Individual Project on Clock House
VerifiedAdded on 2021/01/02
|9
|1440
|423
AI Summary
Contribute Materials
Your contribution can guide someone’s learning journey. Share your
documents today.
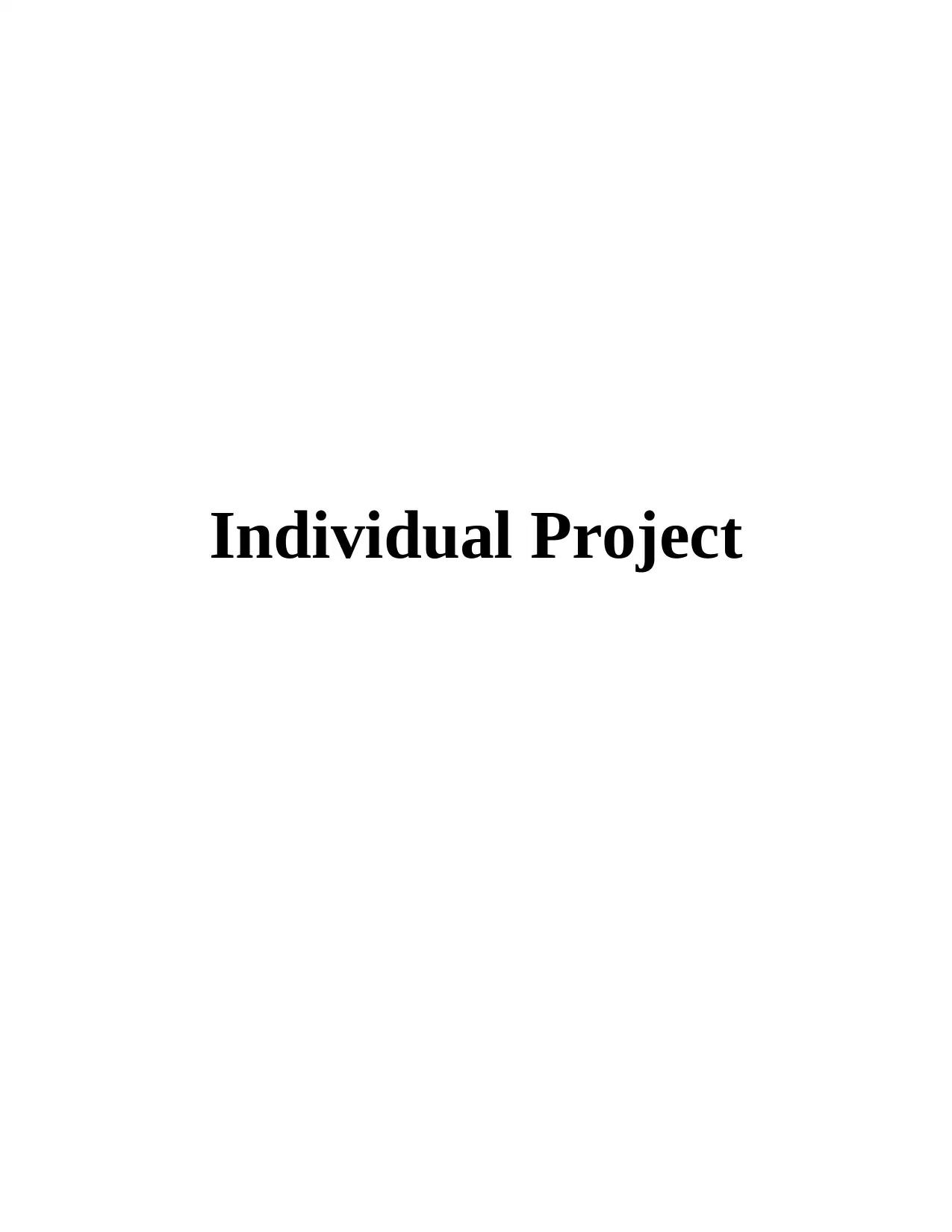
Individual Project
Secure Best Marks with AI Grader
Need help grading? Try our AI Grader for instant feedback on your assignments.
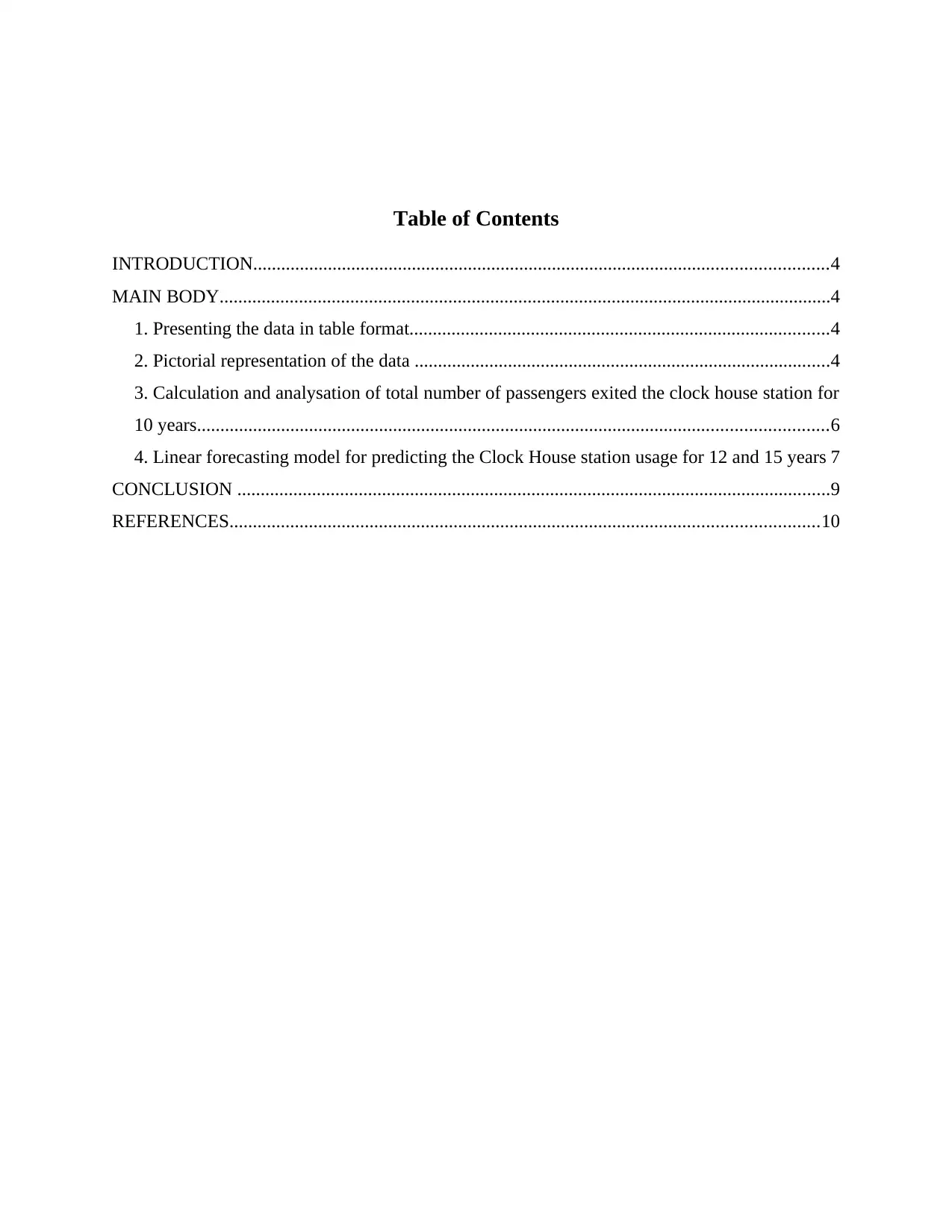
Table of Contents
INTRODUCTION...........................................................................................................................4
MAIN BODY...................................................................................................................................4
1. Presenting the data in table format..........................................................................................4
2. Pictorial representation of the data .........................................................................................4
3. Calculation and analysation of total number of passengers exited the clock house station for
10 years.......................................................................................................................................6
4. Linear forecasting model for predicting the Clock House station usage for 12 and 15 years 7
CONCLUSION ...............................................................................................................................9
REFERENCES..............................................................................................................................10
INTRODUCTION...........................................................................................................................4
MAIN BODY...................................................................................................................................4
1. Presenting the data in table format..........................................................................................4
2. Pictorial representation of the data .........................................................................................4
3. Calculation and analysation of total number of passengers exited the clock house station for
10 years.......................................................................................................................................6
4. Linear forecasting model for predicting the Clock House station usage for 12 and 15 years 7
CONCLUSION ...............................................................................................................................9
REFERENCES..............................................................................................................................10
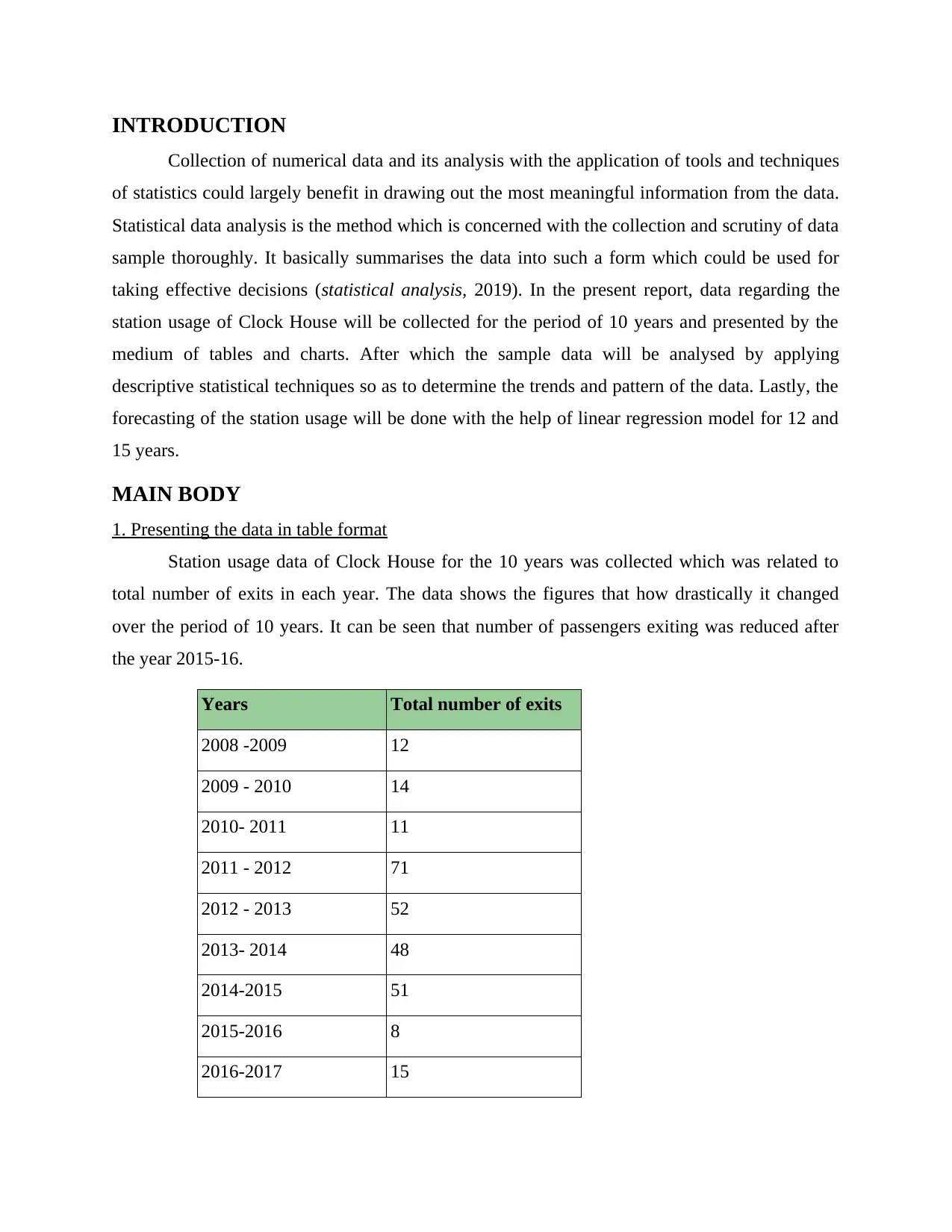
INTRODUCTION
Collection of numerical data and its analysis with the application of tools and techniques
of statistics could largely benefit in drawing out the most meaningful information from the data.
Statistical data analysis is the method which is concerned with the collection and scrutiny of data
sample thoroughly. It basically summarises the data into such a form which could be used for
taking effective decisions (statistical analysis, 2019). In the present report, data regarding the
station usage of Clock House will be collected for the period of 10 years and presented by the
medium of tables and charts. After which the sample data will be analysed by applying
descriptive statistical techniques so as to determine the trends and pattern of the data. Lastly, the
forecasting of the station usage will be done with the help of linear regression model for 12 and
15 years.
MAIN BODY
1. Presenting the data in table format
Station usage data of Clock House for the 10 years was collected which was related to
total number of exits in each year. The data shows the figures that how drastically it changed
over the period of 10 years. It can be seen that number of passengers exiting was reduced after
the year 2015-16.
Years Total number of exits
2008 -2009 12
2009 - 2010 14
2010- 2011 11
2011 - 2012 71
2012 - 2013 52
2013- 2014 48
2014-2015 51
2015-2016 8
2016-2017 15
Collection of numerical data and its analysis with the application of tools and techniques
of statistics could largely benefit in drawing out the most meaningful information from the data.
Statistical data analysis is the method which is concerned with the collection and scrutiny of data
sample thoroughly. It basically summarises the data into such a form which could be used for
taking effective decisions (statistical analysis, 2019). In the present report, data regarding the
station usage of Clock House will be collected for the period of 10 years and presented by the
medium of tables and charts. After which the sample data will be analysed by applying
descriptive statistical techniques so as to determine the trends and pattern of the data. Lastly, the
forecasting of the station usage will be done with the help of linear regression model for 12 and
15 years.
MAIN BODY
1. Presenting the data in table format
Station usage data of Clock House for the 10 years was collected which was related to
total number of exits in each year. The data shows the figures that how drastically it changed
over the period of 10 years. It can be seen that number of passengers exiting was reduced after
the year 2015-16.
Years Total number of exits
2008 -2009 12
2009 - 2010 14
2010- 2011 11
2011 - 2012 71
2012 - 2013 52
2013- 2014 48
2014-2015 51
2015-2016 8
2016-2017 15
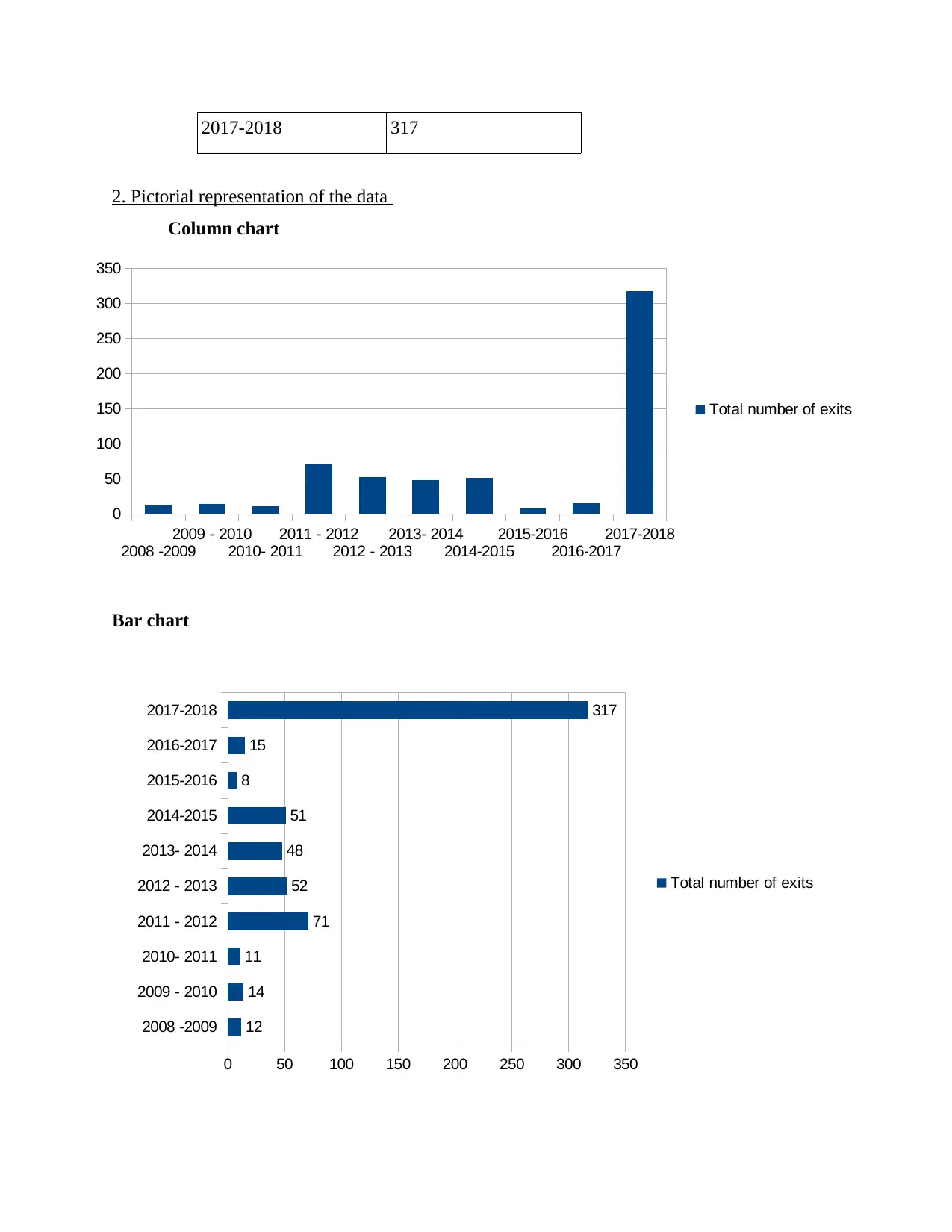
2017-2018 317
2. Pictorial representation of the data
Column chart
Bar chart
2008 -2009
2009 - 2010
2010- 2011
2011 - 2012
2012 - 2013
2013- 2014
2014-2015
2015-2016
2016-2017
2017-2018
0
50
100
150
200
250
300
350
Total number of exits
2008 -2009
2009 - 2010
2010- 2011
2011 - 2012
2012 - 2013
2013- 2014
2014-2015
2015-2016
2016-2017
2017-2018
0 50 100 150 200 250 300 350
12
14
11
71
52
48
51
8
15
317
Total number of exits
2. Pictorial representation of the data
Column chart
Bar chart
2008 -2009
2009 - 2010
2010- 2011
2011 - 2012
2012 - 2013
2013- 2014
2014-2015
2015-2016
2016-2017
2017-2018
0
50
100
150
200
250
300
350
Total number of exits
2008 -2009
2009 - 2010
2010- 2011
2011 - 2012
2012 - 2013
2013- 2014
2014-2015
2015-2016
2016-2017
2017-2018
0 50 100 150 200 250 300 350
12
14
11
71
52
48
51
8
15
317
Total number of exits
Secure Best Marks with AI Grader
Need help grading? Try our AI Grader for instant feedback on your assignments.
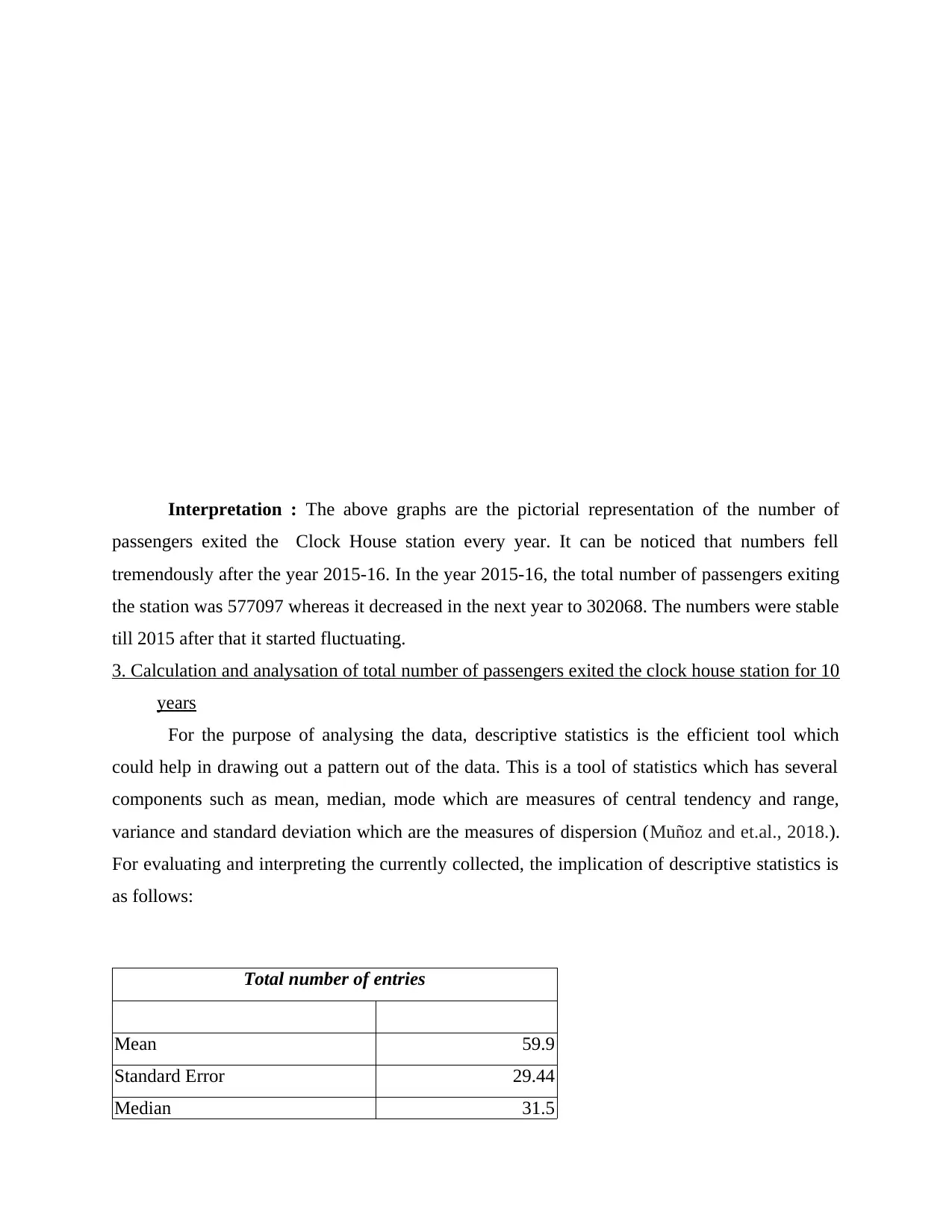
Interpretation : The above graphs are the pictorial representation of the number of
passengers exited the Clock House station every year. It can be noticed that numbers fell
tremendously after the year 2015-16. In the year 2015-16, the total number of passengers exiting
the station was 577097 whereas it decreased in the next year to 302068. The numbers were stable
till 2015 after that it started fluctuating.
3. Calculation and analysation of total number of passengers exited the clock house station for 10
years
For the purpose of analysing the data, descriptive statistics is the efficient tool which
could help in drawing out a pattern out of the data. This is a tool of statistics which has several
components such as mean, median, mode which are measures of central tendency and range,
variance and standard deviation which are the measures of dispersion (Muñoz and et.al., 2018.).
For evaluating and interpreting the currently collected, the implication of descriptive statistics is
as follows:
Total number of entries
Mean 59.9
Standard Error 29.44
Median 31.5
passengers exited the Clock House station every year. It can be noticed that numbers fell
tremendously after the year 2015-16. In the year 2015-16, the total number of passengers exiting
the station was 577097 whereas it decreased in the next year to 302068. The numbers were stable
till 2015 after that it started fluctuating.
3. Calculation and analysation of total number of passengers exited the clock house station for 10
years
For the purpose of analysing the data, descriptive statistics is the efficient tool which
could help in drawing out a pattern out of the data. This is a tool of statistics which has several
components such as mean, median, mode which are measures of central tendency and range,
variance and standard deviation which are the measures of dispersion (Muñoz and et.al., 2018.).
For evaluating and interpreting the currently collected, the implication of descriptive statistics is
as follows:
Total number of entries
Mean 59.9
Standard Error 29.44
Median 31.5
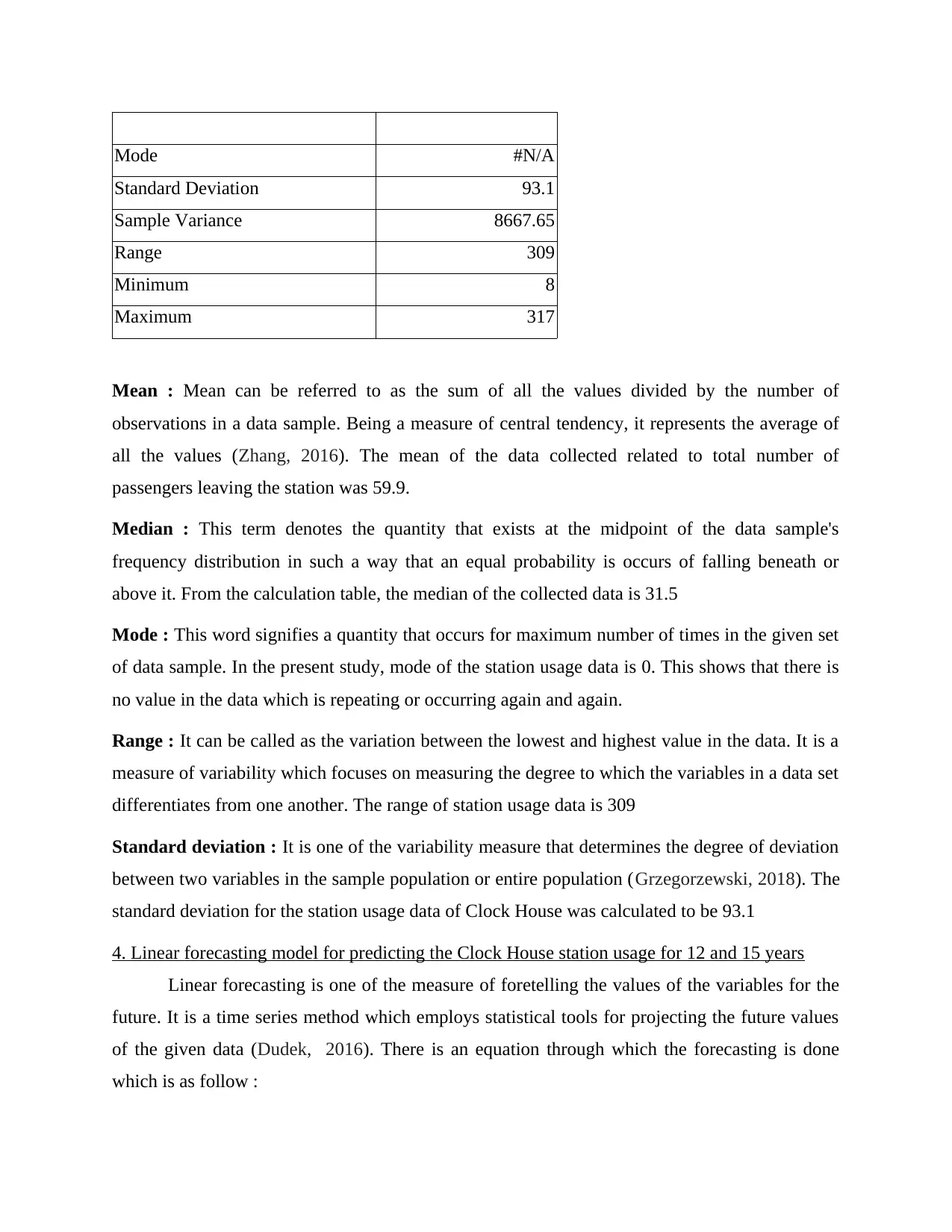
Mode #N/A
Standard Deviation 93.1
Sample Variance 8667.65
Range 309
Minimum 8
Maximum 317
Mean : Mean can be referred to as the sum of all the values divided by the number of
observations in a data sample. Being a measure of central tendency, it represents the average of
all the values (Zhang, 2016). The mean of the data collected related to total number of
passengers leaving the station was 59.9.
Median : This term denotes the quantity that exists at the midpoint of the data sample's
frequency distribution in such a way that an equal probability is occurs of falling beneath or
above it. From the calculation table, the median of the collected data is 31.5
Mode : This word signifies a quantity that occurs for maximum number of times in the given set
of data sample. In the present study, mode of the station usage data is 0. This shows that there is
no value in the data which is repeating or occurring again and again.
Range : It can be called as the variation between the lowest and highest value in the data. It is a
measure of variability which focuses on measuring the degree to which the variables in a data set
differentiates from one another. The range of station usage data is 309
Standard deviation : It is one of the variability measure that determines the degree of deviation
between two variables in the sample population or entire population (Grzegorzewski, 2018). The
standard deviation for the station usage data of Clock House was calculated to be 93.1
4. Linear forecasting model for predicting the Clock House station usage for 12 and 15 years
Linear forecasting is one of the measure of foretelling the values of the variables for the
future. It is a time series method which employs statistical tools for projecting the future values
of the given data (Dudek, 2016). There is an equation through which the forecasting is done
which is as follow :
Standard Deviation 93.1
Sample Variance 8667.65
Range 309
Minimum 8
Maximum 317
Mean : Mean can be referred to as the sum of all the values divided by the number of
observations in a data sample. Being a measure of central tendency, it represents the average of
all the values (Zhang, 2016). The mean of the data collected related to total number of
passengers leaving the station was 59.9.
Median : This term denotes the quantity that exists at the midpoint of the data sample's
frequency distribution in such a way that an equal probability is occurs of falling beneath or
above it. From the calculation table, the median of the collected data is 31.5
Mode : This word signifies a quantity that occurs for maximum number of times in the given set
of data sample. In the present study, mode of the station usage data is 0. This shows that there is
no value in the data which is repeating or occurring again and again.
Range : It can be called as the variation between the lowest and highest value in the data. It is a
measure of variability which focuses on measuring the degree to which the variables in a data set
differentiates from one another. The range of station usage data is 309
Standard deviation : It is one of the variability measure that determines the degree of deviation
between two variables in the sample population or entire population (Grzegorzewski, 2018). The
standard deviation for the station usage data of Clock House was calculated to be 93.1
4. Linear forecasting model for predicting the Clock House station usage for 12 and 15 years
Linear forecasting is one of the measure of foretelling the values of the variables for the
future. It is a time series method which employs statistical tools for projecting the future values
of the given data (Dudek, 2016). There is an equation through which the forecasting is done
which is as follow :
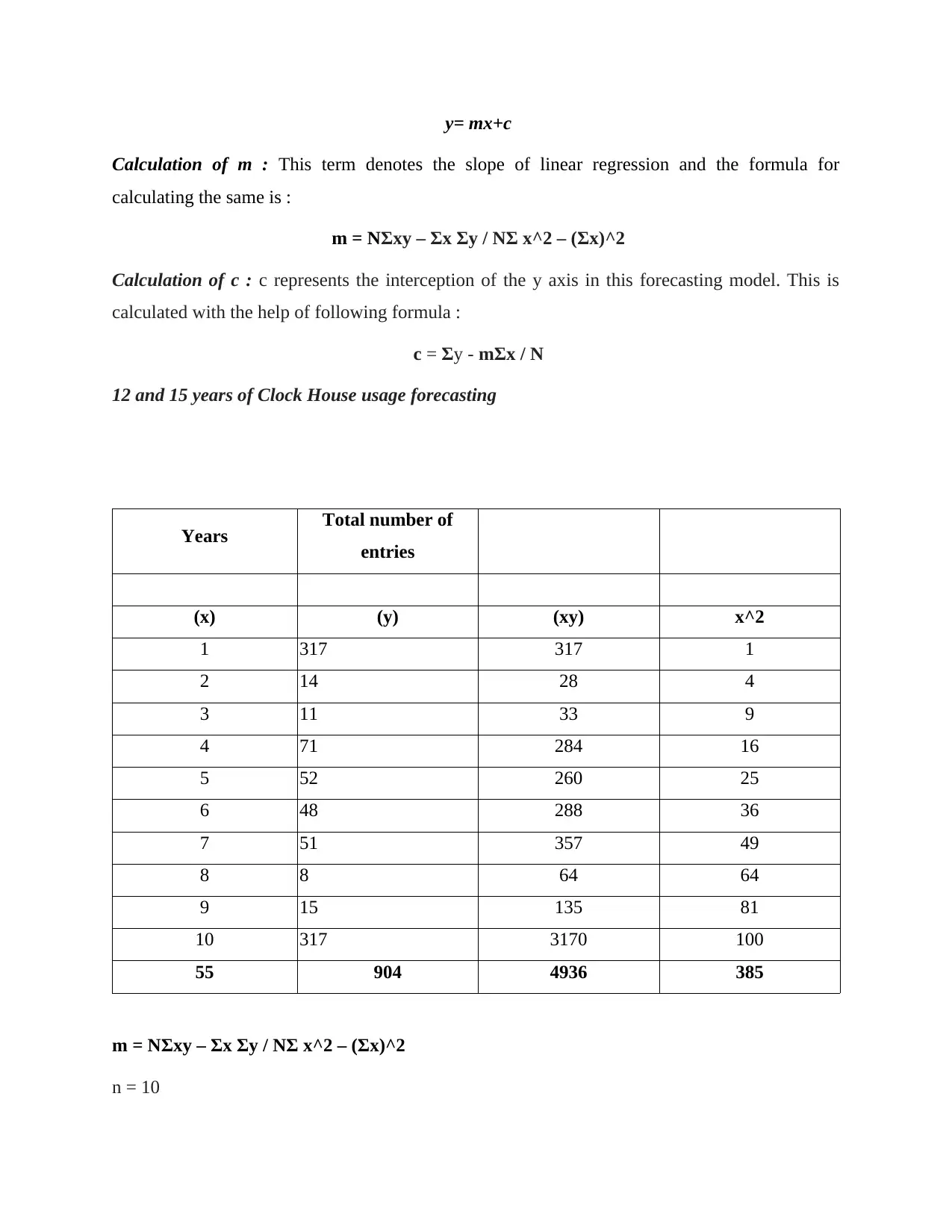
y= mx+c
Calculation of m : This term denotes the slope of linear regression and the formula for
calculating the same is :
m = NΣxy – Σx Σy / NΣ x^2 – (Σx)^2
Calculation of c : c represents the interception of the y axis in this forecasting model. This is
calculated with the help of following formula :
c = Σy - mΣx / N
12 and 15 years of Clock House usage forecasting
Years Total number of
entries
(x) (y) (xy) x^2
1 317 317 1
2 14 28 4
3 11 33 9
4 71 284 16
5 52 260 25
6 48 288 36
7 51 357 49
8 8 64 64
9 15 135 81
10 317 3170 100
55 904 4936 385
m = NΣxy – Σx Σy / NΣ x^2 – (Σx)^2
n = 10
Calculation of m : This term denotes the slope of linear regression and the formula for
calculating the same is :
m = NΣxy – Σx Σy / NΣ x^2 – (Σx)^2
Calculation of c : c represents the interception of the y axis in this forecasting model. This is
calculated with the help of following formula :
c = Σy - mΣx / N
12 and 15 years of Clock House usage forecasting
Years Total number of
entries
(x) (y) (xy) x^2
1 317 317 1
2 14 28 4
3 11 33 9
4 71 284 16
5 52 260 25
6 48 288 36
7 51 357 49
8 8 64 64
9 15 135 81
10 317 3170 100
55 904 4936 385
m = NΣxy – Σx Σy / NΣ x^2 – (Σx)^2
n = 10
Paraphrase This Document
Need a fresh take? Get an instant paraphrase of this document with our AI Paraphraser
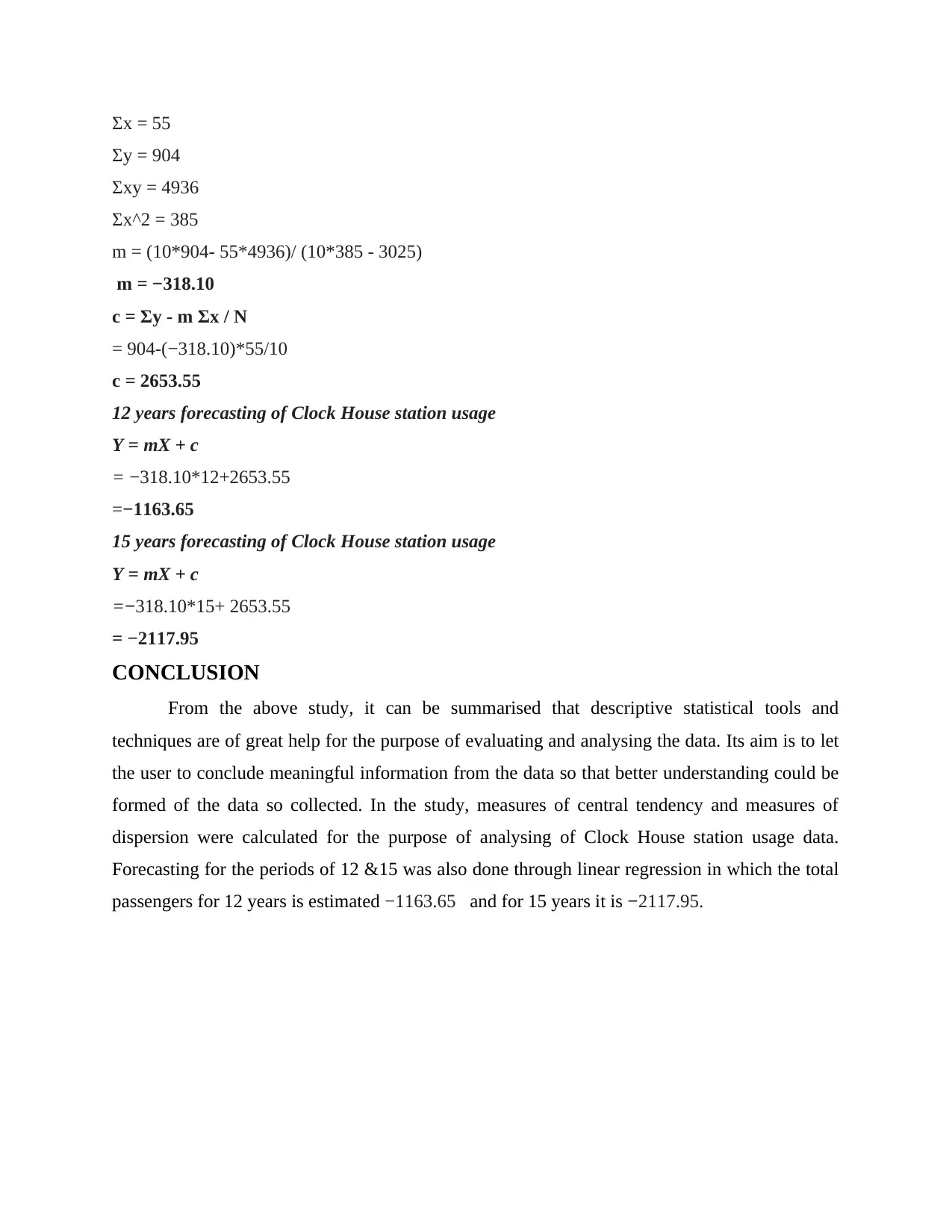
Σx = 55
Σy = 904
Σxy = 4936
Σx^2 = 385
m = (10*904- 55*4936)/ (10*385 - 3025)
m = −318.10
c = Σy - m Σx / N
= 904-(−318.10)*55/10
c = 2653.55
12 years forecasting of Clock House station usage
Y = mX + c
= −318.10*12+2653.55
=−1163.65
15 years forecasting of Clock House station usage
Y = mX + c
=−318.10*15+ 2653.55
= −2117.95
CONCLUSION
From the above study, it can be summarised that descriptive statistical tools and
techniques are of great help for the purpose of evaluating and analysing the data. Its aim is to let
the user to conclude meaningful information from the data so that better understanding could be
formed of the data so collected. In the study, measures of central tendency and measures of
dispersion were calculated for the purpose of analysing of Clock House station usage data.
Forecasting for the periods of 12 &15 was also done through linear regression in which the total
passengers for 12 years is estimated −1163.65 and for 15 years it is −2117.95.
Σy = 904
Σxy = 4936
Σx^2 = 385
m = (10*904- 55*4936)/ (10*385 - 3025)
m = −318.10
c = Σy - m Σx / N
= 904-(−318.10)*55/10
c = 2653.55
12 years forecasting of Clock House station usage
Y = mX + c
= −318.10*12+2653.55
=−1163.65
15 years forecasting of Clock House station usage
Y = mX + c
=−318.10*15+ 2653.55
= −2117.95
CONCLUSION
From the above study, it can be summarised that descriptive statistical tools and
techniques are of great help for the purpose of evaluating and analysing the data. Its aim is to let
the user to conclude meaningful information from the data so that better understanding could be
formed of the data so collected. In the study, measures of central tendency and measures of
dispersion were calculated for the purpose of analysing of Clock House station usage data.
Forecasting for the periods of 12 &15 was also done through linear regression in which the total
passengers for 12 years is estimated −1163.65 and for 15 years it is −2117.95.
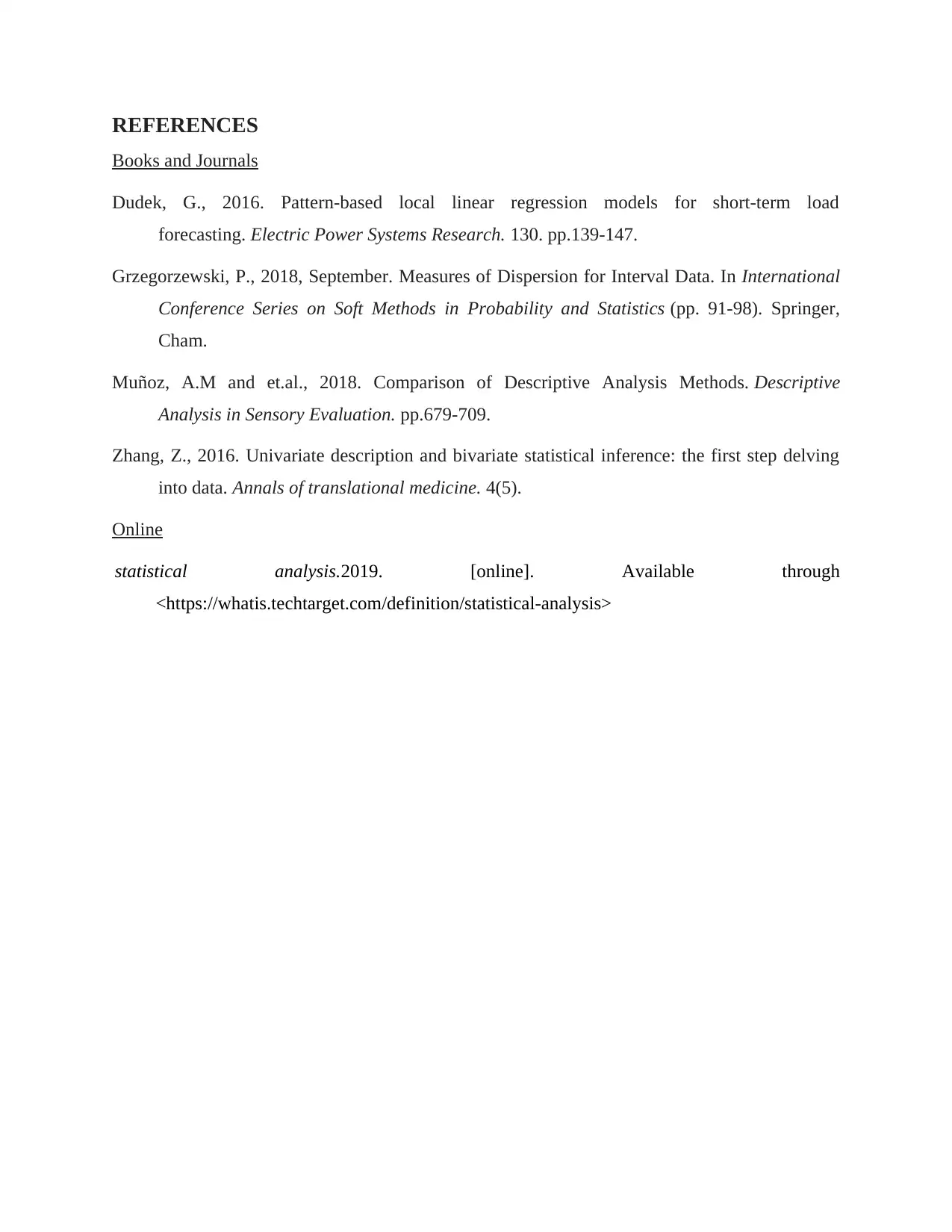
REFERENCES
Books and Journals
Dudek, G., 2016. Pattern-based local linear regression models for short-term load
forecasting. Electric Power Systems Research. 130. pp.139-147.
Grzegorzewski, P., 2018, September. Measures of Dispersion for Interval Data. In International
Conference Series on Soft Methods in Probability and Statistics (pp. 91-98). Springer,
Cham.
Muñoz, A.M and et.al., 2018. Comparison of Descriptive Analysis Methods. Descriptive
Analysis in Sensory Evaluation. pp.679-709.
Zhang, Z., 2016. Univariate description and bivariate statistical inference: the first step delving
into data. Annals of translational medicine. 4(5).
Online
statistical analysis.2019. [online]. Available through
<https://whatis.techtarget.com/definition/statistical-analysis>
Books and Journals
Dudek, G., 2016. Pattern-based local linear regression models for short-term load
forecasting. Electric Power Systems Research. 130. pp.139-147.
Grzegorzewski, P., 2018, September. Measures of Dispersion for Interval Data. In International
Conference Series on Soft Methods in Probability and Statistics (pp. 91-98). Springer,
Cham.
Muñoz, A.M and et.al., 2018. Comparison of Descriptive Analysis Methods. Descriptive
Analysis in Sensory Evaluation. pp.679-709.
Zhang, Z., 2016. Univariate description and bivariate statistical inference: the first step delving
into data. Annals of translational medicine. 4(5).
Online
statistical analysis.2019. [online]. Available through
<https://whatis.techtarget.com/definition/statistical-analysis>
1 out of 9
Related Documents
![[object Object]](/_next/image/?url=%2F_next%2Fstatic%2Fmedia%2Flogo.6d15ce61.png&w=640&q=75)
Your All-in-One AI-Powered Toolkit for Academic Success.
+13062052269
info@desklib.com
Available 24*7 on WhatsApp / Email
Unlock your academic potential
© 2024 | Zucol Services PVT LTD | All rights reserved.