Investigating Flow Characteristics within Powells Creek using Storm Water Management Model
VerifiedAdded on 2022/11/18
|8
|1715
|393
AI Summary
This report investigates the flow characteristics within Powells Creek using Storm Water Management Model. The report evaluates the output of the model and records data, and discusses methods of improving its efficiency and output.
Contribute Materials
Your contribution can guide someone’s learning journey. Share your
documents today.
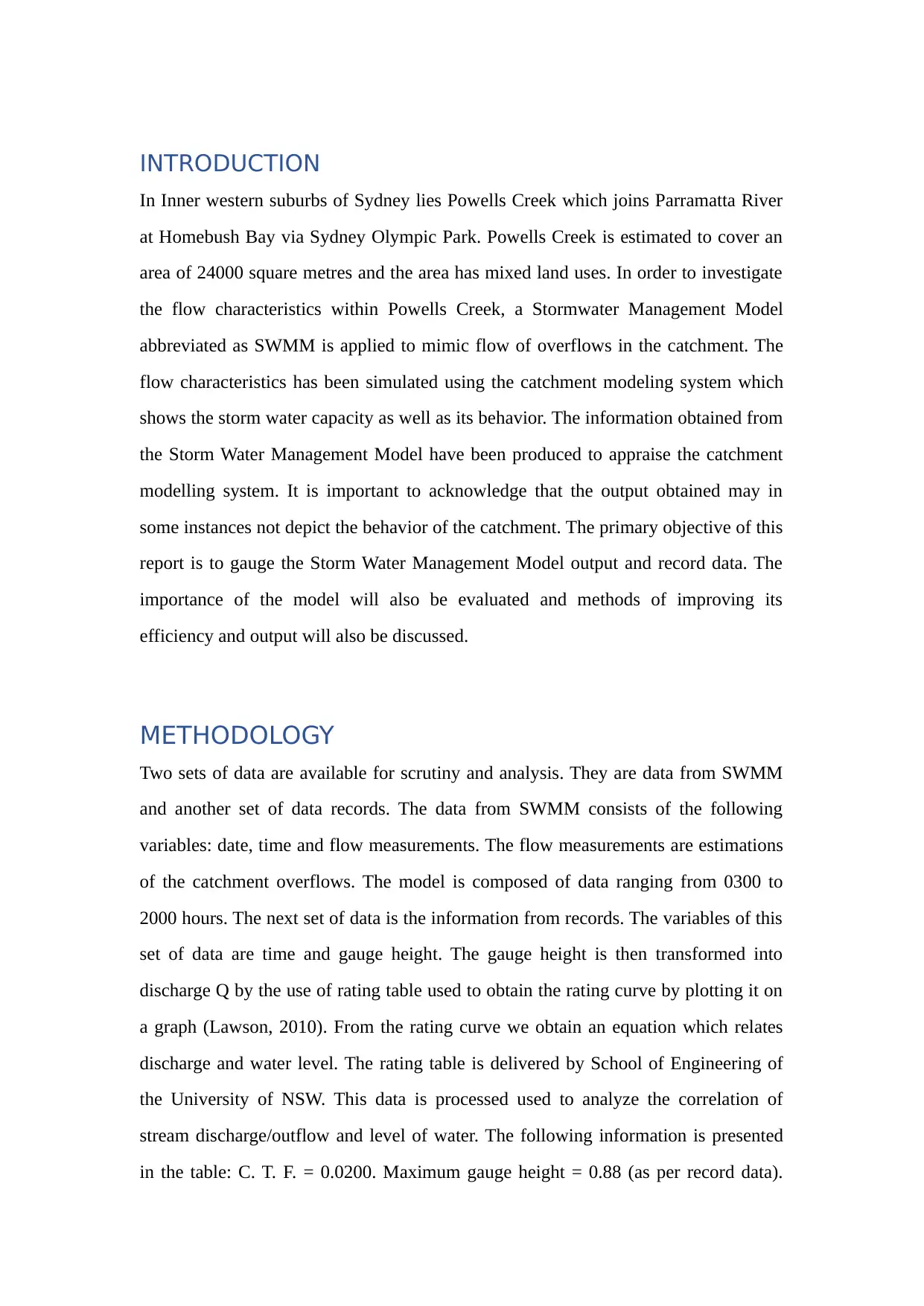
INTRODUCTION
In Inner western suburbs of Sydney lies Powells Creek which joins Parramatta River
at Homebush Bay via Sydney Olympic Park. Powells Creek is estimated to cover an
area of 24000 square metres and the area has mixed land uses. In order to investigate
the flow characteristics within Powells Creek, a Stormwater Management Model
abbreviated as SWMM is applied to mimic flow of overflows in the catchment. The
flow characteristics has been simulated using the catchment modeling system which
shows the storm water capacity as well as its behavior. The information obtained from
the Storm Water Management Model have been produced to appraise the catchment
modelling system. It is important to acknowledge that the output obtained may in
some instances not depict the behavior of the catchment. The primary objective of this
report is to gauge the Storm Water Management Model output and record data. The
importance of the model will also be evaluated and methods of improving its
efficiency and output will also be discussed.
METHODOLOGY
Two sets of data are available for scrutiny and analysis. They are data from SWMM
and another set of data records. The data from SWMM consists of the following
variables: date, time and flow measurements. The flow measurements are estimations
of the catchment overflows. The model is composed of data ranging from 0300 to
2000 hours. The next set of data is the information from records. The variables of this
set of data are time and gauge height. The gauge height is then transformed into
discharge Q by the use of rating table used to obtain the rating curve by plotting it on
a graph (Lawson, 2010). From the rating curve we obtain an equation which relates
discharge and water level. The rating table is delivered by School of Engineering of
the University of NSW. This data is processed used to analyze the correlation of
stream discharge/outflow and level of water. The following information is presented
in the table: C. T. F. = 0.0200. Maximum gauge height = 0.88 (as per record data).
In Inner western suburbs of Sydney lies Powells Creek which joins Parramatta River
at Homebush Bay via Sydney Olympic Park. Powells Creek is estimated to cover an
area of 24000 square metres and the area has mixed land uses. In order to investigate
the flow characteristics within Powells Creek, a Stormwater Management Model
abbreviated as SWMM is applied to mimic flow of overflows in the catchment. The
flow characteristics has been simulated using the catchment modeling system which
shows the storm water capacity as well as its behavior. The information obtained from
the Storm Water Management Model have been produced to appraise the catchment
modelling system. It is important to acknowledge that the output obtained may in
some instances not depict the behavior of the catchment. The primary objective of this
report is to gauge the Storm Water Management Model output and record data. The
importance of the model will also be evaluated and methods of improving its
efficiency and output will also be discussed.
METHODOLOGY
Two sets of data are available for scrutiny and analysis. They are data from SWMM
and another set of data records. The data from SWMM consists of the following
variables: date, time and flow measurements. The flow measurements are estimations
of the catchment overflows. The model is composed of data ranging from 0300 to
2000 hours. The next set of data is the information from records. The variables of this
set of data are time and gauge height. The gauge height is then transformed into
discharge Q by the use of rating table used to obtain the rating curve by plotting it on
a graph (Lawson, 2010). From the rating curve we obtain an equation which relates
discharge and water level. The rating table is delivered by School of Engineering of
the University of NSW. This data is processed used to analyze the correlation of
stream discharge/outflow and level of water. The following information is presented
in the table: C. T. F. = 0.0200. Maximum gauge height = 0.88 (as per record data).
Secure Best Marks with AI Grader
Need help grading? Try our AI Grader for instant feedback on your assignments.
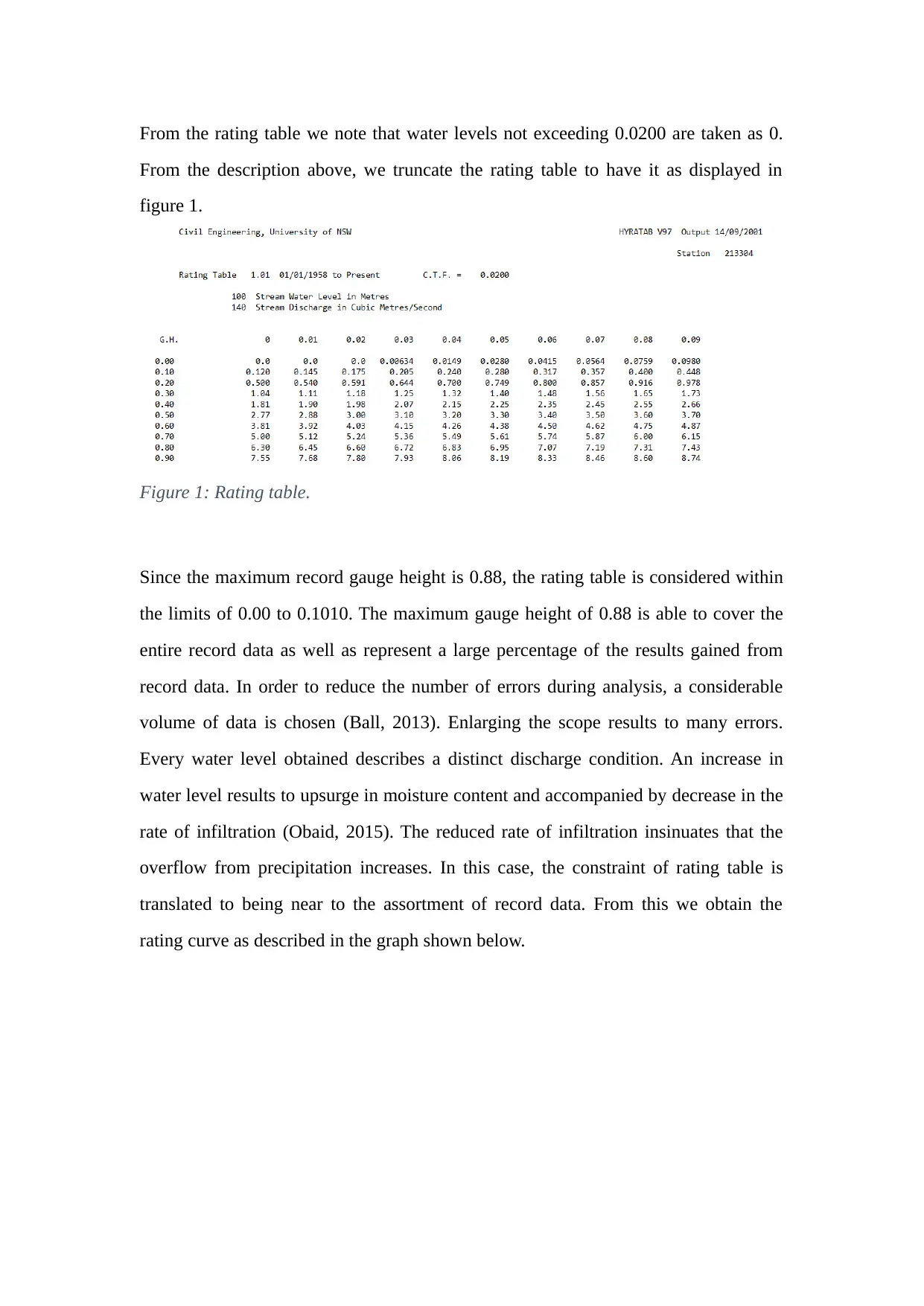
From the rating table we note that water levels not exceeding 0.0200 are taken as 0.
From the description above, we truncate the rating table to have it as displayed in
figure 1.
Figure 1: Rating table.
Since the maximum record gauge height is 0.88, the rating table is considered within
the limits of 0.00 to 0.1010. The maximum gauge height of 0.88 is able to cover the
entire record data as well as represent a large percentage of the results gained from
record data. In order to reduce the number of errors during analysis, a considerable
volume of data is chosen (Ball, 2013). Enlarging the scope results to many errors.
Every water level obtained describes a distinct discharge condition. An increase in
water level results to upsurge in moisture content and accompanied by decrease in the
rate of infiltration (Obaid, 2015). The reduced rate of infiltration insinuates that the
overflow from precipitation increases. In this case, the constraint of rating table is
translated to being near to the assortment of record data. From this we obtain the
rating curve as described in the graph shown below.
From the description above, we truncate the rating table to have it as displayed in
figure 1.
Figure 1: Rating table.
Since the maximum record gauge height is 0.88, the rating table is considered within
the limits of 0.00 to 0.1010. The maximum gauge height of 0.88 is able to cover the
entire record data as well as represent a large percentage of the results gained from
record data. In order to reduce the number of errors during analysis, a considerable
volume of data is chosen (Ball, 2013). Enlarging the scope results to many errors.
Every water level obtained describes a distinct discharge condition. An increase in
water level results to upsurge in moisture content and accompanied by decrease in the
rate of infiltration (Obaid, 2015). The reduced rate of infiltration insinuates that the
overflow from precipitation increases. In this case, the constraint of rating table is
translated to being near to the assortment of record data. From this we obtain the
rating curve as described in the graph shown below.
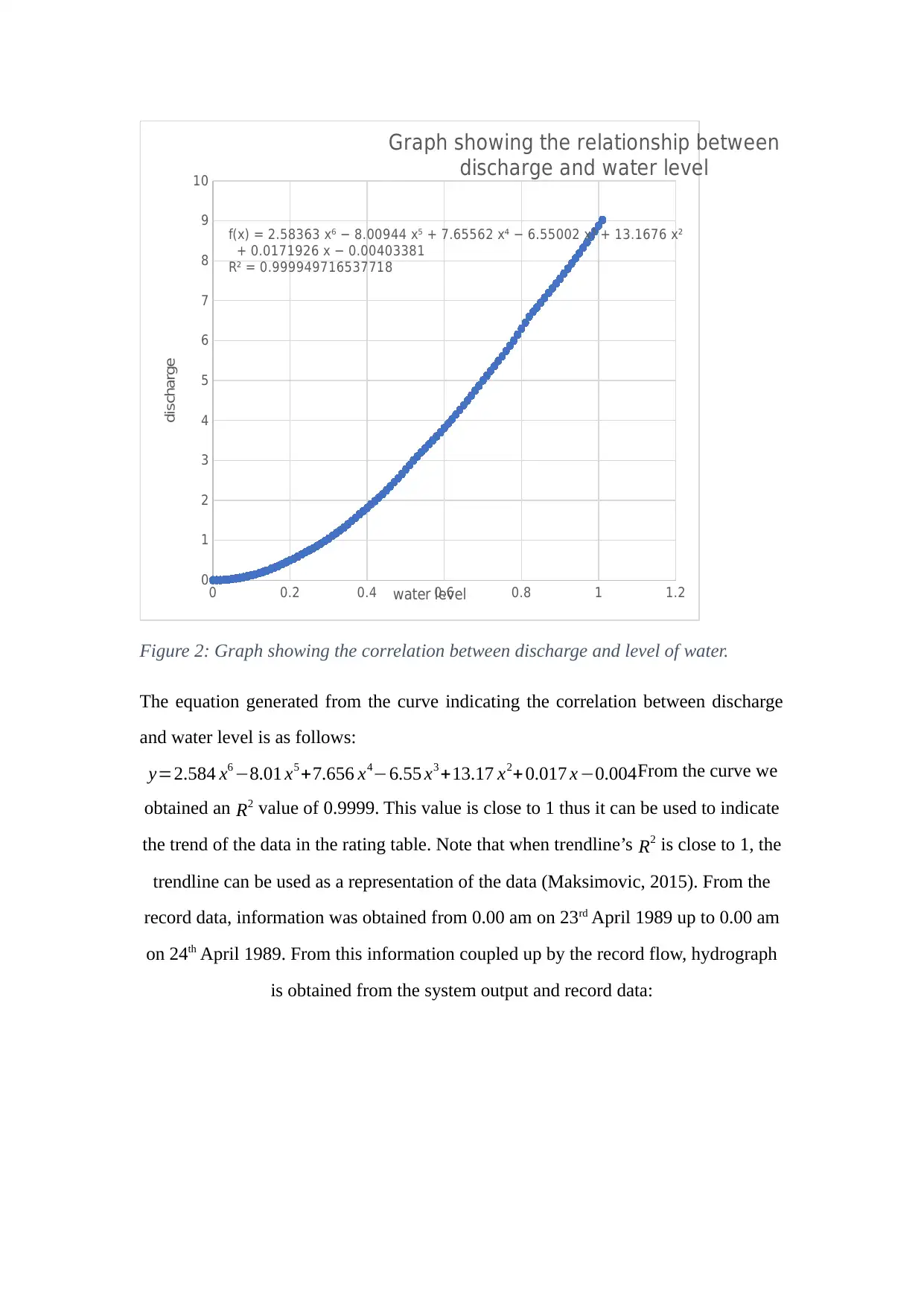
0 0.2 0.4 0.6 0.8 1 1.2
0
1
2
3
4
5
6
7
8
9
10
f(x) = 2.58363 x⁶ − 8.00944 x⁵ + 7.65562 x⁴ − 6.55002 x³ + 13.1676 x²
+ 0.0171926 x − 0.00403381
R² = 0.999949716537718
Graph showing the relationship between
discharge and water level
water level
discharge
Figure 2: Graph showing the correlation between discharge and level of water.
The equation generated from the curve indicating the correlation between discharge
and water level is as follows:
y=2.584 x6 −8.01 x5 +7.656 x4−6.55 x3 +13.17 x2+ 0.017 x −0.004From the curve we
obtained an R2 value of 0.9999. This value is close to 1 thus it can be used to indicate
the trend of the data in the rating table. Note that when trendline’s R2 is close to 1, the
trendline can be used as a representation of the data (Maksimovic, 2015). From the
record data, information was obtained from 0.00 am on 23rd April 1989 up to 0.00 am
on 24th April 1989. From this information coupled up by the record flow, hydrograph
is obtained from the system output and record data:
0
1
2
3
4
5
6
7
8
9
10
f(x) = 2.58363 x⁶ − 8.00944 x⁵ + 7.65562 x⁴ − 6.55002 x³ + 13.1676 x²
+ 0.0171926 x − 0.00403381
R² = 0.999949716537718
Graph showing the relationship between
discharge and water level
water level
discharge
Figure 2: Graph showing the correlation between discharge and level of water.
The equation generated from the curve indicating the correlation between discharge
and water level is as follows:
y=2.584 x6 −8.01 x5 +7.656 x4−6.55 x3 +13.17 x2+ 0.017 x −0.004From the curve we
obtained an R2 value of 0.9999. This value is close to 1 thus it can be used to indicate
the trend of the data in the rating table. Note that when trendline’s R2 is close to 1, the
trendline can be used as a representation of the data (Maksimovic, 2015). From the
record data, information was obtained from 0.00 am on 23rd April 1989 up to 0.00 am
on 24th April 1989. From this information coupled up by the record flow, hydrograph
is obtained from the system output and record data:
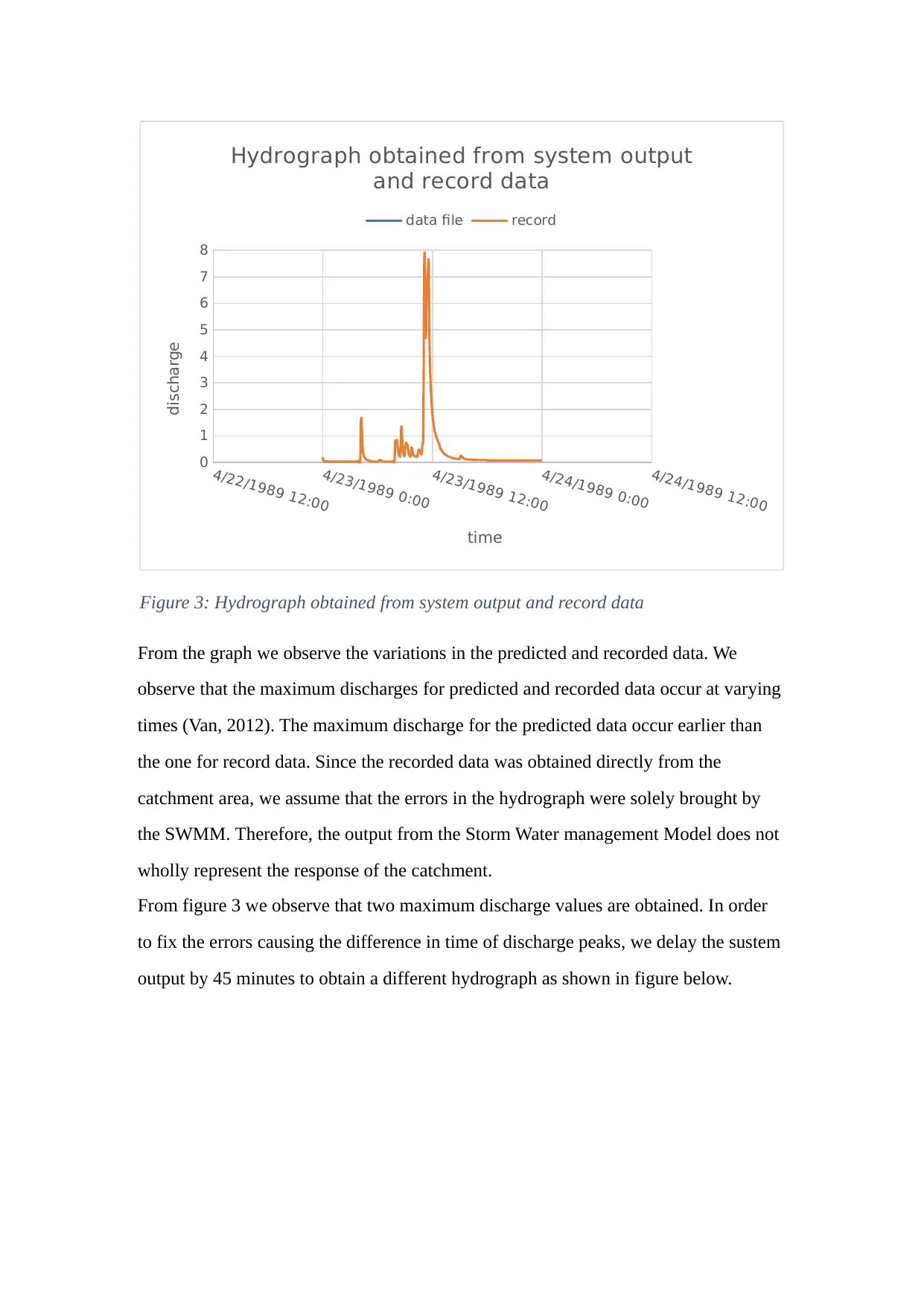
4/22/1989 12:00
4/23/1989 0:00
4/23/1989 12:00
4/24/1989 0:00
4/24/1989 12:00
0
1
2
3
4
5
6
7
8
Hydrograph obtained from system output
and record data
data file record
time
discharge
Figure 3: Hydrograph obtained from system output and record data
From the graph we observe the variations in the predicted and recorded data. We
observe that the maximum discharges for predicted and recorded data occur at varying
times (Van, 2012). The maximum discharge for the predicted data occur earlier than
the one for record data. Since the recorded data was obtained directly from the
catchment area, we assume that the errors in the hydrograph were solely brought by
the SWMM. Therefore, the output from the Storm Water management Model does not
wholly represent the response of the catchment.
From figure 3 we observe that two maximum discharge values are obtained. In order
to fix the errors causing the difference in time of discharge peaks, we delay the sustem
output by 45 minutes to obtain a different hydrograph as shown in figure below.
4/23/1989 0:00
4/23/1989 12:00
4/24/1989 0:00
4/24/1989 12:00
0
1
2
3
4
5
6
7
8
Hydrograph obtained from system output
and record data
data file record
time
discharge
Figure 3: Hydrograph obtained from system output and record data
From the graph we observe the variations in the predicted and recorded data. We
observe that the maximum discharges for predicted and recorded data occur at varying
times (Van, 2012). The maximum discharge for the predicted data occur earlier than
the one for record data. Since the recorded data was obtained directly from the
catchment area, we assume that the errors in the hydrograph were solely brought by
the SWMM. Therefore, the output from the Storm Water management Model does not
wholly represent the response of the catchment.
From figure 3 we observe that two maximum discharge values are obtained. In order
to fix the errors causing the difference in time of discharge peaks, we delay the sustem
output by 45 minutes to obtain a different hydrograph as shown in figure below.
Secure Best Marks with AI Grader
Need help grading? Try our AI Grader for instant feedback on your assignments.
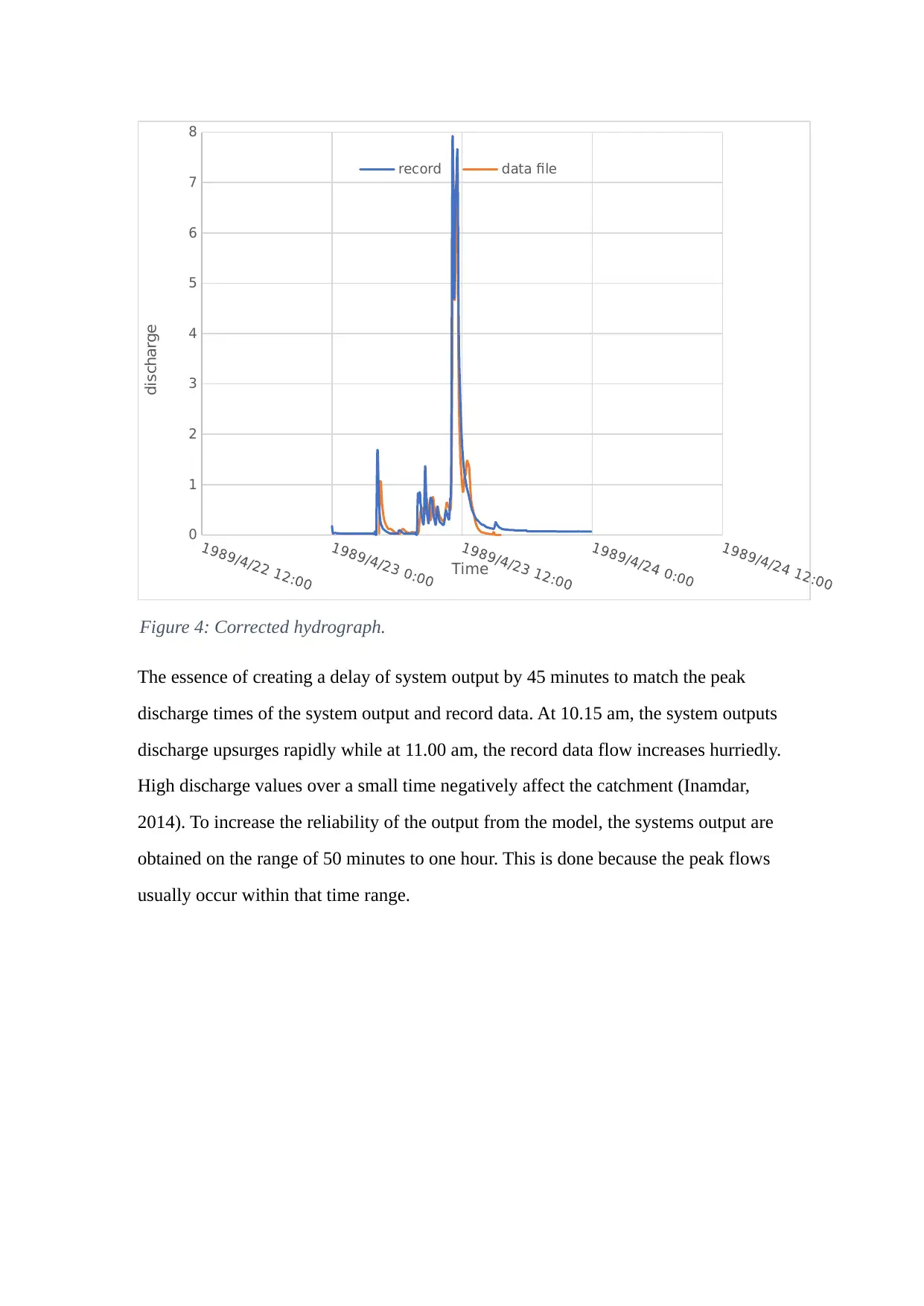
0
1
2
3
4
5
6
7
8
record data file
Time
discharge
Figure 4: Corrected hydrograph.
The essence of creating a delay of system output by 45 minutes to match the peak
discharge times of the system output and record data. At 10.15 am, the system outputs
discharge upsurges rapidly while at 11.00 am, the record data flow increases hurriedly.
High discharge values over a small time negatively affect the catchment (Inamdar,
2014). To increase the reliability of the output from the model, the systems output are
obtained on the range of 50 minutes to one hour. This is done because the peak flows
usually occur within that time range.
1
2
3
4
5
6
7
8
record data file
Time
discharge
Figure 4: Corrected hydrograph.
The essence of creating a delay of system output by 45 minutes to match the peak
discharge times of the system output and record data. At 10.15 am, the system outputs
discharge upsurges rapidly while at 11.00 am, the record data flow increases hurriedly.
High discharge values over a small time negatively affect the catchment (Inamdar,
2014). To increase the reliability of the output from the model, the systems output are
obtained on the range of 50 minutes to one hour. This is done because the peak flows
usually occur within that time range.
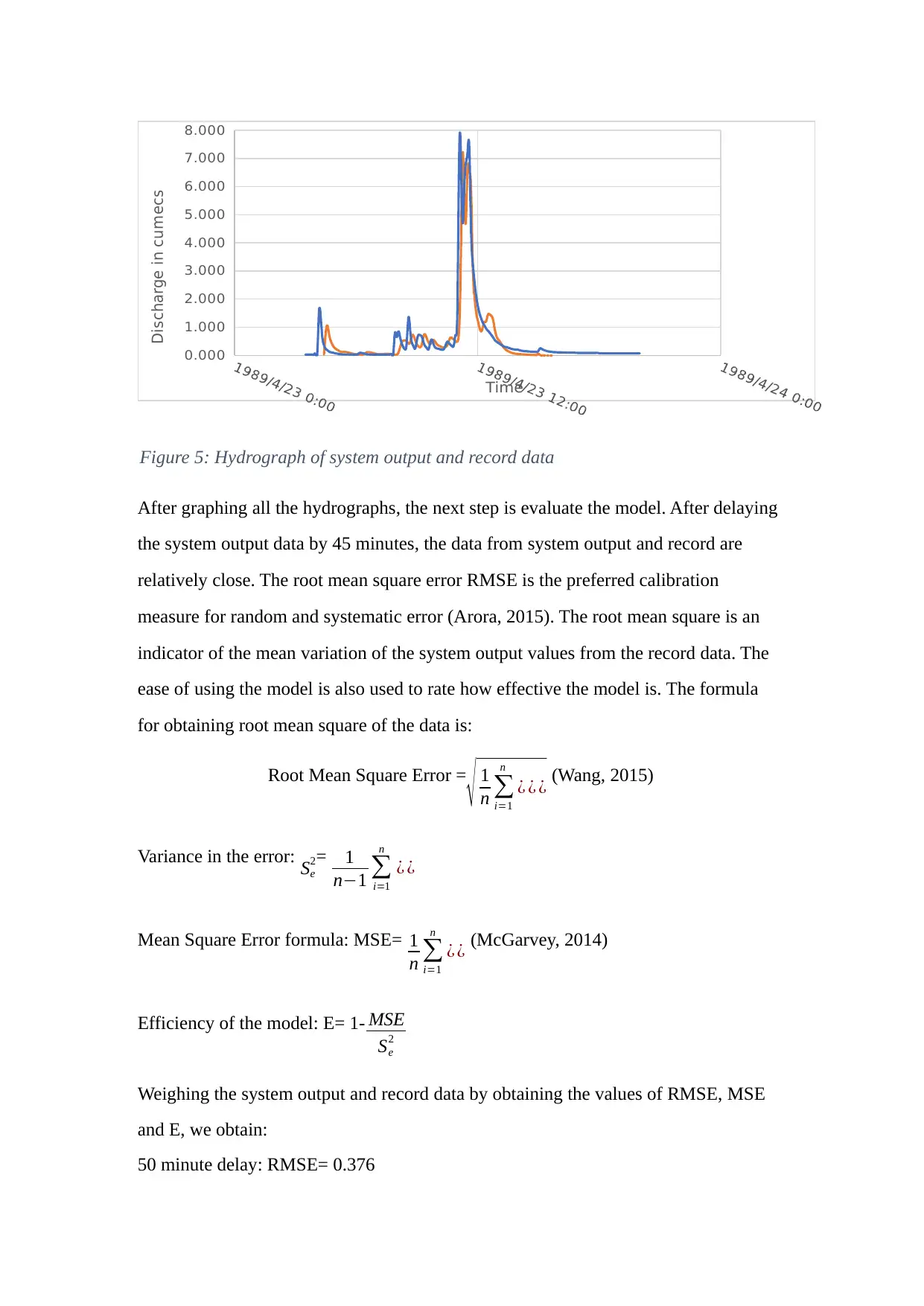
0.000
1.000
2.000
3.000
4.000
5.000
6.000
7.000
8.000
Time
Discharge in cumecs
Figure 5: Hydrograph of system output and record data
After graphing all the hydrographs, the next step is evaluate the model. After delaying
the system output data by 45 minutes, the data from system output and record are
relatively close. The root mean square error RMSE is the preferred calibration
measure for random and systematic error (Arora, 2015). The root mean square is an
indicator of the mean variation of the system output values from the record data. The
ease of using the model is also used to rate how effective the model is. The formula
for obtaining root mean square of the data is:
Root Mean Square Error =
√ 1
n ∑
i=1
n
¿ ¿ ¿ (Wang, 2015)
Variance in the error: Se
2= 1
n−1 ∑
i=1
n
¿ ¿
Mean Square Error formula: MSE= 1
n ∑
i=1
n
¿ ¿ (McGarvey, 2014)
Efficiency of the model: E= 1- MSE
Se
2
Weighing the system output and record data by obtaining the values of RMSE, MSE
and E, we obtain:
50 minute delay: RMSE= 0.376
1.000
2.000
3.000
4.000
5.000
6.000
7.000
8.000
Time
Discharge in cumecs
Figure 5: Hydrograph of system output and record data
After graphing all the hydrographs, the next step is evaluate the model. After delaying
the system output data by 45 minutes, the data from system output and record are
relatively close. The root mean square error RMSE is the preferred calibration
measure for random and systematic error (Arora, 2015). The root mean square is an
indicator of the mean variation of the system output values from the record data. The
ease of using the model is also used to rate how effective the model is. The formula
for obtaining root mean square of the data is:
Root Mean Square Error =
√ 1
n ∑
i=1
n
¿ ¿ ¿ (Wang, 2015)
Variance in the error: Se
2= 1
n−1 ∑
i=1
n
¿ ¿
Mean Square Error formula: MSE= 1
n ∑
i=1
n
¿ ¿ (McGarvey, 2014)
Efficiency of the model: E= 1- MSE
Se
2
Weighing the system output and record data by obtaining the values of RMSE, MSE
and E, we obtain:
50 minute delay: RMSE= 0.376
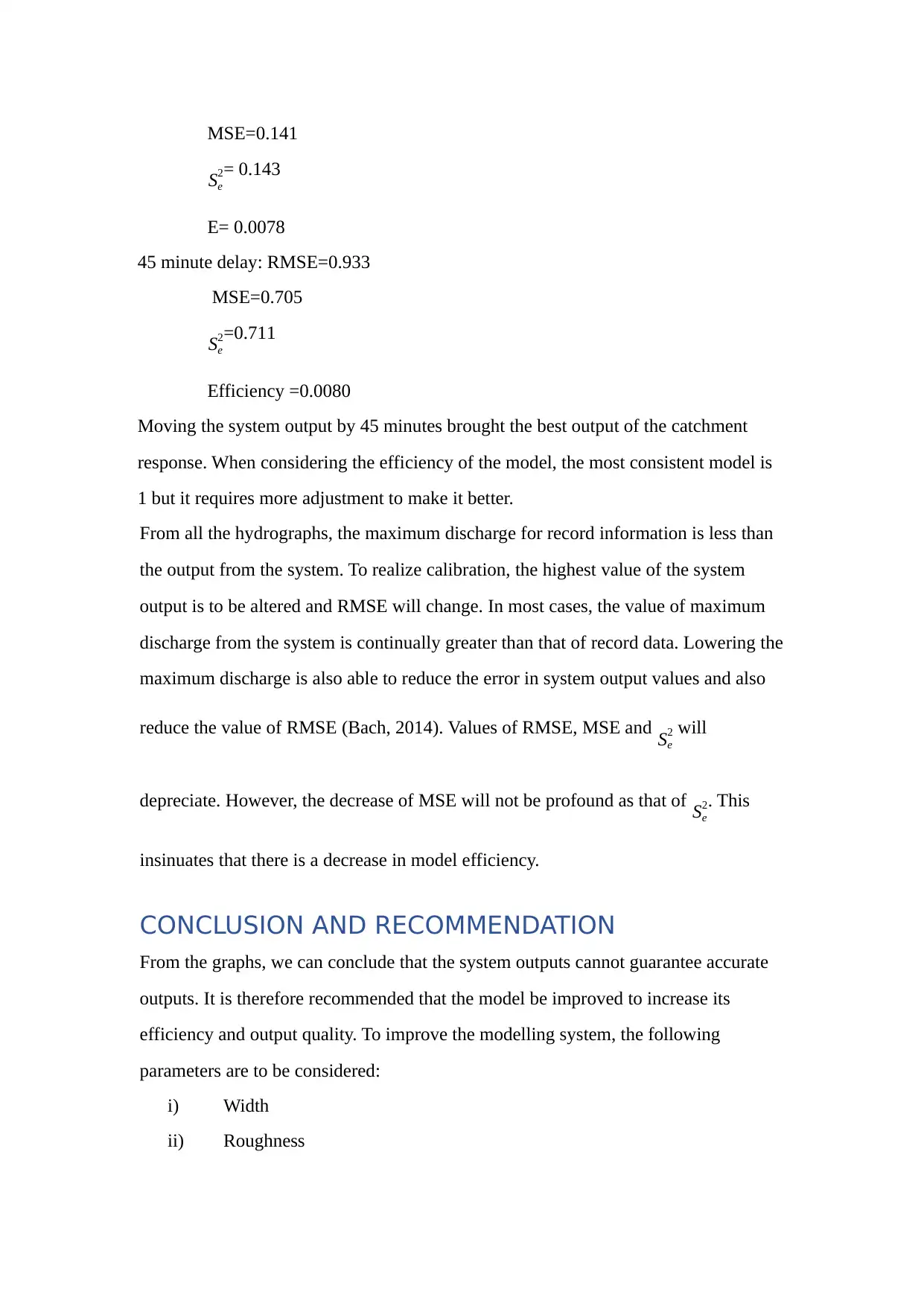
MSE=0.141
Se
2= 0.143
E= 0.0078
45 minute delay: RMSE=0.933
MSE=0.705
Se
2=0.711
Efficiency =0.0080
Moving the system output by 45 minutes brought the best output of the catchment
response. When considering the efficiency of the model, the most consistent model is
1 but it requires more adjustment to make it better.
From all the hydrographs, the maximum discharge for record information is less than
the output from the system. To realize calibration, the highest value of the system
output is to be altered and RMSE will change. In most cases, the value of maximum
discharge from the system is continually greater than that of record data. Lowering the
maximum discharge is also able to reduce the error in system output values and also
reduce the value of RMSE (Bach, 2014). Values of RMSE, MSE and Se
2 will
depreciate. However, the decrease of MSE will not be profound as that of Se
2. This
insinuates that there is a decrease in model efficiency.
CONCLUSION AND RECOMMENDATION
From the graphs, we can conclude that the system outputs cannot guarantee accurate
outputs. It is therefore recommended that the model be improved to increase its
efficiency and output quality. To improve the modelling system, the following
parameters are to be considered:
i) Width
ii) Roughness
Se
2= 0.143
E= 0.0078
45 minute delay: RMSE=0.933
MSE=0.705
Se
2=0.711
Efficiency =0.0080
Moving the system output by 45 minutes brought the best output of the catchment
response. When considering the efficiency of the model, the most consistent model is
1 but it requires more adjustment to make it better.
From all the hydrographs, the maximum discharge for record information is less than
the output from the system. To realize calibration, the highest value of the system
output is to be altered and RMSE will change. In most cases, the value of maximum
discharge from the system is continually greater than that of record data. Lowering the
maximum discharge is also able to reduce the error in system output values and also
reduce the value of RMSE (Bach, 2014). Values of RMSE, MSE and Se
2 will
depreciate. However, the decrease of MSE will not be profound as that of Se
2. This
insinuates that there is a decrease in model efficiency.
CONCLUSION AND RECOMMENDATION
From the graphs, we can conclude that the system outputs cannot guarantee accurate
outputs. It is therefore recommended that the model be improved to increase its
efficiency and output quality. To improve the modelling system, the following
parameters are to be considered:
i) Width
ii) Roughness
Paraphrase This Document
Need a fresh take? Get an instant paraphrase of this document with our AI Paraphraser
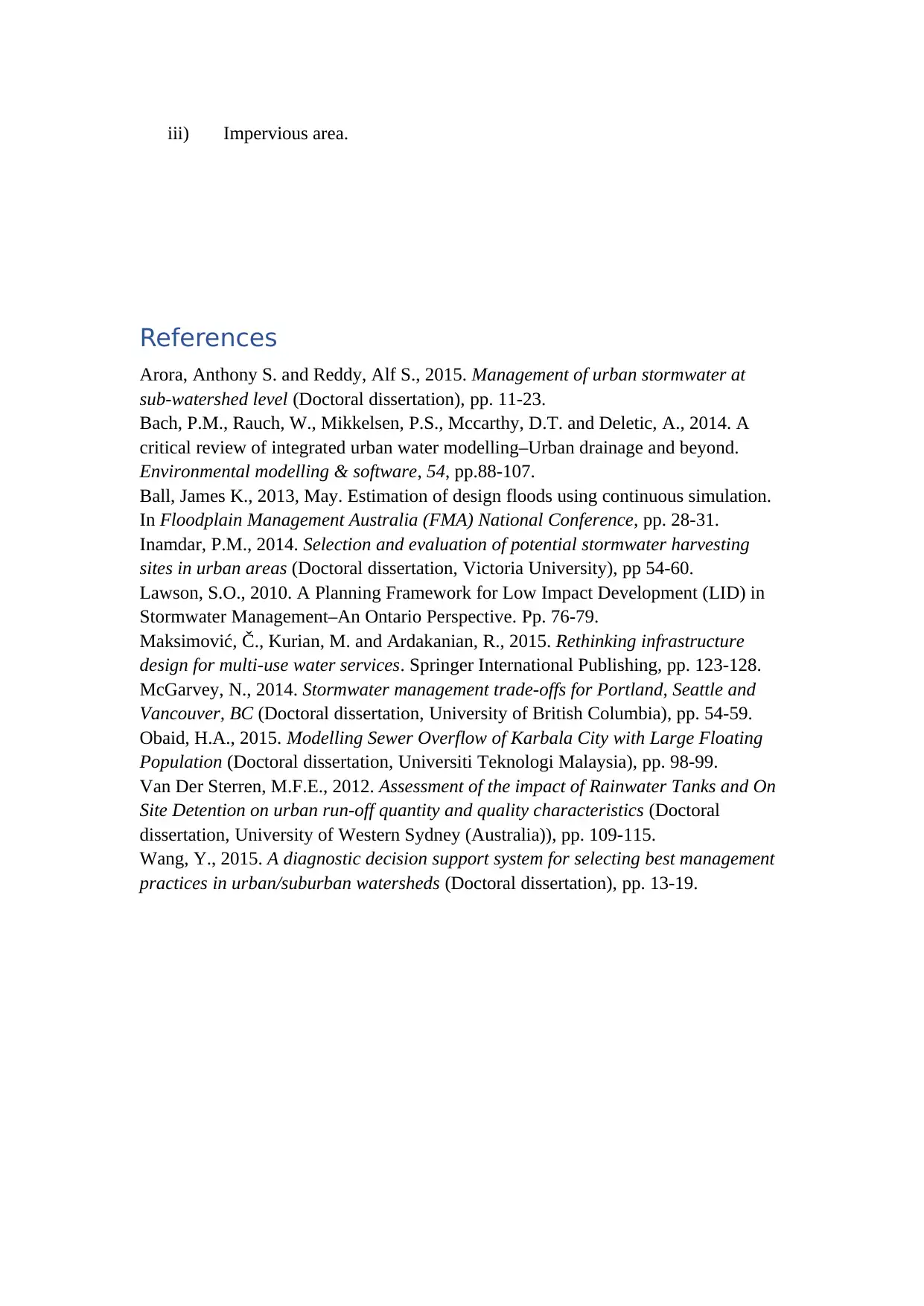
iii) Impervious area.
References
Arora, Anthony S. and Reddy, Alf S., 2015. Management of urban stormwater at
sub-watershed level (Doctoral dissertation), pp. 11-23.
Bach, P.M., Rauch, W., Mikkelsen, P.S., Mccarthy, D.T. and Deletic, A., 2014. A
critical review of integrated urban water modelling–Urban drainage and beyond.
Environmental modelling & software, 54, pp.88-107.
Ball, James K., 2013, May. Estimation of design floods using continuous simulation.
In Floodplain Management Australia (FMA) National Conference, pp. 28-31.
Inamdar, P.M., 2014. Selection and evaluation of potential stormwater harvesting
sites in urban areas (Doctoral dissertation, Victoria University), pp 54-60.
Lawson, S.O., 2010. A Planning Framework for Low Impact Development (LID) in
Stormwater Management–An Ontario Perspective. Pp. 76-79.
Maksimović, Č., Kurian, M. and Ardakanian, R., 2015. Rethinking infrastructure
design for multi-use water services. Springer International Publishing, pp. 123-128.
McGarvey, N., 2014. Stormwater management trade-offs for Portland, Seattle and
Vancouver, BC (Doctoral dissertation, University of British Columbia), pp. 54-59.
Obaid, H.A., 2015. Modelling Sewer Overflow of Karbala City with Large Floating
Population (Doctoral dissertation, Universiti Teknologi Malaysia), pp. 98-99.
Van Der Sterren, M.F.E., 2012. Assessment of the impact of Rainwater Tanks and On
Site Detention on urban run-off quantity and quality characteristics (Doctoral
dissertation, University of Western Sydney (Australia)), pp. 109-115.
Wang, Y., 2015. A diagnostic decision support system for selecting best management
practices in urban/suburban watersheds (Doctoral dissertation), pp. 13-19.
References
Arora, Anthony S. and Reddy, Alf S., 2015. Management of urban stormwater at
sub-watershed level (Doctoral dissertation), pp. 11-23.
Bach, P.M., Rauch, W., Mikkelsen, P.S., Mccarthy, D.T. and Deletic, A., 2014. A
critical review of integrated urban water modelling–Urban drainage and beyond.
Environmental modelling & software, 54, pp.88-107.
Ball, James K., 2013, May. Estimation of design floods using continuous simulation.
In Floodplain Management Australia (FMA) National Conference, pp. 28-31.
Inamdar, P.M., 2014. Selection and evaluation of potential stormwater harvesting
sites in urban areas (Doctoral dissertation, Victoria University), pp 54-60.
Lawson, S.O., 2010. A Planning Framework for Low Impact Development (LID) in
Stormwater Management–An Ontario Perspective. Pp. 76-79.
Maksimović, Č., Kurian, M. and Ardakanian, R., 2015. Rethinking infrastructure
design for multi-use water services. Springer International Publishing, pp. 123-128.
McGarvey, N., 2014. Stormwater management trade-offs for Portland, Seattle and
Vancouver, BC (Doctoral dissertation, University of British Columbia), pp. 54-59.
Obaid, H.A., 2015. Modelling Sewer Overflow of Karbala City with Large Floating
Population (Doctoral dissertation, Universiti Teknologi Malaysia), pp. 98-99.
Van Der Sterren, M.F.E., 2012. Assessment of the impact of Rainwater Tanks and On
Site Detention on urban run-off quantity and quality characteristics (Doctoral
dissertation, University of Western Sydney (Australia)), pp. 109-115.
Wang, Y., 2015. A diagnostic decision support system for selecting best management
practices in urban/suburban watersheds (Doctoral dissertation), pp. 13-19.
1 out of 8
![[object Object]](/_next/image/?url=%2F_next%2Fstatic%2Fmedia%2Flogo.6d15ce61.png&w=640&q=75)
Your All-in-One AI-Powered Toolkit for Academic Success.
+13062052269
info@desklib.com
Available 24*7 on WhatsApp / Email
Unlock your academic potential
© 2024 | Zucol Services PVT LTD | All rights reserved.