of times has been obtained to be 0.266. The average number
of real estate loans have been found to be 1.018, the average number of times a person is 60 – 89
days late in paying the loans is 0.24 and it has also been observed that in most of the families,
there are no dependents.
The graphs related to each of the variables are provided in the following figures.
From the results of the exploratory data analysis, it can be seen very clearly that the
average value of the first variable is 75000. It is an integral variable with a minimum of 1 and a
maximum of 150000. The second variable that has been considered here is whether a person has
experienced a delay in paying the loan for more than 90 days. There are two outcomes to this
variable, Yes and No. This has been identified as the variable that has to be predicted. From the
frequency distribution, it can be observed that the variable observed the value “0” in 139974 of
the cases and the value “1” in 10026 number of cases. The next variable that has been considered
is the “revolving utilization of unsecured lines”. The values of the variable range between 0 and
50708 with an average of 5.748. The next variable that has been considered is the age of the
respondents. This is a continuous variable present in the dataset. The values of the variable
ranges between 0 and 109, with an average age of 52.295 years. The next variable that has been
analyzed is the “number of times 30 – 59 days past due not worse”. The values of this variable
are integral values and the values lie between the range of 0 and 98, with an average of 0.421.
The next considered variable is the “Debt Ratio”, which is a continuous variable and the values
range between 0 and 329664. The average debt ratio has been obtained to be 353.005. It has also
been observed that most of the respondents have a monthly income of $5000. The average
number of open credit lines and loans has been found to be 8.453 and ranging between 0 and 58.
The number of times a person is 90 days late in paying the loan lies between the values 0 and 98.
From the data, the average number of times has been obtained to be 0.266. The average number
of real estate loans have been found to be 1.018, the average number of times a person is 60 – 89
days late in paying the loans is 0.24 and it has also been observed that in most of the families,
there are no dependents.
The graphs related to each of the variables are provided in the following figures.
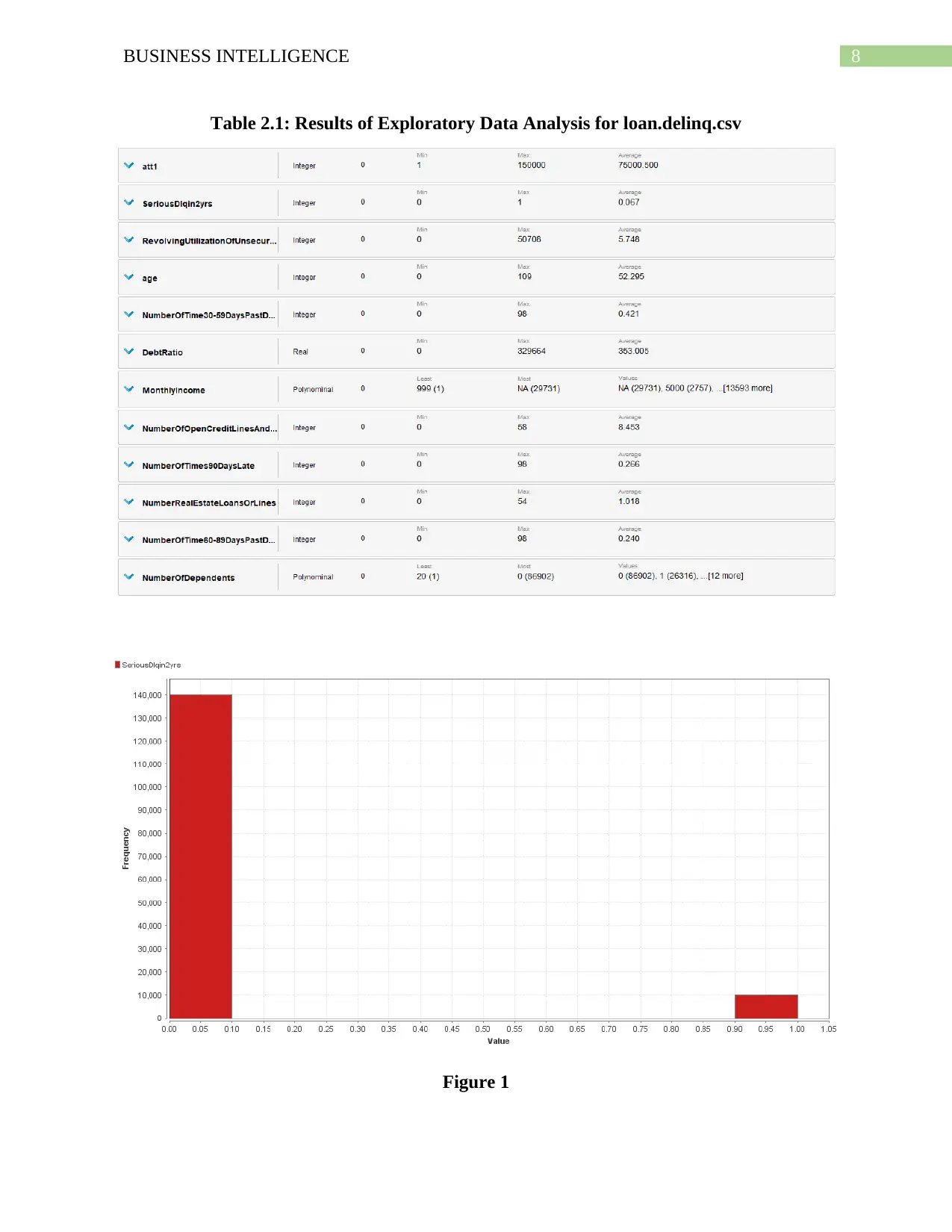
8BUSINESS INTELLIGENCE
Table 2.1: Results of Exploratory Data Analysis for loan.delinq.csv
Figure 1
Table 2.1: Results of Exploratory Data Analysis for loan.delinq.csv
Figure 1
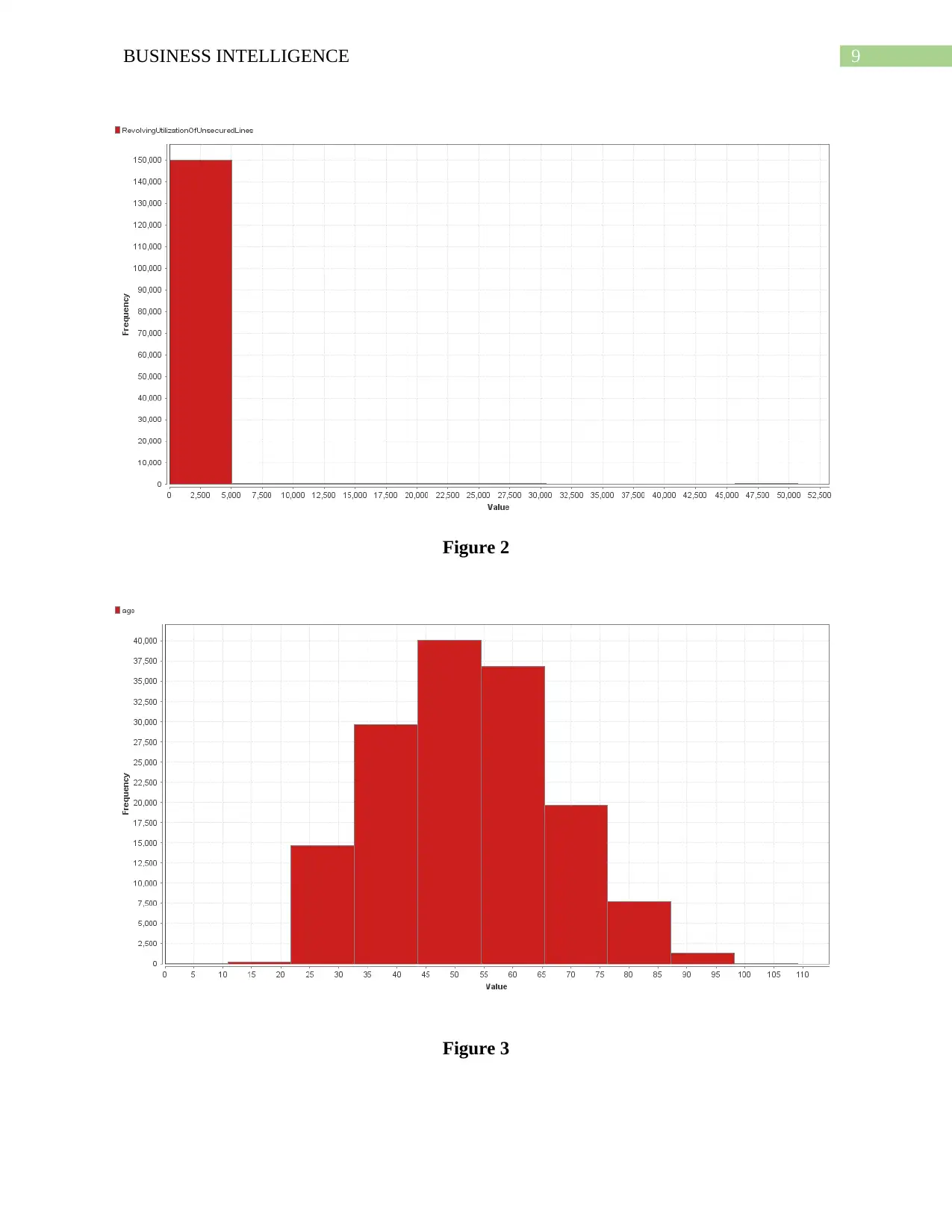
9BUSINESS INTELLIGENCE
Figure 2
Figure 3
Figure 2
Figure 3
Secure Best Marks with AI Grader
Need help grading? Try our AI Grader for instant feedback on your assignments.
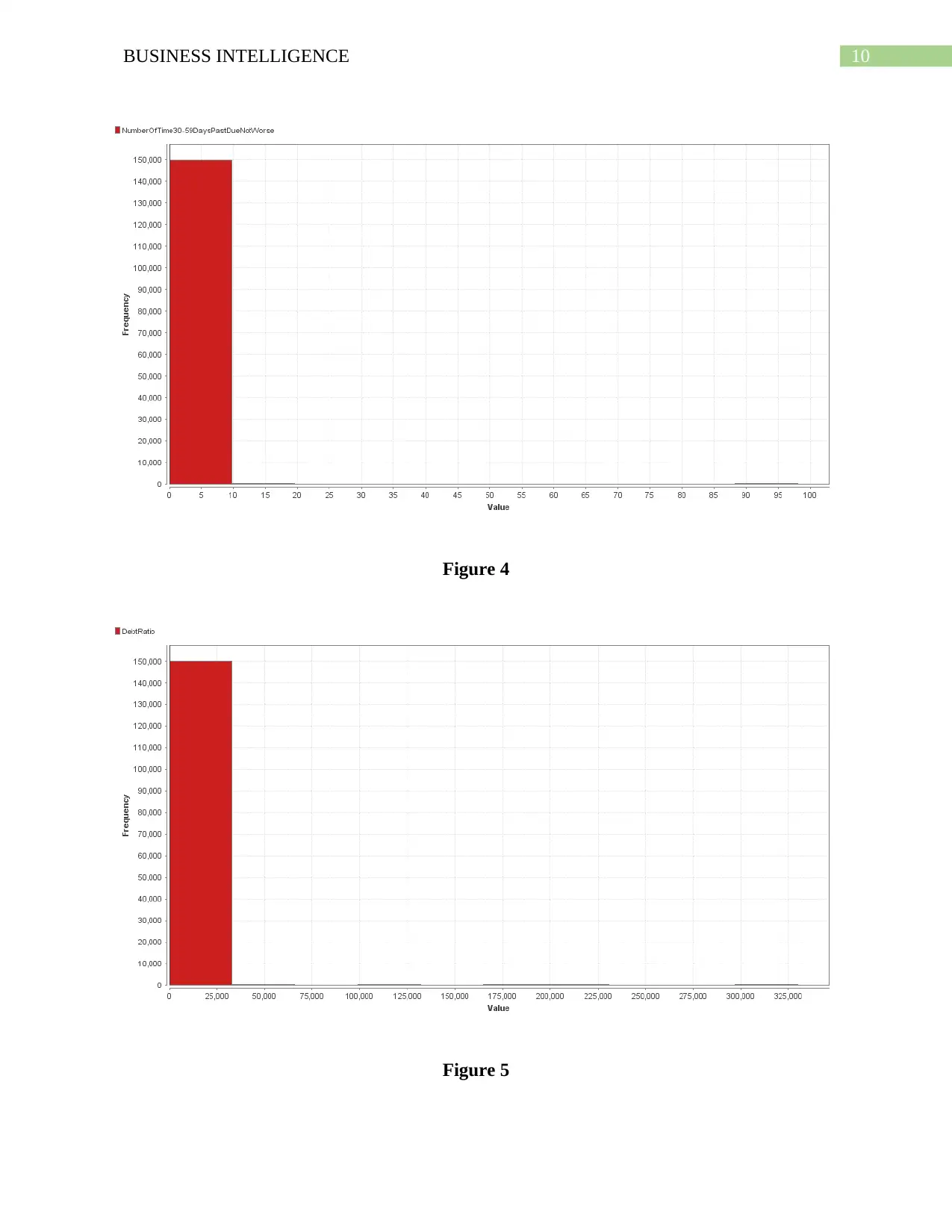
10BUSINESS INTELLIGENCE
Figure 4
Figure 5
Figure 4
Figure 5
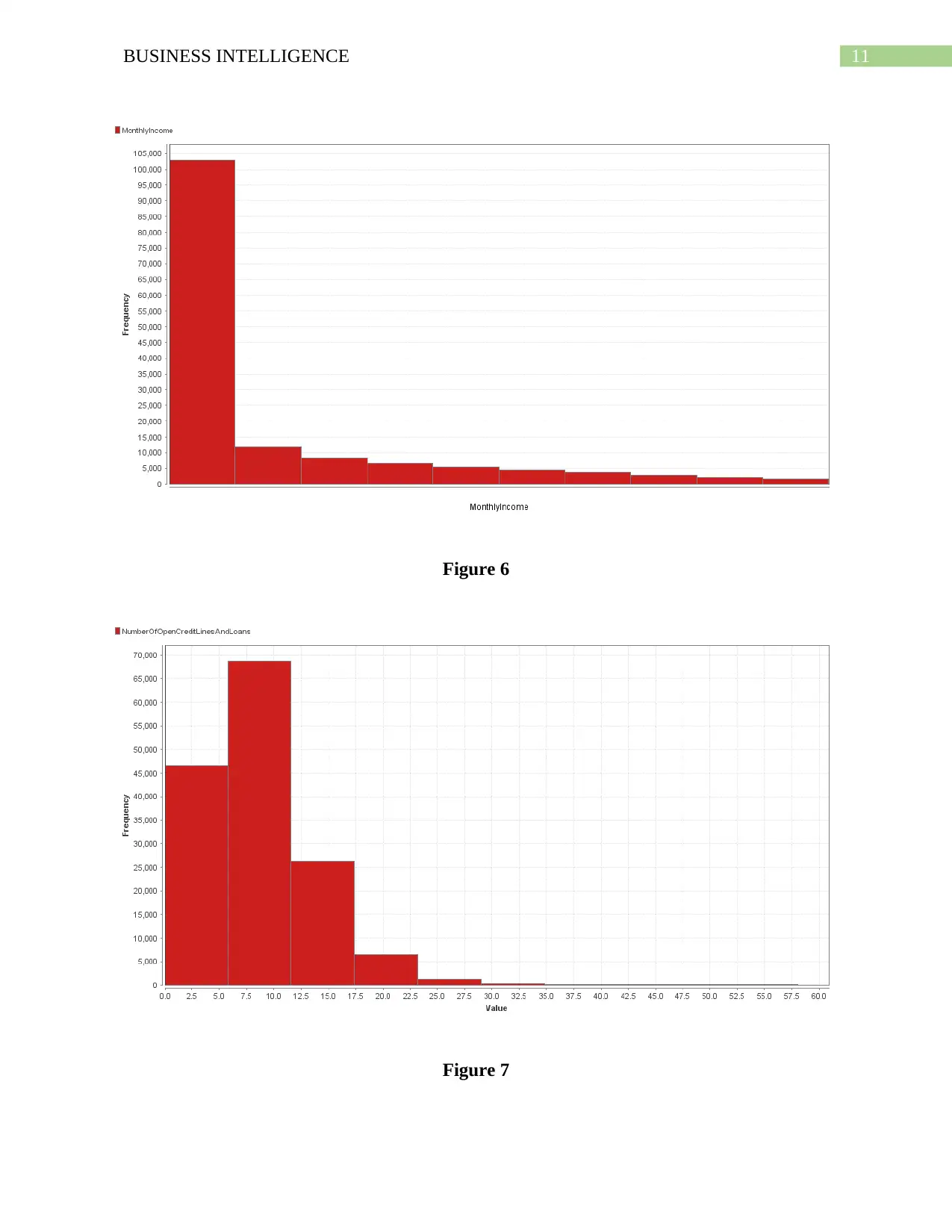
11BUSINESS INTELLIGENCE
Figure 6
Figure 7
Figure 6
Figure 7
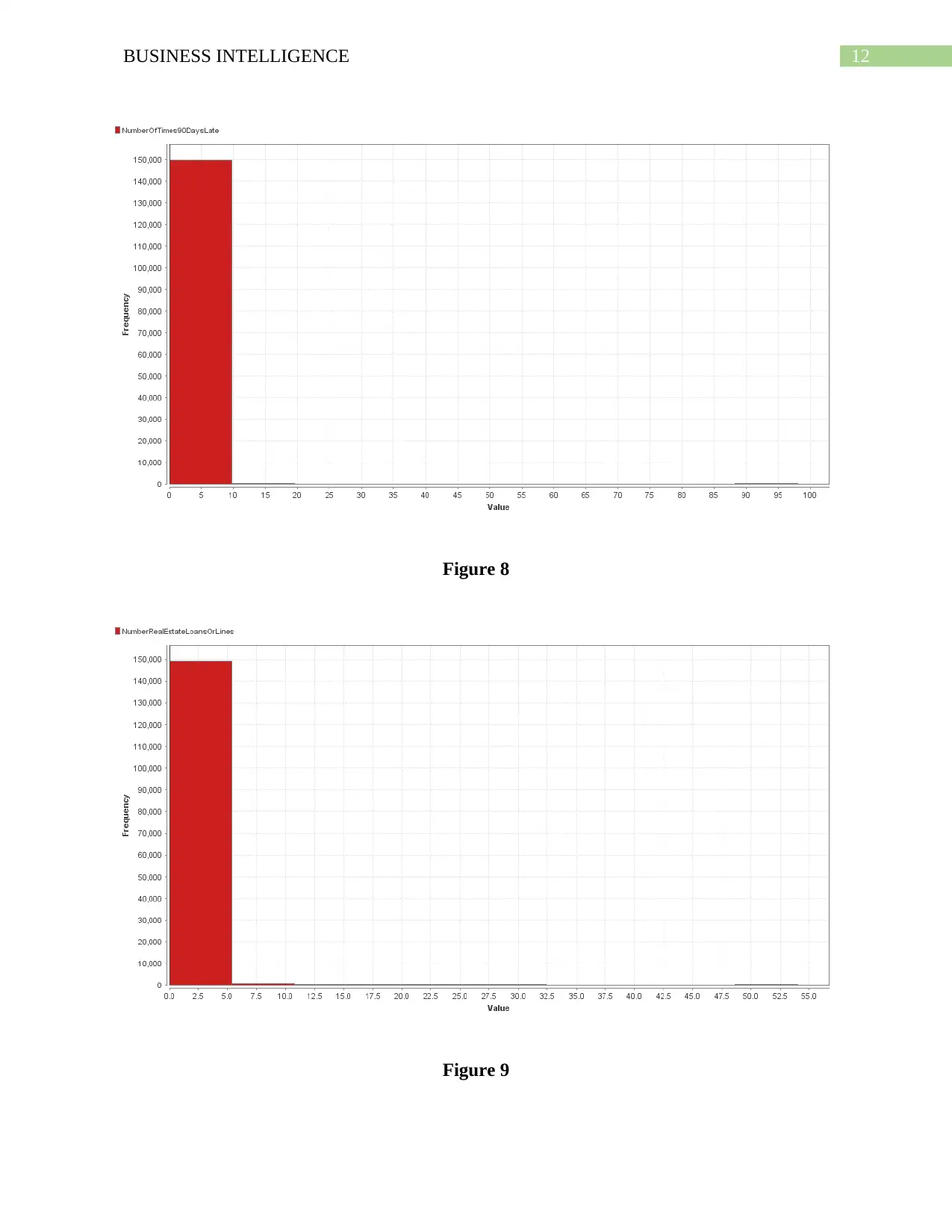
12BUSINESS INTELLIGENCE
Figure 8
Figure 9
Figure 8
Figure 9
Paraphrase This Document
Need a fresh take? Get an instant paraphrase of this document with our AI Paraphraser
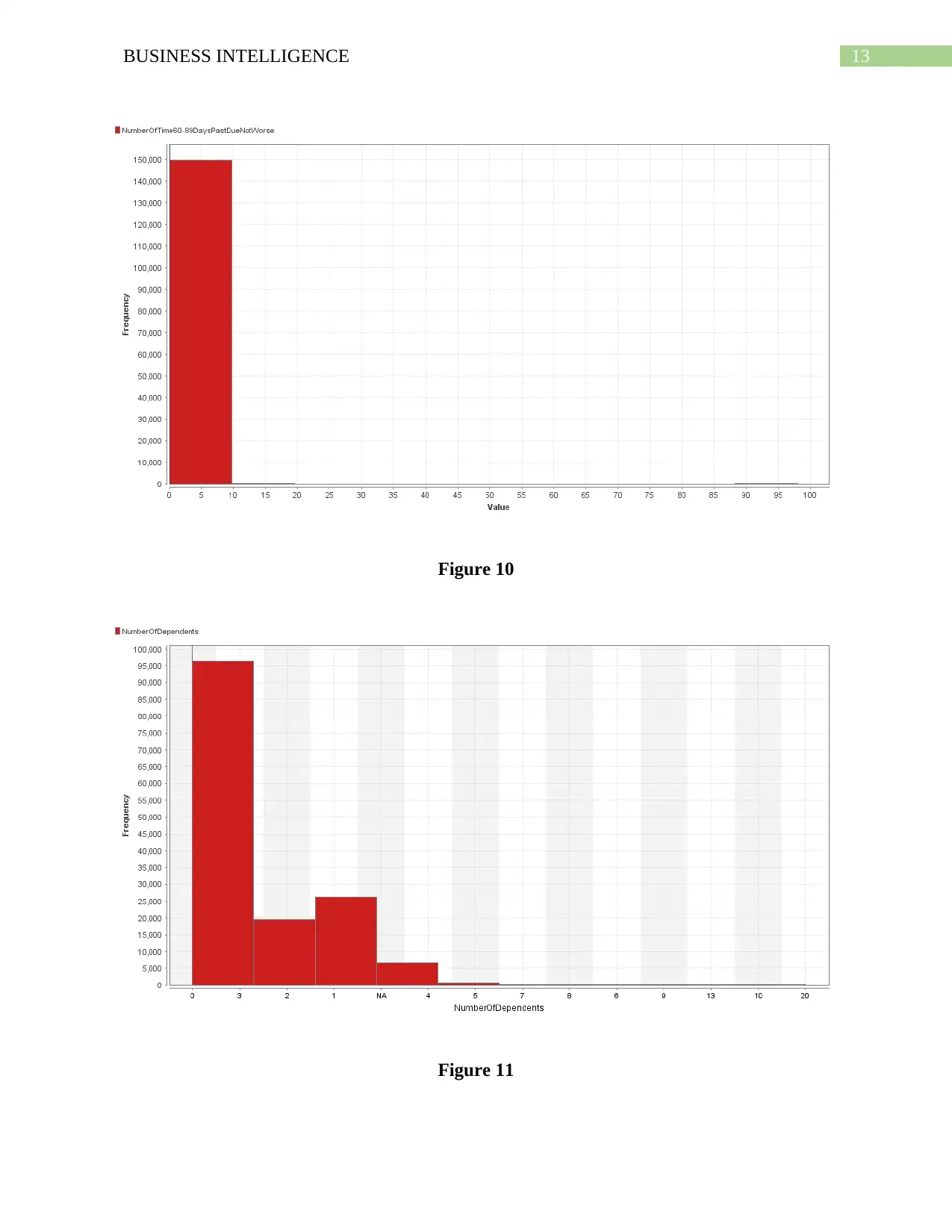
13BUSINESS INTELLIGENCE
Figure 10
Figure 11
Figure 10
Figure 11
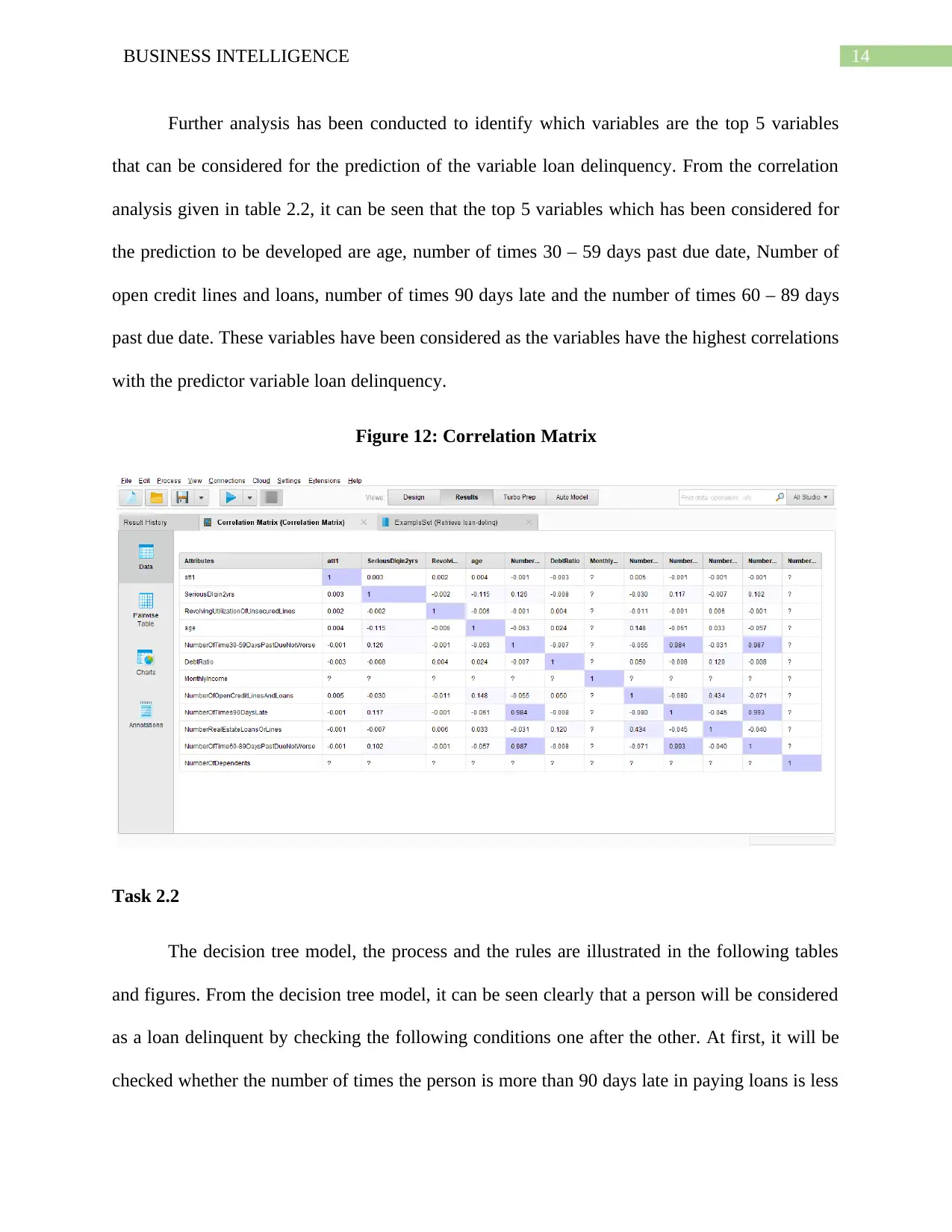
14BUSINESS INTELLIGENCE
Further analysis has been conducted to identify which variables are the top 5 variables
that can be considered for the prediction of the variable loan delinquency. From the correlation
analysis given in table 2.2, it can be seen that the top 5 variables which has been considered for
the prediction to be developed are age, number of times 30 – 59 days past due date, Number of
open credit lines and loans, number of times 90 days late and the number of times 60 – 89 days
past due date. These variables have been considered as the variables have the highest correlations
with the predictor variable loan delinquency.
Figure 12: Correlation Matrix
Task 2.2
The decision tree model, the process and the rules are illustrated in the following tables
and figures. From the decision tree model, it can be seen clearly that a person will be considered
as a loan delinquent by checking the following conditions one after the other. At first, it will be
checked whether the number of times the person is more than 90 days late in paying loans is less
Further analysis has been conducted to identify which variables are the top 5 variables
that can be considered for the prediction of the variable loan delinquency. From the correlation
analysis given in table 2.2, it can be seen that the top 5 variables which has been considered for
the prediction to be developed are age, number of times 30 – 59 days past due date, Number of
open credit lines and loans, number of times 90 days late and the number of times 60 – 89 days
past due date. These variables have been considered as the variables have the highest correlations
with the predictor variable loan delinquency.
Figure 12: Correlation Matrix
Task 2.2
The decision tree model, the process and the rules are illustrated in the following tables
and figures. From the decision tree model, it can be seen clearly that a person will be considered
as a loan delinquent by checking the following conditions one after the other. At first, it will be
checked whether the number of times the person is more than 90 days late in paying loans is less
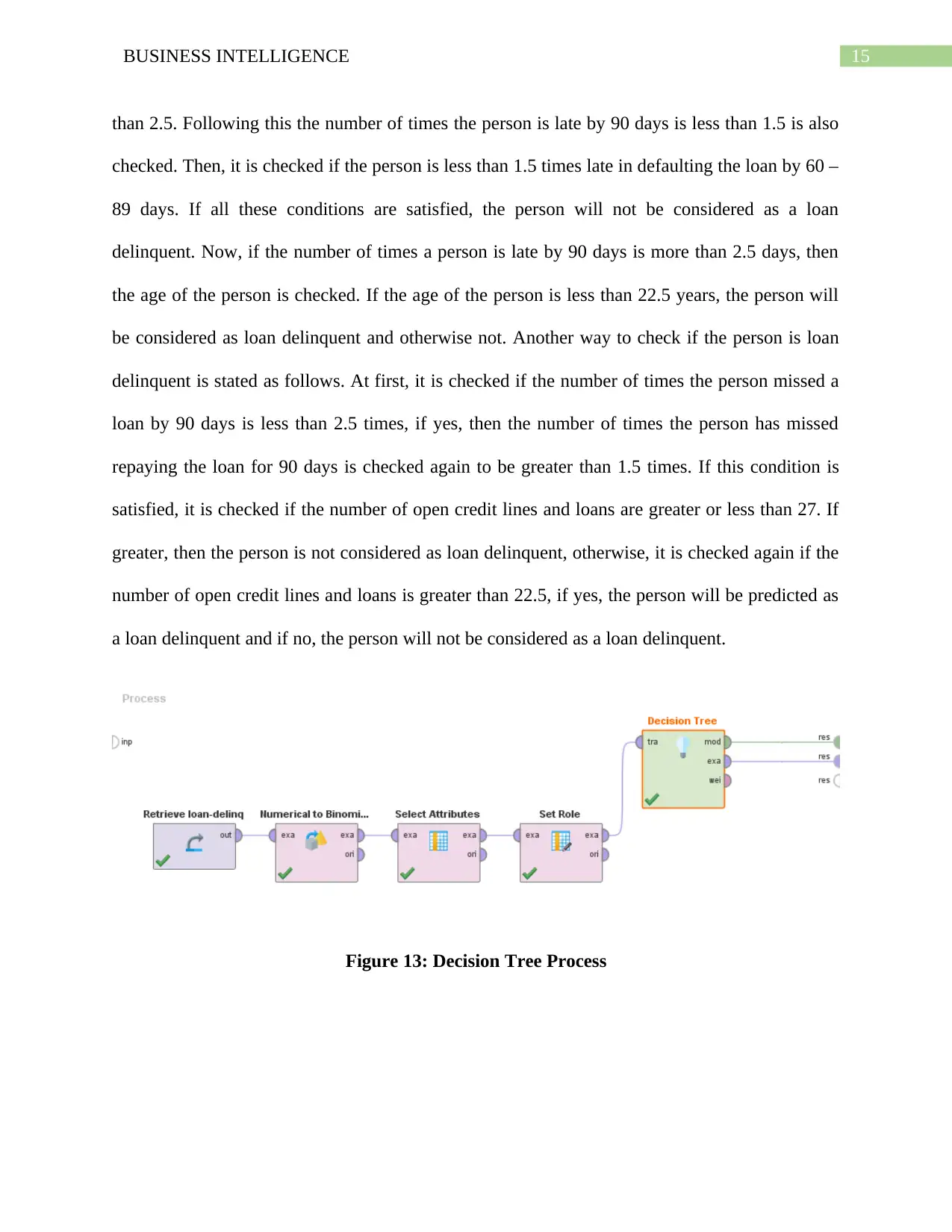
15BUSINESS INTELLIGENCE
than 2.5. Following this the number of times the person is late by 90 days is less than 1.5 is also
checked. Then, it is checked if the person is less than 1.5 times late in defaulting the loan by 60 –
89 days. If all these conditions are satisfied, the person will not be considered as a loan
delinquent. Now, if the number of times a person is late by 90 days is more than 2.5 days, then
the age of the person is checked. If the age of the person is less than 22.5 years, the person will
be considered as loan delinquent and otherwise not. Another way to check if the person is loan
delinquent is stated as follows. At first, it is checked if the number of times the person missed a
loan by 90 days is less than 2.5 times, if yes, then the number of times the person has missed
repaying the loan for 90 days is checked again to be greater than 1.5 times. If this condition is
satisfied, it is checked if the number of open credit lines and loans are greater or less than 27. If
greater, then the person is not considered as loan delinquent, otherwise, it is checked again if the
number of open credit lines and loans is greater than 22.5, if yes, the person will be predicted as
a loan delinquent and if no, the person will not be considered as a loan delinquent.
Figure 13: Decision Tree Process
than 2.5. Following this the number of times the person is late by 90 days is less than 1.5 is also
checked. Then, it is checked if the person is less than 1.5 times late in defaulting the loan by 60 –
89 days. If all these conditions are satisfied, the person will not be considered as a loan
delinquent. Now, if the number of times a person is late by 90 days is more than 2.5 days, then
the age of the person is checked. If the age of the person is less than 22.5 years, the person will
be considered as loan delinquent and otherwise not. Another way to check if the person is loan
delinquent is stated as follows. At first, it is checked if the number of times the person missed a
loan by 90 days is less than 2.5 times, if yes, then the number of times the person has missed
repaying the loan for 90 days is checked again to be greater than 1.5 times. If this condition is
satisfied, it is checked if the number of open credit lines and loans are greater or less than 27. If
greater, then the person is not considered as loan delinquent, otherwise, it is checked again if the
number of open credit lines and loans is greater than 22.5, if yes, the person will be predicted as
a loan delinquent and if no, the person will not be considered as a loan delinquent.
Figure 13: Decision Tree Process
Secure Best Marks with AI Grader
Need help grading? Try our AI Grader for instant feedback on your assignments.
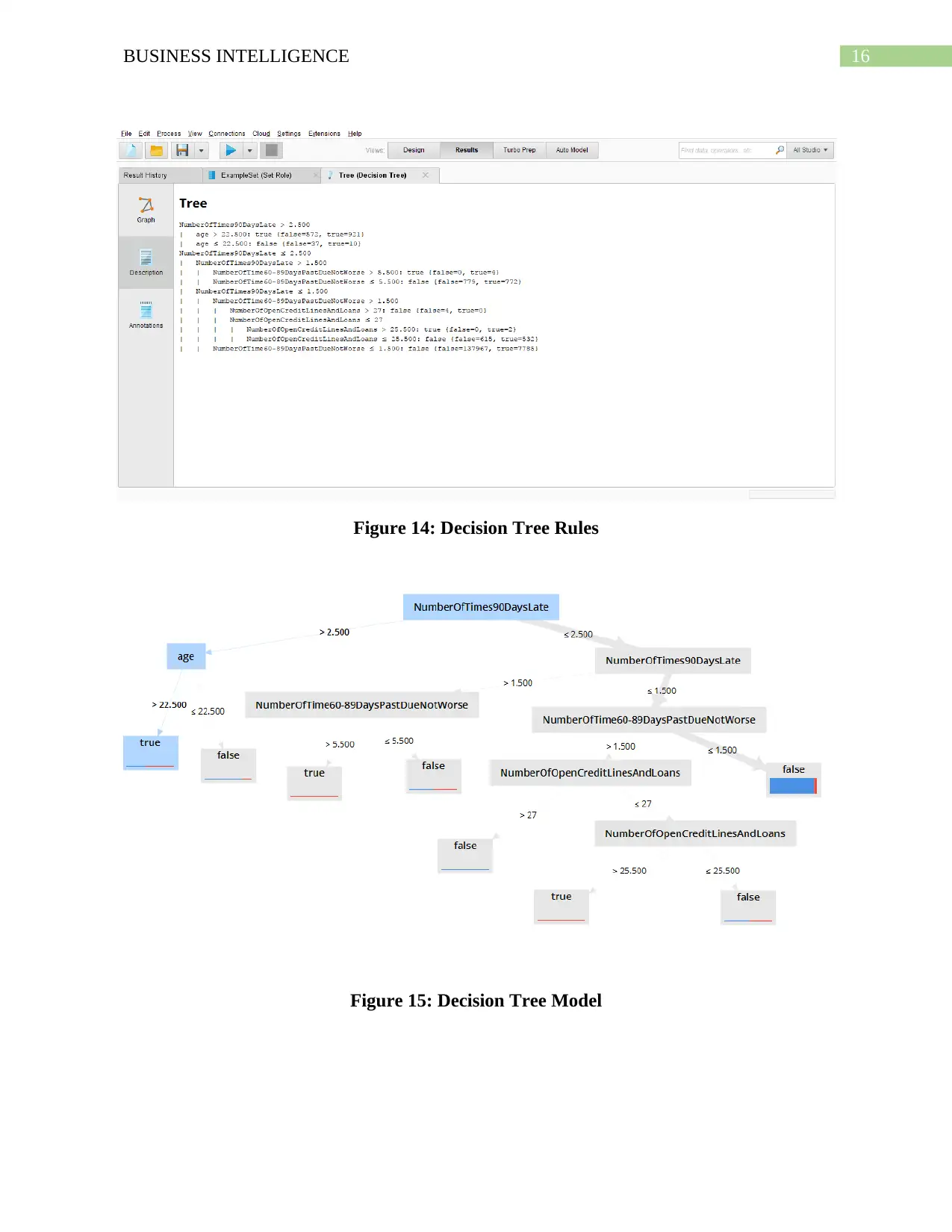
16BUSINESS INTELLIGENCE
Figure 14: Decision Tree Rules
Figure 15: Decision Tree Model
Figure 14: Decision Tree Rules
Figure 15: Decision Tree Model
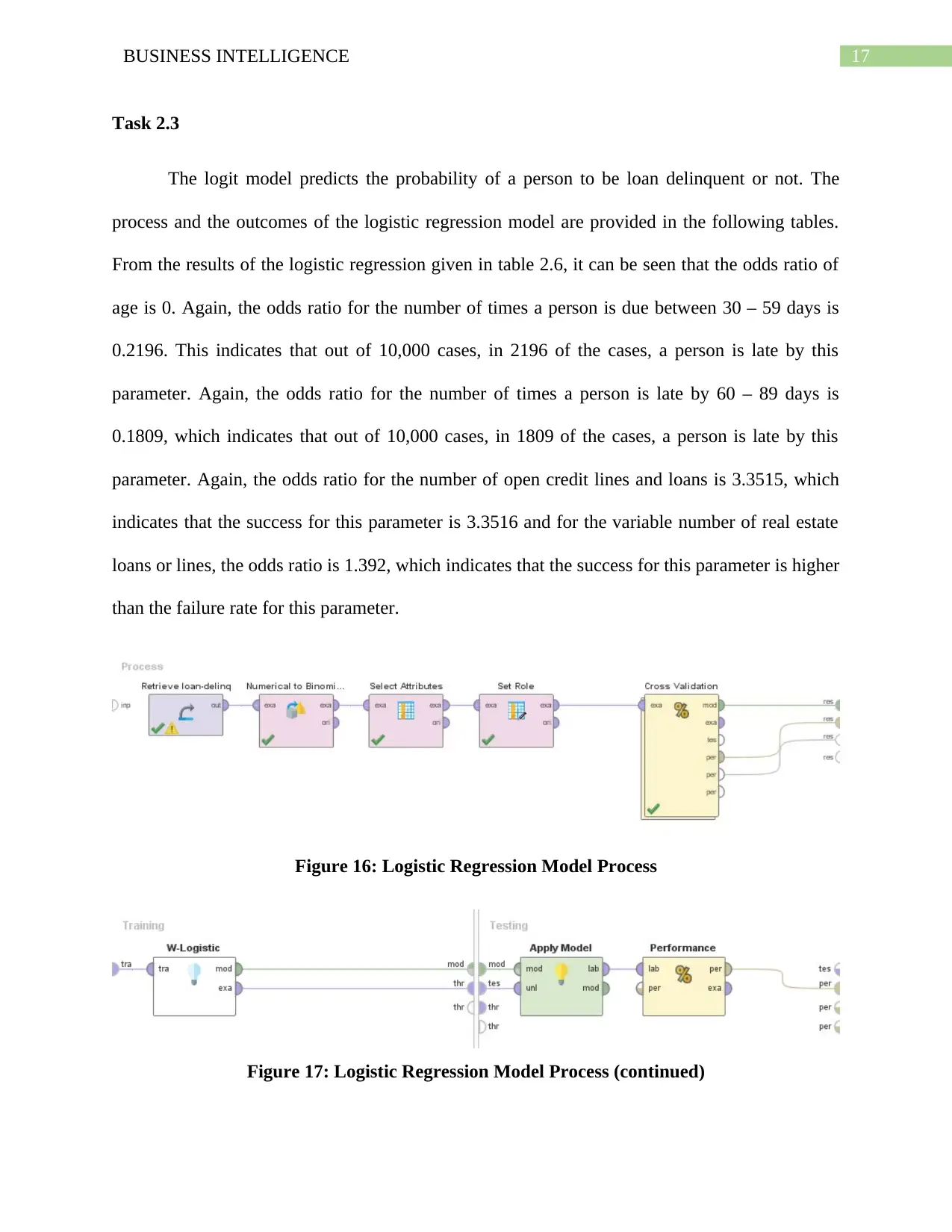
17BUSINESS INTELLIGENCE
Task 2.3
The logit model predicts the probability of a person to be loan delinquent or not. The
process and the outcomes of the logistic regression model are provided in the following tables.
From the results of the logistic regression given in table 2.6, it can be seen that the odds ratio of
age is 0. Again, the odds ratio for the number of times a person is due between 30 – 59 days is
0.2196. This indicates that out of 10,000 cases, in 2196 of the cases, a person is late by this
parameter. Again, the odds ratio for the number of times a person is late by 60 – 89 days is
0.1809, which indicates that out of 10,000 cases, in 1809 of the cases, a person is late by this
parameter. Again, the odds ratio for the number of open credit lines and loans is 3.3515, which
indicates that the success for this parameter is 3.3516 and for the variable number of real estate
loans or lines, the odds ratio is 1.392, which indicates that the success for this parameter is higher
than the failure rate for this parameter.
Figure 16: Logistic Regression Model Process
Figure 17: Logistic Regression Model Process (continued)
Task 2.3
The logit model predicts the probability of a person to be loan delinquent or not. The
process and the outcomes of the logistic regression model are provided in the following tables.
From the results of the logistic regression given in table 2.6, it can be seen that the odds ratio of
age is 0. Again, the odds ratio for the number of times a person is due between 30 – 59 days is
0.2196. This indicates that out of 10,000 cases, in 2196 of the cases, a person is late by this
parameter. Again, the odds ratio for the number of times a person is late by 60 – 89 days is
0.1809, which indicates that out of 10,000 cases, in 1809 of the cases, a person is late by this
parameter. Again, the odds ratio for the number of open credit lines and loans is 3.3515, which
indicates that the success for this parameter is 3.3516 and for the variable number of real estate
loans or lines, the odds ratio is 1.392, which indicates that the success for this parameter is higher
than the failure rate for this parameter.
Figure 16: Logistic Regression Model Process
Figure 17: Logistic Regression Model Process (continued)
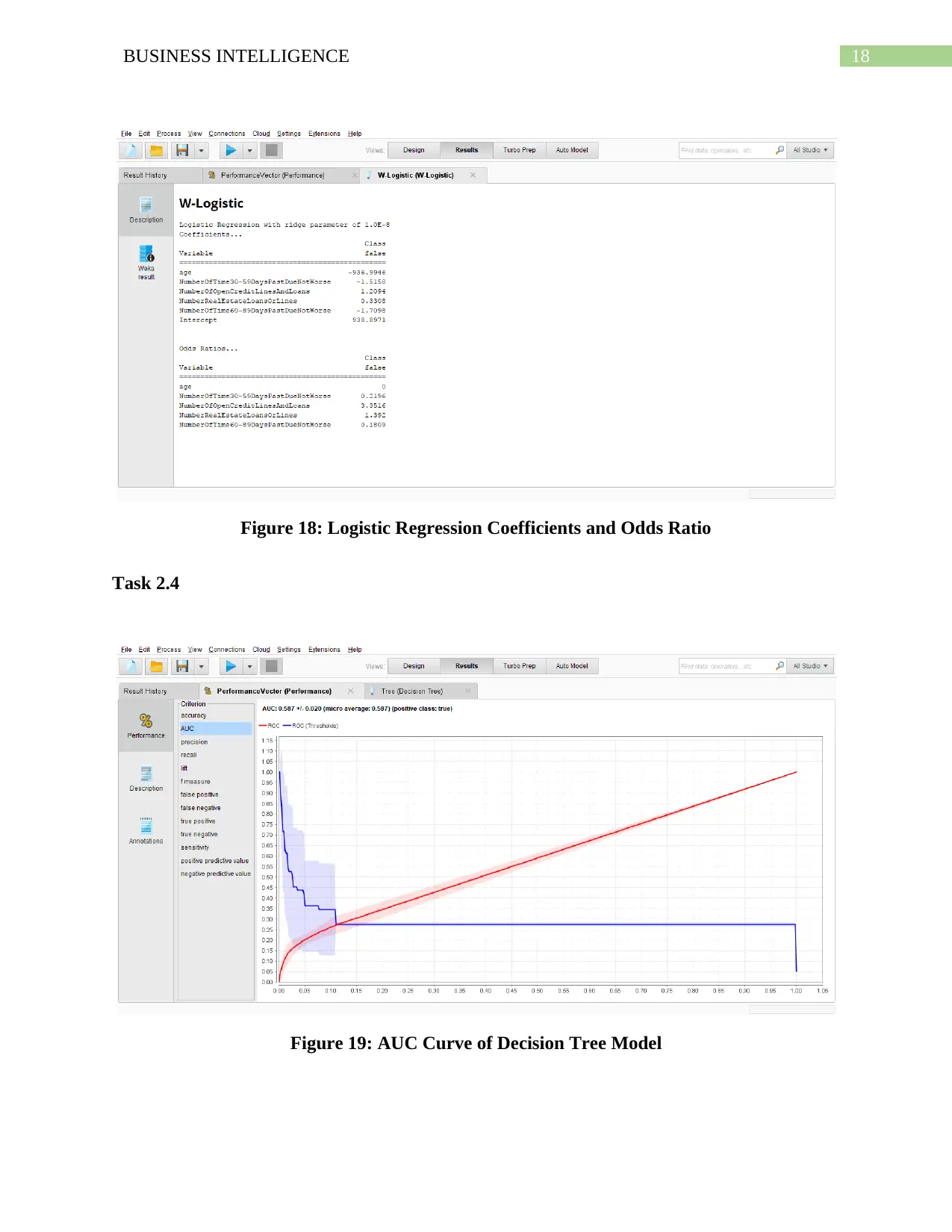
18BUSINESS INTELLIGENCE
Figure 18: Logistic Regression Coefficients and Odds Ratio
Task 2.4
Figure 19: AUC Curve of Decision Tree Model
Figure 18: Logistic Regression Coefficients and Odds Ratio
Task 2.4
Figure 19: AUC Curve of Decision Tree Model
Paraphrase This Document
Need a fresh take? Get an instant paraphrase of this document with our AI Paraphraser
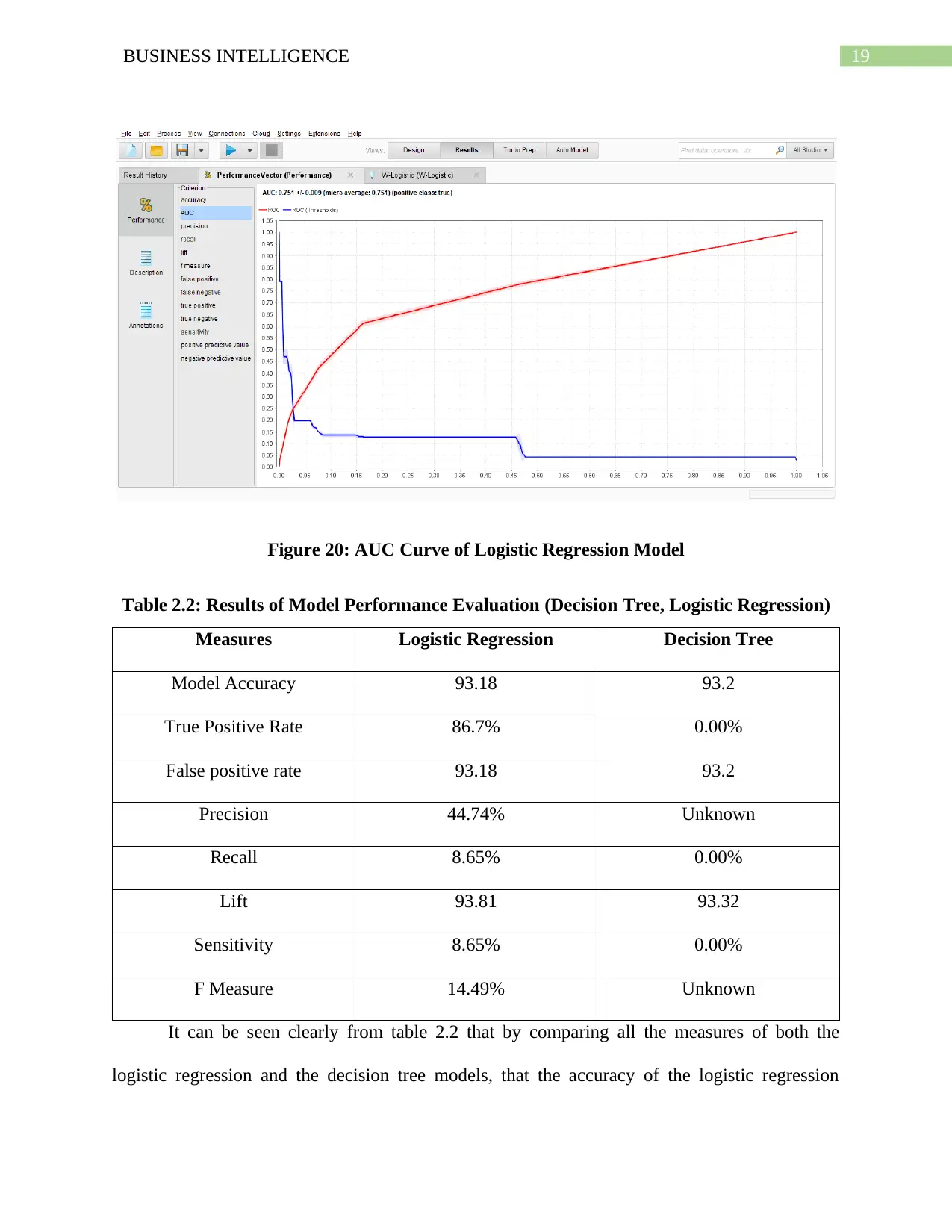
19BUSINESS INTELLIGENCE
Figure 20: AUC Curve of Logistic Regression Model
Table 2.2: Results of Model Performance Evaluation (Decision Tree, Logistic Regression)
Measures Logistic Regression Decision Tree
Model Accuracy 93.18 93.2
True Positive Rate 86.7% 0.00%
False positive rate 93.18 93.2
Precision 44.74% Unknown
Recall 8.65% 0.00%
Lift 93.81 93.32
Sensitivity 8.65% 0.00%
F Measure 14.49% Unknown
It can be seen clearly from table 2.2 that by comparing all the measures of both the
logistic regression and the decision tree models, that the accuracy of the logistic regression
Figure 20: AUC Curve of Logistic Regression Model
Table 2.2: Results of Model Performance Evaluation (Decision Tree, Logistic Regression)
Measures Logistic Regression Decision Tree
Model Accuracy 93.18 93.2
True Positive Rate 86.7% 0.00%
False positive rate 93.18 93.2
Precision 44.74% Unknown
Recall 8.65% 0.00%
Lift 93.81 93.32
Sensitivity 8.65% 0.00%
F Measure 14.49% Unknown
It can be seen clearly from table 2.2 that by comparing all the measures of both the
logistic regression and the decision tree models, that the accuracy of the logistic regression
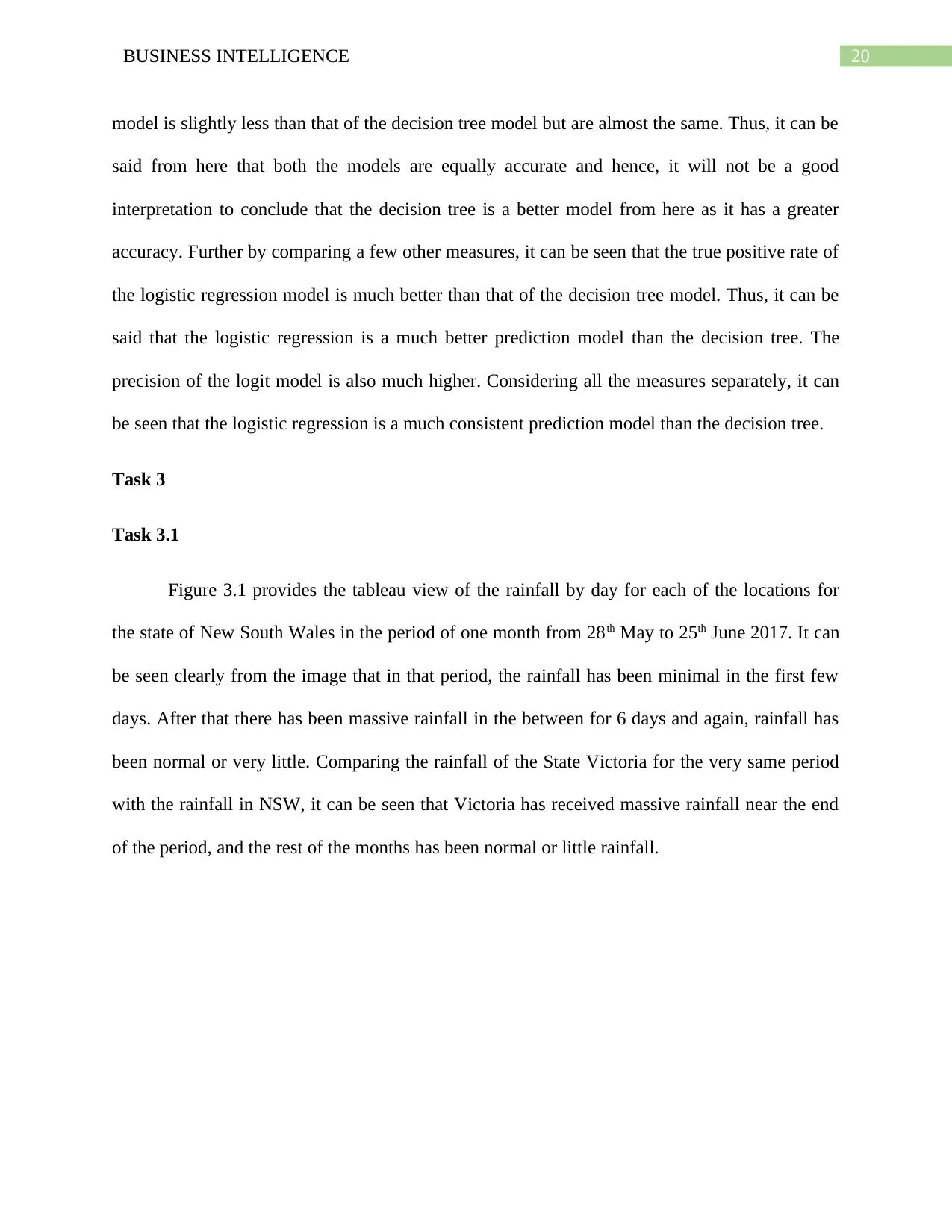
20BUSINESS INTELLIGENCE
model is slightly less than that of the decision tree model but are almost the same. Thus, it can be
said from here that both the models are equally accurate and hence, it will not be a good
interpretation to conclude that the decision tree is a better model from here as it has a greater
accuracy. Further by comparing a few other measures, it can be seen that the true positive rate of
the logistic regression model is much better than that of the decision tree model. Thus, it can be
said that the logistic regression is a much better prediction model than the decision tree. The
precision of the logit model is also much higher. Considering all the measures separately, it can
be seen that the logistic regression is a much consistent prediction model than the decision tree.
Task 3
Task 3.1
Figure 3.1 provides the tableau view of the rainfall by day for each of the locations for
the state of New South Wales in the period of one month from 28th May to 25th June 2017. It can
be seen clearly from the image that in that period, the rainfall has been minimal in the first few
days. After that there has been massive rainfall in the between for 6 days and again, rainfall has
been normal or very little. Comparing the rainfall of the State Victoria for the very same period
with the rainfall in NSW, it can be seen that Victoria has received massive rainfall near the end
of the period, and the rest of the months has been normal or little rainfall.
model is slightly less than that of the decision tree model but are almost the same. Thus, it can be
said from here that both the models are equally accurate and hence, it will not be a good
interpretation to conclude that the decision tree is a better model from here as it has a greater
accuracy. Further by comparing a few other measures, it can be seen that the true positive rate of
the logistic regression model is much better than that of the decision tree model. Thus, it can be
said that the logistic regression is a much better prediction model than the decision tree. The
precision of the logit model is also much higher. Considering all the measures separately, it can
be seen that the logistic regression is a much consistent prediction model than the decision tree.
Task 3
Task 3.1
Figure 3.1 provides the tableau view of the rainfall by day for each of the locations for
the state of New South Wales in the period of one month from 28th May to 25th June 2017. It can
be seen clearly from the image that in that period, the rainfall has been minimal in the first few
days. After that there has been massive rainfall in the between for 6 days and again, rainfall has
been normal or very little. Comparing the rainfall of the State Victoria for the very same period
with the rainfall in NSW, it can be seen that Victoria has received massive rainfall near the end
of the period, and the rest of the months has been normal or little rainfall.
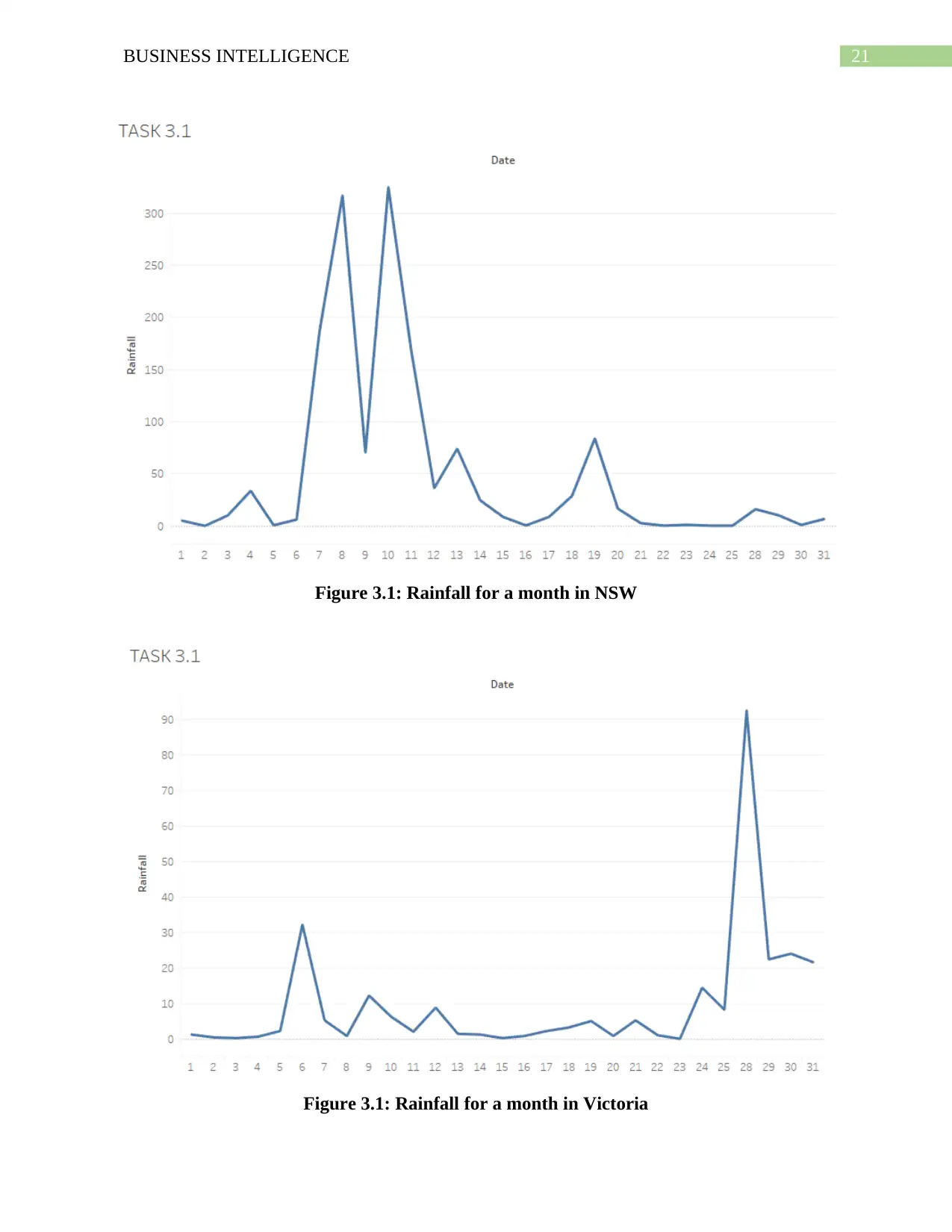
21BUSINESS INTELLIGENCE
Figure 3.1: Rainfall for a month in NSW
Figure 3.1: Rainfall for a month in Victoria
Figure 3.1: Rainfall for a month in NSW
Figure 3.1: Rainfall for a month in Victoria
Secure Best Marks with AI Grader
Need help grading? Try our AI Grader for instant feedback on your assignments.
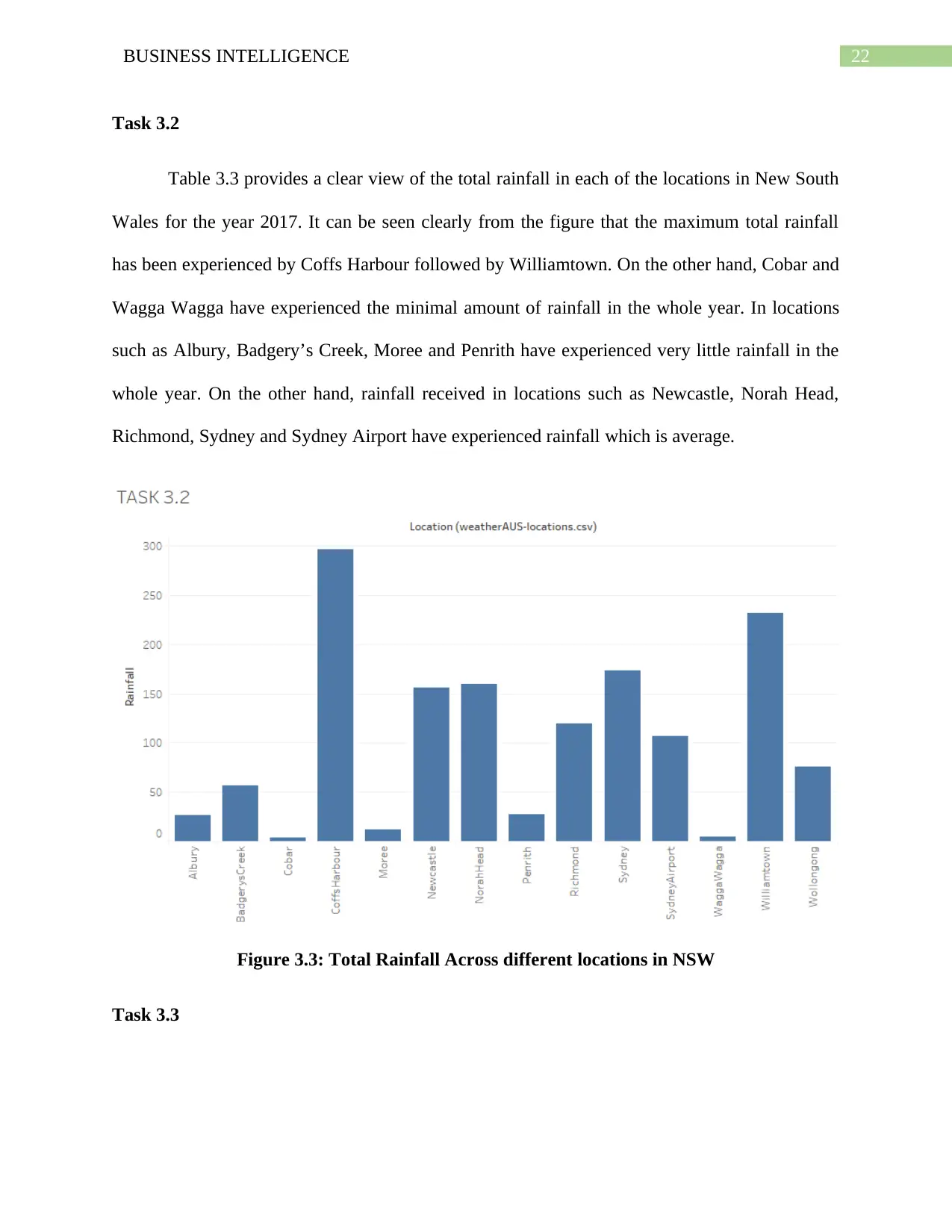
22BUSINESS INTELLIGENCE
Task 3.2
Table 3.3 provides a clear view of the total rainfall in each of the locations in New South
Wales for the year 2017. It can be seen clearly from the figure that the maximum total rainfall
has been experienced by Coffs Harbour followed by Williamtown. On the other hand, Cobar and
Wagga Wagga have experienced the minimal amount of rainfall in the whole year. In locations
such as Albury, Badgery’s Creek, Moree and Penrith have experienced very little rainfall in the
whole year. On the other hand, rainfall received in locations such as Newcastle, Norah Head,
Richmond, Sydney and Sydney Airport have experienced rainfall which is average.
Figure 3.3: Total Rainfall Across different locations in NSW
Task 3.3
Task 3.2
Table 3.3 provides a clear view of the total rainfall in each of the locations in New South
Wales for the year 2017. It can be seen clearly from the figure that the maximum total rainfall
has been experienced by Coffs Harbour followed by Williamtown. On the other hand, Cobar and
Wagga Wagga have experienced the minimal amount of rainfall in the whole year. In locations
such as Albury, Badgery’s Creek, Moree and Penrith have experienced very little rainfall in the
whole year. On the other hand, rainfall received in locations such as Newcastle, Norah Head,
Richmond, Sydney and Sydney Airport have experienced rainfall which is average.
Figure 3.3: Total Rainfall Across different locations in NSW
Task 3.3
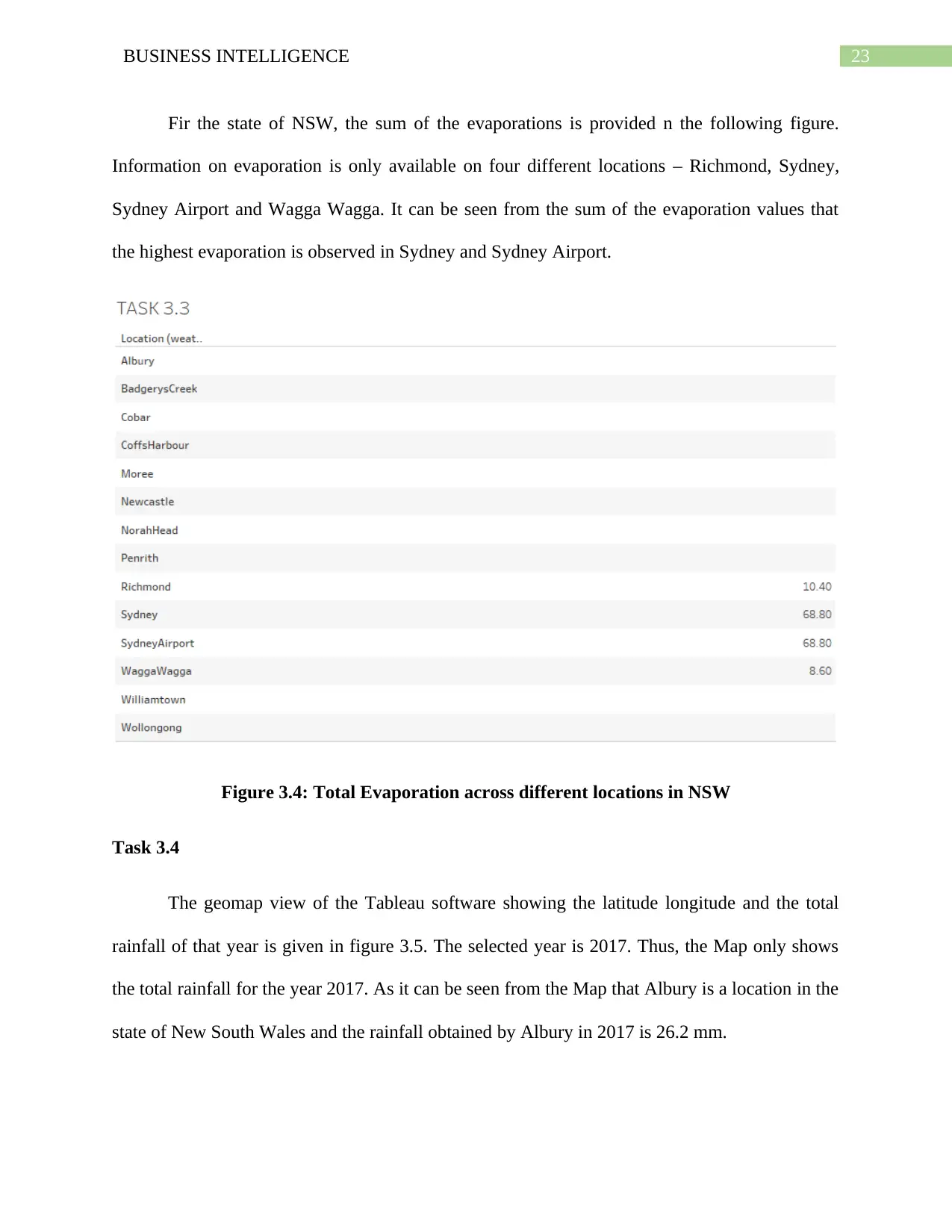
23BUSINESS INTELLIGENCE
Fir the state of NSW, the sum of the evaporations is provided n the following figure.
Information on evaporation is only available on four different locations – Richmond, Sydney,
Sydney Airport and Wagga Wagga. It can be seen from the sum of the evaporation values that
the highest evaporation is observed in Sydney and Sydney Airport.
Figure 3.4: Total Evaporation across different locations in NSW
Task 3.4
The geomap view of the Tableau software showing the latitude longitude and the total
rainfall of that year is given in figure 3.5. The selected year is 2017. Thus, the Map only shows
the total rainfall for the year 2017. As it can be seen from the Map that Albury is a location in the
state of New South Wales and the rainfall obtained by Albury in 2017 is 26.2 mm.
Fir the state of NSW, the sum of the evaporations is provided n the following figure.
Information on evaporation is only available on four different locations – Richmond, Sydney,
Sydney Airport and Wagga Wagga. It can be seen from the sum of the evaporation values that
the highest evaporation is observed in Sydney and Sydney Airport.
Figure 3.4: Total Evaporation across different locations in NSW
Task 3.4
The geomap view of the Tableau software showing the latitude longitude and the total
rainfall of that year is given in figure 3.5. The selected year is 2017. Thus, the Map only shows
the total rainfall for the year 2017. As it can be seen from the Map that Albury is a location in the
state of New South Wales and the rainfall obtained by Albury in 2017 is 26.2 mm.
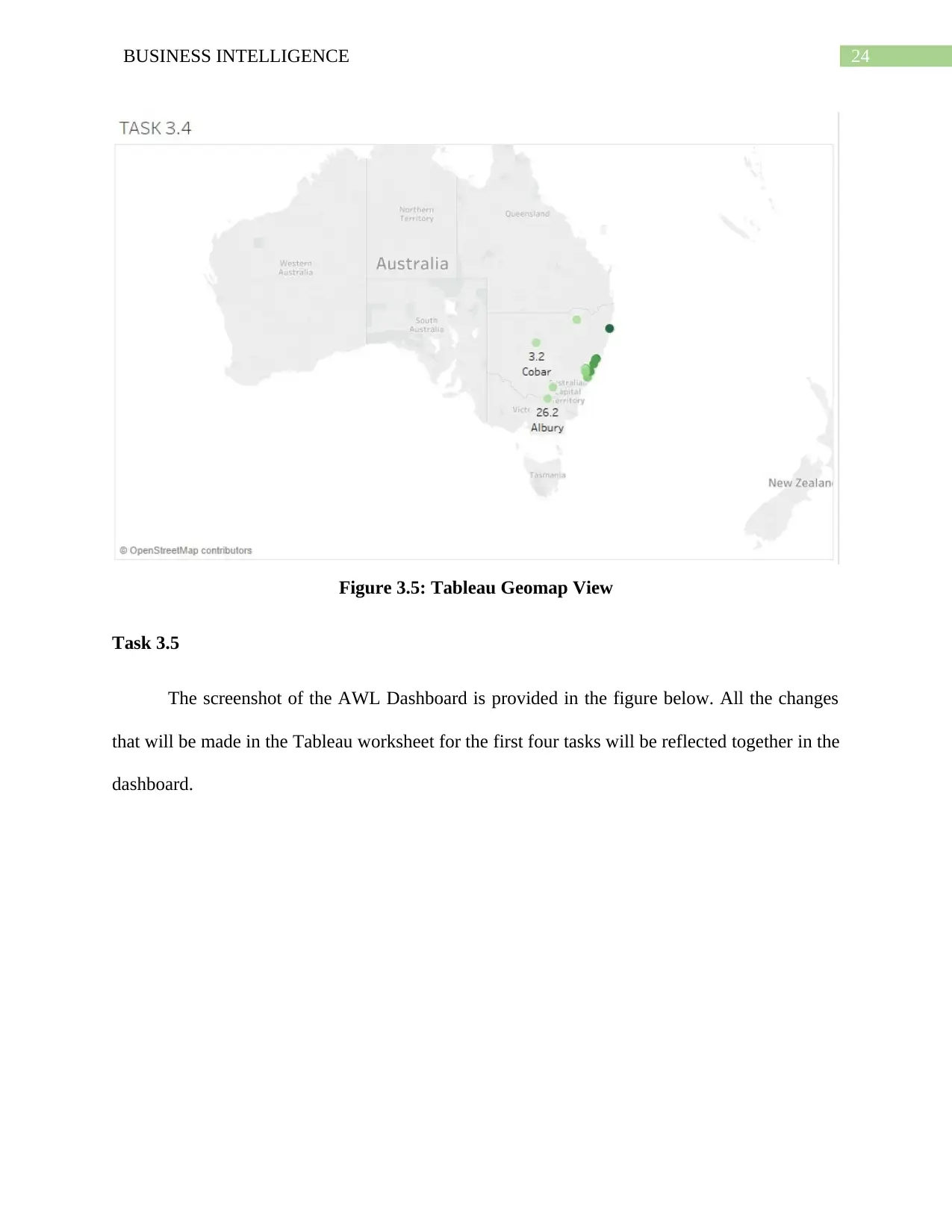
24BUSINESS INTELLIGENCE
Figure 3.5: Tableau Geomap View
Task 3.5
The screenshot of the AWL Dashboard is provided in the figure below. All the changes
that will be made in the Tableau worksheet for the first four tasks will be reflected together in the
dashboard.
Figure 3.5: Tableau Geomap View
Task 3.5
The screenshot of the AWL Dashboard is provided in the figure below. All the changes
that will be made in the Tableau worksheet for the first four tasks will be reflected together in the
dashboard.
Paraphrase This Document
Need a fresh take? Get an instant paraphrase of this document with our AI Paraphraser
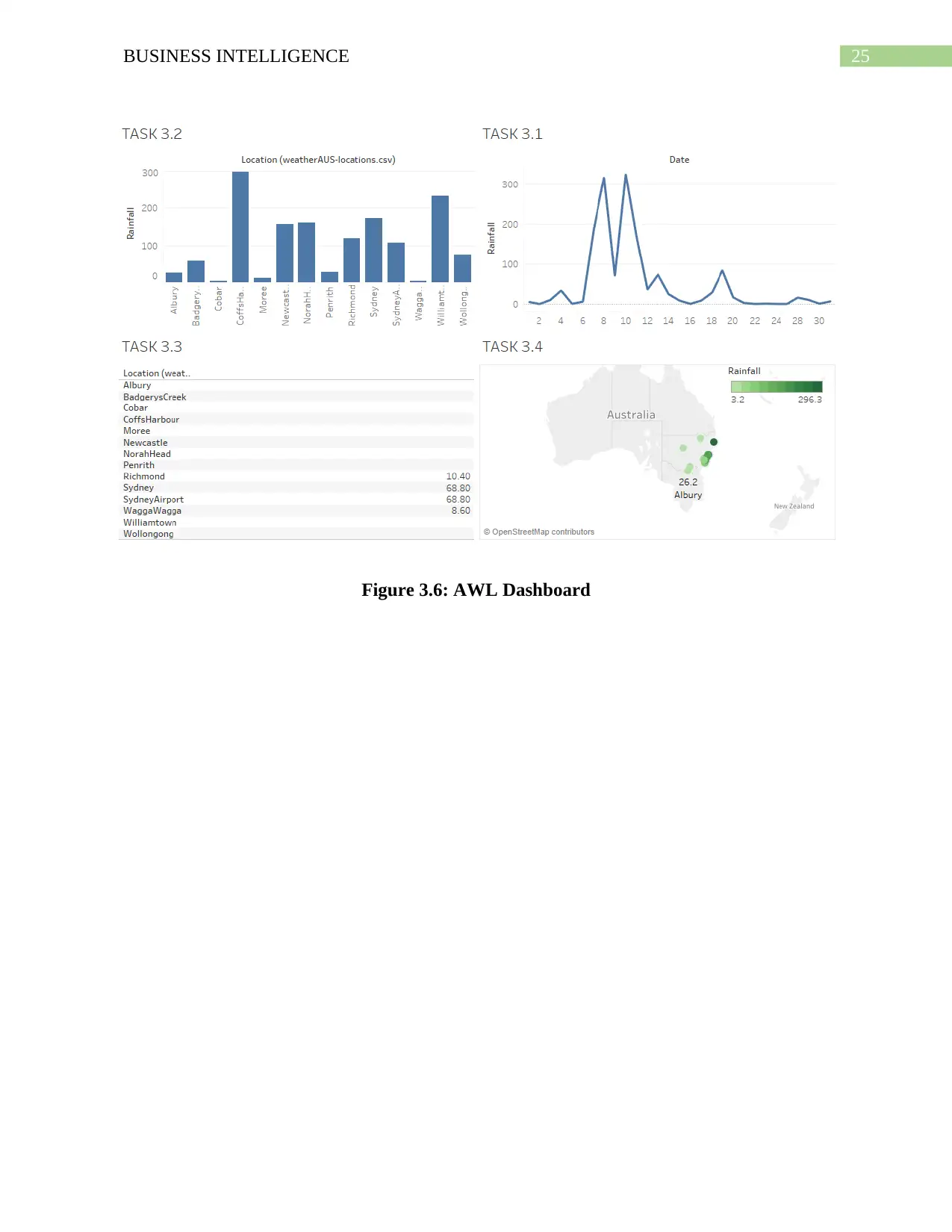
25BUSINESS INTELLIGENCE
Figure 3.6: AWL Dashboard
Figure 3.6: AWL Dashboard
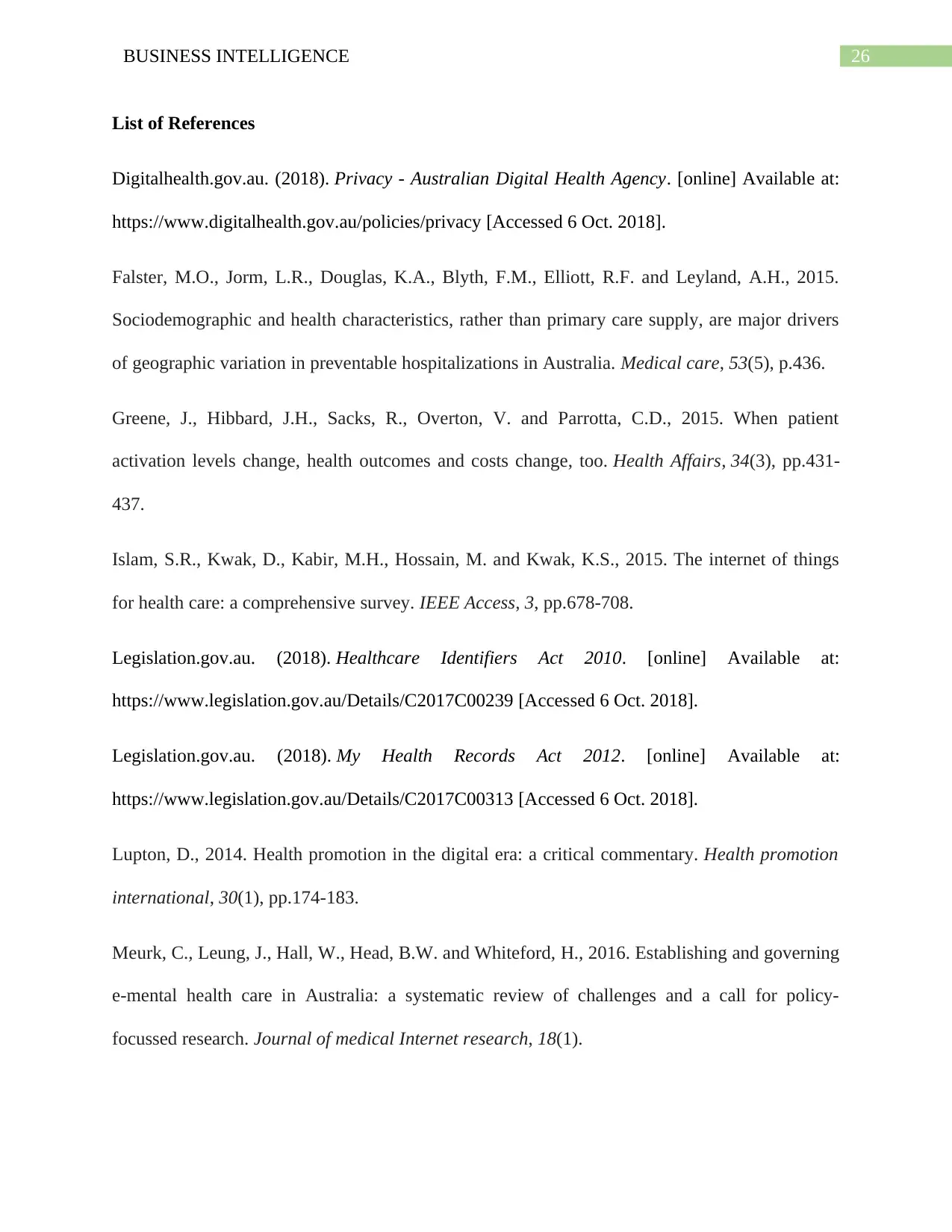
26BUSINESS INTELLIGENCE
List of References
Digitalhealth.gov.au. (2018). Privacy - Australian Digital Health Agency. [online] Available at:
https://www.digitalhealth.gov.au/policies/privacy [Accessed 6 Oct. 2018].
Falster, M.O., Jorm, L.R., Douglas, K.A., Blyth, F.M., Elliott, R.F. and Leyland, A.H., 2015.
Sociodemographic and health characteristics, rather than primary care supply, are major drivers
of geographic variation in preventable hospitalizations in Australia. Medical care, 53(5), p.436.
Greene, J., Hibbard, J.H., Sacks, R., Overton, V. and Parrotta, C.D., 2015. When patient
activation levels change, health outcomes and costs change, too. Health Affairs, 34(3), pp.431-
437.
Islam, S.R., Kwak, D., Kabir, M.H., Hossain, M. and Kwak, K.S., 2015. The internet of things
for health care: a comprehensive survey. IEEE Access, 3, pp.678-708.
Legislation.gov.au. (2018). Healthcare Identifiers Act 2010. [online] Available at:
https://www.legislation.gov.au/Details/C2017C00239 [Accessed 6 Oct. 2018].
Legislation.gov.au. (2018). My Health Records Act 2012. [online] Available at:
https://www.legislation.gov.au/Details/C2017C00313 [Accessed 6 Oct. 2018].
Lupton, D., 2014. Health promotion in the digital era: a critical commentary. Health promotion
international, 30(1), pp.174-183.
Meurk, C., Leung, J., Hall, W., Head, B.W. and Whiteford, H., 2016. Establishing and governing
e-mental health care in Australia: a systematic review of challenges and a call for policy-
focussed research. Journal of medical Internet research, 18(1).
List of References
Digitalhealth.gov.au. (2018). Privacy - Australian Digital Health Agency. [online] Available at:
https://www.digitalhealth.gov.au/policies/privacy [Accessed 6 Oct. 2018].
Falster, M.O., Jorm, L.R., Douglas, K.A., Blyth, F.M., Elliott, R.F. and Leyland, A.H., 2015.
Sociodemographic and health characteristics, rather than primary care supply, are major drivers
of geographic variation in preventable hospitalizations in Australia. Medical care, 53(5), p.436.
Greene, J., Hibbard, J.H., Sacks, R., Overton, V. and Parrotta, C.D., 2015. When patient
activation levels change, health outcomes and costs change, too. Health Affairs, 34(3), pp.431-
437.
Islam, S.R., Kwak, D., Kabir, M.H., Hossain, M. and Kwak, K.S., 2015. The internet of things
for health care: a comprehensive survey. IEEE Access, 3, pp.678-708.
Legislation.gov.au. (2018). Healthcare Identifiers Act 2010. [online] Available at:
https://www.legislation.gov.au/Details/C2017C00239 [Accessed 6 Oct. 2018].
Legislation.gov.au. (2018). My Health Records Act 2012. [online] Available at:
https://www.legislation.gov.au/Details/C2017C00313 [Accessed 6 Oct. 2018].
Lupton, D., 2014. Health promotion in the digital era: a critical commentary. Health promotion
international, 30(1), pp.174-183.
Meurk, C., Leung, J., Hall, W., Head, B.W. and Whiteford, H., 2016. Establishing and governing
e-mental health care in Australia: a systematic review of challenges and a call for policy-
focussed research. Journal of medical Internet research, 18(1).
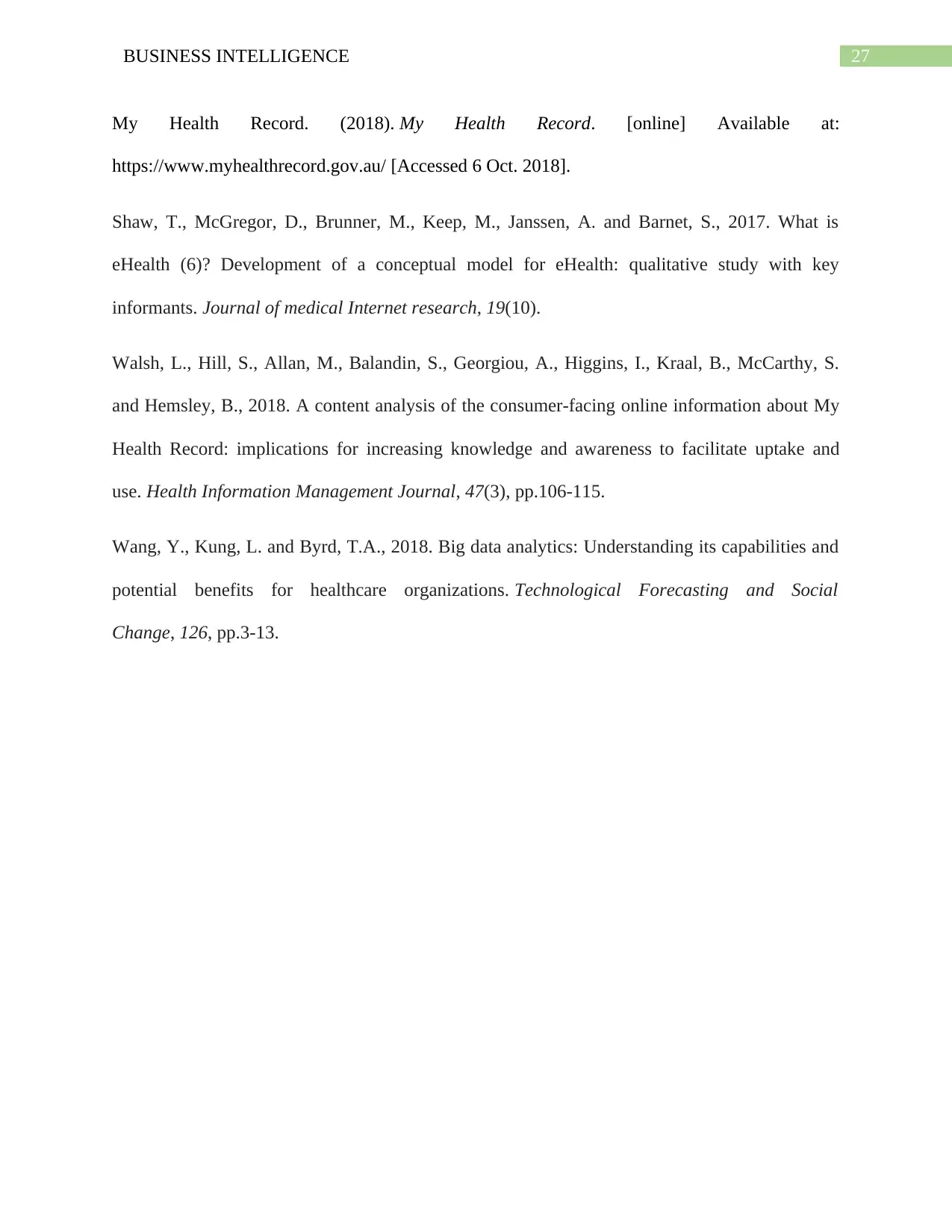
27BUSINESS INTELLIGENCE
My Health Record. (2018). My Health Record. [online] Available at:
https://www.myhealthrecord.gov.au/ [Accessed 6 Oct. 2018].
Shaw, T., McGregor, D., Brunner, M., Keep, M., Janssen, A. and Barnet, S., 2017. What is
eHealth (6)? Development of a conceptual model for eHealth: qualitative study with key
informants. Journal of medical Internet research, 19(10).
Walsh, L., Hill, S., Allan, M., Balandin, S., Georgiou, A., Higgins, I., Kraal, B., McCarthy, S.
and Hemsley, B., 2018. A content analysis of the consumer-facing online information about My
Health Record: implications for increasing knowledge and awareness to facilitate uptake and
use. Health Information Management Journal, 47(3), pp.106-115.
Wang, Y., Kung, L. and Byrd, T.A., 2018. Big data analytics: Understanding its capabilities and
potential benefits for healthcare organizations. Technological Forecasting and Social
Change, 126, pp.3-13.
My Health Record. (2018). My Health Record. [online] Available at:
https://www.myhealthrecord.gov.au/ [Accessed 6 Oct. 2018].
Shaw, T., McGregor, D., Brunner, M., Keep, M., Janssen, A. and Barnet, S., 2017. What is
eHealth (6)? Development of a conceptual model for eHealth: qualitative study with key
informants. Journal of medical Internet research, 19(10).
Walsh, L., Hill, S., Allan, M., Balandin, S., Georgiou, A., Higgins, I., Kraal, B., McCarthy, S.
and Hemsley, B., 2018. A content analysis of the consumer-facing online information about My
Health Record: implications for increasing knowledge and awareness to facilitate uptake and
use. Health Information Management Journal, 47(3), pp.106-115.
Wang, Y., Kung, L. and Byrd, T.A., 2018. Big data analytics: Understanding its capabilities and
potential benefits for healthcare organizations. Technological Forecasting and Social
Change, 126, pp.3-13.
1 out of 28
Related Documents
![[object Object]](/_next/image/?url=%2F_next%2Fstatic%2Fmedia%2Flogo.6d15ce61.png&w=640&q=75)
Your All-in-One AI-Powered Toolkit for Academic Success.
+13062052269
info@desklib.com
Available 24*7 on WhatsApp / Email
Unlock your academic potential
© 2024 | Zucol Services PVT LTD | All rights reserved.