Information Extraction from Images
VerifiedAdded on 2023/01/18
|16
|2143
|51
AI Summary
This article discusses the process of extracting information from images using radiometric enhancement, band ratios, and image classification. It explores the results and analysis of the extracted data, including the role of histogram manipulation in visualizing ratio images and NDVI. The article also highlights the classes identified in the classified image and discusses classification errors. Overall, it provides insights into the utilization of band ratios and combinations for analyzing images and extracting valuable information.
Contribute Materials
Your contribution can guide someone’s learning journey. Share your
documents today.
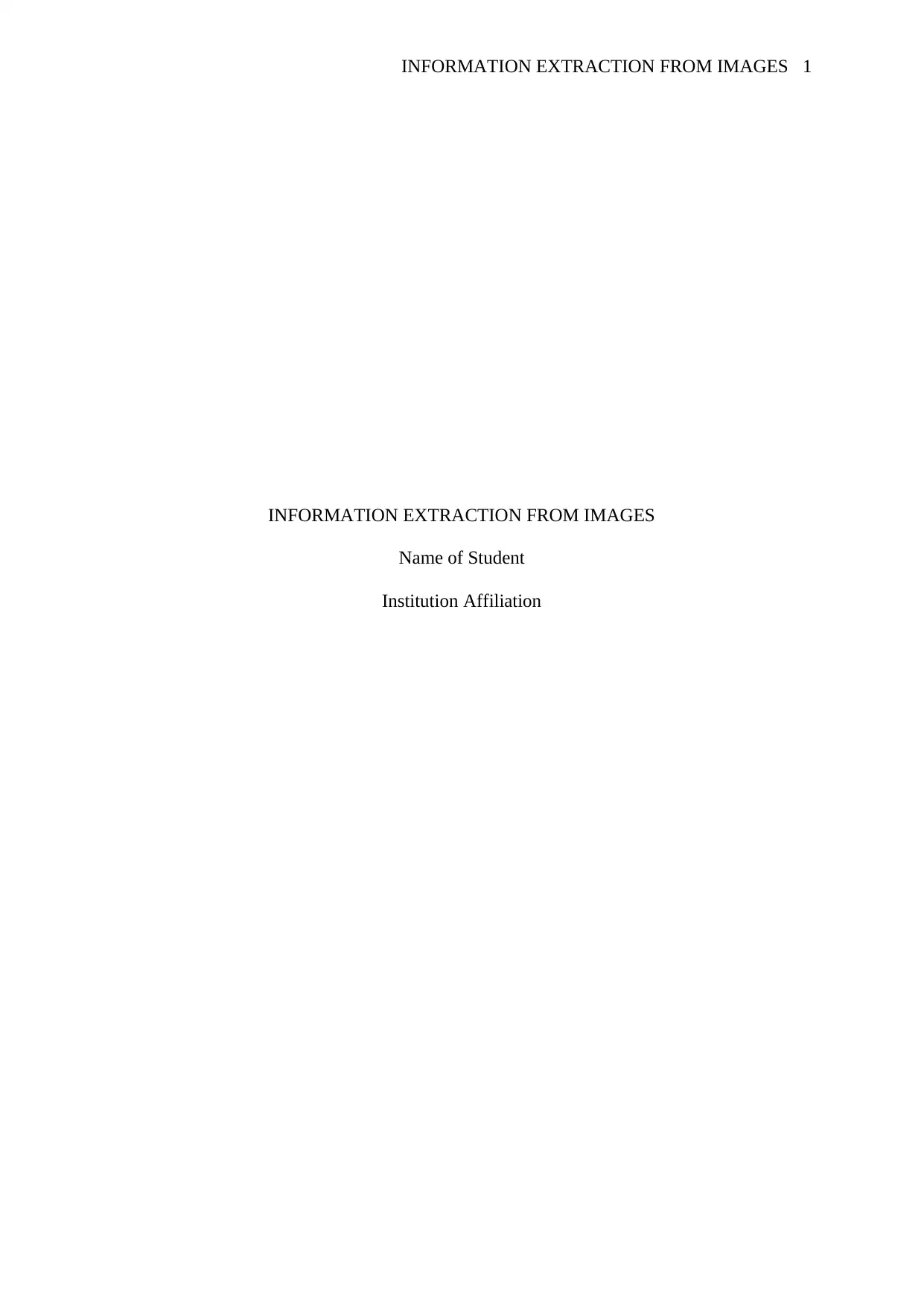
INFORMATION EXTRACTION FROM IMAGES 1
INFORMATION EXTRACTION FROM IMAGES
Name of Student
Institution Affiliation
INFORMATION EXTRACTION FROM IMAGES
Name of Student
Institution Affiliation
Secure Best Marks with AI Grader
Need help grading? Try our AI Grader for instant feedback on your assignments.
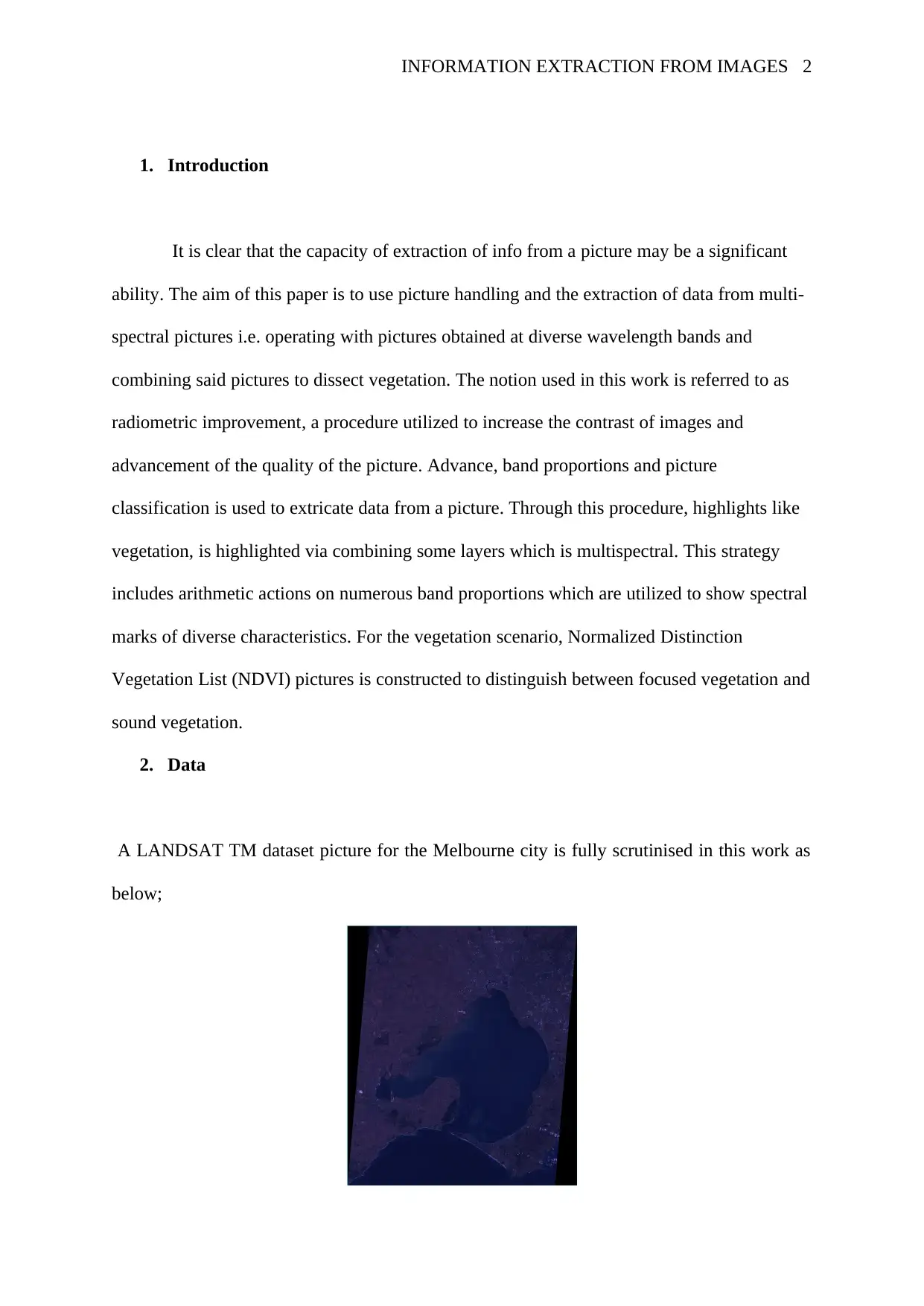
INFORMATION EXTRACTION FROM IMAGES 2
1. Introduction
It is clear that the capacity of extraction of info from a picture may be a significant
ability. The aim of this paper is to use picture handling and the extraction of data from multi-
spectral pictures i.e. operating with pictures obtained at diverse wavelength bands and
combining said pictures to dissect vegetation. The notion used in this work is referred to as
radiometric improvement, a procedure utilized to increase the contrast of images and
advancement of the quality of the picture. Advance, band proportions and picture
classification is used to extricate data from a picture. Through this procedure, highlights like
vegetation, is highlighted via combining some layers which is multispectral. This strategy
includes arithmetic actions on numerous band proportions which are utilized to show spectral
marks of diverse characteristics. For the vegetation scenario, Normalized Distinction
Vegetation List (NDVI) pictures is constructed to distinguish between focused vegetation and
sound vegetation.
2. Data
A LANDSAT TM dataset picture for the Melbourne city is fully scrutinised in this work as
below;
1. Introduction
It is clear that the capacity of extraction of info from a picture may be a significant
ability. The aim of this paper is to use picture handling and the extraction of data from multi-
spectral pictures i.e. operating with pictures obtained at diverse wavelength bands and
combining said pictures to dissect vegetation. The notion used in this work is referred to as
radiometric improvement, a procedure utilized to increase the contrast of images and
advancement of the quality of the picture. Advance, band proportions and picture
classification is used to extricate data from a picture. Through this procedure, highlights like
vegetation, is highlighted via combining some layers which is multispectral. This strategy
includes arithmetic actions on numerous band proportions which are utilized to show spectral
marks of diverse characteristics. For the vegetation scenario, Normalized Distinction
Vegetation List (NDVI) pictures is constructed to distinguish between focused vegetation and
sound vegetation.
2. Data
A LANDSAT TM dataset picture for the Melbourne city is fully scrutinised in this work as
below;
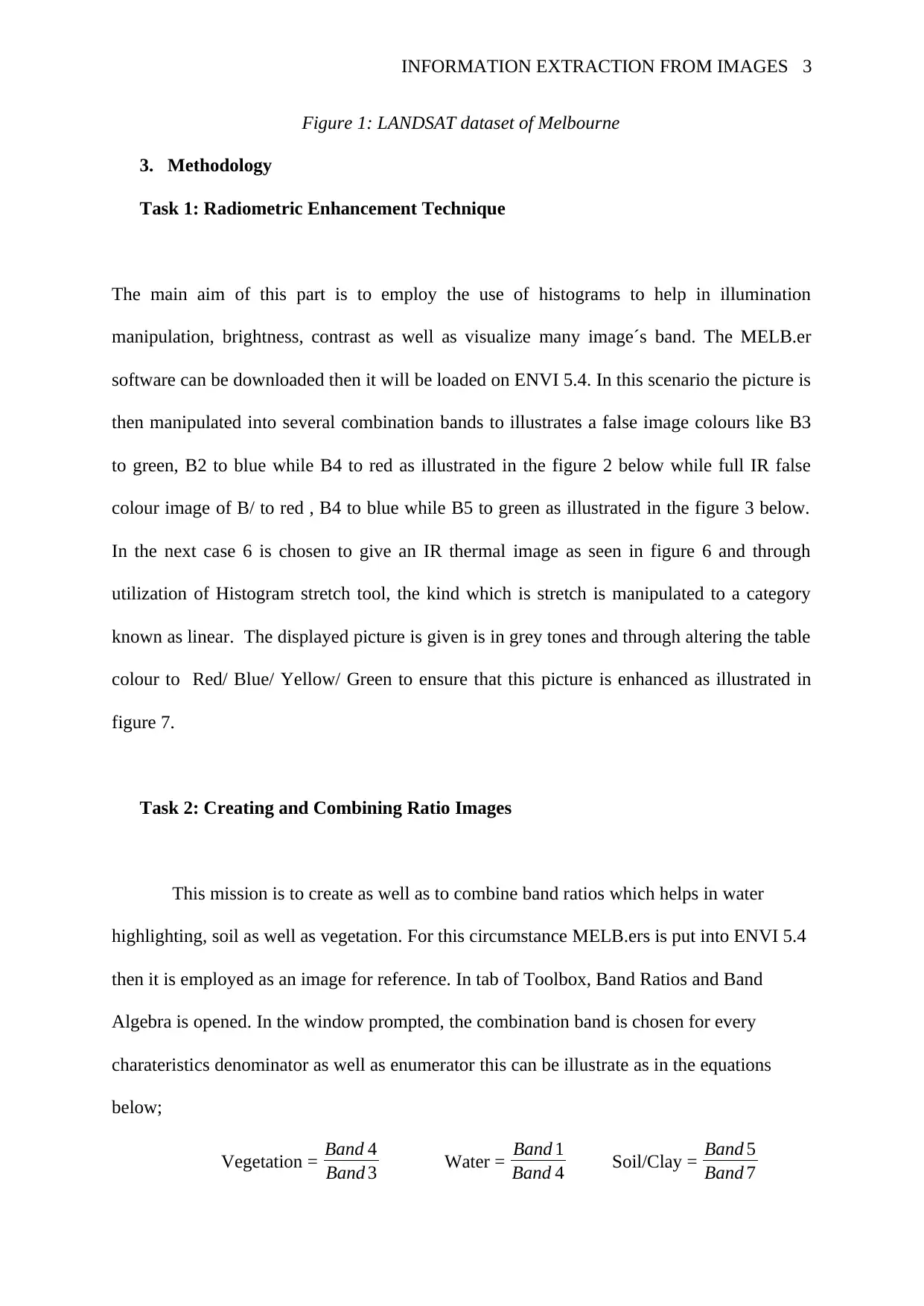
INFORMATION EXTRACTION FROM IMAGES 3
Figure 1: LANDSAT dataset of Melbourne
3. Methodology
Task 1: Radiometric Enhancement Technique
The main aim of this part is to employ the use of histograms to help in illumination
manipulation, brightness, contrast as well as visualize many image´s band. The MELB.er
software can be downloaded then it will be loaded on ENVI 5.4. In this scenario the picture is
then manipulated into several combination bands to illustrates a false image colours like B3
to green, B2 to blue while B4 to red as illustrated in the figure 2 below while full IR false
colour image of B/ to red , B4 to blue while B5 to green as illustrated in the figure 3 below.
In the next case 6 is chosen to give an IR thermal image as seen in figure 6 and through
utilization of Histogram stretch tool, the kind which is stretch is manipulated to a category
known as linear. The displayed picture is given is in grey tones and through altering the table
colour to Red/ Blue/ Yellow/ Green to ensure that this picture is enhanced as illustrated in
figure 7.
Task 2: Creating and Combining Ratio Images
This mission is to create as well as to combine band ratios which helps in water
highlighting, soil as well as vegetation. For this circumstance MELB.ers is put into ENVI 5.4
then it is employed as an image for reference. In tab of Toolbox, Band Ratios and Band
Algebra is opened. In the window prompted, the combination band is chosen for every
charateristics denominator as well as enumerator this can be illustrate as in the equations
below;
Vegetation = Band 4
Band 3 Water = Band 1
Band 4 Soil/Clay = Band 5
Band 7
Figure 1: LANDSAT dataset of Melbourne
3. Methodology
Task 1: Radiometric Enhancement Technique
The main aim of this part is to employ the use of histograms to help in illumination
manipulation, brightness, contrast as well as visualize many image´s band. The MELB.er
software can be downloaded then it will be loaded on ENVI 5.4. In this scenario the picture is
then manipulated into several combination bands to illustrates a false image colours like B3
to green, B2 to blue while B4 to red as illustrated in the figure 2 below while full IR false
colour image of B/ to red , B4 to blue while B5 to green as illustrated in the figure 3 below.
In the next case 6 is chosen to give an IR thermal image as seen in figure 6 and through
utilization of Histogram stretch tool, the kind which is stretch is manipulated to a category
known as linear. The displayed picture is given is in grey tones and through altering the table
colour to Red/ Blue/ Yellow/ Green to ensure that this picture is enhanced as illustrated in
figure 7.
Task 2: Creating and Combining Ratio Images
This mission is to create as well as to combine band ratios which helps in water
highlighting, soil as well as vegetation. For this circumstance MELB.ers is put into ENVI 5.4
then it is employed as an image for reference. In tab of Toolbox, Band Ratios and Band
Algebra is opened. In the window prompted, the combination band is chosen for every
charateristics denominator as well as enumerator this can be illustrate as in the equations
below;
Vegetation = Band 4
Band 3 Water = Band 1
Band 4 Soil/Clay = Band 5
Band 7
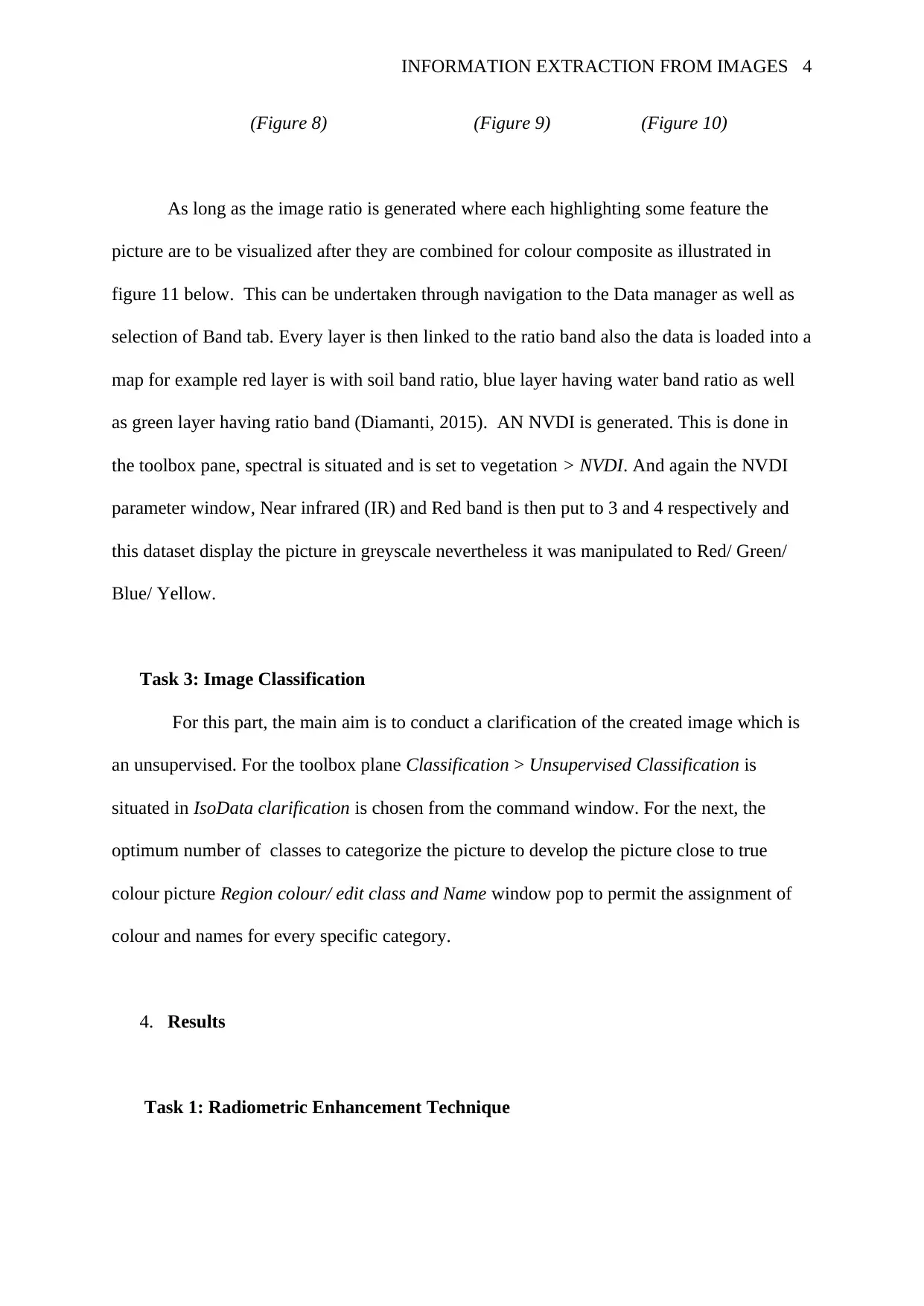
INFORMATION EXTRACTION FROM IMAGES 4
(Figure 8) (Figure 9) (Figure 10)
As long as the image ratio is generated where each highlighting some feature the
picture are to be visualized after they are combined for colour composite as illustrated in
figure 11 below. This can be undertaken through navigation to the Data manager as well as
selection of Band tab. Every layer is then linked to the ratio band also the data is loaded into a
map for example red layer is with soil band ratio, blue layer having water band ratio as well
as green layer having ratio band (Diamanti, 2015). AN NVDI is generated. This is done in
the toolbox pane, spectral is situated and is set to vegetation > NVDI. And again the NVDI
parameter window, Near infrared (IR) and Red band is then put to 3 and 4 respectively and
this dataset display the picture in greyscale nevertheless it was manipulated to Red/ Green/
Blue/ Yellow.
Task 3: Image Classification
For this part, the main aim is to conduct a clarification of the created image which is
an unsupervised. For the toolbox plane Classification > Unsupervised Classification is
situated in IsoData clarification is chosen from the command window. For the next, the
optimum number of classes to categorize the picture to develop the picture close to true
colour picture Region colour/ edit class and Name window pop to permit the assignment of
colour and names for every specific category.
4. Results
Task 1: Radiometric Enhancement Technique
(Figure 8) (Figure 9) (Figure 10)
As long as the image ratio is generated where each highlighting some feature the
picture are to be visualized after they are combined for colour composite as illustrated in
figure 11 below. This can be undertaken through navigation to the Data manager as well as
selection of Band tab. Every layer is then linked to the ratio band also the data is loaded into a
map for example red layer is with soil band ratio, blue layer having water band ratio as well
as green layer having ratio band (Diamanti, 2015). AN NVDI is generated. This is done in
the toolbox pane, spectral is situated and is set to vegetation > NVDI. And again the NVDI
parameter window, Near infrared (IR) and Red band is then put to 3 and 4 respectively and
this dataset display the picture in greyscale nevertheless it was manipulated to Red/ Green/
Blue/ Yellow.
Task 3: Image Classification
For this part, the main aim is to conduct a clarification of the created image which is
an unsupervised. For the toolbox plane Classification > Unsupervised Classification is
situated in IsoData clarification is chosen from the command window. For the next, the
optimum number of classes to categorize the picture to develop the picture close to true
colour picture Region colour/ edit class and Name window pop to permit the assignment of
colour and names for every specific category.
4. Results
Task 1: Radiometric Enhancement Technique
Secure Best Marks with AI Grader
Need help grading? Try our AI Grader for instant feedback on your assignments.
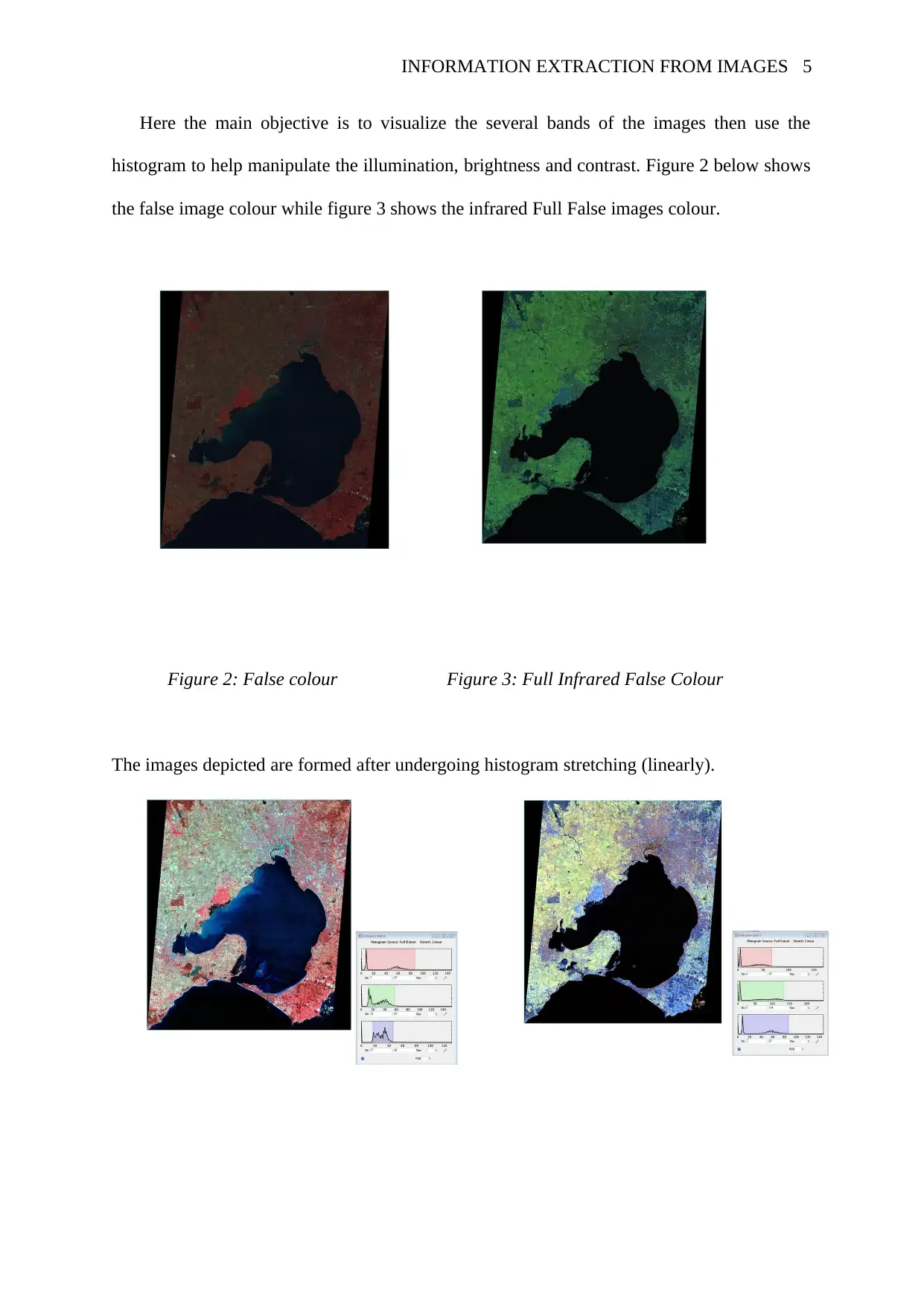
INFORMATION EXTRACTION FROM IMAGES 5
Here the main objective is to visualize the several bands of the images then use the
histogram to help manipulate the illumination, brightness and contrast. Figure 2 below shows
the false image colour while figure 3 shows the infrared Full False images colour.
Figure 2: False colour Figure 3: Full Infrared False Colour
The images depicted are formed after undergoing histogram stretching (linearly).
Here the main objective is to visualize the several bands of the images then use the
histogram to help manipulate the illumination, brightness and contrast. Figure 2 below shows
the false image colour while figure 3 shows the infrared Full False images colour.
Figure 2: False colour Figure 3: Full Infrared False Colour
The images depicted are formed after undergoing histogram stretching (linearly).
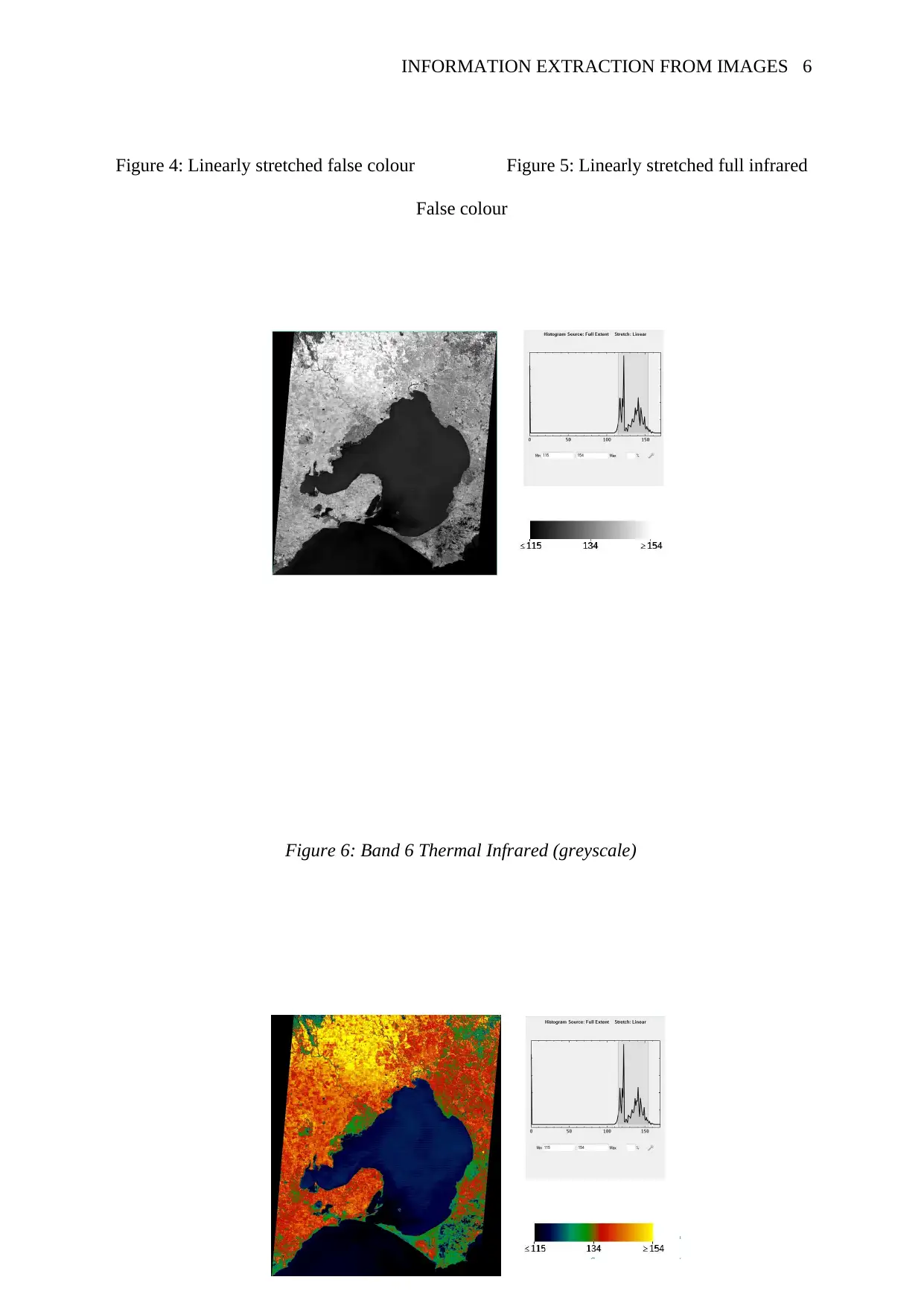
INFORMATION EXTRACTION FROM IMAGES 6
Figure 4: Linearly stretched false colour Figure 5: Linearly stretched full infrared
False colour
Figure 6: Band 6 Thermal Infrared (greyscale)
Figure 4: Linearly stretched false colour Figure 5: Linearly stretched full infrared
False colour
Figure 6: Band 6 Thermal Infrared (greyscale)
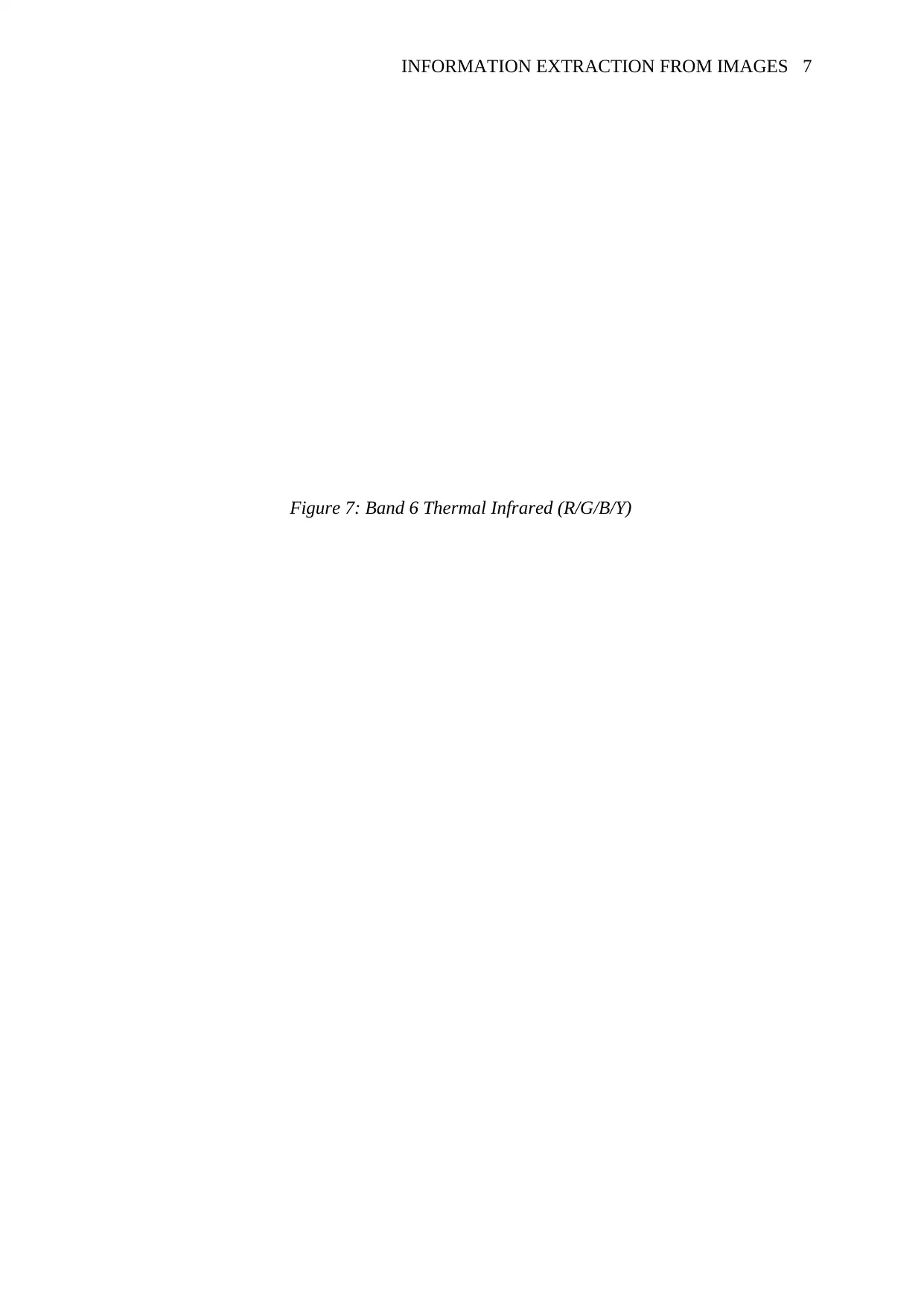
INFORMATION EXTRACTION FROM IMAGES 7
Figure 7: Band 6 Thermal Infrared (R/G/B/Y)
Figure 7: Band 6 Thermal Infrared (R/G/B/Y)
Paraphrase This Document
Need a fresh take? Get an instant paraphrase of this document with our AI Paraphraser
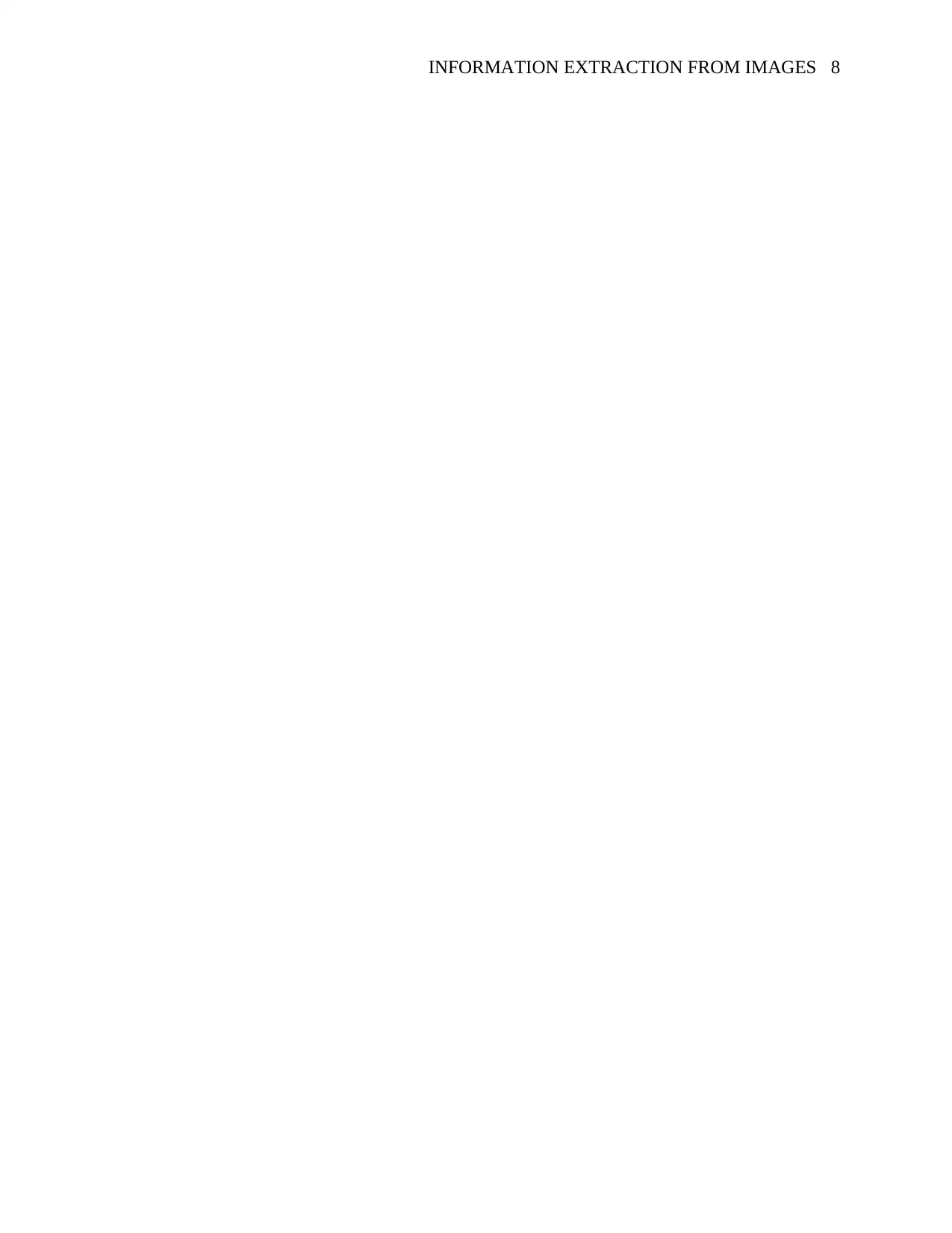
INFORMATION EXTRACTION FROM IMAGES 8
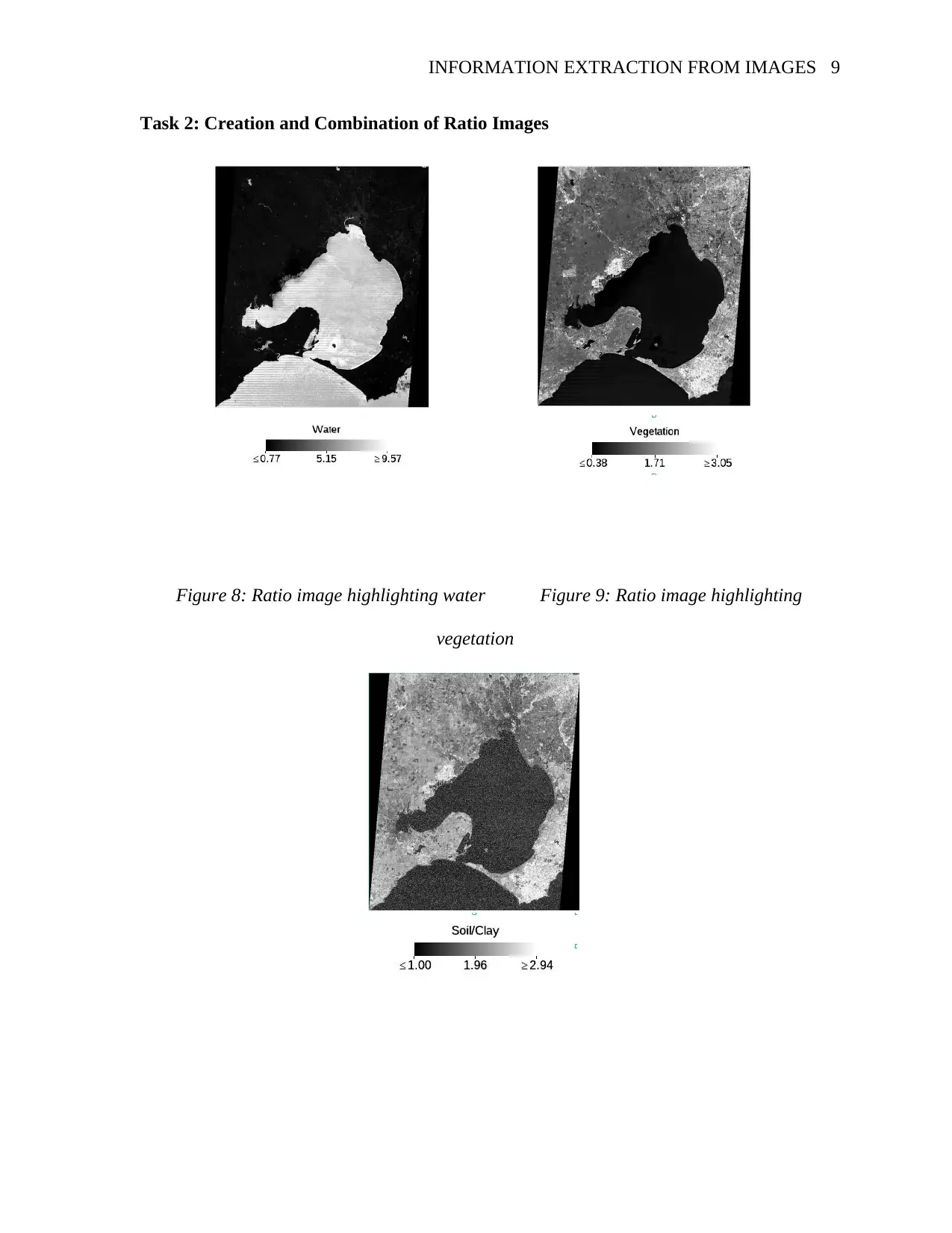
INFORMATION EXTRACTION FROM IMAGES 9
Task 2: Creation and Combination of Ratio Images
Figure 8: Ratio image highlighting water Figure 9: Ratio image highlighting
vegetation
Task 2: Creation and Combination of Ratio Images
Figure 8: Ratio image highlighting water Figure 9: Ratio image highlighting
vegetation
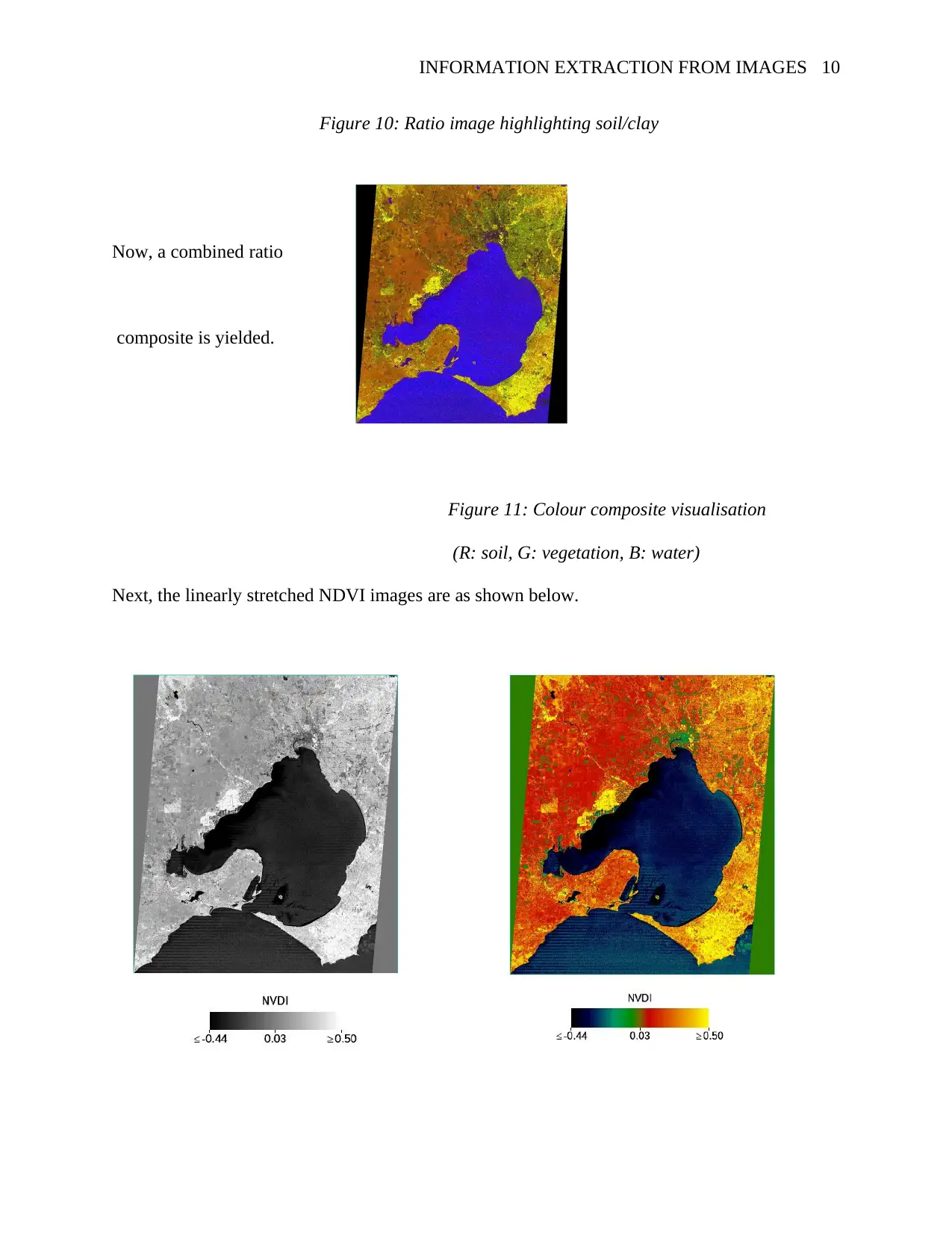
INFORMATION EXTRACTION FROM IMAGES 10
Figure 10: Ratio image highlighting soil/clay
Now, a combined ratio
composite is yielded.
Figure 11: Colour composite visualisation
(R: soil, G: vegetation, B: water)
Next, the linearly stretched NDVI images are as shown below.
Figure 10: Ratio image highlighting soil/clay
Now, a combined ratio
composite is yielded.
Figure 11: Colour composite visualisation
(R: soil, G: vegetation, B: water)
Next, the linearly stretched NDVI images are as shown below.
Secure Best Marks with AI Grader
Need help grading? Try our AI Grader for instant feedback on your assignments.
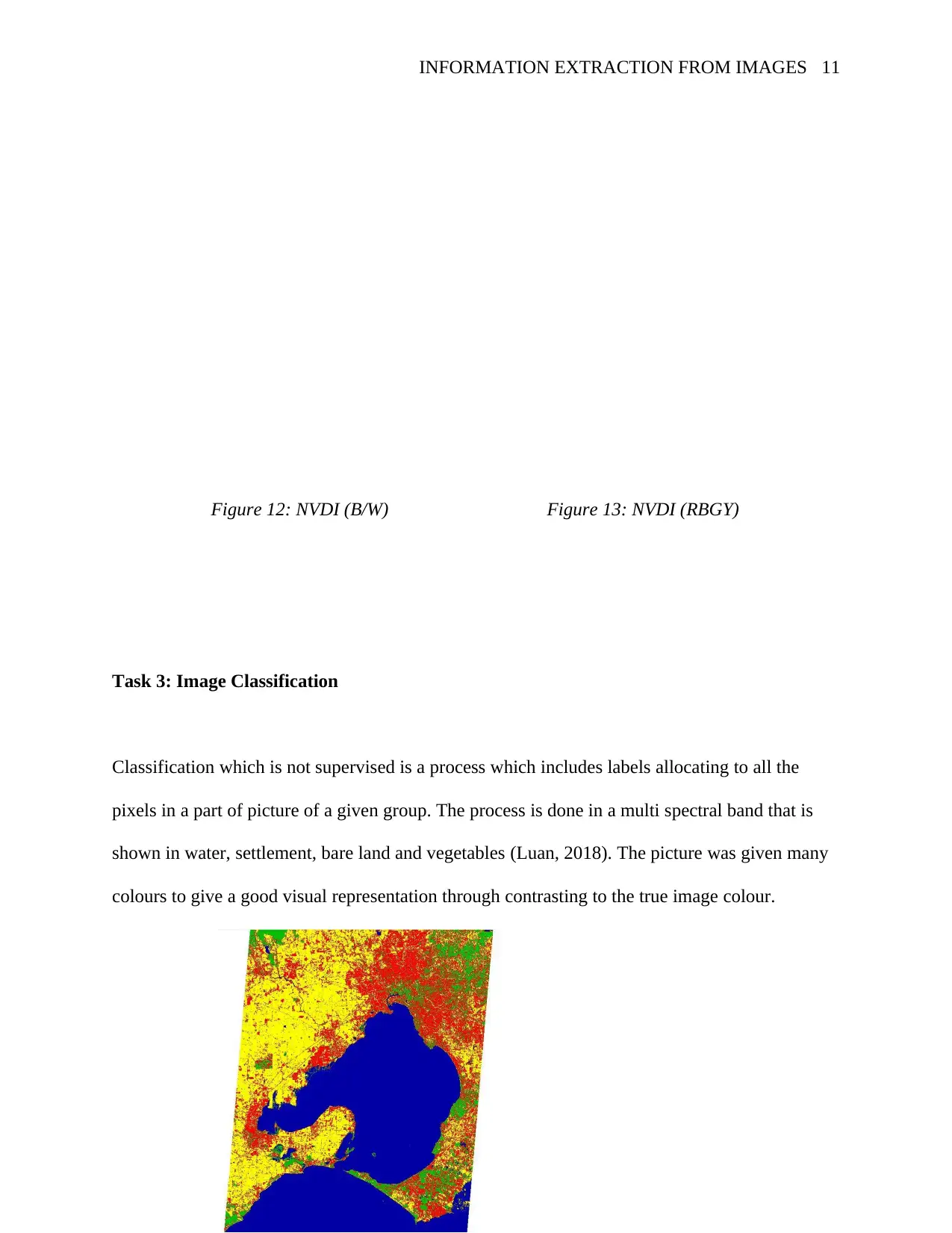
INFORMATION EXTRACTION FROM IMAGES 11
Figure 12: NVDI (B/W) Figure 13: NVDI (RBGY)
Task 3: Image Classification
Classification which is not supervised is a process which includes labels allocating to all the
pixels in a part of picture of a given group. The process is done in a multi spectral band that is
shown in water, settlement, bare land and vegetables (Luan, 2018). The picture was given many
colours to give a good visual representation through contrasting to the true image colour.
Figure 12: NVDI (B/W) Figure 13: NVDI (RBGY)
Task 3: Image Classification
Classification which is not supervised is a process which includes labels allocating to all the
pixels in a part of picture of a given group. The process is done in a multi spectral band that is
shown in water, settlement, bare land and vegetables (Luan, 2018). The picture was given many
colours to give a good visual representation through contrasting to the true image colour.
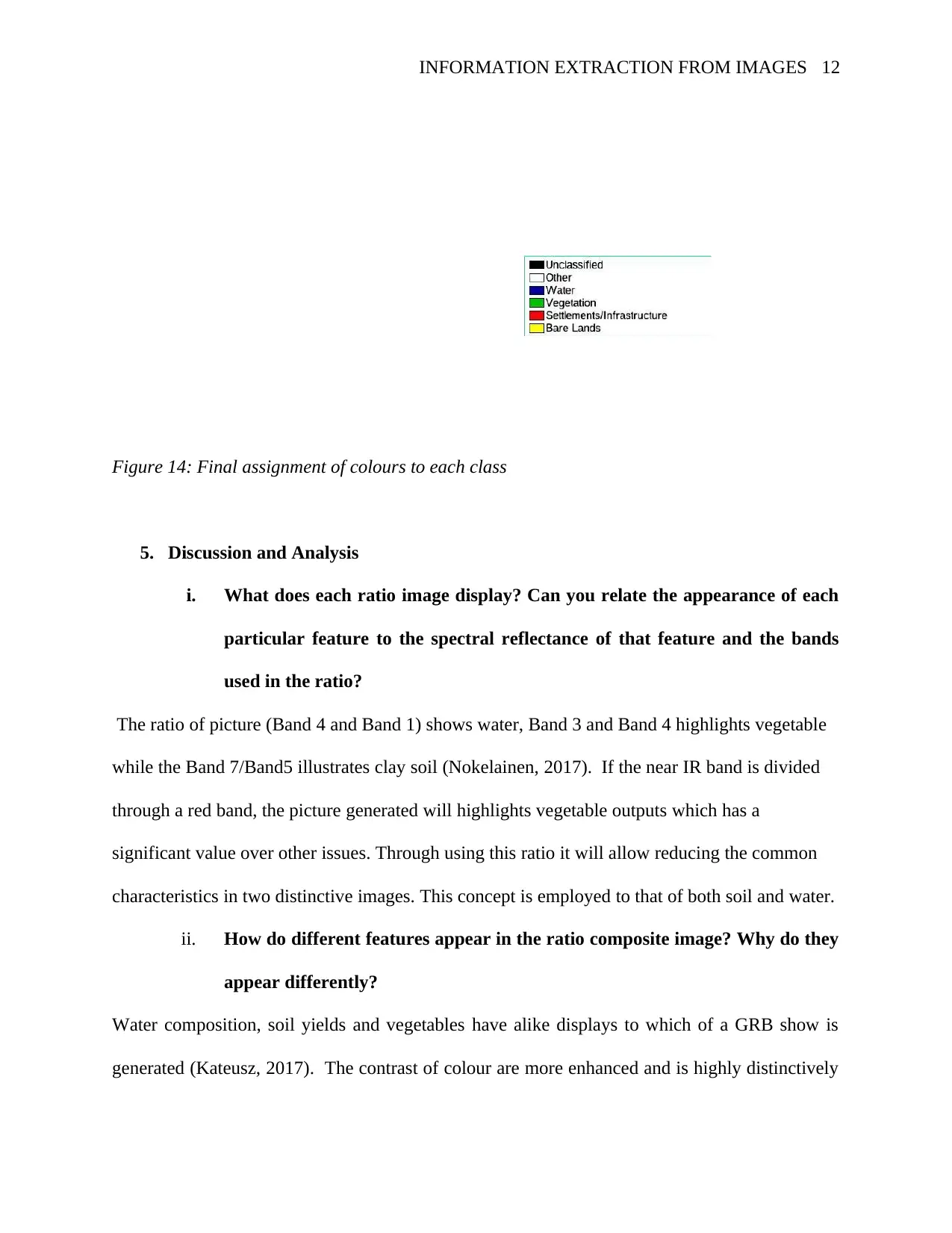
INFORMATION EXTRACTION FROM IMAGES 12
Figure 14: Final assignment of colours to each class
5. Discussion and Analysis
i. What does each ratio image display? Can you relate the appearance of each
particular feature to the spectral reflectance of that feature and the bands
used in the ratio?
The ratio of picture (Band 4 and Band 1) shows water, Band 3 and Band 4 highlights vegetable
while the Band 7/Band5 illustrates clay soil (Nokelainen, 2017). If the near IR band is divided
through a red band, the picture generated will highlights vegetable outputs which has a
significant value over other issues. Through using this ratio it will allow reducing the common
characteristics in two distinctive images. This concept is employed to that of both soil and water.
ii. How do different features appear in the ratio composite image? Why do they
appear differently?
Water composition, soil yields and vegetables have alike displays to which of a GRB show is
generated (Kateusz, 2017). The contrast of colour are more enhanced and is highly distinctively
Figure 14: Final assignment of colours to each class
5. Discussion and Analysis
i. What does each ratio image display? Can you relate the appearance of each
particular feature to the spectral reflectance of that feature and the bands
used in the ratio?
The ratio of picture (Band 4 and Band 1) shows water, Band 3 and Band 4 highlights vegetable
while the Band 7/Band5 illustrates clay soil (Nokelainen, 2017). If the near IR band is divided
through a red band, the picture generated will highlights vegetable outputs which has a
significant value over other issues. Through using this ratio it will allow reducing the common
characteristics in two distinctive images. This concept is employed to that of both soil and water.
ii. How do different features appear in the ratio composite image? Why do they
appear differently?
Water composition, soil yields and vegetables have alike displays to which of a GRB show is
generated (Kateusz, 2017). The contrast of colour are more enhanced and is highly distinctively
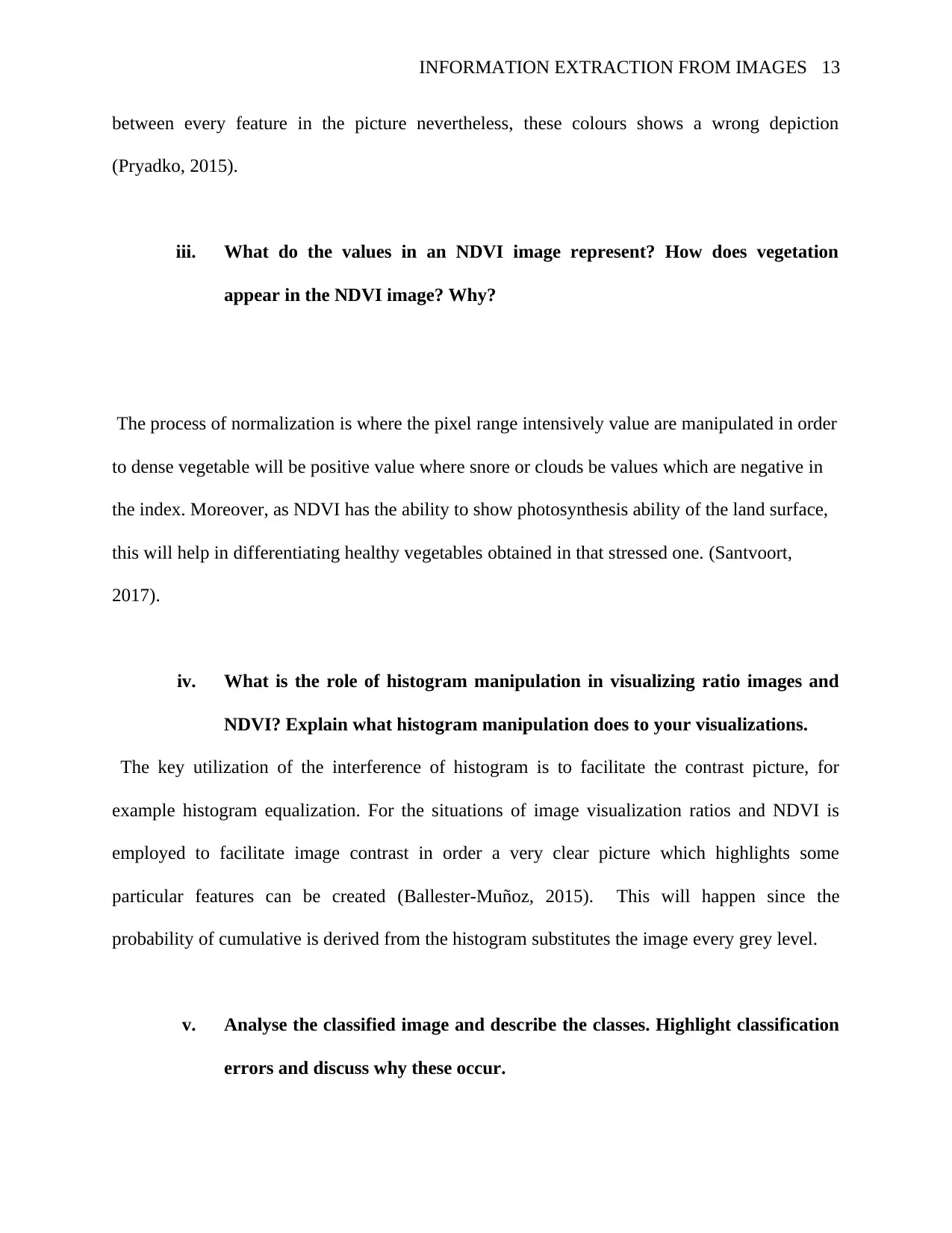
INFORMATION EXTRACTION FROM IMAGES 13
between every feature in the picture nevertheless, these colours shows a wrong depiction
(Pryadko, 2015).
iii. What do the values in an NDVI image represent? How does vegetation
appear in the NDVI image? Why?
The process of normalization is where the pixel range intensively value are manipulated in order
to dense vegetable will be positive value where snore or clouds be values which are negative in
the index. Moreover, as NDVI has the ability to show photosynthesis ability of the land surface,
this will help in differentiating healthy vegetables obtained in that stressed one. (Santvoort,
2017).
iv. What is the role of histogram manipulation in visualizing ratio images and
NDVI? Explain what histogram manipulation does to your visualizations.
The key utilization of the interference of histogram is to facilitate the contrast picture, for
example histogram equalization. For the situations of image visualization ratios and NDVI is
employed to facilitate image contrast in order a very clear picture which highlights some
particular features can be created (Ballester-Muñoz, 2015). This will happen since the
probability of cumulative is derived from the histogram substitutes the image every grey level.
v. Analyse the classified image and describe the classes. Highlight classification
errors and discuss why these occur.
between every feature in the picture nevertheless, these colours shows a wrong depiction
(Pryadko, 2015).
iii. What do the values in an NDVI image represent? How does vegetation
appear in the NDVI image? Why?
The process of normalization is where the pixel range intensively value are manipulated in order
to dense vegetable will be positive value where snore or clouds be values which are negative in
the index. Moreover, as NDVI has the ability to show photosynthesis ability of the land surface,
this will help in differentiating healthy vegetables obtained in that stressed one. (Santvoort,
2017).
iv. What is the role of histogram manipulation in visualizing ratio images and
NDVI? Explain what histogram manipulation does to your visualizations.
The key utilization of the interference of histogram is to facilitate the contrast picture, for
example histogram equalization. For the situations of image visualization ratios and NDVI is
employed to facilitate image contrast in order a very clear picture which highlights some
particular features can be created (Ballester-Muñoz, 2015). This will happen since the
probability of cumulative is derived from the histogram substitutes the image every grey level.
v. Analyse the classified image and describe the classes. Highlight classification
errors and discuss why these occur.
Paraphrase This Document
Need a fresh take? Get an instant paraphrase of this document with our AI Paraphraser
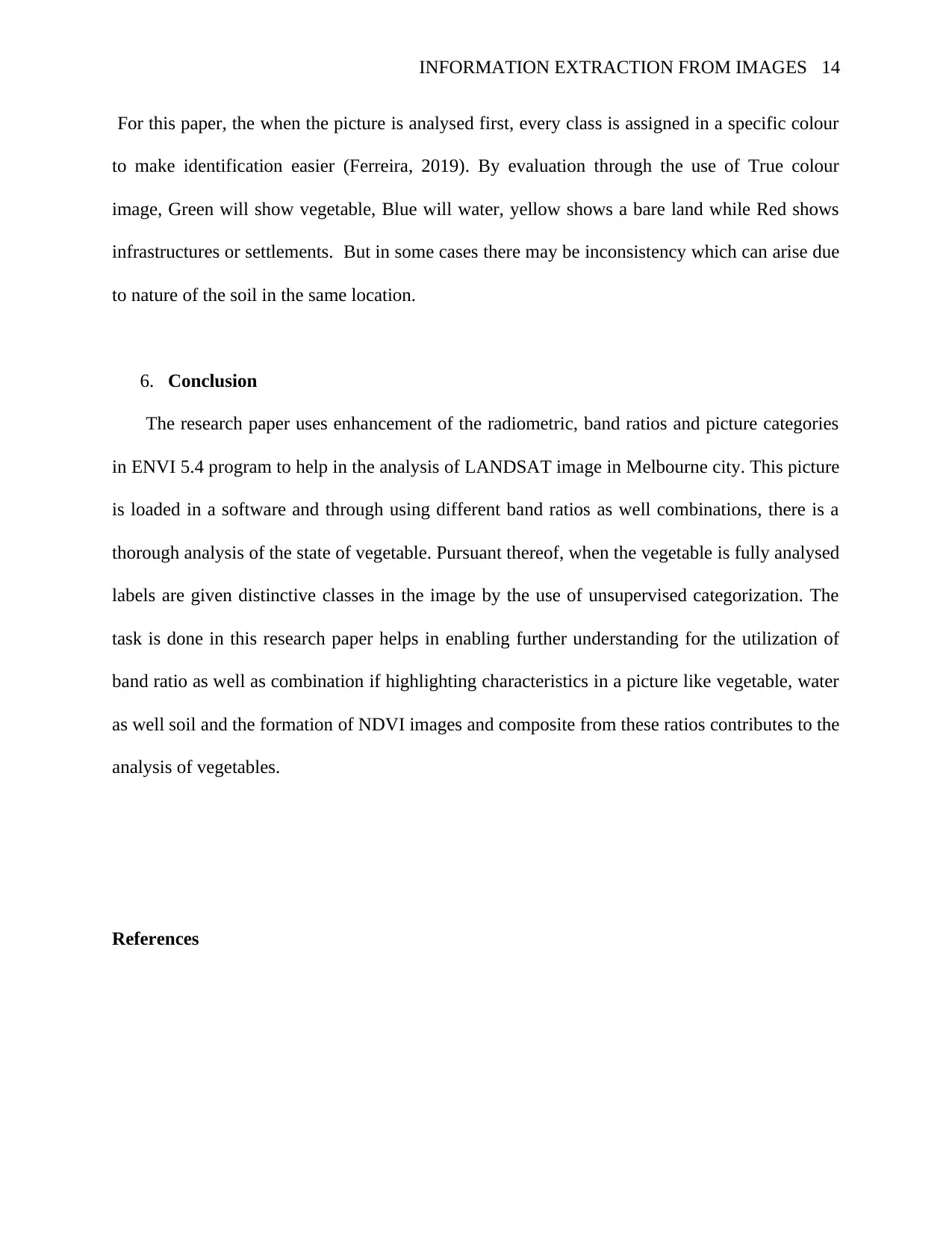
INFORMATION EXTRACTION FROM IMAGES 14
For this paper, the when the picture is analysed first, every class is assigned in a specific colour
to make identification easier (Ferreira, 2019). By evaluation through the use of True colour
image, Green will show vegetable, Blue will water, yellow shows a bare land while Red shows
infrastructures or settlements. But in some cases there may be inconsistency which can arise due
to nature of the soil in the same location.
6. Conclusion
The research paper uses enhancement of the radiometric, band ratios and picture categories
in ENVI 5.4 program to help in the analysis of LANDSAT image in Melbourne city. This picture
is loaded in a software and through using different band ratios as well combinations, there is a
thorough analysis of the state of vegetable. Pursuant thereof, when the vegetable is fully analysed
labels are given distinctive classes in the image by the use of unsupervised categorization. The
task is done in this research paper helps in enabling further understanding for the utilization of
band ratio as well as combination if highlighting characteristics in a picture like vegetable, water
as well soil and the formation of NDVI images and composite from these ratios contributes to the
analysis of vegetables.
References
For this paper, the when the picture is analysed first, every class is assigned in a specific colour
to make identification easier (Ferreira, 2019). By evaluation through the use of True colour
image, Green will show vegetable, Blue will water, yellow shows a bare land while Red shows
infrastructures or settlements. But in some cases there may be inconsistency which can arise due
to nature of the soil in the same location.
6. Conclusion
The research paper uses enhancement of the radiometric, band ratios and picture categories
in ENVI 5.4 program to help in the analysis of LANDSAT image in Melbourne city. This picture
is loaded in a software and through using different band ratios as well combinations, there is a
thorough analysis of the state of vegetable. Pursuant thereof, when the vegetable is fully analysed
labels are given distinctive classes in the image by the use of unsupervised categorization. The
task is done in this research paper helps in enabling further understanding for the utilization of
band ratio as well as combination if highlighting characteristics in a picture like vegetable, water
as well soil and the formation of NDVI images and composite from these ratios contributes to the
analysis of vegetables.
References
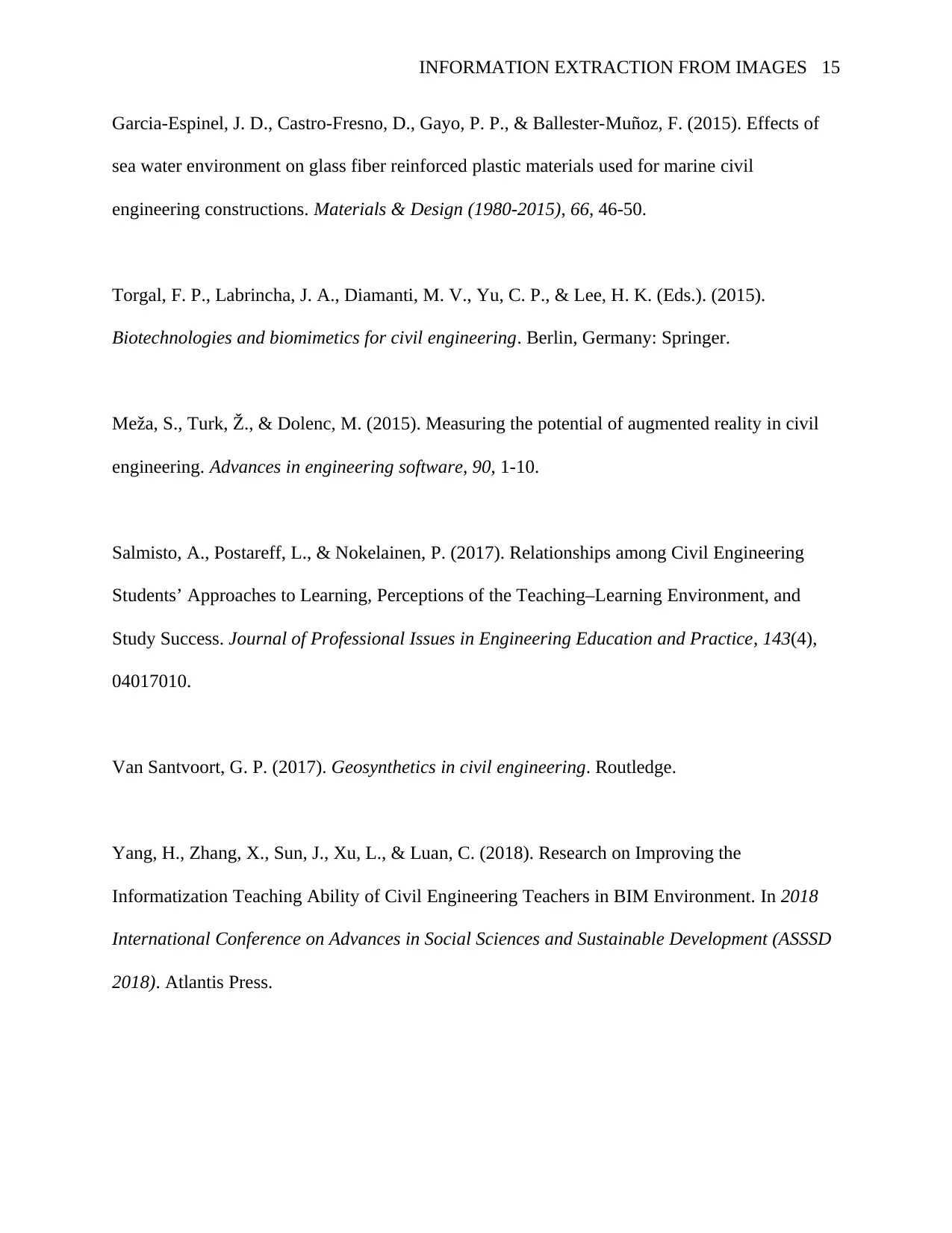
INFORMATION EXTRACTION FROM IMAGES 15
Garcia-Espinel, J. D., Castro-Fresno, D., Gayo, P. P., & Ballester-Muñoz, F. (2015). Effects of
sea water environment on glass fiber reinforced plastic materials used for marine civil
engineering constructions. Materials & Design (1980-2015), 66, 46-50.
Torgal, F. P., Labrincha, J. A., Diamanti, M. V., Yu, C. P., & Lee, H. K. (Eds.). (2015).
Biotechnologies and biomimetics for civil engineering. Berlin, Germany: Springer.
Meža, S., Turk, Ž., & Dolenc, M. (2015). Measuring the potential of augmented reality in civil
engineering. Advances in engineering software, 90, 1-10.
Salmisto, A., Postareff, L., & Nokelainen, P. (2017). Relationships among Civil Engineering
Students’ Approaches to Learning, Perceptions of the Teaching–Learning Environment, and
Study Success. Journal of Professional Issues in Engineering Education and Practice, 143(4),
04017010.
Van Santvoort, G. P. (2017). Geosynthetics in civil engineering. Routledge.
Yang, H., Zhang, X., Sun, J., Xu, L., & Luan, C. (2018). Research on Improving the
Informatization Teaching Ability of Civil Engineering Teachers in BIM Environment. In 2018
International Conference on Advances in Social Sciences and Sustainable Development (ASSSD
2018). Atlantis Press.
Garcia-Espinel, J. D., Castro-Fresno, D., Gayo, P. P., & Ballester-Muñoz, F. (2015). Effects of
sea water environment on glass fiber reinforced plastic materials used for marine civil
engineering constructions. Materials & Design (1980-2015), 66, 46-50.
Torgal, F. P., Labrincha, J. A., Diamanti, M. V., Yu, C. P., & Lee, H. K. (Eds.). (2015).
Biotechnologies and biomimetics for civil engineering. Berlin, Germany: Springer.
Meža, S., Turk, Ž., & Dolenc, M. (2015). Measuring the potential of augmented reality in civil
engineering. Advances in engineering software, 90, 1-10.
Salmisto, A., Postareff, L., & Nokelainen, P. (2017). Relationships among Civil Engineering
Students’ Approaches to Learning, Perceptions of the Teaching–Learning Environment, and
Study Success. Journal of Professional Issues in Engineering Education and Practice, 143(4),
04017010.
Van Santvoort, G. P. (2017). Geosynthetics in civil engineering. Routledge.
Yang, H., Zhang, X., Sun, J., Xu, L., & Luan, C. (2018). Research on Improving the
Informatization Teaching Ability of Civil Engineering Teachers in BIM Environment. In 2018
International Conference on Advances in Social Sciences and Sustainable Development (ASSSD
2018). Atlantis Press.
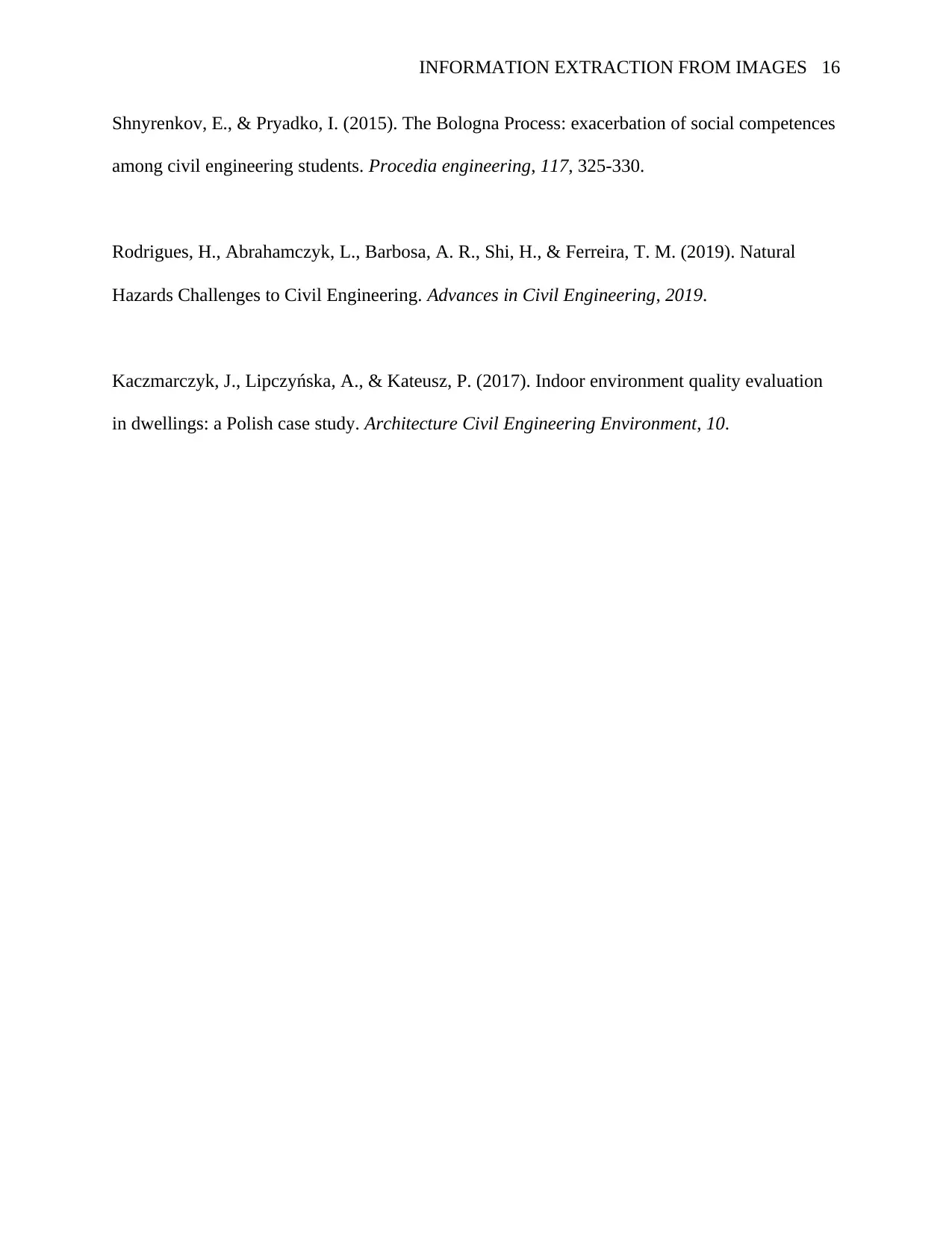
INFORMATION EXTRACTION FROM IMAGES 16
Shnyrenkov, E., & Pryadko, I. (2015). The Bologna Process: exacerbation of social competences
among civil engineering students. Procedia engineering, 117, 325-330.
Rodrigues, H., Abrahamczyk, L., Barbosa, A. R., Shi, H., & Ferreira, T. M. (2019). Natural
Hazards Challenges to Civil Engineering. Advances in Civil Engineering, 2019.
Kaczmarczyk, J., Lipczyńska, A., & Kateusz, P. (2017). Indoor environment quality evaluation
in dwellings: a Polish case study. Architecture Civil Engineering Environment, 10.
Shnyrenkov, E., & Pryadko, I. (2015). The Bologna Process: exacerbation of social competences
among civil engineering students. Procedia engineering, 117, 325-330.
Rodrigues, H., Abrahamczyk, L., Barbosa, A. R., Shi, H., & Ferreira, T. M. (2019). Natural
Hazards Challenges to Civil Engineering. Advances in Civil Engineering, 2019.
Kaczmarczyk, J., Lipczyńska, A., & Kateusz, P. (2017). Indoor environment quality evaluation
in dwellings: a Polish case study. Architecture Civil Engineering Environment, 10.
1 out of 16
![[object Object]](/_next/image/?url=%2F_next%2Fstatic%2Fmedia%2Flogo.6d15ce61.png&w=640&q=75)
Your All-in-One AI-Powered Toolkit for Academic Success.
+13062052269
info@desklib.com
Available 24*7 on WhatsApp / Email
Unlock your academic potential
© 2024 | Zucol Services PVT LTD | All rights reserved.