Introduction to Business Analytics docx.
VerifiedAdded on 2022/07/28
|11
|2745
|13
AI Summary
Contribute Materials
Your contribution can guide someone’s learning journey. Share your
documents today.
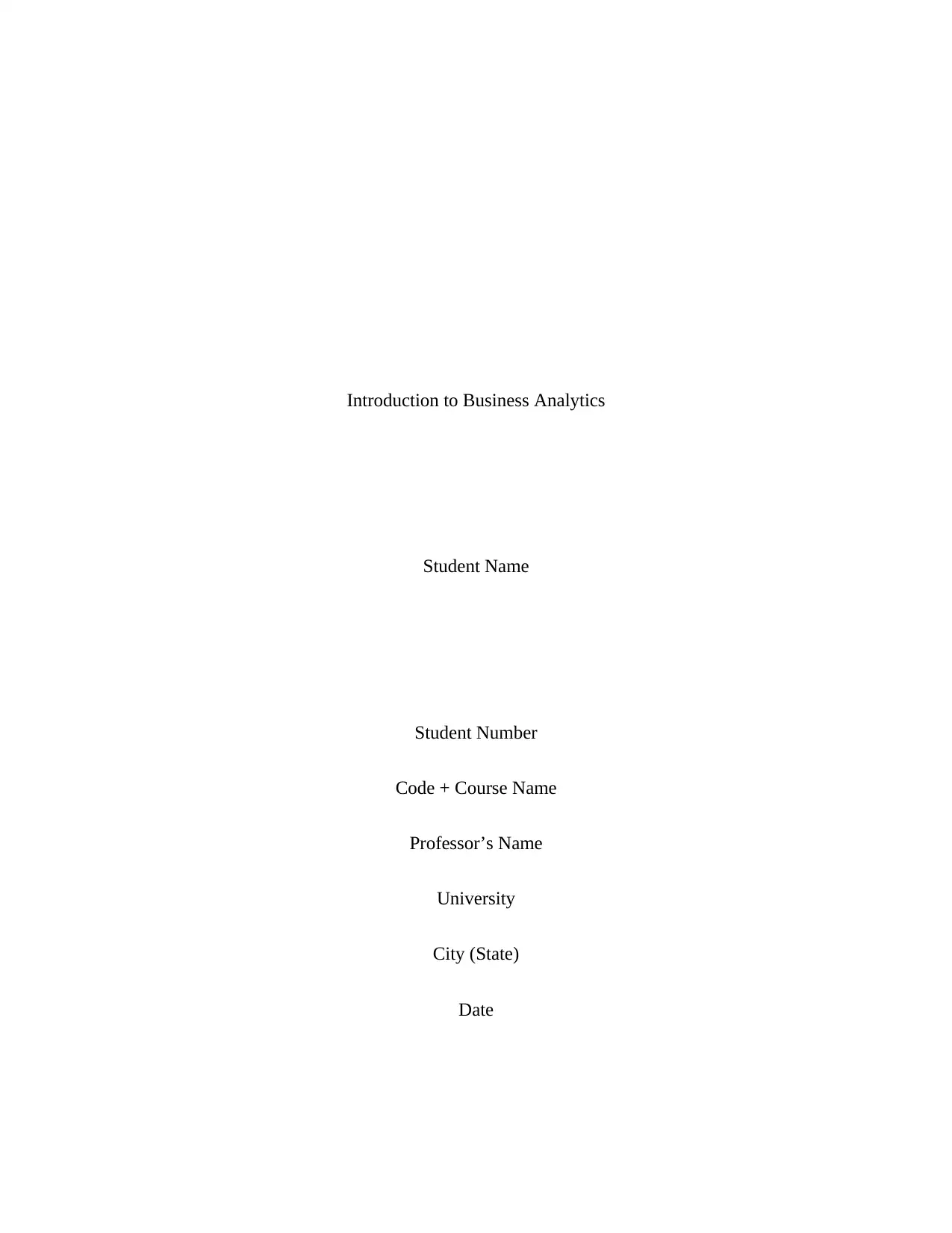
Introduction to Business Analytics
Student Name
Student Number
Code + Course Name
Professor’s Name
University
City (State)
Date
Student Name
Student Number
Code + Course Name
Professor’s Name
University
City (State)
Date
Secure Best Marks with AI Grader
Need help grading? Try our AI Grader for instant feedback on your assignments.
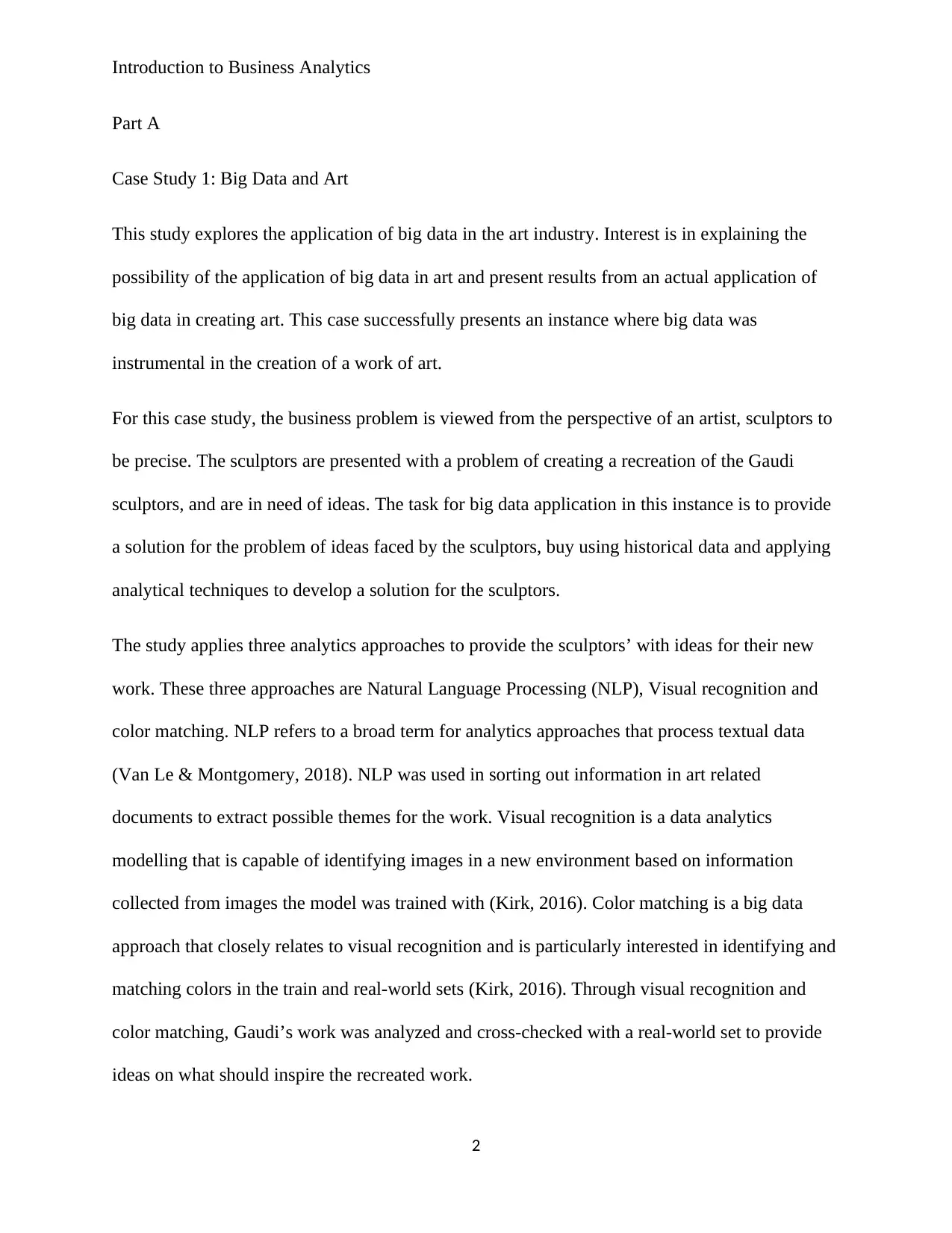
Introduction to Business Analytics
Part A
Case Study 1: Big Data and Art
This study explores the application of big data in the art industry. Interest is in explaining the
possibility of the application of big data in art and present results from an actual application of
big data in creating art. This case successfully presents an instance where big data was
instrumental in the creation of a work of art.
For this case study, the business problem is viewed from the perspective of an artist, sculptors to
be precise. The sculptors are presented with a problem of creating a recreation of the Gaudi
sculptors, and are in need of ideas. The task for big data application in this instance is to provide
a solution for the problem of ideas faced by the sculptors, buy using historical data and applying
analytical techniques to develop a solution for the sculptors.
The study applies three analytics approaches to provide the sculptors’ with ideas for their new
work. These three approaches are Natural Language Processing (NLP), Visual recognition and
color matching. NLP refers to a broad term for analytics approaches that process textual data
(Van Le & Montgomery, 2018). NLP was used in sorting out information in art related
documents to extract possible themes for the work. Visual recognition is a data analytics
modelling that is capable of identifying images in a new environment based on information
collected from images the model was trained with (Kirk, 2016). Color matching is a big data
approach that closely relates to visual recognition and is particularly interested in identifying and
matching colors in the train and real-world sets (Kirk, 2016). Through visual recognition and
color matching, Gaudi’s work was analyzed and cross-checked with a real-world set to provide
ideas on what should inspire the recreated work.
2
Part A
Case Study 1: Big Data and Art
This study explores the application of big data in the art industry. Interest is in explaining the
possibility of the application of big data in art and present results from an actual application of
big data in creating art. This case successfully presents an instance where big data was
instrumental in the creation of a work of art.
For this case study, the business problem is viewed from the perspective of an artist, sculptors to
be precise. The sculptors are presented with a problem of creating a recreation of the Gaudi
sculptors, and are in need of ideas. The task for big data application in this instance is to provide
a solution for the problem of ideas faced by the sculptors, buy using historical data and applying
analytical techniques to develop a solution for the sculptors.
The study applies three analytics approaches to provide the sculptors’ with ideas for their new
work. These three approaches are Natural Language Processing (NLP), Visual recognition and
color matching. NLP refers to a broad term for analytics approaches that process textual data
(Van Le & Montgomery, 2018). NLP was used in sorting out information in art related
documents to extract possible themes for the work. Visual recognition is a data analytics
modelling that is capable of identifying images in a new environment based on information
collected from images the model was trained with (Kirk, 2016). Color matching is a big data
approach that closely relates to visual recognition and is particularly interested in identifying and
matching colors in the train and real-world sets (Kirk, 2016). Through visual recognition and
color matching, Gaudi’s work was analyzed and cross-checked with a real-world set to provide
ideas on what should inspire the recreated work.
2
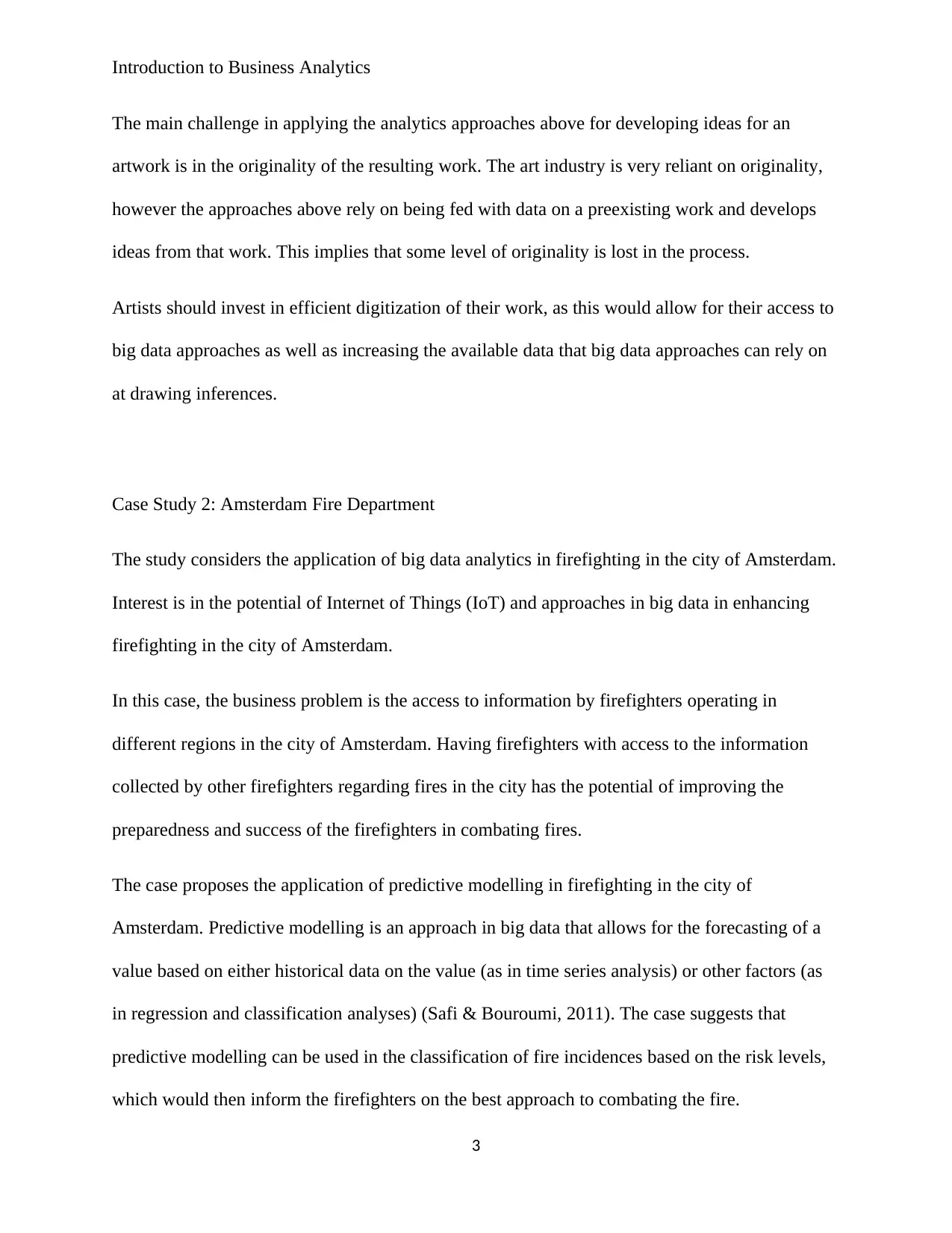
Introduction to Business Analytics
The main challenge in applying the analytics approaches above for developing ideas for an
artwork is in the originality of the resulting work. The art industry is very reliant on originality,
however the approaches above rely on being fed with data on a preexisting work and develops
ideas from that work. This implies that some level of originality is lost in the process.
Artists should invest in efficient digitization of their work, as this would allow for their access to
big data approaches as well as increasing the available data that big data approaches can rely on
at drawing inferences.
Case Study 2: Amsterdam Fire Department
The study considers the application of big data analytics in firefighting in the city of Amsterdam.
Interest is in the potential of Internet of Things (IoT) and approaches in big data in enhancing
firefighting in the city of Amsterdam.
In this case, the business problem is the access to information by firefighters operating in
different regions in the city of Amsterdam. Having firefighters with access to the information
collected by other firefighters regarding fires in the city has the potential of improving the
preparedness and success of the firefighters in combating fires.
The case proposes the application of predictive modelling in firefighting in the city of
Amsterdam. Predictive modelling is an approach in big data that allows for the forecasting of a
value based on either historical data on the value (as in time series analysis) or other factors (as
in regression and classification analyses) (Safi & Bouroumi, 2011). The case suggests that
predictive modelling can be used in the classification of fire incidences based on the risk levels,
which would then inform the firefighters on the best approach to combating the fire.
3
The main challenge in applying the analytics approaches above for developing ideas for an
artwork is in the originality of the resulting work. The art industry is very reliant on originality,
however the approaches above rely on being fed with data on a preexisting work and develops
ideas from that work. This implies that some level of originality is lost in the process.
Artists should invest in efficient digitization of their work, as this would allow for their access to
big data approaches as well as increasing the available data that big data approaches can rely on
at drawing inferences.
Case Study 2: Amsterdam Fire Department
The study considers the application of big data analytics in firefighting in the city of Amsterdam.
Interest is in the potential of Internet of Things (IoT) and approaches in big data in enhancing
firefighting in the city of Amsterdam.
In this case, the business problem is the access to information by firefighters operating in
different regions in the city of Amsterdam. Having firefighters with access to the information
collected by other firefighters regarding fires in the city has the potential of improving the
preparedness and success of the firefighters in combating fires.
The case proposes the application of predictive modelling in firefighting in the city of
Amsterdam. Predictive modelling is an approach in big data that allows for the forecasting of a
value based on either historical data on the value (as in time series analysis) or other factors (as
in regression and classification analyses) (Safi & Bouroumi, 2011). The case suggests that
predictive modelling can be used in the classification of fire incidences based on the risk levels,
which would then inform the firefighters on the best approach to combating the fire.
3
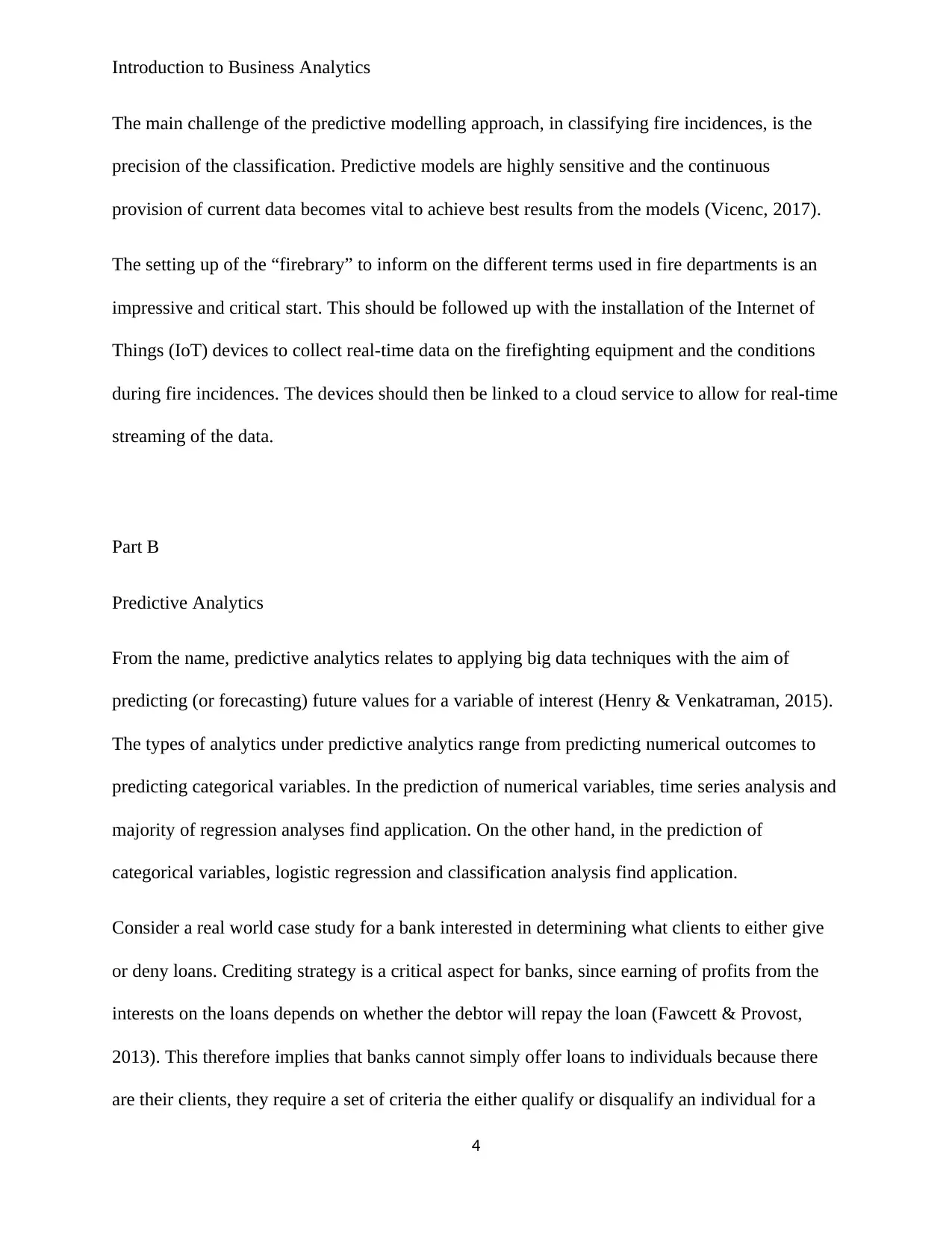
Introduction to Business Analytics
The main challenge of the predictive modelling approach, in classifying fire incidences, is the
precision of the classification. Predictive models are highly sensitive and the continuous
provision of current data becomes vital to achieve best results from the models (Vicenc, 2017).
The setting up of the “firebrary” to inform on the different terms used in fire departments is an
impressive and critical start. This should be followed up with the installation of the Internet of
Things (IoT) devices to collect real-time data on the firefighting equipment and the conditions
during fire incidences. The devices should then be linked to a cloud service to allow for real-time
streaming of the data.
Part B
Predictive Analytics
From the name, predictive analytics relates to applying big data techniques with the aim of
predicting (or forecasting) future values for a variable of interest (Henry & Venkatraman, 2015).
The types of analytics under predictive analytics range from predicting numerical outcomes to
predicting categorical variables. In the prediction of numerical variables, time series analysis and
majority of regression analyses find application. On the other hand, in the prediction of
categorical variables, logistic regression and classification analysis find application.
Consider a real world case study for a bank interested in determining what clients to either give
or deny loans. Crediting strategy is a critical aspect for banks, since earning of profits from the
interests on the loans depends on whether the debtor will repay the loan (Fawcett & Provost,
2013). This therefore implies that banks cannot simply offer loans to individuals because there
are their clients, they require a set of criteria the either qualify or disqualify an individual for a
4
The main challenge of the predictive modelling approach, in classifying fire incidences, is the
precision of the classification. Predictive models are highly sensitive and the continuous
provision of current data becomes vital to achieve best results from the models (Vicenc, 2017).
The setting up of the “firebrary” to inform on the different terms used in fire departments is an
impressive and critical start. This should be followed up with the installation of the Internet of
Things (IoT) devices to collect real-time data on the firefighting equipment and the conditions
during fire incidences. The devices should then be linked to a cloud service to allow for real-time
streaming of the data.
Part B
Predictive Analytics
From the name, predictive analytics relates to applying big data techniques with the aim of
predicting (or forecasting) future values for a variable of interest (Henry & Venkatraman, 2015).
The types of analytics under predictive analytics range from predicting numerical outcomes to
predicting categorical variables. In the prediction of numerical variables, time series analysis and
majority of regression analyses find application. On the other hand, in the prediction of
categorical variables, logistic regression and classification analysis find application.
Consider a real world case study for a bank interested in determining what clients to either give
or deny loans. Crediting strategy is a critical aspect for banks, since earning of profits from the
interests on the loans depends on whether the debtor will repay the loan (Fawcett & Provost,
2013). This therefore implies that banks cannot simply offer loans to individuals because there
are their clients, they require a set of criteria the either qualify or disqualify an individual for a
4
Paraphrase This Document
Need a fresh take? Get an instant paraphrase of this document with our AI Paraphraser
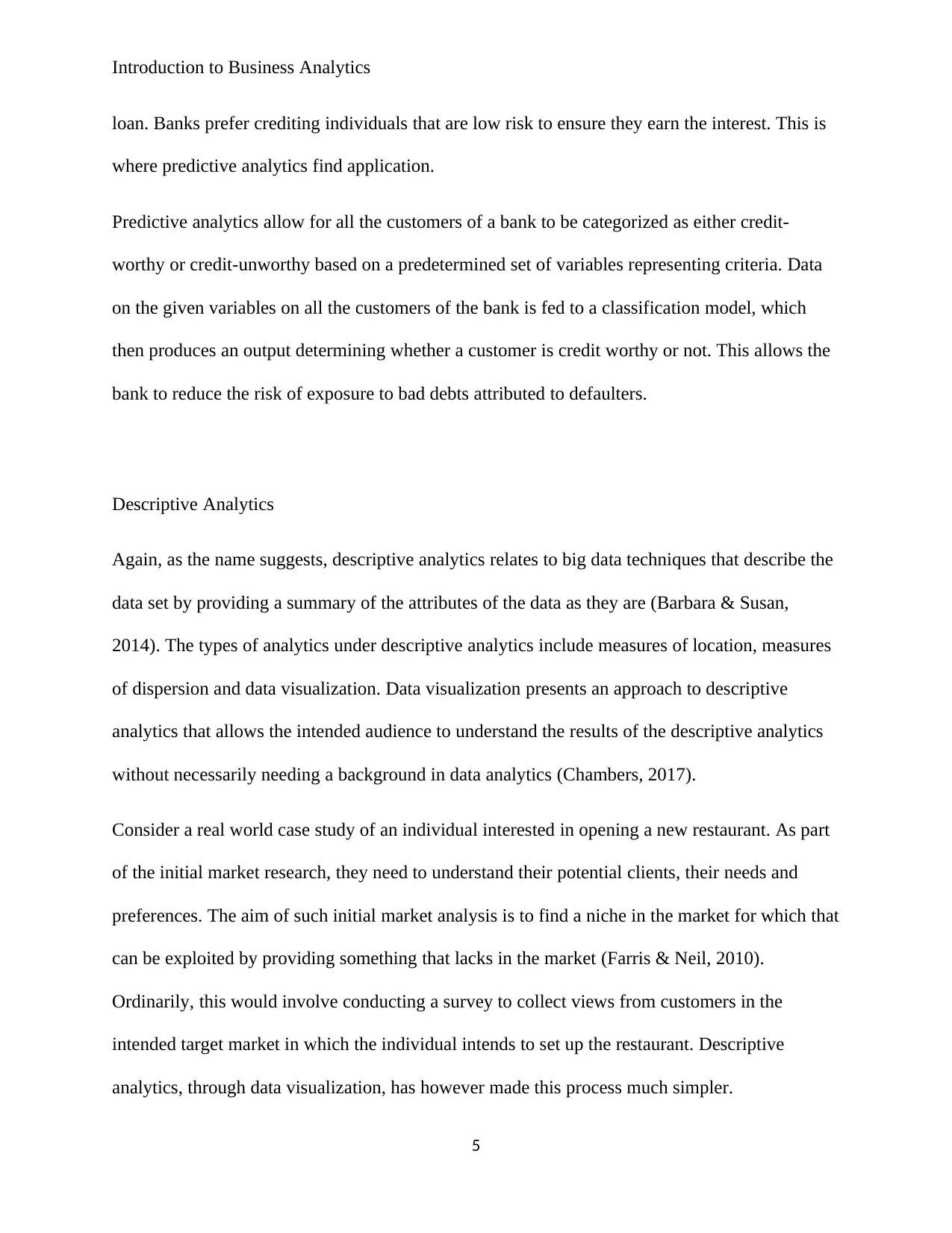
Introduction to Business Analytics
loan. Banks prefer crediting individuals that are low risk to ensure they earn the interest. This is
where predictive analytics find application.
Predictive analytics allow for all the customers of a bank to be categorized as either credit-
worthy or credit-unworthy based on a predetermined set of variables representing criteria. Data
on the given variables on all the customers of the bank is fed to a classification model, which
then produces an output determining whether a customer is credit worthy or not. This allows the
bank to reduce the risk of exposure to bad debts attributed to defaulters.
Descriptive Analytics
Again, as the name suggests, descriptive analytics relates to big data techniques that describe the
data set by providing a summary of the attributes of the data as they are (Barbara & Susan,
2014). The types of analytics under descriptive analytics include measures of location, measures
of dispersion and data visualization. Data visualization presents an approach to descriptive
analytics that allows the intended audience to understand the results of the descriptive analytics
without necessarily needing a background in data analytics (Chambers, 2017).
Consider a real world case study of an individual interested in opening a new restaurant. As part
of the initial market research, they need to understand their potential clients, their needs and
preferences. The aim of such initial market analysis is to find a niche in the market for which that
can be exploited by providing something that lacks in the market (Farris & Neil, 2010).
Ordinarily, this would involve conducting a survey to collect views from customers in the
intended target market in which the individual intends to set up the restaurant. Descriptive
analytics, through data visualization, has however made this process much simpler.
5
loan. Banks prefer crediting individuals that are low risk to ensure they earn the interest. This is
where predictive analytics find application.
Predictive analytics allow for all the customers of a bank to be categorized as either credit-
worthy or credit-unworthy based on a predetermined set of variables representing criteria. Data
on the given variables on all the customers of the bank is fed to a classification model, which
then produces an output determining whether a customer is credit worthy or not. This allows the
bank to reduce the risk of exposure to bad debts attributed to defaulters.
Descriptive Analytics
Again, as the name suggests, descriptive analytics relates to big data techniques that describe the
data set by providing a summary of the attributes of the data as they are (Barbara & Susan,
2014). The types of analytics under descriptive analytics include measures of location, measures
of dispersion and data visualization. Data visualization presents an approach to descriptive
analytics that allows the intended audience to understand the results of the descriptive analytics
without necessarily needing a background in data analytics (Chambers, 2017).
Consider a real world case study of an individual interested in opening a new restaurant. As part
of the initial market research, they need to understand their potential clients, their needs and
preferences. The aim of such initial market analysis is to find a niche in the market for which that
can be exploited by providing something that lacks in the market (Farris & Neil, 2010).
Ordinarily, this would involve conducting a survey to collect views from customers in the
intended target market in which the individual intends to set up the restaurant. Descriptive
analytics, through data visualization, has however made this process much simpler.
5
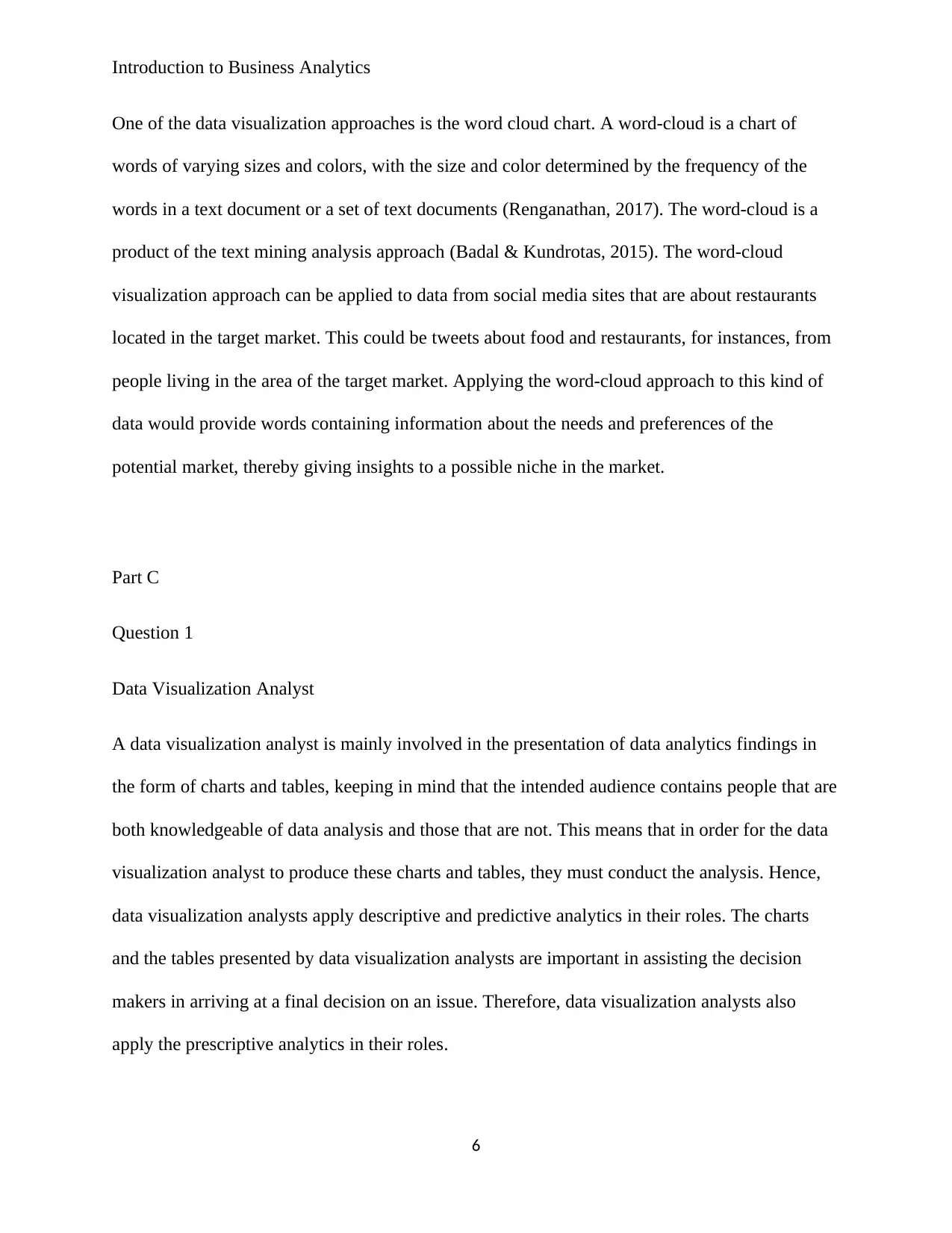
Introduction to Business Analytics
One of the data visualization approaches is the word cloud chart. A word-cloud is a chart of
words of varying sizes and colors, with the size and color determined by the frequency of the
words in a text document or a set of text documents (Renganathan, 2017). The word-cloud is a
product of the text mining analysis approach (Badal & Kundrotas, 2015). The word-cloud
visualization approach can be applied to data from social media sites that are about restaurants
located in the target market. This could be tweets about food and restaurants, for instances, from
people living in the area of the target market. Applying the word-cloud approach to this kind of
data would provide words containing information about the needs and preferences of the
potential market, thereby giving insights to a possible niche in the market.
Part C
Question 1
Data Visualization Analyst
A data visualization analyst is mainly involved in the presentation of data analytics findings in
the form of charts and tables, keeping in mind that the intended audience contains people that are
both knowledgeable of data analysis and those that are not. This means that in order for the data
visualization analyst to produce these charts and tables, they must conduct the analysis. Hence,
data visualization analysts apply descriptive and predictive analytics in their roles. The charts
and the tables presented by data visualization analysts are important in assisting the decision
makers in arriving at a final decision on an issue. Therefore, data visualization analysts also
apply the prescriptive analytics in their roles.
6
One of the data visualization approaches is the word cloud chart. A word-cloud is a chart of
words of varying sizes and colors, with the size and color determined by the frequency of the
words in a text document or a set of text documents (Renganathan, 2017). The word-cloud is a
product of the text mining analysis approach (Badal & Kundrotas, 2015). The word-cloud
visualization approach can be applied to data from social media sites that are about restaurants
located in the target market. This could be tweets about food and restaurants, for instances, from
people living in the area of the target market. Applying the word-cloud approach to this kind of
data would provide words containing information about the needs and preferences of the
potential market, thereby giving insights to a possible niche in the market.
Part C
Question 1
Data Visualization Analyst
A data visualization analyst is mainly involved in the presentation of data analytics findings in
the form of charts and tables, keeping in mind that the intended audience contains people that are
both knowledgeable of data analysis and those that are not. This means that in order for the data
visualization analyst to produce these charts and tables, they must conduct the analysis. Hence,
data visualization analysts apply descriptive and predictive analytics in their roles. The charts
and the tables presented by data visualization analysts are important in assisting the decision
makers in arriving at a final decision on an issue. Therefore, data visualization analysts also
apply the prescriptive analytics in their roles.
6
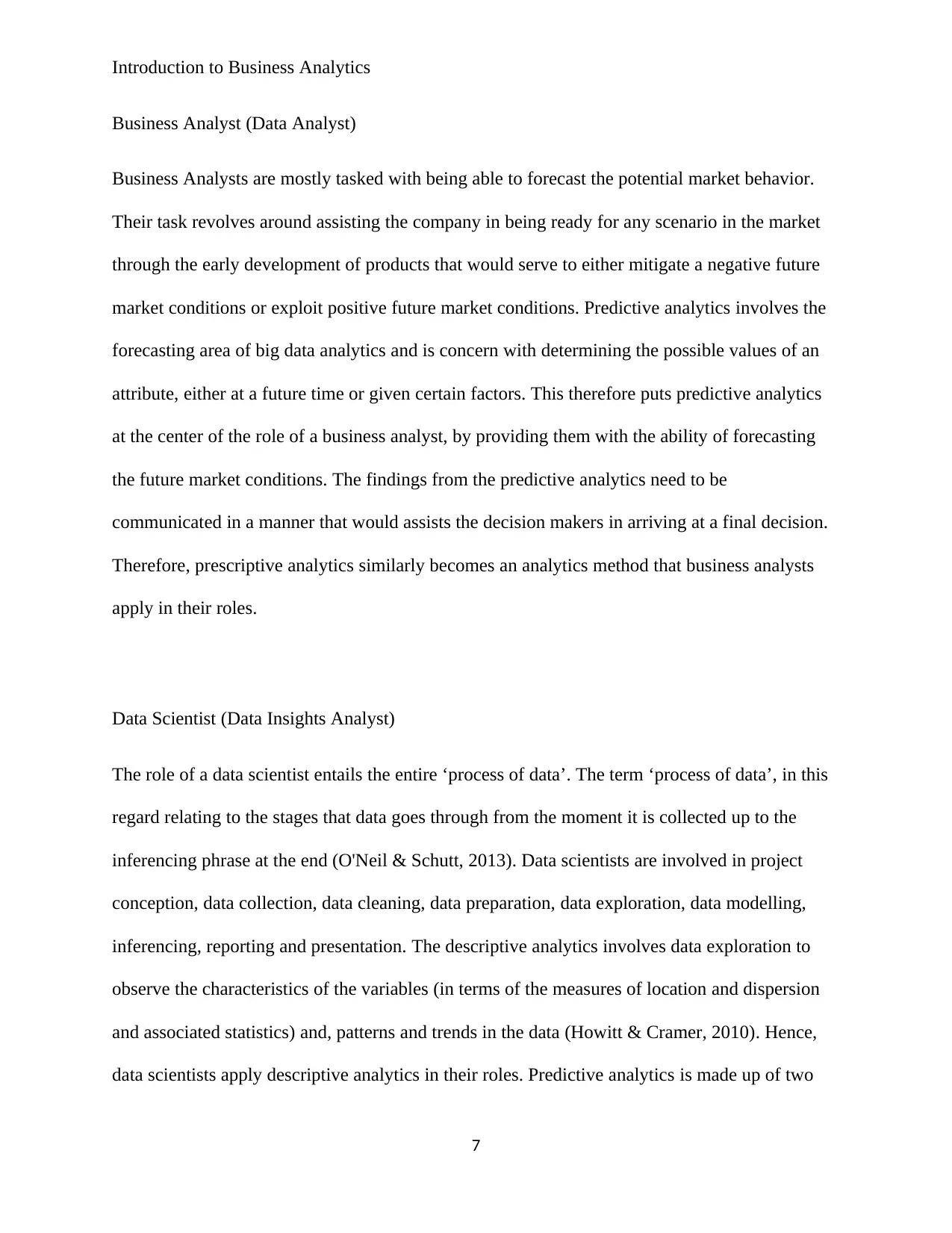
Introduction to Business Analytics
Business Analyst (Data Analyst)
Business Analysts are mostly tasked with being able to forecast the potential market behavior.
Their task revolves around assisting the company in being ready for any scenario in the market
through the early development of products that would serve to either mitigate a negative future
market conditions or exploit positive future market conditions. Predictive analytics involves the
forecasting area of big data analytics and is concern with determining the possible values of an
attribute, either at a future time or given certain factors. This therefore puts predictive analytics
at the center of the role of a business analyst, by providing them with the ability of forecasting
the future market conditions. The findings from the predictive analytics need to be
communicated in a manner that would assists the decision makers in arriving at a final decision.
Therefore, prescriptive analytics similarly becomes an analytics method that business analysts
apply in their roles.
Data Scientist (Data Insights Analyst)
The role of a data scientist entails the entire ‘process of data’. The term ‘process of data’, in this
regard relating to the stages that data goes through from the moment it is collected up to the
inferencing phrase at the end (O'Neil & Schutt, 2013). Data scientists are involved in project
conception, data collection, data cleaning, data preparation, data exploration, data modelling,
inferencing, reporting and presentation. The descriptive analytics involves data exploration to
observe the characteristics of the variables (in terms of the measures of location and dispersion
and associated statistics) and, patterns and trends in the data (Howitt & Cramer, 2010). Hence,
data scientists apply descriptive analytics in their roles. Predictive analytics is made up of two
7
Business Analyst (Data Analyst)
Business Analysts are mostly tasked with being able to forecast the potential market behavior.
Their task revolves around assisting the company in being ready for any scenario in the market
through the early development of products that would serve to either mitigate a negative future
market conditions or exploit positive future market conditions. Predictive analytics involves the
forecasting area of big data analytics and is concern with determining the possible values of an
attribute, either at a future time or given certain factors. This therefore puts predictive analytics
at the center of the role of a business analyst, by providing them with the ability of forecasting
the future market conditions. The findings from the predictive analytics need to be
communicated in a manner that would assists the decision makers in arriving at a final decision.
Therefore, prescriptive analytics similarly becomes an analytics method that business analysts
apply in their roles.
Data Scientist (Data Insights Analyst)
The role of a data scientist entails the entire ‘process of data’. The term ‘process of data’, in this
regard relating to the stages that data goes through from the moment it is collected up to the
inferencing phrase at the end (O'Neil & Schutt, 2013). Data scientists are involved in project
conception, data collection, data cleaning, data preparation, data exploration, data modelling,
inferencing, reporting and presentation. The descriptive analytics involves data exploration to
observe the characteristics of the variables (in terms of the measures of location and dispersion
and associated statistics) and, patterns and trends in the data (Howitt & Cramer, 2010). Hence,
data scientists apply descriptive analytics in their roles. Predictive analytics is made up of two
7
Secure Best Marks with AI Grader
Need help grading? Try our AI Grader for instant feedback on your assignments.

Introduction to Business Analytics
parts: data modeling and inferencing (Albright & Winston, 2014). The data modelling handles
the developing of the predictive model depending on the nature of the data, assumptions and the
intended purpose for the analysis process. The inferencing handles the interpretation and
contextualization of the results from the developed and applied predictive model. Since
predicting analytics involves both data modelling and inferencing, then data scientists apply
predictive analytics in their role. Prescriptive analytics involves the reporting and presentation of
study findings to assist decision makers in arriving at a final decision. Hence, data scientists
apply prescriptive analytics in their roles.
Analytics Translator
Analytics Translators handles the interpretation and application of the findings from the data
analysis process. Whereas other professionals in the field of data are involved in handling of the
data, there is need for individuals that can relate the findings with the market (Prescott, 2014;
Halaweh & El, 2014). This necessitates a professional with both the knowledge of the market
and data, the analytics translator. Analytics translators are less knowledgeable in the field of
data, but more knowledgeable in market dynamics of the market in which a company operates.
Due to their limited knowledge of data, analytics translators are more likely to apply automated
analytics in their roles.
Case Study 1: Big Data and Art
In the application of big data in the art industry, a data visualization analyst would be the most
useful analyst. The outputs from the NLP, visual recognition and color matching are mostly
8
parts: data modeling and inferencing (Albright & Winston, 2014). The data modelling handles
the developing of the predictive model depending on the nature of the data, assumptions and the
intended purpose for the analysis process. The inferencing handles the interpretation and
contextualization of the results from the developed and applied predictive model. Since
predicting analytics involves both data modelling and inferencing, then data scientists apply
predictive analytics in their role. Prescriptive analytics involves the reporting and presentation of
study findings to assist decision makers in arriving at a final decision. Hence, data scientists
apply prescriptive analytics in their roles.
Analytics Translator
Analytics Translators handles the interpretation and application of the findings from the data
analysis process. Whereas other professionals in the field of data are involved in handling of the
data, there is need for individuals that can relate the findings with the market (Prescott, 2014;
Halaweh & El, 2014). This necessitates a professional with both the knowledge of the market
and data, the analytics translator. Analytics translators are less knowledgeable in the field of
data, but more knowledgeable in market dynamics of the market in which a company operates.
Due to their limited knowledge of data, analytics translators are more likely to apply automated
analytics in their roles.
Case Study 1: Big Data and Art
In the application of big data in the art industry, a data visualization analyst would be the most
useful analyst. The outputs from the NLP, visual recognition and color matching are mostly
8
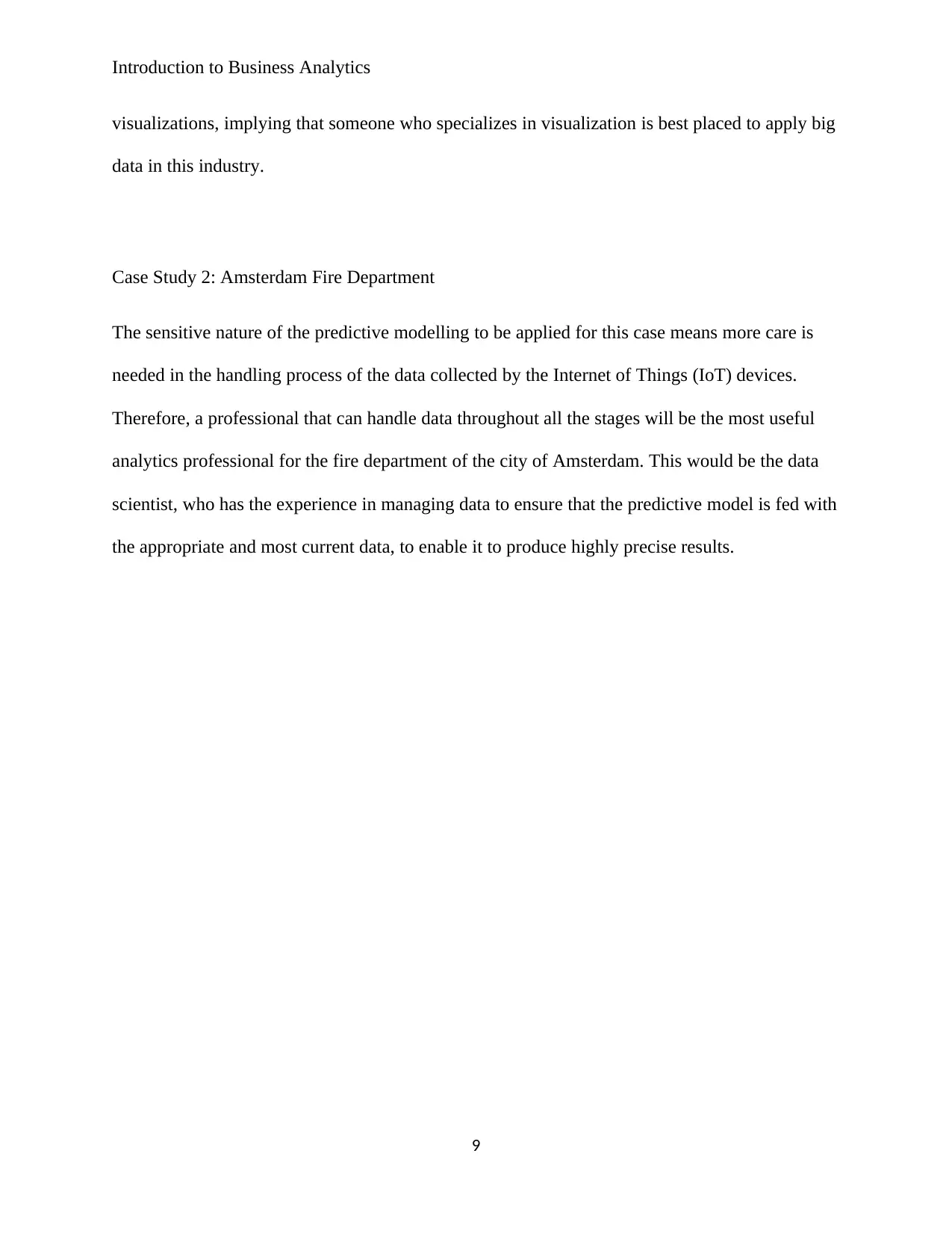
Introduction to Business Analytics
visualizations, implying that someone who specializes in visualization is best placed to apply big
data in this industry.
Case Study 2: Amsterdam Fire Department
The sensitive nature of the predictive modelling to be applied for this case means more care is
needed in the handling process of the data collected by the Internet of Things (IoT) devices.
Therefore, a professional that can handle data throughout all the stages will be the most useful
analytics professional for the fire department of the city of Amsterdam. This would be the data
scientist, who has the experience in managing data to ensure that the predictive model is fed with
the appropriate and most current data, to enable it to produce highly precise results.
9
visualizations, implying that someone who specializes in visualization is best placed to apply big
data in this industry.
Case Study 2: Amsterdam Fire Department
The sensitive nature of the predictive modelling to be applied for this case means more care is
needed in the handling process of the data collected by the Internet of Things (IoT) devices.
Therefore, a professional that can handle data throughout all the stages will be the most useful
analytics professional for the fire department of the city of Amsterdam. This would be the data
scientist, who has the experience in managing data to ensure that the predictive model is fed with
the appropriate and most current data, to enable it to produce highly precise results.
9
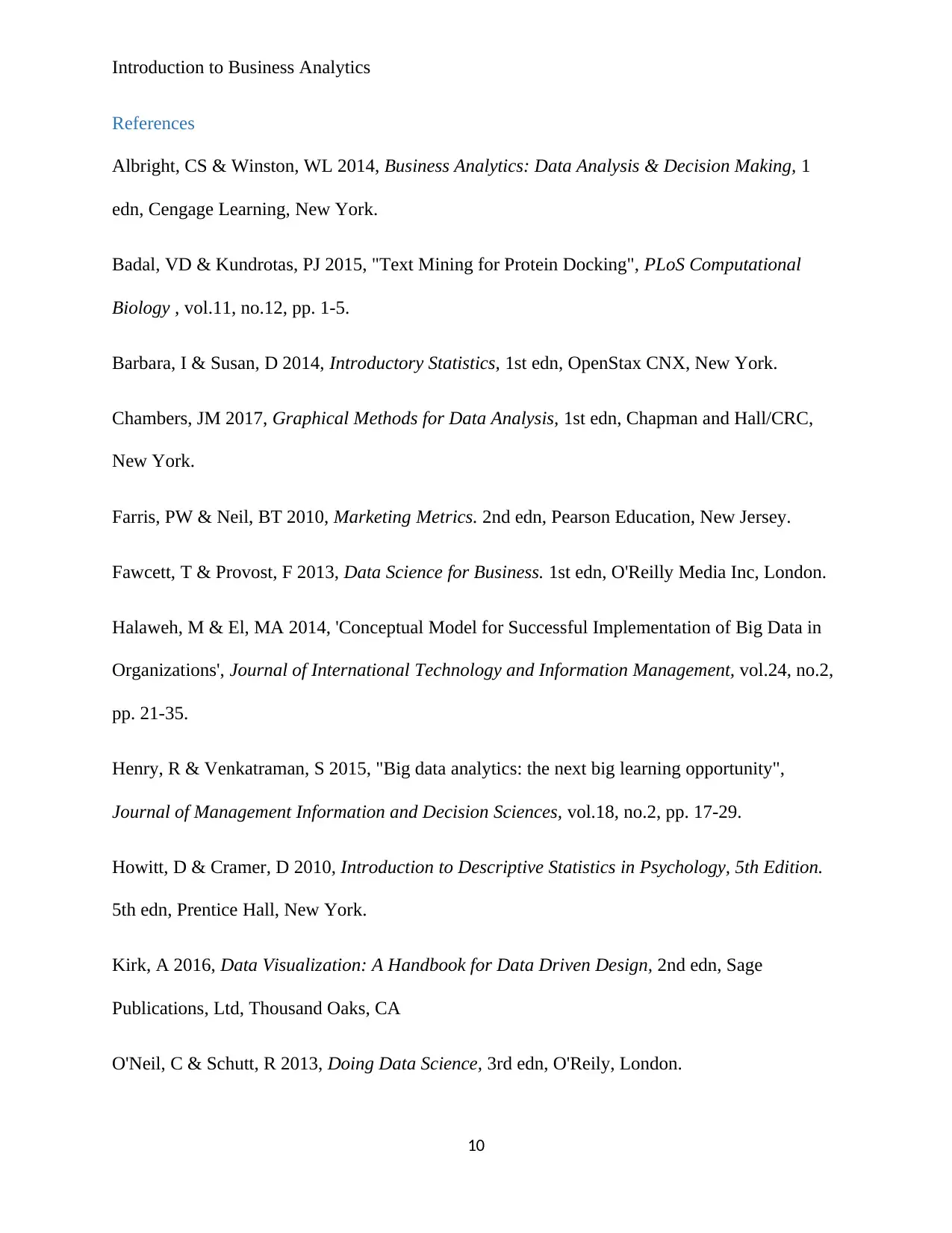
Introduction to Business Analytics
References
Albright, CS & Winston, WL 2014, Business Analytics: Data Analysis & Decision Making, 1
edn, Cengage Learning, New York.
Badal, VD & Kundrotas, PJ 2015, "Text Mining for Protein Docking", PLoS Computational
Biology , vol.11, no.12, pp. 1-5.
Barbara, I & Susan, D 2014, Introductory Statistics, 1st edn, OpenStax CNX, New York.
Chambers, JM 2017, Graphical Methods for Data Analysis, 1st edn, Chapman and Hall/CRC,
New York.
Farris, PW & Neil, BT 2010, Marketing Metrics. 2nd edn, Pearson Education, New Jersey.
Fawcett, T & Provost, F 2013, Data Science for Business. 1st edn, O'Reilly Media Inc, London.
Halaweh, M & El, MA 2014, 'Conceptual Model for Successful Implementation of Big Data in
Organizations', Journal of International Technology and Information Management, vol.24, no.2,
pp. 21-35.
Henry, R & Venkatraman, S 2015, "Big data analytics: the next big learning opportunity",
Journal of Management Information and Decision Sciences, vol.18, no.2, pp. 17-29.
Howitt, D & Cramer, D 2010, Introduction to Descriptive Statistics in Psychology, 5th Edition.
5th edn, Prentice Hall, New York.
Kirk, A 2016, Data Visualization: A Handbook for Data Driven Design, 2nd edn, Sage
Publications, Ltd, Thousand Oaks, CA
O'Neil, C & Schutt, R 2013, Doing Data Science, 3rd edn, O'Reily, London.
10
References
Albright, CS & Winston, WL 2014, Business Analytics: Data Analysis & Decision Making, 1
edn, Cengage Learning, New York.
Badal, VD & Kundrotas, PJ 2015, "Text Mining for Protein Docking", PLoS Computational
Biology , vol.11, no.12, pp. 1-5.
Barbara, I & Susan, D 2014, Introductory Statistics, 1st edn, OpenStax CNX, New York.
Chambers, JM 2017, Graphical Methods for Data Analysis, 1st edn, Chapman and Hall/CRC,
New York.
Farris, PW & Neil, BT 2010, Marketing Metrics. 2nd edn, Pearson Education, New Jersey.
Fawcett, T & Provost, F 2013, Data Science for Business. 1st edn, O'Reilly Media Inc, London.
Halaweh, M & El, MA 2014, 'Conceptual Model for Successful Implementation of Big Data in
Organizations', Journal of International Technology and Information Management, vol.24, no.2,
pp. 21-35.
Henry, R & Venkatraman, S 2015, "Big data analytics: the next big learning opportunity",
Journal of Management Information and Decision Sciences, vol.18, no.2, pp. 17-29.
Howitt, D & Cramer, D 2010, Introduction to Descriptive Statistics in Psychology, 5th Edition.
5th edn, Prentice Hall, New York.
Kirk, A 2016, Data Visualization: A Handbook for Data Driven Design, 2nd edn, Sage
Publications, Ltd, Thousand Oaks, CA
O'Neil, C & Schutt, R 2013, Doing Data Science, 3rd edn, O'Reily, London.
10
Paraphrase This Document
Need a fresh take? Get an instant paraphrase of this document with our AI Paraphraser
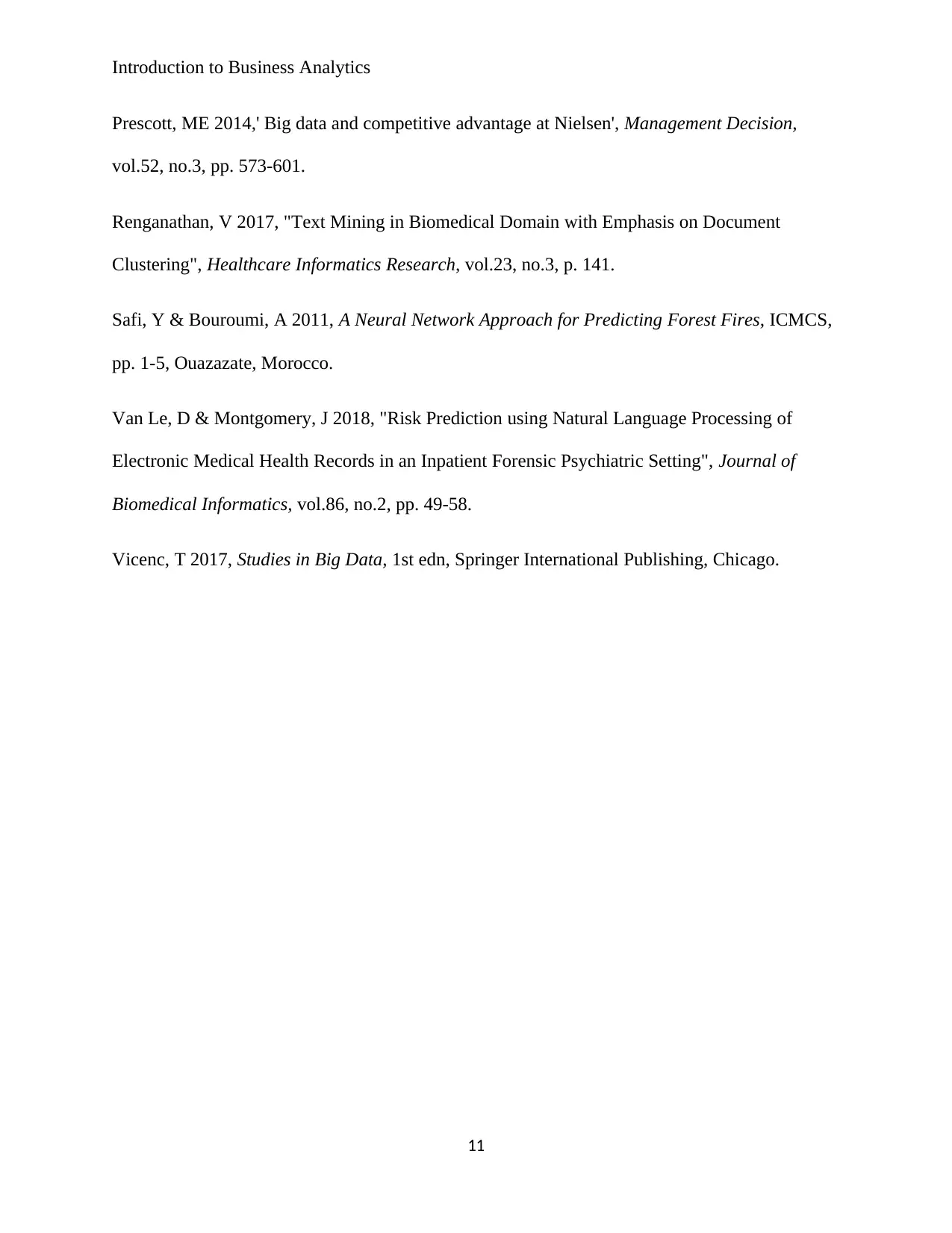
Introduction to Business Analytics
Prescott, ME 2014,' Big data and competitive advantage at Nielsen', Management Decision,
vol.52, no.3, pp. 573-601.
Renganathan, V 2017, "Text Mining in Biomedical Domain with Emphasis on Document
Clustering", Healthcare Informatics Research, vol.23, no.3, p. 141.
Safi, Y & Bouroumi, A 2011, A Neural Network Approach for Predicting Forest Fires, ICMCS,
pp. 1-5, Ouazazate, Morocco.
Van Le, D & Montgomery, J 2018, "Risk Prediction using Natural Language Processing of
Electronic Medical Health Records in an Inpatient Forensic Psychiatric Setting", Journal of
Biomedical Informatics, vol.86, no.2, pp. 49-58.
Vicenc, T 2017, Studies in Big Data, 1st edn, Springer International Publishing, Chicago.
11
Prescott, ME 2014,' Big data and competitive advantage at Nielsen', Management Decision,
vol.52, no.3, pp. 573-601.
Renganathan, V 2017, "Text Mining in Biomedical Domain with Emphasis on Document
Clustering", Healthcare Informatics Research, vol.23, no.3, p. 141.
Safi, Y & Bouroumi, A 2011, A Neural Network Approach for Predicting Forest Fires, ICMCS,
pp. 1-5, Ouazazate, Morocco.
Van Le, D & Montgomery, J 2018, "Risk Prediction using Natural Language Processing of
Electronic Medical Health Records in an Inpatient Forensic Psychiatric Setting", Journal of
Biomedical Informatics, vol.86, no.2, pp. 49-58.
Vicenc, T 2017, Studies in Big Data, 1st edn, Springer International Publishing, Chicago.
11
1 out of 11
Related Documents
![[object Object]](/_next/image/?url=%2F_next%2Fstatic%2Fmedia%2Flogo.6d15ce61.png&w=640&q=75)
Your All-in-One AI-Powered Toolkit for Academic Success.
+13062052269
info@desklib.com
Available 24*7 on WhatsApp / Email
Unlock your academic potential
© 2024 | Zucol Services PVT LTD | All rights reserved.