Literature Review: Machine Learning Techniques for Cancer Diagnosis
VerifiedAdded on 2022/08/20
|13
|4745
|7
Literature Review
AI Summary
This literature review delves into the transformative role of machine learning (ML) in cancer diagnosis, highlighting its potential to revolutionize clinical testing. It explores the limitations of traditional clinical testing and the advancements brought by ML, including the use of big data, advanced data analytics, and regulatory changes. The review examines various ML techniques, such as Artificial Neural Networks (ANNs), Support Vector Machines (SVMs), Decision Trees, and Bayesian Networks, and their applications in cancer detection, classification, and treatment. It also discusses the challenges and future directions of ML in cancer diagnosis, emphasizing the importance of data privacy, high-quality data, and regulatory frameworks. The review provides an overview of how ML algorithms predict pharmaceutical characteristics of targets and molecular compounds for drug development and discovery, ultimately aiming to improve patient outcomes and accelerate medical research. The paper discusses the use of techniques like deep learning methodologies on multimodal sources of data like the combination of clinical data and genomic data to be able to come up with new predictive frameworks and models. The use of segmentation and pattern recognition technologies on medical images like internal organs, retina scans, and body scans to enable speedy diagnosis and monitoring of the progress of the disease. Also, the use of generative innovations and algorithms for computational augmentation of current clinical testing data and imaging data sets.
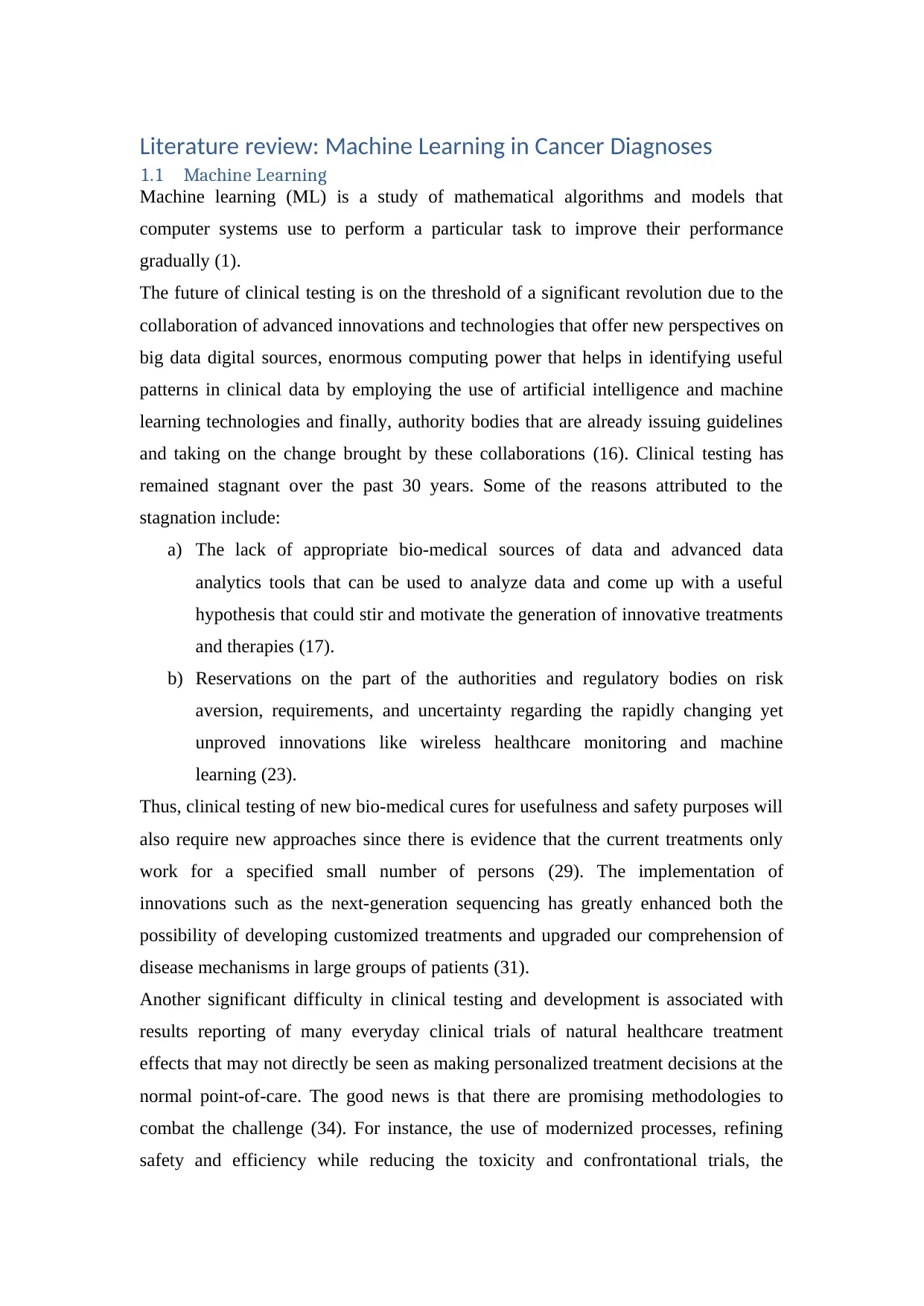
Literature review: Machine Learning in Cancer Diagnoses
1.1 Machine Learning
Machine learning (ML) is a study of mathematical algorithms and models that
computer systems use to perform a particular task to improve their performance
gradually (1).
The future of clinical testing is on the threshold of a significant revolution due to the
collaboration of advanced innovations and technologies that offer new perspectives on
big data digital sources, enormous computing power that helps in identifying useful
patterns in clinical data by employing the use of artificial intelligence and machine
learning technologies and finally, authority bodies that are already issuing guidelines
and taking on the change brought by these collaborations (16). Clinical testing has
remained stagnant over the past 30 years. Some of the reasons attributed to the
stagnation include:
a) The lack of appropriate bio-medical sources of data and advanced data
analytics tools that can be used to analyze data and come up with a useful
hypothesis that could stir and motivate the generation of innovative treatments
and therapies (17).
b) Reservations on the part of the authorities and regulatory bodies on risk
aversion, requirements, and uncertainty regarding the rapidly changing yet
unproved innovations like wireless healthcare monitoring and machine
learning (23).
Thus, clinical testing of new bio-medical cures for usefulness and safety purposes will
also require new approaches since there is evidence that the current treatments only
work for a specified small number of persons (29). The implementation of
innovations such as the next-generation sequencing has greatly enhanced both the
possibility of developing customized treatments and upgraded our comprehension of
disease mechanisms in large groups of patients (31).
Another significant difficulty in clinical testing and development is associated with
results reporting of many everyday clinical trials of natural healthcare treatment
effects that may not directly be seen as making personalized treatment decisions at the
normal point-of-care. The good news is that there are promising methodologies to
combat the challenge (34). For instance, the use of modernized processes, refining
safety and efficiency while reducing the toxicity and confrontational trials, the
1.1 Machine Learning
Machine learning (ML) is a study of mathematical algorithms and models that
computer systems use to perform a particular task to improve their performance
gradually (1).
The future of clinical testing is on the threshold of a significant revolution due to the
collaboration of advanced innovations and technologies that offer new perspectives on
big data digital sources, enormous computing power that helps in identifying useful
patterns in clinical data by employing the use of artificial intelligence and machine
learning technologies and finally, authority bodies that are already issuing guidelines
and taking on the change brought by these collaborations (16). Clinical testing has
remained stagnant over the past 30 years. Some of the reasons attributed to the
stagnation include:
a) The lack of appropriate bio-medical sources of data and advanced data
analytics tools that can be used to analyze data and come up with a useful
hypothesis that could stir and motivate the generation of innovative treatments
and therapies (17).
b) Reservations on the part of the authorities and regulatory bodies on risk
aversion, requirements, and uncertainty regarding the rapidly changing yet
unproved innovations like wireless healthcare monitoring and machine
learning (23).
Thus, clinical testing of new bio-medical cures for usefulness and safety purposes will
also require new approaches since there is evidence that the current treatments only
work for a specified small number of persons (29). The implementation of
innovations such as the next-generation sequencing has greatly enhanced both the
possibility of developing customized treatments and upgraded our comprehension of
disease mechanisms in large groups of patients (31).
Another significant difficulty in clinical testing and development is associated with
results reporting of many everyday clinical trials of natural healthcare treatment
effects that may not directly be seen as making personalized treatment decisions at the
normal point-of-care. The good news is that there are promising methodologies to
combat the challenge (34). For instance, the use of modernized processes, refining
safety and efficiency while reducing the toxicity and confrontational trials, the
Paraphrase This Document
Need a fresh take? Get an instant paraphrase of this document with our AI Paraphraser
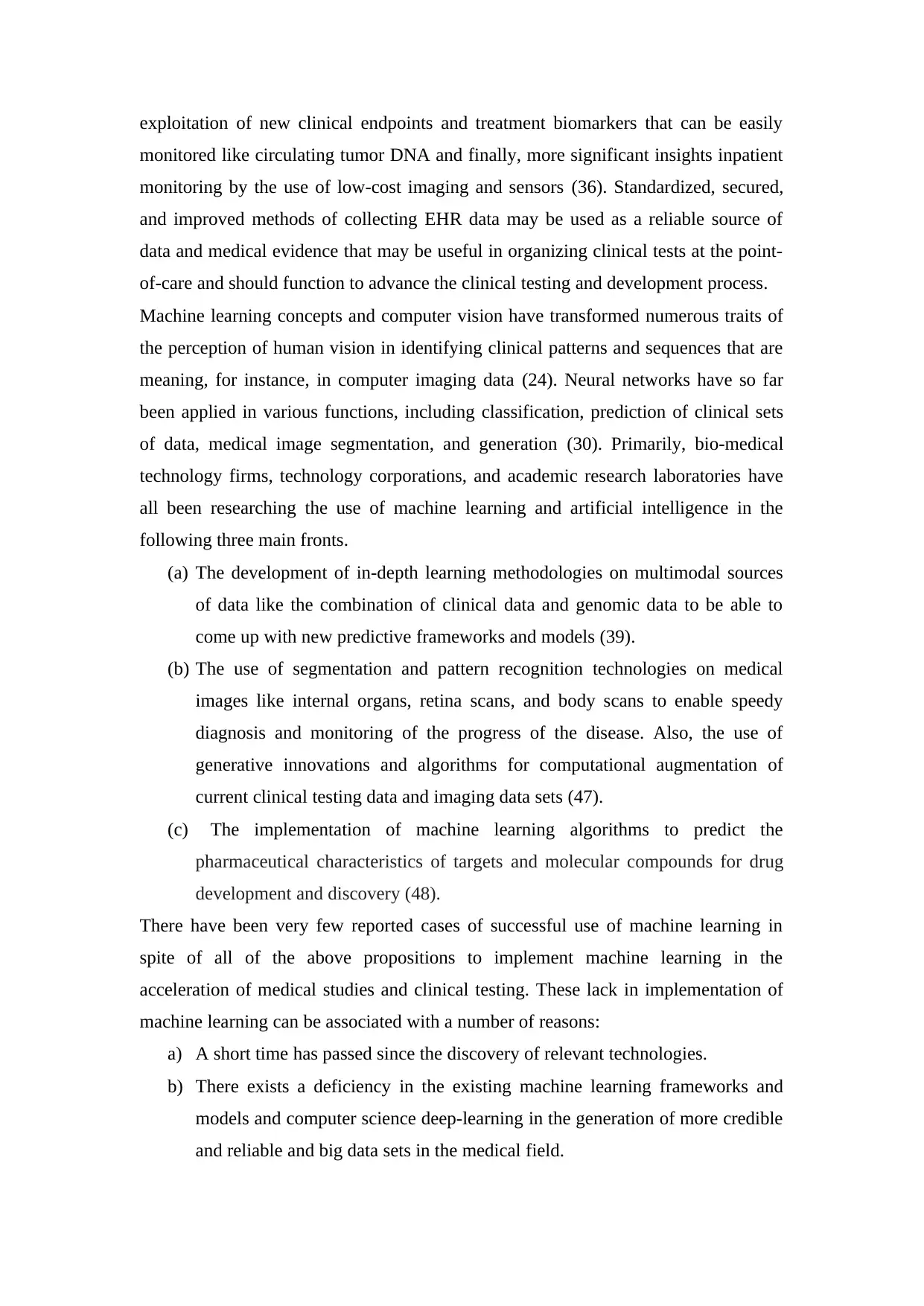
exploitation of new clinical endpoints and treatment biomarkers that can be easily
monitored like circulating tumor DNA and finally, more significant insights inpatient
monitoring by the use of low-cost imaging and sensors (36). Standardized, secured,
and improved methods of collecting EHR data may be used as a reliable source of
data and medical evidence that may be useful in organizing clinical tests at the point-
of-care and should function to advance the clinical testing and development process.
Machine learning concepts and computer vision have transformed numerous traits of
the perception of human vision in identifying clinical patterns and sequences that are
meaning, for instance, in computer imaging data (24). Neural networks have so far
been applied in various functions, including classification, prediction of clinical sets
of data, medical image segmentation, and generation (30). Primarily, bio-medical
technology firms, technology corporations, and academic research laboratories have
all been researching the use of machine learning and artificial intelligence in the
following three main fronts.
(a) The development of in-depth learning methodologies on multimodal sources
of data like the combination of clinical data and genomic data to be able to
come up with new predictive frameworks and models (39).
(b) The use of segmentation and pattern recognition technologies on medical
images like internal organs, retina scans, and body scans to enable speedy
diagnosis and monitoring of the progress of the disease. Also, the use of
generative innovations and algorithms for computational augmentation of
current clinical testing data and imaging data sets (47).
(c) The implementation of machine learning algorithms to predict the
pharmaceutical characteristics of targets and molecular compounds for drug
development and discovery (48).
There have been very few reported cases of successful use of machine learning in
spite of all of the above propositions to implement machine learning in the
acceleration of medical studies and clinical testing. These lack in implementation of
machine learning can be associated with a number of reasons:
a) A short time has passed since the discovery of relevant technologies.
b) There exists a deficiency in the existing machine learning frameworks and
models and computer science deep-learning in the generation of more credible
and reliable and big data sets in the medical field.
monitored like circulating tumor DNA and finally, more significant insights inpatient
monitoring by the use of low-cost imaging and sensors (36). Standardized, secured,
and improved methods of collecting EHR data may be used as a reliable source of
data and medical evidence that may be useful in organizing clinical tests at the point-
of-care and should function to advance the clinical testing and development process.
Machine learning concepts and computer vision have transformed numerous traits of
the perception of human vision in identifying clinical patterns and sequences that are
meaning, for instance, in computer imaging data (24). Neural networks have so far
been applied in various functions, including classification, prediction of clinical sets
of data, medical image segmentation, and generation (30). Primarily, bio-medical
technology firms, technology corporations, and academic research laboratories have
all been researching the use of machine learning and artificial intelligence in the
following three main fronts.
(a) The development of in-depth learning methodologies on multimodal sources
of data like the combination of clinical data and genomic data to be able to
come up with new predictive frameworks and models (39).
(b) The use of segmentation and pattern recognition technologies on medical
images like internal organs, retina scans, and body scans to enable speedy
diagnosis and monitoring of the progress of the disease. Also, the use of
generative innovations and algorithms for computational augmentation of
current clinical testing data and imaging data sets (47).
(c) The implementation of machine learning algorithms to predict the
pharmaceutical characteristics of targets and molecular compounds for drug
development and discovery (48).
There have been very few reported cases of successful use of machine learning in
spite of all of the above propositions to implement machine learning in the
acceleration of medical studies and clinical testing. These lack in implementation of
machine learning can be associated with a number of reasons:
a) A short time has passed since the discovery of relevant technologies.
b) There exists a deficiency in the existing machine learning frameworks and
models and computer science deep-learning in the generation of more credible
and reliable and big data sets in the medical field.
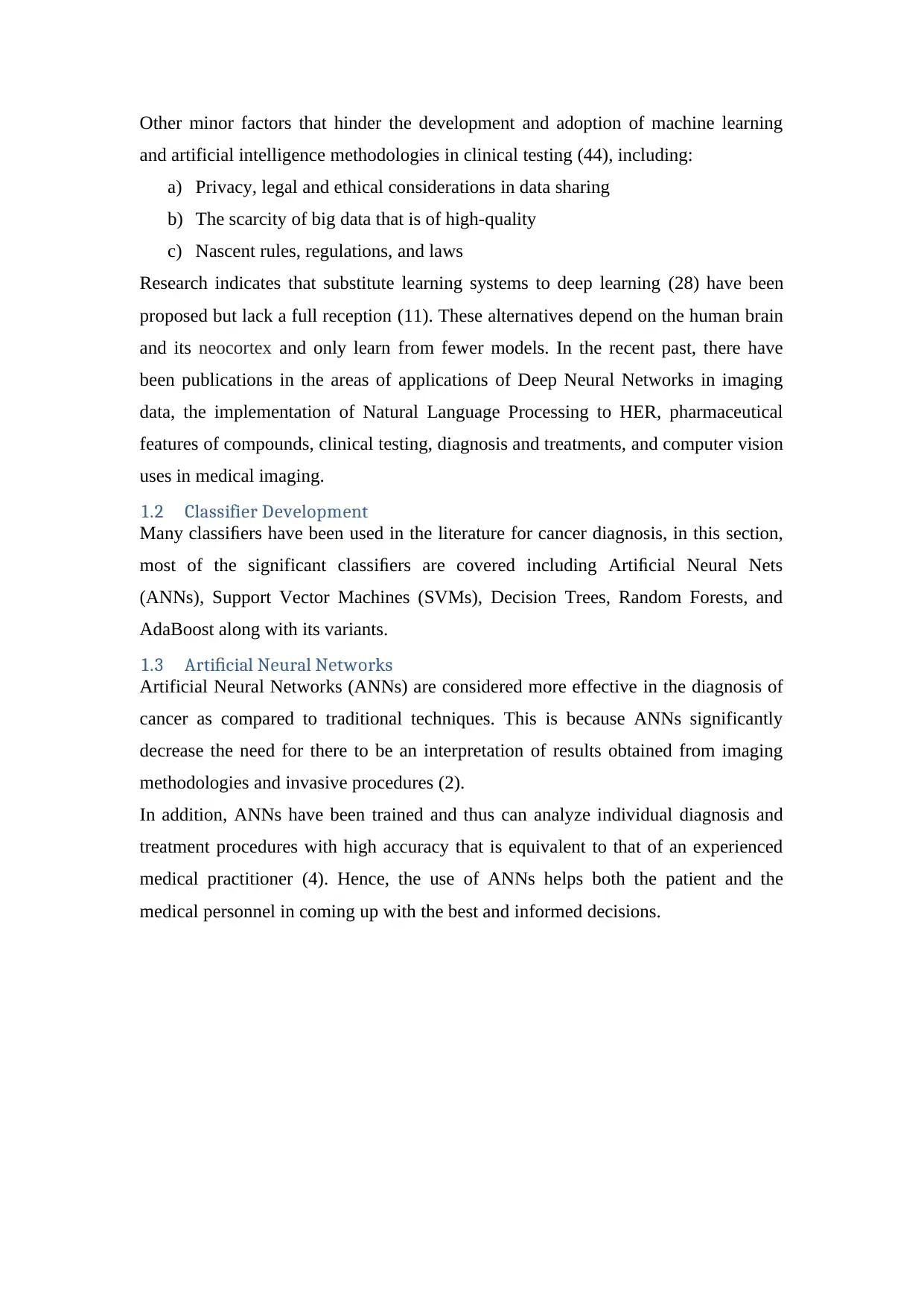
Other minor factors that hinder the development and adoption of machine learning
and artificial intelligence methodologies in clinical testing (44), including:
a) Privacy, legal and ethical considerations in data sharing
b) The scarcity of big data that is of high-quality
c) Nascent rules, regulations, and laws
Research indicates that substitute learning systems to deep learning (28) have been
proposed but lack a full reception (11). These alternatives depend on the human brain
and its neocortex and only learn from fewer models. In the recent past, there have
been publications in the areas of applications of Deep Neural Networks in imaging
data, the implementation of Natural Language Processing to HER, pharmaceutical
features of compounds, clinical testing, diagnosis and treatments, and computer vision
uses in medical imaging.
1.2 Classifier Development
Many classifiers have been used in the literature for cancer diagnosis, in this section,
most of the significant classifiers are covered including Artificial Neural Nets
(ANNs), Support Vector Machines (SVMs), Decision Trees, Random Forests, and
AdaBoost along with its variants.
1.3 Artificial Neural Networks
Artificial Neural Networks (ANNs) are considered more effective in the diagnosis of
cancer as compared to traditional techniques. This is because ANNs significantly
decrease the need for there to be an interpretation of results obtained from imaging
methodologies and invasive procedures (2).
In addition, ANNs have been trained and thus can analyze individual diagnosis and
treatment procedures with high accuracy that is equivalent to that of an experienced
medical practitioner (4). Hence, the use of ANNs helps both the patient and the
medical personnel in coming up with the best and informed decisions.
and artificial intelligence methodologies in clinical testing (44), including:
a) Privacy, legal and ethical considerations in data sharing
b) The scarcity of big data that is of high-quality
c) Nascent rules, regulations, and laws
Research indicates that substitute learning systems to deep learning (28) have been
proposed but lack a full reception (11). These alternatives depend on the human brain
and its neocortex and only learn from fewer models. In the recent past, there have
been publications in the areas of applications of Deep Neural Networks in imaging
data, the implementation of Natural Language Processing to HER, pharmaceutical
features of compounds, clinical testing, diagnosis and treatments, and computer vision
uses in medical imaging.
1.2 Classifier Development
Many classifiers have been used in the literature for cancer diagnosis, in this section,
most of the significant classifiers are covered including Artificial Neural Nets
(ANNs), Support Vector Machines (SVMs), Decision Trees, Random Forests, and
AdaBoost along with its variants.
1.3 Artificial Neural Networks
Artificial Neural Networks (ANNs) are considered more effective in the diagnosis of
cancer as compared to traditional techniques. This is because ANNs significantly
decrease the need for there to be an interpretation of results obtained from imaging
methodologies and invasive procedures (2).
In addition, ANNs have been trained and thus can analyze individual diagnosis and
treatment procedures with high accuracy that is equivalent to that of an experienced
medical practitioner (4). Hence, the use of ANNs helps both the patient and the
medical personnel in coming up with the best and informed decisions.
⊘ This is a preview!⊘
Do you want full access?
Subscribe today to unlock all pages.

Trusted by 1+ million students worldwide
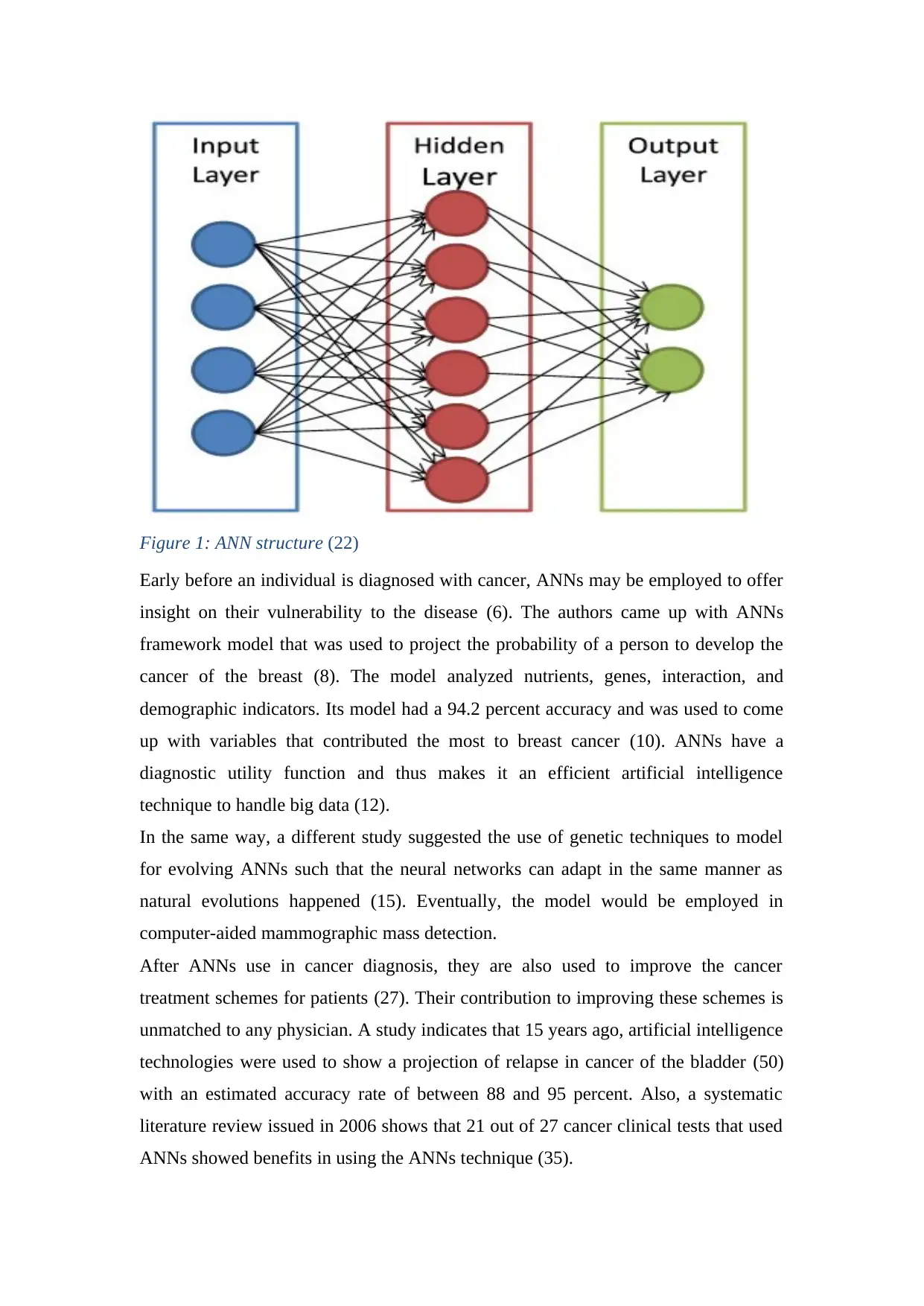
Figure 1: ANN structure (22)
Early before an individual is diagnosed with cancer, ANNs may be employed to offer
insight on their vulnerability to the disease (6). The authors came up with ANNs
framework model that was used to project the probability of a person to develop the
cancer of the breast (8). The model analyzed nutrients, genes, interaction, and
demographic indicators. Its model had a 94.2 percent accuracy and was used to come
up with variables that contributed the most to breast cancer (10). ANNs have a
diagnostic utility function and thus makes it an efficient artificial intelligence
technique to handle big data (12).
In the same way, a different study suggested the use of genetic techniques to model
for evolving ANNs such that the neural networks can adapt in the same manner as
natural evolutions happened (15). Eventually, the model would be employed in
computer-aided mammographic mass detection.
After ANNs use in cancer diagnosis, they are also used to improve the cancer
treatment schemes for patients (27). Their contribution to improving these schemes is
unmatched to any physician. A study indicates that 15 years ago, artificial intelligence
technologies were used to show a projection of relapse in cancer of the bladder (50)
with an estimated accuracy rate of between 88 and 95 percent. Also, a systematic
literature review issued in 2006 shows that 21 out of 27 cancer clinical tests that used
ANNs showed benefits in using the ANNs technique (35).
Early before an individual is diagnosed with cancer, ANNs may be employed to offer
insight on their vulnerability to the disease (6). The authors came up with ANNs
framework model that was used to project the probability of a person to develop the
cancer of the breast (8). The model analyzed nutrients, genes, interaction, and
demographic indicators. Its model had a 94.2 percent accuracy and was used to come
up with variables that contributed the most to breast cancer (10). ANNs have a
diagnostic utility function and thus makes it an efficient artificial intelligence
technique to handle big data (12).
In the same way, a different study suggested the use of genetic techniques to model
for evolving ANNs such that the neural networks can adapt in the same manner as
natural evolutions happened (15). Eventually, the model would be employed in
computer-aided mammographic mass detection.
After ANNs use in cancer diagnosis, they are also used to improve the cancer
treatment schemes for patients (27). Their contribution to improving these schemes is
unmatched to any physician. A study indicates that 15 years ago, artificial intelligence
technologies were used to show a projection of relapse in cancer of the bladder (50)
with an estimated accuracy rate of between 88 and 95 percent. Also, a systematic
literature review issued in 2006 shows that 21 out of 27 cancer clinical tests that used
ANNs showed benefits in using the ANNs technique (35).
Paraphrase This Document
Need a fresh take? Get an instant paraphrase of this document with our AI Paraphraser

1.4 Decision Trees
Decision Trees (DTs) use a classification scheme that is tree-like in structure. The
nodes in the tree represent the input variable in the problem we are addressing while
the leaves show the decision outcome (5). Decision Trees is a branch of machine
learning techniques that have been much employed for classification purposes (13).
One of the reasons why Decision Trees have remained in use for so long is because
they are quick to learn and are very simple to interpret (40). During the transversal
processes for classification of new specimens, the resulted decisions from DTs allow
for sufficient reasoning, making them a very engaging machine learning
methodology.
Figure 2: A DT with its elements and rules (22)
1.5 Support Vector Machine
The Support Vector Machine (SVM) is a state of the art machine learning and pattern
classification algorithm, which has been extensively applied in classification (42) and
regression tasks (7). SVM aims to a find maximum margin hyperplane to minimize
classification error. A function called the kernel K is used to predict the data from
input space to a new feature space, and if this projection is non-linear, it allows non-
linear decision boundaries (18).
SVM can be used to recognize fake credit/debit cards, handwriting, recognize a
speaker, or even detect a face (25). Cancer is considered as a genetic ailment that has
genomic pattern characteristics (45) that may be used as representations for the cancer
subtypes, the resulting prognosis, or even a tumor-specific genetic process (19).
Hence, the use of SVM is useful in analyzing and identifying these patterns.
Decision Trees (DTs) use a classification scheme that is tree-like in structure. The
nodes in the tree represent the input variable in the problem we are addressing while
the leaves show the decision outcome (5). Decision Trees is a branch of machine
learning techniques that have been much employed for classification purposes (13).
One of the reasons why Decision Trees have remained in use for so long is because
they are quick to learn and are very simple to interpret (40). During the transversal
processes for classification of new specimens, the resulted decisions from DTs allow
for sufficient reasoning, making them a very engaging machine learning
methodology.
Figure 2: A DT with its elements and rules (22)
1.5 Support Vector Machine
The Support Vector Machine (SVM) is a state of the art machine learning and pattern
classification algorithm, which has been extensively applied in classification (42) and
regression tasks (7). SVM aims to a find maximum margin hyperplane to minimize
classification error. A function called the kernel K is used to predict the data from
input space to a new feature space, and if this projection is non-linear, it allows non-
linear decision boundaries (18).
SVM can be used to recognize fake credit/debit cards, handwriting, recognize a
speaker, or even detect a face (25). Cancer is considered as a genetic ailment that has
genomic pattern characteristics (45) that may be used as representations for the cancer
subtypes, the resulting prognosis, or even a tumor-specific genetic process (19).
Hence, the use of SVM is useful in analyzing and identifying these patterns.
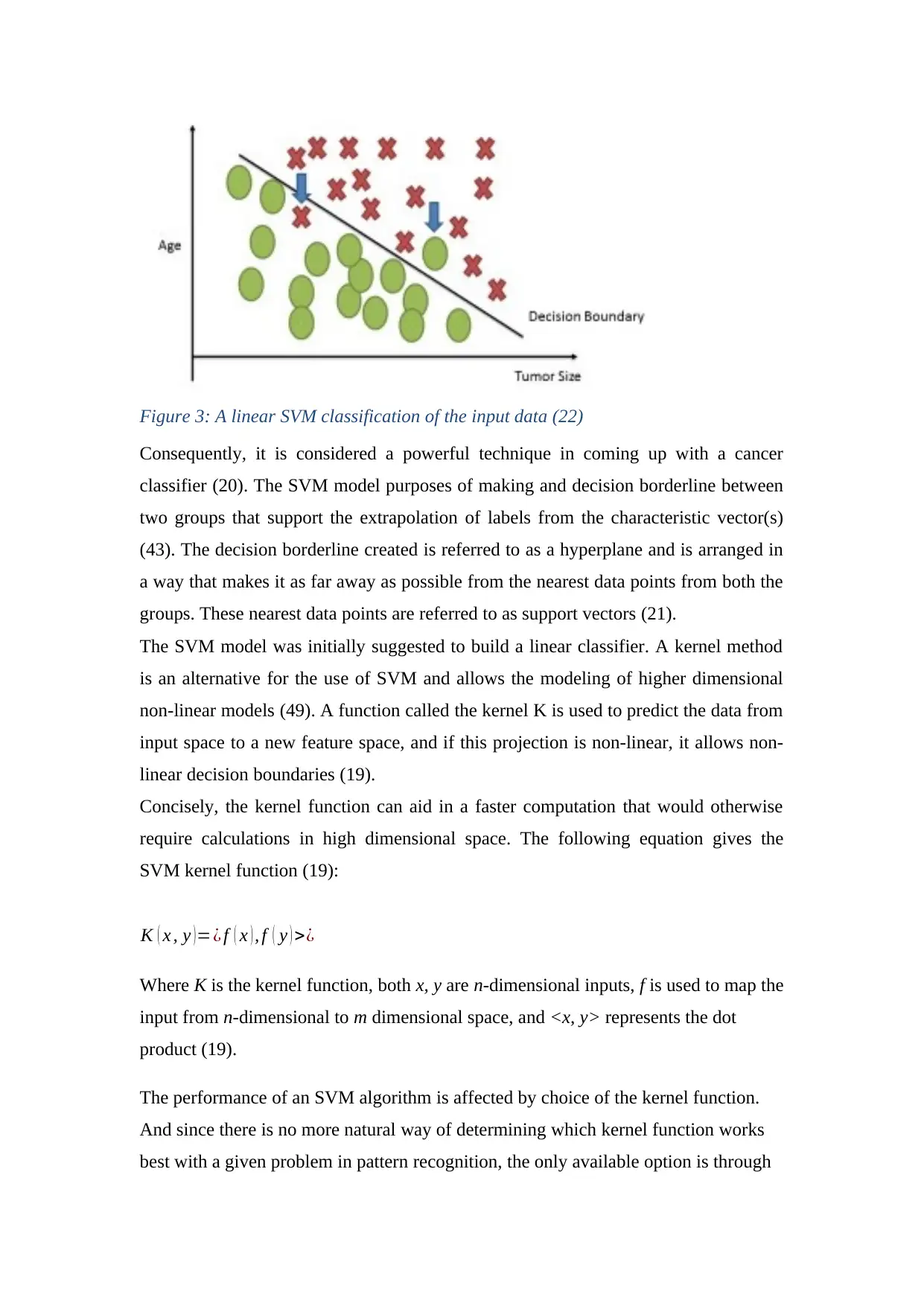
Figure 3: A linear SVM classification of the input data (22)
Consequently, it is considered a powerful technique in coming up with a cancer
classifier (20). The SVM model purposes of making and decision borderline between
two groups that support the extrapolation of labels from the characteristic vector(s)
(43). The decision borderline created is referred to as a hyperplane and is arranged in
a way that makes it as far away as possible from the nearest data points from both the
groups. These nearest data points are referred to as support vectors (21).
The SVM model was initially suggested to build a linear classifier. A kernel method
is an alternative for the use of SVM and allows the modeling of higher dimensional
non-linear models (49). A function called the kernel K is used to predict the data from
input space to a new feature space, and if this projection is non-linear, it allows non-
linear decision boundaries (19).
Concisely, the kernel function can aid in a faster computation that would otherwise
require calculations in high dimensional space. The following equation gives the
SVM kernel function (19):
K ( x , y )=¿ f ( x ) , f ( y ) >¿
Where K is the kernel function, both x, y are n-dimensional inputs, f is used to map the
input from n-dimensional to m dimensional space, and <x, y> represents the dot
product (19).
The performance of an SVM algorithm is affected by choice of the kernel function.
And since there is no more natural way of determining which kernel function works
best with a given problem in pattern recognition, the only available option is through
Consequently, it is considered a powerful technique in coming up with a cancer
classifier (20). The SVM model purposes of making and decision borderline between
two groups that support the extrapolation of labels from the characteristic vector(s)
(43). The decision borderline created is referred to as a hyperplane and is arranged in
a way that makes it as far away as possible from the nearest data points from both the
groups. These nearest data points are referred to as support vectors (21).
The SVM model was initially suggested to build a linear classifier. A kernel method
is an alternative for the use of SVM and allows the modeling of higher dimensional
non-linear models (49). A function called the kernel K is used to predict the data from
input space to a new feature space, and if this projection is non-linear, it allows non-
linear decision boundaries (19).
Concisely, the kernel function can aid in a faster computation that would otherwise
require calculations in high dimensional space. The following equation gives the
SVM kernel function (19):
K ( x , y )=¿ f ( x ) , f ( y ) >¿
Where K is the kernel function, both x, y are n-dimensional inputs, f is used to map the
input from n-dimensional to m dimensional space, and <x, y> represents the dot
product (19).
The performance of an SVM algorithm is affected by choice of the kernel function.
And since there is no more natural way of determining which kernel function works
best with a given problem in pattern recognition, the only available option is through
⊘ This is a preview!⊘
Do you want full access?
Subscribe today to unlock all pages.

Trusted by 1+ million students worldwide
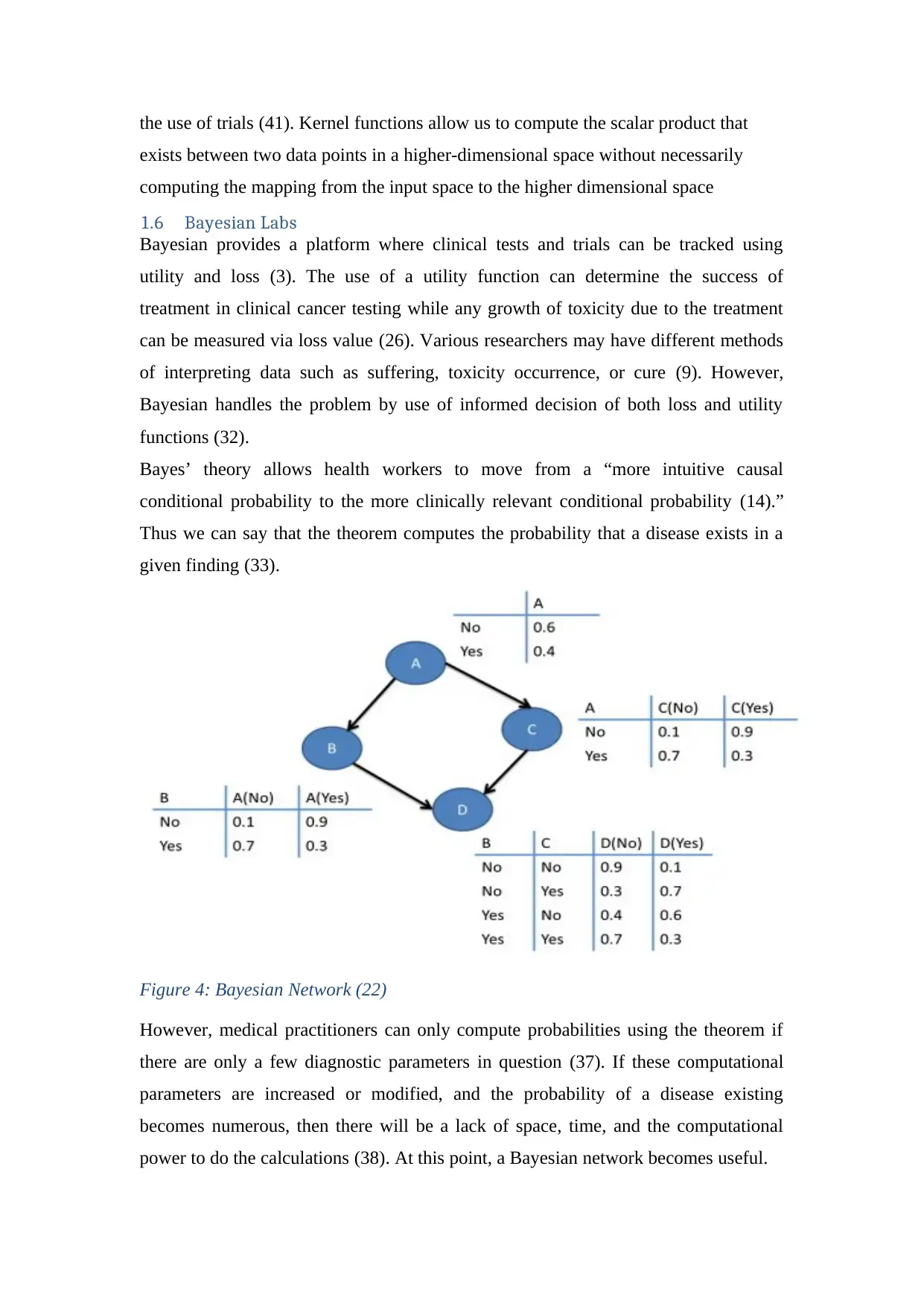
the use of trials (41). Kernel functions allow us to compute the scalar product that
exists between two data points in a higher-dimensional space without necessarily
computing the mapping from the input space to the higher dimensional space
1.6 Bayesian Labs
Bayesian provides a platform where clinical tests and trials can be tracked using
utility and loss (3). The use of a utility function can determine the success of
treatment in clinical cancer testing while any growth of toxicity due to the treatment
can be measured via loss value (26). Various researchers may have different methods
of interpreting data such as suffering, toxicity occurrence, or cure (9). However,
Bayesian handles the problem by use of informed decision of both loss and utility
functions (32).
Bayes’ theory allows health workers to move from a “more intuitive causal
conditional probability to the more clinically relevant conditional probability (14).”
Thus we can say that the theorem computes the probability that a disease exists in a
given finding (33).
Figure 4: Bayesian Network (22)
However, medical practitioners can only compute probabilities using the theorem if
there are only a few diagnostic parameters in question (37). If these computational
parameters are increased or modified, and the probability of a disease existing
becomes numerous, then there will be a lack of space, time, and the computational
power to do the calculations (38). At this point, a Bayesian network becomes useful.
exists between two data points in a higher-dimensional space without necessarily
computing the mapping from the input space to the higher dimensional space
1.6 Bayesian Labs
Bayesian provides a platform where clinical tests and trials can be tracked using
utility and loss (3). The use of a utility function can determine the success of
treatment in clinical cancer testing while any growth of toxicity due to the treatment
can be measured via loss value (26). Various researchers may have different methods
of interpreting data such as suffering, toxicity occurrence, or cure (9). However,
Bayesian handles the problem by use of informed decision of both loss and utility
functions (32).
Bayes’ theory allows health workers to move from a “more intuitive causal
conditional probability to the more clinically relevant conditional probability (14).”
Thus we can say that the theorem computes the probability that a disease exists in a
given finding (33).
Figure 4: Bayesian Network (22)
However, medical practitioners can only compute probabilities using the theorem if
there are only a few diagnostic parameters in question (37). If these computational
parameters are increased or modified, and the probability of a disease existing
becomes numerous, then there will be a lack of space, time, and the computational
power to do the calculations (38). At this point, a Bayesian network becomes useful.
Paraphrase This Document
Need a fresh take? Get an instant paraphrase of this document with our AI Paraphraser
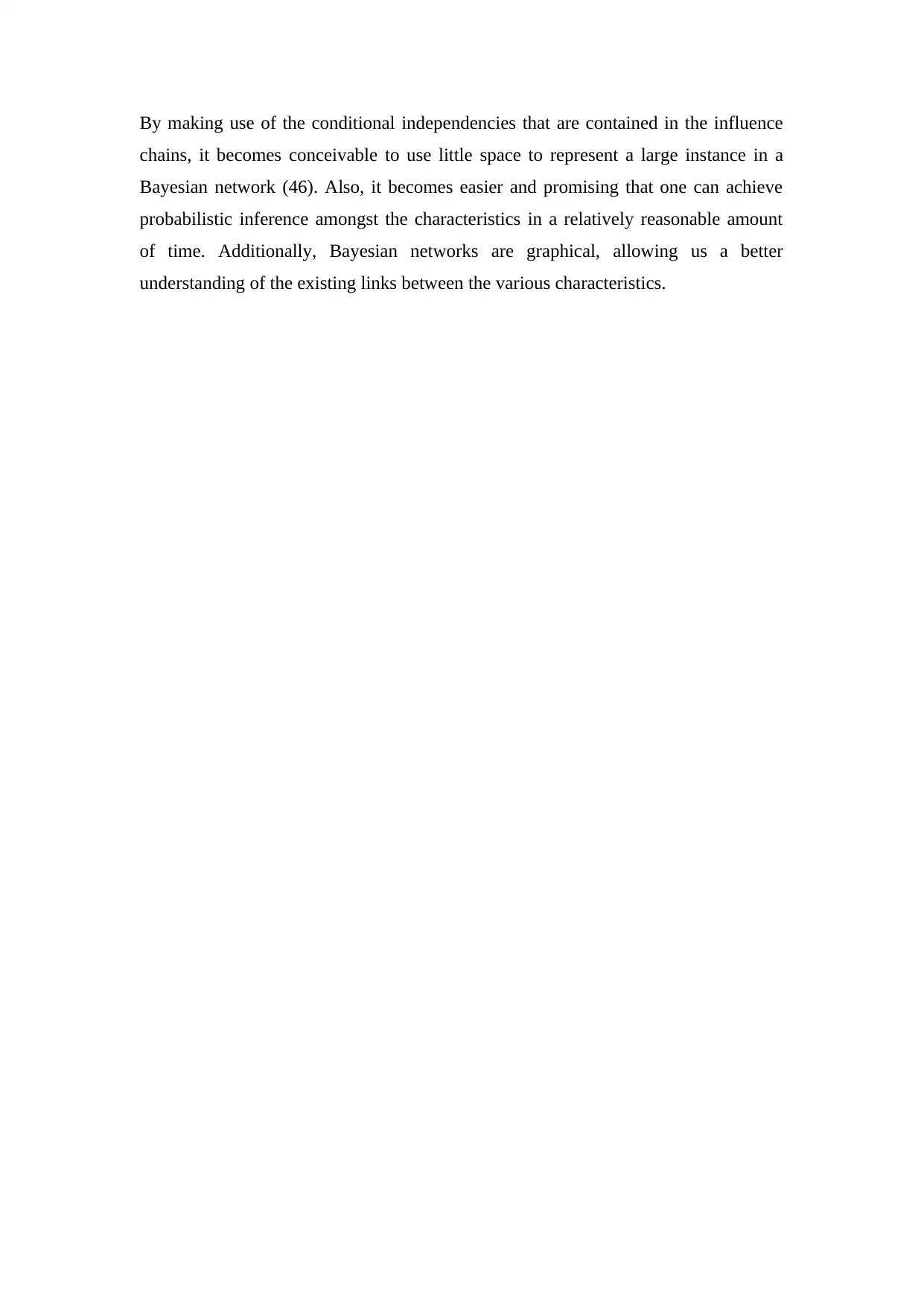
By making use of the conditional independencies that are contained in the influence
chains, it becomes conceivable to use little space to represent a large instance in a
Bayesian network (46). Also, it becomes easier and promising that one can achieve
probabilistic inference amongst the characteristics in a relatively reasonable amount
of time. Additionally, Bayesian networks are graphical, allowing us a better
understanding of the existing links between the various characteristics.
chains, it becomes conceivable to use little space to represent a large instance in a
Bayesian network (46). Also, it becomes easier and promising that one can achieve
probabilistic inference amongst the characteristics in a relatively reasonable amount
of time. Additionally, Bayesian networks are graphical, allowing us a better
understanding of the existing links between the various characteristics.
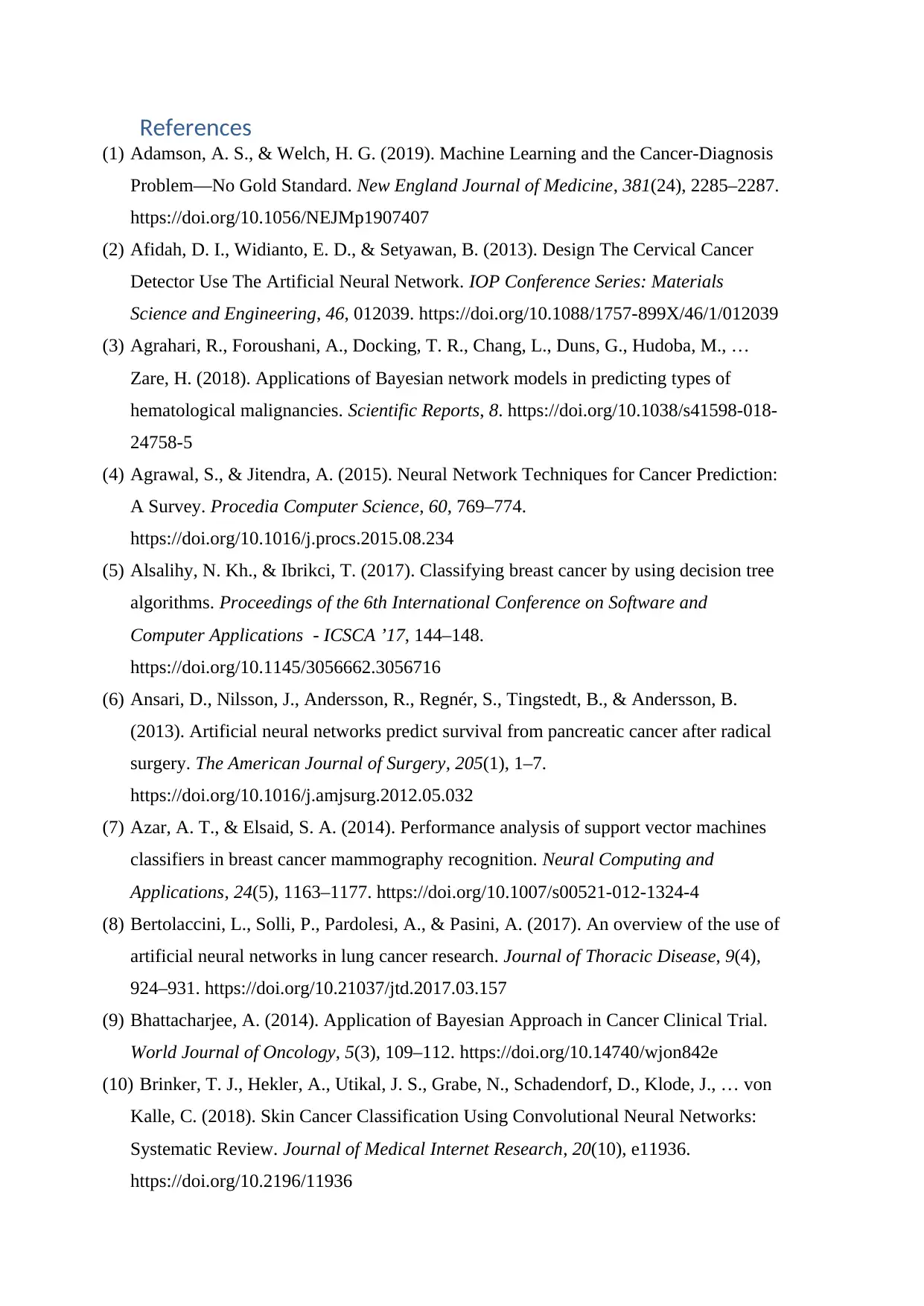
References
(1) Adamson, A. S., & Welch, H. G. (2019). Machine Learning and the Cancer-Diagnosis
Problem—No Gold Standard. New England Journal of Medicine, 381(24), 2285–2287.
https://doi.org/10.1056/NEJMp1907407
(2) Afidah, D. I., Widianto, E. D., & Setyawan, B. (2013). Design The Cervical Cancer
Detector Use The Artificial Neural Network. IOP Conference Series: Materials
Science and Engineering, 46, 012039. https://doi.org/10.1088/1757-899X/46/1/012039
(3) Agrahari, R., Foroushani, A., Docking, T. R., Chang, L., Duns, G., Hudoba, M., …
Zare, H. (2018). Applications of Bayesian network models in predicting types of
hematological malignancies. Scientific Reports, 8. https://doi.org/10.1038/s41598-018-
24758-5
(4) Agrawal, S., & Jitendra, A. (2015). Neural Network Techniques for Cancer Prediction:
A Survey. Procedia Computer Science, 60, 769–774.
https://doi.org/10.1016/j.procs.2015.08.234
(5) Alsalihy, N. Kh., & Ibrikci, T. (2017). Classifying breast cancer by using decision tree
algorithms. Proceedings of the 6th International Conference on Software and
Computer Applications - ICSCA ’17, 144–148.
https://doi.org/10.1145/3056662.3056716
(6) Ansari, D., Nilsson, J., Andersson, R., Regnér, S., Tingstedt, B., & Andersson, B.
(2013). Artificial neural networks predict survival from pancreatic cancer after radical
surgery. The American Journal of Surgery, 205(1), 1–7.
https://doi.org/10.1016/j.amjsurg.2012.05.032
(7) Azar, A. T., & Elsaid, S. A. (2014). Performance analysis of support vector machines
classifiers in breast cancer mammography recognition. Neural Computing and
Applications, 24(5), 1163–1177. https://doi.org/10.1007/s00521-012-1324-4
(8) Bertolaccini, L., Solli, P., Pardolesi, A., & Pasini, A. (2017). An overview of the use of
artificial neural networks in lung cancer research. Journal of Thoracic Disease, 9(4),
924–931. https://doi.org/10.21037/jtd.2017.03.157
(9) Bhattacharjee, A. (2014). Application of Bayesian Approach in Cancer Clinical Trial.
World Journal of Oncology, 5(3), 109–112. https://doi.org/10.14740/wjon842e
(10) Brinker, T. J., Hekler, A., Utikal, J. S., Grabe, N., Schadendorf, D., Klode, J., … von
Kalle, C. (2018). Skin Cancer Classification Using Convolutional Neural Networks:
Systematic Review. Journal of Medical Internet Research, 20(10), e11936.
https://doi.org/10.2196/11936
(1) Adamson, A. S., & Welch, H. G. (2019). Machine Learning and the Cancer-Diagnosis
Problem—No Gold Standard. New England Journal of Medicine, 381(24), 2285–2287.
https://doi.org/10.1056/NEJMp1907407
(2) Afidah, D. I., Widianto, E. D., & Setyawan, B. (2013). Design The Cervical Cancer
Detector Use The Artificial Neural Network. IOP Conference Series: Materials
Science and Engineering, 46, 012039. https://doi.org/10.1088/1757-899X/46/1/012039
(3) Agrahari, R., Foroushani, A., Docking, T. R., Chang, L., Duns, G., Hudoba, M., …
Zare, H. (2018). Applications of Bayesian network models in predicting types of
hematological malignancies. Scientific Reports, 8. https://doi.org/10.1038/s41598-018-
24758-5
(4) Agrawal, S., & Jitendra, A. (2015). Neural Network Techniques for Cancer Prediction:
A Survey. Procedia Computer Science, 60, 769–774.
https://doi.org/10.1016/j.procs.2015.08.234
(5) Alsalihy, N. Kh., & Ibrikci, T. (2017). Classifying breast cancer by using decision tree
algorithms. Proceedings of the 6th International Conference on Software and
Computer Applications - ICSCA ’17, 144–148.
https://doi.org/10.1145/3056662.3056716
(6) Ansari, D., Nilsson, J., Andersson, R., Regnér, S., Tingstedt, B., & Andersson, B.
(2013). Artificial neural networks predict survival from pancreatic cancer after radical
surgery. The American Journal of Surgery, 205(1), 1–7.
https://doi.org/10.1016/j.amjsurg.2012.05.032
(7) Azar, A. T., & Elsaid, S. A. (2014). Performance analysis of support vector machines
classifiers in breast cancer mammography recognition. Neural Computing and
Applications, 24(5), 1163–1177. https://doi.org/10.1007/s00521-012-1324-4
(8) Bertolaccini, L., Solli, P., Pardolesi, A., & Pasini, A. (2017). An overview of the use of
artificial neural networks in lung cancer research. Journal of Thoracic Disease, 9(4),
924–931. https://doi.org/10.21037/jtd.2017.03.157
(9) Bhattacharjee, A. (2014). Application of Bayesian Approach in Cancer Clinical Trial.
World Journal of Oncology, 5(3), 109–112. https://doi.org/10.14740/wjon842e
(10) Brinker, T. J., Hekler, A., Utikal, J. S., Grabe, N., Schadendorf, D., Klode, J., … von
Kalle, C. (2018). Skin Cancer Classification Using Convolutional Neural Networks:
Systematic Review. Journal of Medical Internet Research, 20(10), e11936.
https://doi.org/10.2196/11936
⊘ This is a preview!⊘
Do you want full access?
Subscribe today to unlock all pages.

Trusted by 1+ million students worldwide
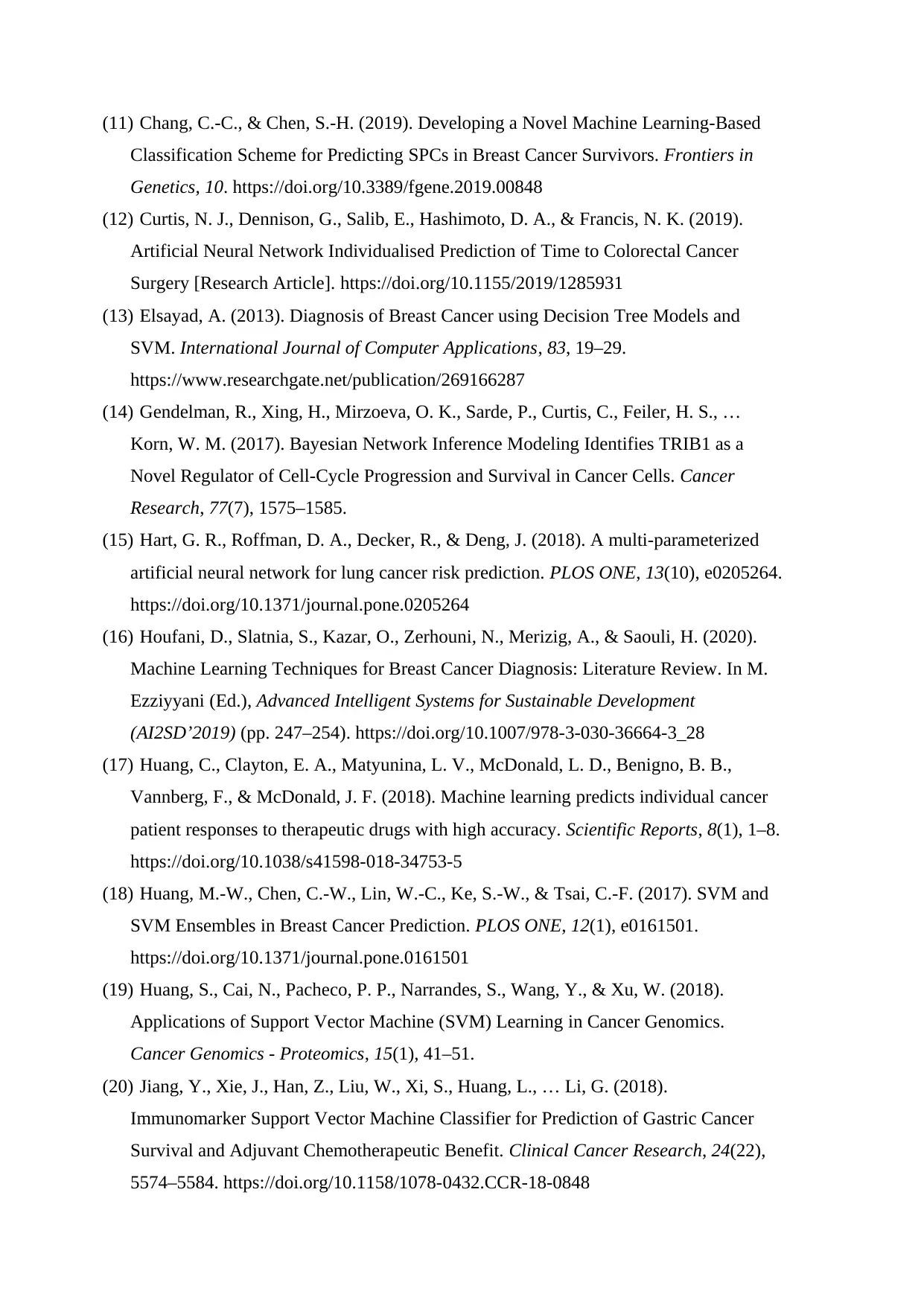
(11) Chang, C.-C., & Chen, S.-H. (2019). Developing a Novel Machine Learning-Based
Classification Scheme for Predicting SPCs in Breast Cancer Survivors. Frontiers in
Genetics, 10. https://doi.org/10.3389/fgene.2019.00848
(12) Curtis, N. J., Dennison, G., Salib, E., Hashimoto, D. A., & Francis, N. K. (2019).
Artificial Neural Network Individualised Prediction of Time to Colorectal Cancer
Surgery [Research Article]. https://doi.org/10.1155/2019/1285931
(13) Elsayad, A. (2013). Diagnosis of Breast Cancer using Decision Tree Models and
SVM. International Journal of Computer Applications, 83, 19–29.
https://www.researchgate.net/publication/269166287
(14) Gendelman, R., Xing, H., Mirzoeva, O. K., Sarde, P., Curtis, C., Feiler, H. S., …
Korn, W. M. (2017). Bayesian Network Inference Modeling Identifies TRIB1 as a
Novel Regulator of Cell-Cycle Progression and Survival in Cancer Cells. Cancer
Research, 77(7), 1575–1585.
(15) Hart, G. R., Roffman, D. A., Decker, R., & Deng, J. (2018). A multi-parameterized
artificial neural network for lung cancer risk prediction. PLOS ONE, 13(10), e0205264.
https://doi.org/10.1371/journal.pone.0205264
(16) Houfani, D., Slatnia, S., Kazar, O., Zerhouni, N., Merizig, A., & Saouli, H. (2020).
Machine Learning Techniques for Breast Cancer Diagnosis: Literature Review. In M.
Ezziyyani (Ed.), Advanced Intelligent Systems for Sustainable Development
(AI2SD’2019) (pp. 247–254). https://doi.org/10.1007/978-3-030-36664-3_28
(17) Huang, C., Clayton, E. A., Matyunina, L. V., McDonald, L. D., Benigno, B. B.,
Vannberg, F., & McDonald, J. F. (2018). Machine learning predicts individual cancer
patient responses to therapeutic drugs with high accuracy. Scientific Reports, 8(1), 1–8.
https://doi.org/10.1038/s41598-018-34753-5
(18) Huang, M.-W., Chen, C.-W., Lin, W.-C., Ke, S.-W., & Tsai, C.-F. (2017). SVM and
SVM Ensembles in Breast Cancer Prediction. PLOS ONE, 12(1), e0161501.
https://doi.org/10.1371/journal.pone.0161501
(19) Huang, S., Cai, N., Pacheco, P. P., Narrandes, S., Wang, Y., & Xu, W. (2018).
Applications of Support Vector Machine (SVM) Learning in Cancer Genomics.
Cancer Genomics - Proteomics, 15(1), 41–51.
(20) Jiang, Y., Xie, J., Han, Z., Liu, W., Xi, S., Huang, L., … Li, G. (2018).
Immunomarker Support Vector Machine Classifier for Prediction of Gastric Cancer
Survival and Adjuvant Chemotherapeutic Benefit. Clinical Cancer Research, 24(22),
5574–5584. https://doi.org/10.1158/1078-0432.CCR-18-0848
Classification Scheme for Predicting SPCs in Breast Cancer Survivors. Frontiers in
Genetics, 10. https://doi.org/10.3389/fgene.2019.00848
(12) Curtis, N. J., Dennison, G., Salib, E., Hashimoto, D. A., & Francis, N. K. (2019).
Artificial Neural Network Individualised Prediction of Time to Colorectal Cancer
Surgery [Research Article]. https://doi.org/10.1155/2019/1285931
(13) Elsayad, A. (2013). Diagnosis of Breast Cancer using Decision Tree Models and
SVM. International Journal of Computer Applications, 83, 19–29.
https://www.researchgate.net/publication/269166287
(14) Gendelman, R., Xing, H., Mirzoeva, O. K., Sarde, P., Curtis, C., Feiler, H. S., …
Korn, W. M. (2017). Bayesian Network Inference Modeling Identifies TRIB1 as a
Novel Regulator of Cell-Cycle Progression and Survival in Cancer Cells. Cancer
Research, 77(7), 1575–1585.
(15) Hart, G. R., Roffman, D. A., Decker, R., & Deng, J. (2018). A multi-parameterized
artificial neural network for lung cancer risk prediction. PLOS ONE, 13(10), e0205264.
https://doi.org/10.1371/journal.pone.0205264
(16) Houfani, D., Slatnia, S., Kazar, O., Zerhouni, N., Merizig, A., & Saouli, H. (2020).
Machine Learning Techniques for Breast Cancer Diagnosis: Literature Review. In M.
Ezziyyani (Ed.), Advanced Intelligent Systems for Sustainable Development
(AI2SD’2019) (pp. 247–254). https://doi.org/10.1007/978-3-030-36664-3_28
(17) Huang, C., Clayton, E. A., Matyunina, L. V., McDonald, L. D., Benigno, B. B.,
Vannberg, F., & McDonald, J. F. (2018). Machine learning predicts individual cancer
patient responses to therapeutic drugs with high accuracy. Scientific Reports, 8(1), 1–8.
https://doi.org/10.1038/s41598-018-34753-5
(18) Huang, M.-W., Chen, C.-W., Lin, W.-C., Ke, S.-W., & Tsai, C.-F. (2017). SVM and
SVM Ensembles in Breast Cancer Prediction. PLOS ONE, 12(1), e0161501.
https://doi.org/10.1371/journal.pone.0161501
(19) Huang, S., Cai, N., Pacheco, P. P., Narrandes, S., Wang, Y., & Xu, W. (2018).
Applications of Support Vector Machine (SVM) Learning in Cancer Genomics.
Cancer Genomics - Proteomics, 15(1), 41–51.
(20) Jiang, Y., Xie, J., Han, Z., Liu, W., Xi, S., Huang, L., … Li, G. (2018).
Immunomarker Support Vector Machine Classifier for Prediction of Gastric Cancer
Survival and Adjuvant Chemotherapeutic Benefit. Clinical Cancer Research, 24(22),
5574–5584. https://doi.org/10.1158/1078-0432.CCR-18-0848
Paraphrase This Document
Need a fresh take? Get an instant paraphrase of this document with our AI Paraphraser

(21) Kamel, S. R., YaghoubZadeh, R., & Kheirabadi, M. (2019). Improving the
performance of support-vector machine by selecting the best features by Gray Wolf
algorithm to increase the accuracy of diagnosis of breast cancer. Journal of Big Data,
6(1), 90. https://doi.org/10.1186/s40537-019-0247-7
(22) Kourou, K., Exarchos, T. P., Exarchos, K. P., Karamouzis, M. V., & Fotiadis, D. I.
(2015). Machine learning applications in cancer prognosis and prediction.
Computational and Structural Biotechnology Journal, 13, 8–17.
https://doi.org/10.1016/j.csbj.2014.11.005
(23) Kumar, M., & Udayan, J. (2019). A Survey of Machine Learning Techniques for
Cancer Disease Prediction and Diagnosis. Indian Journal of Public Health Research &
Development, 10, 157. https://doi.org/10.5958/0976-5506.2019.00682.X
(24) Linda, W., Hosny, A., Schabath, M. B., Giger, M. L., Birkbak, N. J., Mehrtash, A., …
Aerts, H. J. W. L. (2019). Artificial intelligence in cancer imaging: Clinical challenges
and applications. CA: A Cancer Journal for Clinicians, 69(2), 127–157.
https://doi.org/10.3322/caac.21552
(25) Liu, N., Shen, J., Xu, M., Gan, D., Qi, E.-S., & Gao, B. (2018). Improved Cost-
Sensitive Support Vector Machine Classifier for Breast Cancer Diagnosis [Research
Article]. https://doi.org/10.1155/2018/3875082
(26) McCabe, R. M., Grutsch, J. F., Braun, D. P., & Nutakki, S. B. (2015). Fatigue as a
Driver of Overall Quality of Life in Cancer Patients. PLOS ONE, 10(6), e0130023.
https://doi.org/10.1371/journal.pone.0130023
(27) Muhammad, W., Hart, G. R., Nartowt, B., Farrell, J. J., Johung, K., Liang, Y., &
Deng, J. (2019). Pancreatic Cancer Prediction Through an Artificial Neural Network.
Frontiers in Artificial Intelligence, 2. https://doi.org/10.3389/frai.2019.00002
(28) Munir, K., Elahi, H., Ayub, A., Frezza, F., & Rizzi, A. (2019). Cancer Diagnosis
Using Deep Learning: A Bibliographic Review. Cancers, 11(9), 1235.
https://doi.org/10.3390/cancers11091235
(29) Nindrea, R. D., Aryandono, T., Lazuardi, L., & Dwiprahasto, I. (2018). Diagnostic
Accuracy of Different Machine Learning Algorithms for Breast Cancer Risk
Calculation: A Meta-Analysis. Asian Pacific Journal of Cancer Prevention : APJCP,
19(7), 1747–1752. https://doi.org/10.22034/APJCP.2018.19.7.1747
(30) Omondiagbe, D. A., Veeramani, S., & Sidhu, A. S. (2019). Machine Learning
Classification Techniques for Breast Cancer Diagnosis. IOP Conference Series:
performance of support-vector machine by selecting the best features by Gray Wolf
algorithm to increase the accuracy of diagnosis of breast cancer. Journal of Big Data,
6(1), 90. https://doi.org/10.1186/s40537-019-0247-7
(22) Kourou, K., Exarchos, T. P., Exarchos, K. P., Karamouzis, M. V., & Fotiadis, D. I.
(2015). Machine learning applications in cancer prognosis and prediction.
Computational and Structural Biotechnology Journal, 13, 8–17.
https://doi.org/10.1016/j.csbj.2014.11.005
(23) Kumar, M., & Udayan, J. (2019). A Survey of Machine Learning Techniques for
Cancer Disease Prediction and Diagnosis. Indian Journal of Public Health Research &
Development, 10, 157. https://doi.org/10.5958/0976-5506.2019.00682.X
(24) Linda, W., Hosny, A., Schabath, M. B., Giger, M. L., Birkbak, N. J., Mehrtash, A., …
Aerts, H. J. W. L. (2019). Artificial intelligence in cancer imaging: Clinical challenges
and applications. CA: A Cancer Journal for Clinicians, 69(2), 127–157.
https://doi.org/10.3322/caac.21552
(25) Liu, N., Shen, J., Xu, M., Gan, D., Qi, E.-S., & Gao, B. (2018). Improved Cost-
Sensitive Support Vector Machine Classifier for Breast Cancer Diagnosis [Research
Article]. https://doi.org/10.1155/2018/3875082
(26) McCabe, R. M., Grutsch, J. F., Braun, D. P., & Nutakki, S. B. (2015). Fatigue as a
Driver of Overall Quality of Life in Cancer Patients. PLOS ONE, 10(6), e0130023.
https://doi.org/10.1371/journal.pone.0130023
(27) Muhammad, W., Hart, G. R., Nartowt, B., Farrell, J. J., Johung, K., Liang, Y., &
Deng, J. (2019). Pancreatic Cancer Prediction Through an Artificial Neural Network.
Frontiers in Artificial Intelligence, 2. https://doi.org/10.3389/frai.2019.00002
(28) Munir, K., Elahi, H., Ayub, A., Frezza, F., & Rizzi, A. (2019). Cancer Diagnosis
Using Deep Learning: A Bibliographic Review. Cancers, 11(9), 1235.
https://doi.org/10.3390/cancers11091235
(29) Nindrea, R. D., Aryandono, T., Lazuardi, L., & Dwiprahasto, I. (2018). Diagnostic
Accuracy of Different Machine Learning Algorithms for Breast Cancer Risk
Calculation: A Meta-Analysis. Asian Pacific Journal of Cancer Prevention : APJCP,
19(7), 1747–1752. https://doi.org/10.22034/APJCP.2018.19.7.1747
(30) Omondiagbe, D. A., Veeramani, S., & Sidhu, A. S. (2019). Machine Learning
Classification Techniques for Breast Cancer Diagnosis. IOP Conference Series:
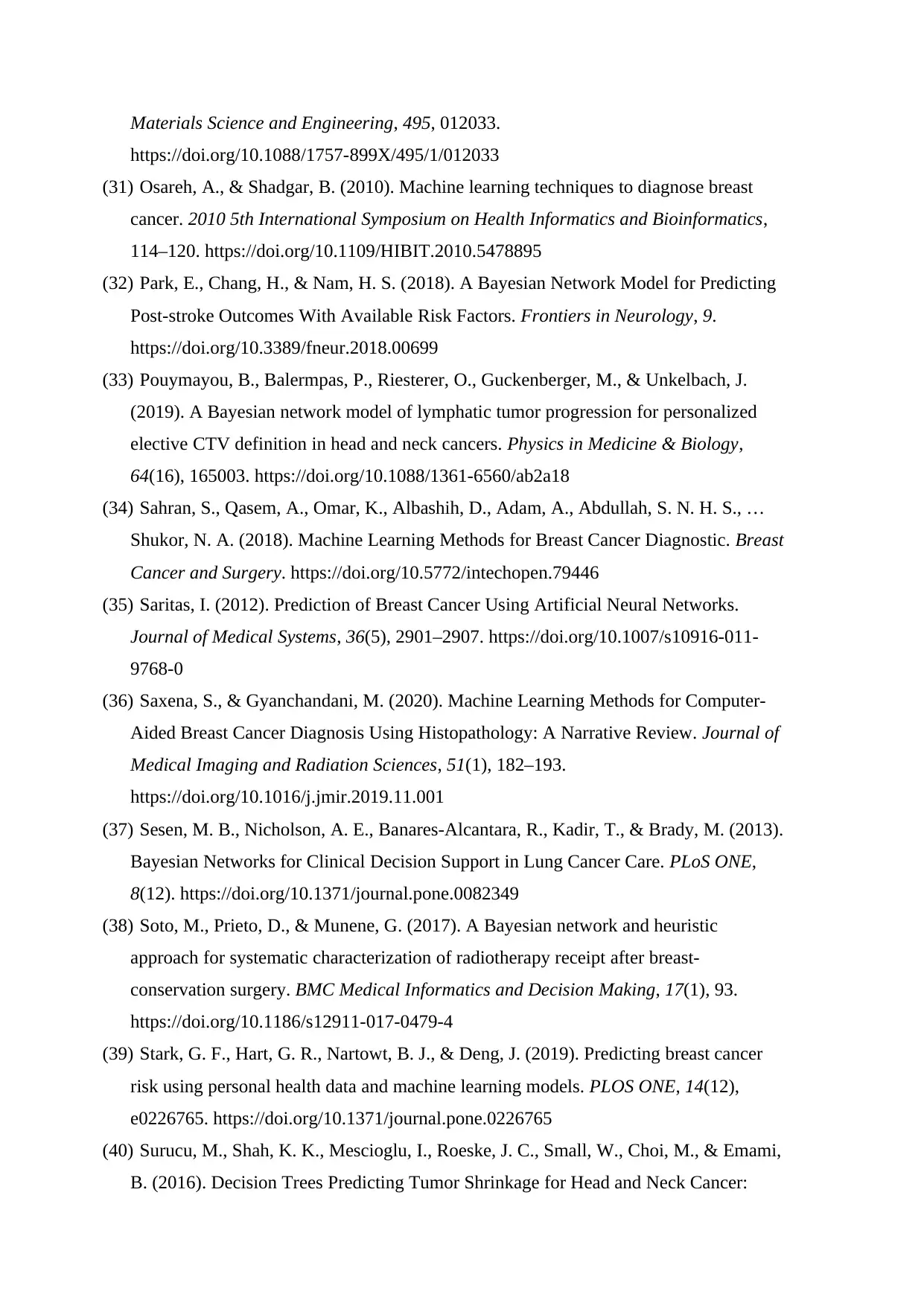
Materials Science and Engineering, 495, 012033.
https://doi.org/10.1088/1757-899X/495/1/012033
(31) Osareh, A., & Shadgar, B. (2010). Machine learning techniques to diagnose breast
cancer. 2010 5th International Symposium on Health Informatics and Bioinformatics,
114–120. https://doi.org/10.1109/HIBIT.2010.5478895
(32) Park, E., Chang, H., & Nam, H. S. (2018). A Bayesian Network Model for Predicting
Post-stroke Outcomes With Available Risk Factors. Frontiers in Neurology, 9.
https://doi.org/10.3389/fneur.2018.00699
(33) Pouymayou, B., Balermpas, P., Riesterer, O., Guckenberger, M., & Unkelbach, J.
(2019). A Bayesian network model of lymphatic tumor progression for personalized
elective CTV definition in head and neck cancers. Physics in Medicine & Biology,
64(16), 165003. https://doi.org/10.1088/1361-6560/ab2a18
(34) Sahran, S., Qasem, A., Omar, K., Albashih, D., Adam, A., Abdullah, S. N. H. S., …
Shukor, N. A. (2018). Machine Learning Methods for Breast Cancer Diagnostic. Breast
Cancer and Surgery. https://doi.org/10.5772/intechopen.79446
(35) Saritas, I. (2012). Prediction of Breast Cancer Using Artificial Neural Networks.
Journal of Medical Systems, 36(5), 2901–2907. https://doi.org/10.1007/s10916-011-
9768-0
(36) Saxena, S., & Gyanchandani, M. (2020). Machine Learning Methods for Computer-
Aided Breast Cancer Diagnosis Using Histopathology: A Narrative Review. Journal of
Medical Imaging and Radiation Sciences, 51(1), 182–193.
https://doi.org/10.1016/j.jmir.2019.11.001
(37) Sesen, M. B., Nicholson, A. E., Banares-Alcantara, R., Kadir, T., & Brady, M. (2013).
Bayesian Networks for Clinical Decision Support in Lung Cancer Care. PLoS ONE,
8(12). https://doi.org/10.1371/journal.pone.0082349
(38) Soto, M., Prieto, D., & Munene, G. (2017). A Bayesian network and heuristic
approach for systematic characterization of radiotherapy receipt after breast-
conservation surgery. BMC Medical Informatics and Decision Making, 17(1), 93.
https://doi.org/10.1186/s12911-017-0479-4
(39) Stark, G. F., Hart, G. R., Nartowt, B. J., & Deng, J. (2019). Predicting breast cancer
risk using personal health data and machine learning models. PLOS ONE, 14(12),
e0226765. https://doi.org/10.1371/journal.pone.0226765
(40) Surucu, M., Shah, K. K., Mescioglu, I., Roeske, J. C., Small, W., Choi, M., & Emami,
B. (2016). Decision Trees Predicting Tumor Shrinkage for Head and Neck Cancer:
https://doi.org/10.1088/1757-899X/495/1/012033
(31) Osareh, A., & Shadgar, B. (2010). Machine learning techniques to diagnose breast
cancer. 2010 5th International Symposium on Health Informatics and Bioinformatics,
114–120. https://doi.org/10.1109/HIBIT.2010.5478895
(32) Park, E., Chang, H., & Nam, H. S. (2018). A Bayesian Network Model for Predicting
Post-stroke Outcomes With Available Risk Factors. Frontiers in Neurology, 9.
https://doi.org/10.3389/fneur.2018.00699
(33) Pouymayou, B., Balermpas, P., Riesterer, O., Guckenberger, M., & Unkelbach, J.
(2019). A Bayesian network model of lymphatic tumor progression for personalized
elective CTV definition in head and neck cancers. Physics in Medicine & Biology,
64(16), 165003. https://doi.org/10.1088/1361-6560/ab2a18
(34) Sahran, S., Qasem, A., Omar, K., Albashih, D., Adam, A., Abdullah, S. N. H. S., …
Shukor, N. A. (2018). Machine Learning Methods for Breast Cancer Diagnostic. Breast
Cancer and Surgery. https://doi.org/10.5772/intechopen.79446
(35) Saritas, I. (2012). Prediction of Breast Cancer Using Artificial Neural Networks.
Journal of Medical Systems, 36(5), 2901–2907. https://doi.org/10.1007/s10916-011-
9768-0
(36) Saxena, S., & Gyanchandani, M. (2020). Machine Learning Methods for Computer-
Aided Breast Cancer Diagnosis Using Histopathology: A Narrative Review. Journal of
Medical Imaging and Radiation Sciences, 51(1), 182–193.
https://doi.org/10.1016/j.jmir.2019.11.001
(37) Sesen, M. B., Nicholson, A. E., Banares-Alcantara, R., Kadir, T., & Brady, M. (2013).
Bayesian Networks for Clinical Decision Support in Lung Cancer Care. PLoS ONE,
8(12). https://doi.org/10.1371/journal.pone.0082349
(38) Soto, M., Prieto, D., & Munene, G. (2017). A Bayesian network and heuristic
approach for systematic characterization of radiotherapy receipt after breast-
conservation surgery. BMC Medical Informatics and Decision Making, 17(1), 93.
https://doi.org/10.1186/s12911-017-0479-4
(39) Stark, G. F., Hart, G. R., Nartowt, B. J., & Deng, J. (2019). Predicting breast cancer
risk using personal health data and machine learning models. PLOS ONE, 14(12),
e0226765. https://doi.org/10.1371/journal.pone.0226765
(40) Surucu, M., Shah, K. K., Mescioglu, I., Roeske, J. C., Small, W., Choi, M., & Emami,
B. (2016). Decision Trees Predicting Tumor Shrinkage for Head and Neck Cancer:
⊘ This is a preview!⊘
Do you want full access?
Subscribe today to unlock all pages.

Trusted by 1+ million students worldwide
1 out of 13