In Silico Modelling Toxicology | Assignment
VerifiedAdded on 2022/09/01
|25
|7727
|74
AI Summary
Contribute Materials
Your contribution can guide someone’s learning journey. Share your
documents today.
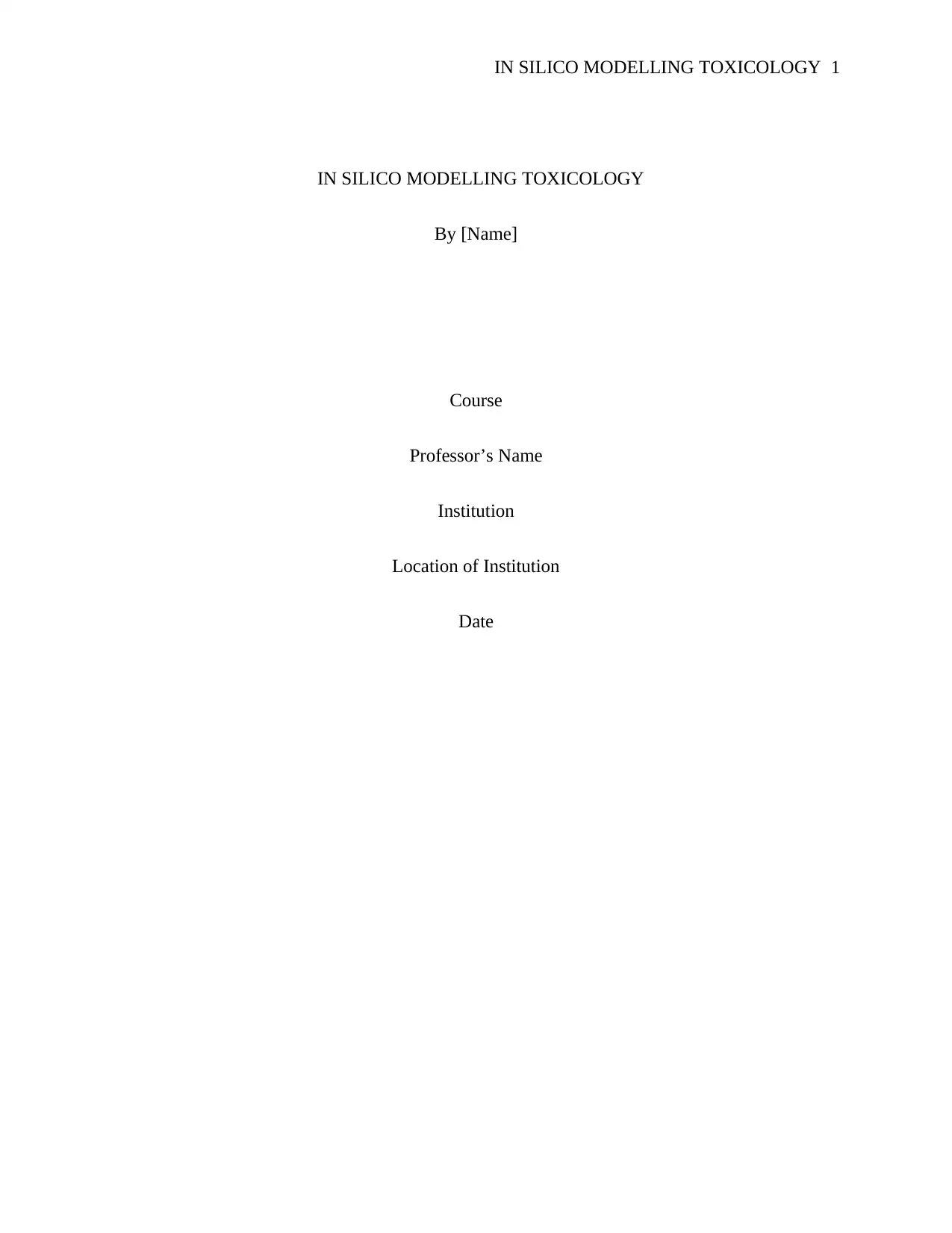
IN SILICO MODELLING TOXICOLOGY 1
IN SILICO MODELLING TOXICOLOGY
By [Name]
Course
Professor’s Name
Institution
Location of Institution
Date
IN SILICO MODELLING TOXICOLOGY
By [Name]
Course
Professor’s Name
Institution
Location of Institution
Date
Secure Best Marks with AI Grader
Need help grading? Try our AI Grader for instant feedback on your assignments.
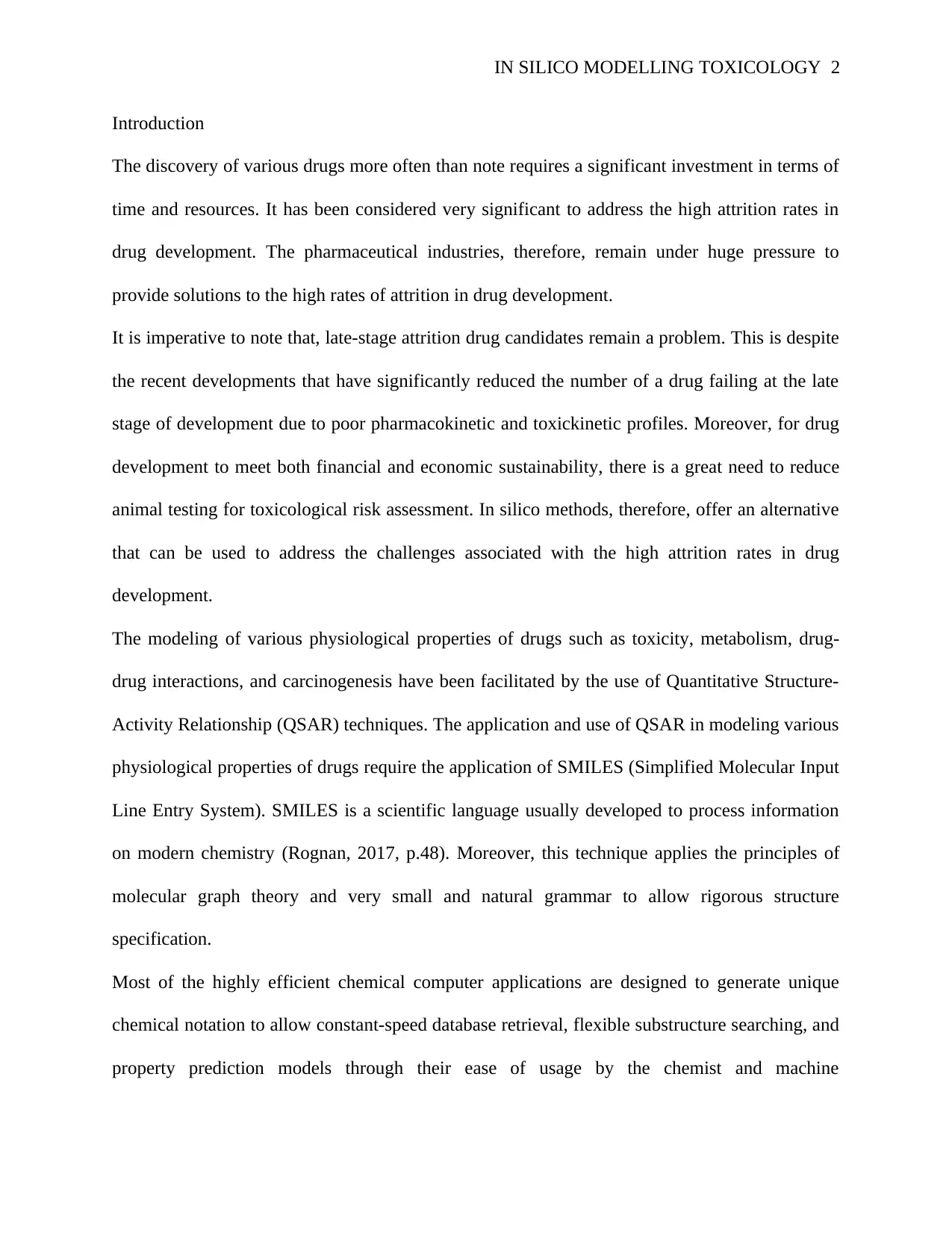
IN SILICO MODELLING TOXICOLOGY 2
Introduction
The discovery of various drugs more often than note requires a significant investment in terms of
time and resources. It has been considered very significant to address the high attrition rates in
drug development. The pharmaceutical industries, therefore, remain under huge pressure to
provide solutions to the high rates of attrition in drug development.
It is imperative to note that, late-stage attrition drug candidates remain a problem. This is despite
the recent developments that have significantly reduced the number of a drug failing at the late
stage of development due to poor pharmacokinetic and toxickinetic profiles. Moreover, for drug
development to meet both financial and economic sustainability, there is a great need to reduce
animal testing for toxicological risk assessment. In silico methods, therefore, offer an alternative
that can be used to address the challenges associated with the high attrition rates in drug
development.
The modeling of various physiological properties of drugs such as toxicity, metabolism, drug-
drug interactions, and carcinogenesis have been facilitated by the use of Quantitative Structure-
Activity Relationship (QSAR) techniques. The application and use of QSAR in modeling various
physiological properties of drugs require the application of SMILES (Simplified Molecular Input
Line Entry System). SMILES is a scientific language usually developed to process information
on modern chemistry (Rognan, 2017, p.48). Moreover, this technique applies the principles of
molecular graph theory and very small and natural grammar to allow rigorous structure
specification.
Most of the highly efficient chemical computer applications are designed to generate unique
chemical notation to allow constant-speed database retrieval, flexible substructure searching, and
property prediction models through their ease of usage by the chemist and machine
Introduction
The discovery of various drugs more often than note requires a significant investment in terms of
time and resources. It has been considered very significant to address the high attrition rates in
drug development. The pharmaceutical industries, therefore, remain under huge pressure to
provide solutions to the high rates of attrition in drug development.
It is imperative to note that, late-stage attrition drug candidates remain a problem. This is despite
the recent developments that have significantly reduced the number of a drug failing at the late
stage of development due to poor pharmacokinetic and toxickinetic profiles. Moreover, for drug
development to meet both financial and economic sustainability, there is a great need to reduce
animal testing for toxicological risk assessment. In silico methods, therefore, offer an alternative
that can be used to address the challenges associated with the high attrition rates in drug
development.
The modeling of various physiological properties of drugs such as toxicity, metabolism, drug-
drug interactions, and carcinogenesis have been facilitated by the use of Quantitative Structure-
Activity Relationship (QSAR) techniques. The application and use of QSAR in modeling various
physiological properties of drugs require the application of SMILES (Simplified Molecular Input
Line Entry System). SMILES is a scientific language usually developed to process information
on modern chemistry (Rognan, 2017, p.48). Moreover, this technique applies the principles of
molecular graph theory and very small and natural grammar to allow rigorous structure
specification.
Most of the highly efficient chemical computer applications are designed to generate unique
chemical notation to allow constant-speed database retrieval, flexible substructure searching, and
property prediction models through their ease of usage by the chemist and machine
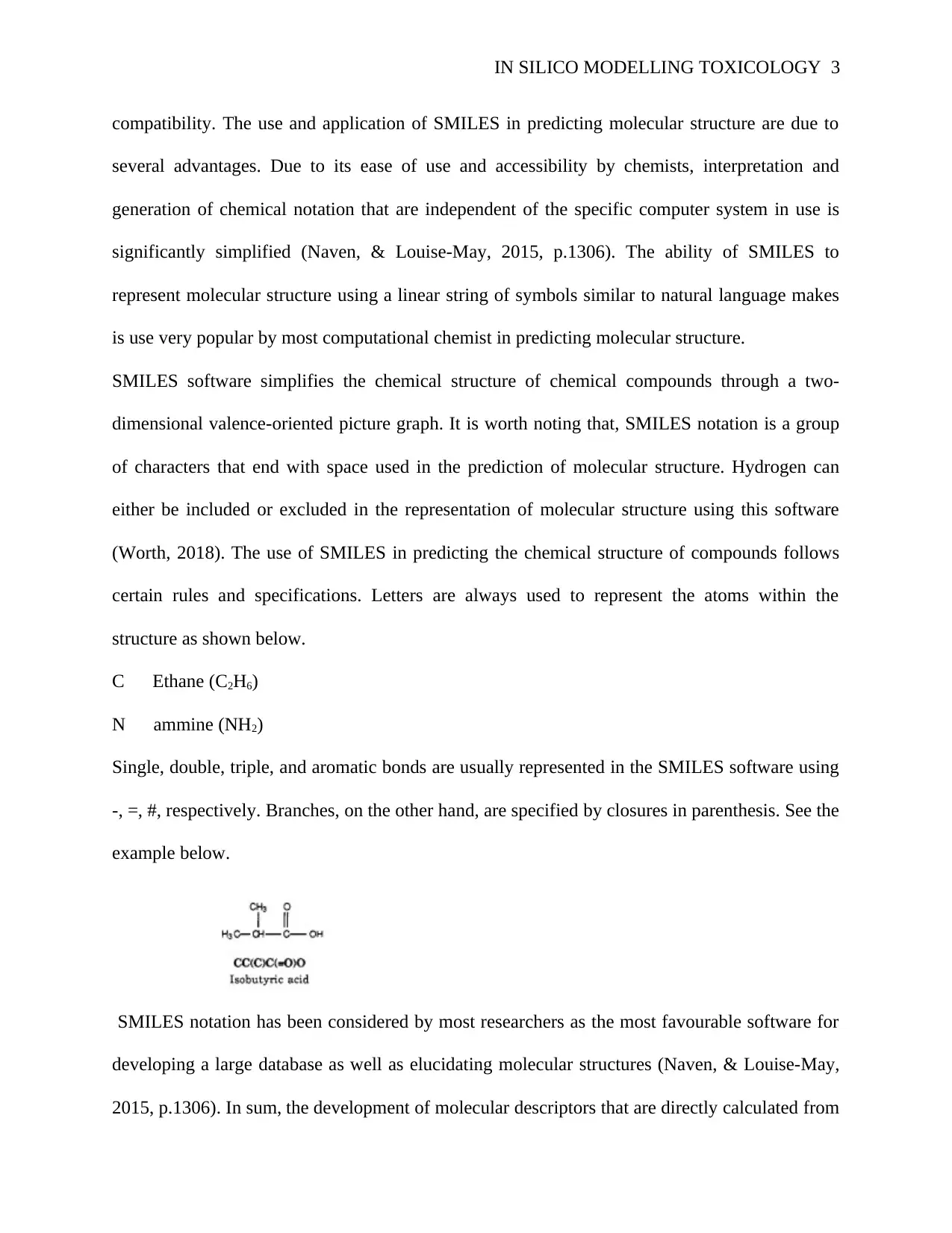
IN SILICO MODELLING TOXICOLOGY 3
compatibility. The use and application of SMILES in predicting molecular structure are due to
several advantages. Due to its ease of use and accessibility by chemists, interpretation and
generation of chemical notation that are independent of the specific computer system in use is
significantly simplified (Naven, & Louise-May, 2015, p.1306). The ability of SMILES to
represent molecular structure using a linear string of symbols similar to natural language makes
is use very popular by most computational chemist in predicting molecular structure.
SMILES software simplifies the chemical structure of chemical compounds through a two-
dimensional valence-oriented picture graph. It is worth noting that, SMILES notation is a group
of characters that end with space used in the prediction of molecular structure. Hydrogen can
either be included or excluded in the representation of molecular structure using this software
(Worth, 2018). The use of SMILES in predicting the chemical structure of compounds follows
certain rules and specifications. Letters are always used to represent the atoms within the
structure as shown below.
C Ethane (C2H6)
N ammine (NH2)
Single, double, triple, and aromatic bonds are usually represented in the SMILES software using
-, =, #, respectively. Branches, on the other hand, are specified by closures in parenthesis. See the
example below.
SMILES notation has been considered by most researchers as the most favourable software for
developing a large database as well as elucidating molecular structures (Naven, & Louise-May,
2015, p.1306). In sum, the development of molecular descriptors that are directly calculated from
compatibility. The use and application of SMILES in predicting molecular structure are due to
several advantages. Due to its ease of use and accessibility by chemists, interpretation and
generation of chemical notation that are independent of the specific computer system in use is
significantly simplified (Naven, & Louise-May, 2015, p.1306). The ability of SMILES to
represent molecular structure using a linear string of symbols similar to natural language makes
is use very popular by most computational chemist in predicting molecular structure.
SMILES software simplifies the chemical structure of chemical compounds through a two-
dimensional valence-oriented picture graph. It is worth noting that, SMILES notation is a group
of characters that end with space used in the prediction of molecular structure. Hydrogen can
either be included or excluded in the representation of molecular structure using this software
(Worth, 2018). The use of SMILES in predicting the chemical structure of compounds follows
certain rules and specifications. Letters are always used to represent the atoms within the
structure as shown below.
C Ethane (C2H6)
N ammine (NH2)
Single, double, triple, and aromatic bonds are usually represented in the SMILES software using
-, =, #, respectively. Branches, on the other hand, are specified by closures in parenthesis. See the
example below.
SMILES notation has been considered by most researchers as the most favourable software for
developing a large database as well as elucidating molecular structures (Naven, & Louise-May,
2015, p.1306). In sum, the development of molecular descriptors that are directly calculated from
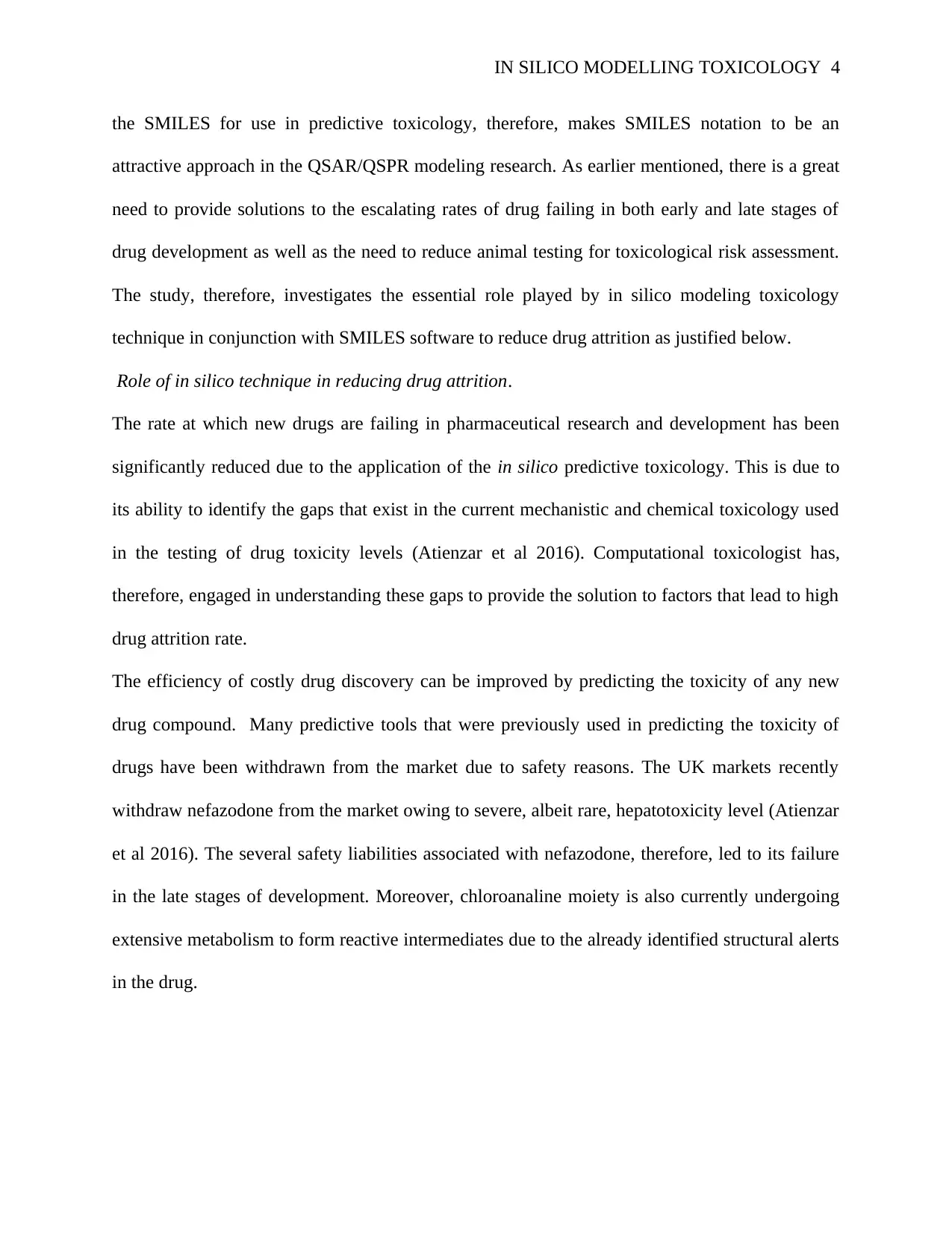
IN SILICO MODELLING TOXICOLOGY 4
the SMILES for use in predictive toxicology, therefore, makes SMILES notation to be an
attractive approach in the QSAR/QSPR modeling research. As earlier mentioned, there is a great
need to provide solutions to the escalating rates of drug failing in both early and late stages of
drug development as well as the need to reduce animal testing for toxicological risk assessment.
The study, therefore, investigates the essential role played by in silico modeling toxicology
technique in conjunction with SMILES software to reduce drug attrition as justified below.
Role of in silico technique in reducing drug attrition.
The rate at which new drugs are failing in pharmaceutical research and development has been
significantly reduced due to the application of the in silico predictive toxicology. This is due to
its ability to identify the gaps that exist in the current mechanistic and chemical toxicology used
in the testing of drug toxicity levels (Atienzar et al 2016). Computational toxicologist has,
therefore, engaged in understanding these gaps to provide the solution to factors that lead to high
drug attrition rate.
The efficiency of costly drug discovery can be improved by predicting the toxicity of any new
drug compound. Many predictive tools that were previously used in predicting the toxicity of
drugs have been withdrawn from the market due to safety reasons. The UK markets recently
withdraw nefazodone from the market owing to severe, albeit rare, hepatotoxicity level (Atienzar
et al 2016). The several safety liabilities associated with nefazodone, therefore, led to its failure
in the late stages of development. Moreover, chloroanaline moiety is also currently undergoing
extensive metabolism to form reactive intermediates due to the already identified structural alerts
in the drug.
the SMILES for use in predictive toxicology, therefore, makes SMILES notation to be an
attractive approach in the QSAR/QSPR modeling research. As earlier mentioned, there is a great
need to provide solutions to the escalating rates of drug failing in both early and late stages of
drug development as well as the need to reduce animal testing for toxicological risk assessment.
The study, therefore, investigates the essential role played by in silico modeling toxicology
technique in conjunction with SMILES software to reduce drug attrition as justified below.
Role of in silico technique in reducing drug attrition.
The rate at which new drugs are failing in pharmaceutical research and development has been
significantly reduced due to the application of the in silico predictive toxicology. This is due to
its ability to identify the gaps that exist in the current mechanistic and chemical toxicology used
in the testing of drug toxicity levels (Atienzar et al 2016). Computational toxicologist has,
therefore, engaged in understanding these gaps to provide the solution to factors that lead to high
drug attrition rate.
The efficiency of costly drug discovery can be improved by predicting the toxicity of any new
drug compound. Many predictive tools that were previously used in predicting the toxicity of
drugs have been withdrawn from the market due to safety reasons. The UK markets recently
withdraw nefazodone from the market owing to severe, albeit rare, hepatotoxicity level (Atienzar
et al 2016). The several safety liabilities associated with nefazodone, therefore, led to its failure
in the late stages of development. Moreover, chloroanaline moiety is also currently undergoing
extensive metabolism to form reactive intermediates due to the already identified structural alerts
in the drug.
Secure Best Marks with AI Grader
Need help grading? Try our AI Grader for instant feedback on your assignments.
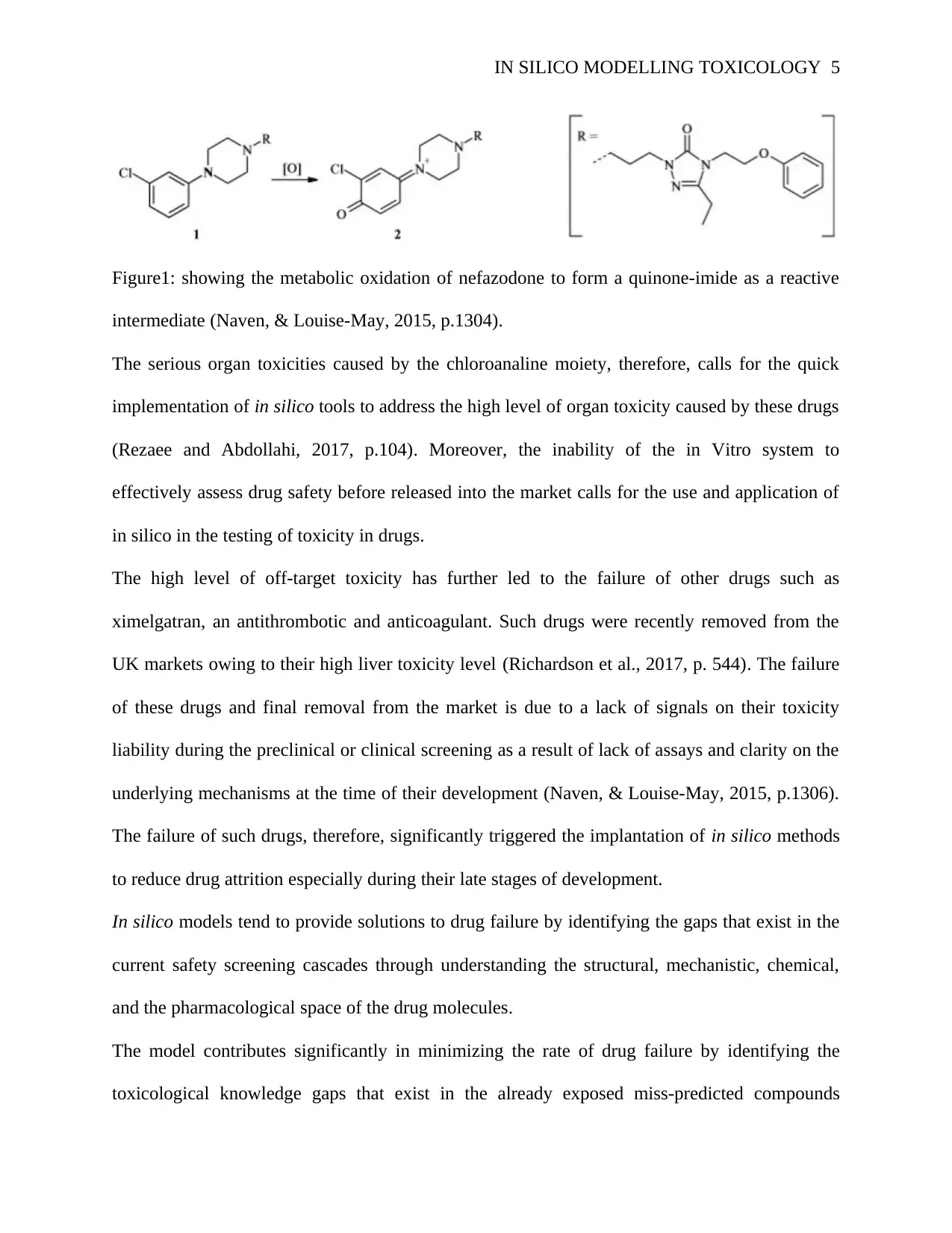
IN SILICO MODELLING TOXICOLOGY 5
Figure1: showing the metabolic oxidation of nefazodone to form a quinone-imide as a reactive
intermediate (Naven, & Louise-May, 2015, p.1304).
The serious organ toxicities caused by the chloroanaline moiety, therefore, calls for the quick
implementation of in silico tools to address the high level of organ toxicity caused by these drugs
(Rezaee and Abdollahi, 2017, p.104). Moreover, the inability of the in Vitro system to
effectively assess drug safety before released into the market calls for the use and application of
in silico in the testing of toxicity in drugs.
The high level of off-target toxicity has further led to the failure of other drugs such as
ximelgatran, an antithrombotic and anticoagulant. Such drugs were recently removed from the
UK markets owing to their high liver toxicity level (Richardson et al., 2017, p. 544). The failure
of these drugs and final removal from the market is due to a lack of signals on their toxicity
liability during the preclinical or clinical screening as a result of lack of assays and clarity on the
underlying mechanisms at the time of their development (Naven, & Louise-May, 2015, p.1306).
The failure of such drugs, therefore, significantly triggered the implantation of in silico methods
to reduce drug attrition especially during their late stages of development.
In silico models tend to provide solutions to drug failure by identifying the gaps that exist in the
current safety screening cascades through understanding the structural, mechanistic, chemical,
and the pharmacological space of the drug molecules.
The model contributes significantly in minimizing the rate of drug failure by identifying the
toxicological knowledge gaps that exist in the already exposed miss-predicted compounds
Figure1: showing the metabolic oxidation of nefazodone to form a quinone-imide as a reactive
intermediate (Naven, & Louise-May, 2015, p.1304).
The serious organ toxicities caused by the chloroanaline moiety, therefore, calls for the quick
implementation of in silico tools to address the high level of organ toxicity caused by these drugs
(Rezaee and Abdollahi, 2017, p.104). Moreover, the inability of the in Vitro system to
effectively assess drug safety before released into the market calls for the use and application of
in silico in the testing of toxicity in drugs.
The high level of off-target toxicity has further led to the failure of other drugs such as
ximelgatran, an antithrombotic and anticoagulant. Such drugs were recently removed from the
UK markets owing to their high liver toxicity level (Richardson et al., 2017, p. 544). The failure
of these drugs and final removal from the market is due to a lack of signals on their toxicity
liability during the preclinical or clinical screening as a result of lack of assays and clarity on the
underlying mechanisms at the time of their development (Naven, & Louise-May, 2015, p.1306).
The failure of such drugs, therefore, significantly triggered the implantation of in silico methods
to reduce drug attrition especially during their late stages of development.
In silico models tend to provide solutions to drug failure by identifying the gaps that exist in the
current safety screening cascades through understanding the structural, mechanistic, chemical,
and the pharmacological space of the drug molecules.
The model contributes significantly in minimizing the rate of drug failure by identifying the
toxicological knowledge gaps that exist in the already exposed miss-predicted compounds
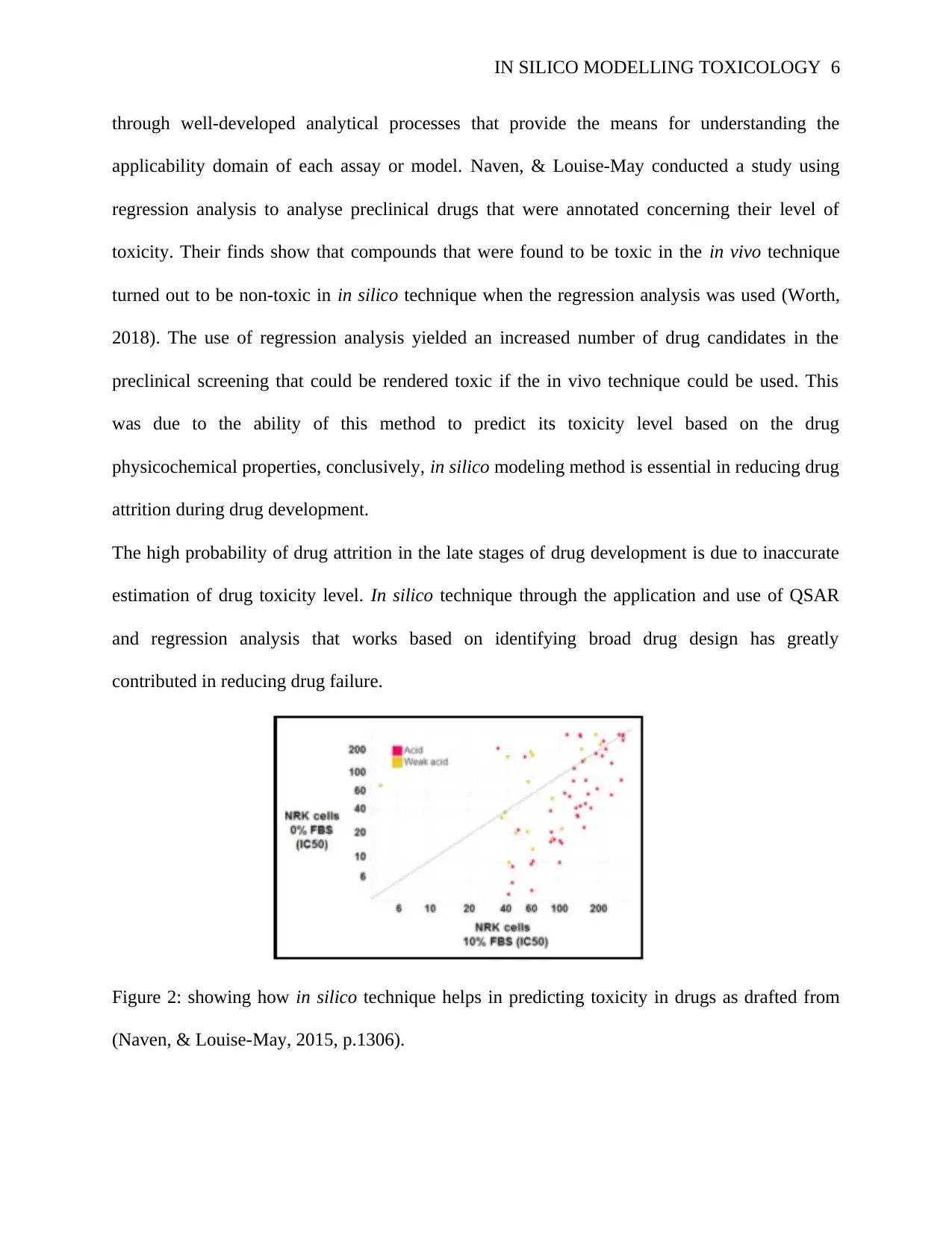
IN SILICO MODELLING TOXICOLOGY 6
through well-developed analytical processes that provide the means for understanding the
applicability domain of each assay or model. Naven, & Louise-May conducted a study using
regression analysis to analyse preclinical drugs that were annotated concerning their level of
toxicity. Their finds show that compounds that were found to be toxic in the in vivo technique
turned out to be non-toxic in in silico technique when the regression analysis was used (Worth,
2018). The use of regression analysis yielded an increased number of drug candidates in the
preclinical screening that could be rendered toxic if the in vivo technique could be used. This
was due to the ability of this method to predict its toxicity level based on the drug
physicochemical properties, conclusively, in silico modeling method is essential in reducing drug
attrition during drug development.
The high probability of drug attrition in the late stages of drug development is due to inaccurate
estimation of drug toxicity level. In silico technique through the application and use of QSAR
and regression analysis that works based on identifying broad drug design has greatly
contributed in reducing drug failure.
Figure 2: showing how in silico technique helps in predicting toxicity in drugs as drafted from
(Naven, & Louise-May, 2015, p.1306).
through well-developed analytical processes that provide the means for understanding the
applicability domain of each assay or model. Naven, & Louise-May conducted a study using
regression analysis to analyse preclinical drugs that were annotated concerning their level of
toxicity. Their finds show that compounds that were found to be toxic in the in vivo technique
turned out to be non-toxic in in silico technique when the regression analysis was used (Worth,
2018). The use of regression analysis yielded an increased number of drug candidates in the
preclinical screening that could be rendered toxic if the in vivo technique could be used. This
was due to the ability of this method to predict its toxicity level based on the drug
physicochemical properties, conclusively, in silico modeling method is essential in reducing drug
attrition during drug development.
The high probability of drug attrition in the late stages of drug development is due to inaccurate
estimation of drug toxicity level. In silico technique through the application and use of QSAR
and regression analysis that works based on identifying broad drug design has greatly
contributed in reducing drug failure.
Figure 2: showing how in silico technique helps in predicting toxicity in drugs as drafted from
(Naven, & Louise-May, 2015, p.1306).
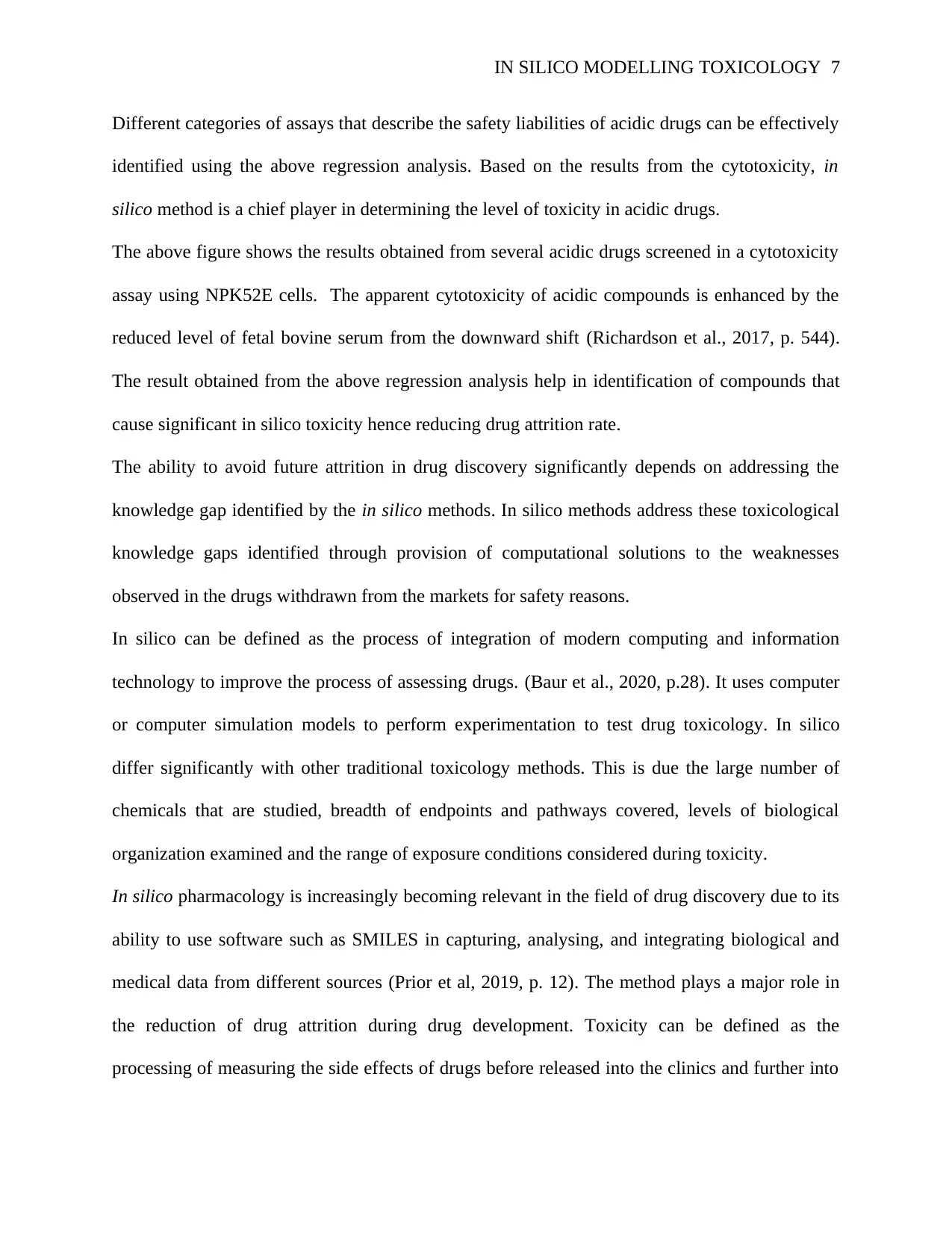
IN SILICO MODELLING TOXICOLOGY 7
Different categories of assays that describe the safety liabilities of acidic drugs can be effectively
identified using the above regression analysis. Based on the results from the cytotoxicity, in
silico method is a chief player in determining the level of toxicity in acidic drugs.
The above figure shows the results obtained from several acidic drugs screened in a cytotoxicity
assay using NPK52E cells. The apparent cytotoxicity of acidic compounds is enhanced by the
reduced level of fetal bovine serum from the downward shift (Richardson et al., 2017, p. 544).
The result obtained from the above regression analysis help in identification of compounds that
cause significant in silico toxicity hence reducing drug attrition rate.
The ability to avoid future attrition in drug discovery significantly depends on addressing the
knowledge gap identified by the in silico methods. In silico methods address these toxicological
knowledge gaps identified through provision of computational solutions to the weaknesses
observed in the drugs withdrawn from the markets for safety reasons.
In silico can be defined as the process of integration of modern computing and information
technology to improve the process of assessing drugs. (Baur et al., 2020, p.28). It uses computer
or computer simulation models to perform experimentation to test drug toxicology. In silico
differ significantly with other traditional toxicology methods. This is due the large number of
chemicals that are studied, breadth of endpoints and pathways covered, levels of biological
organization examined and the range of exposure conditions considered during toxicity.
In silico pharmacology is increasingly becoming relevant in the field of drug discovery due to its
ability to use software such as SMILES in capturing, analysing, and integrating biological and
medical data from different sources (Prior et al, 2019, p. 12). The method plays a major role in
the reduction of drug attrition during drug development. Toxicity can be defined as the
processing of measuring the side effects of drugs before released into the clinics and further into
Different categories of assays that describe the safety liabilities of acidic drugs can be effectively
identified using the above regression analysis. Based on the results from the cytotoxicity, in
silico method is a chief player in determining the level of toxicity in acidic drugs.
The above figure shows the results obtained from several acidic drugs screened in a cytotoxicity
assay using NPK52E cells. The apparent cytotoxicity of acidic compounds is enhanced by the
reduced level of fetal bovine serum from the downward shift (Richardson et al., 2017, p. 544).
The result obtained from the above regression analysis help in identification of compounds that
cause significant in silico toxicity hence reducing drug attrition rate.
The ability to avoid future attrition in drug discovery significantly depends on addressing the
knowledge gap identified by the in silico methods. In silico methods address these toxicological
knowledge gaps identified through provision of computational solutions to the weaknesses
observed in the drugs withdrawn from the markets for safety reasons.
In silico can be defined as the process of integration of modern computing and information
technology to improve the process of assessing drugs. (Baur et al., 2020, p.28). It uses computer
or computer simulation models to perform experimentation to test drug toxicology. In silico
differ significantly with other traditional toxicology methods. This is due the large number of
chemicals that are studied, breadth of endpoints and pathways covered, levels of biological
organization examined and the range of exposure conditions considered during toxicity.
In silico pharmacology is increasingly becoming relevant in the field of drug discovery due to its
ability to use software such as SMILES in capturing, analysing, and integrating biological and
medical data from different sources (Prior et al, 2019, p. 12). The method plays a major role in
the reduction of drug attrition during drug development. Toxicity can be defined as the
processing of measuring the side effects of drugs before released into the clinics and further into
Paraphrase This Document
Need a fresh take? Get an instant paraphrase of this document with our AI Paraphraser
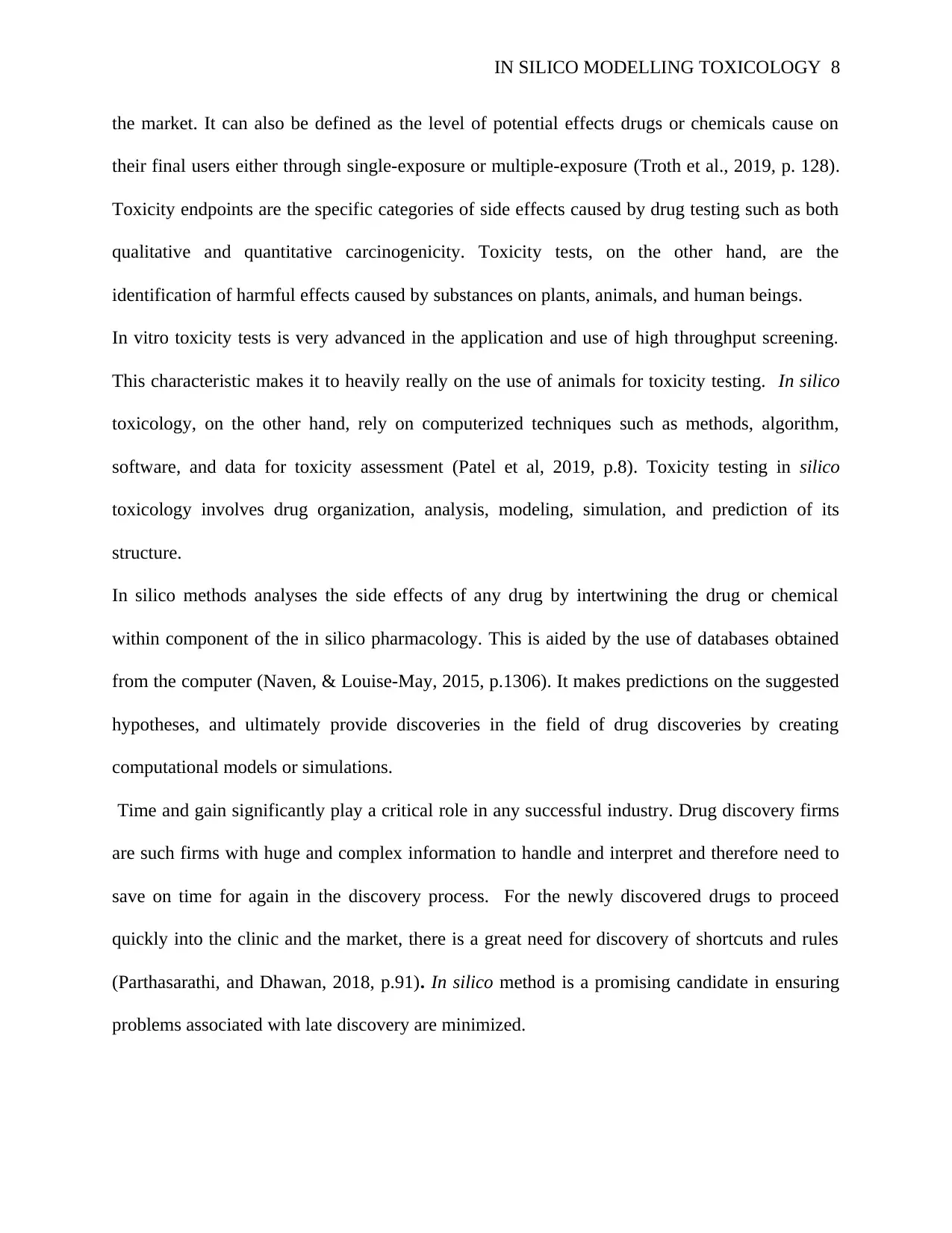
IN SILICO MODELLING TOXICOLOGY 8
the market. It can also be defined as the level of potential effects drugs or chemicals cause on
their final users either through single-exposure or multiple-exposure (Troth et al., 2019, p. 128).
Toxicity endpoints are the specific categories of side effects caused by drug testing such as both
qualitative and quantitative carcinogenicity. Toxicity tests, on the other hand, are the
identification of harmful effects caused by substances on plants, animals, and human beings.
In vitro toxicity tests is very advanced in the application and use of high throughput screening.
This characteristic makes it to heavily really on the use of animals for toxicity testing. In silico
toxicology, on the other hand, rely on computerized techniques such as methods, algorithm,
software, and data for toxicity assessment (Patel et al, 2019, p.8). Toxicity testing in silico
toxicology involves drug organization, analysis, modeling, simulation, and prediction of its
structure.
In silico methods analyses the side effects of any drug by intertwining the drug or chemical
within component of the in silico pharmacology. This is aided by the use of databases obtained
from the computer (Naven, & Louise-May, 2015, p.1306). It makes predictions on the suggested
hypotheses, and ultimately provide discoveries in the field of drug discoveries by creating
computational models or simulations.
Time and gain significantly play a critical role in any successful industry. Drug discovery firms
are such firms with huge and complex information to handle and interpret and therefore need to
save on time for again in the discovery process. For the newly discovered drugs to proceed
quickly into the clinic and the market, there is a great need for discovery of shortcuts and rules
(Parthasarathi, and Dhawan, 2018, p.91). In silico method is a promising candidate in ensuring
problems associated with late discovery are minimized.
the market. It can also be defined as the level of potential effects drugs or chemicals cause on
their final users either through single-exposure or multiple-exposure (Troth et al., 2019, p. 128).
Toxicity endpoints are the specific categories of side effects caused by drug testing such as both
qualitative and quantitative carcinogenicity. Toxicity tests, on the other hand, are the
identification of harmful effects caused by substances on plants, animals, and human beings.
In vitro toxicity tests is very advanced in the application and use of high throughput screening.
This characteristic makes it to heavily really on the use of animals for toxicity testing. In silico
toxicology, on the other hand, rely on computerized techniques such as methods, algorithm,
software, and data for toxicity assessment (Patel et al, 2019, p.8). Toxicity testing in silico
toxicology involves drug organization, analysis, modeling, simulation, and prediction of its
structure.
In silico methods analyses the side effects of any drug by intertwining the drug or chemical
within component of the in silico pharmacology. This is aided by the use of databases obtained
from the computer (Naven, & Louise-May, 2015, p.1306). It makes predictions on the suggested
hypotheses, and ultimately provide discoveries in the field of drug discoveries by creating
computational models or simulations.
Time and gain significantly play a critical role in any successful industry. Drug discovery firms
are such firms with huge and complex information to handle and interpret and therefore need to
save on time for again in the discovery process. For the newly discovered drugs to proceed
quickly into the clinic and the market, there is a great need for discovery of shortcuts and rules
(Parthasarathi, and Dhawan, 2018, p.91). In silico method is a promising candidate in ensuring
problems associated with late discovery are minimized.
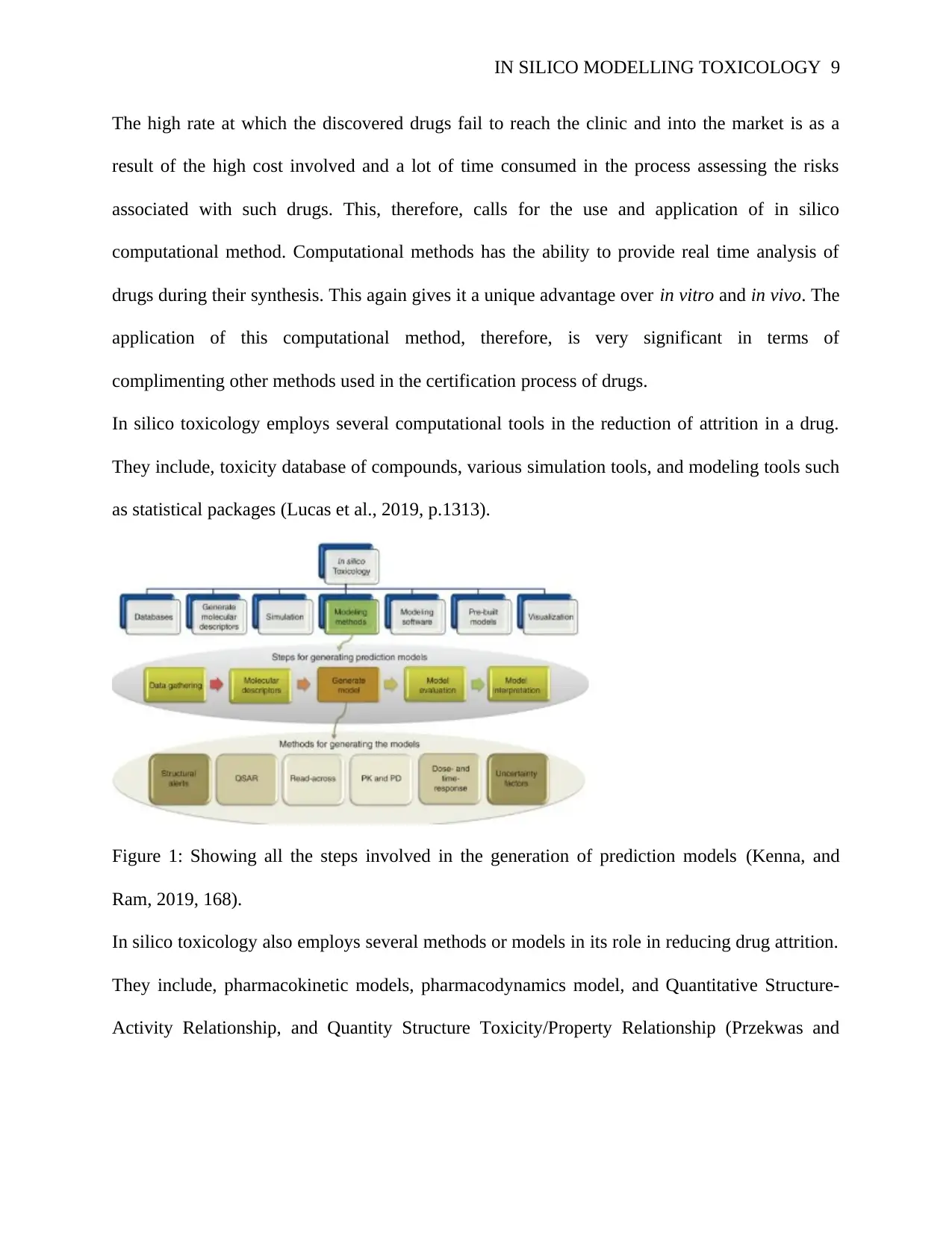
IN SILICO MODELLING TOXICOLOGY 9
The high rate at which the discovered drugs fail to reach the clinic and into the market is as a
result of the high cost involved and a lot of time consumed in the process assessing the risks
associated with such drugs. This, therefore, calls for the use and application of in silico
computational method. Computational methods has the ability to provide real time analysis of
drugs during their synthesis. This again gives it a unique advantage over in vitro and in vivo. The
application of this computational method, therefore, is very significant in terms of
complimenting other methods used in the certification process of drugs.
In silico toxicology employs several computational tools in the reduction of attrition in a drug.
They include, toxicity database of compounds, various simulation tools, and modeling tools such
as statistical packages (Lucas et al., 2019, p.1313).
Figure 1: Showing all the steps involved in the generation of prediction models (Kenna, and
Ram, 2019, 168).
In silico toxicology also employs several methods or models in its role in reducing drug attrition.
They include, pharmacokinetic models, pharmacodynamics model, and Quantitative Structure-
Activity Relationship, and Quantity Structure Toxicity/Property Relationship (Przekwas and
The high rate at which the discovered drugs fail to reach the clinic and into the market is as a
result of the high cost involved and a lot of time consumed in the process assessing the risks
associated with such drugs. This, therefore, calls for the use and application of in silico
computational method. Computational methods has the ability to provide real time analysis of
drugs during their synthesis. This again gives it a unique advantage over in vitro and in vivo. The
application of this computational method, therefore, is very significant in terms of
complimenting other methods used in the certification process of drugs.
In silico toxicology employs several computational tools in the reduction of attrition in a drug.
They include, toxicity database of compounds, various simulation tools, and modeling tools such
as statistical packages (Lucas et al., 2019, p.1313).
Figure 1: Showing all the steps involved in the generation of prediction models (Kenna, and
Ram, 2019, 168).
In silico toxicology also employs several methods or models in its role in reducing drug attrition.
They include, pharmacokinetic models, pharmacodynamics model, and Quantitative Structure-
Activity Relationship, and Quantity Structure Toxicity/Property Relationship (Przekwas and
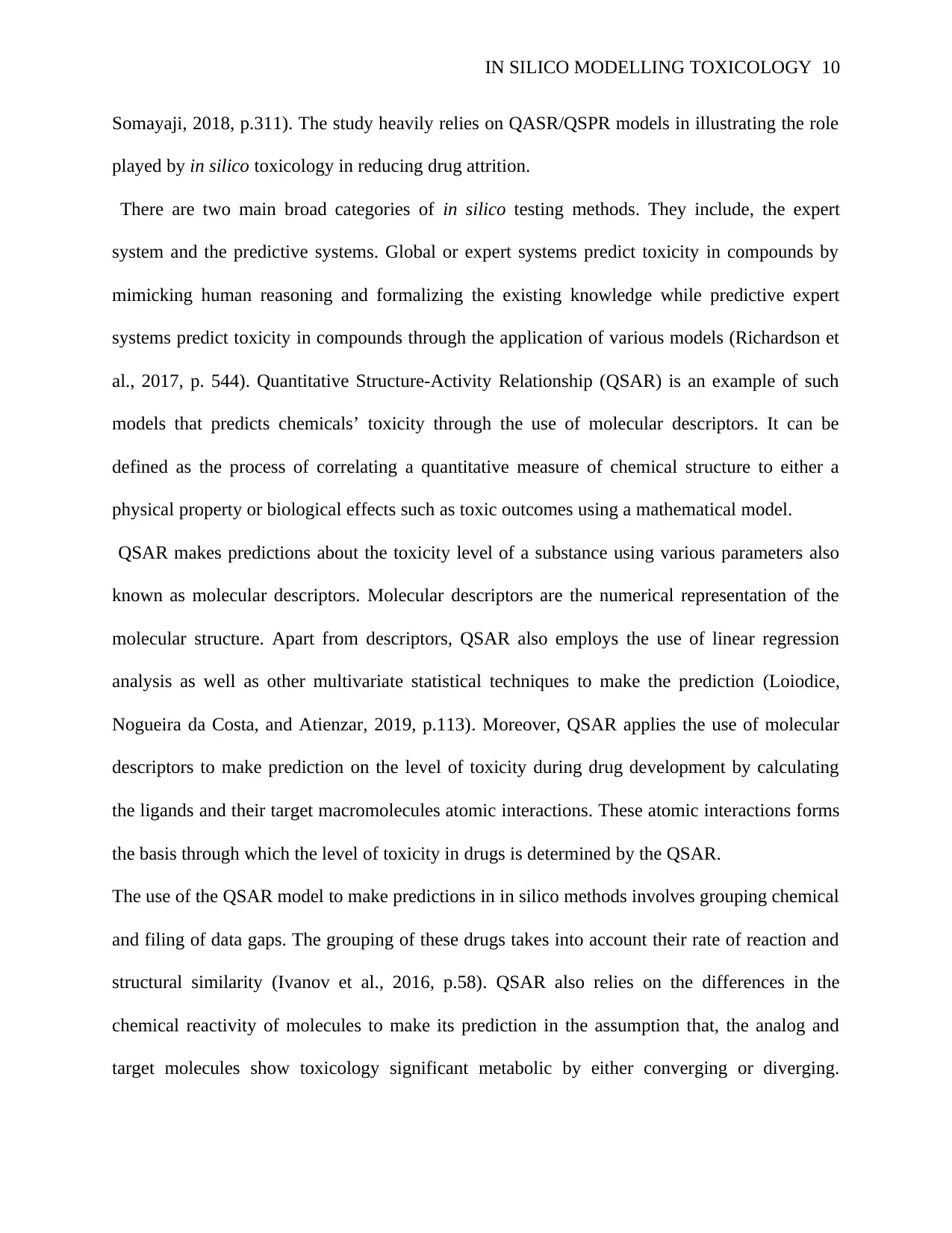
IN SILICO MODELLING TOXICOLOGY 10
Somayaji, 2018, p.311). The study heavily relies on QASR/QSPR models in illustrating the role
played by in silico toxicology in reducing drug attrition.
There are two main broad categories of in silico testing methods. They include, the expert
system and the predictive systems. Global or expert systems predict toxicity in compounds by
mimicking human reasoning and formalizing the existing knowledge while predictive expert
systems predict toxicity in compounds through the application of various models (Richardson et
al., 2017, p. 544). Quantitative Structure-Activity Relationship (QSAR) is an example of such
models that predicts chemicals’ toxicity through the use of molecular descriptors. It can be
defined as the process of correlating a quantitative measure of chemical structure to either a
physical property or biological effects such as toxic outcomes using a mathematical model.
QSAR makes predictions about the toxicity level of a substance using various parameters also
known as molecular descriptors. Molecular descriptors are the numerical representation of the
molecular structure. Apart from descriptors, QSAR also employs the use of linear regression
analysis as well as other multivariate statistical techniques to make the prediction (Loiodice,
Nogueira da Costa, and Atienzar, 2019, p.113). Moreover, QSAR applies the use of molecular
descriptors to make prediction on the level of toxicity during drug development by calculating
the ligands and their target macromolecules atomic interactions. These atomic interactions forms
the basis through which the level of toxicity in drugs is determined by the QSAR.
The use of the QSAR model to make predictions in in silico methods involves grouping chemical
and filing of data gaps. The grouping of these drugs takes into account their rate of reaction and
structural similarity (Ivanov et al., 2016, p.58). QSAR also relies on the differences in the
chemical reactivity of molecules to make its prediction in the assumption that, the analog and
target molecules show toxicology significant metabolic by either converging or diverging.
Somayaji, 2018, p.311). The study heavily relies on QASR/QSPR models in illustrating the role
played by in silico toxicology in reducing drug attrition.
There are two main broad categories of in silico testing methods. They include, the expert
system and the predictive systems. Global or expert systems predict toxicity in compounds by
mimicking human reasoning and formalizing the existing knowledge while predictive expert
systems predict toxicity in compounds through the application of various models (Richardson et
al., 2017, p. 544). Quantitative Structure-Activity Relationship (QSAR) is an example of such
models that predicts chemicals’ toxicity through the use of molecular descriptors. It can be
defined as the process of correlating a quantitative measure of chemical structure to either a
physical property or biological effects such as toxic outcomes using a mathematical model.
QSAR makes predictions about the toxicity level of a substance using various parameters also
known as molecular descriptors. Molecular descriptors are the numerical representation of the
molecular structure. Apart from descriptors, QSAR also employs the use of linear regression
analysis as well as other multivariate statistical techniques to make the prediction (Loiodice,
Nogueira da Costa, and Atienzar, 2019, p.113). Moreover, QSAR applies the use of molecular
descriptors to make prediction on the level of toxicity during drug development by calculating
the ligands and their target macromolecules atomic interactions. These atomic interactions forms
the basis through which the level of toxicity in drugs is determined by the QSAR.
The use of the QSAR model to make predictions in in silico methods involves grouping chemical
and filing of data gaps. The grouping of these drugs takes into account their rate of reaction and
structural similarity (Ivanov et al., 2016, p.58). QSAR also relies on the differences in the
chemical reactivity of molecules to make its prediction in the assumption that, the analog and
target molecules show toxicology significant metabolic by either converging or diverging.
Secure Best Marks with AI Grader
Need help grading? Try our AI Grader for instant feedback on your assignments.
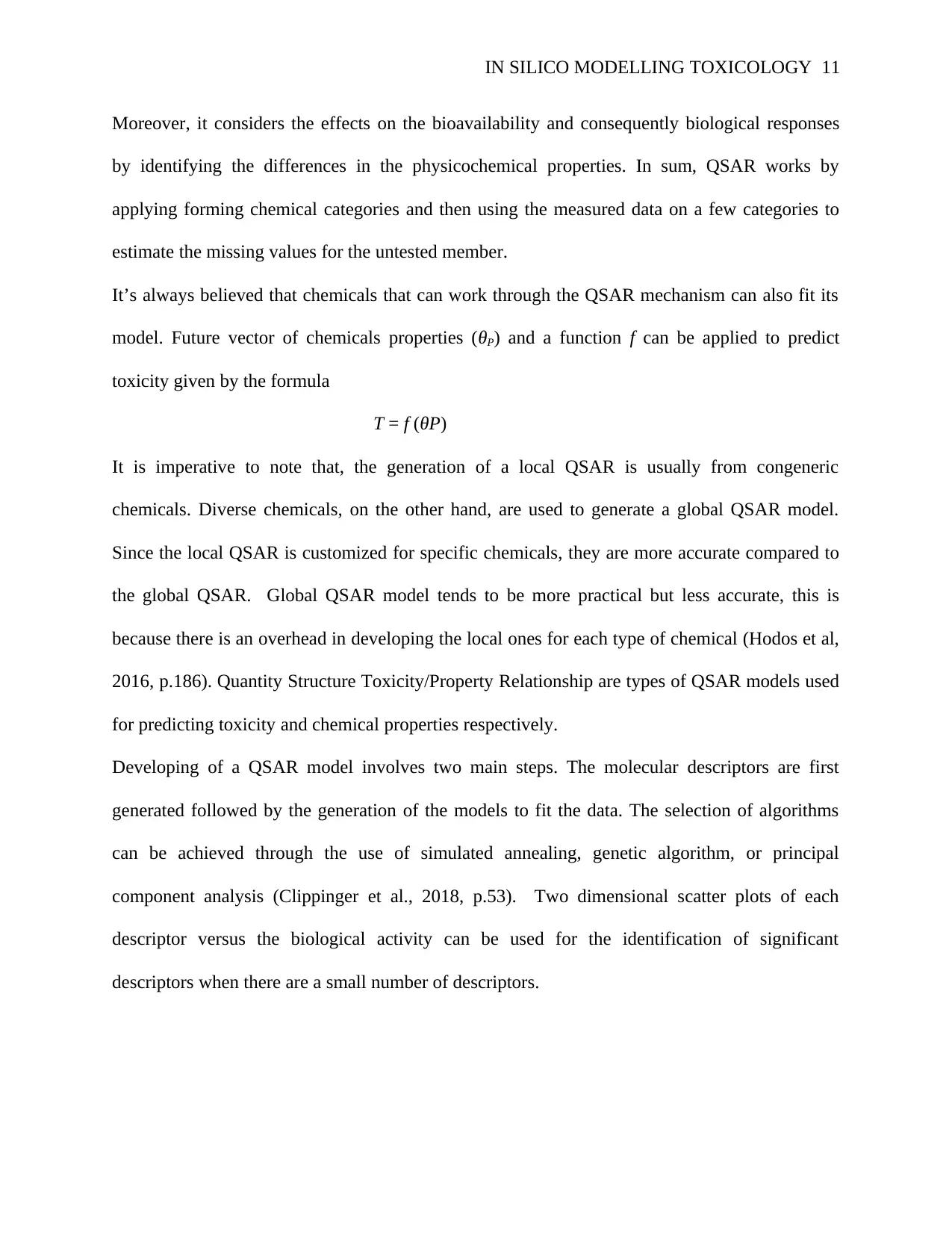
IN SILICO MODELLING TOXICOLOGY 11
Moreover, it considers the effects on the bioavailability and consequently biological responses
by identifying the differences in the physicochemical properties. In sum, QSAR works by
applying forming chemical categories and then using the measured data on a few categories to
estimate the missing values for the untested member.
It’s always believed that chemicals that can work through the QSAR mechanism can also fit its
model. Future vector of chemicals properties (θP) and a function f can be applied to predict
toxicity given by the formula
T = f (θP)
It is imperative to note that, the generation of a local QSAR is usually from congeneric
chemicals. Diverse chemicals, on the other hand, are used to generate a global QSAR model.
Since the local QSAR is customized for specific chemicals, they are more accurate compared to
the global QSAR. Global QSAR model tends to be more practical but less accurate, this is
because there is an overhead in developing the local ones for each type of chemical (Hodos et al,
2016, p.186). Quantity Structure Toxicity/Property Relationship are types of QSAR models used
for predicting toxicity and chemical properties respectively.
Developing of a QSAR model involves two main steps. The molecular descriptors are first
generated followed by the generation of the models to fit the data. The selection of algorithms
can be achieved through the use of simulated annealing, genetic algorithm, or principal
component analysis (Clippinger et al., 2018, p.53). Two dimensional scatter plots of each
descriptor versus the biological activity can be used for the identification of significant
descriptors when there are a small number of descriptors.
Moreover, it considers the effects on the bioavailability and consequently biological responses
by identifying the differences in the physicochemical properties. In sum, QSAR works by
applying forming chemical categories and then using the measured data on a few categories to
estimate the missing values for the untested member.
It’s always believed that chemicals that can work through the QSAR mechanism can also fit its
model. Future vector of chemicals properties (θP) and a function f can be applied to predict
toxicity given by the formula
T = f (θP)
It is imperative to note that, the generation of a local QSAR is usually from congeneric
chemicals. Diverse chemicals, on the other hand, are used to generate a global QSAR model.
Since the local QSAR is customized for specific chemicals, they are more accurate compared to
the global QSAR. Global QSAR model tends to be more practical but less accurate, this is
because there is an overhead in developing the local ones for each type of chemical (Hodos et al,
2016, p.186). Quantity Structure Toxicity/Property Relationship are types of QSAR models used
for predicting toxicity and chemical properties respectively.
Developing of a QSAR model involves two main steps. The molecular descriptors are first
generated followed by the generation of the models to fit the data. The selection of algorithms
can be achieved through the use of simulated annealing, genetic algorithm, or principal
component analysis (Clippinger et al., 2018, p.53). Two dimensional scatter plots of each
descriptor versus the biological activity can be used for the identification of significant
descriptors when there are a small number of descriptors.
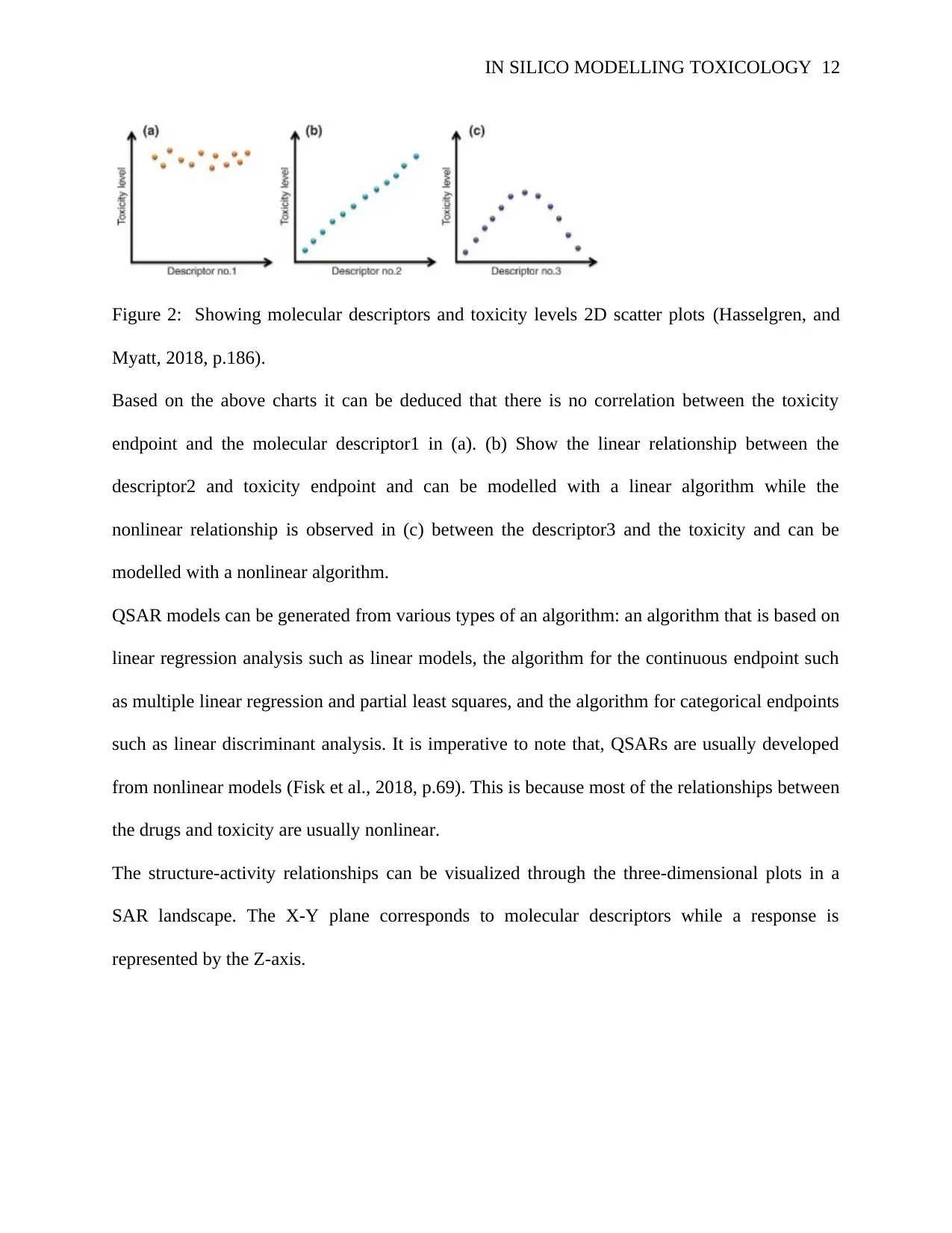
IN SILICO MODELLING TOXICOLOGY 12
Figure 2: Showing molecular descriptors and toxicity levels 2D scatter plots (Hasselgren, and
Myatt, 2018, p.186).
Based on the above charts it can be deduced that there is no correlation between the toxicity
endpoint and the molecular descriptor1 in (a). (b) Show the linear relationship between the
descriptor2 and toxicity endpoint and can be modelled with a linear algorithm while the
nonlinear relationship is observed in (c) between the descriptor3 and the toxicity and can be
modelled with a nonlinear algorithm.
QSAR models can be generated from various types of an algorithm: an algorithm that is based on
linear regression analysis such as linear models, the algorithm for the continuous endpoint such
as multiple linear regression and partial least squares, and the algorithm for categorical endpoints
such as linear discriminant analysis. It is imperative to note that, QSARs are usually developed
from nonlinear models (Fisk et al., 2018, p.69). This is because most of the relationships between
the drugs and toxicity are usually nonlinear.
The structure-activity relationships can be visualized through the three-dimensional plots in a
SAR landscape. The X-Y plane corresponds to molecular descriptors while a response is
represented by the Z-axis.
Figure 2: Showing molecular descriptors and toxicity levels 2D scatter plots (Hasselgren, and
Myatt, 2018, p.186).
Based on the above charts it can be deduced that there is no correlation between the toxicity
endpoint and the molecular descriptor1 in (a). (b) Show the linear relationship between the
descriptor2 and toxicity endpoint and can be modelled with a linear algorithm while the
nonlinear relationship is observed in (c) between the descriptor3 and the toxicity and can be
modelled with a nonlinear algorithm.
QSAR models can be generated from various types of an algorithm: an algorithm that is based on
linear regression analysis such as linear models, the algorithm for the continuous endpoint such
as multiple linear regression and partial least squares, and the algorithm for categorical endpoints
such as linear discriminant analysis. It is imperative to note that, QSARs are usually developed
from nonlinear models (Fisk et al., 2018, p.69). This is because most of the relationships between
the drugs and toxicity are usually nonlinear.
The structure-activity relationships can be visualized through the three-dimensional plots in a
SAR landscape. The X-Y plane corresponds to molecular descriptors while a response is
represented by the Z-axis.
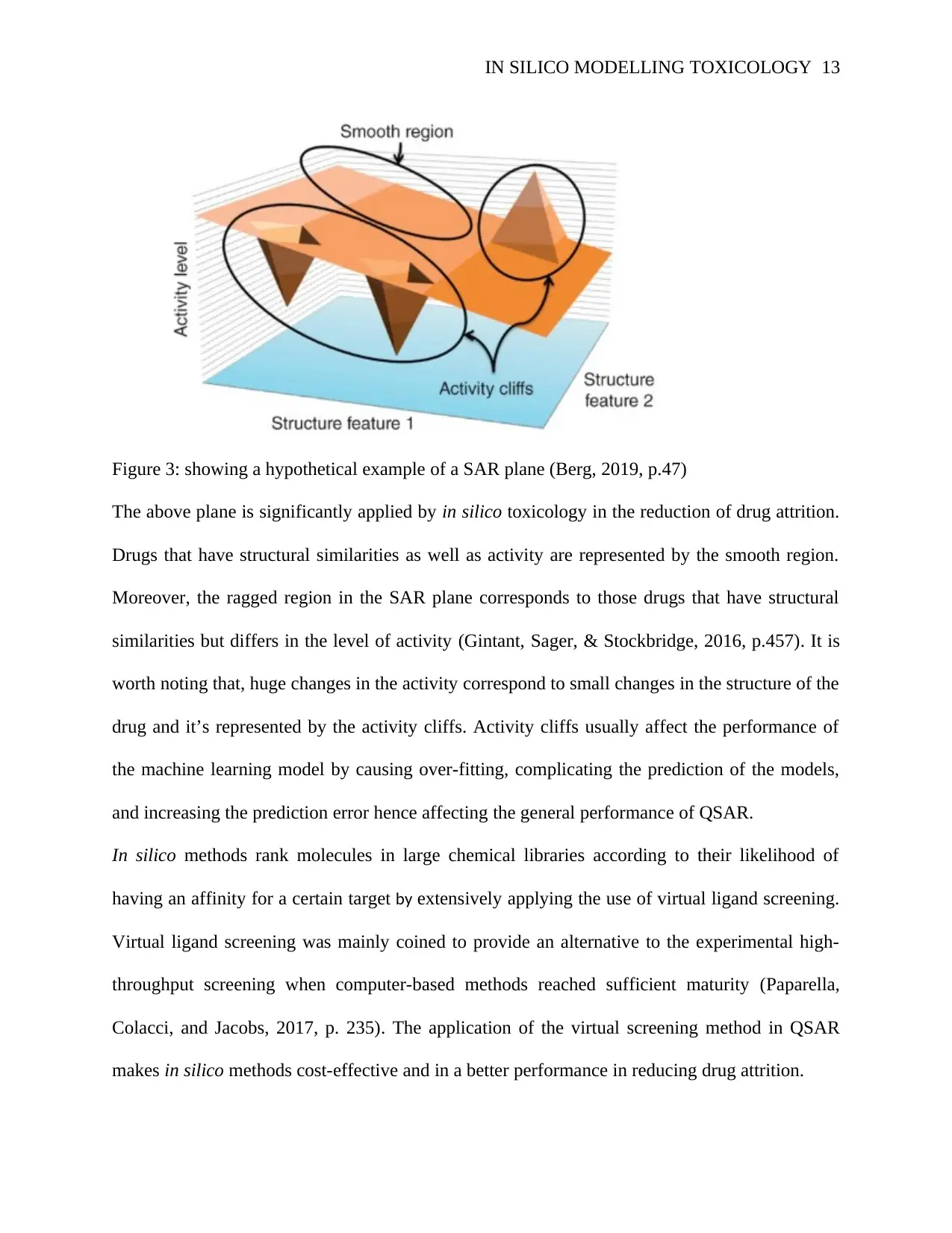
IN SILICO MODELLING TOXICOLOGY 13
Figure 3: showing a hypothetical example of a SAR plane (Berg, 2019, p.47)
The above plane is significantly applied by in silico toxicology in the reduction of drug attrition.
Drugs that have structural similarities as well as activity are represented by the smooth region.
Moreover, the ragged region in the SAR plane corresponds to those drugs that have structural
similarities but differs in the level of activity (Gintant, Sager, & Stockbridge, 2016, p.457). It is
worth noting that, huge changes in the activity correspond to small changes in the structure of the
drug and it’s represented by the activity cliffs. Activity cliffs usually affect the performance of
the machine learning model by causing over-fitting, complicating the prediction of the models,
and increasing the prediction error hence affecting the general performance of QSAR.
In silico methods rank molecules in large chemical libraries according to their likelihood of
having an affinity for a certain target by extensively applying the use of virtual ligand screening.
Virtual ligand screening was mainly coined to provide an alternative to the experimental high-
throughput screening when computer-based methods reached sufficient maturity (Paparella,
Colacci, and Jacobs, 2017, p. 235). The application of the virtual screening method in QSAR
makes in silico methods cost-effective and in a better performance in reducing drug attrition.
Figure 3: showing a hypothetical example of a SAR plane (Berg, 2019, p.47)
The above plane is significantly applied by in silico toxicology in the reduction of drug attrition.
Drugs that have structural similarities as well as activity are represented by the smooth region.
Moreover, the ragged region in the SAR plane corresponds to those drugs that have structural
similarities but differs in the level of activity (Gintant, Sager, & Stockbridge, 2016, p.457). It is
worth noting that, huge changes in the activity correspond to small changes in the structure of the
drug and it’s represented by the activity cliffs. Activity cliffs usually affect the performance of
the machine learning model by causing over-fitting, complicating the prediction of the models,
and increasing the prediction error hence affecting the general performance of QSAR.
In silico methods rank molecules in large chemical libraries according to their likelihood of
having an affinity for a certain target by extensively applying the use of virtual ligand screening.
Virtual ligand screening was mainly coined to provide an alternative to the experimental high-
throughput screening when computer-based methods reached sufficient maturity (Paparella,
Colacci, and Jacobs, 2017, p. 235). The application of the virtual screening method in QSAR
makes in silico methods cost-effective and in a better performance in reducing drug attrition.
Paraphrase This Document
Need a fresh take? Get an instant paraphrase of this document with our AI Paraphraser
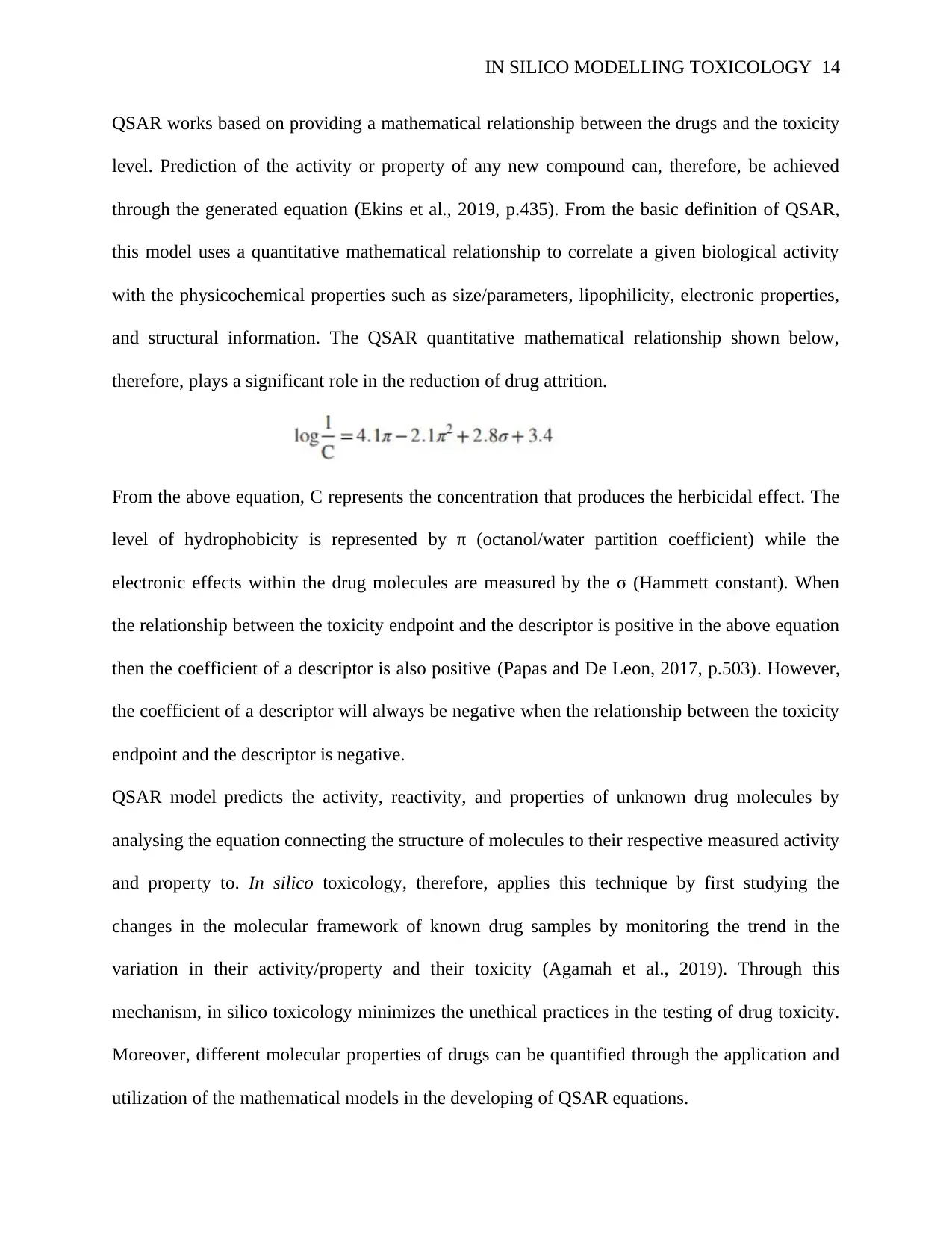
IN SILICO MODELLING TOXICOLOGY 14
QSAR works based on providing a mathematical relationship between the drugs and the toxicity
level. Prediction of the activity or property of any new compound can, therefore, be achieved
through the generated equation (Ekins et al., 2019, p.435). From the basic definition of QSAR,
this model uses a quantitative mathematical relationship to correlate a given biological activity
with the physicochemical properties such as size/parameters, lipophilicity, electronic properties,
and structural information. The QSAR quantitative mathematical relationship shown below,
therefore, plays a significant role in the reduction of drug attrition.
From the above equation, C represents the concentration that produces the herbicidal effect. The
level of hydrophobicity is represented by π (octanol/water partition coefficient) while the
electronic effects within the drug molecules are measured by the σ (Hammett constant). When
the relationship between the toxicity endpoint and the descriptor is positive in the above equation
then the coefficient of a descriptor is also positive (Papas and De Leon, 2017, p.503). However,
the coefficient of a descriptor will always be negative when the relationship between the toxicity
endpoint and the descriptor is negative.
QSAR model predicts the activity, reactivity, and properties of unknown drug molecules by
analysing the equation connecting the structure of molecules to their respective measured activity
and property to. In silico toxicology, therefore, applies this technique by first studying the
changes in the molecular framework of known drug samples by monitoring the trend in the
variation in their activity/property and their toxicity (Agamah et al., 2019). Through this
mechanism, in silico toxicology minimizes the unethical practices in the testing of drug toxicity.
Moreover, different molecular properties of drugs can be quantified through the application and
utilization of the mathematical models in the developing of QSAR equations.
QSAR works based on providing a mathematical relationship between the drugs and the toxicity
level. Prediction of the activity or property of any new compound can, therefore, be achieved
through the generated equation (Ekins et al., 2019, p.435). From the basic definition of QSAR,
this model uses a quantitative mathematical relationship to correlate a given biological activity
with the physicochemical properties such as size/parameters, lipophilicity, electronic properties,
and structural information. The QSAR quantitative mathematical relationship shown below,
therefore, plays a significant role in the reduction of drug attrition.
From the above equation, C represents the concentration that produces the herbicidal effect. The
level of hydrophobicity is represented by π (octanol/water partition coefficient) while the
electronic effects within the drug molecules are measured by the σ (Hammett constant). When
the relationship between the toxicity endpoint and the descriptor is positive in the above equation
then the coefficient of a descriptor is also positive (Papas and De Leon, 2017, p.503). However,
the coefficient of a descriptor will always be negative when the relationship between the toxicity
endpoint and the descriptor is negative.
QSAR model predicts the activity, reactivity, and properties of unknown drug molecules by
analysing the equation connecting the structure of molecules to their respective measured activity
and property to. In silico toxicology, therefore, applies this technique by first studying the
changes in the molecular framework of known drug samples by monitoring the trend in the
variation in their activity/property and their toxicity (Agamah et al., 2019). Through this
mechanism, in silico toxicology minimizes the unethical practices in the testing of drug toxicity.
Moreover, different molecular properties of drugs can be quantified through the application and
utilization of the mathematical models in the developing of QSAR equations.
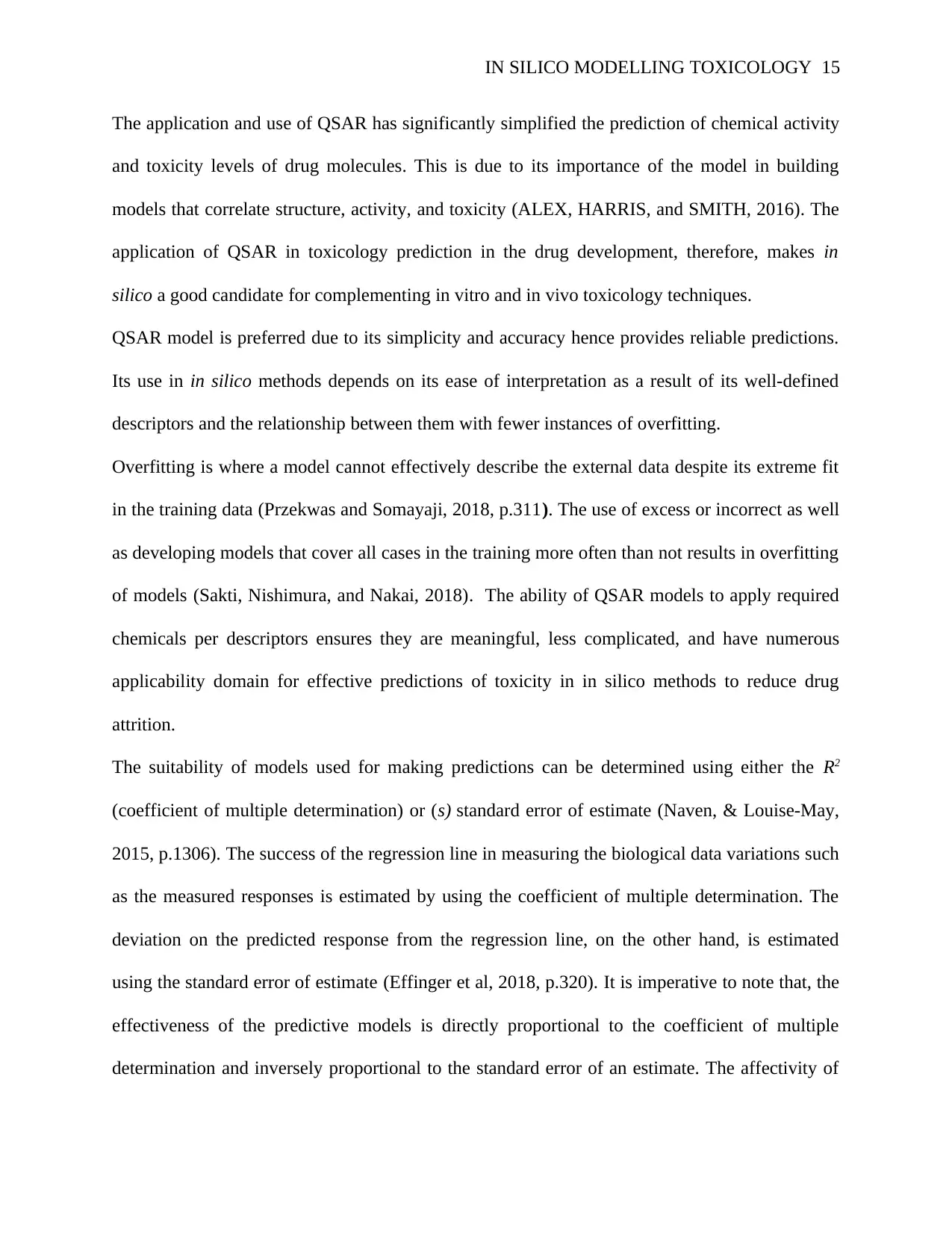
IN SILICO MODELLING TOXICOLOGY 15
The application and use of QSAR has significantly simplified the prediction of chemical activity
and toxicity levels of drug molecules. This is due to its importance of the model in building
models that correlate structure, activity, and toxicity (ALEX, HARRIS, and SMITH, 2016). The
application of QSAR in toxicology prediction in the drug development, therefore, makes in
silico a good candidate for complementing in vitro and in vivo toxicology techniques.
QSAR model is preferred due to its simplicity and accuracy hence provides reliable predictions.
Its use in in silico methods depends on its ease of interpretation as a result of its well-defined
descriptors and the relationship between them with fewer instances of overfitting.
Overfitting is where a model cannot effectively describe the external data despite its extreme fit
in the training data (Przekwas and Somayaji, 2018, p.311). The use of excess or incorrect as well
as developing models that cover all cases in the training more often than not results in overfitting
of models (Sakti, Nishimura, and Nakai, 2018). The ability of QSAR models to apply required
chemicals per descriptors ensures they are meaningful, less complicated, and have numerous
applicability domain for effective predictions of toxicity in in silico methods to reduce drug
attrition.
The suitability of models used for making predictions can be determined using either the R2
(coefficient of multiple determination) or (s) standard error of estimate (Naven, & Louise-May,
2015, p.1306). The success of the regression line in measuring the biological data variations such
as the measured responses is estimated by using the coefficient of multiple determination. The
deviation on the predicted response from the regression line, on the other hand, is estimated
using the standard error of estimate (Effinger et al, 2018, p.320). It is imperative to note that, the
effectiveness of the predictive models is directly proportional to the coefficient of multiple
determination and inversely proportional to the standard error of an estimate. The affectivity of
The application and use of QSAR has significantly simplified the prediction of chemical activity
and toxicity levels of drug molecules. This is due to its importance of the model in building
models that correlate structure, activity, and toxicity (ALEX, HARRIS, and SMITH, 2016). The
application of QSAR in toxicology prediction in the drug development, therefore, makes in
silico a good candidate for complementing in vitro and in vivo toxicology techniques.
QSAR model is preferred due to its simplicity and accuracy hence provides reliable predictions.
Its use in in silico methods depends on its ease of interpretation as a result of its well-defined
descriptors and the relationship between them with fewer instances of overfitting.
Overfitting is where a model cannot effectively describe the external data despite its extreme fit
in the training data (Przekwas and Somayaji, 2018, p.311). The use of excess or incorrect as well
as developing models that cover all cases in the training more often than not results in overfitting
of models (Sakti, Nishimura, and Nakai, 2018). The ability of QSAR models to apply required
chemicals per descriptors ensures they are meaningful, less complicated, and have numerous
applicability domain for effective predictions of toxicity in in silico methods to reduce drug
attrition.
The suitability of models used for making predictions can be determined using either the R2
(coefficient of multiple determination) or (s) standard error of estimate (Naven, & Louise-May,
2015, p.1306). The success of the regression line in measuring the biological data variations such
as the measured responses is estimated by using the coefficient of multiple determination. The
deviation on the predicted response from the regression line, on the other hand, is estimated
using the standard error of estimate (Effinger et al, 2018, p.320). It is imperative to note that, the
effectiveness of the predictive models is directly proportional to the coefficient of multiple
determination and inversely proportional to the standard error of an estimate. The affectivity of
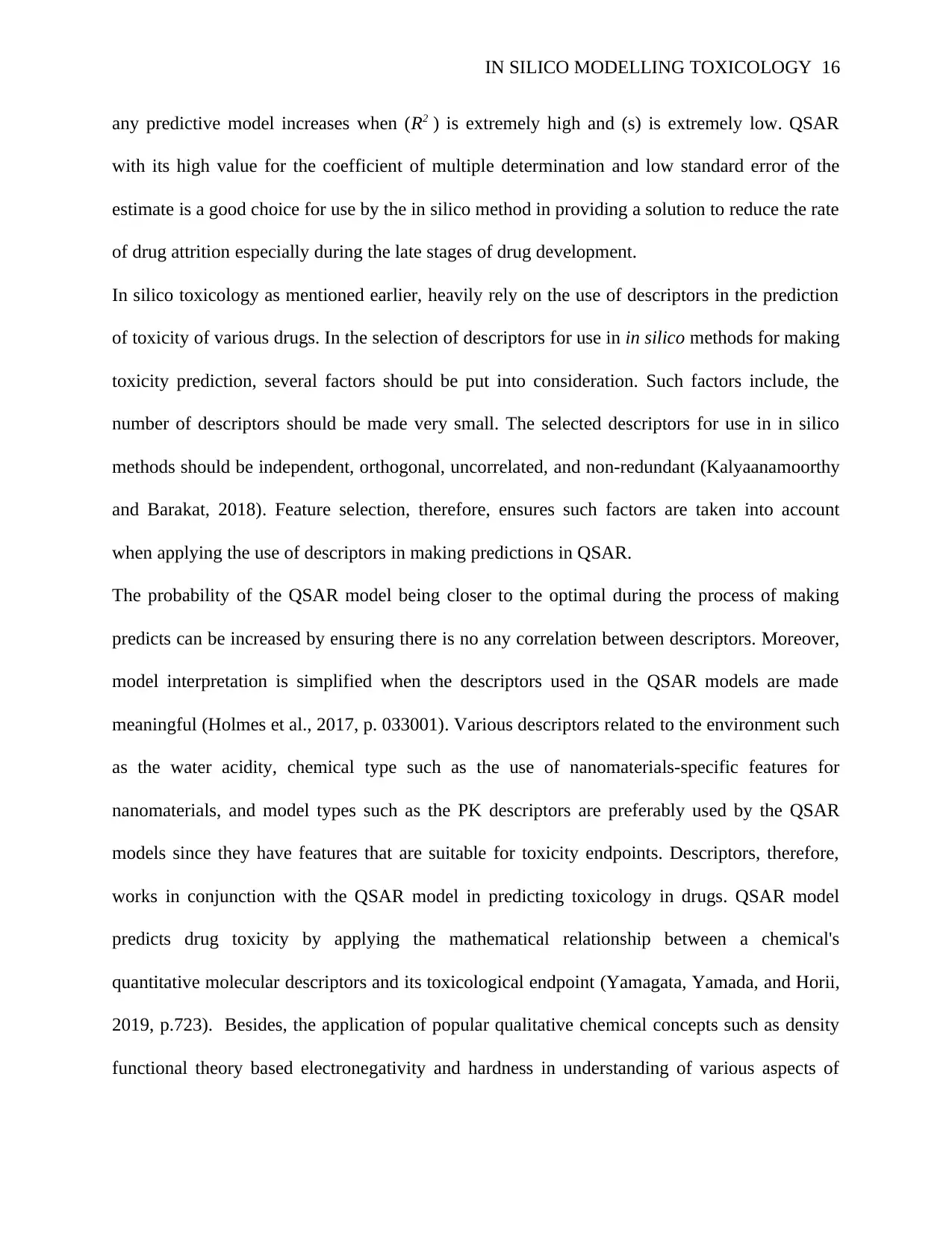
IN SILICO MODELLING TOXICOLOGY 16
any predictive model increases when (R2 ) is extremely high and (s) is extremely low. QSAR
with its high value for the coefficient of multiple determination and low standard error of the
estimate is a good choice for use by the in silico method in providing a solution to reduce the rate
of drug attrition especially during the late stages of drug development.
In silico toxicology as mentioned earlier, heavily rely on the use of descriptors in the prediction
of toxicity of various drugs. In the selection of descriptors for use in in silico methods for making
toxicity prediction, several factors should be put into consideration. Such factors include, the
number of descriptors should be made very small. The selected descriptors for use in in silico
methods should be independent, orthogonal, uncorrelated, and non-redundant (Kalyaanamoorthy
and Barakat, 2018). Feature selection, therefore, ensures such factors are taken into account
when applying the use of descriptors in making predictions in QSAR.
The probability of the QSAR model being closer to the optimal during the process of making
predicts can be increased by ensuring there is no any correlation between descriptors. Moreover,
model interpretation is simplified when the descriptors used in the QSAR models are made
meaningful (Holmes et al., 2017, p. 033001). Various descriptors related to the environment such
as the water acidity, chemical type such as the use of nanomaterials-specific features for
nanomaterials, and model types such as the PK descriptors are preferably used by the QSAR
models since they have features that are suitable for toxicity endpoints. Descriptors, therefore,
works in conjunction with the QSAR model in predicting toxicology in drugs. QSAR model
predicts drug toxicity by applying the mathematical relationship between a chemical's
quantitative molecular descriptors and its toxicological endpoint (Yamagata, Yamada, and Horii,
2019, p.723). Besides, the application of popular qualitative chemical concepts such as density
functional theory based electronegativity and hardness in understanding of various aspects of
any predictive model increases when (R2 ) is extremely high and (s) is extremely low. QSAR
with its high value for the coefficient of multiple determination and low standard error of the
estimate is a good choice for use by the in silico method in providing a solution to reduce the rate
of drug attrition especially during the late stages of drug development.
In silico toxicology as mentioned earlier, heavily rely on the use of descriptors in the prediction
of toxicity of various drugs. In the selection of descriptors for use in in silico methods for making
toxicity prediction, several factors should be put into consideration. Such factors include, the
number of descriptors should be made very small. The selected descriptors for use in in silico
methods should be independent, orthogonal, uncorrelated, and non-redundant (Kalyaanamoorthy
and Barakat, 2018). Feature selection, therefore, ensures such factors are taken into account
when applying the use of descriptors in making predictions in QSAR.
The probability of the QSAR model being closer to the optimal during the process of making
predicts can be increased by ensuring there is no any correlation between descriptors. Moreover,
model interpretation is simplified when the descriptors used in the QSAR models are made
meaningful (Holmes et al., 2017, p. 033001). Various descriptors related to the environment such
as the water acidity, chemical type such as the use of nanomaterials-specific features for
nanomaterials, and model types such as the PK descriptors are preferably used by the QSAR
models since they have features that are suitable for toxicity endpoints. Descriptors, therefore,
works in conjunction with the QSAR model in predicting toxicology in drugs. QSAR model
predicts drug toxicity by applying the mathematical relationship between a chemical's
quantitative molecular descriptors and its toxicological endpoint (Yamagata, Yamada, and Horii,
2019, p.723). Besides, the application of popular qualitative chemical concepts such as density
functional theory based electronegativity and hardness in understanding of various aspects of
Secure Best Marks with AI Grader
Need help grading? Try our AI Grader for instant feedback on your assignments.
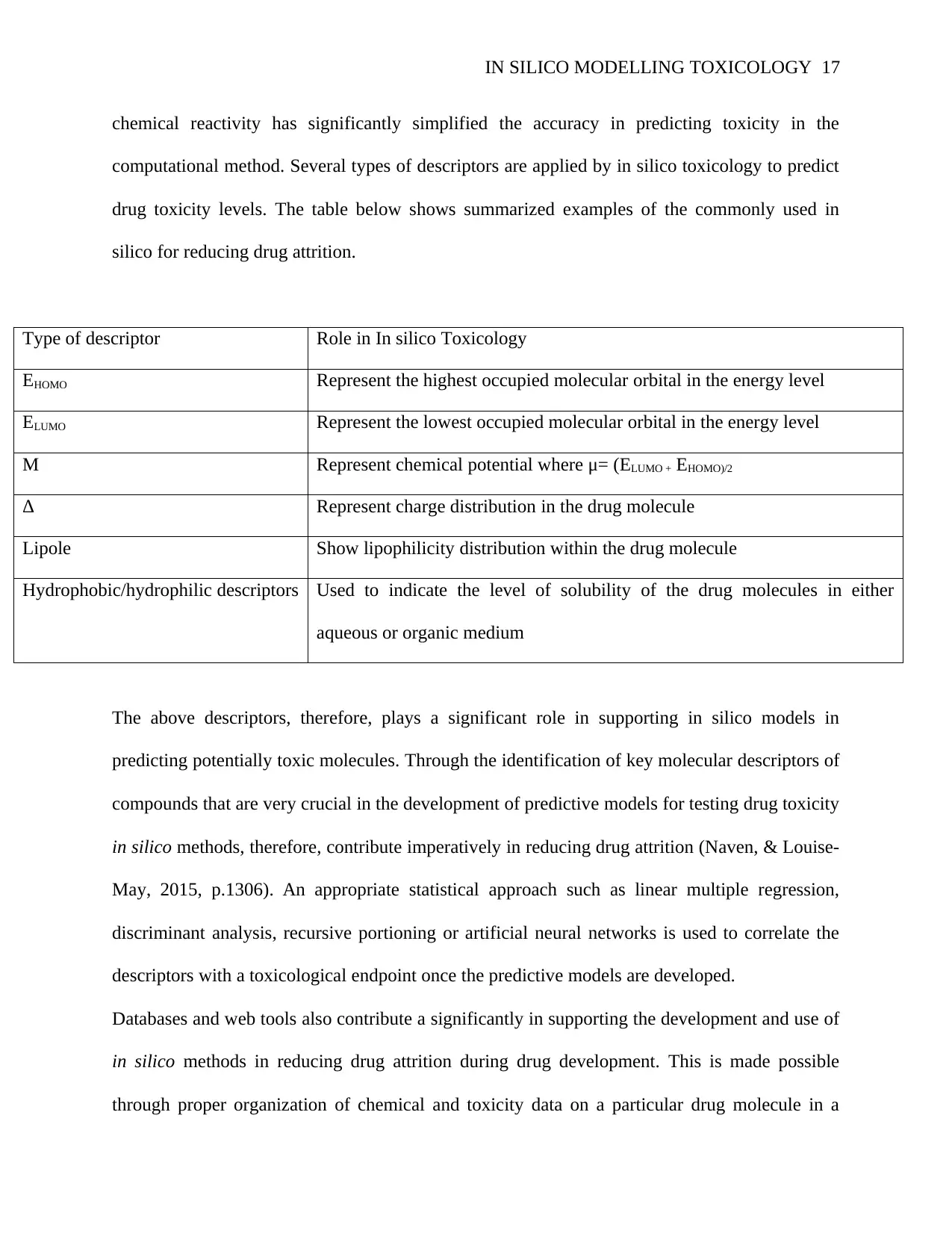
IN SILICO MODELLING TOXICOLOGY 17
chemical reactivity has significantly simplified the accuracy in predicting toxicity in the
computational method. Several types of descriptors are applied by in silico toxicology to predict
drug toxicity levels. The table below shows summarized examples of the commonly used in
silico for reducing drug attrition.
Type of descriptor Role in In silico Toxicology
EHOMO Represent the highest occupied molecular orbital in the energy level
ELUMO Represent the lowest occupied molecular orbital in the energy level
Μ Represent chemical potential where μ= (ELUMO + EHOMO)/2
Δ Represent charge distribution in the drug molecule
Lipole Show lipophilicity distribution within the drug molecule
Hydrophobic/hydrophilic descriptors Used to indicate the level of solubility of the drug molecules in either
aqueous or organic medium
The above descriptors, therefore, plays a significant role in supporting in silico models in
predicting potentially toxic molecules. Through the identification of key molecular descriptors of
compounds that are very crucial in the development of predictive models for testing drug toxicity
in silico methods, therefore, contribute imperatively in reducing drug attrition (Naven, & Louise-
May, 2015, p.1306). An appropriate statistical approach such as linear multiple regression,
discriminant analysis, recursive portioning or artificial neural networks is used to correlate the
descriptors with a toxicological endpoint once the predictive models are developed.
Databases and web tools also contribute a significantly in supporting the development and use of
in silico methods in reducing drug attrition during drug development. This is made possible
through proper organization of chemical and toxicity data on a particular drug molecule in a
chemical reactivity has significantly simplified the accuracy in predicting toxicity in the
computational method. Several types of descriptors are applied by in silico toxicology to predict
drug toxicity levels. The table below shows summarized examples of the commonly used in
silico for reducing drug attrition.
Type of descriptor Role in In silico Toxicology
EHOMO Represent the highest occupied molecular orbital in the energy level
ELUMO Represent the lowest occupied molecular orbital in the energy level
Μ Represent chemical potential where μ= (ELUMO + EHOMO)/2
Δ Represent charge distribution in the drug molecule
Lipole Show lipophilicity distribution within the drug molecule
Hydrophobic/hydrophilic descriptors Used to indicate the level of solubility of the drug molecules in either
aqueous or organic medium
The above descriptors, therefore, plays a significant role in supporting in silico models in
predicting potentially toxic molecules. Through the identification of key molecular descriptors of
compounds that are very crucial in the development of predictive models for testing drug toxicity
in silico methods, therefore, contribute imperatively in reducing drug attrition (Naven, & Louise-
May, 2015, p.1306). An appropriate statistical approach such as linear multiple regression,
discriminant analysis, recursive portioning or artificial neural networks is used to correlate the
descriptors with a toxicological endpoint once the predictive models are developed.
Databases and web tools also contribute a significantly in supporting the development and use of
in silico methods in reducing drug attrition during drug development. This is made possible
through proper organization of chemical and toxicity data on a particular drug molecule in a
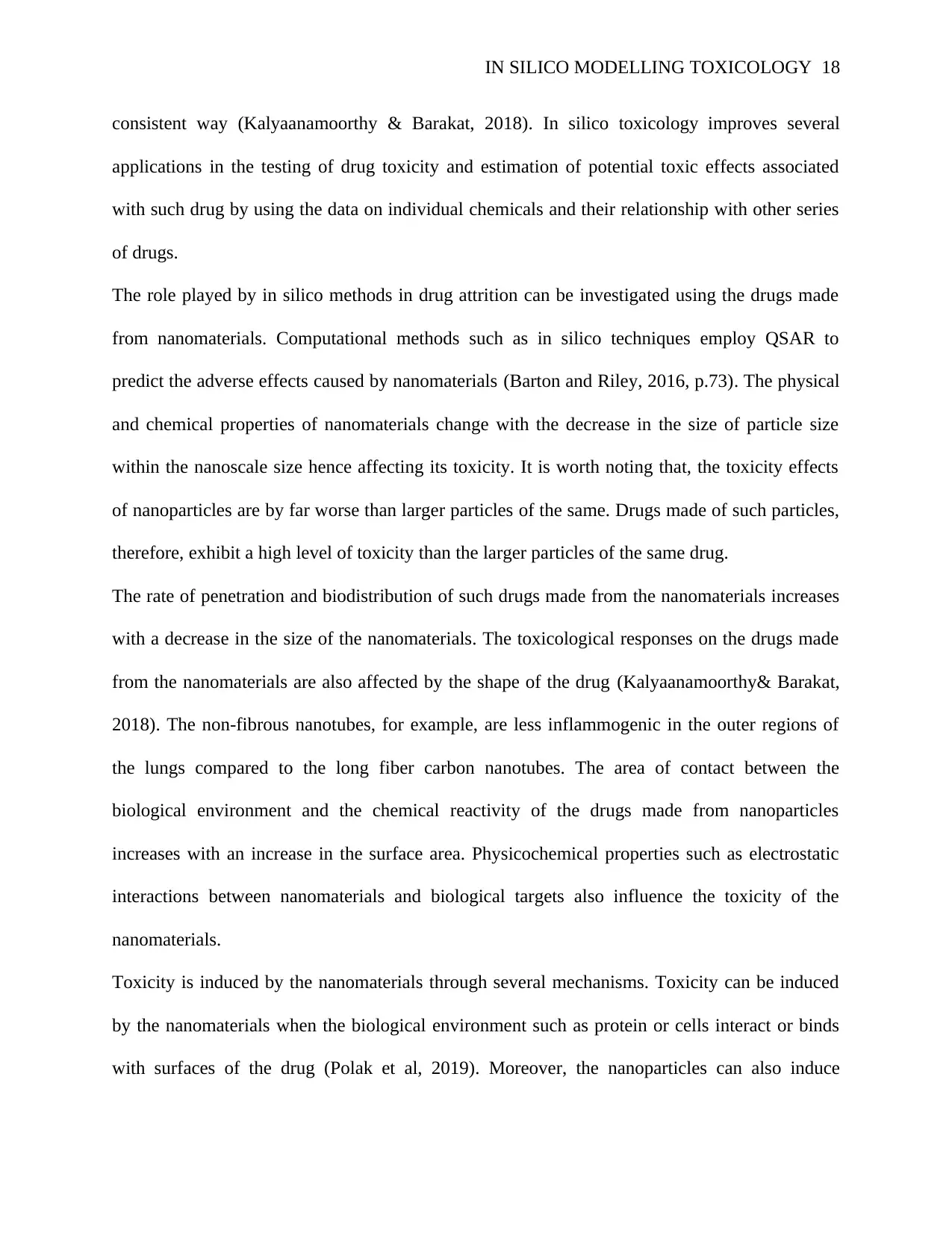
IN SILICO MODELLING TOXICOLOGY 18
consistent way (Kalyaanamoorthy & Barakat, 2018). In silico toxicology improves several
applications in the testing of drug toxicity and estimation of potential toxic effects associated
with such drug by using the data on individual chemicals and their relationship with other series
of drugs.
The role played by in silico methods in drug attrition can be investigated using the drugs made
from nanomaterials. Computational methods such as in silico techniques employ QSAR to
predict the adverse effects caused by nanomaterials (Barton and Riley, 2016, p.73). The physical
and chemical properties of nanomaterials change with the decrease in the size of particle size
within the nanoscale size hence affecting its toxicity. It is worth noting that, the toxicity effects
of nanoparticles are by far worse than larger particles of the same. Drugs made of such particles,
therefore, exhibit a high level of toxicity than the larger particles of the same drug.
The rate of penetration and biodistribution of such drugs made from the nanomaterials increases
with a decrease in the size of the nanomaterials. The toxicological responses on the drugs made
from the nanomaterials are also affected by the shape of the drug (Kalyaanamoorthy& Barakat,
2018). The non-fibrous nanotubes, for example, are less inflammogenic in the outer regions of
the lungs compared to the long fiber carbon nanotubes. The area of contact between the
biological environment and the chemical reactivity of the drugs made from nanoparticles
increases with an increase in the surface area. Physicochemical properties such as electrostatic
interactions between nanomaterials and biological targets also influence the toxicity of the
nanomaterials.
Toxicity is induced by the nanomaterials through several mechanisms. Toxicity can be induced
by the nanomaterials when the biological environment such as protein or cells interact or binds
with surfaces of the drug (Polak et al, 2019). Moreover, the nanoparticles can also induce
consistent way (Kalyaanamoorthy & Barakat, 2018). In silico toxicology improves several
applications in the testing of drug toxicity and estimation of potential toxic effects associated
with such drug by using the data on individual chemicals and their relationship with other series
of drugs.
The role played by in silico methods in drug attrition can be investigated using the drugs made
from nanomaterials. Computational methods such as in silico techniques employ QSAR to
predict the adverse effects caused by nanomaterials (Barton and Riley, 2016, p.73). The physical
and chemical properties of nanomaterials change with the decrease in the size of particle size
within the nanoscale size hence affecting its toxicity. It is worth noting that, the toxicity effects
of nanoparticles are by far worse than larger particles of the same. Drugs made of such particles,
therefore, exhibit a high level of toxicity than the larger particles of the same drug.
The rate of penetration and biodistribution of such drugs made from the nanomaterials increases
with a decrease in the size of the nanomaterials. The toxicological responses on the drugs made
from the nanomaterials are also affected by the shape of the drug (Kalyaanamoorthy& Barakat,
2018). The non-fibrous nanotubes, for example, are less inflammogenic in the outer regions of
the lungs compared to the long fiber carbon nanotubes. The area of contact between the
biological environment and the chemical reactivity of the drugs made from nanoparticles
increases with an increase in the surface area. Physicochemical properties such as electrostatic
interactions between nanomaterials and biological targets also influence the toxicity of the
nanomaterials.
Toxicity is induced by the nanomaterials through several mechanisms. Toxicity can be induced
by the nanomaterials when the biological environment such as protein or cells interact or binds
with surfaces of the drug (Polak et al, 2019). Moreover, the nanoparticles can also induce
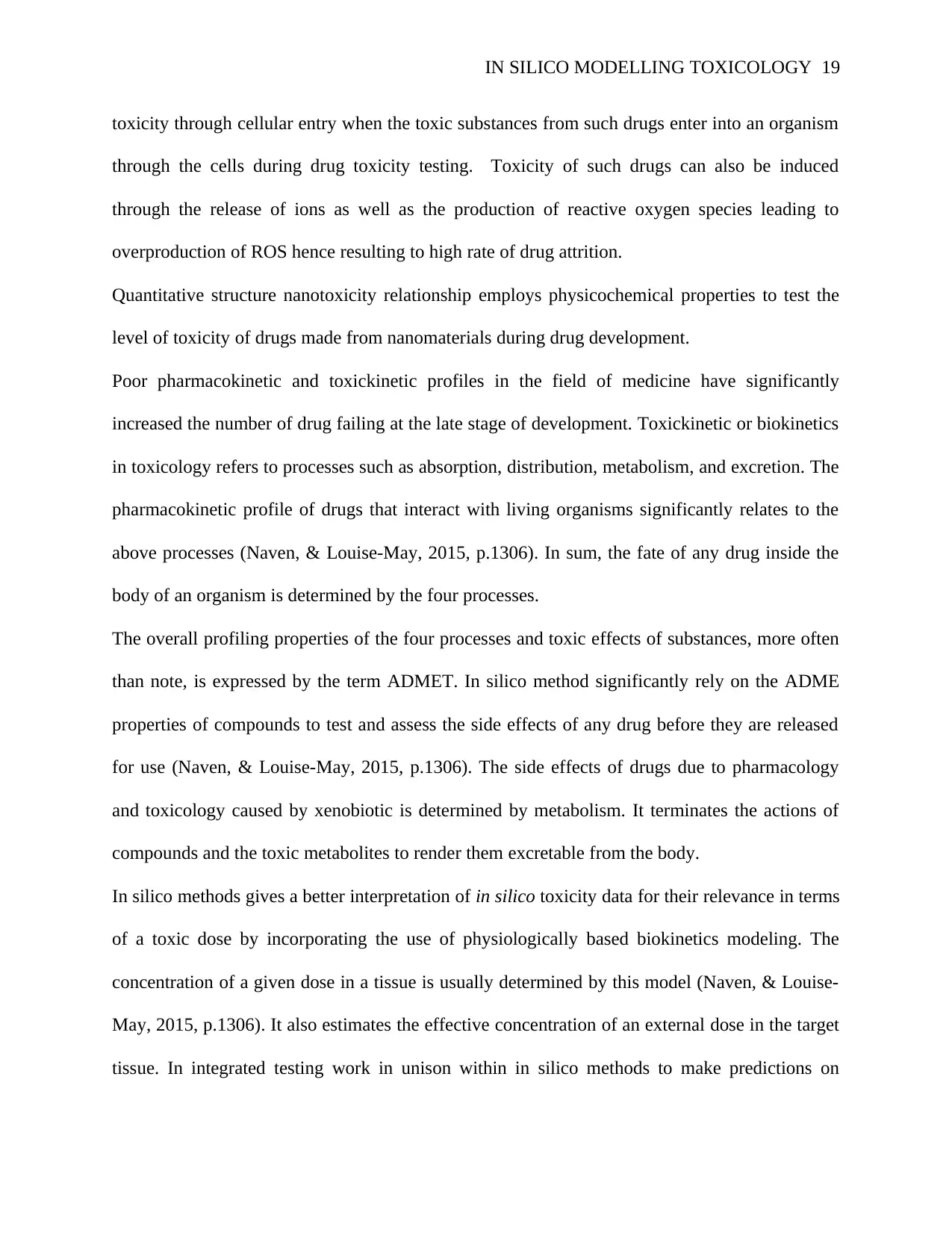
IN SILICO MODELLING TOXICOLOGY 19
toxicity through cellular entry when the toxic substances from such drugs enter into an organism
through the cells during drug toxicity testing. Toxicity of such drugs can also be induced
through the release of ions as well as the production of reactive oxygen species leading to
overproduction of ROS hence resulting to high rate of drug attrition.
Quantitative structure nanotoxicity relationship employs physicochemical properties to test the
level of toxicity of drugs made from nanomaterials during drug development.
Poor pharmacokinetic and toxickinetic profiles in the field of medicine have significantly
increased the number of drug failing at the late stage of development. Toxickinetic or biokinetics
in toxicology refers to processes such as absorption, distribution, metabolism, and excretion. The
pharmacokinetic profile of drugs that interact with living organisms significantly relates to the
above processes (Naven, & Louise-May, 2015, p.1306). In sum, the fate of any drug inside the
body of an organism is determined by the four processes.
The overall profiling properties of the four processes and toxic effects of substances, more often
than note, is expressed by the term ADMET. In silico method significantly rely on the ADME
properties of compounds to test and assess the side effects of any drug before they are released
for use (Naven, & Louise-May, 2015, p.1306). The side effects of drugs due to pharmacology
and toxicology caused by xenobiotic is determined by metabolism. It terminates the actions of
compounds and the toxic metabolites to render them excretable from the body.
In silico methods gives a better interpretation of in silico toxicity data for their relevance in terms
of a toxic dose by incorporating the use of physiologically based biokinetics modeling. The
concentration of a given dose in a tissue is usually determined by this model (Naven, & Louise-
May, 2015, p.1306). It also estimates the effective concentration of an external dose in the target
tissue. In integrated testing work in unison within in silico methods to make predictions on
toxicity through cellular entry when the toxic substances from such drugs enter into an organism
through the cells during drug toxicity testing. Toxicity of such drugs can also be induced
through the release of ions as well as the production of reactive oxygen species leading to
overproduction of ROS hence resulting to high rate of drug attrition.
Quantitative structure nanotoxicity relationship employs physicochemical properties to test the
level of toxicity of drugs made from nanomaterials during drug development.
Poor pharmacokinetic and toxickinetic profiles in the field of medicine have significantly
increased the number of drug failing at the late stage of development. Toxickinetic or biokinetics
in toxicology refers to processes such as absorption, distribution, metabolism, and excretion. The
pharmacokinetic profile of drugs that interact with living organisms significantly relates to the
above processes (Naven, & Louise-May, 2015, p.1306). In sum, the fate of any drug inside the
body of an organism is determined by the four processes.
The overall profiling properties of the four processes and toxic effects of substances, more often
than note, is expressed by the term ADMET. In silico method significantly rely on the ADME
properties of compounds to test and assess the side effects of any drug before they are released
for use (Naven, & Louise-May, 2015, p.1306). The side effects of drugs due to pharmacology
and toxicology caused by xenobiotic is determined by metabolism. It terminates the actions of
compounds and the toxic metabolites to render them excretable from the body.
In silico methods gives a better interpretation of in silico toxicity data for their relevance in terms
of a toxic dose by incorporating the use of physiologically based biokinetics modeling. The
concentration of a given dose in a tissue is usually determined by this model (Naven, & Louise-
May, 2015, p.1306). It also estimates the effective concentration of an external dose in the target
tissue. In integrated testing work in unison within in silico methods to make predictions on
Paraphrase This Document
Need a fresh take? Get an instant paraphrase of this document with our AI Paraphraser
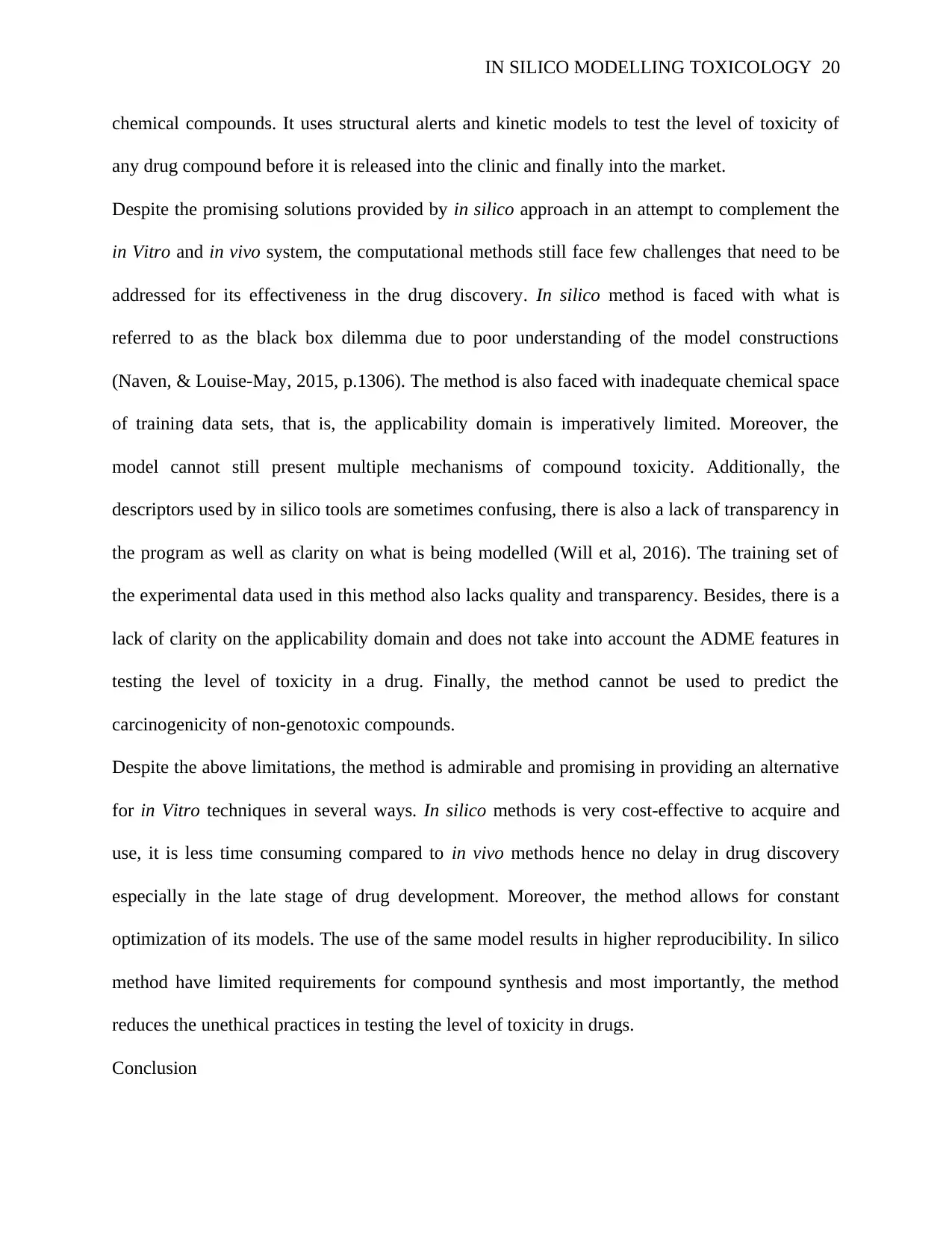
IN SILICO MODELLING TOXICOLOGY 20
chemical compounds. It uses structural alerts and kinetic models to test the level of toxicity of
any drug compound before it is released into the clinic and finally into the market.
Despite the promising solutions provided by in silico approach in an attempt to complement the
in Vitro and in vivo system, the computational methods still face few challenges that need to be
addressed for its effectiveness in the drug discovery. In silico method is faced with what is
referred to as the black box dilemma due to poor understanding of the model constructions
(Naven, & Louise-May, 2015, p.1306). The method is also faced with inadequate chemical space
of training data sets, that is, the applicability domain is imperatively limited. Moreover, the
model cannot still present multiple mechanisms of compound toxicity. Additionally, the
descriptors used by in silico tools are sometimes confusing, there is also a lack of transparency in
the program as well as clarity on what is being modelled (Will et al, 2016). The training set of
the experimental data used in this method also lacks quality and transparency. Besides, there is a
lack of clarity on the applicability domain and does not take into account the ADME features in
testing the level of toxicity in a drug. Finally, the method cannot be used to predict the
carcinogenicity of non-genotoxic compounds.
Despite the above limitations, the method is admirable and promising in providing an alternative
for in Vitro techniques in several ways. In silico methods is very cost-effective to acquire and
use, it is less time consuming compared to in vivo methods hence no delay in drug discovery
especially in the late stage of drug development. Moreover, the method allows for constant
optimization of its models. The use of the same model results in higher reproducibility. In silico
method have limited requirements for compound synthesis and most importantly, the method
reduces the unethical practices in testing the level of toxicity in drugs.
Conclusion
chemical compounds. It uses structural alerts and kinetic models to test the level of toxicity of
any drug compound before it is released into the clinic and finally into the market.
Despite the promising solutions provided by in silico approach in an attempt to complement the
in Vitro and in vivo system, the computational methods still face few challenges that need to be
addressed for its effectiveness in the drug discovery. In silico method is faced with what is
referred to as the black box dilemma due to poor understanding of the model constructions
(Naven, & Louise-May, 2015, p.1306). The method is also faced with inadequate chemical space
of training data sets, that is, the applicability domain is imperatively limited. Moreover, the
model cannot still present multiple mechanisms of compound toxicity. Additionally, the
descriptors used by in silico tools are sometimes confusing, there is also a lack of transparency in
the program as well as clarity on what is being modelled (Will et al, 2016). The training set of
the experimental data used in this method also lacks quality and transparency. Besides, there is a
lack of clarity on the applicability domain and does not take into account the ADME features in
testing the level of toxicity in a drug. Finally, the method cannot be used to predict the
carcinogenicity of non-genotoxic compounds.
Despite the above limitations, the method is admirable and promising in providing an alternative
for in Vitro techniques in several ways. In silico methods is very cost-effective to acquire and
use, it is less time consuming compared to in vivo methods hence no delay in drug discovery
especially in the late stage of drug development. Moreover, the method allows for constant
optimization of its models. The use of the same model results in higher reproducibility. In silico
method have limited requirements for compound synthesis and most importantly, the method
reduces the unethical practices in testing the level of toxicity in drugs.
Conclusion
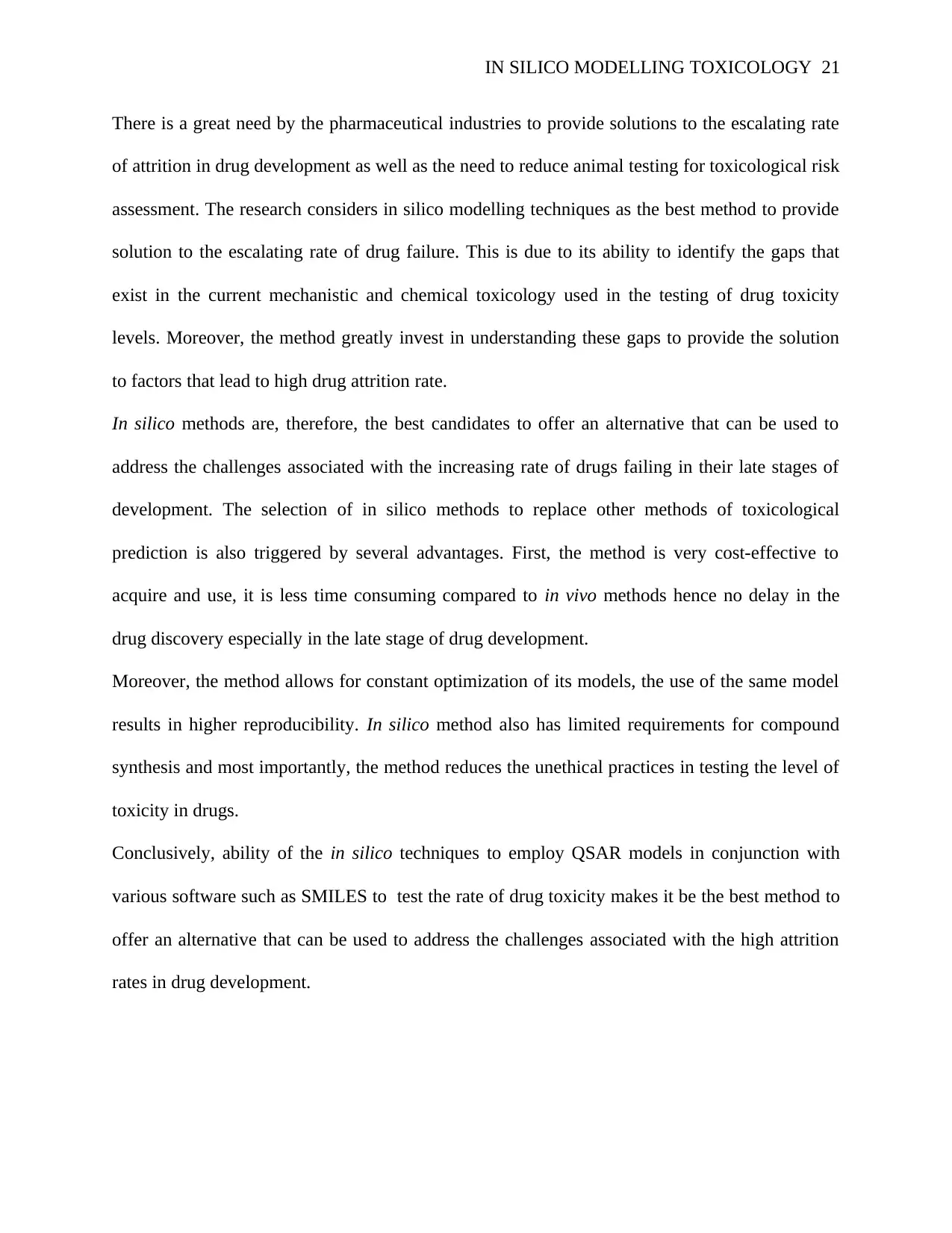
IN SILICO MODELLING TOXICOLOGY 21
There is a great need by the pharmaceutical industries to provide solutions to the escalating rate
of attrition in drug development as well as the need to reduce animal testing for toxicological risk
assessment. The research considers in silico modelling techniques as the best method to provide
solution to the escalating rate of drug failure. This is due to its ability to identify the gaps that
exist in the current mechanistic and chemical toxicology used in the testing of drug toxicity
levels. Moreover, the method greatly invest in understanding these gaps to provide the solution
to factors that lead to high drug attrition rate.
In silico methods are, therefore, the best candidates to offer an alternative that can be used to
address the challenges associated with the increasing rate of drugs failing in their late stages of
development. The selection of in silico methods to replace other methods of toxicological
prediction is also triggered by several advantages. First, the method is very cost-effective to
acquire and use, it is less time consuming compared to in vivo methods hence no delay in the
drug discovery especially in the late stage of drug development.
Moreover, the method allows for constant optimization of its models, the use of the same model
results in higher reproducibility. In silico method also has limited requirements for compound
synthesis and most importantly, the method reduces the unethical practices in testing the level of
toxicity in drugs.
Conclusively, ability of the in silico techniques to employ QSAR models in conjunction with
various software such as SMILES to test the rate of drug toxicity makes it be the best method to
offer an alternative that can be used to address the challenges associated with the high attrition
rates in drug development.
There is a great need by the pharmaceutical industries to provide solutions to the escalating rate
of attrition in drug development as well as the need to reduce animal testing for toxicological risk
assessment. The research considers in silico modelling techniques as the best method to provide
solution to the escalating rate of drug failure. This is due to its ability to identify the gaps that
exist in the current mechanistic and chemical toxicology used in the testing of drug toxicity
levels. Moreover, the method greatly invest in understanding these gaps to provide the solution
to factors that lead to high drug attrition rate.
In silico methods are, therefore, the best candidates to offer an alternative that can be used to
address the challenges associated with the increasing rate of drugs failing in their late stages of
development. The selection of in silico methods to replace other methods of toxicological
prediction is also triggered by several advantages. First, the method is very cost-effective to
acquire and use, it is less time consuming compared to in vivo methods hence no delay in the
drug discovery especially in the late stage of drug development.
Moreover, the method allows for constant optimization of its models, the use of the same model
results in higher reproducibility. In silico method also has limited requirements for compound
synthesis and most importantly, the method reduces the unethical practices in testing the level of
toxicity in drugs.
Conclusively, ability of the in silico techniques to employ QSAR models in conjunction with
various software such as SMILES to test the rate of drug toxicity makes it be the best method to
offer an alternative that can be used to address the challenges associated with the high attrition
rates in drug development.
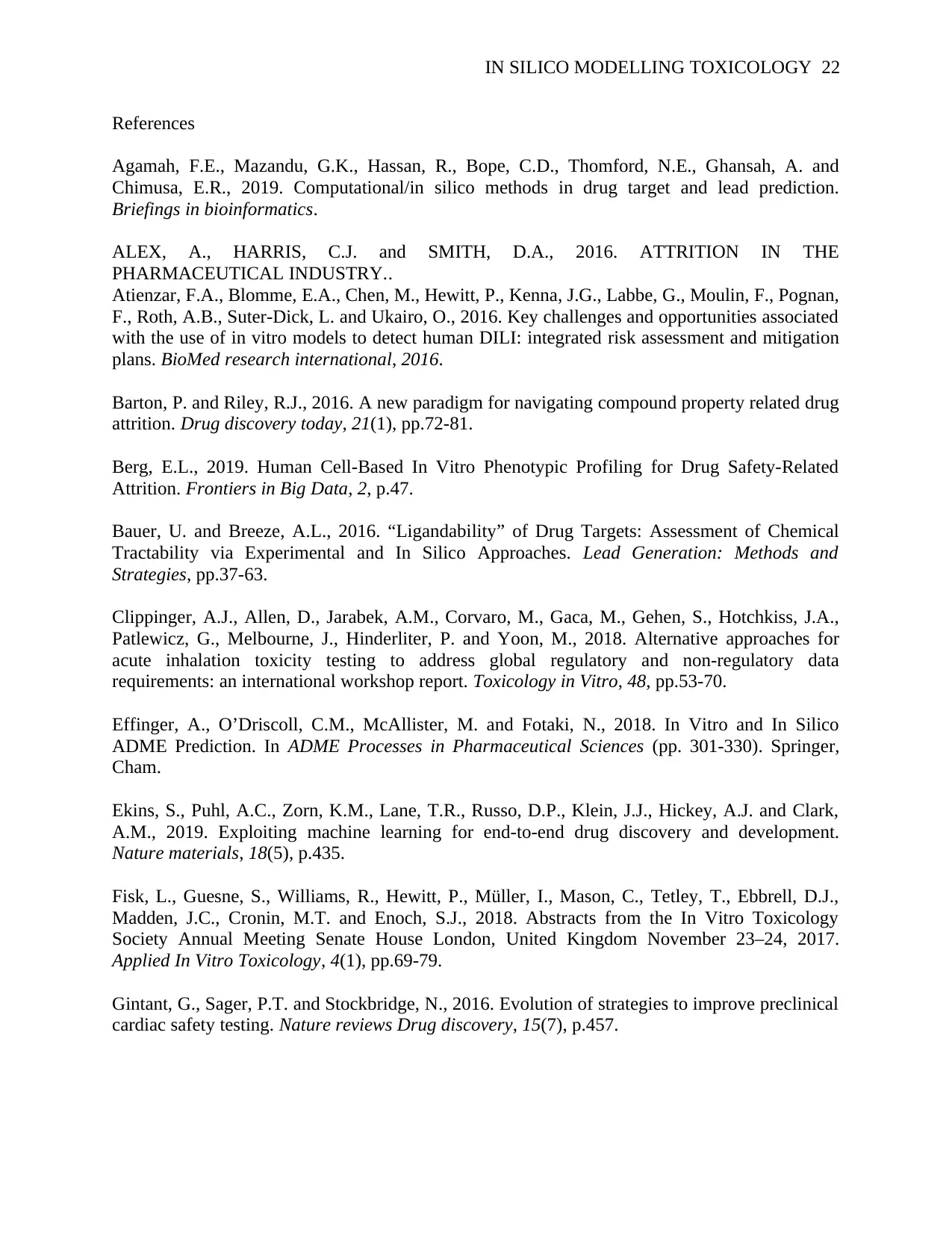
IN SILICO MODELLING TOXICOLOGY 22
References
Agamah, F.E., Mazandu, G.K., Hassan, R., Bope, C.D., Thomford, N.E., Ghansah, A. and
Chimusa, E.R., 2019. Computational/in silico methods in drug target and lead prediction.
Briefings in bioinformatics.
ALEX, A., HARRIS, C.J. and SMITH, D.A., 2016. ATTRITION IN THE
PHARMACEUTICAL INDUSTRY..
Atienzar, F.A., Blomme, E.A., Chen, M., Hewitt, P., Kenna, J.G., Labbe, G., Moulin, F., Pognan,
F., Roth, A.B., Suter-Dick, L. and Ukairo, O., 2016. Key challenges and opportunities associated
with the use of in vitro models to detect human DILI: integrated risk assessment and mitigation
plans. BioMed research international, 2016.
Barton, P. and Riley, R.J., 2016. A new paradigm for navigating compound property related drug
attrition. Drug discovery today, 21(1), pp.72-81.
Berg, E.L., 2019. Human Cell-Based In Vitro Phenotypic Profiling for Drug Safety-Related
Attrition. Frontiers in Big Data, 2, p.47.
Bauer, U. and Breeze, A.L., 2016. “Ligandability” of Drug Targets: Assessment of Chemical
Tractability via Experimental and In Silico Approaches. Lead Generation: Methods and
Strategies, pp.37-63.
Clippinger, A.J., Allen, D., Jarabek, A.M., Corvaro, M., Gaca, M., Gehen, S., Hotchkiss, J.A.,
Patlewicz, G., Melbourne, J., Hinderliter, P. and Yoon, M., 2018. Alternative approaches for
acute inhalation toxicity testing to address global regulatory and non-regulatory data
requirements: an international workshop report. Toxicology in Vitro, 48, pp.53-70.
Effinger, A., O’Driscoll, C.M., McAllister, M. and Fotaki, N., 2018. In Vitro and In Silico
ADME Prediction. In ADME Processes in Pharmaceutical Sciences (pp. 301-330). Springer,
Cham.
Ekins, S., Puhl, A.C., Zorn, K.M., Lane, T.R., Russo, D.P., Klein, J.J., Hickey, A.J. and Clark,
A.M., 2019. Exploiting machine learning for end-to-end drug discovery and development.
Nature materials, 18(5), p.435.
Fisk, L., Guesne, S., Williams, R., Hewitt, P., Müller, I., Mason, C., Tetley, T., Ebbrell, D.J.,
Madden, J.C., Cronin, M.T. and Enoch, S.J., 2018. Abstracts from the In Vitro Toxicology
Society Annual Meeting Senate House London, United Kingdom November 23–24, 2017.
Applied In Vitro Toxicology, 4(1), pp.69-79.
Gintant, G., Sager, P.T. and Stockbridge, N., 2016. Evolution of strategies to improve preclinical
cardiac safety testing. Nature reviews Drug discovery, 15(7), p.457.
References
Agamah, F.E., Mazandu, G.K., Hassan, R., Bope, C.D., Thomford, N.E., Ghansah, A. and
Chimusa, E.R., 2019. Computational/in silico methods in drug target and lead prediction.
Briefings in bioinformatics.
ALEX, A., HARRIS, C.J. and SMITH, D.A., 2016. ATTRITION IN THE
PHARMACEUTICAL INDUSTRY..
Atienzar, F.A., Blomme, E.A., Chen, M., Hewitt, P., Kenna, J.G., Labbe, G., Moulin, F., Pognan,
F., Roth, A.B., Suter-Dick, L. and Ukairo, O., 2016. Key challenges and opportunities associated
with the use of in vitro models to detect human DILI: integrated risk assessment and mitigation
plans. BioMed research international, 2016.
Barton, P. and Riley, R.J., 2016. A new paradigm for navigating compound property related drug
attrition. Drug discovery today, 21(1), pp.72-81.
Berg, E.L., 2019. Human Cell-Based In Vitro Phenotypic Profiling for Drug Safety-Related
Attrition. Frontiers in Big Data, 2, p.47.
Bauer, U. and Breeze, A.L., 2016. “Ligandability” of Drug Targets: Assessment of Chemical
Tractability via Experimental and In Silico Approaches. Lead Generation: Methods and
Strategies, pp.37-63.
Clippinger, A.J., Allen, D., Jarabek, A.M., Corvaro, M., Gaca, M., Gehen, S., Hotchkiss, J.A.,
Patlewicz, G., Melbourne, J., Hinderliter, P. and Yoon, M., 2018. Alternative approaches for
acute inhalation toxicity testing to address global regulatory and non-regulatory data
requirements: an international workshop report. Toxicology in Vitro, 48, pp.53-70.
Effinger, A., O’Driscoll, C.M., McAllister, M. and Fotaki, N., 2018. In Vitro and In Silico
ADME Prediction. In ADME Processes in Pharmaceutical Sciences (pp. 301-330). Springer,
Cham.
Ekins, S., Puhl, A.C., Zorn, K.M., Lane, T.R., Russo, D.P., Klein, J.J., Hickey, A.J. and Clark,
A.M., 2019. Exploiting machine learning for end-to-end drug discovery and development.
Nature materials, 18(5), p.435.
Fisk, L., Guesne, S., Williams, R., Hewitt, P., Müller, I., Mason, C., Tetley, T., Ebbrell, D.J.,
Madden, J.C., Cronin, M.T. and Enoch, S.J., 2018. Abstracts from the In Vitro Toxicology
Society Annual Meeting Senate House London, United Kingdom November 23–24, 2017.
Applied In Vitro Toxicology, 4(1), pp.69-79.
Gintant, G., Sager, P.T. and Stockbridge, N., 2016. Evolution of strategies to improve preclinical
cardiac safety testing. Nature reviews Drug discovery, 15(7), p.457.
Secure Best Marks with AI Grader
Need help grading? Try our AI Grader for instant feedback on your assignments.
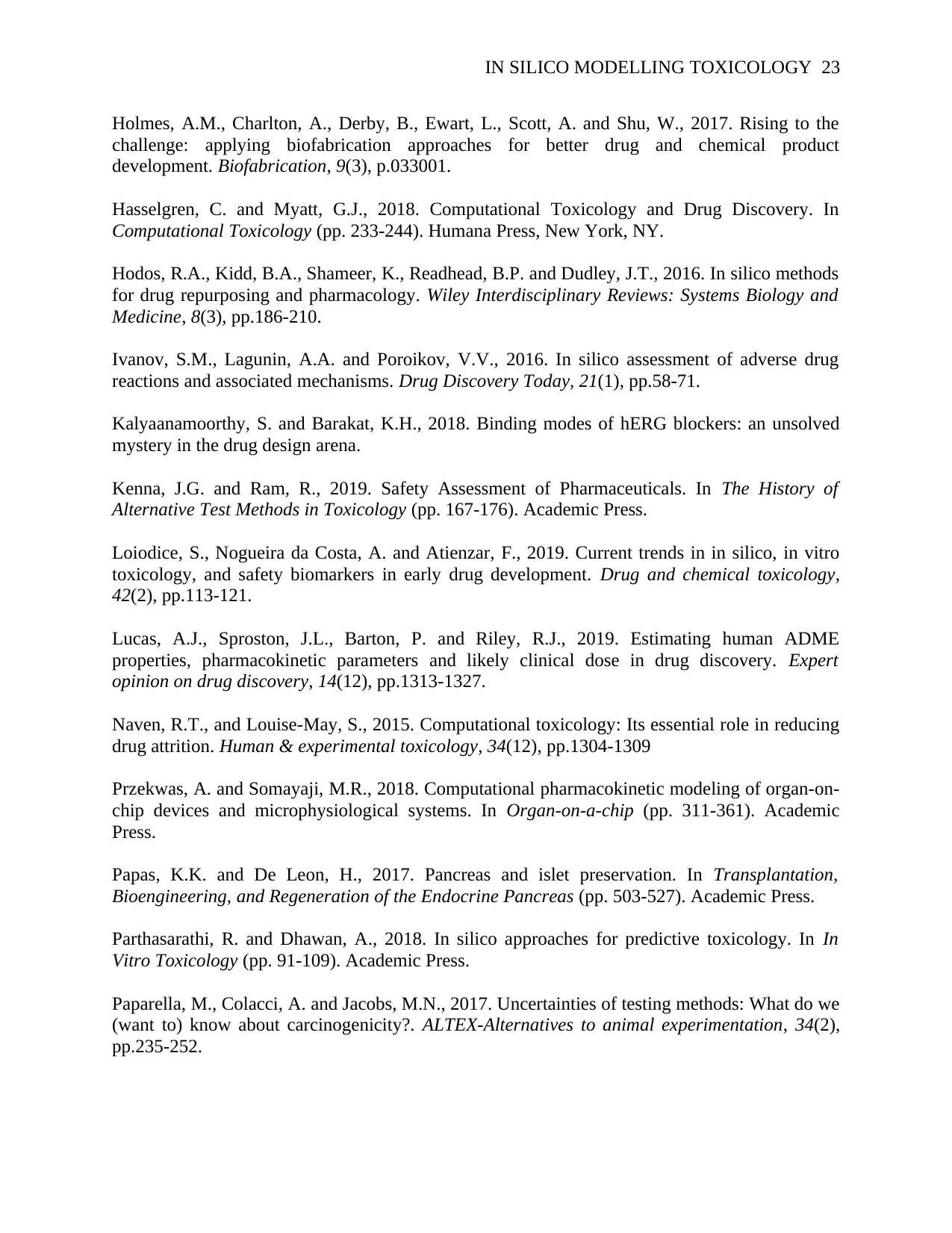
IN SILICO MODELLING TOXICOLOGY 23
Holmes, A.M., Charlton, A., Derby, B., Ewart, L., Scott, A. and Shu, W., 2017. Rising to the
challenge: applying biofabrication approaches for better drug and chemical product
development. Biofabrication, 9(3), p.033001.
Hasselgren, C. and Myatt, G.J., 2018. Computational Toxicology and Drug Discovery. In
Computational Toxicology (pp. 233-244). Humana Press, New York, NY.
Hodos, R.A., Kidd, B.A., Shameer, K., Readhead, B.P. and Dudley, J.T., 2016. In silico methods
for drug repurposing and pharmacology. Wiley Interdisciplinary Reviews: Systems Biology and
Medicine, 8(3), pp.186-210.
Ivanov, S.M., Lagunin, A.A. and Poroikov, V.V., 2016. In silico assessment of adverse drug
reactions and associated mechanisms. Drug Discovery Today, 21(1), pp.58-71.
Kalyaanamoorthy, S. and Barakat, K.H., 2018. Binding modes of hERG blockers: an unsolved
mystery in the drug design arena.
Kenna, J.G. and Ram, R., 2019. Safety Assessment of Pharmaceuticals. In The History of
Alternative Test Methods in Toxicology (pp. 167-176). Academic Press.
Loiodice, S., Nogueira da Costa, A. and Atienzar, F., 2019. Current trends in in silico, in vitro
toxicology, and safety biomarkers in early drug development. Drug and chemical toxicology,
42(2), pp.113-121.
Lucas, A.J., Sproston, J.L., Barton, P. and Riley, R.J., 2019. Estimating human ADME
properties, pharmacokinetic parameters and likely clinical dose in drug discovery. Expert
opinion on drug discovery, 14(12), pp.1313-1327.
Naven, R.T., and Louise-May, S., 2015. Computational toxicology: Its essential role in reducing
drug attrition. Human & experimental toxicology, 34(12), pp.1304-1309
Przekwas, A. and Somayaji, M.R., 2018. Computational pharmacokinetic modeling of organ-on-
chip devices and microphysiological systems. In Organ-on-a-chip (pp. 311-361). Academic
Press.
Papas, K.K. and De Leon, H., 2017. Pancreas and islet preservation. In Transplantation,
Bioengineering, and Regeneration of the Endocrine Pancreas (pp. 503-527). Academic Press.
Parthasarathi, R. and Dhawan, A., 2018. In silico approaches for predictive toxicology. In In
Vitro Toxicology (pp. 91-109). Academic Press.
Paparella, M., Colacci, A. and Jacobs, M.N., 2017. Uncertainties of testing methods: What do we
(want to) know about carcinogenicity?. ALTEX-Alternatives to animal experimentation, 34(2),
pp.235-252.
Holmes, A.M., Charlton, A., Derby, B., Ewart, L., Scott, A. and Shu, W., 2017. Rising to the
challenge: applying biofabrication approaches for better drug and chemical product
development. Biofabrication, 9(3), p.033001.
Hasselgren, C. and Myatt, G.J., 2018. Computational Toxicology and Drug Discovery. In
Computational Toxicology (pp. 233-244). Humana Press, New York, NY.
Hodos, R.A., Kidd, B.A., Shameer, K., Readhead, B.P. and Dudley, J.T., 2016. In silico methods
for drug repurposing and pharmacology. Wiley Interdisciplinary Reviews: Systems Biology and
Medicine, 8(3), pp.186-210.
Ivanov, S.M., Lagunin, A.A. and Poroikov, V.V., 2016. In silico assessment of adverse drug
reactions and associated mechanisms. Drug Discovery Today, 21(1), pp.58-71.
Kalyaanamoorthy, S. and Barakat, K.H., 2018. Binding modes of hERG blockers: an unsolved
mystery in the drug design arena.
Kenna, J.G. and Ram, R., 2019. Safety Assessment of Pharmaceuticals. In The History of
Alternative Test Methods in Toxicology (pp. 167-176). Academic Press.
Loiodice, S., Nogueira da Costa, A. and Atienzar, F., 2019. Current trends in in silico, in vitro
toxicology, and safety biomarkers in early drug development. Drug and chemical toxicology,
42(2), pp.113-121.
Lucas, A.J., Sproston, J.L., Barton, P. and Riley, R.J., 2019. Estimating human ADME
properties, pharmacokinetic parameters and likely clinical dose in drug discovery. Expert
opinion on drug discovery, 14(12), pp.1313-1327.
Naven, R.T., and Louise-May, S., 2015. Computational toxicology: Its essential role in reducing
drug attrition. Human & experimental toxicology, 34(12), pp.1304-1309
Przekwas, A. and Somayaji, M.R., 2018. Computational pharmacokinetic modeling of organ-on-
chip devices and microphysiological systems. In Organ-on-a-chip (pp. 311-361). Academic
Press.
Papas, K.K. and De Leon, H., 2017. Pancreas and islet preservation. In Transplantation,
Bioengineering, and Regeneration of the Endocrine Pancreas (pp. 503-527). Academic Press.
Parthasarathi, R. and Dhawan, A., 2018. In silico approaches for predictive toxicology. In In
Vitro Toxicology (pp. 91-109). Academic Press.
Paparella, M., Colacci, A. and Jacobs, M.N., 2017. Uncertainties of testing methods: What do we
(want to) know about carcinogenicity?. ALTEX-Alternatives to animal experimentation, 34(2),
pp.235-252.
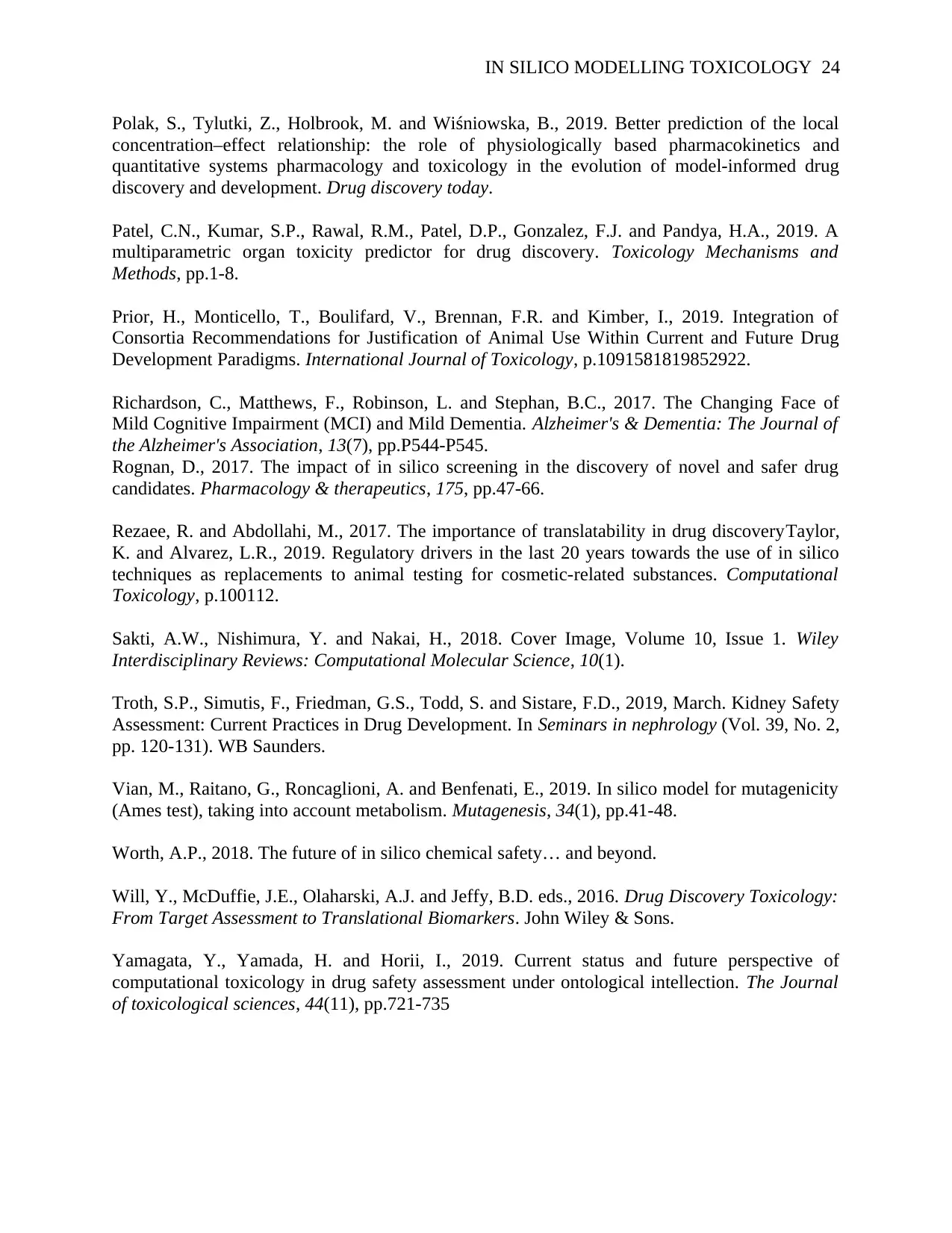
IN SILICO MODELLING TOXICOLOGY 24
Polak, S., Tylutki, Z., Holbrook, M. and Wiśniowska, B., 2019. Better prediction of the local
concentration–effect relationship: the role of physiologically based pharmacokinetics and
quantitative systems pharmacology and toxicology in the evolution of model-informed drug
discovery and development. Drug discovery today.
Patel, C.N., Kumar, S.P., Rawal, R.M., Patel, D.P., Gonzalez, F.J. and Pandya, H.A., 2019. A
multiparametric organ toxicity predictor for drug discovery. Toxicology Mechanisms and
Methods, pp.1-8.
Prior, H., Monticello, T., Boulifard, V., Brennan, F.R. and Kimber, I., 2019. Integration of
Consortia Recommendations for Justification of Animal Use Within Current and Future Drug
Development Paradigms. International Journal of Toxicology, p.1091581819852922.
Richardson, C., Matthews, F., Robinson, L. and Stephan, B.C., 2017. The Changing Face of
Mild Cognitive Impairment (MCI) and Mild Dementia. Alzheimer's & Dementia: The Journal of
the Alzheimer's Association, 13(7), pp.P544-P545.
Rognan, D., 2017. The impact of in silico screening in the discovery of novel and safer drug
candidates. Pharmacology & therapeutics, 175, pp.47-66.
Rezaee, R. and Abdollahi, M., 2017. The importance of translatability in drug discoveryTaylor,
K. and Alvarez, L.R., 2019. Regulatory drivers in the last 20 years towards the use of in silico
techniques as replacements to animal testing for cosmetic-related substances. Computational
Toxicology, p.100112.
Sakti, A.W., Nishimura, Y. and Nakai, H., 2018. Cover Image, Volume 10, Issue 1. Wiley
Interdisciplinary Reviews: Computational Molecular Science, 10(1).
Troth, S.P., Simutis, F., Friedman, G.S., Todd, S. and Sistare, F.D., 2019, March. Kidney Safety
Assessment: Current Practices in Drug Development. In Seminars in nephrology (Vol. 39, No. 2,
pp. 120-131). WB Saunders.
Vian, M., Raitano, G., Roncaglioni, A. and Benfenati, E., 2019. In silico model for mutagenicity
(Ames test), taking into account metabolism. Mutagenesis, 34(1), pp.41-48.
Worth, A.P., 2018. The future of in silico chemical safety… and beyond.
Will, Y., McDuffie, J.E., Olaharski, A.J. and Jeffy, B.D. eds., 2016. Drug Discovery Toxicology:
From Target Assessment to Translational Biomarkers. John Wiley & Sons.
Yamagata, Y., Yamada, H. and Horii, I., 2019. Current status and future perspective of
computational toxicology in drug safety assessment under ontological intellection. The Journal
of toxicological sciences, 44(11), pp.721-735
Polak, S., Tylutki, Z., Holbrook, M. and Wiśniowska, B., 2019. Better prediction of the local
concentration–effect relationship: the role of physiologically based pharmacokinetics and
quantitative systems pharmacology and toxicology in the evolution of model-informed drug
discovery and development. Drug discovery today.
Patel, C.N., Kumar, S.P., Rawal, R.M., Patel, D.P., Gonzalez, F.J. and Pandya, H.A., 2019. A
multiparametric organ toxicity predictor for drug discovery. Toxicology Mechanisms and
Methods, pp.1-8.
Prior, H., Monticello, T., Boulifard, V., Brennan, F.R. and Kimber, I., 2019. Integration of
Consortia Recommendations for Justification of Animal Use Within Current and Future Drug
Development Paradigms. International Journal of Toxicology, p.1091581819852922.
Richardson, C., Matthews, F., Robinson, L. and Stephan, B.C., 2017. The Changing Face of
Mild Cognitive Impairment (MCI) and Mild Dementia. Alzheimer's & Dementia: The Journal of
the Alzheimer's Association, 13(7), pp.P544-P545.
Rognan, D., 2017. The impact of in silico screening in the discovery of novel and safer drug
candidates. Pharmacology & therapeutics, 175, pp.47-66.
Rezaee, R. and Abdollahi, M., 2017. The importance of translatability in drug discoveryTaylor,
K. and Alvarez, L.R., 2019. Regulatory drivers in the last 20 years towards the use of in silico
techniques as replacements to animal testing for cosmetic-related substances. Computational
Toxicology, p.100112.
Sakti, A.W., Nishimura, Y. and Nakai, H., 2018. Cover Image, Volume 10, Issue 1. Wiley
Interdisciplinary Reviews: Computational Molecular Science, 10(1).
Troth, S.P., Simutis, F., Friedman, G.S., Todd, S. and Sistare, F.D., 2019, March. Kidney Safety
Assessment: Current Practices in Drug Development. In Seminars in nephrology (Vol. 39, No. 2,
pp. 120-131). WB Saunders.
Vian, M., Raitano, G., Roncaglioni, A. and Benfenati, E., 2019. In silico model for mutagenicity
(Ames test), taking into account metabolism. Mutagenesis, 34(1), pp.41-48.
Worth, A.P., 2018. The future of in silico chemical safety… and beyond.
Will, Y., McDuffie, J.E., Olaharski, A.J. and Jeffy, B.D. eds., 2016. Drug Discovery Toxicology:
From Target Assessment to Translational Biomarkers. John Wiley & Sons.
Yamagata, Y., Yamada, H. and Horii, I., 2019. Current status and future perspective of
computational toxicology in drug safety assessment under ontological intellection. The Journal
of toxicological sciences, 44(11), pp.721-735
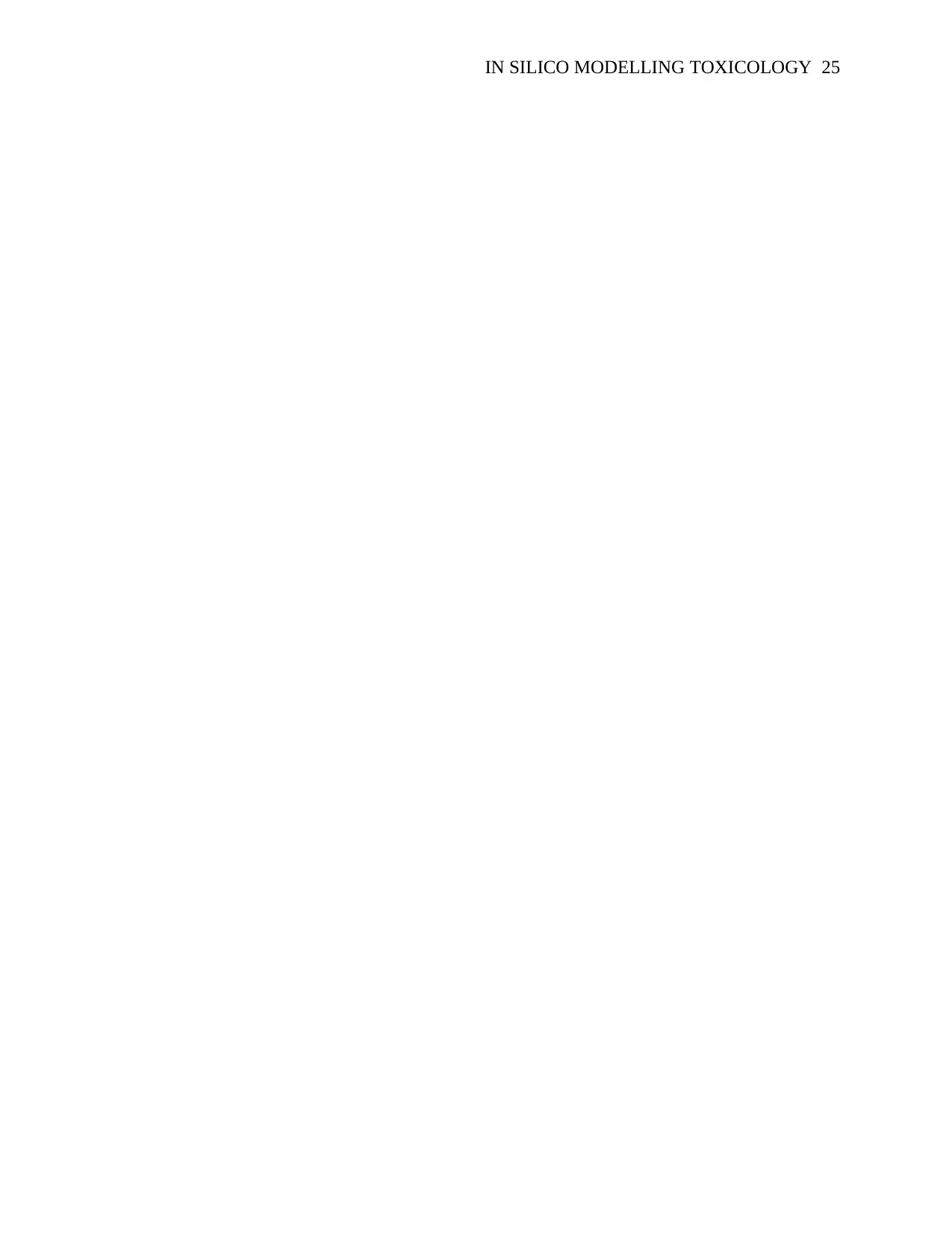
IN SILICO MODELLING TOXICOLOGY 25
1 out of 25
![[object Object]](/_next/image/?url=%2F_next%2Fstatic%2Fmedia%2Flogo.6d15ce61.png&w=640&q=75)
Your All-in-One AI-Powered Toolkit for Academic Success.
+13062052269
info@desklib.com
Available 24*7 on WhatsApp / Email
Unlock your academic potential
© 2024 | Zucol Services PVT LTD | All rights reserved.