Intelligent Machines: Autonomous Driverless Cars, Deep Learning, Reinforcement Learning, 5G Technology
VerifiedAdded on 2023/01/12
|7
|1976
|63
AI Summary
This assignment explores the applications of autonomous driverless cars, the main components involved, the use of deep learning and reinforcement learning techniques, and the impact of 5G technology on driverless cars within smart cities and smart transportation.
Contribute Materials
Your contribution can guide someone’s learning journey. Share your
documents today.
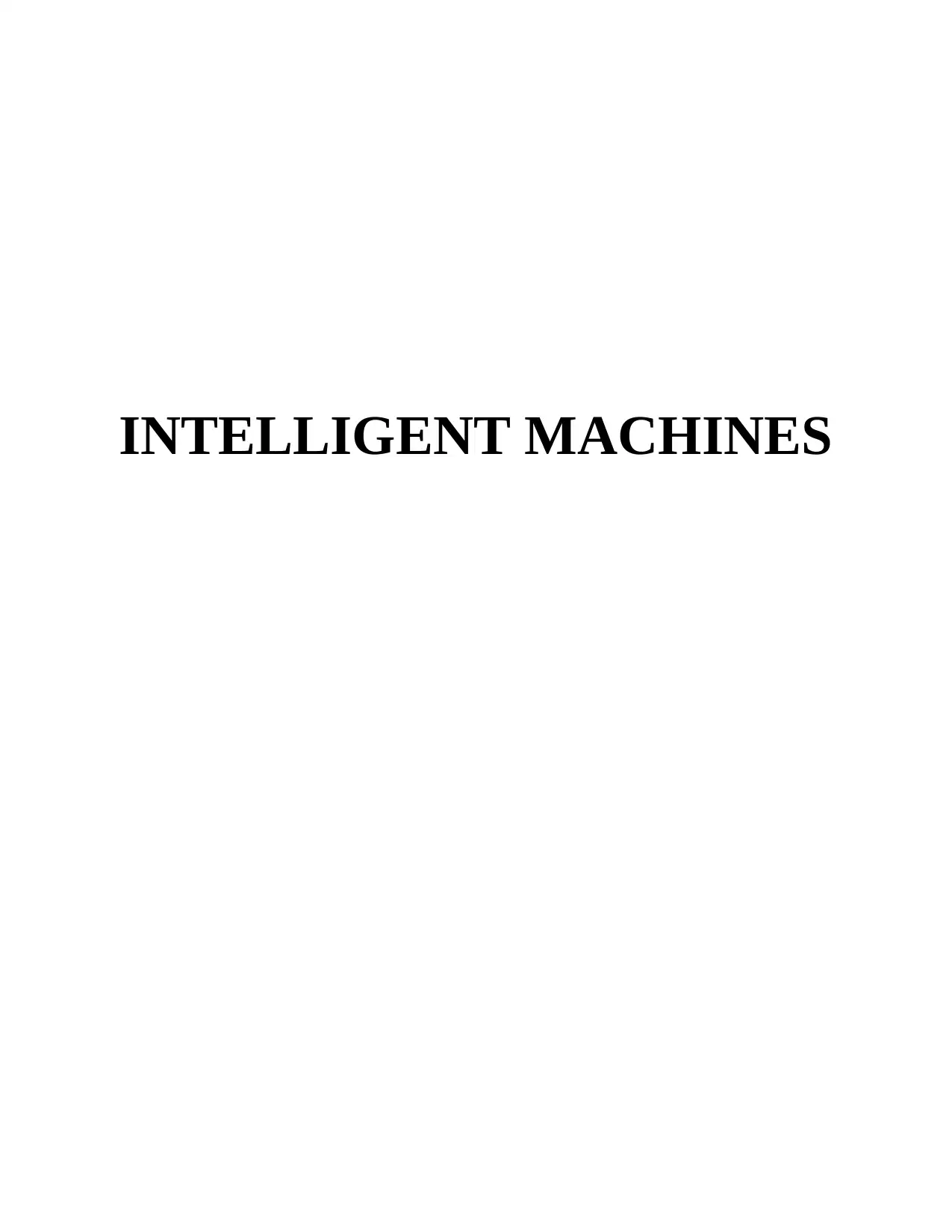
INTELLIGENT MACHINES
Secure Best Marks with AI Grader
Need help grading? Try our AI Grader for instant feedback on your assignments.
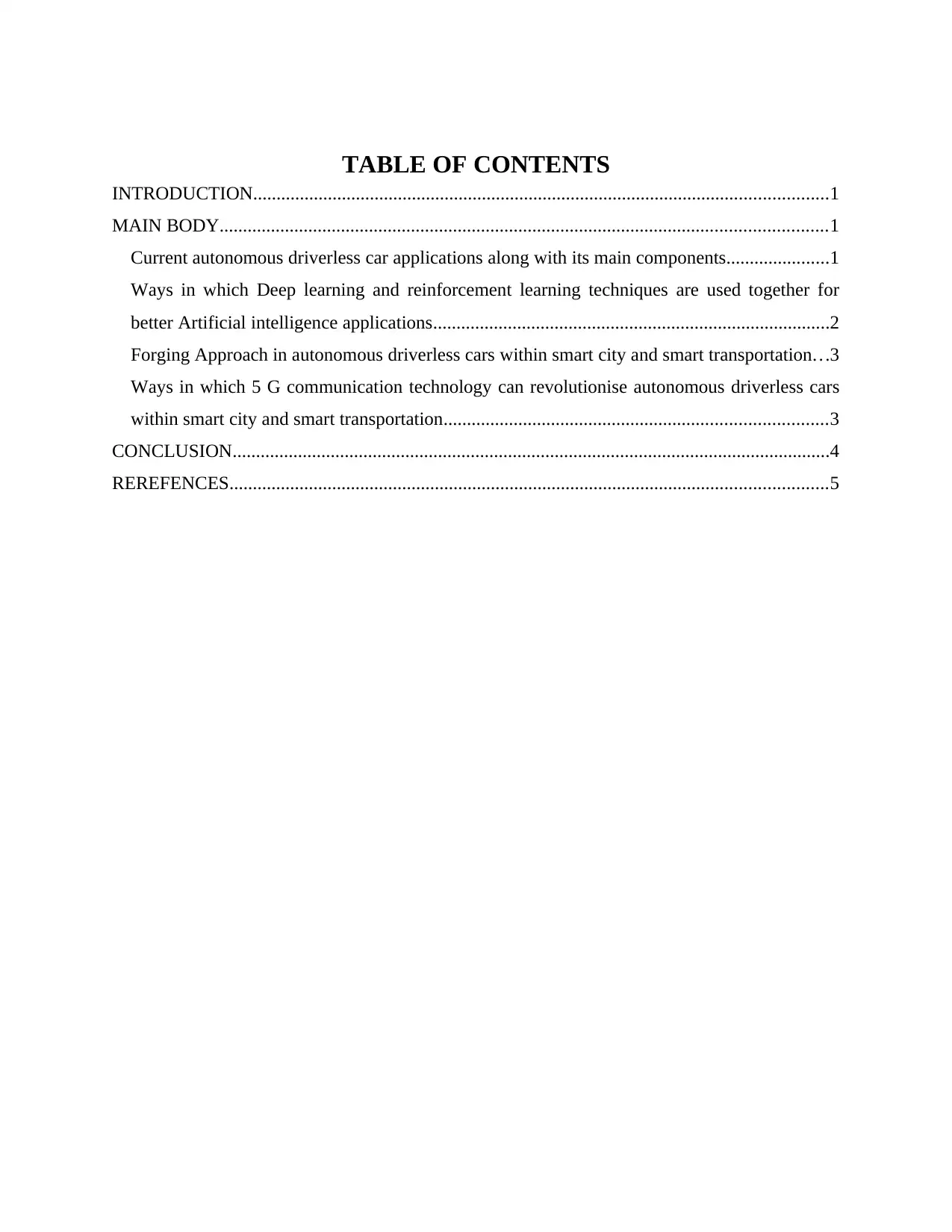
TABLE OF CONTENTS
INTRODUCTION...........................................................................................................................1
MAIN BODY..................................................................................................................................1
Current autonomous driverless car applications along with its main components......................1
Ways in which Deep learning and reinforcement learning techniques are used together for
better Artificial intelligence applications.....................................................................................2
Forging Approach in autonomous driverless cars within smart city and smart transportation...3
Ways in which 5 G communication technology can revolutionise autonomous driverless cars
within smart city and smart transportation..................................................................................3
CONCLUSION................................................................................................................................4
REREFENCES................................................................................................................................5
INTRODUCTION...........................................................................................................................1
MAIN BODY..................................................................................................................................1
Current autonomous driverless car applications along with its main components......................1
Ways in which Deep learning and reinforcement learning techniques are used together for
better Artificial intelligence applications.....................................................................................2
Forging Approach in autonomous driverless cars within smart city and smart transportation...3
Ways in which 5 G communication technology can revolutionise autonomous driverless cars
within smart city and smart transportation..................................................................................3
CONCLUSION................................................................................................................................4
REREFENCES................................................................................................................................5
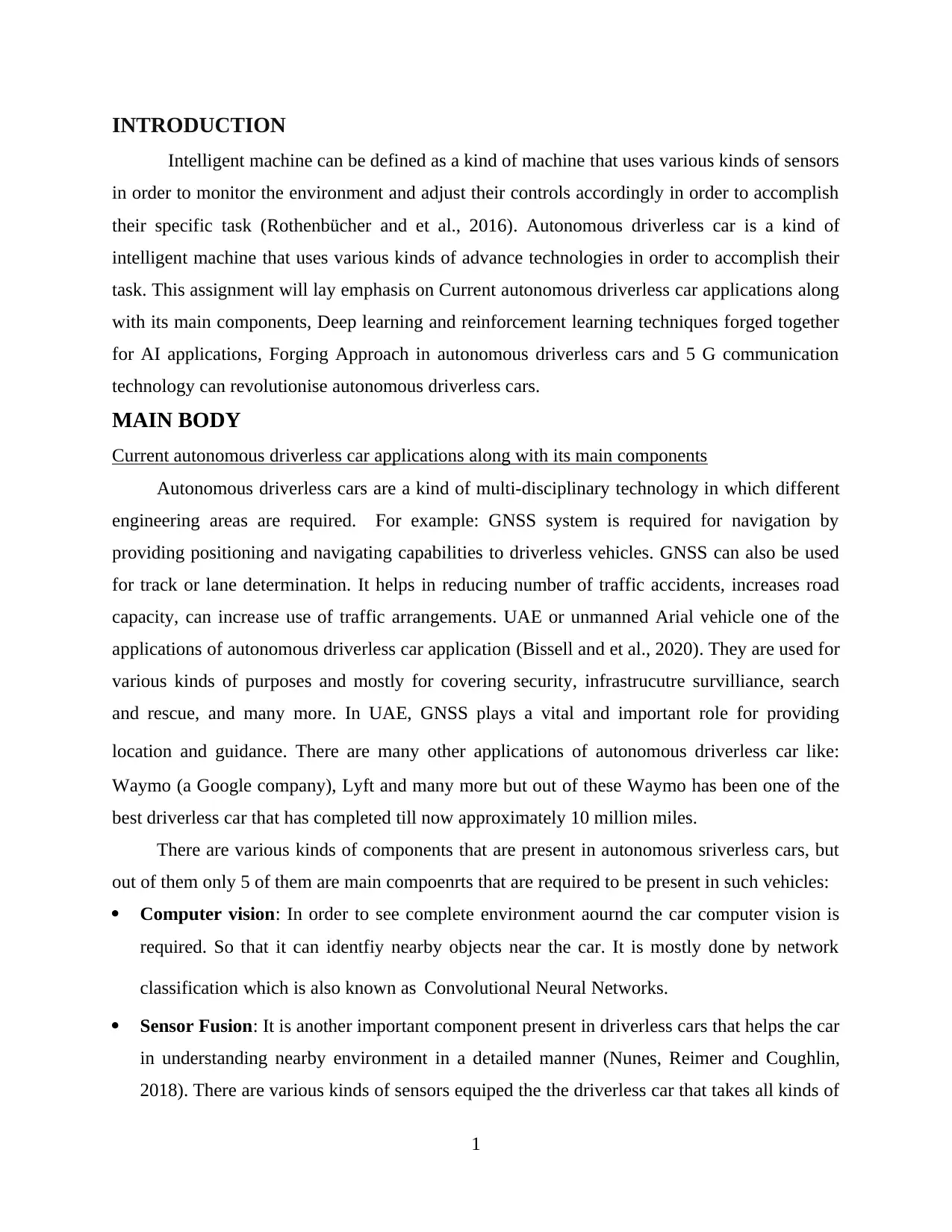
INTRODUCTION
Intelligent machine can be defined as a kind of machine that uses various kinds of sensors
in order to monitor the environment and adjust their controls accordingly in order to accomplish
their specific task (Rothenbücher and et al., 2016). Autonomous driverless car is a kind of
intelligent machine that uses various kinds of advance technologies in order to accomplish their
task. This assignment will lay emphasis on Current autonomous driverless car applications along
with its main components, Deep learning and reinforcement learning techniques forged together
for AI applications, Forging Approach in autonomous driverless cars and 5 G communication
technology can revolutionise autonomous driverless cars.
MAIN BODY
Current autonomous driverless car applications along with its main components
Autonomous driverless cars are a kind of multi-disciplinary technology in which different
engineering areas are required. For example: GNSS system is required for navigation by
providing positioning and navigating capabilities to driverless vehicles. GNSS can also be used
for track or lane determination. It helps in reducing number of traffic accidents, increases road
capacity, can increase use of traffic arrangements. UAE or unmanned Arial vehicle one of the
applications of autonomous driverless car application (Bissell and et al., 2020). They are used for
various kinds of purposes and mostly for covering security, infrastrucutre survilliance, search
and rescue, and many more. In UAE, GNSS plays a vital and important role for providing
location and guidance. There are many other applications of autonomous driverless car like:
Waymo (a Google company), Lyft and many more but out of these Waymo has been one of the
best driverless car that has completed till now approximately 10 million miles.
There are various kinds of components that are present in autonomous sriverless cars, but
out of them only 5 of them are main compoenrts that are required to be present in such vehicles:
Computer vision: In order to see complete environment aournd the car computer vision is
required. So that it can identfiy nearby objects near the car. It is mostly done by network
classification which is also known as Convolutional Neural Networks.
Sensor Fusion: It is another important component present in driverless cars that helps the car
in understanding nearby environment in a detailed manner (Nunes, Reimer and Coughlin,
2018). There are various kinds of sensors equiped the the driverless car that takes all kinds of
1
Intelligent machine can be defined as a kind of machine that uses various kinds of sensors
in order to monitor the environment and adjust their controls accordingly in order to accomplish
their specific task (Rothenbücher and et al., 2016). Autonomous driverless car is a kind of
intelligent machine that uses various kinds of advance technologies in order to accomplish their
task. This assignment will lay emphasis on Current autonomous driverless car applications along
with its main components, Deep learning and reinforcement learning techniques forged together
for AI applications, Forging Approach in autonomous driverless cars and 5 G communication
technology can revolutionise autonomous driverless cars.
MAIN BODY
Current autonomous driverless car applications along with its main components
Autonomous driverless cars are a kind of multi-disciplinary technology in which different
engineering areas are required. For example: GNSS system is required for navigation by
providing positioning and navigating capabilities to driverless vehicles. GNSS can also be used
for track or lane determination. It helps in reducing number of traffic accidents, increases road
capacity, can increase use of traffic arrangements. UAE or unmanned Arial vehicle one of the
applications of autonomous driverless car application (Bissell and et al., 2020). They are used for
various kinds of purposes and mostly for covering security, infrastrucutre survilliance, search
and rescue, and many more. In UAE, GNSS plays a vital and important role for providing
location and guidance. There are many other applications of autonomous driverless car like:
Waymo (a Google company), Lyft and many more but out of these Waymo has been one of the
best driverless car that has completed till now approximately 10 million miles.
There are various kinds of components that are present in autonomous sriverless cars, but
out of them only 5 of them are main compoenrts that are required to be present in such vehicles:
Computer vision: In order to see complete environment aournd the car computer vision is
required. So that it can identfiy nearby objects near the car. It is mostly done by network
classification which is also known as Convolutional Neural Networks.
Sensor Fusion: It is another important component present in driverless cars that helps the car
in understanding nearby environment in a detailed manner (Nunes, Reimer and Coughlin,
2018). There are various kinds of sensors equiped the the driverless car that takes all kinds of
1
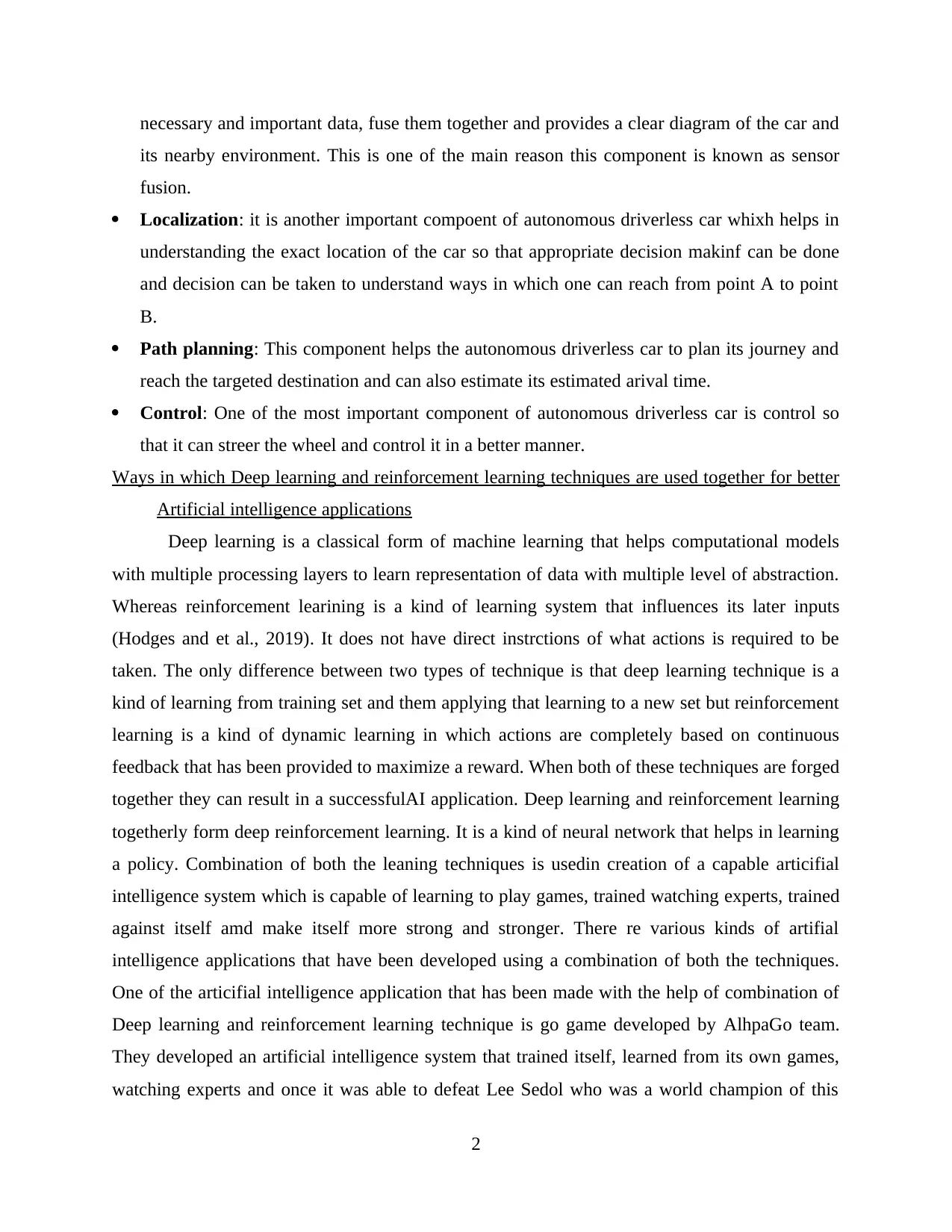
necessary and important data, fuse them together and provides a clear diagram of the car and
its nearby environment. This is one of the main reason this component is known as sensor
fusion.
Localization: it is another important compoent of autonomous driverless car whixh helps in
understanding the exact location of the car so that appropriate decision makinf can be done
and decision can be taken to understand ways in which one can reach from point A to point
B.
Path planning: This component helps the autonomous driverless car to plan its journey and
reach the targeted destination and can also estimate its estimated arival time.
Control: One of the most important component of autonomous driverless car is control so
that it can streer the wheel and control it in a better manner.
Ways in which Deep learning and reinforcement learning techniques are used together for better
Artificial intelligence applications
Deep learning is a classical form of machine learning that helps computational models
with multiple processing layers to learn representation of data with multiple level of abstraction.
Whereas reinforcement learining is a kind of learning system that influences its later inputs
(Hodges and et al., 2019). It does not have direct instrctions of what actions is required to be
taken. The only difference between two types of technique is that deep learning technique is a
kind of learning from training set and them applying that learning to a new set but reinforcement
learning is a kind of dynamic learning in which actions are completely based on continuous
feedback that has been provided to maximize a reward. When both of these techniques are forged
together they can result in a successfulAI application. Deep learning and reinforcement learning
togetherly form deep reinforcement learning. It is a kind of neural network that helps in learning
a policy. Combination of both the leaning techniques is usedin creation of a capable articifial
intelligence system which is capable of learning to play games, trained watching experts, trained
against itself amd make itself more strong and stronger. There re various kinds of artifial
intelligence applications that have been developed using a combination of both the techniques.
One of the articifial intelligence application that has been made with the help of combination of
Deep learning and reinforcement learning technique is go game developed by AlhpaGo team.
They developed an artificial intelligence system that trained itself, learned from its own games,
watching experts and once it was able to defeat Lee Sedol who was a world champion of this
2
its nearby environment. This is one of the main reason this component is known as sensor
fusion.
Localization: it is another important compoent of autonomous driverless car whixh helps in
understanding the exact location of the car so that appropriate decision makinf can be done
and decision can be taken to understand ways in which one can reach from point A to point
B.
Path planning: This component helps the autonomous driverless car to plan its journey and
reach the targeted destination and can also estimate its estimated arival time.
Control: One of the most important component of autonomous driverless car is control so
that it can streer the wheel and control it in a better manner.
Ways in which Deep learning and reinforcement learning techniques are used together for better
Artificial intelligence applications
Deep learning is a classical form of machine learning that helps computational models
with multiple processing layers to learn representation of data with multiple level of abstraction.
Whereas reinforcement learining is a kind of learning system that influences its later inputs
(Hodges and et al., 2019). It does not have direct instrctions of what actions is required to be
taken. The only difference between two types of technique is that deep learning technique is a
kind of learning from training set and them applying that learning to a new set but reinforcement
learning is a kind of dynamic learning in which actions are completely based on continuous
feedback that has been provided to maximize a reward. When both of these techniques are forged
together they can result in a successfulAI application. Deep learning and reinforcement learning
togetherly form deep reinforcement learning. It is a kind of neural network that helps in learning
a policy. Combination of both the leaning techniques is usedin creation of a capable articifial
intelligence system which is capable of learning to play games, trained watching experts, trained
against itself amd make itself more strong and stronger. There re various kinds of artifial
intelligence applications that have been developed using a combination of both the techniques.
One of the articifial intelligence application that has been made with the help of combination of
Deep learning and reinforcement learning technique is go game developed by AlhpaGo team.
They developed an artificial intelligence system that trained itself, learned from its own games,
watching experts and once it was able to defeat Lee Sedol who was a world champion of this
2
Paraphrase This Document
Need a fresh take? Get an instant paraphrase of this document with our AI Paraphraser
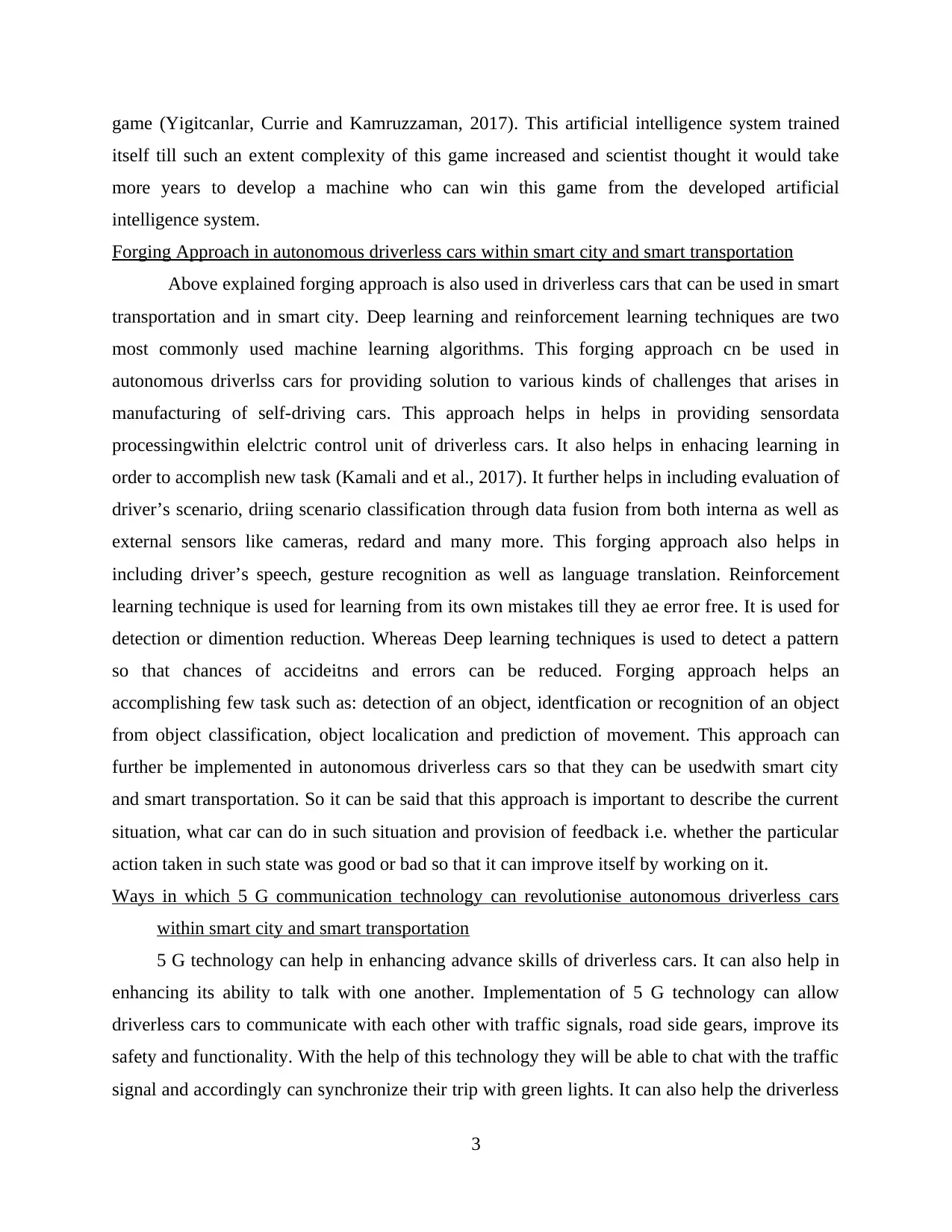
game (Yigitcanlar, Currie and Kamruzzaman, 2017). This artificial intelligence system trained
itself till such an extent complexity of this game increased and scientist thought it would take
more years to develop a machine who can win this game from the developed artificial
intelligence system.
Forging Approach in autonomous driverless cars within smart city and smart transportation
Above explained forging approach is also used in driverless cars that can be used in smart
transportation and in smart city. Deep learning and reinforcement learning techniques are two
most commonly used machine learning algorithms. This forging approach cn be used in
autonomous driverlss cars for providing solution to various kinds of challenges that arises in
manufacturing of self-driving cars. This approach helps in helps in providing sensordata
processingwithin elelctric control unit of driverless cars. It also helps in enhacing learning in
order to accomplish new task (Kamali and et al., 2017). It further helps in including evaluation of
driver’s scenario, driing scenario classification through data fusion from both interna as well as
external sensors like cameras, redard and many more. This forging approach also helps in
including driver’s speech, gesture recognition as well as language translation. Reinforcement
learning technique is used for learning from its own mistakes till they ae error free. It is used for
detection or dimention reduction. Whereas Deep learning techniques is used to detect a pattern
so that chances of accideitns and errors can be reduced. Forging approach helps an
accomplishing few task such as: detection of an object, identfication or recognition of an object
from object classification, object localication and prediction of movement. This approach can
further be implemented in autonomous driverless cars so that they can be usedwith smart city
and smart transportation. So it can be said that this approach is important to describe the current
situation, what car can do in such situation and provision of feedback i.e. whether the particular
action taken in such state was good or bad so that it can improve itself by working on it.
Ways in which 5 G communication technology can revolutionise autonomous driverless cars
within smart city and smart transportation
5 G technology can help in enhancing advance skills of driverless cars. It can also help in
enhancing its ability to talk with one another. Implementation of 5 G technology can allow
driverless cars to communicate with each other with traffic signals, road side gears, improve its
safety and functionality. With the help of this technology they will be able to chat with the traffic
signal and accordingly can synchronize their trip with green lights. It can also help the driverless
3
itself till such an extent complexity of this game increased and scientist thought it would take
more years to develop a machine who can win this game from the developed artificial
intelligence system.
Forging Approach in autonomous driverless cars within smart city and smart transportation
Above explained forging approach is also used in driverless cars that can be used in smart
transportation and in smart city. Deep learning and reinforcement learning techniques are two
most commonly used machine learning algorithms. This forging approach cn be used in
autonomous driverlss cars for providing solution to various kinds of challenges that arises in
manufacturing of self-driving cars. This approach helps in helps in providing sensordata
processingwithin elelctric control unit of driverless cars. It also helps in enhacing learning in
order to accomplish new task (Kamali and et al., 2017). It further helps in including evaluation of
driver’s scenario, driing scenario classification through data fusion from both interna as well as
external sensors like cameras, redard and many more. This forging approach also helps in
including driver’s speech, gesture recognition as well as language translation. Reinforcement
learning technique is used for learning from its own mistakes till they ae error free. It is used for
detection or dimention reduction. Whereas Deep learning techniques is used to detect a pattern
so that chances of accideitns and errors can be reduced. Forging approach helps an
accomplishing few task such as: detection of an object, identfication or recognition of an object
from object classification, object localication and prediction of movement. This approach can
further be implemented in autonomous driverless cars so that they can be usedwith smart city
and smart transportation. So it can be said that this approach is important to describe the current
situation, what car can do in such situation and provision of feedback i.e. whether the particular
action taken in such state was good or bad so that it can improve itself by working on it.
Ways in which 5 G communication technology can revolutionise autonomous driverless cars
within smart city and smart transportation
5 G technology can help in enhancing advance skills of driverless cars. It can also help in
enhancing its ability to talk with one another. Implementation of 5 G technology can allow
driverless cars to communicate with each other with traffic signals, road side gears, improve its
safety and functionality. With the help of this technology they will be able to chat with the traffic
signal and accordingly can synchronize their trip with green lights. It can also help the driverless
3
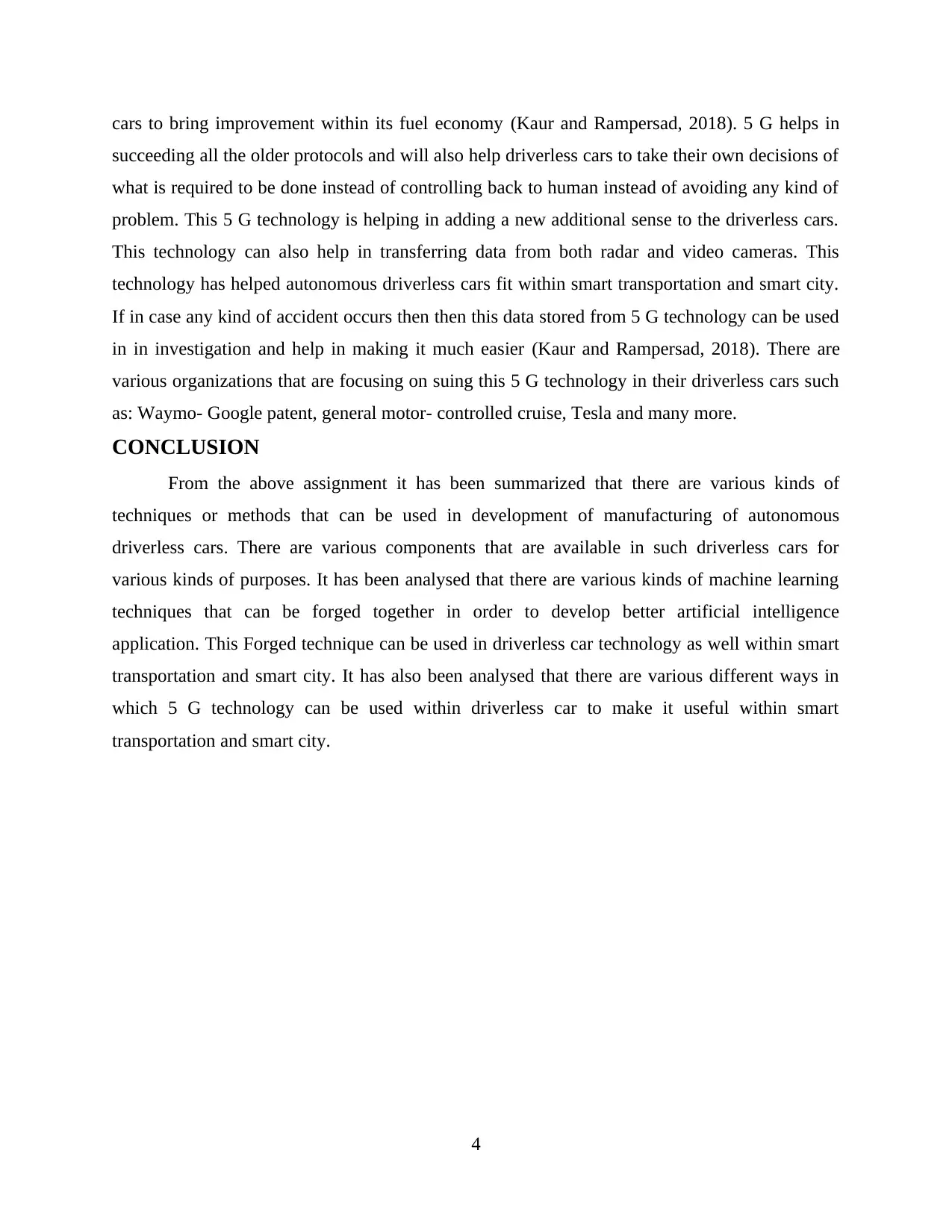
cars to bring improvement within its fuel economy (Kaur and Rampersad, 2018). 5 G helps in
succeeding all the older protocols and will also help driverless cars to take their own decisions of
what is required to be done instead of controlling back to human instead of avoiding any kind of
problem. This 5 G technology is helping in adding a new additional sense to the driverless cars.
This technology can also help in transferring data from both radar and video cameras. This
technology has helped autonomous driverless cars fit within smart transportation and smart city.
If in case any kind of accident occurs then then this data stored from 5 G technology can be used
in in investigation and help in making it much easier (Kaur and Rampersad, 2018). There are
various organizations that are focusing on suing this 5 G technology in their driverless cars such
as: Waymo- Google patent, general motor- controlled cruise, Tesla and many more.
CONCLUSION
From the above assignment it has been summarized that there are various kinds of
techniques or methods that can be used in development of manufacturing of autonomous
driverless cars. There are various components that are available in such driverless cars for
various kinds of purposes. It has been analysed that there are various kinds of machine learning
techniques that can be forged together in order to develop better artificial intelligence
application. This Forged technique can be used in driverless car technology as well within smart
transportation and smart city. It has also been analysed that there are various different ways in
which 5 G technology can be used within driverless car to make it useful within smart
transportation and smart city.
4
succeeding all the older protocols and will also help driverless cars to take their own decisions of
what is required to be done instead of controlling back to human instead of avoiding any kind of
problem. This 5 G technology is helping in adding a new additional sense to the driverless cars.
This technology can also help in transferring data from both radar and video cameras. This
technology has helped autonomous driverless cars fit within smart transportation and smart city.
If in case any kind of accident occurs then then this data stored from 5 G technology can be used
in in investigation and help in making it much easier (Kaur and Rampersad, 2018). There are
various organizations that are focusing on suing this 5 G technology in their driverless cars such
as: Waymo- Google patent, general motor- controlled cruise, Tesla and many more.
CONCLUSION
From the above assignment it has been summarized that there are various kinds of
techniques or methods that can be used in development of manufacturing of autonomous
driverless cars. There are various components that are available in such driverless cars for
various kinds of purposes. It has been analysed that there are various kinds of machine learning
techniques that can be forged together in order to develop better artificial intelligence
application. This Forged technique can be used in driverless car technology as well within smart
transportation and smart city. It has also been analysed that there are various different ways in
which 5 G technology can be used within driverless car to make it useful within smart
transportation and smart city.
4
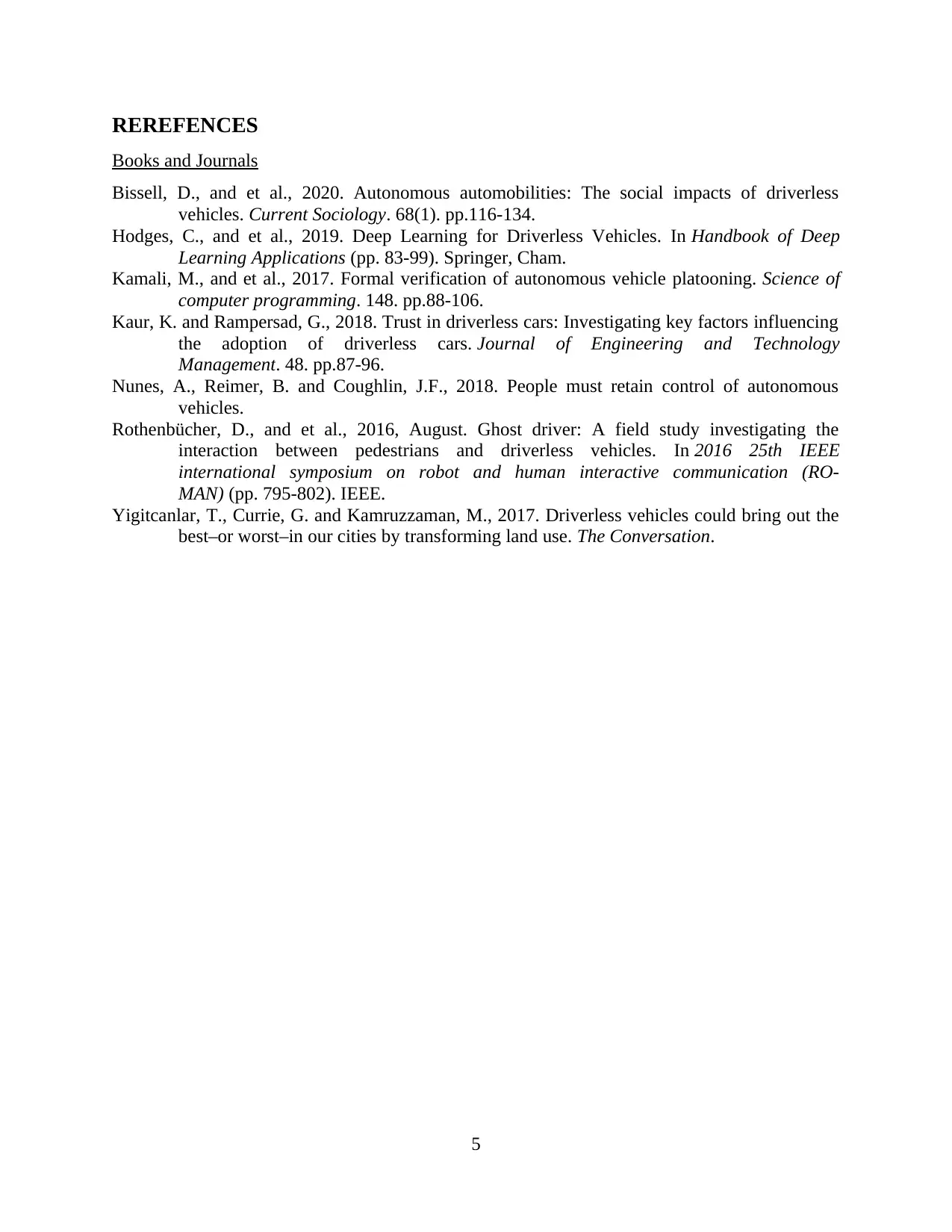
REREFENCES
Books and Journals
Bissell, D., and et al., 2020. Autonomous automobilities: The social impacts of driverless
vehicles. Current Sociology. 68(1). pp.116-134.
Hodges, C., and et al., 2019. Deep Learning for Driverless Vehicles. In Handbook of Deep
Learning Applications (pp. 83-99). Springer, Cham.
Kamali, M., and et al., 2017. Formal verification of autonomous vehicle platooning. Science of
computer programming. 148. pp.88-106.
Kaur, K. and Rampersad, G., 2018. Trust in driverless cars: Investigating key factors influencing
the adoption of driverless cars. Journal of Engineering and Technology
Management. 48. pp.87-96.
Nunes, A., Reimer, B. and Coughlin, J.F., 2018. People must retain control of autonomous
vehicles.
Rothenbücher, D., and et al., 2016, August. Ghost driver: A field study investigating the
interaction between pedestrians and driverless vehicles. In 2016 25th IEEE
international symposium on robot and human interactive communication (RO-
MAN) (pp. 795-802). IEEE.
Yigitcanlar, T., Currie, G. and Kamruzzaman, M., 2017. Driverless vehicles could bring out the
best–or worst–in our cities by transforming land use. The Conversation.
5
Books and Journals
Bissell, D., and et al., 2020. Autonomous automobilities: The social impacts of driverless
vehicles. Current Sociology. 68(1). pp.116-134.
Hodges, C., and et al., 2019. Deep Learning for Driverless Vehicles. In Handbook of Deep
Learning Applications (pp. 83-99). Springer, Cham.
Kamali, M., and et al., 2017. Formal verification of autonomous vehicle platooning. Science of
computer programming. 148. pp.88-106.
Kaur, K. and Rampersad, G., 2018. Trust in driverless cars: Investigating key factors influencing
the adoption of driverless cars. Journal of Engineering and Technology
Management. 48. pp.87-96.
Nunes, A., Reimer, B. and Coughlin, J.F., 2018. People must retain control of autonomous
vehicles.
Rothenbücher, D., and et al., 2016, August. Ghost driver: A field study investigating the
interaction between pedestrians and driverless vehicles. In 2016 25th IEEE
international symposium on robot and human interactive communication (RO-
MAN) (pp. 795-802). IEEE.
Yigitcanlar, T., Currie, G. and Kamruzzaman, M., 2017. Driverless vehicles could bring out the
best–or worst–in our cities by transforming land use. The Conversation.
5
1 out of 7
![[object Object]](/_next/image/?url=%2F_next%2Fstatic%2Fmedia%2Flogo.6d15ce61.png&w=640&q=75)
Your All-in-One AI-Powered Toolkit for Academic Success.
+13062052269
info@desklib.com
Available 24*7 on WhatsApp / Email
Unlock your academic potential
© 2024 | Zucol Services PVT LTD | All rights reserved.