M350 Statistics II, Spring 2020: Regression Analysis
VerifiedAdded on 2022/09/23
|6
|1354
|25
Homework Assignment
AI Summary
This assignment solution addresses a statistics problem involving multiple linear regression analysis. The solution begins by analyzing the goodness of fit of a given model, evaluating the R-squared value and the statistical significance of individual slope coefficients. It then discusses the testing of assumptions, specifically addressing the normality and homoscedasticity assumptions. The solution proceeds to compare complete and reduced models using F-statistics, and determines the significance of an interaction model and its coefficients. Furthermore, the assignment explores the significance of a quadratic term and provides the least squares regression equation. Finally, the solution covers the utility of a regression model, multicollinearity, and provides prediction intervals. The assignment utilizes Minitab output to support its analysis and interpretations.
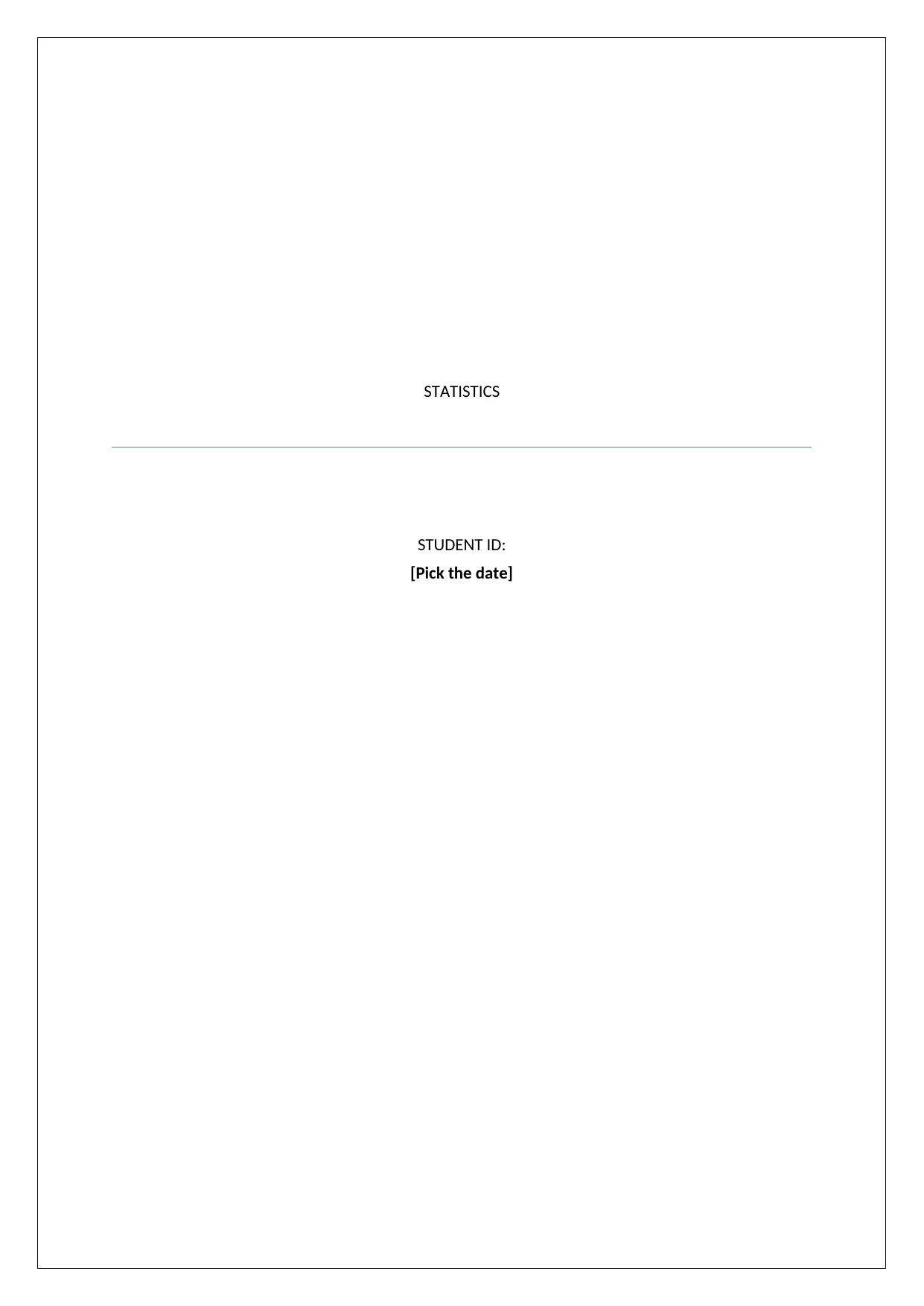
STATISTICS
STUDENT ID:
[Pick the date]
STUDENT ID:
[Pick the date]
Paraphrase This Document
Need a fresh take? Get an instant paraphrase of this document with our AI Paraphraser
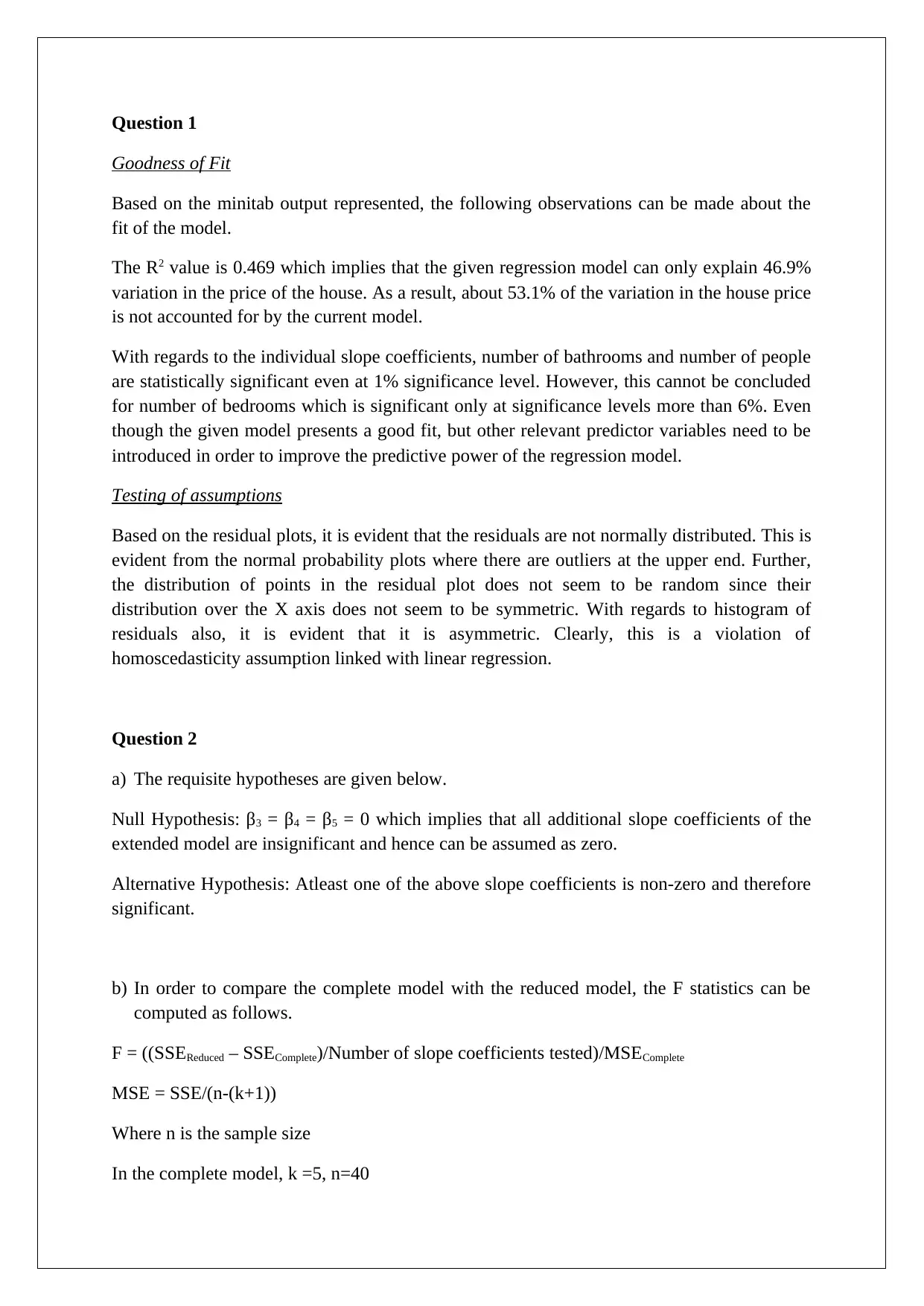
Question 1
Goodness of Fit
Based on the minitab output represented, the following observations can be made about the
fit of the model.
The R2 value is 0.469 which implies that the given regression model can only explain 46.9%
variation in the price of the house. As a result, about 53.1% of the variation in the house price
is not accounted for by the current model.
With regards to the individual slope coefficients, number of bathrooms and number of people
are statistically significant even at 1% significance level. However, this cannot be concluded
for number of bedrooms which is significant only at significance levels more than 6%. Even
though the given model presents a good fit, but other relevant predictor variables need to be
introduced in order to improve the predictive power of the regression model.
Testing of assumptions
Based on the residual plots, it is evident that the residuals are not normally distributed. This is
evident from the normal probability plots where there are outliers at the upper end. Further,
the distribution of points in the residual plot does not seem to be random since their
distribution over the X axis does not seem to be symmetric. With regards to histogram of
residuals also, it is evident that it is asymmetric. Clearly, this is a violation of
homoscedasticity assumption linked with linear regression.
Question 2
a) The requisite hypotheses are given below.
Null Hypothesis: β3 = β4 = β5 = 0 which implies that all additional slope coefficients of the
extended model are insignificant and hence can be assumed as zero.
Alternative Hypothesis: Atleast one of the above slope coefficients is non-zero and therefore
significant.
b) In order to compare the complete model with the reduced model, the F statistics can be
computed as follows.
F = ((SSEReduced – SSEComplete)/Number of slope coefficients tested)/MSEComplete
MSE = SSE/(n-(k+1))
Where n is the sample size
In the complete model, k =5, n=40
Goodness of Fit
Based on the minitab output represented, the following observations can be made about the
fit of the model.
The R2 value is 0.469 which implies that the given regression model can only explain 46.9%
variation in the price of the house. As a result, about 53.1% of the variation in the house price
is not accounted for by the current model.
With regards to the individual slope coefficients, number of bathrooms and number of people
are statistically significant even at 1% significance level. However, this cannot be concluded
for number of bedrooms which is significant only at significance levels more than 6%. Even
though the given model presents a good fit, but other relevant predictor variables need to be
introduced in order to improve the predictive power of the regression model.
Testing of assumptions
Based on the residual plots, it is evident that the residuals are not normally distributed. This is
evident from the normal probability plots where there are outliers at the upper end. Further,
the distribution of points in the residual plot does not seem to be random since their
distribution over the X axis does not seem to be symmetric. With regards to histogram of
residuals also, it is evident that it is asymmetric. Clearly, this is a violation of
homoscedasticity assumption linked with linear regression.
Question 2
a) The requisite hypotheses are given below.
Null Hypothesis: β3 = β4 = β5 = 0 which implies that all additional slope coefficients of the
extended model are insignificant and hence can be assumed as zero.
Alternative Hypothesis: Atleast one of the above slope coefficients is non-zero and therefore
significant.
b) In order to compare the complete model with the reduced model, the F statistics can be
computed as follows.
F = ((SSEReduced – SSEComplete)/Number of slope coefficients tested)/MSEComplete
MSE = SSE/(n-(k+1))
Where n is the sample size
In the complete model, k =5, n=40
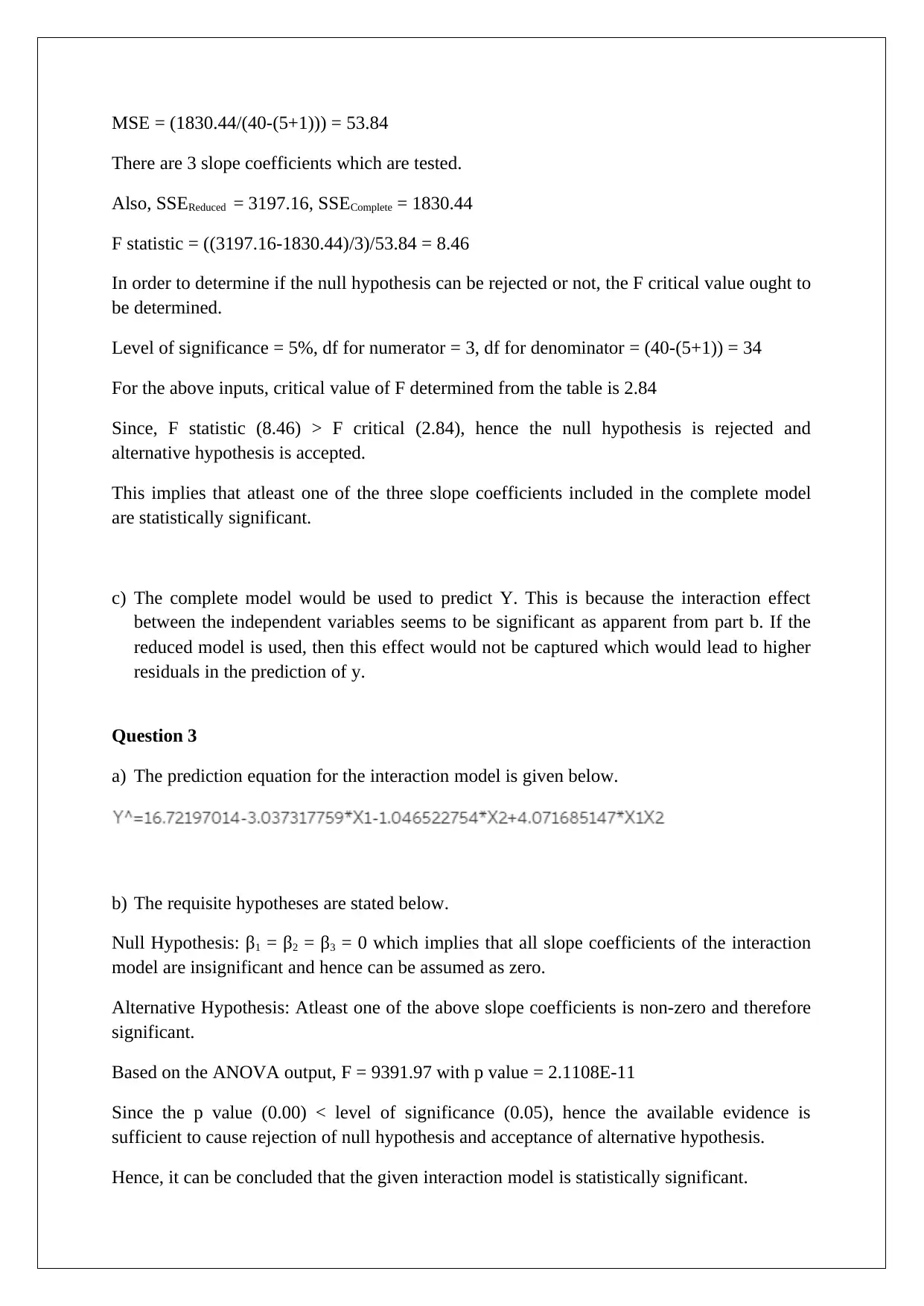
MSE = (1830.44/(40-(5+1))) = 53.84
There are 3 slope coefficients which are tested.
Also, SSEReduced = 3197.16, SSEComplete = 1830.44
F statistic = ((3197.16-1830.44)/3)/53.84 = 8.46
In order to determine if the null hypothesis can be rejected or not, the F critical value ought to
be determined.
Level of significance = 5%, df for numerator = 3, df for denominator = (40-(5+1)) = 34
For the above inputs, critical value of F determined from the table is 2.84
Since, F statistic (8.46) > F critical (2.84), hence the null hypothesis is rejected and
alternative hypothesis is accepted.
This implies that atleast one of the three slope coefficients included in the complete model
are statistically significant.
c) The complete model would be used to predict Y. This is because the interaction effect
between the independent variables seems to be significant as apparent from part b. If the
reduced model is used, then this effect would not be captured which would lead to higher
residuals in the prediction of y.
Question 3
a) The prediction equation for the interaction model is given below.
b) The requisite hypotheses are stated below.
Null Hypothesis: β1 = β2 = β3 = 0 which implies that all slope coefficients of the interaction
model are insignificant and hence can be assumed as zero.
Alternative Hypothesis: Atleast one of the above slope coefficients is non-zero and therefore
significant.
Based on the ANOVA output, F = 9391.97 with p value = 2.1108E-11
Since the p value (0.00) < level of significance (0.05), hence the available evidence is
sufficient to cause rejection of null hypothesis and acceptance of alternative hypothesis.
Hence, it can be concluded that the given interaction model is statistically significant.
There are 3 slope coefficients which are tested.
Also, SSEReduced = 3197.16, SSEComplete = 1830.44
F statistic = ((3197.16-1830.44)/3)/53.84 = 8.46
In order to determine if the null hypothesis can be rejected or not, the F critical value ought to
be determined.
Level of significance = 5%, df for numerator = 3, df for denominator = (40-(5+1)) = 34
For the above inputs, critical value of F determined from the table is 2.84
Since, F statistic (8.46) > F critical (2.84), hence the null hypothesis is rejected and
alternative hypothesis is accepted.
This implies that atleast one of the three slope coefficients included in the complete model
are statistically significant.
c) The complete model would be used to predict Y. This is because the interaction effect
between the independent variables seems to be significant as apparent from part b. If the
reduced model is used, then this effect would not be captured which would lead to higher
residuals in the prediction of y.
Question 3
a) The prediction equation for the interaction model is given below.
b) The requisite hypotheses are stated below.
Null Hypothesis: β1 = β2 = β3 = 0 which implies that all slope coefficients of the interaction
model are insignificant and hence can be assumed as zero.
Alternative Hypothesis: Atleast one of the above slope coefficients is non-zero and therefore
significant.
Based on the ANOVA output, F = 9391.97 with p value = 2.1108E-11
Since the p value (0.00) < level of significance (0.05), hence the available evidence is
sufficient to cause rejection of null hypothesis and acceptance of alternative hypothesis.
Hence, it can be concluded that the given interaction model is statistically significant.
You're viewing a preview
Unlock full access by subscribing today!
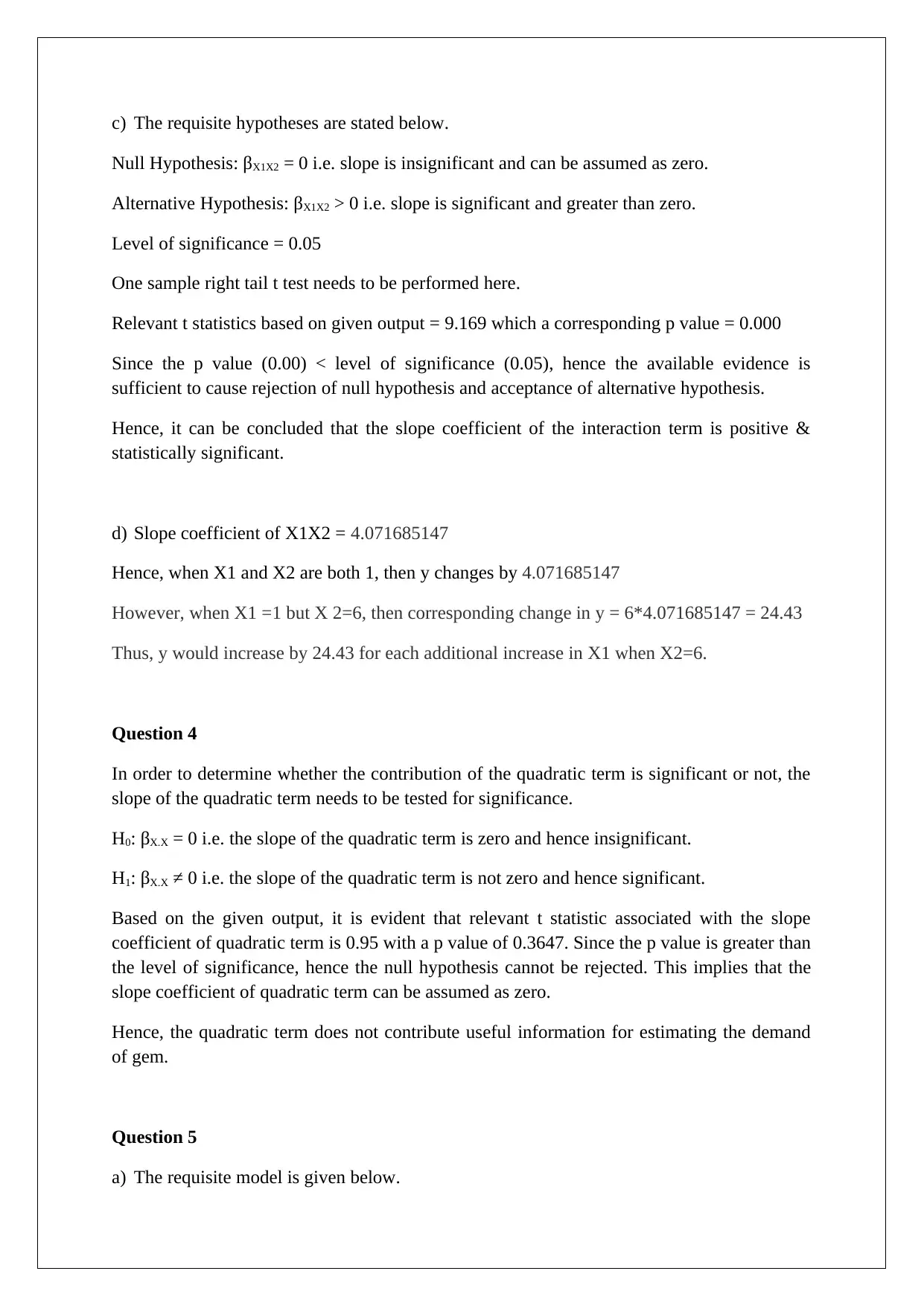
c) The requisite hypotheses are stated below.
Null Hypothesis: βX1X2 = 0 i.e. slope is insignificant and can be assumed as zero.
Alternative Hypothesis: βX1X2 > 0 i.e. slope is significant and greater than zero.
Level of significance = 0.05
One sample right tail t test needs to be performed here.
Relevant t statistics based on given output = 9.169 which a corresponding p value = 0.000
Since the p value (0.00) < level of significance (0.05), hence the available evidence is
sufficient to cause rejection of null hypothesis and acceptance of alternative hypothesis.
Hence, it can be concluded that the slope coefficient of the interaction term is positive &
statistically significant.
d) Slope coefficient of X1X2 = 4.071685147
Hence, when X1 and X2 are both 1, then y changes by 4.071685147
However, when X1 =1 but X 2=6, then corresponding change in y = 6*4.071685147 = 24.43
Thus, y would increase by 24.43 for each additional increase in X1 when X2=6.
Question 4
In order to determine whether the contribution of the quadratic term is significant or not, the
slope of the quadratic term needs to be tested for significance.
H0: βX.X = 0 i.e. the slope of the quadratic term is zero and hence insignificant.
H1: βX.X ≠ 0 i.e. the slope of the quadratic term is not zero and hence significant.
Based on the given output, it is evident that relevant t statistic associated with the slope
coefficient of quadratic term is 0.95 with a p value of 0.3647. Since the p value is greater than
the level of significance, hence the null hypothesis cannot be rejected. This implies that the
slope coefficient of quadratic term can be assumed as zero.
Hence, the quadratic term does not contribute useful information for estimating the demand
of gem.
Question 5
a) The requisite model is given below.
Null Hypothesis: βX1X2 = 0 i.e. slope is insignificant and can be assumed as zero.
Alternative Hypothesis: βX1X2 > 0 i.e. slope is significant and greater than zero.
Level of significance = 0.05
One sample right tail t test needs to be performed here.
Relevant t statistics based on given output = 9.169 which a corresponding p value = 0.000
Since the p value (0.00) < level of significance (0.05), hence the available evidence is
sufficient to cause rejection of null hypothesis and acceptance of alternative hypothesis.
Hence, it can be concluded that the slope coefficient of the interaction term is positive &
statistically significant.
d) Slope coefficient of X1X2 = 4.071685147
Hence, when X1 and X2 are both 1, then y changes by 4.071685147
However, when X1 =1 but X 2=6, then corresponding change in y = 6*4.071685147 = 24.43
Thus, y would increase by 24.43 for each additional increase in X1 when X2=6.
Question 4
In order to determine whether the contribution of the quadratic term is significant or not, the
slope of the quadratic term needs to be tested for significance.
H0: βX.X = 0 i.e. the slope of the quadratic term is zero and hence insignificant.
H1: βX.X ≠ 0 i.e. the slope of the quadratic term is not zero and hence significant.
Based on the given output, it is evident that relevant t statistic associated with the slope
coefficient of quadratic term is 0.95 with a p value of 0.3647. Since the p value is greater than
the level of significance, hence the null hypothesis cannot be rejected. This implies that the
slope coefficient of quadratic term can be assumed as zero.
Hence, the quadratic term does not contribute useful information for estimating the demand
of gem.
Question 5
a) The requisite model is given below.
Paraphrase This Document
Need a fresh take? Get an instant paraphrase of this document with our AI Paraphraser
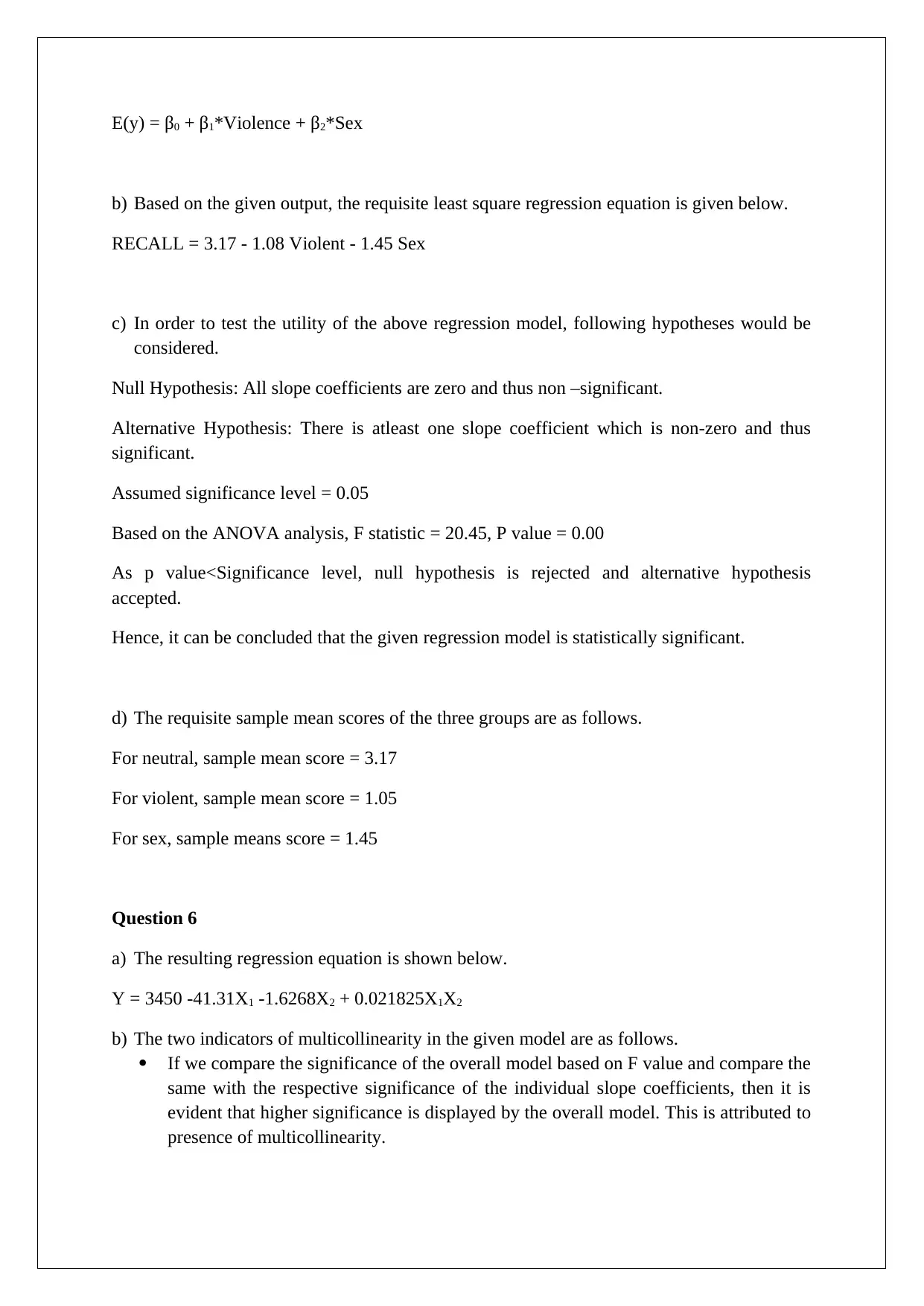
E(y) = β0 + β1*Violence + β2*Sex
b) Based on the given output, the requisite least square regression equation is given below.
RECALL = 3.17 - 1.08 Violent - 1.45 Sex
c) In order to test the utility of the above regression model, following hypotheses would be
considered.
Null Hypothesis: All slope coefficients are zero and thus non –significant.
Alternative Hypothesis: There is atleast one slope coefficient which is non-zero and thus
significant.
Assumed significance level = 0.05
Based on the ANOVA analysis, F statistic = 20.45, P value = 0.00
As p value<Significance level, null hypothesis is rejected and alternative hypothesis
accepted.
Hence, it can be concluded that the given regression model is statistically significant.
d) The requisite sample mean scores of the three groups are as follows.
For neutral, sample mean score = 3.17
For violent, sample mean score = 1.05
For sex, sample means score = 1.45
Question 6
a) The resulting regression equation is shown below.
Y = 3450 -41.31X1 -1.6268X2 + 0.021825X1X2
b) The two indicators of multicollinearity in the given model are as follows.
If we compare the significance of the overall model based on F value and compare the
same with the respective significance of the individual slope coefficients, then it is
evident that higher significance is displayed by the overall model. This is attributed to
presence of multicollinearity.
b) Based on the given output, the requisite least square regression equation is given below.
RECALL = 3.17 - 1.08 Violent - 1.45 Sex
c) In order to test the utility of the above regression model, following hypotheses would be
considered.
Null Hypothesis: All slope coefficients are zero and thus non –significant.
Alternative Hypothesis: There is atleast one slope coefficient which is non-zero and thus
significant.
Assumed significance level = 0.05
Based on the ANOVA analysis, F statistic = 20.45, P value = 0.00
As p value<Significance level, null hypothesis is rejected and alternative hypothesis
accepted.
Hence, it can be concluded that the given regression model is statistically significant.
d) The requisite sample mean scores of the three groups are as follows.
For neutral, sample mean score = 3.17
For violent, sample mean score = 1.05
For sex, sample means score = 1.45
Question 6
a) The resulting regression equation is shown below.
Y = 3450 -41.31X1 -1.6268X2 + 0.021825X1X2
b) The two indicators of multicollinearity in the given model are as follows.
If we compare the significance of the overall model based on F value and compare the
same with the respective significance of the individual slope coefficients, then it is
evident that higher significance is displayed by the overall model. This is attributed to
presence of multicollinearity.
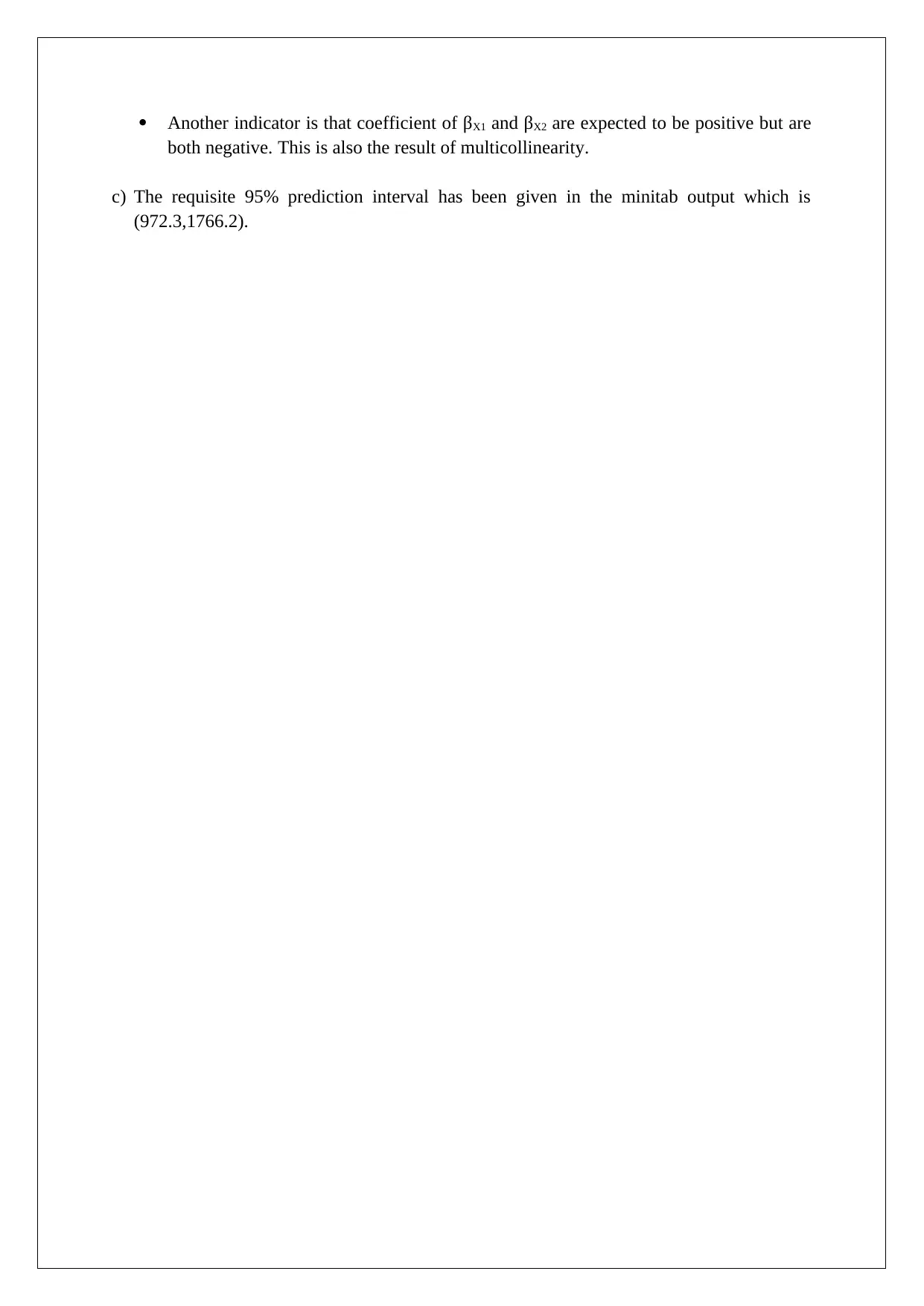
Another indicator is that coefficient of βX1 and βX2 are expected to be positive but are
both negative. This is also the result of multicollinearity.
c) The requisite 95% prediction interval has been given in the minitab output which is
(972.3,1766.2).
both negative. This is also the result of multicollinearity.
c) The requisite 95% prediction interval has been given in the minitab output which is
(972.3,1766.2).
You're viewing a preview
Unlock full access by subscribing today!
1 out of 6
Related Documents

Your All-in-One AI-Powered Toolkit for Academic Success.
+13062052269
info@desklib.com
Available 24*7 on WhatsApp / Email
Unlock your academic potential
© 2024 | Zucol Services PVT LTD | All rights reserved.