Clinical Treatment University of Bristol Project 2022
VerifiedAdded on 2022/09/18
|72
|24997
|29
AI Summary
Contribute Materials
Your contribution can guide someone’s learning journey. Share your
documents today.
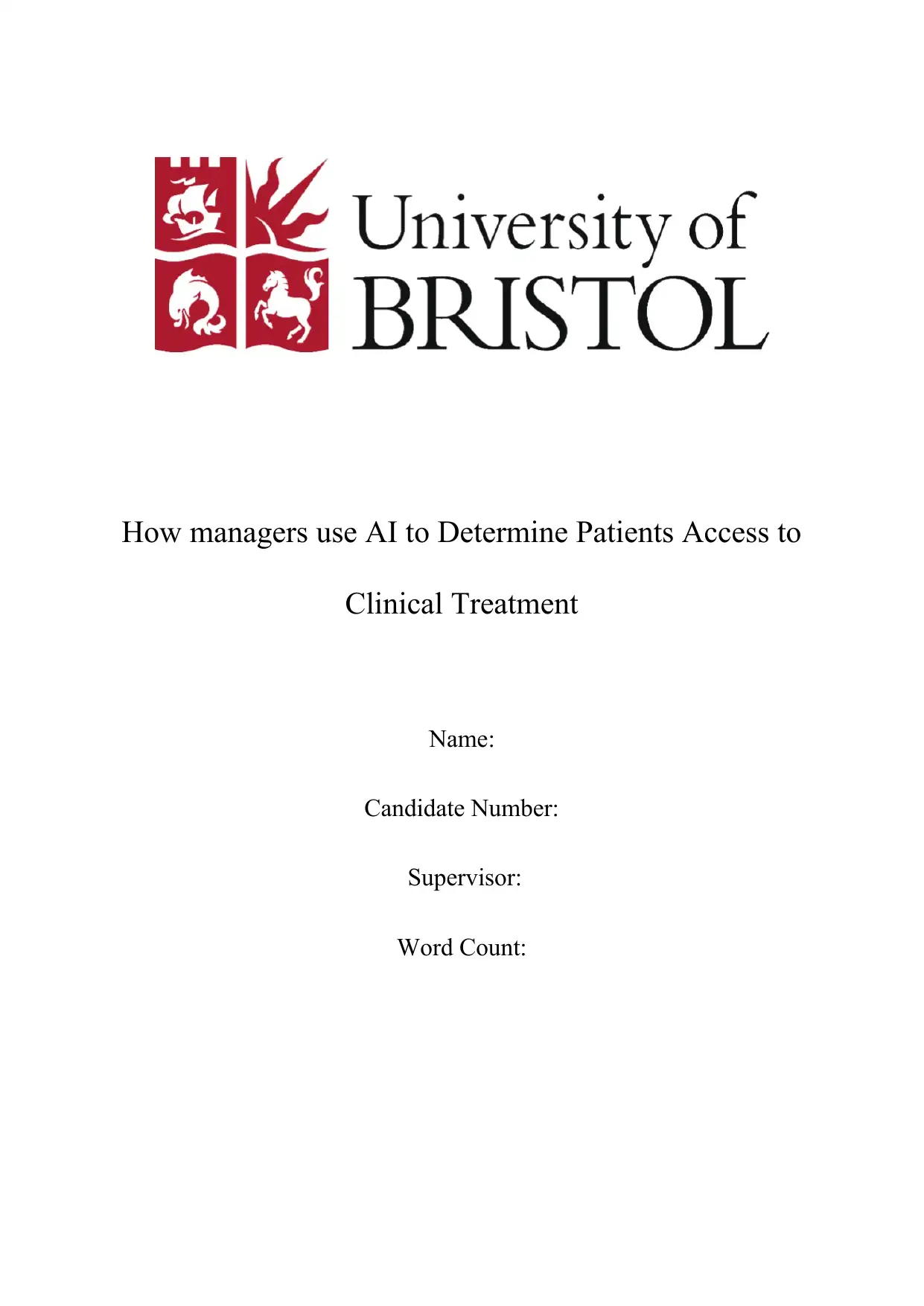
How managers use AI to Determine Patients Access to
Clinical Treatment
Name:
Candidate Number:
Supervisor:
Word Count:
Clinical Treatment
Name:
Candidate Number:
Supervisor:
Word Count:
Secure Best Marks with AI Grader
Need help grading? Try our AI Grader for instant feedback on your assignments.
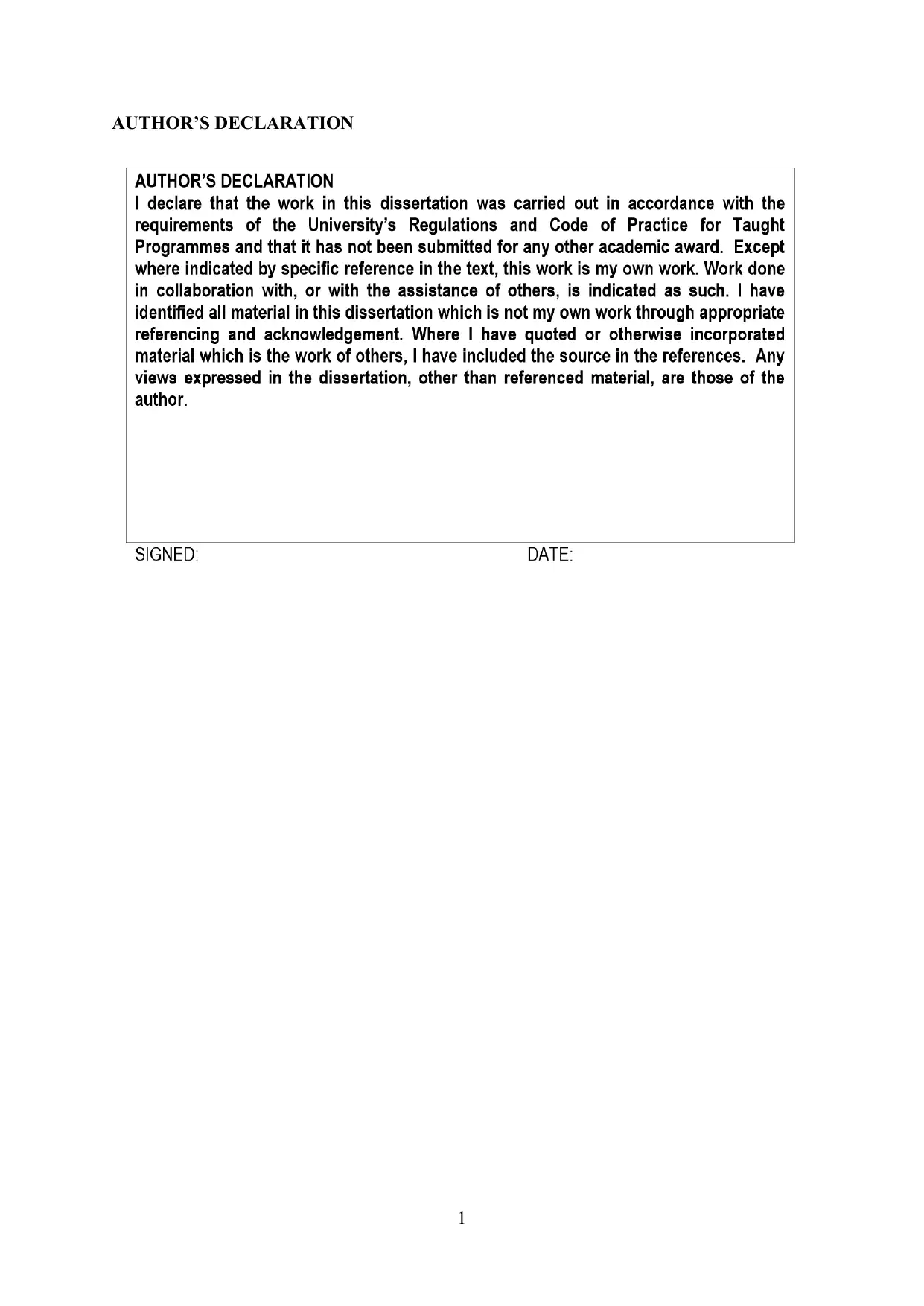
AUTHOR’S DECLARATION
1
1
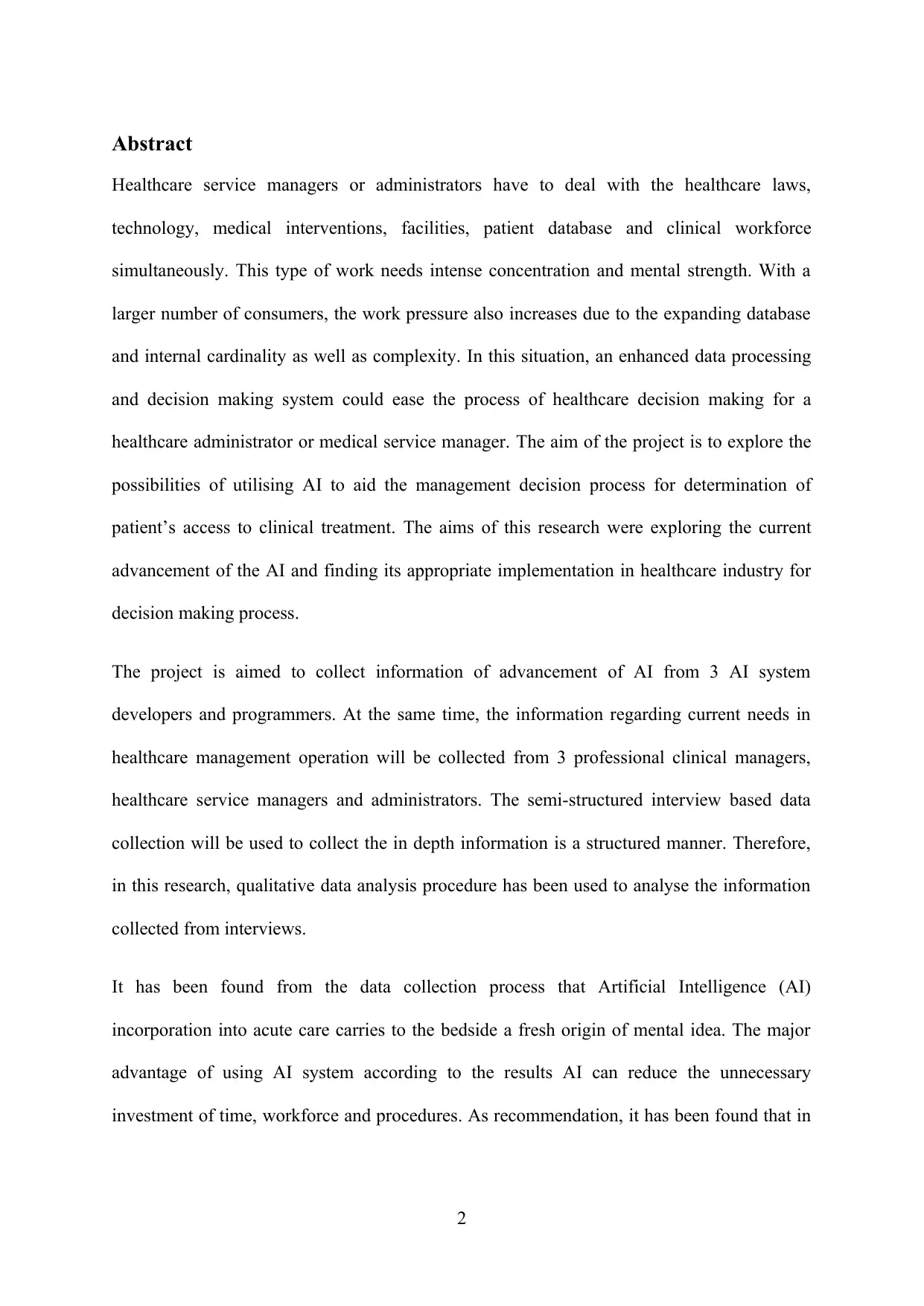
Abstract
Healthcare service managers or administrators have to deal with the healthcare laws,
technology, medical interventions, facilities, patient database and clinical workforce
simultaneously. This type of work needs intense concentration and mental strength. With a
larger number of consumers, the work pressure also increases due to the expanding database
and internal cardinality as well as complexity. In this situation, an enhanced data processing
and decision making system could ease the process of healthcare decision making for a
healthcare administrator or medical service manager. The aim of the project is to explore the
possibilities of utilising AI to aid the management decision process for determination of
patient’s access to clinical treatment. The aims of this research were exploring the current
advancement of the AI and finding its appropriate implementation in healthcare industry for
decision making process.
The project is aimed to collect information of advancement of AI from 3 AI system
developers and programmers. At the same time, the information regarding current needs in
healthcare management operation will be collected from 3 professional clinical managers,
healthcare service managers and administrators. The semi-structured interview based data
collection will be used to collect the in depth information is a structured manner. Therefore,
in this research, qualitative data analysis procedure has been used to analyse the information
collected from interviews.
It has been found from the data collection process that Artificial Intelligence (AI)
incorporation into acute care carries to the bedside a fresh origin of mental idea. The major
advantage of using AI system according to the results AI can reduce the unnecessary
investment of time, workforce and procedures. As recommendation, it has been found that in
2
Healthcare service managers or administrators have to deal with the healthcare laws,
technology, medical interventions, facilities, patient database and clinical workforce
simultaneously. This type of work needs intense concentration and mental strength. With a
larger number of consumers, the work pressure also increases due to the expanding database
and internal cardinality as well as complexity. In this situation, an enhanced data processing
and decision making system could ease the process of healthcare decision making for a
healthcare administrator or medical service manager. The aim of the project is to explore the
possibilities of utilising AI to aid the management decision process for determination of
patient’s access to clinical treatment. The aims of this research were exploring the current
advancement of the AI and finding its appropriate implementation in healthcare industry for
decision making process.
The project is aimed to collect information of advancement of AI from 3 AI system
developers and programmers. At the same time, the information regarding current needs in
healthcare management operation will be collected from 3 professional clinical managers,
healthcare service managers and administrators. The semi-structured interview based data
collection will be used to collect the in depth information is a structured manner. Therefore,
in this research, qualitative data analysis procedure has been used to analyse the information
collected from interviews.
It has been found from the data collection process that Artificial Intelligence (AI)
incorporation into acute care carries to the bedside a fresh origin of mental idea. The major
advantage of using AI system according to the results AI can reduce the unnecessary
investment of time, workforce and procedures. As recommendation, it has been found that in
2
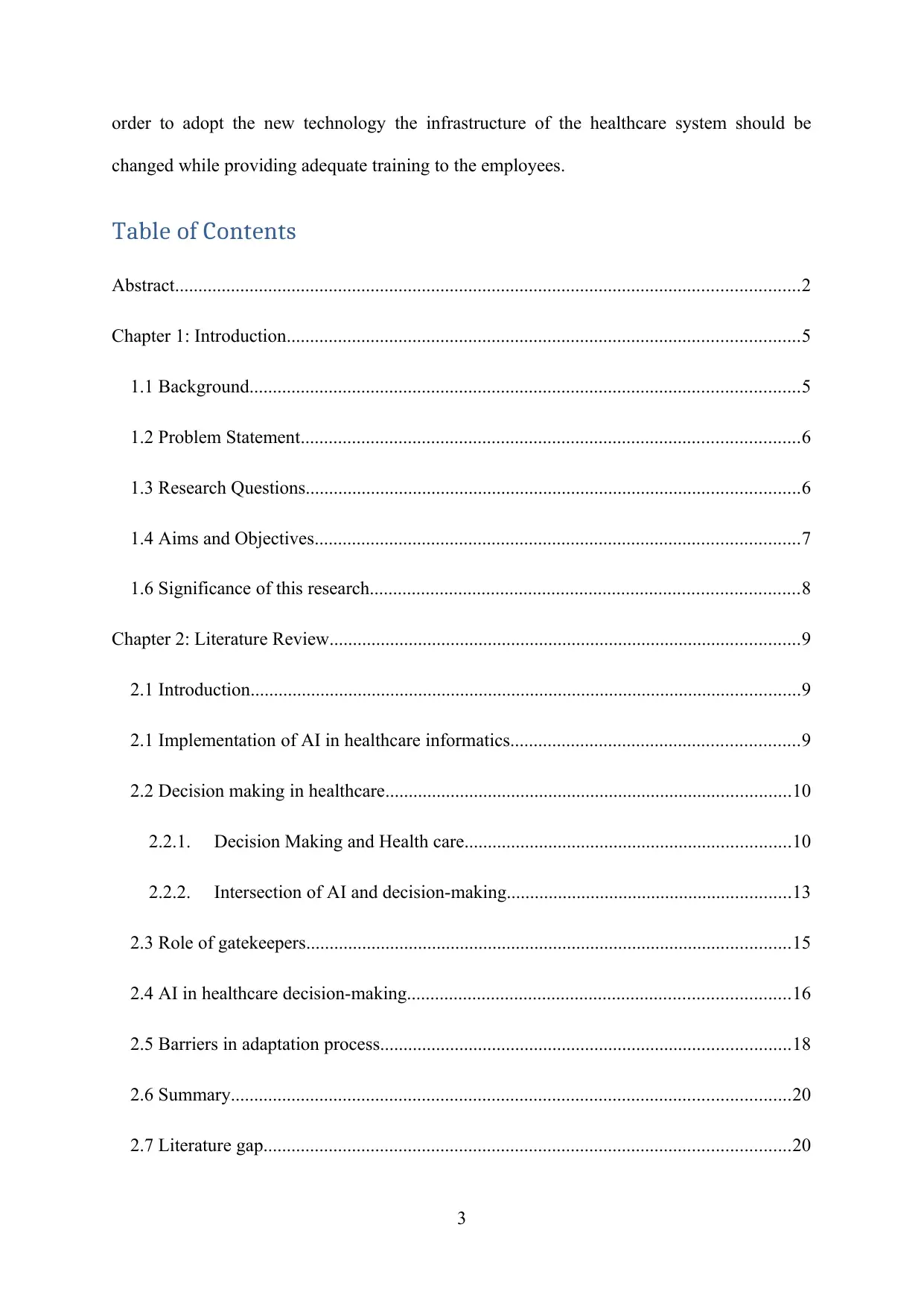
order to adopt the new technology the infrastructure of the healthcare system should be
changed while providing adequate training to the employees.
Table of Contents
Abstract......................................................................................................................................2
Chapter 1: Introduction..............................................................................................................5
1.1 Background......................................................................................................................5
1.2 Problem Statement...........................................................................................................6
1.3 Research Questions..........................................................................................................6
1.4 Aims and Objectives........................................................................................................7
1.6 Significance of this research............................................................................................8
Chapter 2: Literature Review.....................................................................................................9
2.1 Introduction......................................................................................................................9
2.1 Implementation of AI in healthcare informatics..............................................................9
2.2 Decision making in healthcare.......................................................................................10
2.2.1. Decision Making and Health care......................................................................10
2.2.2. Intersection of AI and decision-making.............................................................13
2.3 Role of gatekeepers........................................................................................................15
2.4 AI in healthcare decision-making..................................................................................16
2.5 Barriers in adaptation process........................................................................................18
2.6 Summary........................................................................................................................20
2.7 Literature gap.................................................................................................................20
3
changed while providing adequate training to the employees.
Table of Contents
Abstract......................................................................................................................................2
Chapter 1: Introduction..............................................................................................................5
1.1 Background......................................................................................................................5
1.2 Problem Statement...........................................................................................................6
1.3 Research Questions..........................................................................................................6
1.4 Aims and Objectives........................................................................................................7
1.6 Significance of this research............................................................................................8
Chapter 2: Literature Review.....................................................................................................9
2.1 Introduction......................................................................................................................9
2.1 Implementation of AI in healthcare informatics..............................................................9
2.2 Decision making in healthcare.......................................................................................10
2.2.1. Decision Making and Health care......................................................................10
2.2.2. Intersection of AI and decision-making.............................................................13
2.3 Role of gatekeepers........................................................................................................15
2.4 AI in healthcare decision-making..................................................................................16
2.5 Barriers in adaptation process........................................................................................18
2.6 Summary........................................................................................................................20
2.7 Literature gap.................................................................................................................20
3
Secure Best Marks with AI Grader
Need help grading? Try our AI Grader for instant feedback on your assignments.
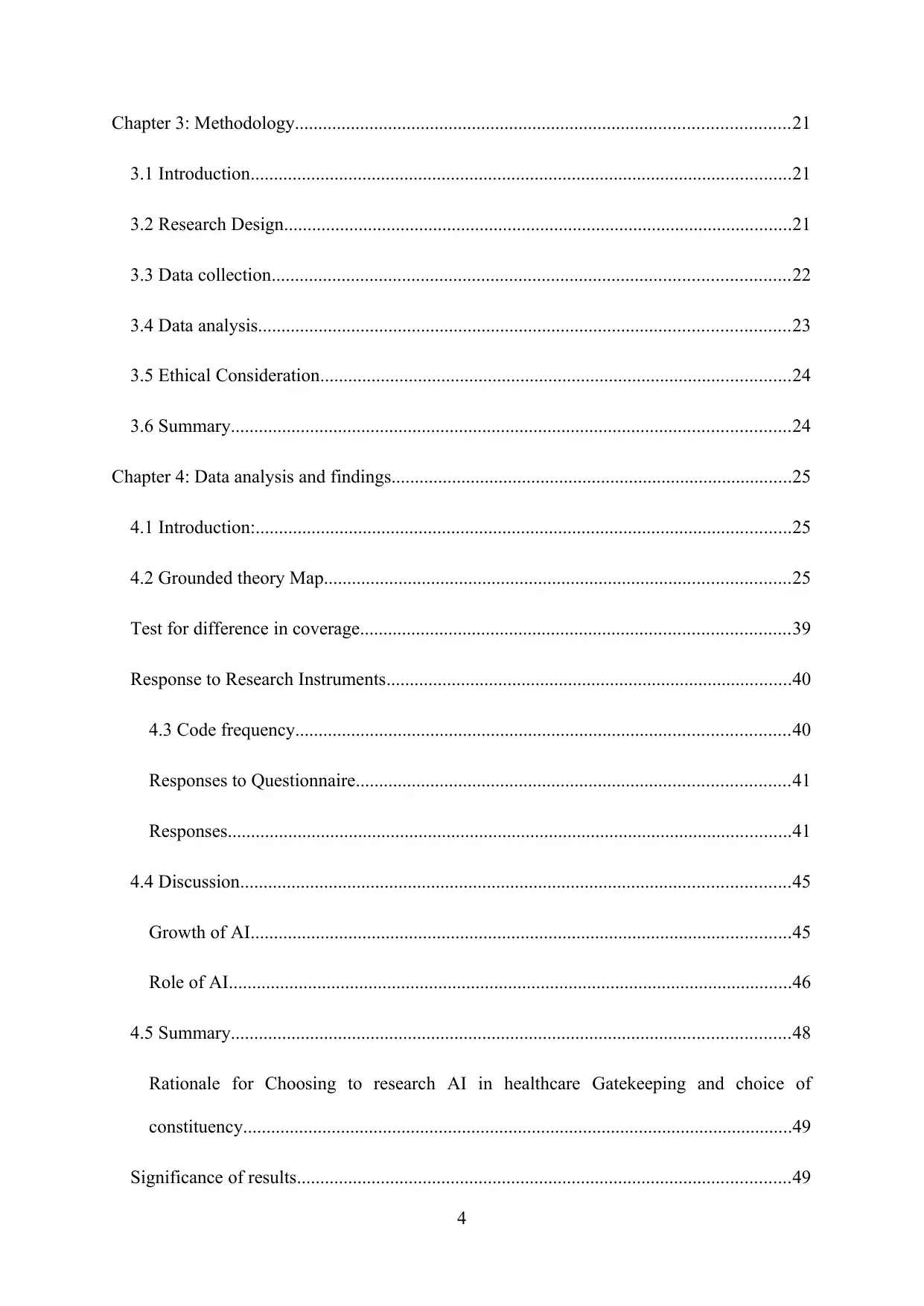
Chapter 3: Methodology..........................................................................................................21
3.1 Introduction....................................................................................................................21
3.2 Research Design.............................................................................................................21
3.3 Data collection...............................................................................................................22
3.4 Data analysis..................................................................................................................23
3.5 Ethical Consideration.....................................................................................................24
3.6 Summary........................................................................................................................24
Chapter 4: Data analysis and findings......................................................................................25
4.1 Introduction:...................................................................................................................25
4.2 Grounded theory Map....................................................................................................25
Test for difference in coverage............................................................................................39
Response to Research Instruments.......................................................................................40
4.3 Code frequency..........................................................................................................40
Responses to Questionnaire.............................................................................................41
Responses.........................................................................................................................41
4.4 Discussion......................................................................................................................45
Growth of AI....................................................................................................................45
Role of AI.........................................................................................................................46
4.5 Summary........................................................................................................................48
Rationale for Choosing to research AI in healthcare Gatekeeping and choice of
constituency......................................................................................................................49
Significance of results..........................................................................................................49
4
3.1 Introduction....................................................................................................................21
3.2 Research Design.............................................................................................................21
3.3 Data collection...............................................................................................................22
3.4 Data analysis..................................................................................................................23
3.5 Ethical Consideration.....................................................................................................24
3.6 Summary........................................................................................................................24
Chapter 4: Data analysis and findings......................................................................................25
4.1 Introduction:...................................................................................................................25
4.2 Grounded theory Map....................................................................................................25
Test for difference in coverage............................................................................................39
Response to Research Instruments.......................................................................................40
4.3 Code frequency..........................................................................................................40
Responses to Questionnaire.............................................................................................41
Responses.........................................................................................................................41
4.4 Discussion......................................................................................................................45
Growth of AI....................................................................................................................45
Role of AI.........................................................................................................................46
4.5 Summary........................................................................................................................48
Rationale for Choosing to research AI in healthcare Gatekeeping and choice of
constituency......................................................................................................................49
Significance of results..........................................................................................................49
4
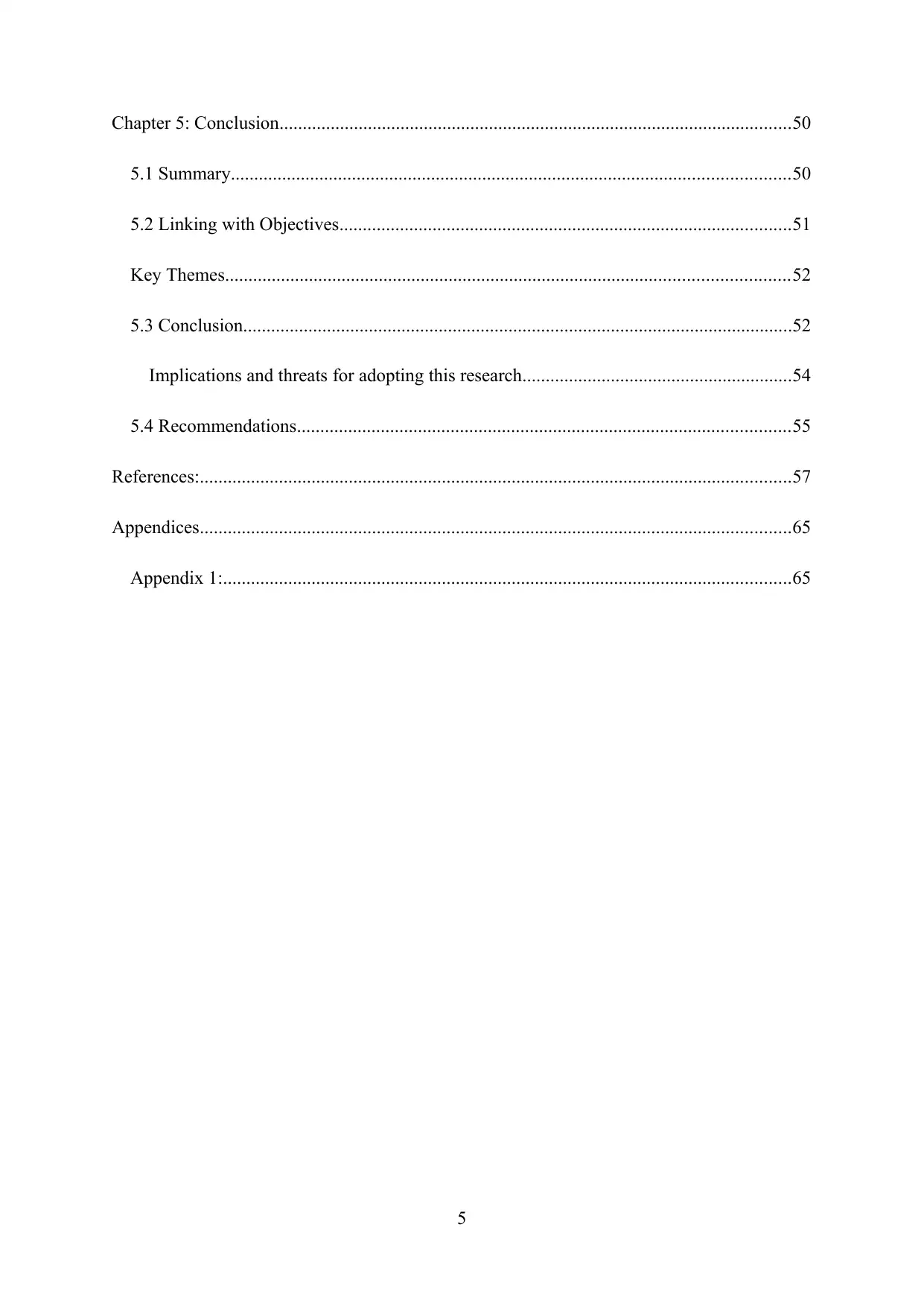
Chapter 5: Conclusion..............................................................................................................50
5.1 Summary........................................................................................................................50
5.2 Linking with Objectives.................................................................................................51
Key Themes.........................................................................................................................52
5.3 Conclusion......................................................................................................................52
Implications and threats for adopting this research..........................................................54
5.4 Recommendations..........................................................................................................55
References:...............................................................................................................................57
Appendices...............................................................................................................................65
Appendix 1:..........................................................................................................................65
5
5.1 Summary........................................................................................................................50
5.2 Linking with Objectives.................................................................................................51
Key Themes.........................................................................................................................52
5.3 Conclusion......................................................................................................................52
Implications and threats for adopting this research..........................................................54
5.4 Recommendations..........................................................................................................55
References:...............................................................................................................................57
Appendices...............................................................................................................................65
Appendix 1:..........................................................................................................................65
5
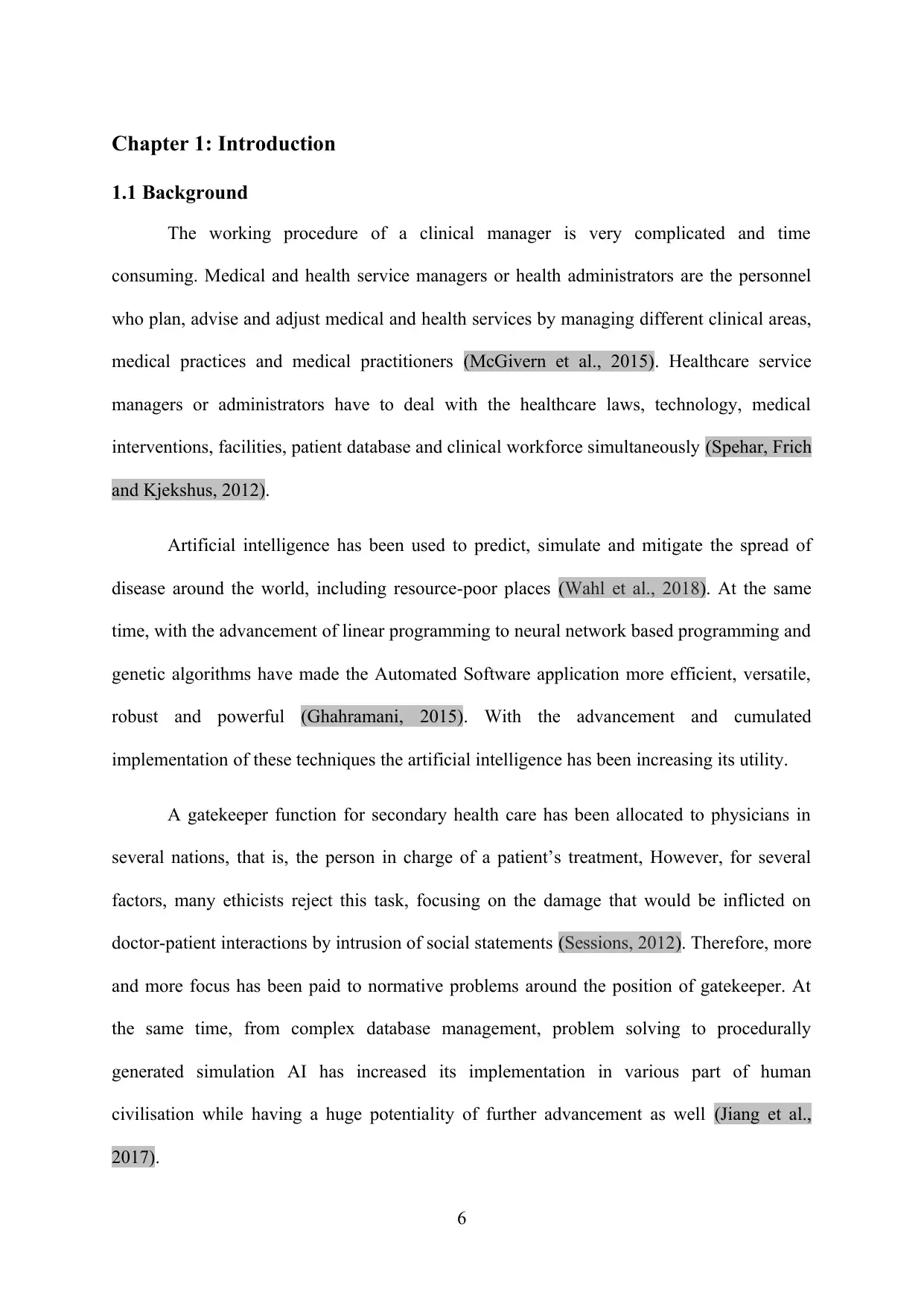
Chapter 1: Introduction
1.1 Background
The working procedure of a clinical manager is very complicated and time
consuming. Medical and health service managers or health administrators are the personnel
who plan, advise and adjust medical and health services by managing different clinical areas,
medical practices and medical practitioners (McGivern et al., 2015). Healthcare service
managers or administrators have to deal with the healthcare laws, technology, medical
interventions, facilities, patient database and clinical workforce simultaneously (Spehar, Frich
and Kjekshus, 2012).
Artificial intelligence has been used to predict, simulate and mitigate the spread of
disease around the world, including resource-poor places (Wahl et al., 2018). At the same
time, with the advancement of linear programming to neural network based programming and
genetic algorithms have made the Automated Software application more efficient, versatile,
robust and powerful (Ghahramani, 2015). With the advancement and cumulated
implementation of these techniques the artificial intelligence has been increasing its utility.
A gatekeeper function for secondary health care has been allocated to physicians in
several nations, that is, the person in charge of a patient’s treatment, However, for several
factors, many ethicists reject this task, focusing on the damage that would be inflicted on
doctor-patient interactions by intrusion of social statements (Sessions, 2012). Therefore, more
and more focus has been paid to normative problems around the position of gatekeeper. At
the same time, from complex database management, problem solving to procedurally
generated simulation AI has increased its implementation in various part of human
civilisation while having a huge potentiality of further advancement as well (Jiang et al.,
2017).
6
1.1 Background
The working procedure of a clinical manager is very complicated and time
consuming. Medical and health service managers or health administrators are the personnel
who plan, advise and adjust medical and health services by managing different clinical areas,
medical practices and medical practitioners (McGivern et al., 2015). Healthcare service
managers or administrators have to deal with the healthcare laws, technology, medical
interventions, facilities, patient database and clinical workforce simultaneously (Spehar, Frich
and Kjekshus, 2012).
Artificial intelligence has been used to predict, simulate and mitigate the spread of
disease around the world, including resource-poor places (Wahl et al., 2018). At the same
time, with the advancement of linear programming to neural network based programming and
genetic algorithms have made the Automated Software application more efficient, versatile,
robust and powerful (Ghahramani, 2015). With the advancement and cumulated
implementation of these techniques the artificial intelligence has been increasing its utility.
A gatekeeper function for secondary health care has been allocated to physicians in
several nations, that is, the person in charge of a patient’s treatment, However, for several
factors, many ethicists reject this task, focusing on the damage that would be inflicted on
doctor-patient interactions by intrusion of social statements (Sessions, 2012). Therefore, more
and more focus has been paid to normative problems around the position of gatekeeper. At
the same time, from complex database management, problem solving to procedurally
generated simulation AI has increased its implementation in various part of human
civilisation while having a huge potentiality of further advancement as well (Jiang et al.,
2017).
6
Paraphrase This Document
Need a fresh take? Get an instant paraphrase of this document with our AI Paraphraser
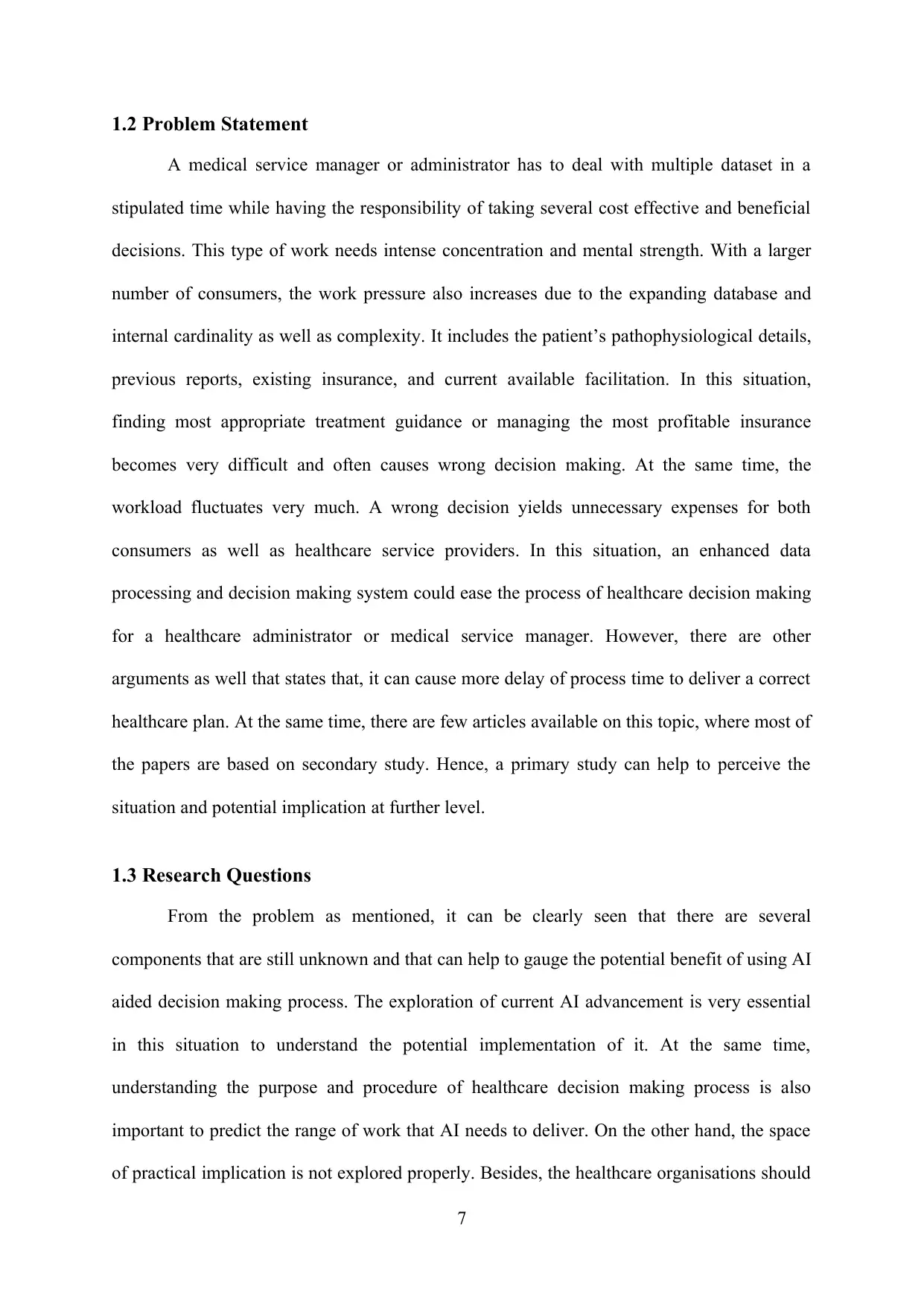
1.2 Problem Statement
A medical service manager or administrator has to deal with multiple dataset in a
stipulated time while having the responsibility of taking several cost effective and beneficial
decisions. This type of work needs intense concentration and mental strength. With a larger
number of consumers, the work pressure also increases due to the expanding database and
internal cardinality as well as complexity. It includes the patient’s pathophysiological details,
previous reports, existing insurance, and current available facilitation. In this situation,
finding most appropriate treatment guidance or managing the most profitable insurance
becomes very difficult and often causes wrong decision making. At the same time, the
workload fluctuates very much. A wrong decision yields unnecessary expenses for both
consumers as well as healthcare service providers. In this situation, an enhanced data
processing and decision making system could ease the process of healthcare decision making
for a healthcare administrator or medical service manager. However, there are other
arguments as well that states that, it can cause more delay of process time to deliver a correct
healthcare plan. At the same time, there are few articles available on this topic, where most of
the papers are based on secondary study. Hence, a primary study can help to perceive the
situation and potential implication at further level.
1.3 Research Questions
From the problem as mentioned, it can be clearly seen that there are several
components that are still unknown and that can help to gauge the potential benefit of using AI
aided decision making process. The exploration of current AI advancement is very essential
in this situation to understand the potential implementation of it. At the same time,
understanding the purpose and procedure of healthcare decision making process is also
important to predict the range of work that AI needs to deliver. On the other hand, the space
of practical implication is not explored properly. Besides, the healthcare organisations should
7
A medical service manager or administrator has to deal with multiple dataset in a
stipulated time while having the responsibility of taking several cost effective and beneficial
decisions. This type of work needs intense concentration and mental strength. With a larger
number of consumers, the work pressure also increases due to the expanding database and
internal cardinality as well as complexity. It includes the patient’s pathophysiological details,
previous reports, existing insurance, and current available facilitation. In this situation,
finding most appropriate treatment guidance or managing the most profitable insurance
becomes very difficult and often causes wrong decision making. At the same time, the
workload fluctuates very much. A wrong decision yields unnecessary expenses for both
consumers as well as healthcare service providers. In this situation, an enhanced data
processing and decision making system could ease the process of healthcare decision making
for a healthcare administrator or medical service manager. However, there are other
arguments as well that states that, it can cause more delay of process time to deliver a correct
healthcare plan. At the same time, there are few articles available on this topic, where most of
the papers are based on secondary study. Hence, a primary study can help to perceive the
situation and potential implication at further level.
1.3 Research Questions
From the problem as mentioned, it can be clearly seen that there are several
components that are still unknown and that can help to gauge the potential benefit of using AI
aided decision making process. The exploration of current AI advancement is very essential
in this situation to understand the potential implementation of it. At the same time,
understanding the purpose and procedure of healthcare decision making process is also
important to predict the range of work that AI needs to deliver. On the other hand, the space
of practical implication is not explored properly. Besides, the healthcare organisations should
7
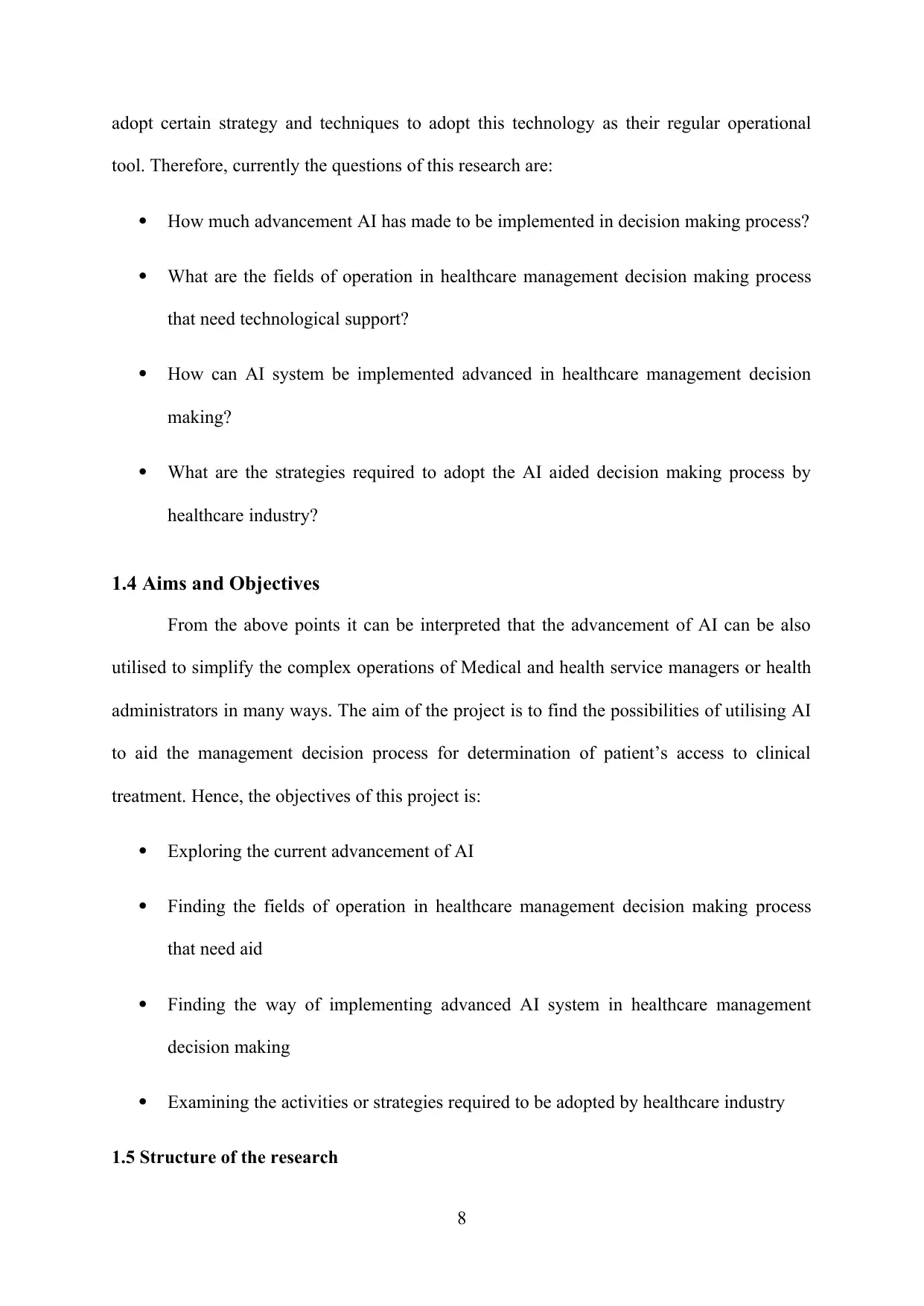
adopt certain strategy and techniques to adopt this technology as their regular operational
tool. Therefore, currently the questions of this research are:
How much advancement AI has made to be implemented in decision making process?
What are the fields of operation in healthcare management decision making process
that need technological support?
How can AI system be implemented advanced in healthcare management decision
making?
What are the strategies required to adopt the AI aided decision making process by
healthcare industry?
1.4 Aims and Objectives
From the above points it can be interpreted that the advancement of AI can be also
utilised to simplify the complex operations of Medical and health service managers or health
administrators in many ways. The aim of the project is to find the possibilities of utilising AI
to aid the management decision process for determination of patient’s access to clinical
treatment. Hence, the objectives of this project is:
Exploring the current advancement of AI
Finding the fields of operation in healthcare management decision making process
that need aid
Finding the way of implementing advanced AI system in healthcare management
decision making
Examining the activities or strategies required to be adopted by healthcare industry
1.5 Structure of the research
8
tool. Therefore, currently the questions of this research are:
How much advancement AI has made to be implemented in decision making process?
What are the fields of operation in healthcare management decision making process
that need technological support?
How can AI system be implemented advanced in healthcare management decision
making?
What are the strategies required to adopt the AI aided decision making process by
healthcare industry?
1.4 Aims and Objectives
From the above points it can be interpreted that the advancement of AI can be also
utilised to simplify the complex operations of Medical and health service managers or health
administrators in many ways. The aim of the project is to find the possibilities of utilising AI
to aid the management decision process for determination of patient’s access to clinical
treatment. Hence, the objectives of this project is:
Exploring the current advancement of AI
Finding the fields of operation in healthcare management decision making process
that need aid
Finding the way of implementing advanced AI system in healthcare management
decision making
Examining the activities or strategies required to be adopted by healthcare industry
1.5 Structure of the research
8
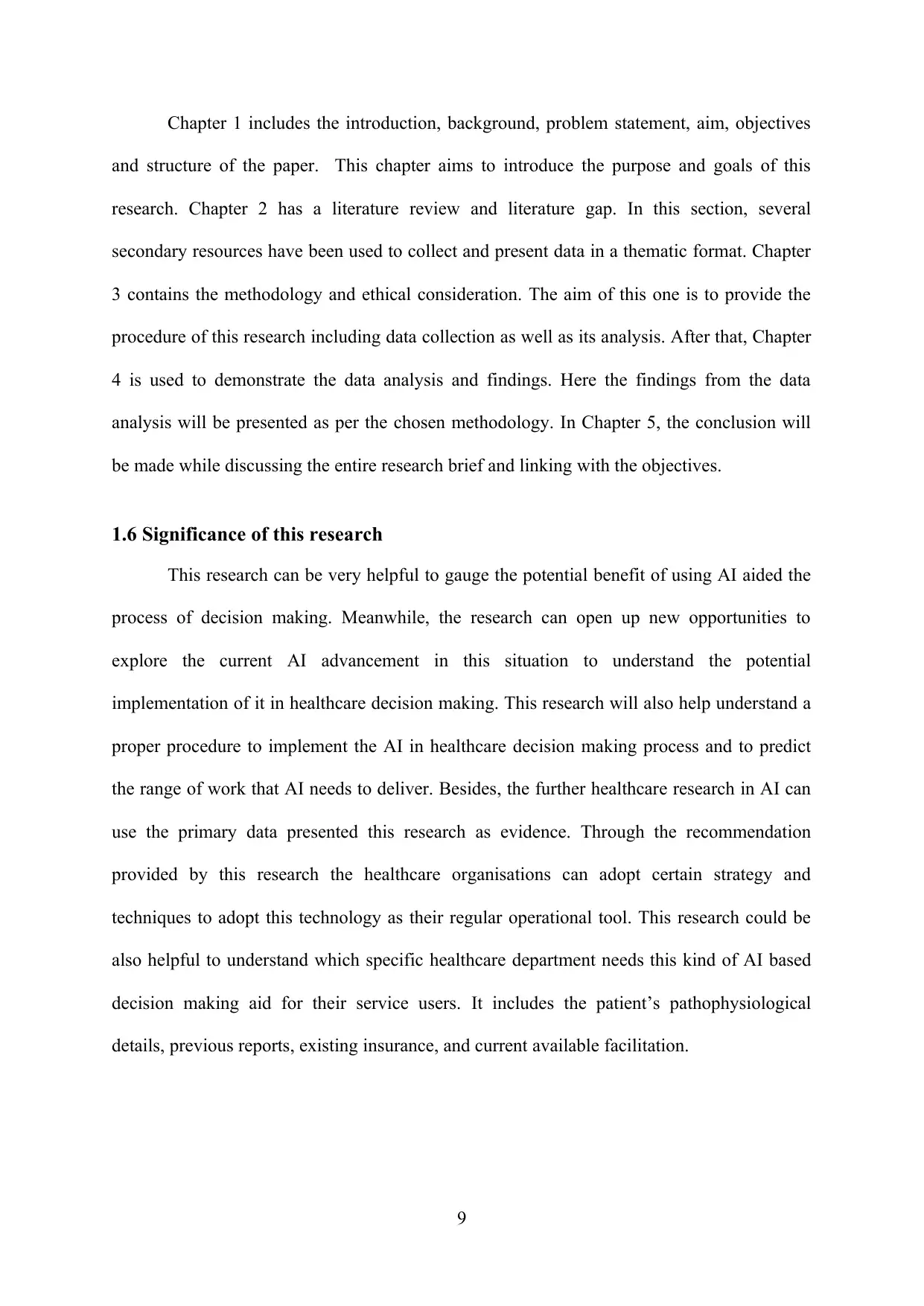
Chapter 1 includes the introduction, background, problem statement, aim, objectives
and structure of the paper. This chapter aims to introduce the purpose and goals of this
research. Chapter 2 has a literature review and literature gap. In this section, several
secondary resources have been used to collect and present data in a thematic format. Chapter
3 contains the methodology and ethical consideration. The aim of this one is to provide the
procedure of this research including data collection as well as its analysis. After that, Chapter
4 is used to demonstrate the data analysis and findings. Here the findings from the data
analysis will be presented as per the chosen methodology. In Chapter 5, the conclusion will
be made while discussing the entire research brief and linking with the objectives.
1.6 Significance of this research
This research can be very helpful to gauge the potential benefit of using AI aided the
process of decision making. Meanwhile, the research can open up new opportunities to
explore the current AI advancement in this situation to understand the potential
implementation of it in healthcare decision making. This research will also help understand a
proper procedure to implement the AI in healthcare decision making process and to predict
the range of work that AI needs to deliver. Besides, the further healthcare research in AI can
use the primary data presented this research as evidence. Through the recommendation
provided by this research the healthcare organisations can adopt certain strategy and
techniques to adopt this technology as their regular operational tool. This research could be
also helpful to understand which specific healthcare department needs this kind of AI based
decision making aid for their service users. It includes the patient’s pathophysiological
details, previous reports, existing insurance, and current available facilitation.
9
and structure of the paper. This chapter aims to introduce the purpose and goals of this
research. Chapter 2 has a literature review and literature gap. In this section, several
secondary resources have been used to collect and present data in a thematic format. Chapter
3 contains the methodology and ethical consideration. The aim of this one is to provide the
procedure of this research including data collection as well as its analysis. After that, Chapter
4 is used to demonstrate the data analysis and findings. Here the findings from the data
analysis will be presented as per the chosen methodology. In Chapter 5, the conclusion will
be made while discussing the entire research brief and linking with the objectives.
1.6 Significance of this research
This research can be very helpful to gauge the potential benefit of using AI aided the
process of decision making. Meanwhile, the research can open up new opportunities to
explore the current AI advancement in this situation to understand the potential
implementation of it in healthcare decision making. This research will also help understand a
proper procedure to implement the AI in healthcare decision making process and to predict
the range of work that AI needs to deliver. Besides, the further healthcare research in AI can
use the primary data presented this research as evidence. Through the recommendation
provided by this research the healthcare organisations can adopt certain strategy and
techniques to adopt this technology as their regular operational tool. This research could be
also helpful to understand which specific healthcare department needs this kind of AI based
decision making aid for their service users. It includes the patient’s pathophysiological
details, previous reports, existing insurance, and current available facilitation.
9
Secure Best Marks with AI Grader
Need help grading? Try our AI Grader for instant feedback on your assignments.
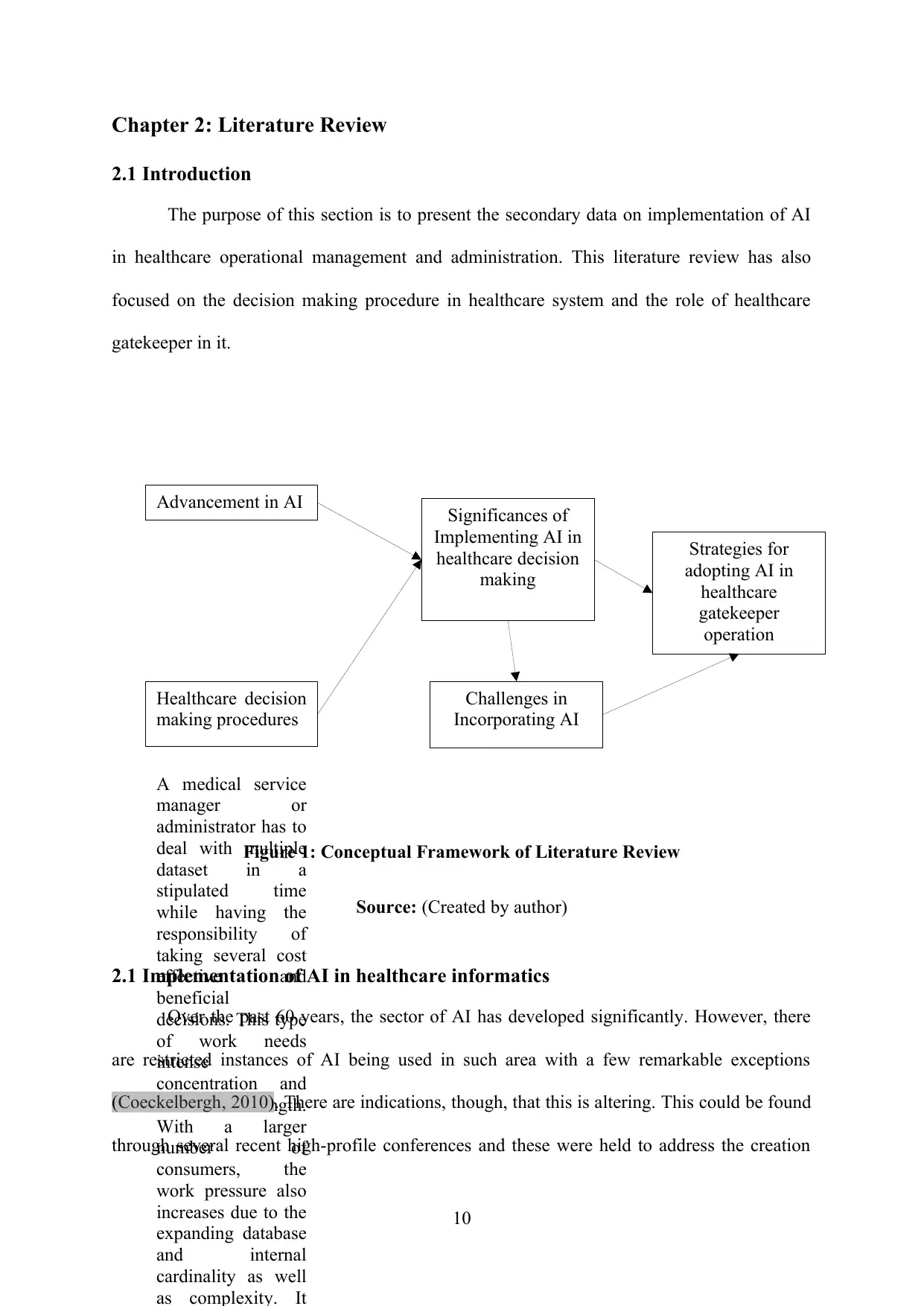
Advancement in AI
Healthcare decision
making procedures
A medical service
manager or
administrator has to
deal with multiple
dataset in a
stipulated time
while having the
responsibility of
taking several cost
effective and
beneficial
decisions. This type
of work needs
intense
concentration and
mental strength.
With a larger
number of
consumers, the
work pressure also
increases due to the
expanding database
and internal
cardinality as well
as complexity. It
Significances of
Implementing AI in
healthcare decision
making
Strategies for
adopting AI in
healthcare
gatekeeper
operation
Challenges in
Incorporating AI
Chapter 2: Literature Review
2.1 Introduction
The purpose of this section is to present the secondary data on implementation of AI
in healthcare operational management and administration. This literature review has also
focused on the decision making procedure in healthcare system and the role of healthcare
gatekeeper in it.
Figure 1: Conceptual Framework of Literature Review
Source: (Created by author)
2.1 Implementation of AI in healthcare informatics
Over the past 60 years, the sector of AI has developed significantly. However, there
are restricted instances of AI being used in such area with a few remarkable exceptions
(Coeckelbergh, 2010). There are indications, though, that this is altering. This could be found
through several recent high-profile conferences and these were held to address the creation
10
Healthcare decision
making procedures
A medical service
manager or
administrator has to
deal with multiple
dataset in a
stipulated time
while having the
responsibility of
taking several cost
effective and
beneficial
decisions. This type
of work needs
intense
concentration and
mental strength.
With a larger
number of
consumers, the
work pressure also
increases due to the
expanding database
and internal
cardinality as well
as complexity. It
Significances of
Implementing AI in
healthcare decision
making
Strategies for
adopting AI in
healthcare
gatekeeper
operation
Challenges in
Incorporating AI
Chapter 2: Literature Review
2.1 Introduction
The purpose of this section is to present the secondary data on implementation of AI
in healthcare operational management and administration. This literature review has also
focused on the decision making procedure in healthcare system and the role of healthcare
gatekeeper in it.
Figure 1: Conceptual Framework of Literature Review
Source: (Created by author)
2.1 Implementation of AI in healthcare informatics
Over the past 60 years, the sector of AI has developed significantly. However, there
are restricted instances of AI being used in such area with a few remarkable exceptions
(Coeckelbergh, 2010). There are indications, though, that this is altering. This could be found
through several recent high-profile conferences and these were held to address the creation
10
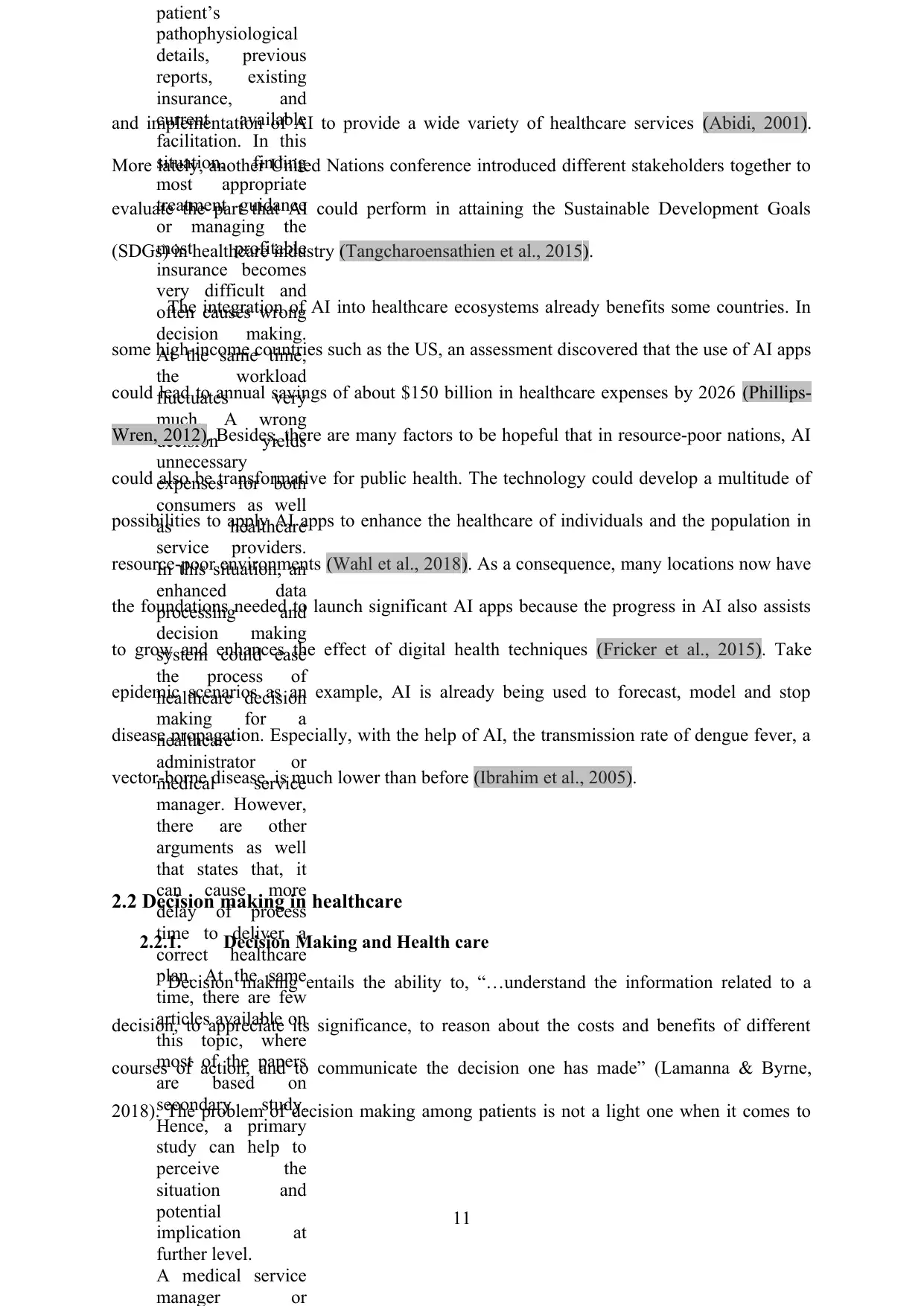
patient’s
pathophysiological
details, previous
reports, existing
insurance, and
current available
facilitation. In this
situation, finding
most appropriate
treatment guidance
or managing the
most profitable
insurance becomes
very difficult and
often causes wrong
decision making.
At the same time,
the workload
fluctuates very
much. A wrong
decision yields
unnecessary
expenses for both
consumers as well
as healthcare
service providers.
In this situation, an
enhanced data
processing and
decision making
system could ease
the process of
healthcare decision
making for a
healthcare
administrator or
medical service
manager. However,
there are other
arguments as well
that states that, it
can cause more
delay of process
time to deliver a
correct healthcare
plan. At the same
time, there are few
articles available on
this topic, where
most of the papers
are based on
secondary study.
Hence, a primary
study can help to
perceive the
situation and
potential
implication at
further level.
A medical service
manager or
and implementation of AI to provide a wide variety of healthcare services (Abidi, 2001).
More lately, another United Nations conference introduced different stakeholders together to
evaluate the part that AI could perform in attaining the Sustainable Development Goals
(SDGs) in healthcare industry (Tangcharoensathien et al., 2015).
The integration of AI into healthcare ecosystems already benefits some countries. In
some high-income countries such as the US, an assessment discovered that the use of AI apps
could lead to annual savings of about $150 billion in healthcare expenses by 2026 (Phillips-
Wren, 2012). Besides, there are many factors to be hopeful that in resource-poor nations, AI
could also be transformative for public health. The technology could develop a multitude of
possibilities to apply AI apps to enhance the healthcare of individuals and the population in
resource-poor environments (Wahl et al., 2018). As a consequence, many locations now have
the foundations needed to launch significant AI apps because the progress in AI also assists
to grow and enhances the effect of digital health techniques (Fricker et al., 2015). Take
epidemic scenarios as an example, AI is already being used to forecast, model and stop
disease propagation. Especially, with the help of AI, the transmission rate of dengue fever, a
vector-borne disease, is much lower than before (Ibrahim et al., 2005).
2.2 Decision making in healthcare
2.2.1. Decision Making and Health care
Decision making entails the ability to, “…understand the information related to a
decision, to appreciate its significance, to reason about the costs and benefits of different
courses of action, and to communicate the decision one has made” (Lamanna & Byrne,
2018). The problem of decision making among patients is not a light one when it comes to
11
pathophysiological
details, previous
reports, existing
insurance, and
current available
facilitation. In this
situation, finding
most appropriate
treatment guidance
or managing the
most profitable
insurance becomes
very difficult and
often causes wrong
decision making.
At the same time,
the workload
fluctuates very
much. A wrong
decision yields
unnecessary
expenses for both
consumers as well
as healthcare
service providers.
In this situation, an
enhanced data
processing and
decision making
system could ease
the process of
healthcare decision
making for a
healthcare
administrator or
medical service
manager. However,
there are other
arguments as well
that states that, it
can cause more
delay of process
time to deliver a
correct healthcare
plan. At the same
time, there are few
articles available on
this topic, where
most of the papers
are based on
secondary study.
Hence, a primary
study can help to
perceive the
situation and
potential
implication at
further level.
A medical service
manager or
and implementation of AI to provide a wide variety of healthcare services (Abidi, 2001).
More lately, another United Nations conference introduced different stakeholders together to
evaluate the part that AI could perform in attaining the Sustainable Development Goals
(SDGs) in healthcare industry (Tangcharoensathien et al., 2015).
The integration of AI into healthcare ecosystems already benefits some countries. In
some high-income countries such as the US, an assessment discovered that the use of AI apps
could lead to annual savings of about $150 billion in healthcare expenses by 2026 (Phillips-
Wren, 2012). Besides, there are many factors to be hopeful that in resource-poor nations, AI
could also be transformative for public health. The technology could develop a multitude of
possibilities to apply AI apps to enhance the healthcare of individuals and the population in
resource-poor environments (Wahl et al., 2018). As a consequence, many locations now have
the foundations needed to launch significant AI apps because the progress in AI also assists
to grow and enhances the effect of digital health techniques (Fricker et al., 2015). Take
epidemic scenarios as an example, AI is already being used to forecast, model and stop
disease propagation. Especially, with the help of AI, the transmission rate of dengue fever, a
vector-borne disease, is much lower than before (Ibrahim et al., 2005).
2.2 Decision making in healthcare
2.2.1. Decision Making and Health care
Decision making entails the ability to, “…understand the information related to a
decision, to appreciate its significance, to reason about the costs and benefits of different
courses of action, and to communicate the decision one has made” (Lamanna & Byrne,
2018). The problem of decision making among patients is not a light one when it comes to
11
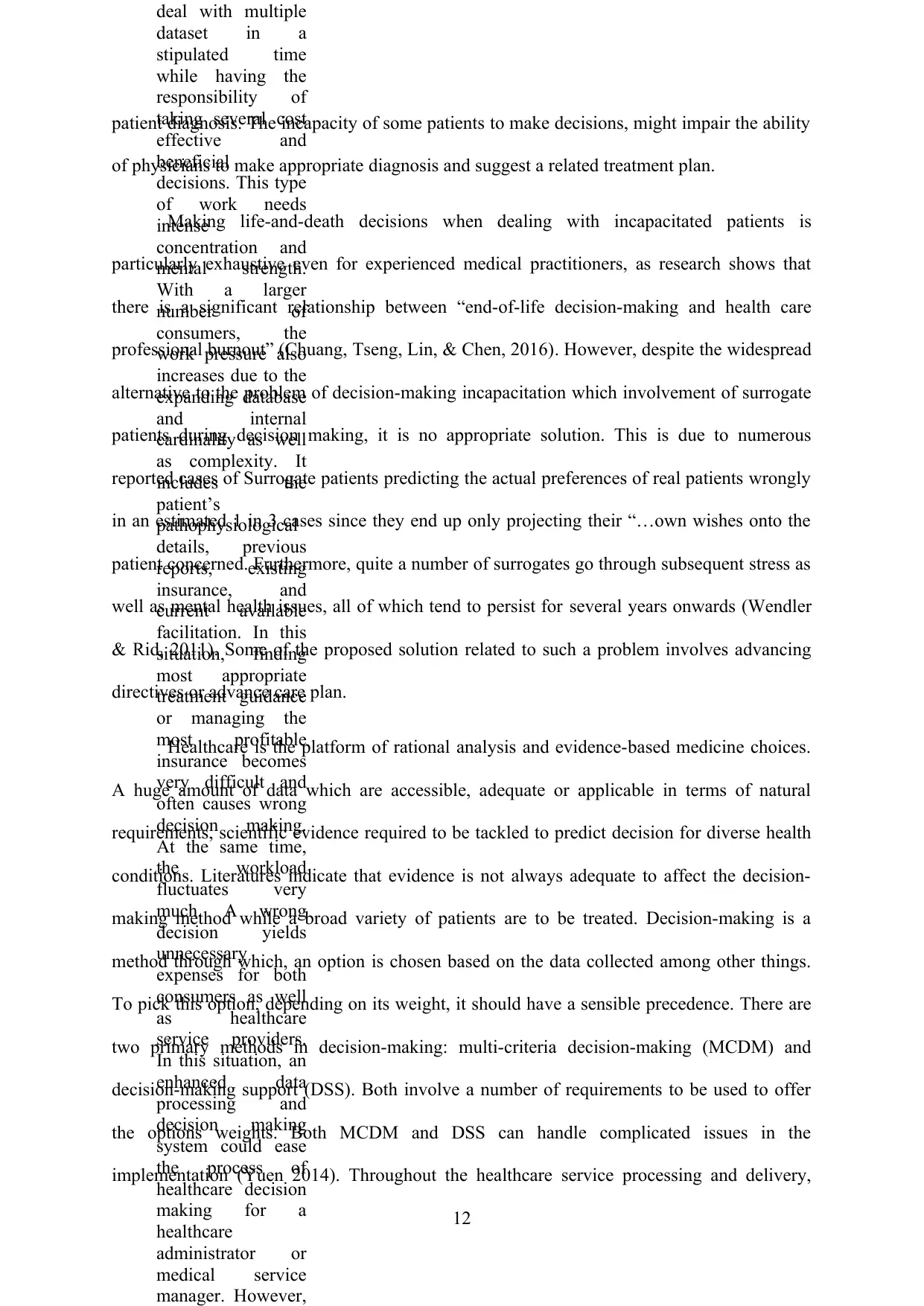
deal with multiple
dataset in a
stipulated time
while having the
responsibility of
taking several cost
effective and
beneficial
decisions. This type
of work needs
intense
concentration and
mental strength.
With a larger
number of
consumers, the
work pressure also
increases due to the
expanding database
and internal
cardinality as well
as complexity. It
includes the
patient’s
pathophysiological
details, previous
reports, existing
insurance, and
current available
facilitation. In this
situation, finding
most appropriate
treatment guidance
or managing the
most profitable
insurance becomes
very difficult and
often causes wrong
decision making.
At the same time,
the workload
fluctuates very
much. A wrong
decision yields
unnecessary
expenses for both
consumers as well
as healthcare
service providers.
In this situation, an
enhanced data
processing and
decision making
system could ease
the process of
healthcare decision
making for a
healthcare
administrator or
medical service
manager. However,
patient diagnosis. The incapacity of some patients to make decisions, might impair the ability
of physicians to make appropriate diagnosis and suggest a related treatment plan.
Making life-and-death decisions when dealing with incapacitated patients is
particularly exhaustive even for experienced medical practitioners, as research shows that
there is a significant relationship between “end-of-life decision-making and health care
professional burnout” (Chuang, Tseng, Lin, & Chen, 2016). However, despite the widespread
alternative to the problem of decision-making incapacitation which involvement of surrogate
patients during decision making, it is no appropriate solution. This is due to numerous
reported cases of Surrogate patients predicting the actual preferences of real patients wrongly
in an estimated 1 in 3 cases since they end up only projecting their “…own wishes onto the
patient concerned. Furthermore, quite a number of surrogates go through subsequent stress as
well as mental health issues, all of which tend to persist for several years onwards (Wendler
& Rid, 2011). Some of the proposed solution related to such a problem involves advancing
directives or advance care plan.
Healthcare is the platform of rational analysis and evidence-based medicine choices.
A huge amount of data which are accessible, adequate or applicable in terms of natural
requirements, scientific evidence required to be tackled to predict decision for diverse health
conditions. Literatures indicate that evidence is not always adequate to affect the decision-
making method while a broad variety of patients are to be treated. Decision-making is a
method through which, an option is chosen based on the data collected among other things.
To pick this option, depending on its weight, it should have a sensible precedence. There are
two primary methods in decision-making: multi-criteria decision-making (MCDM) and
decision-making support (DSS). Both involve a number of requirements to be used to offer
the options weights. Both MCDM and DSS can handle complicated issues in the
implementation (Yuen 2014). Throughout the healthcare service processing and delivery,
12
dataset in a
stipulated time
while having the
responsibility of
taking several cost
effective and
beneficial
decisions. This type
of work needs
intense
concentration and
mental strength.
With a larger
number of
consumers, the
work pressure also
increases due to the
expanding database
and internal
cardinality as well
as complexity. It
includes the
patient’s
pathophysiological
details, previous
reports, existing
insurance, and
current available
facilitation. In this
situation, finding
most appropriate
treatment guidance
or managing the
most profitable
insurance becomes
very difficult and
often causes wrong
decision making.
At the same time,
the workload
fluctuates very
much. A wrong
decision yields
unnecessary
expenses for both
consumers as well
as healthcare
service providers.
In this situation, an
enhanced data
processing and
decision making
system could ease
the process of
healthcare decision
making for a
healthcare
administrator or
medical service
manager. However,
patient diagnosis. The incapacity of some patients to make decisions, might impair the ability
of physicians to make appropriate diagnosis and suggest a related treatment plan.
Making life-and-death decisions when dealing with incapacitated patients is
particularly exhaustive even for experienced medical practitioners, as research shows that
there is a significant relationship between “end-of-life decision-making and health care
professional burnout” (Chuang, Tseng, Lin, & Chen, 2016). However, despite the widespread
alternative to the problem of decision-making incapacitation which involvement of surrogate
patients during decision making, it is no appropriate solution. This is due to numerous
reported cases of Surrogate patients predicting the actual preferences of real patients wrongly
in an estimated 1 in 3 cases since they end up only projecting their “…own wishes onto the
patient concerned. Furthermore, quite a number of surrogates go through subsequent stress as
well as mental health issues, all of which tend to persist for several years onwards (Wendler
& Rid, 2011). Some of the proposed solution related to such a problem involves advancing
directives or advance care plan.
Healthcare is the platform of rational analysis and evidence-based medicine choices.
A huge amount of data which are accessible, adequate or applicable in terms of natural
requirements, scientific evidence required to be tackled to predict decision for diverse health
conditions. Literatures indicate that evidence is not always adequate to affect the decision-
making method while a broad variety of patients are to be treated. Decision-making is a
method through which, an option is chosen based on the data collected among other things.
To pick this option, depending on its weight, it should have a sensible precedence. There are
two primary methods in decision-making: multi-criteria decision-making (MCDM) and
decision-making support (DSS). Both involve a number of requirements to be used to offer
the options weights. Both MCDM and DSS can handle complicated issues in the
implementation (Yuen 2014). Throughout the healthcare service processing and delivery,
12
Paraphrase This Document
Need a fresh take? Get an instant paraphrase of this document with our AI Paraphraser
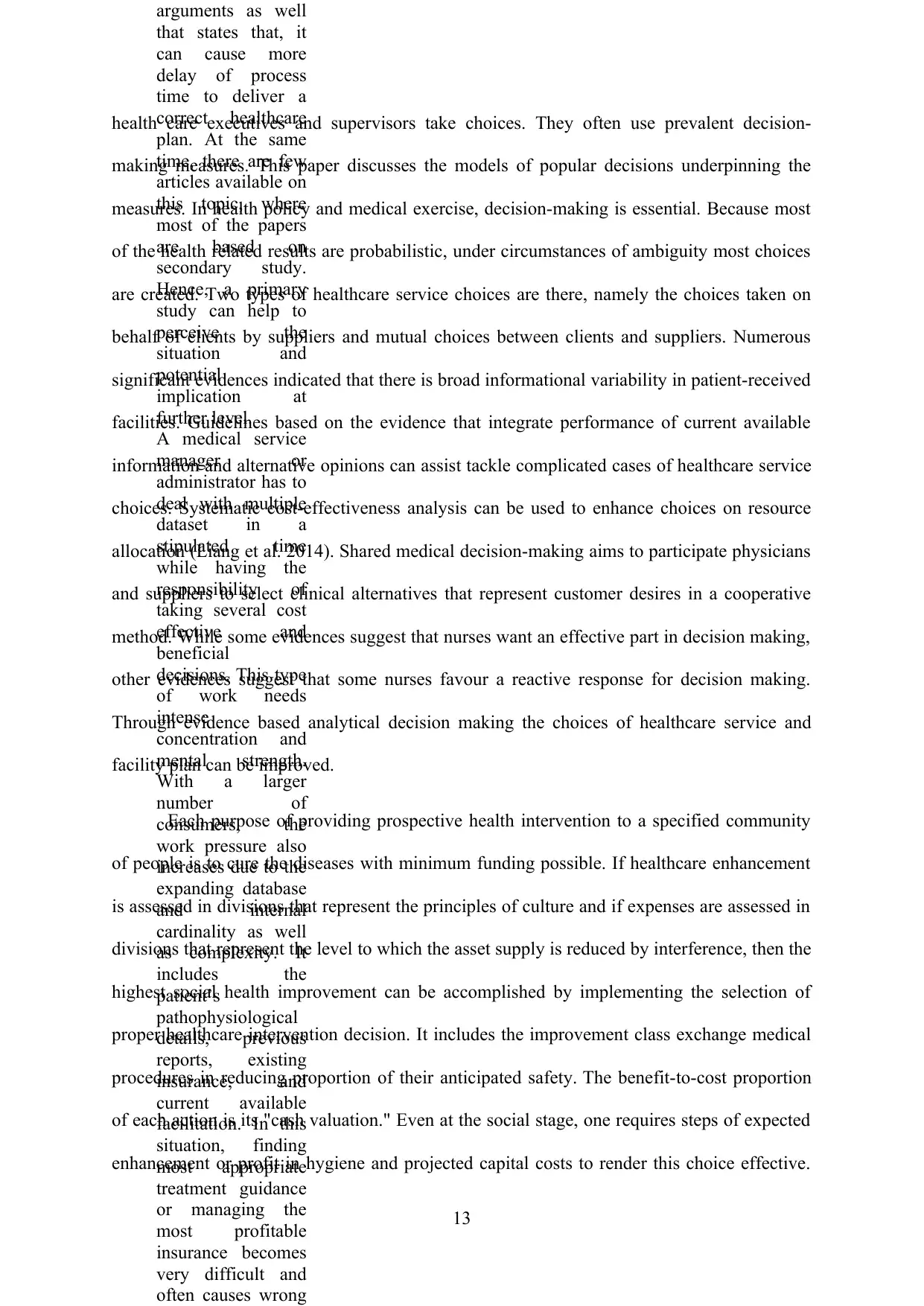
arguments as well
that states that, it
can cause more
delay of process
time to deliver a
correct healthcare
plan. At the same
time, there are few
articles available on
this topic, where
most of the papers
are based on
secondary study.
Hence, a primary
study can help to
perceive the
situation and
potential
implication at
further level.
A medical service
manager or
administrator has to
deal with multiple
dataset in a
stipulated time
while having the
responsibility of
taking several cost
effective and
beneficial
decisions. This type
of work needs
intense
concentration and
mental strength.
With a larger
number of
consumers, the
work pressure also
increases due to the
expanding database
and internal
cardinality as well
as complexity. It
includes the
patient’s
pathophysiological
details, previous
reports, existing
insurance, and
current available
facilitation. In this
situation, finding
most appropriate
treatment guidance
or managing the
most profitable
insurance becomes
very difficult and
often causes wrong
health care executives and supervisors take choices. They often use prevalent decision-
making measures. This paper discusses the models of popular decisions underpinning the
measures. In health policy and medical exercise, decision-making is essential. Because most
of the health related results are probabilistic, under circumstances of ambiguity most choices
are created. Two types of healthcare service choices are there, namely the choices taken on
behalf of clients by suppliers and mutual choices between clients and suppliers. Numerous
significant evidences indicated that there is broad informational variability in patient-received
facilities. Guidelines based on the evidence that integrate performance of current available
information and alternative opinions can assist tackle complicated cases of healthcare service
choices. Systematic cost-effectiveness analysis can be used to enhance choices on resource
allocation (Liang et al. 2014). Shared medical decision-making aims to participate physicians
and suppliers to select clinical alternatives that represent customer desires in a cooperative
method. While some evidences suggest that nurses want an effective part in decision making,
other evidences suggest that some nurses favour a reactive response for decision making.
Through evidence based analytical decision making the choices of healthcare service and
facility plan can be improved.
Each purpose of providing prospective health intervention to a specified community
of people is to cure the diseases with minimum funding possible. If healthcare enhancement
is assessed in divisions that represent the principles of culture and if expenses are assessed in
divisions that represent the level to which the asset supply is reduced by interference, then the
highest social health improvement can be accomplished by implementing the selection of
proper healthcare intervention decision. It includes the improvement class exchange medical
procedures in reducing proportion of their anticipated safety. The benefit-to-cost proportion
of each action is its "cash valuation." Even at the social stage, one requires steps of expected
enhancement or profit in hygiene and projected capital costs to render this choice effective.
13
that states that, it
can cause more
delay of process
time to deliver a
correct healthcare
plan. At the same
time, there are few
articles available on
this topic, where
most of the papers
are based on
secondary study.
Hence, a primary
study can help to
perceive the
situation and
potential
implication at
further level.
A medical service
manager or
administrator has to
deal with multiple
dataset in a
stipulated time
while having the
responsibility of
taking several cost
effective and
beneficial
decisions. This type
of work needs
intense
concentration and
mental strength.
With a larger
number of
consumers, the
work pressure also
increases due to the
expanding database
and internal
cardinality as well
as complexity. It
includes the
patient’s
pathophysiological
details, previous
reports, existing
insurance, and
current available
facilitation. In this
situation, finding
most appropriate
treatment guidance
or managing the
most profitable
insurance becomes
very difficult and
often causes wrong
health care executives and supervisors take choices. They often use prevalent decision-
making measures. This paper discusses the models of popular decisions underpinning the
measures. In health policy and medical exercise, decision-making is essential. Because most
of the health related results are probabilistic, under circumstances of ambiguity most choices
are created. Two types of healthcare service choices are there, namely the choices taken on
behalf of clients by suppliers and mutual choices between clients and suppliers. Numerous
significant evidences indicated that there is broad informational variability in patient-received
facilities. Guidelines based on the evidence that integrate performance of current available
information and alternative opinions can assist tackle complicated cases of healthcare service
choices. Systematic cost-effectiveness analysis can be used to enhance choices on resource
allocation (Liang et al. 2014). Shared medical decision-making aims to participate physicians
and suppliers to select clinical alternatives that represent customer desires in a cooperative
method. While some evidences suggest that nurses want an effective part in decision making,
other evidences suggest that some nurses favour a reactive response for decision making.
Through evidence based analytical decision making the choices of healthcare service and
facility plan can be improved.
Each purpose of providing prospective health intervention to a specified community
of people is to cure the diseases with minimum funding possible. If healthcare enhancement
is assessed in divisions that represent the principles of culture and if expenses are assessed in
divisions that represent the level to which the asset supply is reduced by interference, then the
highest social health improvement can be accomplished by implementing the selection of
proper healthcare intervention decision. It includes the improvement class exchange medical
procedures in reducing proportion of their anticipated safety. The benefit-to-cost proportion
of each action is its "cash valuation." Even at the social stage, one requires steps of expected
enhancement or profit in hygiene and projected capital costs to render this choice effective.
13
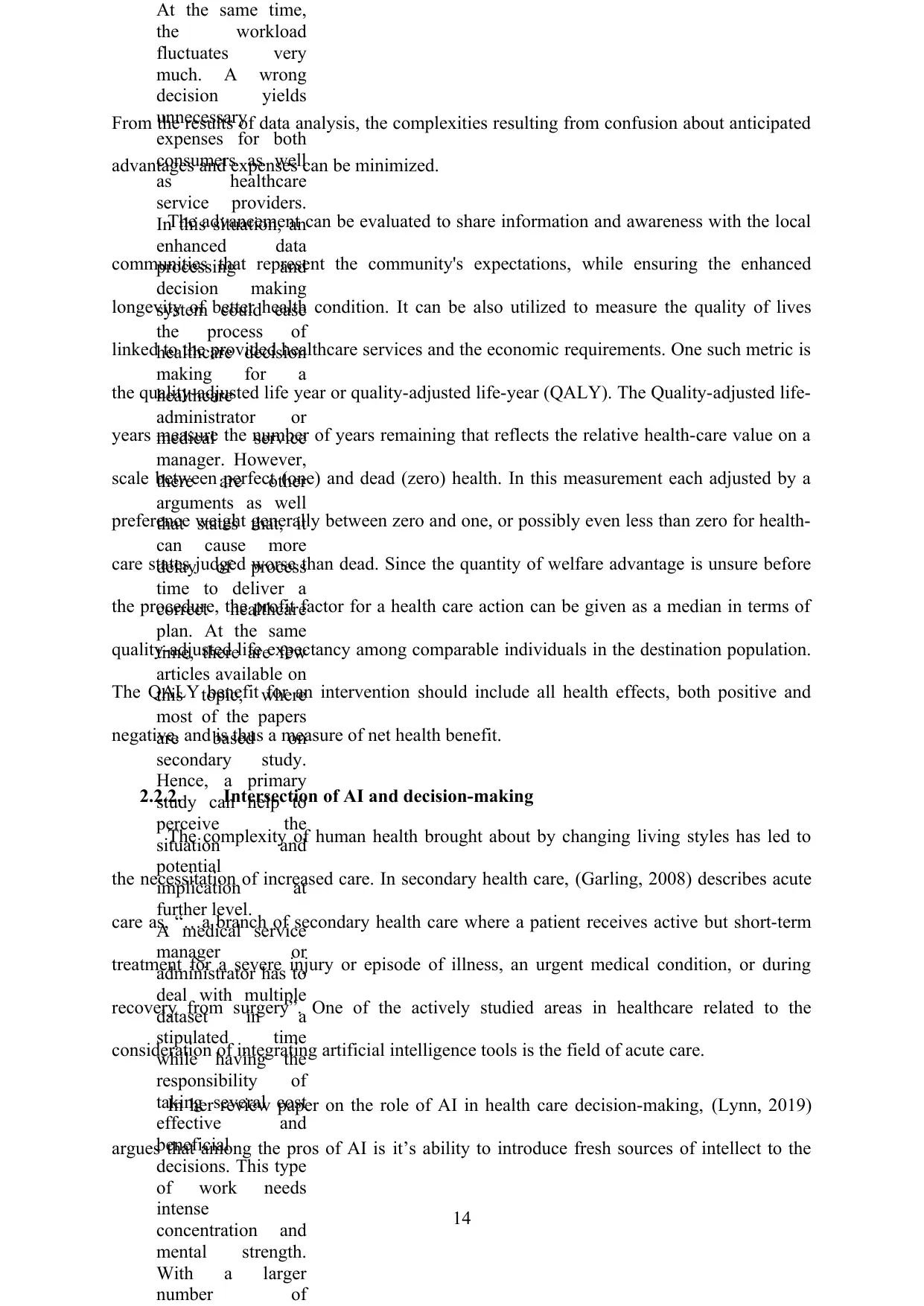
At the same time,
the workload
fluctuates very
much. A wrong
decision yields
unnecessary
expenses for both
consumers as well
as healthcare
service providers.
In this situation, an
enhanced data
processing and
decision making
system could ease
the process of
healthcare decision
making for a
healthcare
administrator or
medical service
manager. However,
there are other
arguments as well
that states that, it
can cause more
delay of process
time to deliver a
correct healthcare
plan. At the same
time, there are few
articles available on
this topic, where
most of the papers
are based on
secondary study.
Hence, a primary
study can help to
perceive the
situation and
potential
implication at
further level.
A medical service
manager or
administrator has to
deal with multiple
dataset in a
stipulated time
while having the
responsibility of
taking several cost
effective and
beneficial
decisions. This type
of work needs
intense
concentration and
mental strength.
With a larger
number of
From the results of data analysis, the complexities resulting from confusion about anticipated
advantages and expenses can be minimized.
The advancement can be evaluated to share information and awareness with the local
communities that represent the community's expectations, while ensuring the enhanced
longevity of better health condition. It can be also utilized to measure the quality of lives
linked to the provided healthcare services and the economic requirements. One such metric is
the quality-adjusted life year or quality-adjusted life-year (QALY). The Quality-adjusted life-
years measure the number of years remaining that reflects the relative health-care value on a
scale between perfect (one) and dead (zero) health. In this measurement each adjusted by a
preference weight generally between zero and one, or possibly even less than zero for health-
care states judged worse than dead. Since the quantity of welfare advantage is unsure before
the procedure, the profit factor for a health care action can be given as a median in terms of
quality-adjusted life expectancy among comparable individuals in the destination population.
The QALY benefit for an intervention should include all health effects, both positive and
negative, and is thus a measure of net health benefit.
2.2.2. Intersection of AI and decision-making
The complexity of human health brought about by changing living styles has led to
the necessitation of increased care. In secondary health care, (Garling, 2008) describes acute
care as, “…a branch of secondary health care where a patient receives active but short-term
treatment for a severe injury or episode of illness, an urgent medical condition, or during
recovery from surgery”. One of the actively studied areas in healthcare related to the
consideration of integrating artificial intelligence tools is the field of acute care.
In her review paper on the role of AI in health care decision-making, (Lynn, 2019)
argues that among the pros of AI is it’s ability to introduce fresh sources of intellect to the
14
the workload
fluctuates very
much. A wrong
decision yields
unnecessary
expenses for both
consumers as well
as healthcare
service providers.
In this situation, an
enhanced data
processing and
decision making
system could ease
the process of
healthcare decision
making for a
healthcare
administrator or
medical service
manager. However,
there are other
arguments as well
that states that, it
can cause more
delay of process
time to deliver a
correct healthcare
plan. At the same
time, there are few
articles available on
this topic, where
most of the papers
are based on
secondary study.
Hence, a primary
study can help to
perceive the
situation and
potential
implication at
further level.
A medical service
manager or
administrator has to
deal with multiple
dataset in a
stipulated time
while having the
responsibility of
taking several cost
effective and
beneficial
decisions. This type
of work needs
intense
concentration and
mental strength.
With a larger
number of
From the results of data analysis, the complexities resulting from confusion about anticipated
advantages and expenses can be minimized.
The advancement can be evaluated to share information and awareness with the local
communities that represent the community's expectations, while ensuring the enhanced
longevity of better health condition. It can be also utilized to measure the quality of lives
linked to the provided healthcare services and the economic requirements. One such metric is
the quality-adjusted life year or quality-adjusted life-year (QALY). The Quality-adjusted life-
years measure the number of years remaining that reflects the relative health-care value on a
scale between perfect (one) and dead (zero) health. In this measurement each adjusted by a
preference weight generally between zero and one, or possibly even less than zero for health-
care states judged worse than dead. Since the quantity of welfare advantage is unsure before
the procedure, the profit factor for a health care action can be given as a median in terms of
quality-adjusted life expectancy among comparable individuals in the destination population.
The QALY benefit for an intervention should include all health effects, both positive and
negative, and is thus a measure of net health benefit.
2.2.2. Intersection of AI and decision-making
The complexity of human health brought about by changing living styles has led to
the necessitation of increased care. In secondary health care, (Garling, 2008) describes acute
care as, “…a branch of secondary health care where a patient receives active but short-term
treatment for a severe injury or episode of illness, an urgent medical condition, or during
recovery from surgery”. One of the actively studied areas in healthcare related to the
consideration of integrating artificial intelligence tools is the field of acute care.
In her review paper on the role of AI in health care decision-making, (Lynn, 2019)
argues that among the pros of AI is it’s ability to introduce fresh sources of intellect to the
14
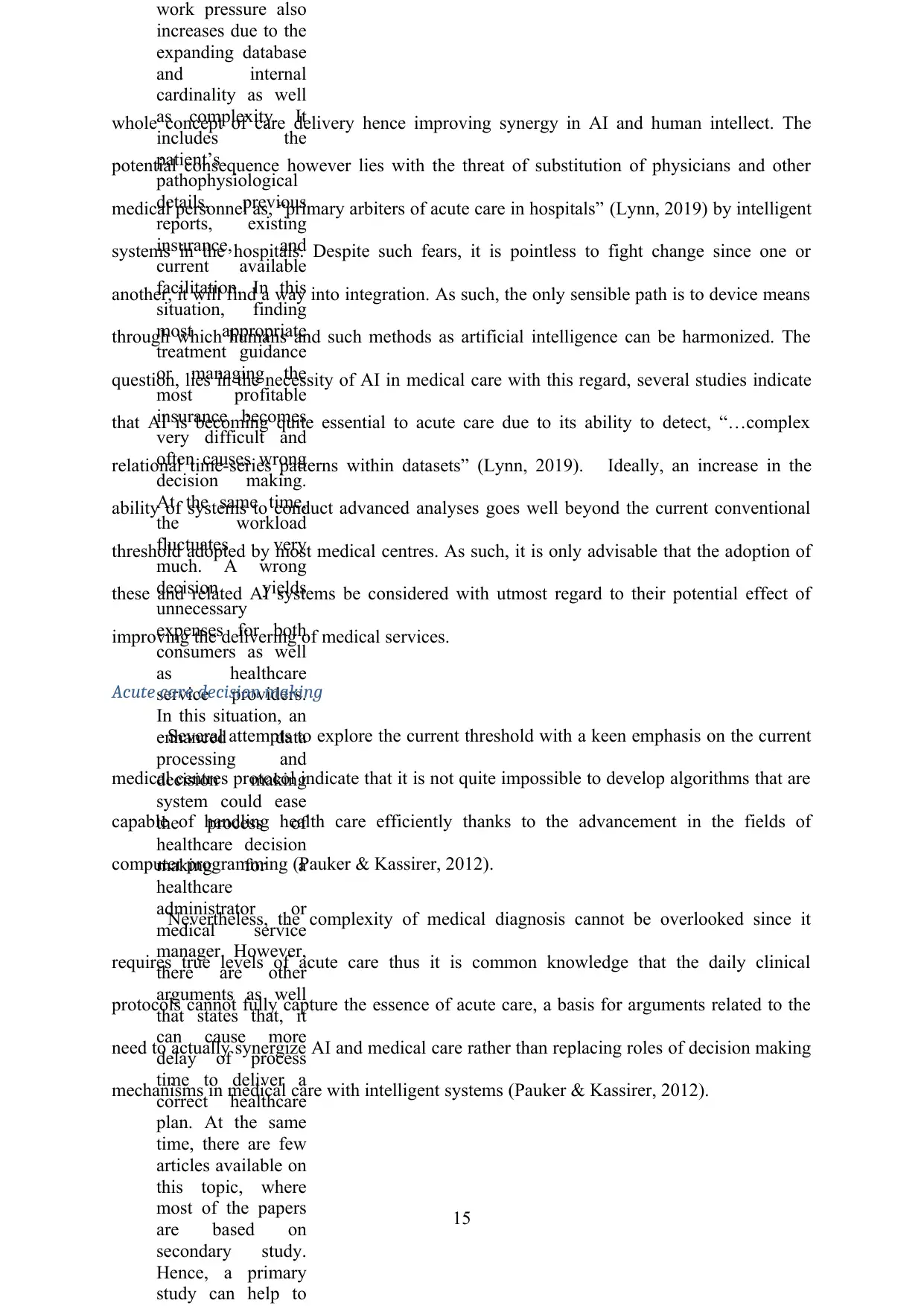
work pressure also
increases due to the
expanding database
and internal
cardinality as well
as complexity. It
includes the
patient’s
pathophysiological
details, previous
reports, existing
insurance, and
current available
facilitation. In this
situation, finding
most appropriate
treatment guidance
or managing the
most profitable
insurance becomes
very difficult and
often causes wrong
decision making.
At the same time,
the workload
fluctuates very
much. A wrong
decision yields
unnecessary
expenses for both
consumers as well
as healthcare
service providers.
In this situation, an
enhanced data
processing and
decision making
system could ease
the process of
healthcare decision
making for a
healthcare
administrator or
medical service
manager. However,
there are other
arguments as well
that states that, it
can cause more
delay of process
time to deliver a
correct healthcare
plan. At the same
time, there are few
articles available on
this topic, where
most of the papers
are based on
secondary study.
Hence, a primary
study can help to
whole concept of care delivery hence improving synergy in AI and human intellect. The
potential consequence however lies with the threat of substitution of physicians and other
medical personnel as, “primary arbiters of acute care in hospitals” (Lynn, 2019) by intelligent
systems in the hospitals. Despite such fears, it is pointless to fight change since one or
another, it will find a way into integration. As such, the only sensible path is to device means
through which humans and such methods as artificial intelligence can be harmonized. The
question, lies in the necessity of AI in medical care with this regard, several studies indicate
that AI is becoming quite essential to acute care due to its ability to detect, “…complex
relational time-series patterns within datasets” (Lynn, 2019). Ideally, an increase in the
ability of systems to conduct advanced analyses goes well beyond the current conventional
threshold adopted by most medical centres. As such, it is only advisable that the adoption of
these and related AI systems be considered with utmost regard to their potential effect of
improving the delivering of medical services.
Acute care decision making
Several attempts to explore the current threshold with a keen emphasis on the current
medical centres protocol indicate that it is not quite impossible to develop algorithms that are
capable of handling health care efficiently thanks to the advancement in the fields of
computer programming (Pauker & Kassirer, 2012).
Nevertheless, the complexity of medical diagnosis cannot be overlooked since it
requires true levels of acute care thus it is common knowledge that the daily clinical
protocols cannot fully capture the essence of acute care, a basis for arguments related to the
need to actually synergize AI and medical care rather than replacing roles of decision making
mechanisms in medical care with intelligent systems (Pauker & Kassirer, 2012).
15
increases due to the
expanding database
and internal
cardinality as well
as complexity. It
includes the
patient’s
pathophysiological
details, previous
reports, existing
insurance, and
current available
facilitation. In this
situation, finding
most appropriate
treatment guidance
or managing the
most profitable
insurance becomes
very difficult and
often causes wrong
decision making.
At the same time,
the workload
fluctuates very
much. A wrong
decision yields
unnecessary
expenses for both
consumers as well
as healthcare
service providers.
In this situation, an
enhanced data
processing and
decision making
system could ease
the process of
healthcare decision
making for a
healthcare
administrator or
medical service
manager. However,
there are other
arguments as well
that states that, it
can cause more
delay of process
time to deliver a
correct healthcare
plan. At the same
time, there are few
articles available on
this topic, where
most of the papers
are based on
secondary study.
Hence, a primary
study can help to
whole concept of care delivery hence improving synergy in AI and human intellect. The
potential consequence however lies with the threat of substitution of physicians and other
medical personnel as, “primary arbiters of acute care in hospitals” (Lynn, 2019) by intelligent
systems in the hospitals. Despite such fears, it is pointless to fight change since one or
another, it will find a way into integration. As such, the only sensible path is to device means
through which humans and such methods as artificial intelligence can be harmonized. The
question, lies in the necessity of AI in medical care with this regard, several studies indicate
that AI is becoming quite essential to acute care due to its ability to detect, “…complex
relational time-series patterns within datasets” (Lynn, 2019). Ideally, an increase in the
ability of systems to conduct advanced analyses goes well beyond the current conventional
threshold adopted by most medical centres. As such, it is only advisable that the adoption of
these and related AI systems be considered with utmost regard to their potential effect of
improving the delivering of medical services.
Acute care decision making
Several attempts to explore the current threshold with a keen emphasis on the current
medical centres protocol indicate that it is not quite impossible to develop algorithms that are
capable of handling health care efficiently thanks to the advancement in the fields of
computer programming (Pauker & Kassirer, 2012).
Nevertheless, the complexity of medical diagnosis cannot be overlooked since it
requires true levels of acute care thus it is common knowledge that the daily clinical
protocols cannot fully capture the essence of acute care, a basis for arguments related to the
need to actually synergize AI and medical care rather than replacing roles of decision making
mechanisms in medical care with intelligent systems (Pauker & Kassirer, 2012).
15
Secure Best Marks with AI Grader
Need help grading? Try our AI Grader for instant feedback on your assignments.
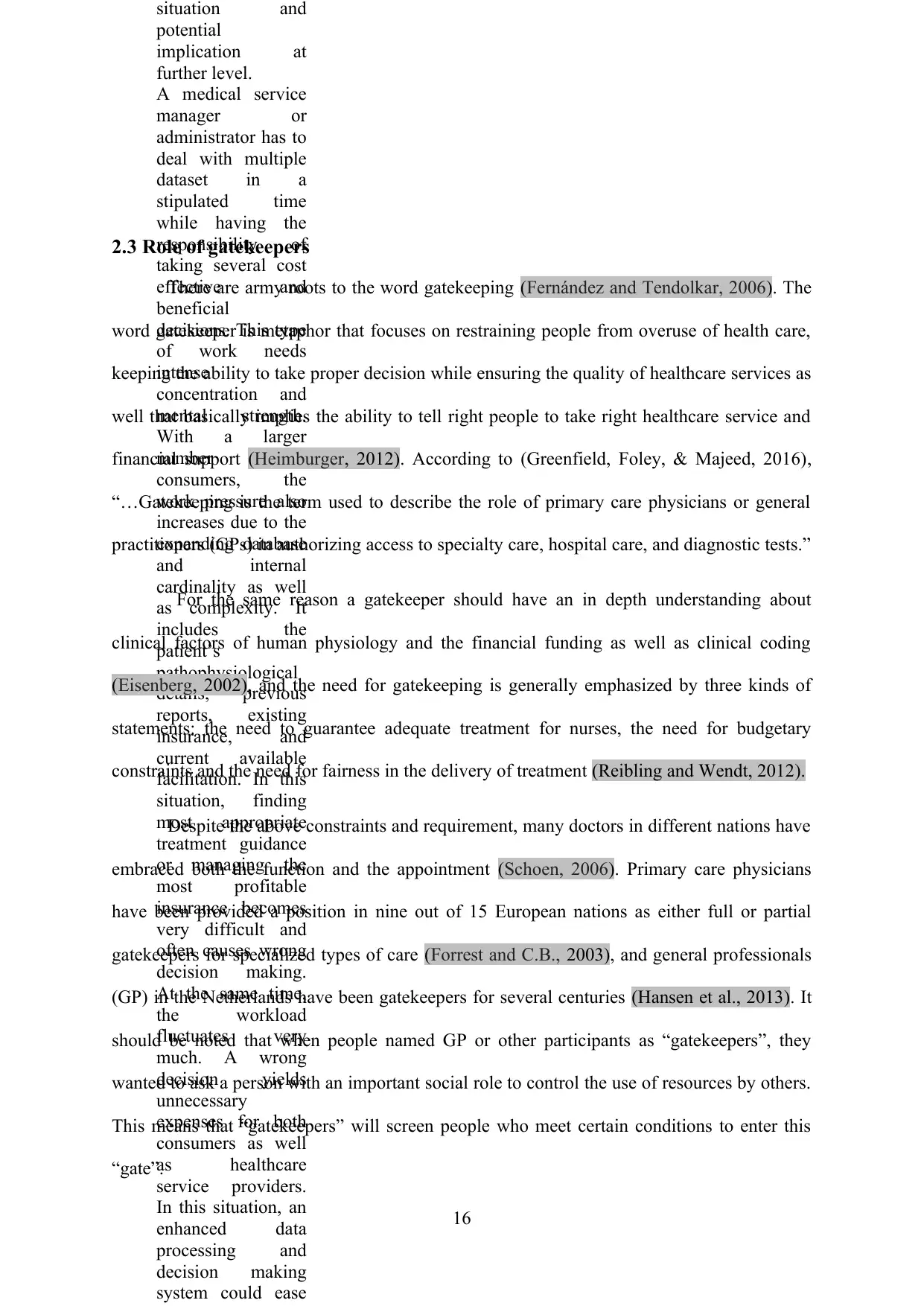
situation and
potential
implication at
further level.
A medical service
manager or
administrator has to
deal with multiple
dataset in a
stipulated time
while having the
responsibility of
taking several cost
effective and
beneficial
decisions. This type
of work needs
intense
concentration and
mental strength.
With a larger
number of
consumers, the
work pressure also
increases due to the
expanding database
and internal
cardinality as well
as complexity. It
includes the
patient’s
pathophysiological
details, previous
reports, existing
insurance, and
current available
facilitation. In this
situation, finding
most appropriate
treatment guidance
or managing the
most profitable
insurance becomes
very difficult and
often causes wrong
decision making.
At the same time,
the workload
fluctuates very
much. A wrong
decision yields
unnecessary
expenses for both
consumers as well
as healthcare
service providers.
In this situation, an
enhanced data
processing and
decision making
system could ease
2.3 Role of gatekeepers
There are army roots to the word gatekeeping (Fernández and Tendolkar, 2006). The
word gatekeeper is metaphor that focuses on restraining people from overuse of health care,
keeping the ability to take proper decision while ensuring the quality of healthcare services as
well that basically implies the ability to tell right people to take right healthcare service and
financial support (Heimburger, 2012). According to (Greenfield, Foley, & Majeed, 2016),
“…Gatekeeping is the term used to describe the role of primary care physicians or general
practitioners (GPs) in authorizing access to specialty care, hospital care, and diagnostic tests.”
For the same reason a gatekeeper should have an in depth understanding about
clinical factors of human physiology and the financial funding as well as clinical coding
(Eisenberg, 2002), and the need for gatekeeping is generally emphasized by three kinds of
statements: the need to guarantee adequate treatment for nurses, the need for budgetary
constraints and the need for fairness in the delivery of treatment (Reibling and Wendt, 2012).
Despite the above constraints and requirement, many doctors in different nations have
embraced both the function and the appointment (Schoen, 2006). Primary care physicians
have been provided a position in nine out of 15 European nations as either full or partial
gatekeepers for specialized types of care (Forrest and C.B., 2003), and general professionals
(GP) in the Netherlands have been gatekeepers for several centuries (Hansen et al., 2013). It
should be noted that when people named GP or other participants as “gatekeepers”, they
wanted to ask a person with an important social role to control the use of resources by others.
This means that “gatekeepers” will screen people who meet certain conditions to enter this
“gate”.
16
potential
implication at
further level.
A medical service
manager or
administrator has to
deal with multiple
dataset in a
stipulated time
while having the
responsibility of
taking several cost
effective and
beneficial
decisions. This type
of work needs
intense
concentration and
mental strength.
With a larger
number of
consumers, the
work pressure also
increases due to the
expanding database
and internal
cardinality as well
as complexity. It
includes the
patient’s
pathophysiological
details, previous
reports, existing
insurance, and
current available
facilitation. In this
situation, finding
most appropriate
treatment guidance
or managing the
most profitable
insurance becomes
very difficult and
often causes wrong
decision making.
At the same time,
the workload
fluctuates very
much. A wrong
decision yields
unnecessary
expenses for both
consumers as well
as healthcare
service providers.
In this situation, an
enhanced data
processing and
decision making
system could ease
2.3 Role of gatekeepers
There are army roots to the word gatekeeping (Fernández and Tendolkar, 2006). The
word gatekeeper is metaphor that focuses on restraining people from overuse of health care,
keeping the ability to take proper decision while ensuring the quality of healthcare services as
well that basically implies the ability to tell right people to take right healthcare service and
financial support (Heimburger, 2012). According to (Greenfield, Foley, & Majeed, 2016),
“…Gatekeeping is the term used to describe the role of primary care physicians or general
practitioners (GPs) in authorizing access to specialty care, hospital care, and diagnostic tests.”
For the same reason a gatekeeper should have an in depth understanding about
clinical factors of human physiology and the financial funding as well as clinical coding
(Eisenberg, 2002), and the need for gatekeeping is generally emphasized by three kinds of
statements: the need to guarantee adequate treatment for nurses, the need for budgetary
constraints and the need for fairness in the delivery of treatment (Reibling and Wendt, 2012).
Despite the above constraints and requirement, many doctors in different nations have
embraced both the function and the appointment (Schoen, 2006). Primary care physicians
have been provided a position in nine out of 15 European nations as either full or partial
gatekeepers for specialized types of care (Forrest and C.B., 2003), and general professionals
(GP) in the Netherlands have been gatekeepers for several centuries (Hansen et al., 2013). It
should be noted that when people named GP or other participants as “gatekeepers”, they
wanted to ask a person with an important social role to control the use of resources by others.
This means that “gatekeepers” will screen people who meet certain conditions to enter this
“gate”.
16
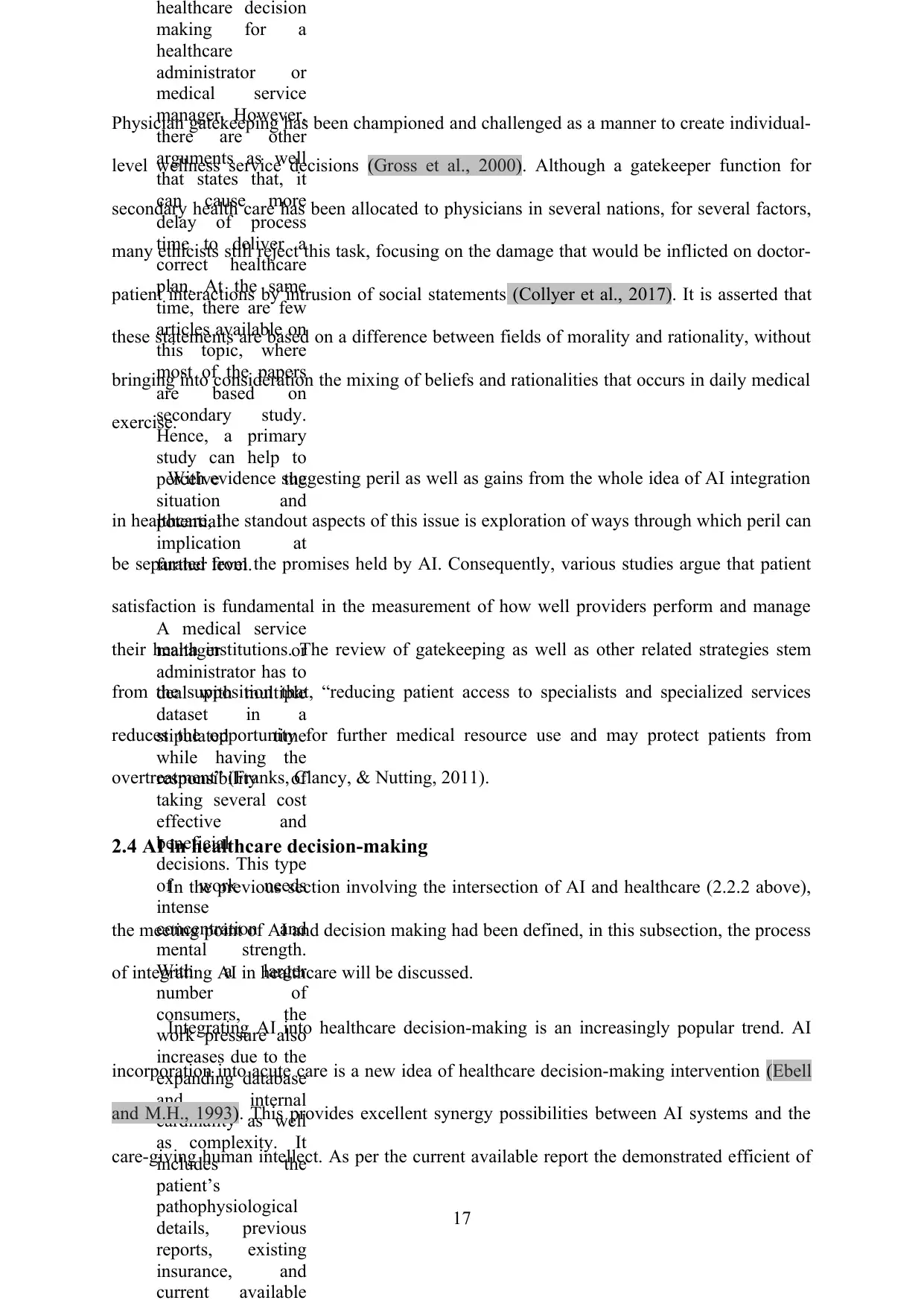
healthcare decision
making for a
healthcare
administrator or
medical service
manager. However,
there are other
arguments as well
that states that, it
can cause more
delay of process
time to deliver a
correct healthcare
plan. At the same
time, there are few
articles available on
this topic, where
most of the papers
are based on
secondary study.
Hence, a primary
study can help to
perceive the
situation and
potential
implication at
further level.
A medical service
manager or
administrator has to
deal with multiple
dataset in a
stipulated time
while having the
responsibility of
taking several cost
effective and
beneficial
decisions. This type
of work needs
intense
concentration and
mental strength.
With a larger
number of
consumers, the
work pressure also
increases due to the
expanding database
and internal
cardinality as well
as complexity. It
includes the
patient’s
pathophysiological
details, previous
reports, existing
insurance, and
current available
Physician gatekeeping has been championed and challenged as a manner to create individual-
level wellness service decisions (Gross et al., 2000). Although a gatekeeper function for
secondary health care has been allocated to physicians in several nations, for several factors,
many ethicists still reject this task, focusing on the damage that would be inflicted on doctor-
patient interactions by intrusion of social statements (Collyer et al., 2017). It is asserted that
these statements are based on a difference between fields of morality and rationality, without
bringing into consideration the mixing of beliefs and rationalities that occurs in daily medical
exercise.
With evidence suggesting peril as well as gains from the whole idea of AI integration
in healthcare, the standout aspects of this issue is exploration of ways through which peril can
be separated from the promises held by AI. Consequently, various studies argue that patient
satisfaction is fundamental in the measurement of how well providers perform and manage
their health institutions. The review of gatekeeping as well as other related strategies stem
from the supposition that, “reducing patient access to specialists and specialized services
reduces the opportunity for further medical resource use and may protect patients from
overtreatment” (Franks, Clancy, & Nutting, 2011).
2.4 AI in healthcare decision-making
In the previous section involving the intersection of AI and healthcare (2.2.2 above),
the meeting point of AI and decision making had been defined, in this subsection, the process
of integrating AI in healthcare will be discussed.
Integrating AI into healthcare decision-making is an increasingly popular trend. AI
incorporation into acute care is a new idea of healthcare decision-making intervention (Ebell
and M.H., 1993). This provides excellent synergy possibilities between AI systems and the
care-giving human intellect. As per the current available report the demonstrated efficient of
17
making for a
healthcare
administrator or
medical service
manager. However,
there are other
arguments as well
that states that, it
can cause more
delay of process
time to deliver a
correct healthcare
plan. At the same
time, there are few
articles available on
this topic, where
most of the papers
are based on
secondary study.
Hence, a primary
study can help to
perceive the
situation and
potential
implication at
further level.
A medical service
manager or
administrator has to
deal with multiple
dataset in a
stipulated time
while having the
responsibility of
taking several cost
effective and
beneficial
decisions. This type
of work needs
intense
concentration and
mental strength.
With a larger
number of
consumers, the
work pressure also
increases due to the
expanding database
and internal
cardinality as well
as complexity. It
includes the
patient’s
pathophysiological
details, previous
reports, existing
insurance, and
current available
Physician gatekeeping has been championed and challenged as a manner to create individual-
level wellness service decisions (Gross et al., 2000). Although a gatekeeper function for
secondary health care has been allocated to physicians in several nations, for several factors,
many ethicists still reject this task, focusing on the damage that would be inflicted on doctor-
patient interactions by intrusion of social statements (Collyer et al., 2017). It is asserted that
these statements are based on a difference between fields of morality and rationality, without
bringing into consideration the mixing of beliefs and rationalities that occurs in daily medical
exercise.
With evidence suggesting peril as well as gains from the whole idea of AI integration
in healthcare, the standout aspects of this issue is exploration of ways through which peril can
be separated from the promises held by AI. Consequently, various studies argue that patient
satisfaction is fundamental in the measurement of how well providers perform and manage
their health institutions. The review of gatekeeping as well as other related strategies stem
from the supposition that, “reducing patient access to specialists and specialized services
reduces the opportunity for further medical resource use and may protect patients from
overtreatment” (Franks, Clancy, & Nutting, 2011).
2.4 AI in healthcare decision-making
In the previous section involving the intersection of AI and healthcare (2.2.2 above),
the meeting point of AI and decision making had been defined, in this subsection, the process
of integrating AI in healthcare will be discussed.
Integrating AI into healthcare decision-making is an increasingly popular trend. AI
incorporation into acute care is a new idea of healthcare decision-making intervention (Ebell
and M.H., 1993). This provides excellent synergy possibilities between AI systems and the
care-giving human intellect. As per the current available report the demonstrated efficient of
17
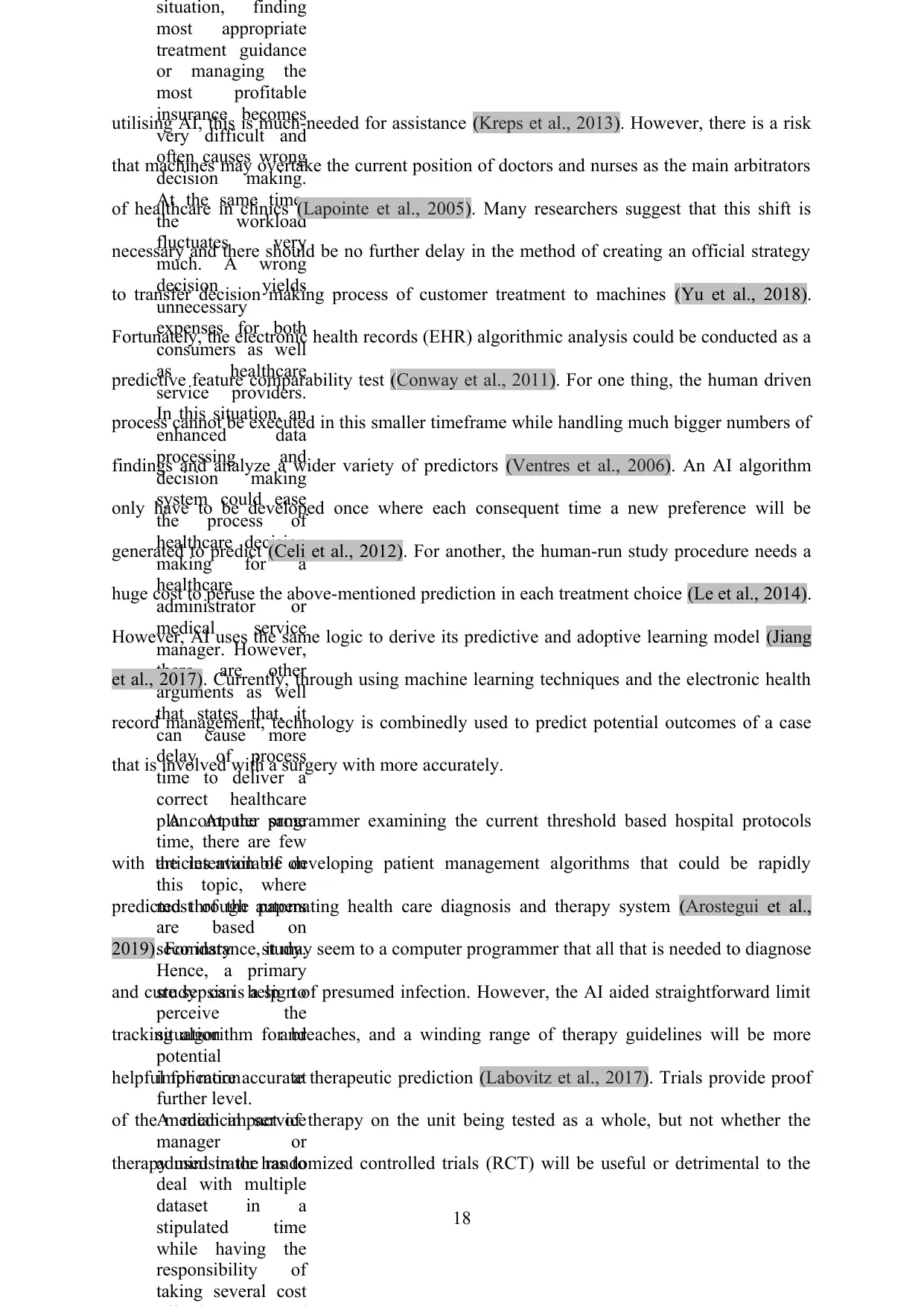
situation, finding
most appropriate
treatment guidance
or managing the
most profitable
insurance becomes
very difficult and
often causes wrong
decision making.
At the same time,
the workload
fluctuates very
much. A wrong
decision yields
unnecessary
expenses for both
consumers as well
as healthcare
service providers.
In this situation, an
enhanced data
processing and
decision making
system could ease
the process of
healthcare decision
making for a
healthcare
administrator or
medical service
manager. However,
there are other
arguments as well
that states that, it
can cause more
delay of process
time to deliver a
correct healthcare
plan. At the same
time, there are few
articles available on
this topic, where
most of the papers
are based on
secondary study.
Hence, a primary
study can help to
perceive the
situation and
potential
implication at
further level.
A medical service
manager or
administrator has to
deal with multiple
dataset in a
stipulated time
while having the
responsibility of
taking several cost
utilising AI, this is much-needed for assistance (Kreps et al., 2013). However, there is a risk
that machines may overtake the current position of doctors and nurses as the main arbitrators
of healthcare in clinics (Lapointe et al., 2005). Many researchers suggest that this shift is
necessary and there should be no further delay in the method of creating an official strategy
to transfer decision making process of customer treatment to machines (Yu et al., 2018).
Fortunately, the electronic health records (EHR) algorithmic analysis could be conducted as a
predictive feature comparability test (Conway et al., 2011). For one thing, the human driven
process cannot be executed in this smaller timeframe while handling much bigger numbers of
findings and analyze a wider variety of predictors (Ventres et al., 2006). An AI algorithm
only have to be developed once where each consequent time a new preference will be
generated to predict (Celi et al., 2012). For another, the human-run study procedure needs a
huge cost to peruse the above-mentioned prediction in each treatment choice (Le et al., 2014).
However, AI uses the same logic to derive its predictive and adoptive learning model (Jiang
et al., 2017). Currently, through using machine learning techniques and the electronic health
record management, technology is combinedly used to predict potential outcomes of a case
that is involved with a surgery with more accurately.
A computer programmer examining the current threshold based hospital protocols
with the intention of developing patient management algorithms that could be rapidly
predicted through automating health care diagnosis and therapy system (Arostegui et al.,
2019). For instance, it may seem to a computer programmer that all that is needed to diagnose
and cure sepsis is a sign of presumed infection. However, the AI aided straightforward limit
tracking algorithm for breaches, and a winding range of therapy guidelines will be more
helpful for more accurate therapeutic prediction (Labovitz et al., 2017). Trials provide proof
of the median impact of therapy on the unit being tested as a whole, but not whether the
therapy used in the randomized controlled trials (RCT) will be useful or detrimental to the
18
most appropriate
treatment guidance
or managing the
most profitable
insurance becomes
very difficult and
often causes wrong
decision making.
At the same time,
the workload
fluctuates very
much. A wrong
decision yields
unnecessary
expenses for both
consumers as well
as healthcare
service providers.
In this situation, an
enhanced data
processing and
decision making
system could ease
the process of
healthcare decision
making for a
healthcare
administrator or
medical service
manager. However,
there are other
arguments as well
that states that, it
can cause more
delay of process
time to deliver a
correct healthcare
plan. At the same
time, there are few
articles available on
this topic, where
most of the papers
are based on
secondary study.
Hence, a primary
study can help to
perceive the
situation and
potential
implication at
further level.
A medical service
manager or
administrator has to
deal with multiple
dataset in a
stipulated time
while having the
responsibility of
taking several cost
utilising AI, this is much-needed for assistance (Kreps et al., 2013). However, there is a risk
that machines may overtake the current position of doctors and nurses as the main arbitrators
of healthcare in clinics (Lapointe et al., 2005). Many researchers suggest that this shift is
necessary and there should be no further delay in the method of creating an official strategy
to transfer decision making process of customer treatment to machines (Yu et al., 2018).
Fortunately, the electronic health records (EHR) algorithmic analysis could be conducted as a
predictive feature comparability test (Conway et al., 2011). For one thing, the human driven
process cannot be executed in this smaller timeframe while handling much bigger numbers of
findings and analyze a wider variety of predictors (Ventres et al., 2006). An AI algorithm
only have to be developed once where each consequent time a new preference will be
generated to predict (Celi et al., 2012). For another, the human-run study procedure needs a
huge cost to peruse the above-mentioned prediction in each treatment choice (Le et al., 2014).
However, AI uses the same logic to derive its predictive and adoptive learning model (Jiang
et al., 2017). Currently, through using machine learning techniques and the electronic health
record management, technology is combinedly used to predict potential outcomes of a case
that is involved with a surgery with more accurately.
A computer programmer examining the current threshold based hospital protocols
with the intention of developing patient management algorithms that could be rapidly
predicted through automating health care diagnosis and therapy system (Arostegui et al.,
2019). For instance, it may seem to a computer programmer that all that is needed to diagnose
and cure sepsis is a sign of presumed infection. However, the AI aided straightforward limit
tracking algorithm for breaches, and a winding range of therapy guidelines will be more
helpful for more accurate therapeutic prediction (Labovitz et al., 2017). Trials provide proof
of the median impact of therapy on the unit being tested as a whole, but not whether the
therapy used in the randomized controlled trials (RCT) will be useful or detrimental to the
18
Paraphrase This Document
Need a fresh take? Get an instant paraphrase of this document with our AI Paraphraser
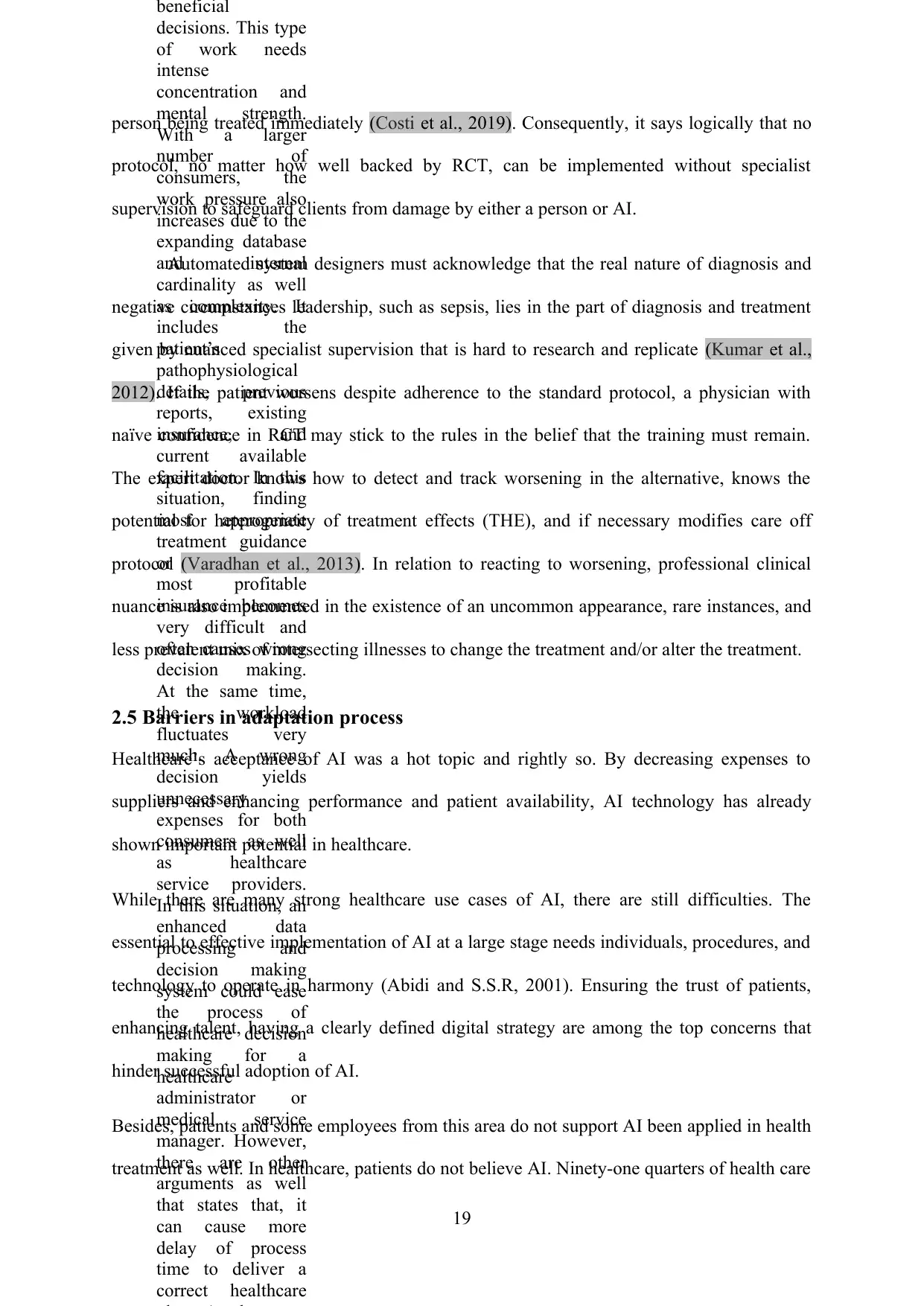
beneficial
decisions. This type
of work needs
intense
concentration and
mental strength.
With a larger
number of
consumers, the
work pressure also
increases due to the
expanding database
and internal
cardinality as well
as complexity. It
includes the
patient’s
pathophysiological
details, previous
reports, existing
insurance, and
current available
facilitation. In this
situation, finding
most appropriate
treatment guidance
or managing the
most profitable
insurance becomes
very difficult and
often causes wrong
decision making.
At the same time,
the workload
fluctuates very
much. A wrong
decision yields
unnecessary
expenses for both
consumers as well
as healthcare
service providers.
In this situation, an
enhanced data
processing and
decision making
system could ease
the process of
healthcare decision
making for a
healthcare
administrator or
medical service
manager. However,
there are other
arguments as well
that states that, it
can cause more
delay of process
time to deliver a
correct healthcare
person being treated immediately (Costi et al., 2019). Consequently, it says logically that no
protocol, no matter how well backed by RCT, can be implemented without specialist
supervision to safeguard clients from damage by either a person or AI.
Automated system designers must acknowledge that the real nature of diagnosis and
negative circumstances leadership, such as sepsis, lies in the part of diagnosis and treatment
given by nuanced specialist supervision that is hard to research and replicate (Kumar et al.,
2012). If the patient worsens despite adherence to the standard protocol, a physician with
naïve confidence in RCT may stick to the rules in the belief that the training must remain.
The expert doctor knows how to detect and track worsening in the alternative, knows the
potential for heterogeneity of treatment effects (THE), and if necessary modifies care off
protocol (Varadhan et al., 2013). In relation to reacting to worsening, professional clinical
nuance is also implemented in the existence of an uncommon appearance, rare instances, and
less prevalent mix of intersecting illnesses to change the treatment and/or alter the treatment.
2.5 Barriers in adaptation process
Healthcare’s acceptance of AI was a hot topic and rightly so. By decreasing expenses to
suppliers and enhancing performance and patient availability, AI technology has already
shown important potential in healthcare.
While there are many strong healthcare use cases of AI, there are still difficulties. The
essential to effective implementation of AI at a large stage needs individuals, procedures, and
technology to operate in harmony (Abidi and S.S.R, 2001). Ensuring the trust of patients,
enhancing talent, having a clearly defined digital strategy are among the top concerns that
hinder successful adoption of AI.
Besides, patients and some employees from this area do not support AI been applied in health
treatment as well. In healthcare, patients do not believe AI. Ninety-one quarters of health care
19
decisions. This type
of work needs
intense
concentration and
mental strength.
With a larger
number of
consumers, the
work pressure also
increases due to the
expanding database
and internal
cardinality as well
as complexity. It
includes the
patient’s
pathophysiological
details, previous
reports, existing
insurance, and
current available
facilitation. In this
situation, finding
most appropriate
treatment guidance
or managing the
most profitable
insurance becomes
very difficult and
often causes wrong
decision making.
At the same time,
the workload
fluctuates very
much. A wrong
decision yields
unnecessary
expenses for both
consumers as well
as healthcare
service providers.
In this situation, an
enhanced data
processing and
decision making
system could ease
the process of
healthcare decision
making for a
healthcare
administrator or
medical service
manager. However,
there are other
arguments as well
that states that, it
can cause more
delay of process
time to deliver a
correct healthcare
person being treated immediately (Costi et al., 2019). Consequently, it says logically that no
protocol, no matter how well backed by RCT, can be implemented without specialist
supervision to safeguard clients from damage by either a person or AI.
Automated system designers must acknowledge that the real nature of diagnosis and
negative circumstances leadership, such as sepsis, lies in the part of diagnosis and treatment
given by nuanced specialist supervision that is hard to research and replicate (Kumar et al.,
2012). If the patient worsens despite adherence to the standard protocol, a physician with
naïve confidence in RCT may stick to the rules in the belief that the training must remain.
The expert doctor knows how to detect and track worsening in the alternative, knows the
potential for heterogeneity of treatment effects (THE), and if necessary modifies care off
protocol (Varadhan et al., 2013). In relation to reacting to worsening, professional clinical
nuance is also implemented in the existence of an uncommon appearance, rare instances, and
less prevalent mix of intersecting illnesses to change the treatment and/or alter the treatment.
2.5 Barriers in adaptation process
Healthcare’s acceptance of AI was a hot topic and rightly so. By decreasing expenses to
suppliers and enhancing performance and patient availability, AI technology has already
shown important potential in healthcare.
While there are many strong healthcare use cases of AI, there are still difficulties. The
essential to effective implementation of AI at a large stage needs individuals, procedures, and
technology to operate in harmony (Abidi and S.S.R, 2001). Ensuring the trust of patients,
enhancing talent, having a clearly defined digital strategy are among the top concerns that
hinder successful adoption of AI.
Besides, patients and some employees from this area do not support AI been applied in health
treatment as well. In healthcare, patients do not believe AI. Ninety-one quarters of health care
19
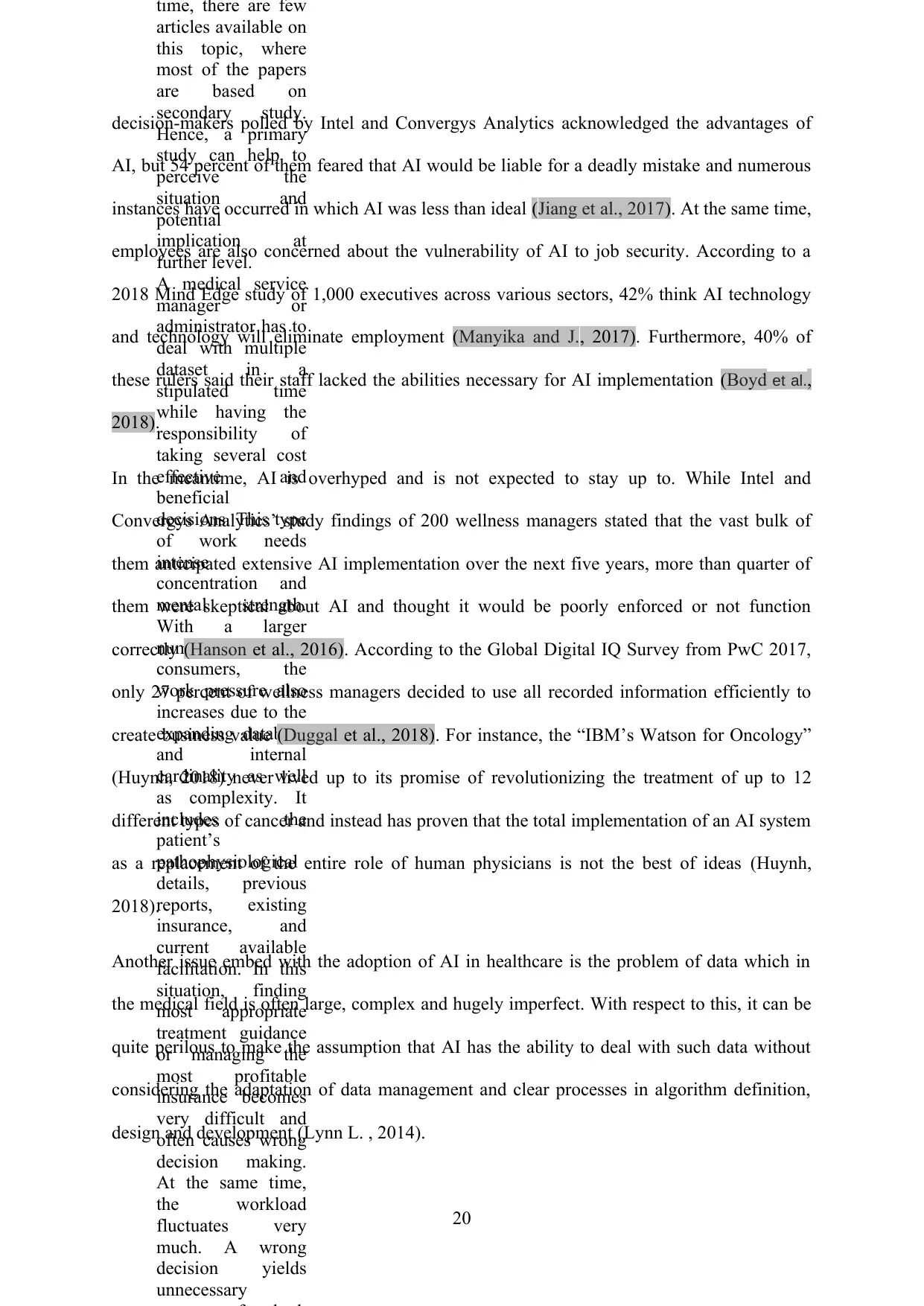
time, there are few
articles available on
this topic, where
most of the papers
are based on
secondary study.
Hence, a primary
study can help to
perceive the
situation and
potential
implication at
further level.
A medical service
manager or
administrator has to
deal with multiple
dataset in a
stipulated time
while having the
responsibility of
taking several cost
effective and
beneficial
decisions. This type
of work needs
intense
concentration and
mental strength.
With a larger
number of
consumers, the
work pressure also
increases due to the
expanding database
and internal
cardinality as well
as complexity. It
includes the
patient’s
pathophysiological
details, previous
reports, existing
insurance, and
current available
facilitation. In this
situation, finding
most appropriate
treatment guidance
or managing the
most profitable
insurance becomes
very difficult and
often causes wrong
decision making.
At the same time,
the workload
fluctuates very
much. A wrong
decision yields
unnecessary
decision-makers polled by Intel and Convergys Analytics acknowledged the advantages of
AI, but 54 percent of them feared that AI would be liable for a deadly mistake and numerous
instances have occurred in which AI was less than ideal (Jiang et al., 2017). At the same time,
employees are also concerned about the vulnerability of AI to job security. According to a
2018 Mind Edge study of 1,000 executives across various sectors, 42% think AI technology
and technology will eliminate employment (Manyika and J., 2017). Furthermore, 40% of
these rulers said their staff lacked the abilities necessary for AI implementation (Boyd et al.,
2018).
In the meantime, AI is overhyped and is not expected to stay up to. While Intel and
Convergys Analytics’ study findings of 200 wellness managers stated that the vast bulk of
them anticipated extensive AI implementation over the next five years, more than quarter of
them were skeptical about AI and thought it would be poorly enforced or not function
correctly (Hanson et al., 2016). According to the Global Digital IQ Survey from PwC 2017,
only 27 percent of wellness managers decided to use all recorded information efficiently to
create business value (Duggal et al., 2018). For instance, the “IBM’s Watson for Oncology”
(Huynh, 2018) never lived up to its promise of revolutionizing the treatment of up to 12
different types of cancer and instead has proven that the total implementation of an AI system
as a replacement of the entire role of human physicians is not the best of ideas (Huynh,
2018).
Another issue embed with the adoption of AI in healthcare is the problem of data which in
the medical field is often large, complex and hugely imperfect. With respect to this, it can be
quite perilous to make the assumption that AI has the ability to deal with such data without
considering the adaptation of data management and clear processes in algorithm definition,
design and development (Lynn L. , 2014).
20
articles available on
this topic, where
most of the papers
are based on
secondary study.
Hence, a primary
study can help to
perceive the
situation and
potential
implication at
further level.
A medical service
manager or
administrator has to
deal with multiple
dataset in a
stipulated time
while having the
responsibility of
taking several cost
effective and
beneficial
decisions. This type
of work needs
intense
concentration and
mental strength.
With a larger
number of
consumers, the
work pressure also
increases due to the
expanding database
and internal
cardinality as well
as complexity. It
includes the
patient’s
pathophysiological
details, previous
reports, existing
insurance, and
current available
facilitation. In this
situation, finding
most appropriate
treatment guidance
or managing the
most profitable
insurance becomes
very difficult and
often causes wrong
decision making.
At the same time,
the workload
fluctuates very
much. A wrong
decision yields
unnecessary
decision-makers polled by Intel and Convergys Analytics acknowledged the advantages of
AI, but 54 percent of them feared that AI would be liable for a deadly mistake and numerous
instances have occurred in which AI was less than ideal (Jiang et al., 2017). At the same time,
employees are also concerned about the vulnerability of AI to job security. According to a
2018 Mind Edge study of 1,000 executives across various sectors, 42% think AI technology
and technology will eliminate employment (Manyika and J., 2017). Furthermore, 40% of
these rulers said their staff lacked the abilities necessary for AI implementation (Boyd et al.,
2018).
In the meantime, AI is overhyped and is not expected to stay up to. While Intel and
Convergys Analytics’ study findings of 200 wellness managers stated that the vast bulk of
them anticipated extensive AI implementation over the next five years, more than quarter of
them were skeptical about AI and thought it would be poorly enforced or not function
correctly (Hanson et al., 2016). According to the Global Digital IQ Survey from PwC 2017,
only 27 percent of wellness managers decided to use all recorded information efficiently to
create business value (Duggal et al., 2018). For instance, the “IBM’s Watson for Oncology”
(Huynh, 2018) never lived up to its promise of revolutionizing the treatment of up to 12
different types of cancer and instead has proven that the total implementation of an AI system
as a replacement of the entire role of human physicians is not the best of ideas (Huynh,
2018).
Another issue embed with the adoption of AI in healthcare is the problem of data which in
the medical field is often large, complex and hugely imperfect. With respect to this, it can be
quite perilous to make the assumption that AI has the ability to deal with such data without
considering the adaptation of data management and clear processes in algorithm definition,
design and development (Lynn L. , 2014).
20
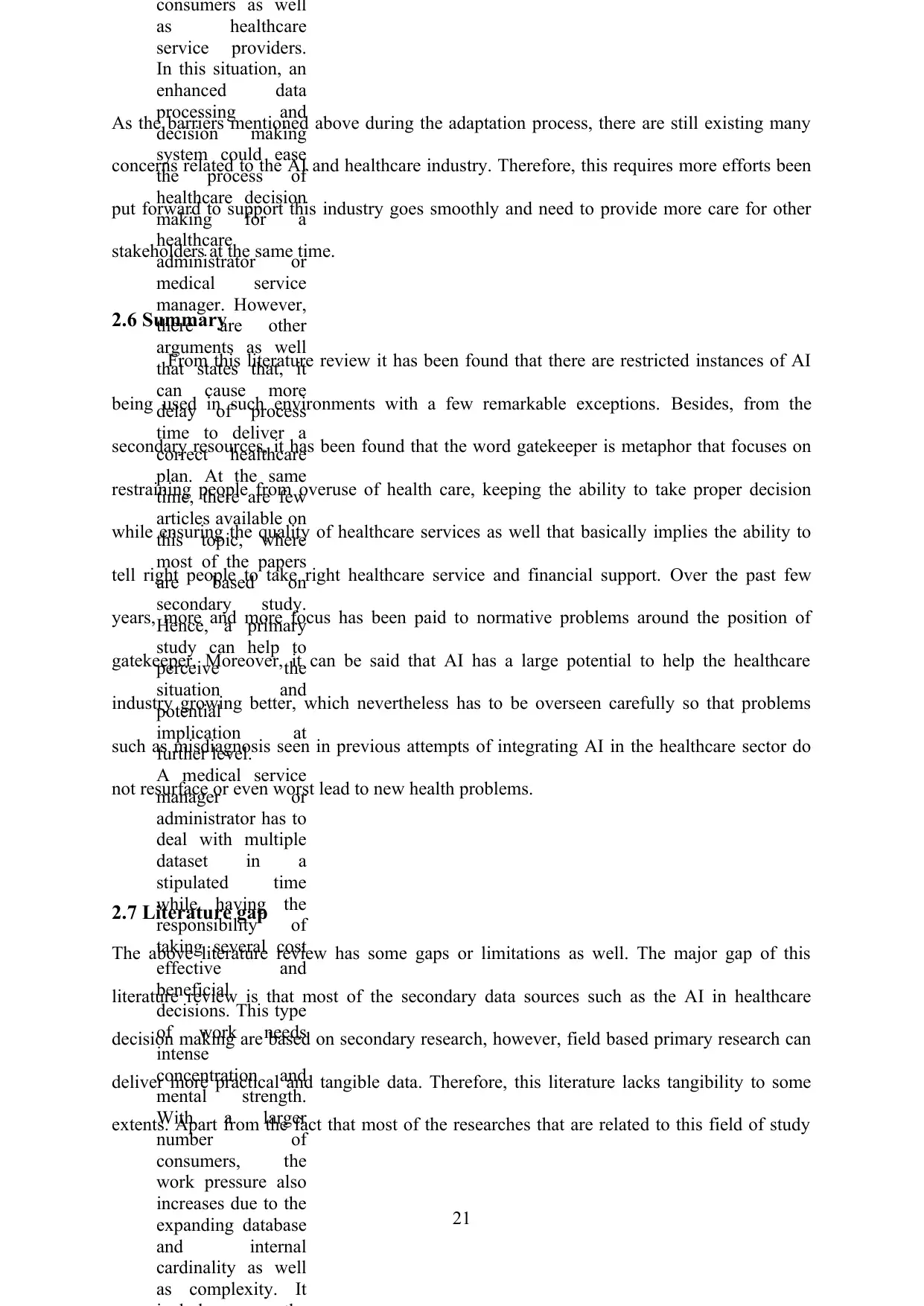
consumers as well
as healthcare
service providers.
In this situation, an
enhanced data
processing and
decision making
system could ease
the process of
healthcare decision
making for a
healthcare
administrator or
medical service
manager. However,
there are other
arguments as well
that states that, it
can cause more
delay of process
time to deliver a
correct healthcare
plan. At the same
time, there are few
articles available on
this topic, where
most of the papers
are based on
secondary study.
Hence, a primary
study can help to
perceive the
situation and
potential
implication at
further level.
A medical service
manager or
administrator has to
deal with multiple
dataset in a
stipulated time
while having the
responsibility of
taking several cost
effective and
beneficial
decisions. This type
of work needs
intense
concentration and
mental strength.
With a larger
number of
consumers, the
work pressure also
increases due to the
expanding database
and internal
cardinality as well
as complexity. It
As the barriers mentioned above during the adaptation process, there are still existing many
concerns related to the AI and healthcare industry. Therefore, this requires more efforts been
put forward to support this industry goes smoothly and need to provide more care for other
stakeholders at the same time.
2.6 Summary
From this literature review it has been found that there are restricted instances of AI
being used in such environments with a few remarkable exceptions. Besides, from the
secondary resources, it has been found that the word gatekeeper is metaphor that focuses on
restraining people from overuse of health care, keeping the ability to take proper decision
while ensuring the quality of healthcare services as well that basically implies the ability to
tell right people to take right healthcare service and financial support. Over the past few
years, more and more focus has been paid to normative problems around the position of
gatekeeper. Moreover, it can be said that AI has a large potential to help the healthcare
industry growing better, which nevertheless has to be overseen carefully so that problems
such as misdiagnosis seen in previous attempts of integrating AI in the healthcare sector do
not resurface or even worst lead to new health problems.
2.7 Literature gap
The above literature review has some gaps or limitations as well. The major gap of this
literature review is that most of the secondary data sources such as the AI in healthcare
decision making are based on secondary research, however, field based primary research can
deliver more practical and tangible data. Therefore, this literature lacks tangibility to some
extents. Apart from the fact that most of the researches that are related to this field of study
21
as healthcare
service providers.
In this situation, an
enhanced data
processing and
decision making
system could ease
the process of
healthcare decision
making for a
healthcare
administrator or
medical service
manager. However,
there are other
arguments as well
that states that, it
can cause more
delay of process
time to deliver a
correct healthcare
plan. At the same
time, there are few
articles available on
this topic, where
most of the papers
are based on
secondary study.
Hence, a primary
study can help to
perceive the
situation and
potential
implication at
further level.
A medical service
manager or
administrator has to
deal with multiple
dataset in a
stipulated time
while having the
responsibility of
taking several cost
effective and
beneficial
decisions. This type
of work needs
intense
concentration and
mental strength.
With a larger
number of
consumers, the
work pressure also
increases due to the
expanding database
and internal
cardinality as well
as complexity. It
As the barriers mentioned above during the adaptation process, there are still existing many
concerns related to the AI and healthcare industry. Therefore, this requires more efforts been
put forward to support this industry goes smoothly and need to provide more care for other
stakeholders at the same time.
2.6 Summary
From this literature review it has been found that there are restricted instances of AI
being used in such environments with a few remarkable exceptions. Besides, from the
secondary resources, it has been found that the word gatekeeper is metaphor that focuses on
restraining people from overuse of health care, keeping the ability to take proper decision
while ensuring the quality of healthcare services as well that basically implies the ability to
tell right people to take right healthcare service and financial support. Over the past few
years, more and more focus has been paid to normative problems around the position of
gatekeeper. Moreover, it can be said that AI has a large potential to help the healthcare
industry growing better, which nevertheless has to be overseen carefully so that problems
such as misdiagnosis seen in previous attempts of integrating AI in the healthcare sector do
not resurface or even worst lead to new health problems.
2.7 Literature gap
The above literature review has some gaps or limitations as well. The major gap of this
literature review is that most of the secondary data sources such as the AI in healthcare
decision making are based on secondary research, however, field based primary research can
deliver more practical and tangible data. Therefore, this literature lacks tangibility to some
extents. Apart from the fact that most of the researches that are related to this field of study
21
Secure Best Marks with AI Grader
Need help grading? Try our AI Grader for instant feedback on your assignments.
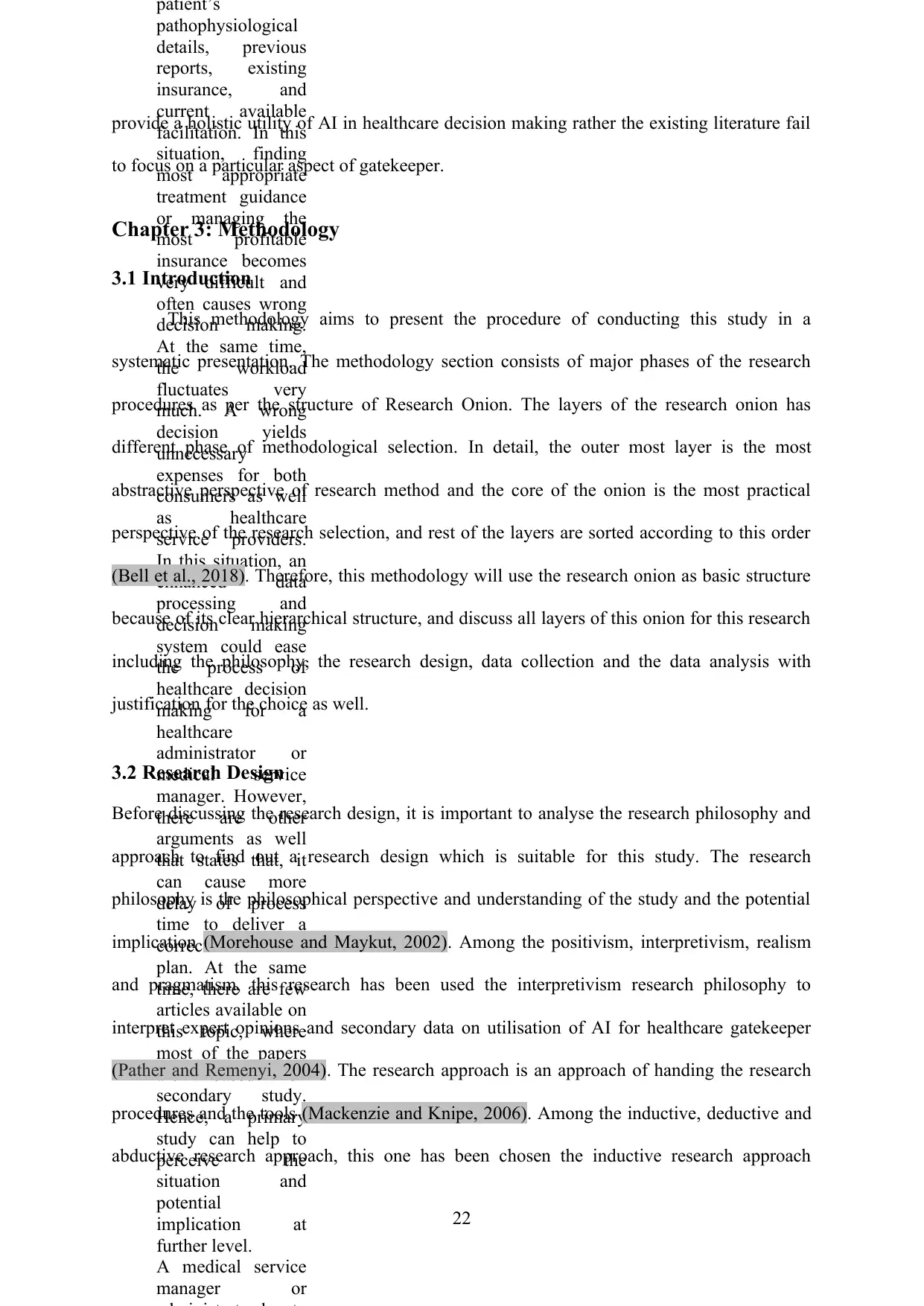
patient’s
pathophysiological
details, previous
reports, existing
insurance, and
current available
facilitation. In this
situation, finding
most appropriate
treatment guidance
or managing the
most profitable
insurance becomes
very difficult and
often causes wrong
decision making.
At the same time,
the workload
fluctuates very
much. A wrong
decision yields
unnecessary
expenses for both
consumers as well
as healthcare
service providers.
In this situation, an
enhanced data
processing and
decision making
system could ease
the process of
healthcare decision
making for a
healthcare
administrator or
medical service
manager. However,
there are other
arguments as well
that states that, it
can cause more
delay of process
time to deliver a
correct healthcare
plan. At the same
time, there are few
articles available on
this topic, where
most of the papers
are based on
secondary study.
Hence, a primary
study can help to
perceive the
situation and
potential
implication at
further level.
A medical service
manager or
provide a holistic utility of AI in healthcare decision making rather the existing literature fail
to focus on a particular aspect of gatekeeper.
Chapter 3: Methodology
3.1 Introduction
This methodology aims to present the procedure of conducting this study in a
systematic presentation. The methodology section consists of major phases of the research
procedures as per the structure of Research Onion. The layers of the research onion has
different phase of methodological selection. In detail, the outer most layer is the most
abstractive perspective of research method and the core of the onion is the most practical
perspective of the research selection, and rest of the layers are sorted according to this order
(Bell et al., 2018). Therefore, this methodology will use the research onion as basic structure
because of its clear hierarchical structure, and discuss all layers of this onion for this research
including the philosophy, the research design, data collection and the data analysis with
justification for the choice as well.
3.2 Research Design
Before discussing the research design, it is important to analyse the research philosophy and
approach to find out a research design which is suitable for this study. The research
philosophy is the philosophical perspective and understanding of the study and the potential
implication (Morehouse and Maykut, 2002). Among the positivism, interpretivism, realism
and pragmatism, this research has been used the interpretivism research philosophy to
interpret expert opinions and secondary data on utilisation of AI for healthcare gatekeeper
(Pather and Remenyi, 2004). The research approach is an approach of handing the research
procedures and the tools (Mackenzie and Knipe, 2006). Among the inductive, deductive and
abductive research approach, this one has been chosen the inductive research approach
22
pathophysiological
details, previous
reports, existing
insurance, and
current available
facilitation. In this
situation, finding
most appropriate
treatment guidance
or managing the
most profitable
insurance becomes
very difficult and
often causes wrong
decision making.
At the same time,
the workload
fluctuates very
much. A wrong
decision yields
unnecessary
expenses for both
consumers as well
as healthcare
service providers.
In this situation, an
enhanced data
processing and
decision making
system could ease
the process of
healthcare decision
making for a
healthcare
administrator or
medical service
manager. However,
there are other
arguments as well
that states that, it
can cause more
delay of process
time to deliver a
correct healthcare
plan. At the same
time, there are few
articles available on
this topic, where
most of the papers
are based on
secondary study.
Hence, a primary
study can help to
perceive the
situation and
potential
implication at
further level.
A medical service
manager or
provide a holistic utility of AI in healthcare decision making rather the existing literature fail
to focus on a particular aspect of gatekeeper.
Chapter 3: Methodology
3.1 Introduction
This methodology aims to present the procedure of conducting this study in a
systematic presentation. The methodology section consists of major phases of the research
procedures as per the structure of Research Onion. The layers of the research onion has
different phase of methodological selection. In detail, the outer most layer is the most
abstractive perspective of research method and the core of the onion is the most practical
perspective of the research selection, and rest of the layers are sorted according to this order
(Bell et al., 2018). Therefore, this methodology will use the research onion as basic structure
because of its clear hierarchical structure, and discuss all layers of this onion for this research
including the philosophy, the research design, data collection and the data analysis with
justification for the choice as well.
3.2 Research Design
Before discussing the research design, it is important to analyse the research philosophy and
approach to find out a research design which is suitable for this study. The research
philosophy is the philosophical perspective and understanding of the study and the potential
implication (Morehouse and Maykut, 2002). Among the positivism, interpretivism, realism
and pragmatism, this research has been used the interpretivism research philosophy to
interpret expert opinions and secondary data on utilisation of AI for healthcare gatekeeper
(Pather and Remenyi, 2004). The research approach is an approach of handing the research
procedures and the tools (Mackenzie and Knipe, 2006). Among the inductive, deductive and
abductive research approach, this one has been chosen the inductive research approach
22
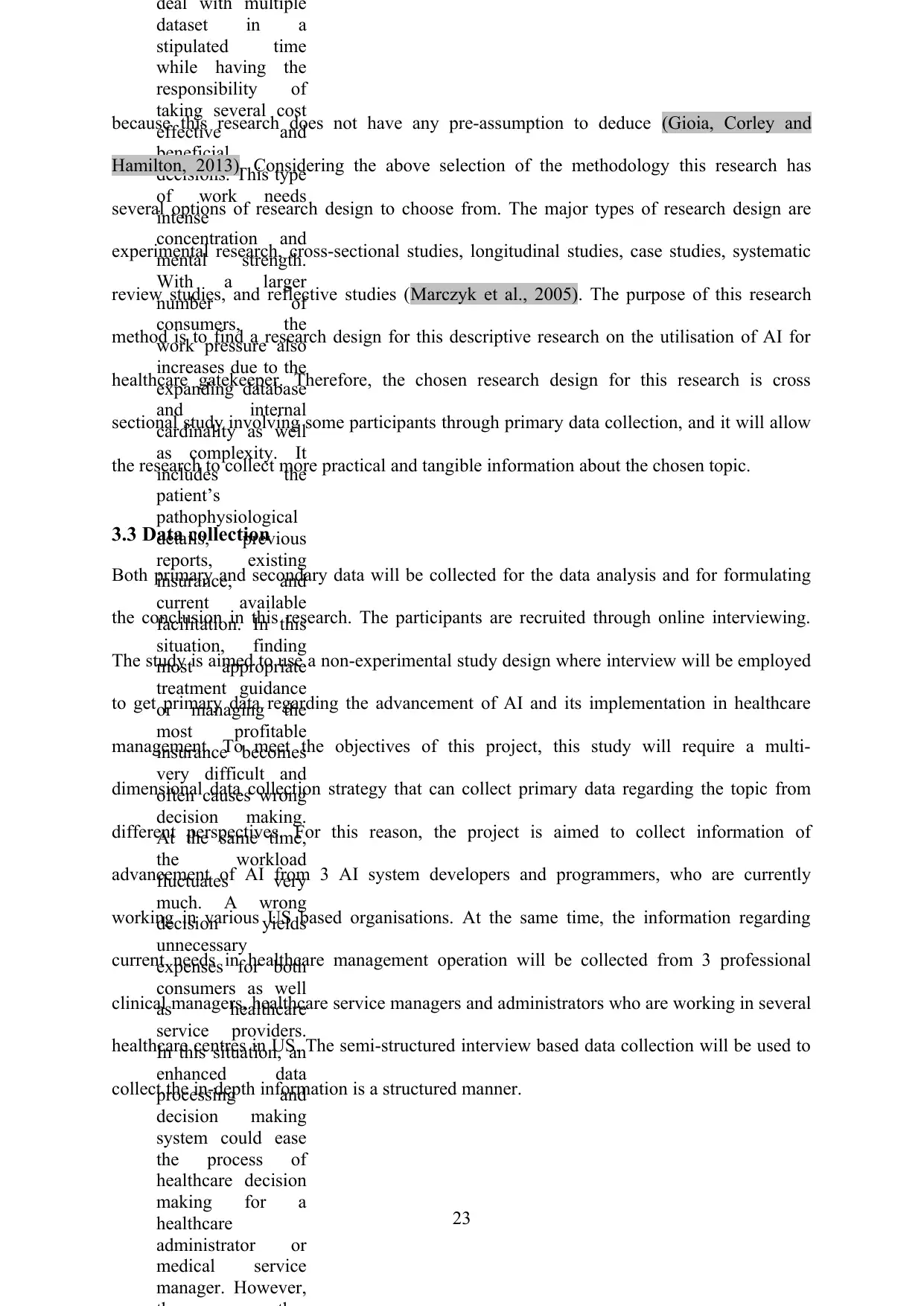
deal with multiple
dataset in a
stipulated time
while having the
responsibility of
taking several cost
effective and
beneficial
decisions. This type
of work needs
intense
concentration and
mental strength.
With a larger
number of
consumers, the
work pressure also
increases due to the
expanding database
and internal
cardinality as well
as complexity. It
includes the
patient’s
pathophysiological
details, previous
reports, existing
insurance, and
current available
facilitation. In this
situation, finding
most appropriate
treatment guidance
or managing the
most profitable
insurance becomes
very difficult and
often causes wrong
decision making.
At the same time,
the workload
fluctuates very
much. A wrong
decision yields
unnecessary
expenses for both
consumers as well
as healthcare
service providers.
In this situation, an
enhanced data
processing and
decision making
system could ease
the process of
healthcare decision
making for a
healthcare
administrator or
medical service
manager. However,
because this research does not have any pre-assumption to deduce (Gioia, Corley and
Hamilton, 2013). Considering the above selection of the methodology this research has
several options of research design to choose from. The major types of research design are
experimental research, cross-sectional studies, longitudinal studies, case studies, systematic
review studies, and reflective studies (Marczyk et al., 2005). The purpose of this research
method is to find a research design for this descriptive research on the utilisation of AI for
healthcare gatekeeper. Therefore, the chosen research design for this research is cross
sectional study involving some participants through primary data collection, and it will allow
the research to collect more practical and tangible information about the chosen topic.
3.3 Data collection
Both primary and secondary data will be collected for the data analysis and for formulating
the conclusion in this research. The participants are recruited through online interviewing.
The study is aimed to use a non-experimental study design where interview will be employed
to get primary data regarding the advancement of AI and its implementation in healthcare
management. To meet the objectives of this project, this study will require a multi-
dimensional data collection strategy that can collect primary data regarding the topic from
different perspectives. For this reason, the project is aimed to collect information of
advancement of AI from 3 AI system developers and programmers, who are currently
working in various US based organisations. At the same time, the information regarding
current needs in healthcare management operation will be collected from 3 professional
clinical managers, healthcare service managers and administrators who are working in several
healthcare centres in US. The semi-structured interview based data collection will be used to
collect the in-depth information is a structured manner.
23
dataset in a
stipulated time
while having the
responsibility of
taking several cost
effective and
beneficial
decisions. This type
of work needs
intense
concentration and
mental strength.
With a larger
number of
consumers, the
work pressure also
increases due to the
expanding database
and internal
cardinality as well
as complexity. It
includes the
patient’s
pathophysiological
details, previous
reports, existing
insurance, and
current available
facilitation. In this
situation, finding
most appropriate
treatment guidance
or managing the
most profitable
insurance becomes
very difficult and
often causes wrong
decision making.
At the same time,
the workload
fluctuates very
much. A wrong
decision yields
unnecessary
expenses for both
consumers as well
as healthcare
service providers.
In this situation, an
enhanced data
processing and
decision making
system could ease
the process of
healthcare decision
making for a
healthcare
administrator or
medical service
manager. However,
because this research does not have any pre-assumption to deduce (Gioia, Corley and
Hamilton, 2013). Considering the above selection of the methodology this research has
several options of research design to choose from. The major types of research design are
experimental research, cross-sectional studies, longitudinal studies, case studies, systematic
review studies, and reflective studies (Marczyk et al., 2005). The purpose of this research
method is to find a research design for this descriptive research on the utilisation of AI for
healthcare gatekeeper. Therefore, the chosen research design for this research is cross
sectional study involving some participants through primary data collection, and it will allow
the research to collect more practical and tangible information about the chosen topic.
3.3 Data collection
Both primary and secondary data will be collected for the data analysis and for formulating
the conclusion in this research. The participants are recruited through online interviewing.
The study is aimed to use a non-experimental study design where interview will be employed
to get primary data regarding the advancement of AI and its implementation in healthcare
management. To meet the objectives of this project, this study will require a multi-
dimensional data collection strategy that can collect primary data regarding the topic from
different perspectives. For this reason, the project is aimed to collect information of
advancement of AI from 3 AI system developers and programmers, who are currently
working in various US based organisations. At the same time, the information regarding
current needs in healthcare management operation will be collected from 3 professional
clinical managers, healthcare service managers and administrators who are working in several
healthcare centres in US. The semi-structured interview based data collection will be used to
collect the in-depth information is a structured manner.
23
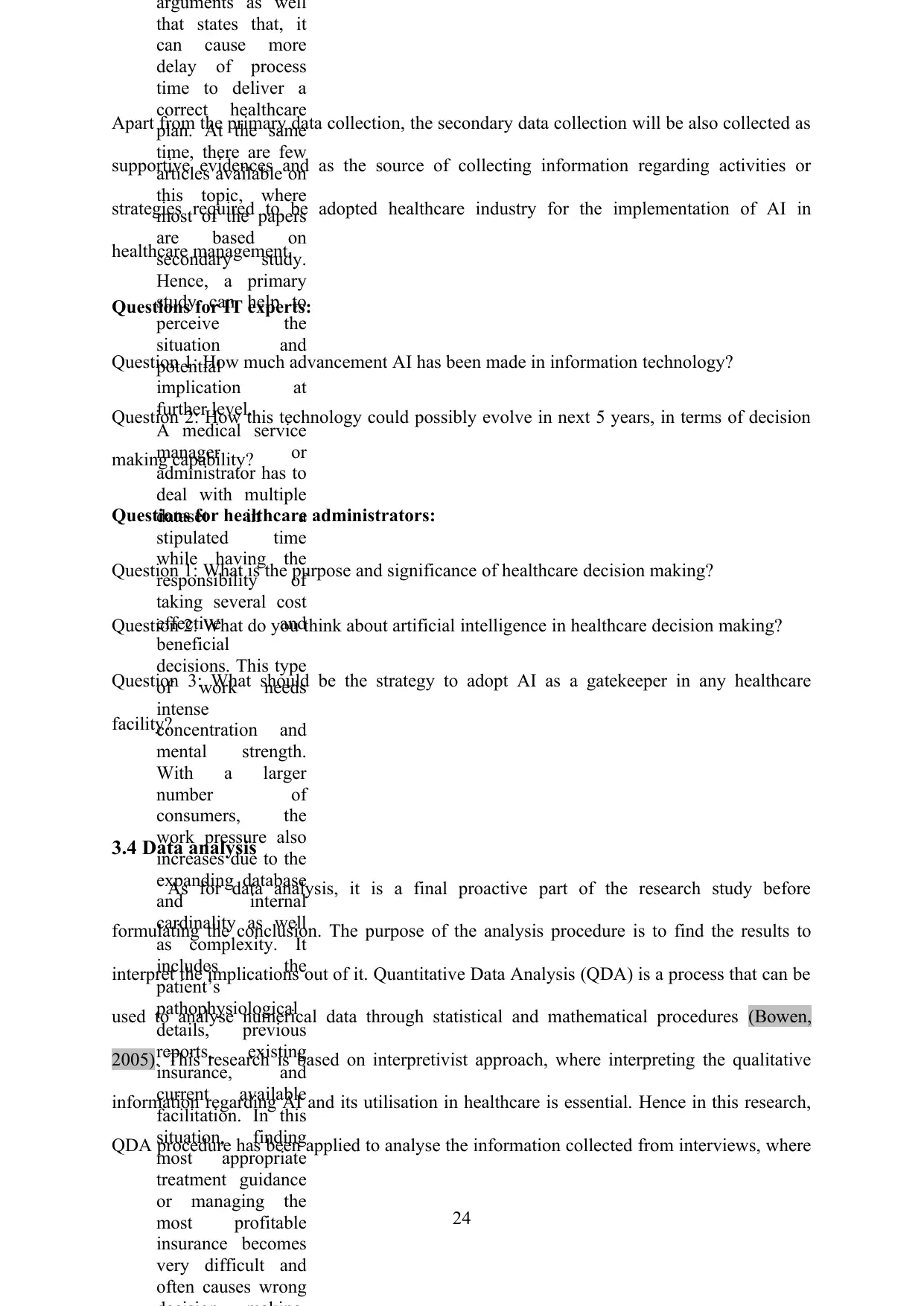
arguments as well
that states that, it
can cause more
delay of process
time to deliver a
correct healthcare
plan. At the same
time, there are few
articles available on
this topic, where
most of the papers
are based on
secondary study.
Hence, a primary
study can help to
perceive the
situation and
potential
implication at
further level.
A medical service
manager or
administrator has to
deal with multiple
dataset in a
stipulated time
while having the
responsibility of
taking several cost
effective and
beneficial
decisions. This type
of work needs
intense
concentration and
mental strength.
With a larger
number of
consumers, the
work pressure also
increases due to the
expanding database
and internal
cardinality as well
as complexity. It
includes the
patient’s
pathophysiological
details, previous
reports, existing
insurance, and
current available
facilitation. In this
situation, finding
most appropriate
treatment guidance
or managing the
most profitable
insurance becomes
very difficult and
often causes wrong
Apart from the primary data collection, the secondary data collection will be also collected as
supportive evidences and as the source of collecting information regarding activities or
strategies required to be adopted healthcare industry for the implementation of AI in
healthcare management.
Questions for IT experts:
Question 1: How much advancement AI has been made in information technology?
Question 2: How this technology could possibly evolve in next 5 years, in terms of decision
making capability?
Questions for healthcare administrators:
Question 1: What is the purpose and significance of healthcare decision making?
Question 2: What do you think about artificial intelligence in healthcare decision making?
Question 3: What should be the strategy to adopt AI as a gatekeeper in any healthcare
facility?
3.4 Data analysis
As for data analysis, it is a final proactive part of the research study before
formulating the conclusion. The purpose of the analysis procedure is to find the results to
interpret the implications out of it. Quantitative Data Analysis (QDA) is a process that can be
used to analyse numerical data through statistical and mathematical procedures (Bowen,
2005). This research is based on interpretivist approach, where interpreting the qualitative
information regarding AI and its utilisation in healthcare is essential. Hence in this research,
QDA procedure has been applied to analyse the information collected from interviews, where
24
that states that, it
can cause more
delay of process
time to deliver a
correct healthcare
plan. At the same
time, there are few
articles available on
this topic, where
most of the papers
are based on
secondary study.
Hence, a primary
study can help to
perceive the
situation and
potential
implication at
further level.
A medical service
manager or
administrator has to
deal with multiple
dataset in a
stipulated time
while having the
responsibility of
taking several cost
effective and
beneficial
decisions. This type
of work needs
intense
concentration and
mental strength.
With a larger
number of
consumers, the
work pressure also
increases due to the
expanding database
and internal
cardinality as well
as complexity. It
includes the
patient’s
pathophysiological
details, previous
reports, existing
insurance, and
current available
facilitation. In this
situation, finding
most appropriate
treatment guidance
or managing the
most profitable
insurance becomes
very difficult and
often causes wrong
Apart from the primary data collection, the secondary data collection will be also collected as
supportive evidences and as the source of collecting information regarding activities or
strategies required to be adopted healthcare industry for the implementation of AI in
healthcare management.
Questions for IT experts:
Question 1: How much advancement AI has been made in information technology?
Question 2: How this technology could possibly evolve in next 5 years, in terms of decision
making capability?
Questions for healthcare administrators:
Question 1: What is the purpose and significance of healthcare decision making?
Question 2: What do you think about artificial intelligence in healthcare decision making?
Question 3: What should be the strategy to adopt AI as a gatekeeper in any healthcare
facility?
3.4 Data analysis
As for data analysis, it is a final proactive part of the research study before
formulating the conclusion. The purpose of the analysis procedure is to find the results to
interpret the implications out of it. Quantitative Data Analysis (QDA) is a process that can be
used to analyse numerical data through statistical and mathematical procedures (Bowen,
2005). This research is based on interpretivist approach, where interpreting the qualitative
information regarding AI and its utilisation in healthcare is essential. Hence in this research,
QDA procedure has been applied to analyse the information collected from interviews, where
24
Paraphrase This Document
Need a fresh take? Get an instant paraphrase of this document with our AI Paraphraser
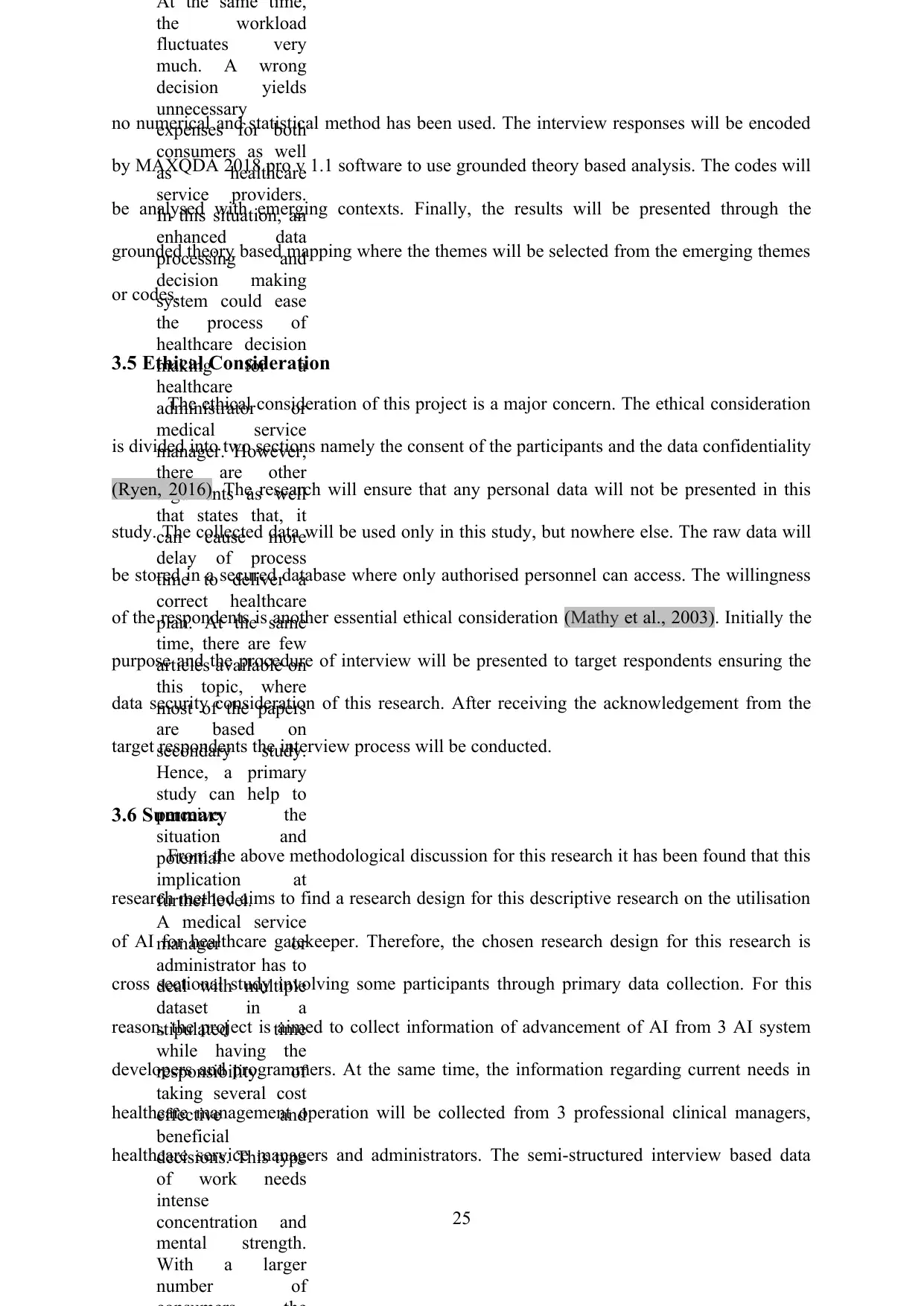
At the same time,
the workload
fluctuates very
much. A wrong
decision yields
unnecessary
expenses for both
consumers as well
as healthcare
service providers.
In this situation, an
enhanced data
processing and
decision making
system could ease
the process of
healthcare decision
making for a
healthcare
administrator or
medical service
manager. However,
there are other
arguments as well
that states that, it
can cause more
delay of process
time to deliver a
correct healthcare
plan. At the same
time, there are few
articles available on
this topic, where
most of the papers
are based on
secondary study.
Hence, a primary
study can help to
perceive the
situation and
potential
implication at
further level.
A medical service
manager or
administrator has to
deal with multiple
dataset in a
stipulated time
while having the
responsibility of
taking several cost
effective and
beneficial
decisions. This type
of work needs
intense
concentration and
mental strength.
With a larger
number of
no numerical and statistical method has been used. The interview responses will be encoded
by MAXQDA 2018 pro v 1.1 software to use grounded theory based analysis. The codes will
be analysed with emerging contexts. Finally, the results will be presented through the
grounded theory based mapping where the themes will be selected from the emerging themes
or codes.
3.5 Ethical Consideration
The ethical consideration of this project is a major concern. The ethical consideration
is divided into two sections namely the consent of the participants and the data confidentiality
(Ryen, 2016). The research will ensure that any personal data will not be presented in this
study. The collected data will be used only in this study, but nowhere else. The raw data will
be stored in a secured database where only authorised personnel can access. The willingness
of the respondents is another essential ethical consideration (Mathy et al., 2003). Initially the
purpose and the procedure of interview will be presented to target respondents ensuring the
data security consideration of this research. After receiving the acknowledgement from the
target respondents the interview process will be conducted.
3.6 Summary
From the above methodological discussion for this research it has been found that this
research method aims to find a research design for this descriptive research on the utilisation
of AI for healthcare gatekeeper. Therefore, the chosen research design for this research is
cross sectional study involving some participants through primary data collection. For this
reason, the project is aimed to collect information of advancement of AI from 3 AI system
developers and programmers. At the same time, the information regarding current needs in
healthcare management operation will be collected from 3 professional clinical managers,
healthcare service managers and administrators. The semi-structured interview based data
25
the workload
fluctuates very
much. A wrong
decision yields
unnecessary
expenses for both
consumers as well
as healthcare
service providers.
In this situation, an
enhanced data
processing and
decision making
system could ease
the process of
healthcare decision
making for a
healthcare
administrator or
medical service
manager. However,
there are other
arguments as well
that states that, it
can cause more
delay of process
time to deliver a
correct healthcare
plan. At the same
time, there are few
articles available on
this topic, where
most of the papers
are based on
secondary study.
Hence, a primary
study can help to
perceive the
situation and
potential
implication at
further level.
A medical service
manager or
administrator has to
deal with multiple
dataset in a
stipulated time
while having the
responsibility of
taking several cost
effective and
beneficial
decisions. This type
of work needs
intense
concentration and
mental strength.
With a larger
number of
no numerical and statistical method has been used. The interview responses will be encoded
by MAXQDA 2018 pro v 1.1 software to use grounded theory based analysis. The codes will
be analysed with emerging contexts. Finally, the results will be presented through the
grounded theory based mapping where the themes will be selected from the emerging themes
or codes.
3.5 Ethical Consideration
The ethical consideration of this project is a major concern. The ethical consideration
is divided into two sections namely the consent of the participants and the data confidentiality
(Ryen, 2016). The research will ensure that any personal data will not be presented in this
study. The collected data will be used only in this study, but nowhere else. The raw data will
be stored in a secured database where only authorised personnel can access. The willingness
of the respondents is another essential ethical consideration (Mathy et al., 2003). Initially the
purpose and the procedure of interview will be presented to target respondents ensuring the
data security consideration of this research. After receiving the acknowledgement from the
target respondents the interview process will be conducted.
3.6 Summary
From the above methodological discussion for this research it has been found that this
research method aims to find a research design for this descriptive research on the utilisation
of AI for healthcare gatekeeper. Therefore, the chosen research design for this research is
cross sectional study involving some participants through primary data collection. For this
reason, the project is aimed to collect information of advancement of AI from 3 AI system
developers and programmers. At the same time, the information regarding current needs in
healthcare management operation will be collected from 3 professional clinical managers,
healthcare service managers and administrators. The semi-structured interview based data
25
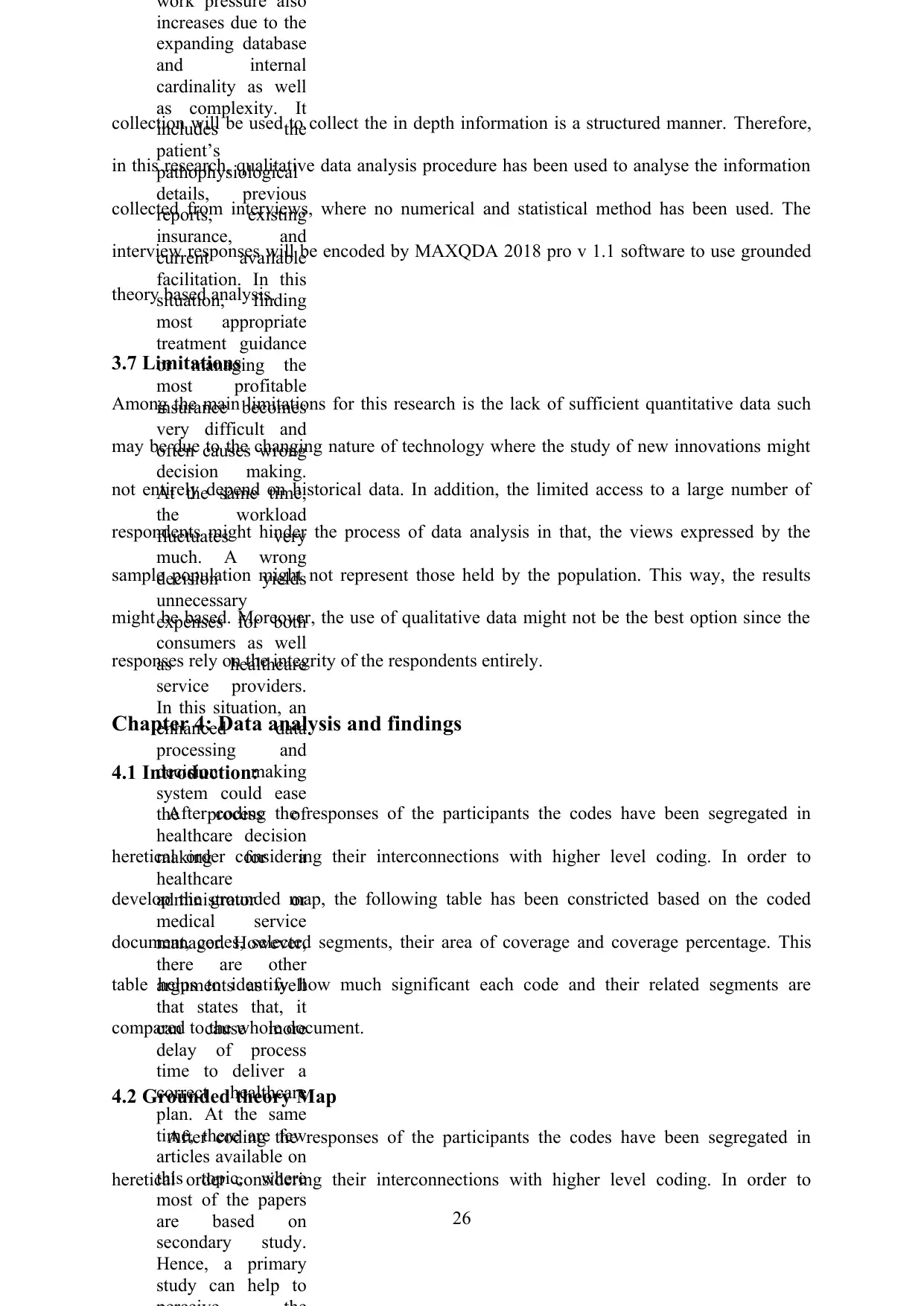
work pressure also
increases due to the
expanding database
and internal
cardinality as well
as complexity. It
includes the
patient’s
pathophysiological
details, previous
reports, existing
insurance, and
current available
facilitation. In this
situation, finding
most appropriate
treatment guidance
or managing the
most profitable
insurance becomes
very difficult and
often causes wrong
decision making.
At the same time,
the workload
fluctuates very
much. A wrong
decision yields
unnecessary
expenses for both
consumers as well
as healthcare
service providers.
In this situation, an
enhanced data
processing and
decision making
system could ease
the process of
healthcare decision
making for a
healthcare
administrator or
medical service
manager. However,
there are other
arguments as well
that states that, it
can cause more
delay of process
time to deliver a
correct healthcare
plan. At the same
time, there are few
articles available on
this topic, where
most of the papers
are based on
secondary study.
Hence, a primary
study can help to
collection will be used to collect the in depth information is a structured manner. Therefore,
in this research, qualitative data analysis procedure has been used to analyse the information
collected from interviews, where no numerical and statistical method has been used. The
interview responses will be encoded by MAXQDA 2018 pro v 1.1 software to use grounded
theory based analysis.
3.7 Limitations
Among the main limitations for this research is the lack of sufficient quantitative data such
may be due to the changing nature of technology where the study of new innovations might
not entirely depend on historical data. In addition, the limited access to a large number of
respondents might hinder the process of data analysis in that, the views expressed by the
sample population might not represent those held by the population. This way, the results
might be based. Moreover, the use of qualitative data might not be the best option since the
responses rely on the integrity of the respondents entirely.
Chapter 4: Data analysis and findings
4.1 Introduction:
After coding the responses of the participants the codes have been segregated in
heretical order considering their interconnections with higher level coding. In order to
develop the grounded map, the following table has been constricted based on the coded
document, codes, selected segments, their area of coverage and coverage percentage. This
table helps to identify how much significant each code and their related segments are
compared to the whole document.
4.2 Grounded theory Map
After coding the responses of the participants the codes have been segregated in
heretical order considering their interconnections with higher level coding. In order to
26
increases due to the
expanding database
and internal
cardinality as well
as complexity. It
includes the
patient’s
pathophysiological
details, previous
reports, existing
insurance, and
current available
facilitation. In this
situation, finding
most appropriate
treatment guidance
or managing the
most profitable
insurance becomes
very difficult and
often causes wrong
decision making.
At the same time,
the workload
fluctuates very
much. A wrong
decision yields
unnecessary
expenses for both
consumers as well
as healthcare
service providers.
In this situation, an
enhanced data
processing and
decision making
system could ease
the process of
healthcare decision
making for a
healthcare
administrator or
medical service
manager. However,
there are other
arguments as well
that states that, it
can cause more
delay of process
time to deliver a
correct healthcare
plan. At the same
time, there are few
articles available on
this topic, where
most of the papers
are based on
secondary study.
Hence, a primary
study can help to
collection will be used to collect the in depth information is a structured manner. Therefore,
in this research, qualitative data analysis procedure has been used to analyse the information
collected from interviews, where no numerical and statistical method has been used. The
interview responses will be encoded by MAXQDA 2018 pro v 1.1 software to use grounded
theory based analysis.
3.7 Limitations
Among the main limitations for this research is the lack of sufficient quantitative data such
may be due to the changing nature of technology where the study of new innovations might
not entirely depend on historical data. In addition, the limited access to a large number of
respondents might hinder the process of data analysis in that, the views expressed by the
sample population might not represent those held by the population. This way, the results
might be based. Moreover, the use of qualitative data might not be the best option since the
responses rely on the integrity of the respondents entirely.
Chapter 4: Data analysis and findings
4.1 Introduction:
After coding the responses of the participants the codes have been segregated in
heretical order considering their interconnections with higher level coding. In order to
develop the grounded map, the following table has been constricted based on the coded
document, codes, selected segments, their area of coverage and coverage percentage. This
table helps to identify how much significant each code and their related segments are
compared to the whole document.
4.2 Grounded theory Map
After coding the responses of the participants the codes have been segregated in
heretical order considering their interconnections with higher level coding. In order to
26
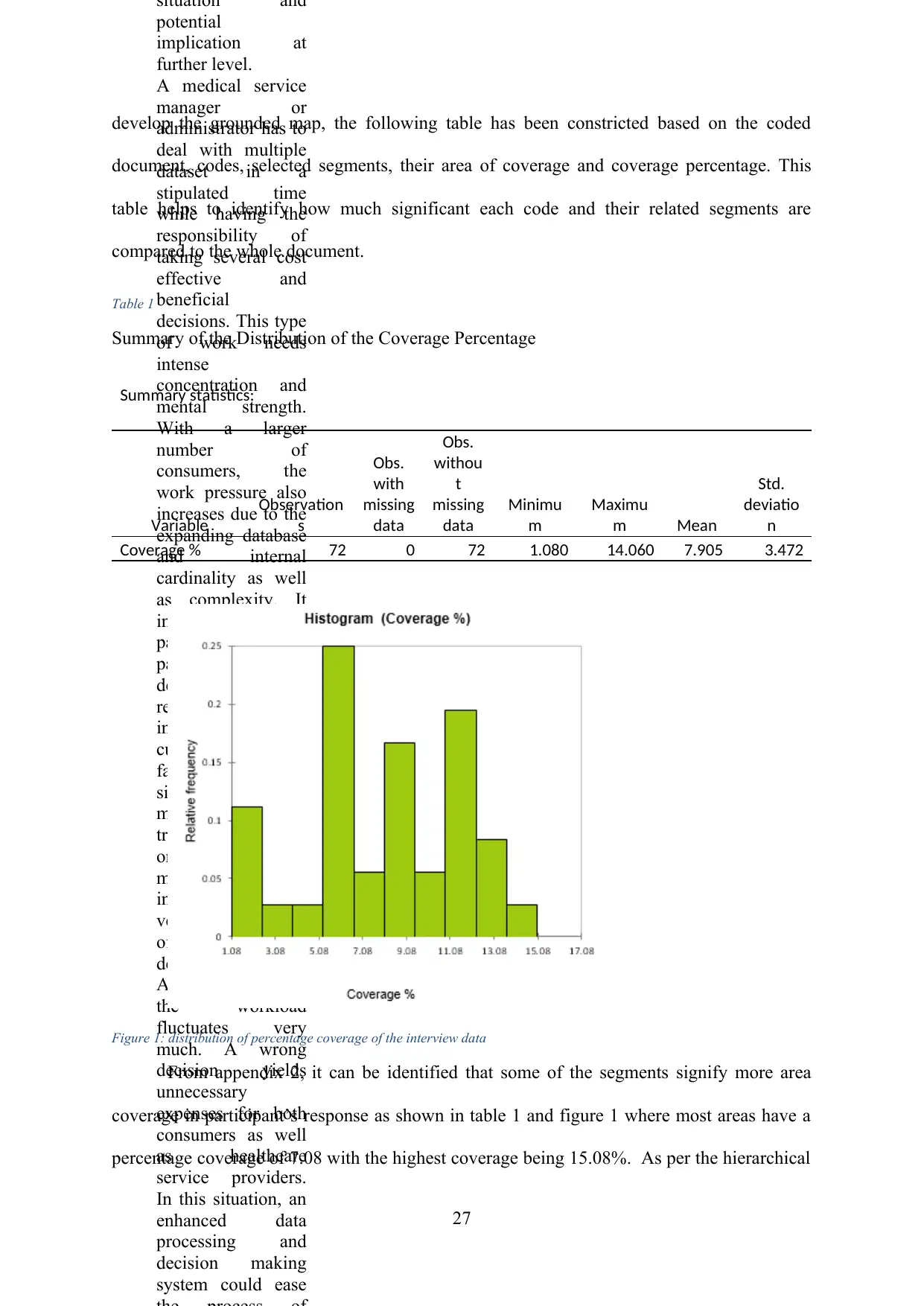
situation and
potential
implication at
further level.
A medical service
manager or
administrator has to
deal with multiple
dataset in a
stipulated time
while having the
responsibility of
taking several cost
effective and
beneficial
decisions. This type
of work needs
intense
concentration and
mental strength.
With a larger
number of
consumers, the
work pressure also
increases due to the
expanding database
and internal
cardinality as well
as complexity. It
includes the
patient’s
pathophysiological
details, previous
reports, existing
insurance, and
current available
facilitation. In this
situation, finding
most appropriate
treatment guidance
or managing the
most profitable
insurance becomes
very difficult and
often causes wrong
decision making.
At the same time,
the workload
fluctuates very
much. A wrong
decision yields
unnecessary
expenses for both
consumers as well
as healthcare
service providers.
In this situation, an
enhanced data
processing and
decision making
system could ease
develop the grounded map, the following table has been constricted based on the coded
document, codes, selected segments, their area of coverage and coverage percentage. This
table helps to identify how much significant each code and their related segments are
compared to the whole document.
Table 1
Summary of the Distribution of the Coverage Percentage
Summary statistics:
Variable
Observation
s
Obs.
with
missing
data
Obs.
withou
t
missing
data
Minimu
m
Maximu
m Mean
Std.
deviatio
n
Coverage % 72 0 72 1.080 14.060 7.905 3.472
Figure 1: distribution of percentage coverage of the interview data
From appendix 2, it can be identified that some of the segments signify more area
coverage in participant’s response as shown in table 1 and figure 1 where most areas have a
percentage coverage of 7.08 with the highest coverage being 15.08%. As per the hierarchical
27
potential
implication at
further level.
A medical service
manager or
administrator has to
deal with multiple
dataset in a
stipulated time
while having the
responsibility of
taking several cost
effective and
beneficial
decisions. This type
of work needs
intense
concentration and
mental strength.
With a larger
number of
consumers, the
work pressure also
increases due to the
expanding database
and internal
cardinality as well
as complexity. It
includes the
patient’s
pathophysiological
details, previous
reports, existing
insurance, and
current available
facilitation. In this
situation, finding
most appropriate
treatment guidance
or managing the
most profitable
insurance becomes
very difficult and
often causes wrong
decision making.
At the same time,
the workload
fluctuates very
much. A wrong
decision yields
unnecessary
expenses for both
consumers as well
as healthcare
service providers.
In this situation, an
enhanced data
processing and
decision making
system could ease
develop the grounded map, the following table has been constricted based on the coded
document, codes, selected segments, their area of coverage and coverage percentage. This
table helps to identify how much significant each code and their related segments are
compared to the whole document.
Table 1
Summary of the Distribution of the Coverage Percentage
Summary statistics:
Variable
Observation
s
Obs.
with
missing
data
Obs.
withou
t
missing
data
Minimu
m
Maximu
m Mean
Std.
deviatio
n
Coverage % 72 0 72 1.080 14.060 7.905 3.472
Figure 1: distribution of percentage coverage of the interview data
From appendix 2, it can be identified that some of the segments signify more area
coverage in participant’s response as shown in table 1 and figure 1 where most areas have a
percentage coverage of 7.08 with the highest coverage being 15.08%. As per the hierarchical
27
Secure Best Marks with AI Grader
Need help grading? Try our AI Grader for instant feedback on your assignments.
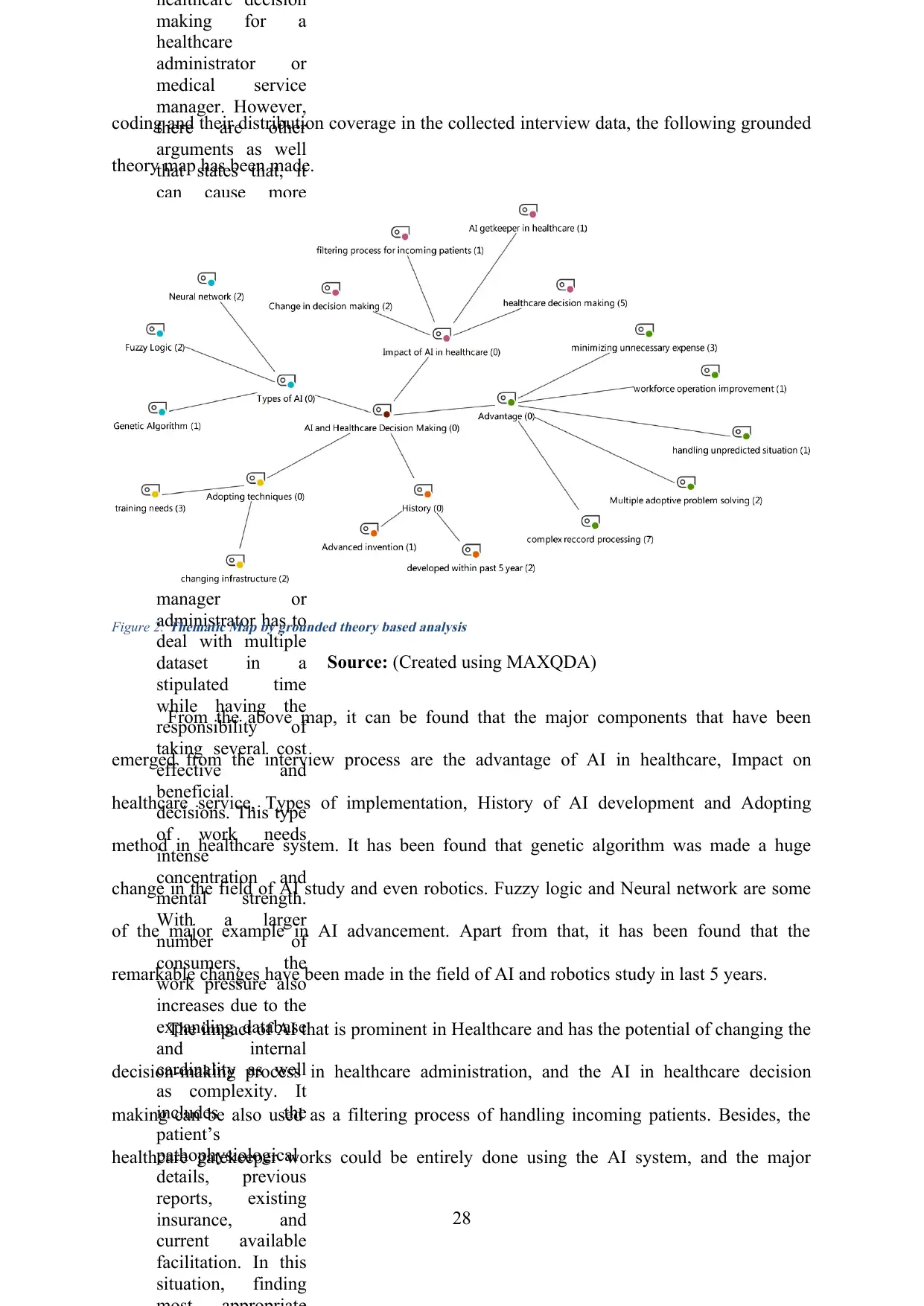
healthcare decision
making for a
healthcare
administrator or
medical service
manager. However,
there are other
arguments as well
that states that, it
can cause more
delay of process
time to deliver a
correct healthcare
plan. At the same
time, there are few
articles available on
this topic, where
most of the papers
are based on
secondary study.
Hence, a primary
study can help to
perceive the
situation and
potential
implication at
further level.
A medical service
manager or
administrator has to
deal with multiple
dataset in a
stipulated time
while having the
responsibility of
taking several cost
effective and
beneficial
decisions. This type
of work needs
intense
concentration and
mental strength.
With a larger
number of
consumers, the
work pressure also
increases due to the
expanding database
and internal
cardinality as well
as complexity. It
includes the
patient’s
pathophysiological
details, previous
reports, existing
insurance, and
current available
facilitation. In this
situation, finding
coding and their distribution coverage in the collected interview data, the following grounded
theory map has been made.
Figure 2:
Thematic Map by grounded theory based analysis
Source: (Created using MAXQDA)
From the above map, it can be found that the major components that have been
emerged from the interview process are the advantage of AI in healthcare, Impact on
healthcare service, Types of implementation, History of AI development and Adopting
method in healthcare system. It has been found that genetic algorithm was made a huge
change in the field of AI study and even robotics. Fuzzy logic and Neural network are some
of the major example in AI advancement. Apart from that, it has been found that the
remarkable changes have been made in the field of AI and robotics study in last 5 years.
The impact of AI that is prominent in Healthcare and has the potential of changing the
decision-making process in healthcare administration, and the AI in healthcare decision
making can be also used as a filtering process of handling incoming patients. Besides, the
healthcare gatekeeper works could be entirely done using the AI system, and the major
28
making for a
healthcare
administrator or
medical service
manager. However,
there are other
arguments as well
that states that, it
can cause more
delay of process
time to deliver a
correct healthcare
plan. At the same
time, there are few
articles available on
this topic, where
most of the papers
are based on
secondary study.
Hence, a primary
study can help to
perceive the
situation and
potential
implication at
further level.
A medical service
manager or
administrator has to
deal with multiple
dataset in a
stipulated time
while having the
responsibility of
taking several cost
effective and
beneficial
decisions. This type
of work needs
intense
concentration and
mental strength.
With a larger
number of
consumers, the
work pressure also
increases due to the
expanding database
and internal
cardinality as well
as complexity. It
includes the
patient’s
pathophysiological
details, previous
reports, existing
insurance, and
current available
facilitation. In this
situation, finding
coding and their distribution coverage in the collected interview data, the following grounded
theory map has been made.
Figure 2:
Thematic Map by grounded theory based analysis
Source: (Created using MAXQDA)
From the above map, it can be found that the major components that have been
emerged from the interview process are the advantage of AI in healthcare, Impact on
healthcare service, Types of implementation, History of AI development and Adopting
method in healthcare system. It has been found that genetic algorithm was made a huge
change in the field of AI study and even robotics. Fuzzy logic and Neural network are some
of the major example in AI advancement. Apart from that, it has been found that the
remarkable changes have been made in the field of AI and robotics study in last 5 years.
The impact of AI that is prominent in Healthcare and has the potential of changing the
decision-making process in healthcare administration, and the AI in healthcare decision
making can be also used as a filtering process of handling incoming patients. Besides, the
healthcare gatekeeper works could be entirely done using the AI system, and the major
28
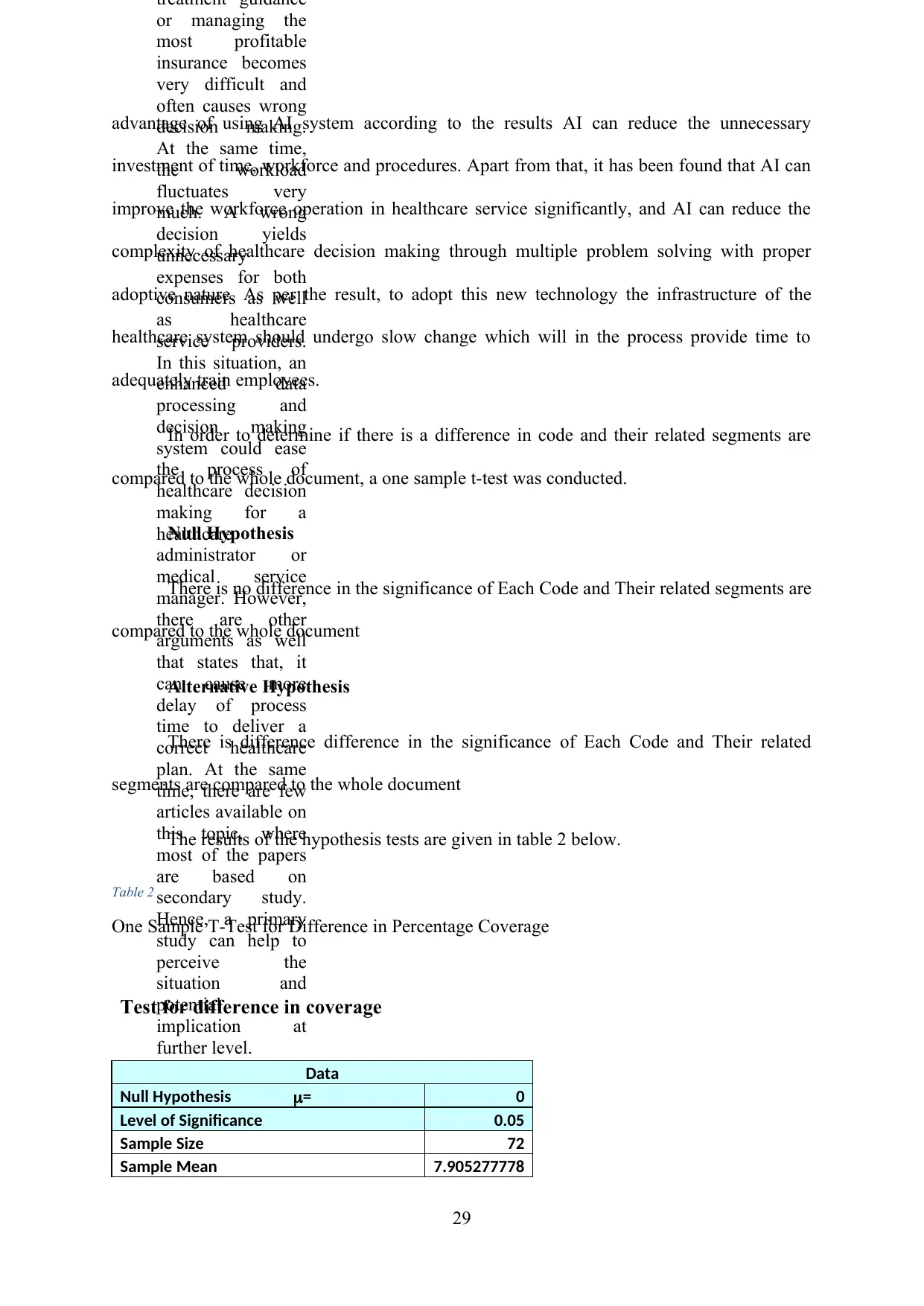
treatment guidance
or managing the
most profitable
insurance becomes
very difficult and
often causes wrong
decision making.
At the same time,
the workload
fluctuates very
much. A wrong
decision yields
unnecessary
expenses for both
consumers as well
as healthcare
service providers.
In this situation, an
enhanced data
processing and
decision making
system could ease
the process of
healthcare decision
making for a
healthcare
administrator or
medical service
manager. However,
there are other
arguments as well
that states that, it
can cause more
delay of process
time to deliver a
correct healthcare
plan. At the same
time, there are few
articles available on
this topic, where
most of the papers
are based on
secondary study.
Hence, a primary
study can help to
perceive the
situation and
potential
implication at
further level.
advantage of using AI system according to the results AI can reduce the unnecessary
investment of time, workforce and procedures. Apart from that, it has been found that AI can
improve the workforce operation in healthcare service significantly, and AI can reduce the
complexity of healthcare decision making through multiple problem solving with proper
adoptive nature. As per the result, to adopt this new technology the infrastructure of the
healthcare system should undergo slow change which will in the process provide time to
adequately train employees.
In order to determine if there is a difference in code and their related segments are
compared to the whole document, a one sample t-test was conducted.
Null Hypothesis
There is no difference in the significance of Each Code and Their related segments are
compared to the whole document
Alternative Hypothesis
There is difference difference in the significance of Each Code and Their related
segments are compared to the whole document
The results of the hypothesis tests are given in table 2 below.
Table 2
One Sample T-Test for Difference in Percentage Coverage
Test for difference in coverage
Data
Null Hypothesis = 0
Level of Significance 0.05
Sample Size 72
Sample Mean 7.905277778
29
or managing the
most profitable
insurance becomes
very difficult and
often causes wrong
decision making.
At the same time,
the workload
fluctuates very
much. A wrong
decision yields
unnecessary
expenses for both
consumers as well
as healthcare
service providers.
In this situation, an
enhanced data
processing and
decision making
system could ease
the process of
healthcare decision
making for a
healthcare
administrator or
medical service
manager. However,
there are other
arguments as well
that states that, it
can cause more
delay of process
time to deliver a
correct healthcare
plan. At the same
time, there are few
articles available on
this topic, where
most of the papers
are based on
secondary study.
Hence, a primary
study can help to
perceive the
situation and
potential
implication at
further level.
advantage of using AI system according to the results AI can reduce the unnecessary
investment of time, workforce and procedures. Apart from that, it has been found that AI can
improve the workforce operation in healthcare service significantly, and AI can reduce the
complexity of healthcare decision making through multiple problem solving with proper
adoptive nature. As per the result, to adopt this new technology the infrastructure of the
healthcare system should undergo slow change which will in the process provide time to
adequately train employees.
In order to determine if there is a difference in code and their related segments are
compared to the whole document, a one sample t-test was conducted.
Null Hypothesis
There is no difference in the significance of Each Code and Their related segments are
compared to the whole document
Alternative Hypothesis
There is difference difference in the significance of Each Code and Their related
segments are compared to the whole document
The results of the hypothesis tests are given in table 2 below.
Table 2
One Sample T-Test for Difference in Percentage Coverage
Test for difference in coverage
Data
Null Hypothesis = 0
Level of Significance 0.05
Sample Size 72
Sample Mean 7.905277778
29
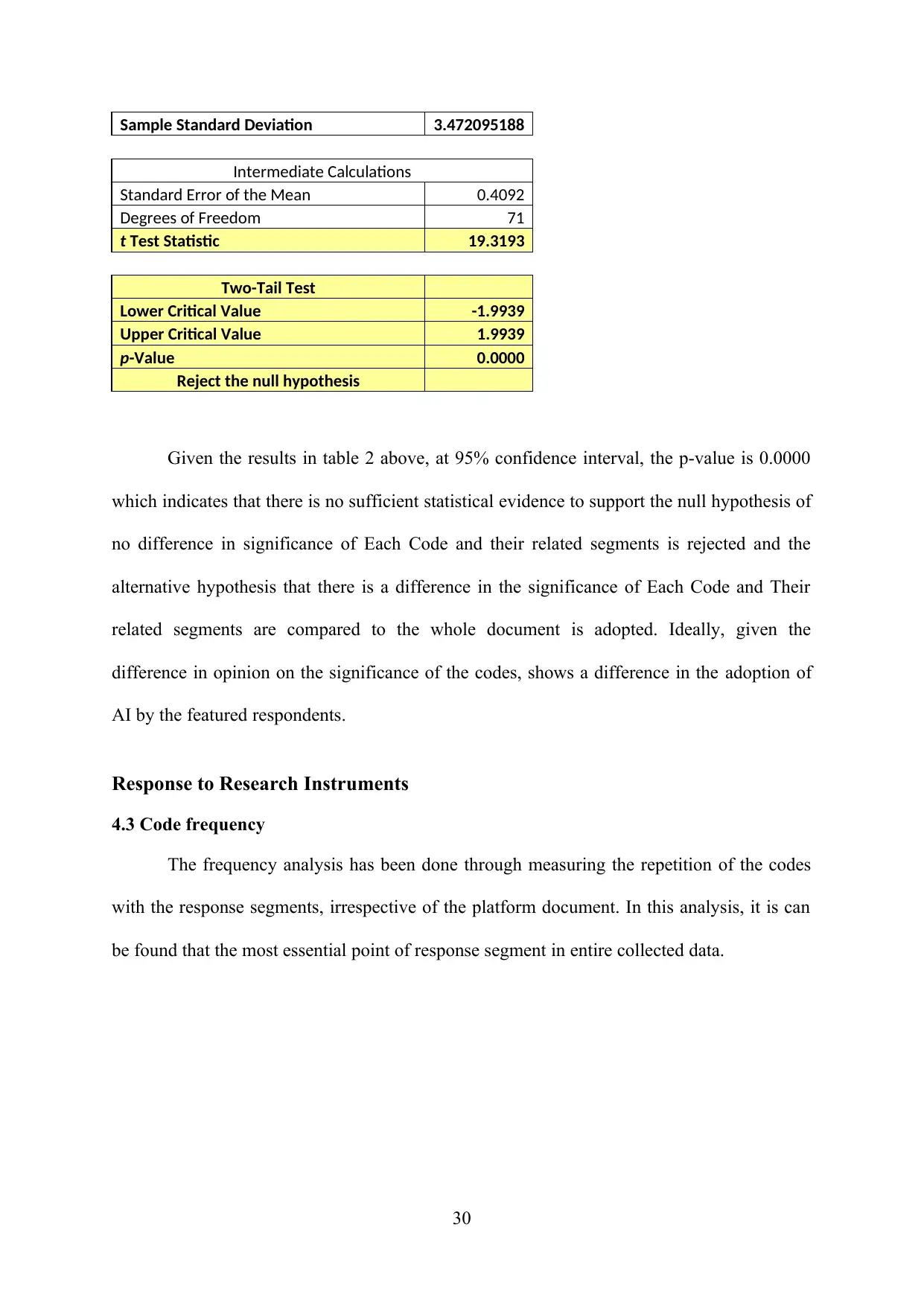
Sample Standard Deviation 3.472095188
Intermediate Calculations
Standard Error of the Mean 0.4092
Degrees of Freedom 71
t Test Statistic 19.3193
Two-Tail Test
Lower Critical Value -1.9939
Upper Critical Value 1.9939
p-Value 0.0000
Reject the null hypothesis
Given the results in table 2 above, at 95% confidence interval, the p-value is 0.0000
which indicates that there is no sufficient statistical evidence to support the null hypothesis of
no difference in significance of Each Code and their related segments is rejected and the
alternative hypothesis that there is a difference in the significance of Each Code and Their
related segments are compared to the whole document is adopted. Ideally, given the
difference in opinion on the significance of the codes, shows a difference in the adoption of
AI by the featured respondents.
Response to Research Instruments
4.3 Code frequency
The frequency analysis has been done through measuring the repetition of the codes
with the response segments, irrespective of the platform document. In this analysis, it is can
be found that the most essential point of response segment in entire collected data.
30
Intermediate Calculations
Standard Error of the Mean 0.4092
Degrees of Freedom 71
t Test Statistic 19.3193
Two-Tail Test
Lower Critical Value -1.9939
Upper Critical Value 1.9939
p-Value 0.0000
Reject the null hypothesis
Given the results in table 2 above, at 95% confidence interval, the p-value is 0.0000
which indicates that there is no sufficient statistical evidence to support the null hypothesis of
no difference in significance of Each Code and their related segments is rejected and the
alternative hypothesis that there is a difference in the significance of Each Code and Their
related segments are compared to the whole document is adopted. Ideally, given the
difference in opinion on the significance of the codes, shows a difference in the adoption of
AI by the featured respondents.
Response to Research Instruments
4.3 Code frequency
The frequency analysis has been done through measuring the repetition of the codes
with the response segments, irrespective of the platform document. In this analysis, it is can
be found that the most essential point of response segment in entire collected data.
30
Paraphrase This Document
Need a fresh take? Get an instant paraphrase of this document with our AI Paraphraser
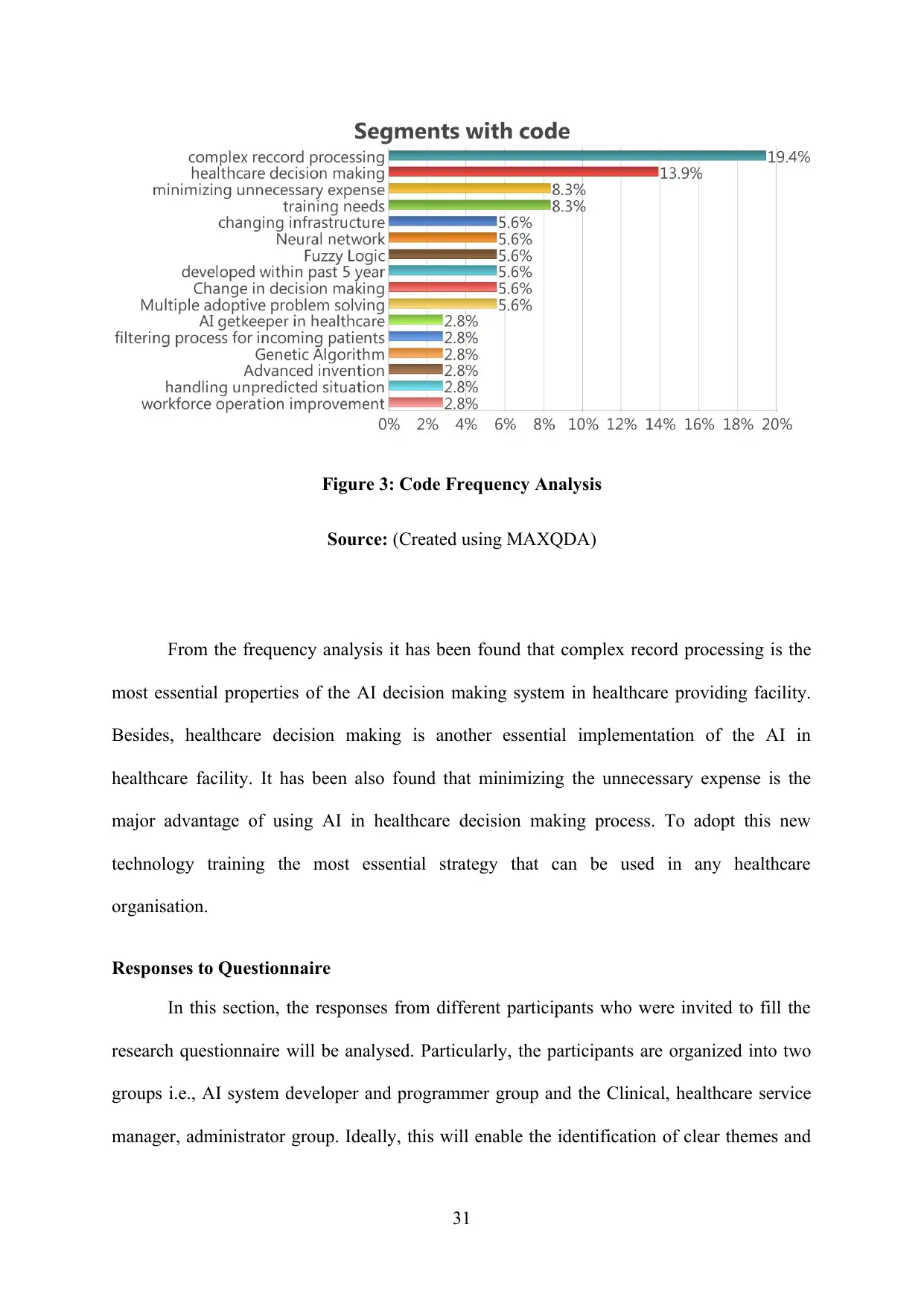
Figure 3: Code Frequency Analysis
Source: (Created using MAXQDA)
From the frequency analysis it has been found that complex record processing is the
most essential properties of the AI decision making system in healthcare providing facility.
Besides, healthcare decision making is another essential implementation of the AI in
healthcare facility. It has been also found that minimizing the unnecessary expense is the
major advantage of using AI in healthcare decision making process. To adopt this new
technology training the most essential strategy that can be used in any healthcare
organisation.
Responses to Questionnaire
In this section, the responses from different participants who were invited to fill the
research questionnaire will be analysed. Particularly, the participants are organized into two
groups i.e., AI system developer and programmer group and the Clinical, healthcare service
manager, administrator group. Ideally, this will enable the identification of clear themes and
31
Source: (Created using MAXQDA)
From the frequency analysis it has been found that complex record processing is the
most essential properties of the AI decision making system in healthcare providing facility.
Besides, healthcare decision making is another essential implementation of the AI in
healthcare facility. It has been also found that minimizing the unnecessary expense is the
major advantage of using AI in healthcare decision making process. To adopt this new
technology training the most essential strategy that can be used in any healthcare
organisation.
Responses to Questionnaire
In this section, the responses from different participants who were invited to fill the
research questionnaire will be analysed. Particularly, the participants are organized into two
groups i.e., AI system developer and programmer group and the Clinical, healthcare service
manager, administrator group. Ideally, this will enable the identification of clear themes and
31
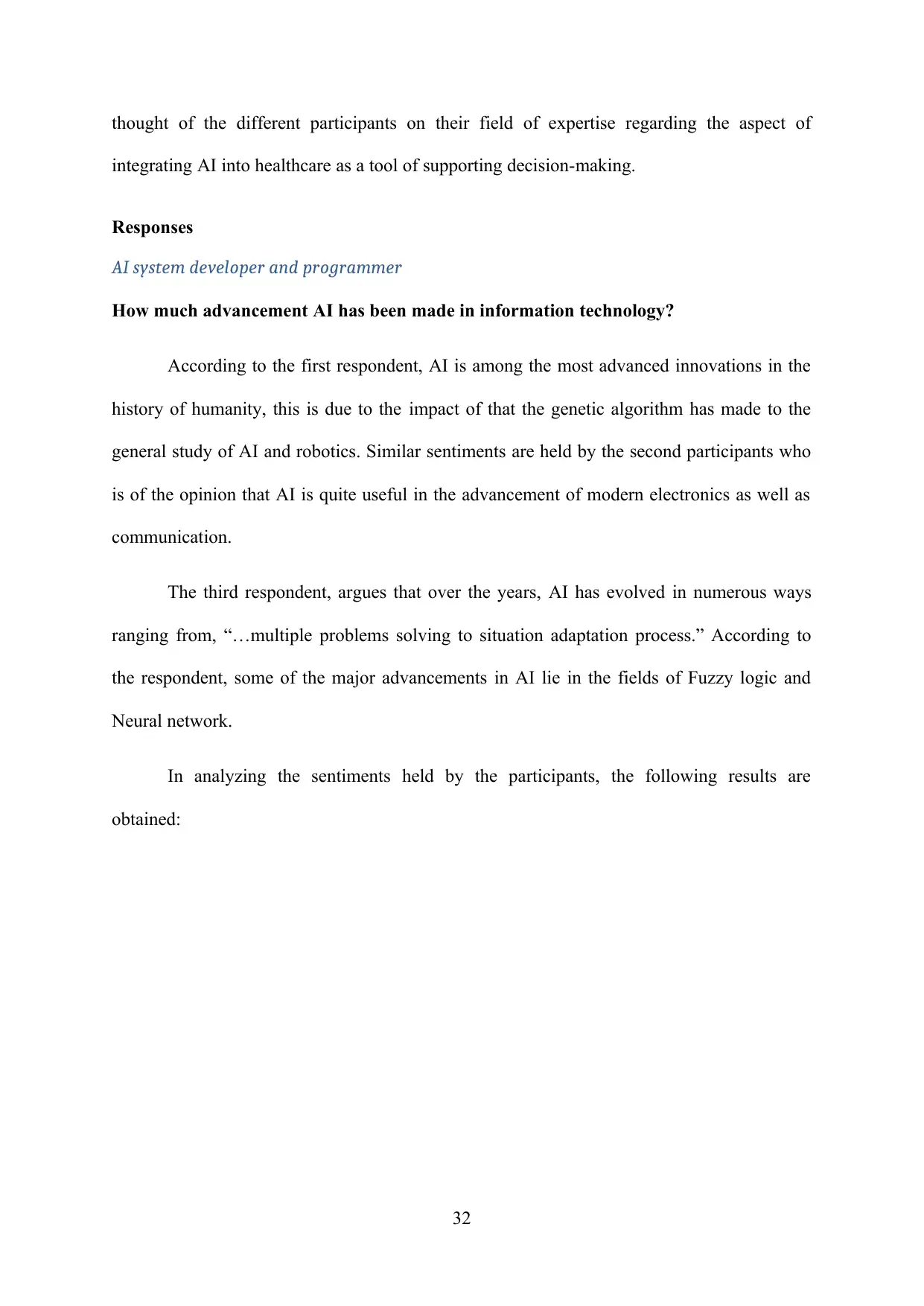
thought of the different participants on their field of expertise regarding the aspect of
integrating AI into healthcare as a tool of supporting decision-making.
Responses
AI system developer and programmer
How much advancement AI has been made in information technology?
According to the first respondent, AI is among the most advanced innovations in the
history of humanity, this is due to the impact of that the genetic algorithm has made to the
general study of AI and robotics. Similar sentiments are held by the second participants who
is of the opinion that AI is quite useful in the advancement of modern electronics as well as
communication.
The third respondent, argues that over the years, AI has evolved in numerous ways
ranging from, “…multiple problems solving to situation adaptation process.” According to
the respondent, some of the major advancements in AI lie in the fields of Fuzzy logic and
Neural network.
In analyzing the sentiments held by the participants, the following results are
obtained:
32
integrating AI into healthcare as a tool of supporting decision-making.
Responses
AI system developer and programmer
How much advancement AI has been made in information technology?
According to the first respondent, AI is among the most advanced innovations in the
history of humanity, this is due to the impact of that the genetic algorithm has made to the
general study of AI and robotics. Similar sentiments are held by the second participants who
is of the opinion that AI is quite useful in the advancement of modern electronics as well as
communication.
The third respondent, argues that over the years, AI has evolved in numerous ways
ranging from, “…multiple problems solving to situation adaptation process.” According to
the respondent, some of the major advancements in AI lie in the fields of Fuzzy logic and
Neural network.
In analyzing the sentiments held by the participants, the following results are
obtained:
32
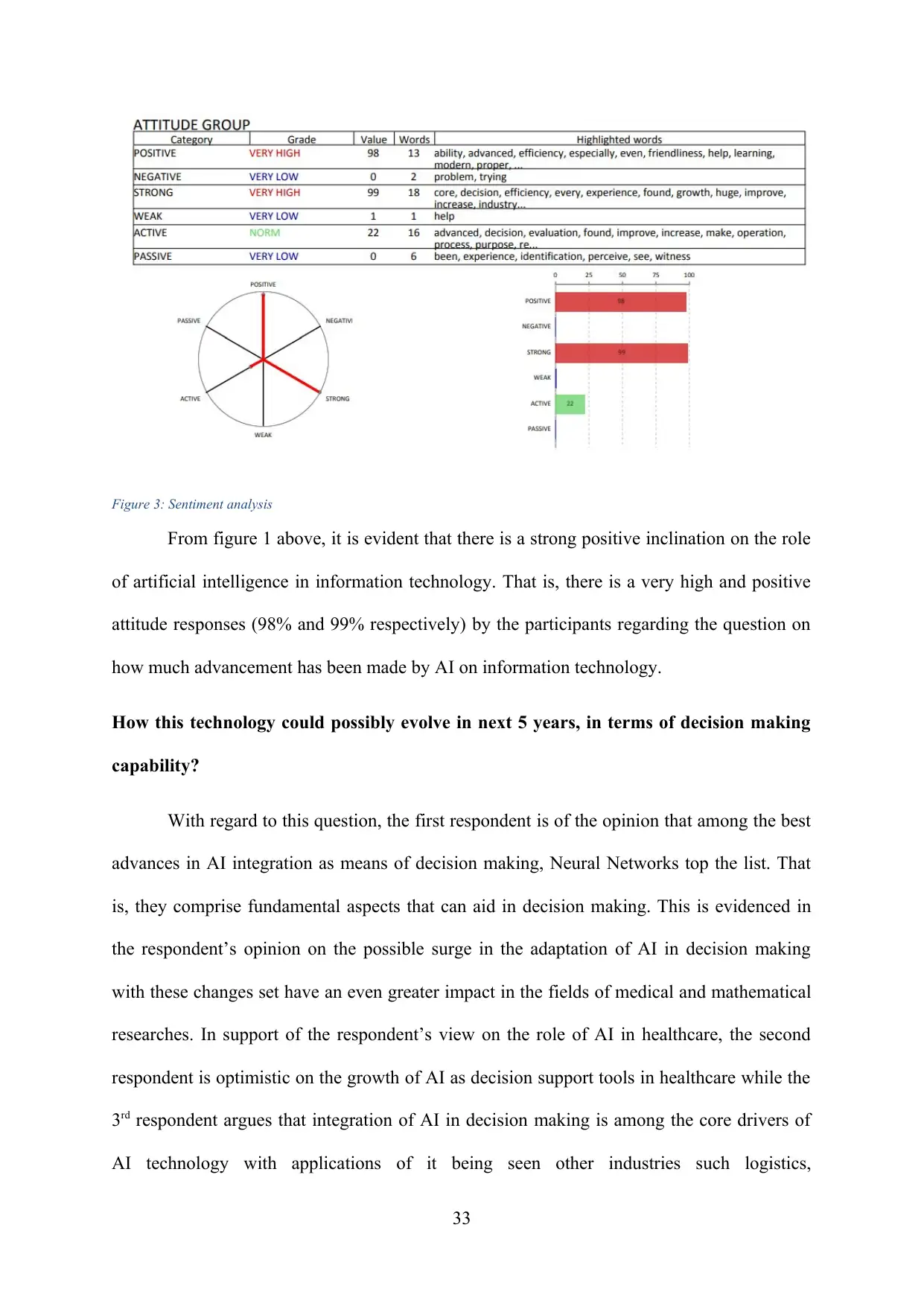
Figure 3: Sentiment analysis
From figure 1 above, it is evident that there is a strong positive inclination on the role
of artificial intelligence in information technology. That is, there is a very high and positive
attitude responses (98% and 99% respectively) by the participants regarding the question on
how much advancement has been made by AI on information technology.
How this technology could possibly evolve in next 5 years, in terms of decision making
capability?
With regard to this question, the first respondent is of the opinion that among the best
advances in AI integration as means of decision making, Neural Networks top the list. That
is, they comprise fundamental aspects that can aid in decision making. This is evidenced in
the respondent’s opinion on the possible surge in the adaptation of AI in decision making
with these changes set have an even greater impact in the fields of medical and mathematical
researches. In support of the respondent’s view on the role of AI in healthcare, the second
respondent is optimistic on the growth of AI as decision support tools in healthcare while the
3rd respondent argues that integration of AI in decision making is among the core drivers of
AI technology with applications of it being seen other industries such logistics,
33
From figure 1 above, it is evident that there is a strong positive inclination on the role
of artificial intelligence in information technology. That is, there is a very high and positive
attitude responses (98% and 99% respectively) by the participants regarding the question on
how much advancement has been made by AI on information technology.
How this technology could possibly evolve in next 5 years, in terms of decision making
capability?
With regard to this question, the first respondent is of the opinion that among the best
advances in AI integration as means of decision making, Neural Networks top the list. That
is, they comprise fundamental aspects that can aid in decision making. This is evidenced in
the respondent’s opinion on the possible surge in the adaptation of AI in decision making
with these changes set have an even greater impact in the fields of medical and mathematical
researches. In support of the respondent’s view on the role of AI in healthcare, the second
respondent is optimistic on the growth of AI as decision support tools in healthcare while the
3rd respondent argues that integration of AI in decision making is among the core drivers of
AI technology with applications of it being seen other industries such logistics,
33
Secure Best Marks with AI Grader
Need help grading? Try our AI Grader for instant feedback on your assignments.
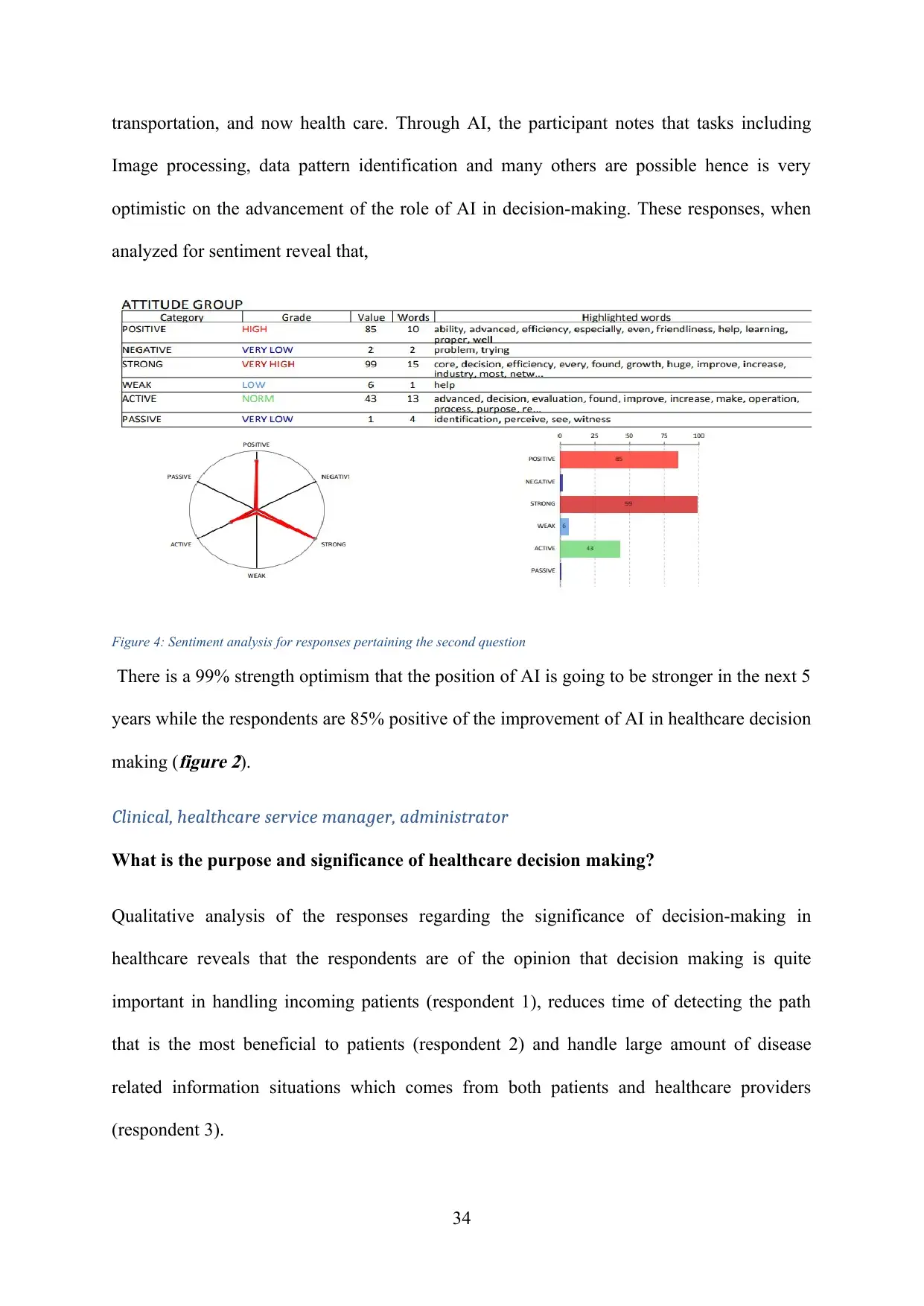
transportation, and now health care. Through AI, the participant notes that tasks including
Image processing, data pattern identification and many others are possible hence is very
optimistic on the advancement of the role of AI in decision-making. These responses, when
analyzed for sentiment reveal that,
Figure 4: Sentiment analysis for responses pertaining the second question
There is a 99% strength optimism that the position of AI is going to be stronger in the next 5
years while the respondents are 85% positive of the improvement of AI in healthcare decision
making (
figure 2).
Clinical, healthcare service manager, administrator
What is the purpose and significance of healthcare decision making?
Qualitative analysis of the responses regarding the significance of decision-making in
healthcare reveals that the respondents are of the opinion that decision making is quite
important in handling incoming patients (respondent 1), reduces time of detecting the path
that is the most beneficial to patients (respondent 2) and handle large amount of disease
related information situations which comes from both patients and healthcare providers
(respondent 3).
34
Image processing, data pattern identification and many others are possible hence is very
optimistic on the advancement of the role of AI in decision-making. These responses, when
analyzed for sentiment reveal that,
Figure 4: Sentiment analysis for responses pertaining the second question
There is a 99% strength optimism that the position of AI is going to be stronger in the next 5
years while the respondents are 85% positive of the improvement of AI in healthcare decision
making (
figure 2).
Clinical, healthcare service manager, administrator
What is the purpose and significance of healthcare decision making?
Qualitative analysis of the responses regarding the significance of decision-making in
healthcare reveals that the respondents are of the opinion that decision making is quite
important in handling incoming patients (respondent 1), reduces time of detecting the path
that is the most beneficial to patients (respondent 2) and handle large amount of disease
related information situations which comes from both patients and healthcare providers
(respondent 3).
34
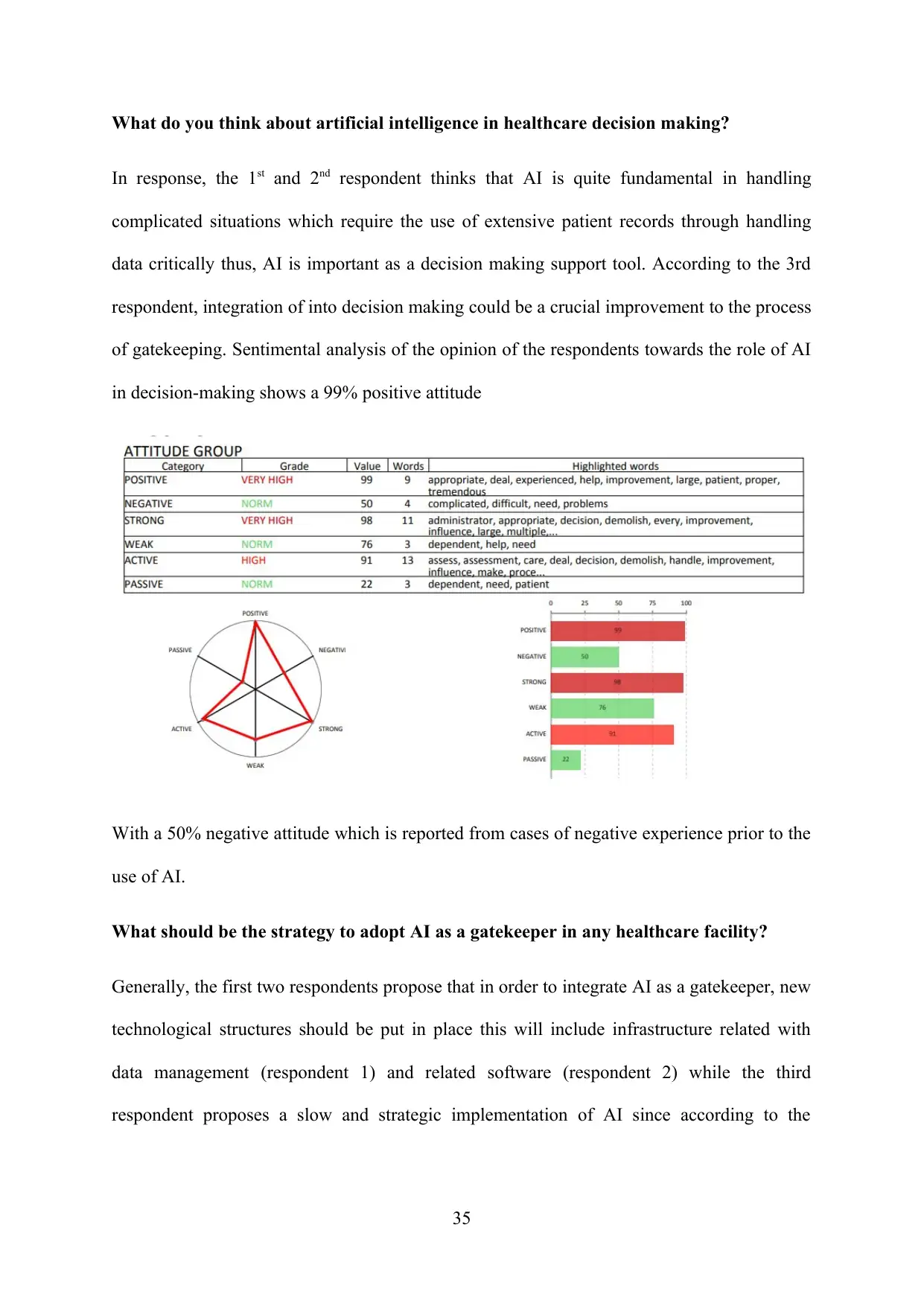
What do you think about artificial intelligence in healthcare decision making?
In response, the 1st and 2nd respondent thinks that AI is quite fundamental in handling
complicated situations which require the use of extensive patient records through handling
data critically thus, AI is important as a decision making support tool. According to the 3rd
respondent, integration of into decision making could be a crucial improvement to the process
of gatekeeping. Sentimental analysis of the opinion of the respondents towards the role of AI
in decision-making shows a 99% positive attitude
With a 50% negative attitude which is reported from cases of negative experience prior to the
use of AI.
What should be the strategy to adopt AI as a gatekeeper in any healthcare facility?
Generally, the first two respondents propose that in order to integrate AI as a gatekeeper, new
technological structures should be put in place this will include infrastructure related with
data management (respondent 1) and related software (respondent 2) while the third
respondent proposes a slow and strategic implementation of AI since according to the
35
In response, the 1st and 2nd respondent thinks that AI is quite fundamental in handling
complicated situations which require the use of extensive patient records through handling
data critically thus, AI is important as a decision making support tool. According to the 3rd
respondent, integration of into decision making could be a crucial improvement to the process
of gatekeeping. Sentimental analysis of the opinion of the respondents towards the role of AI
in decision-making shows a 99% positive attitude
With a 50% negative attitude which is reported from cases of negative experience prior to the
use of AI.
What should be the strategy to adopt AI as a gatekeeper in any healthcare facility?
Generally, the first two respondents propose that in order to integrate AI as a gatekeeper, new
technological structures should be put in place this will include infrastructure related with
data management (respondent 1) and related software (respondent 2) while the third
respondent proposes a slow and strategic implementation of AI since according to the
35
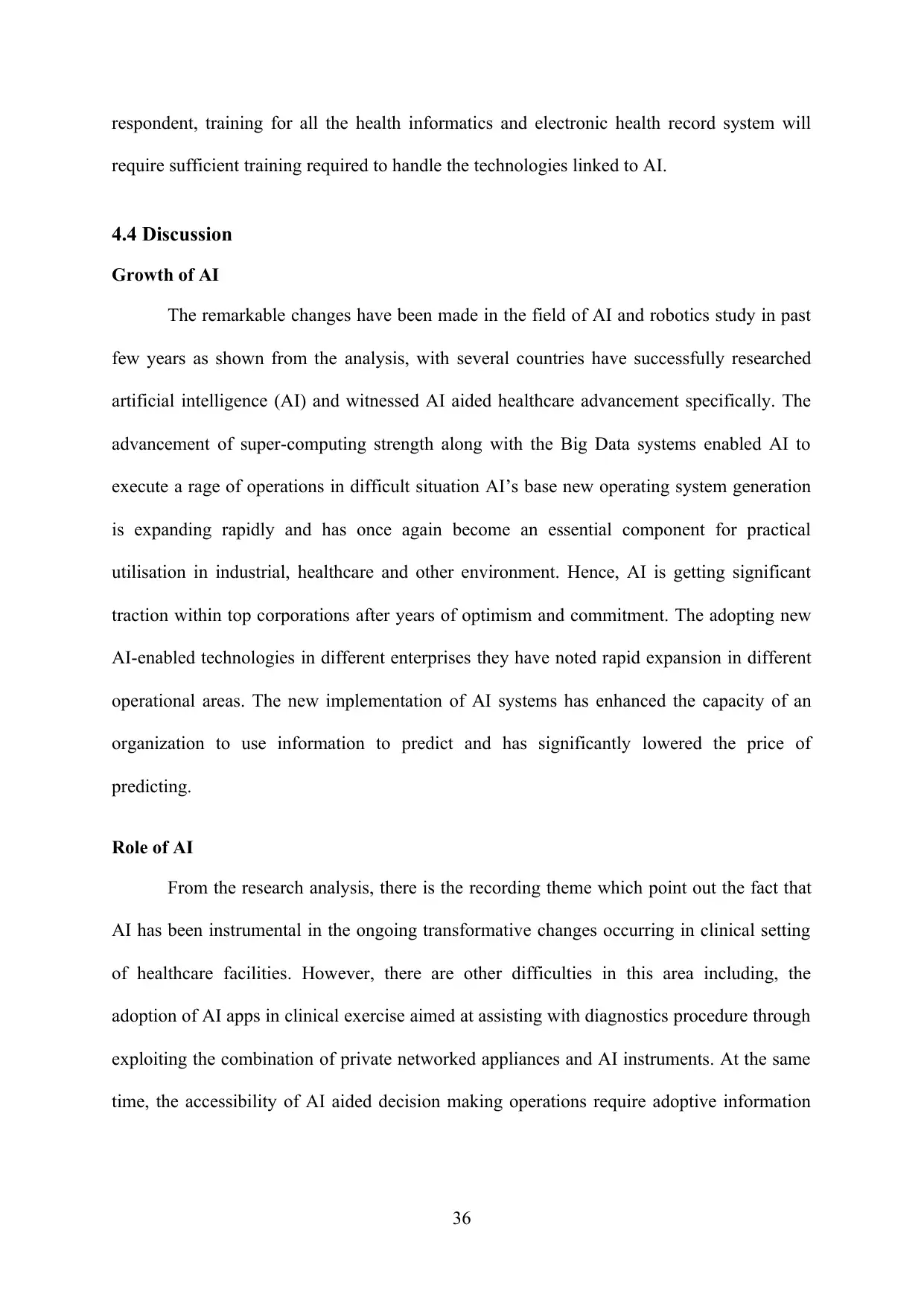
respondent, training for all the health informatics and electronic health record system will
require sufficient training required to handle the technologies linked to AI.
4.4 Discussion
Growth of AI
The remarkable changes have been made in the field of AI and robotics study in past
few years as shown from the analysis, with several countries have successfully researched
artificial intelligence (AI) and witnessed AI aided healthcare advancement specifically. The
advancement of super-computing strength along with the Big Data systems enabled AI to
execute a rage of operations in difficult situation AI’s base new operating system generation
is expanding rapidly and has once again become an essential component for practical
utilisation in industrial, healthcare and other environment. Hence, AI is getting significant
traction within top corporations after years of optimism and commitment. The adopting new
AI-enabled technologies in different enterprises they have noted rapid expansion in different
operational areas. The new implementation of AI systems has enhanced the capacity of an
organization to use information to predict and has significantly lowered the price of
predicting.
Role of AI
From the research analysis, there is the recording theme which point out the fact that
AI has been instrumental in the ongoing transformative changes occurring in clinical setting
of healthcare facilities. However, there are other difficulties in this area including, the
adoption of AI apps in clinical exercise aimed at assisting with diagnostics procedure through
exploiting the combination of private networked appliances and AI instruments. At the same
time, the accessibility of AI aided decision making operations require adoptive information
36
require sufficient training required to handle the technologies linked to AI.
4.4 Discussion
Growth of AI
The remarkable changes have been made in the field of AI and robotics study in past
few years as shown from the analysis, with several countries have successfully researched
artificial intelligence (AI) and witnessed AI aided healthcare advancement specifically. The
advancement of super-computing strength along with the Big Data systems enabled AI to
execute a rage of operations in difficult situation AI’s base new operating system generation
is expanding rapidly and has once again become an essential component for practical
utilisation in industrial, healthcare and other environment. Hence, AI is getting significant
traction within top corporations after years of optimism and commitment. The adopting new
AI-enabled technologies in different enterprises they have noted rapid expansion in different
operational areas. The new implementation of AI systems has enhanced the capacity of an
organization to use information to predict and has significantly lowered the price of
predicting.
Role of AI
From the research analysis, there is the recording theme which point out the fact that
AI has been instrumental in the ongoing transformative changes occurring in clinical setting
of healthcare facilities. However, there are other difficulties in this area including, the
adoption of AI apps in clinical exercise aimed at assisting with diagnostics procedure through
exploiting the combination of private networked appliances and AI instruments. At the same
time, the accessibility of AI aided decision making operations require adoptive information
36
Paraphrase This Document
Need a fresh take? Get an instant paraphrase of this document with our AI Paraphraser
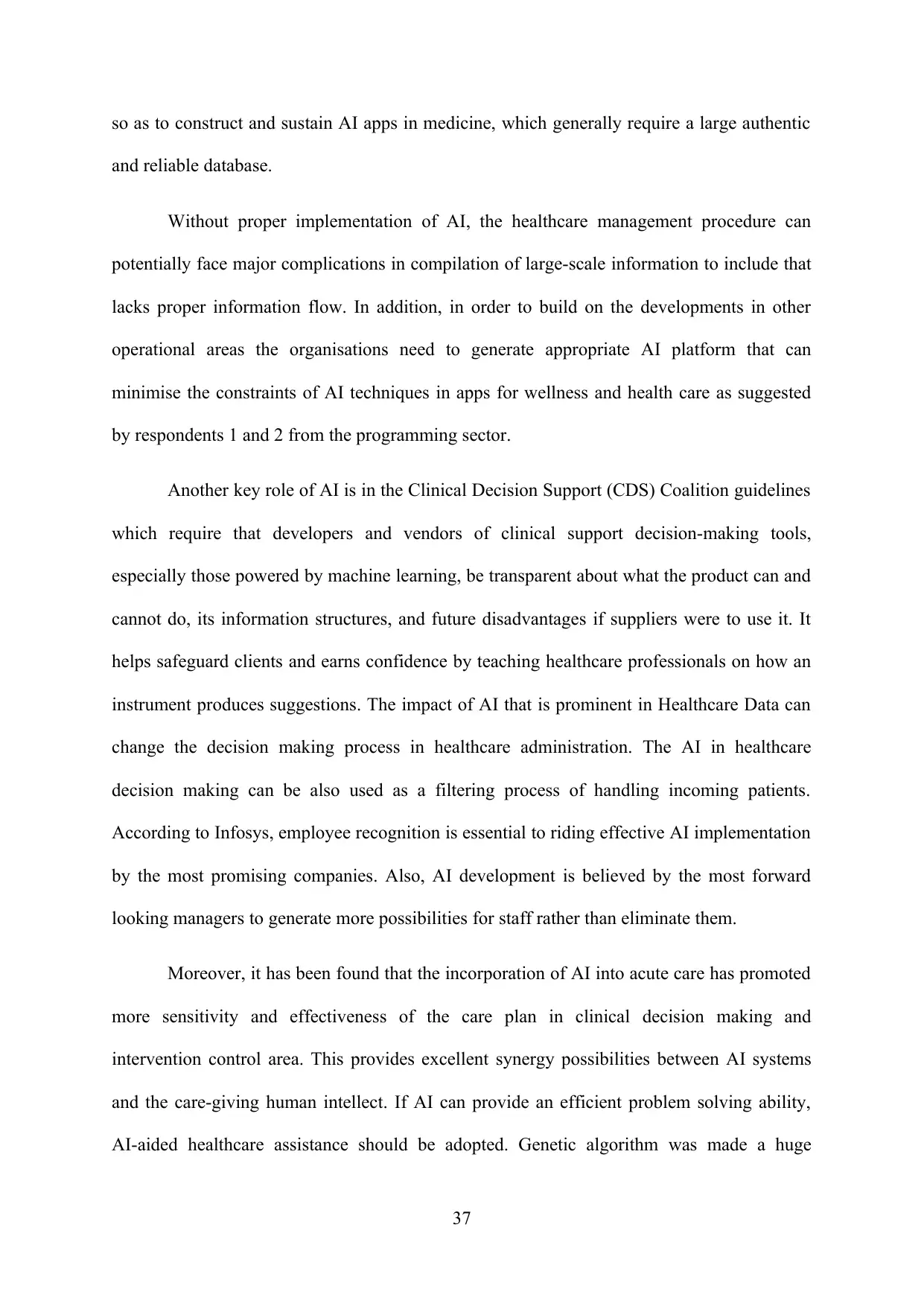
so as to construct and sustain AI apps in medicine, which generally require a large authentic
and reliable database.
Without proper implementation of AI, the healthcare management procedure can
potentially face major complications in compilation of large-scale information to include that
lacks proper information flow. In addition, in order to build on the developments in other
operational areas the organisations need to generate appropriate AI platform that can
minimise the constraints of AI techniques in apps for wellness and health care as suggested
by respondents 1 and 2 from the programming sector.
Another key role of AI is in the Clinical Decision Support (CDS) Coalition guidelines
which require that developers and vendors of clinical support decision-making tools,
especially those powered by machine learning, be transparent about what the product can and
cannot do, its information structures, and future disadvantages if suppliers were to use it. It
helps safeguard clients and earns confidence by teaching healthcare professionals on how an
instrument produces suggestions. The impact of AI that is prominent in Healthcare Data can
change the decision making process in healthcare administration. The AI in healthcare
decision making can be also used as a filtering process of handling incoming patients.
According to Infosys, employee recognition is essential to riding effective AI implementation
by the most promising companies. Also, AI development is believed by the most forward
looking managers to generate more possibilities for staff rather than eliminate them.
Moreover, it has been found that the incorporation of AI into acute care has promoted
more sensitivity and effectiveness of the care plan in clinical decision making and
intervention control area. This provides excellent synergy possibilities between AI systems
and the care-giving human intellect. If AI can provide an efficient problem solving ability,
AI-aided healthcare assistance should be adopted. Genetic algorithm was made a huge
37
and reliable database.
Without proper implementation of AI, the healthcare management procedure can
potentially face major complications in compilation of large-scale information to include that
lacks proper information flow. In addition, in order to build on the developments in other
operational areas the organisations need to generate appropriate AI platform that can
minimise the constraints of AI techniques in apps for wellness and health care as suggested
by respondents 1 and 2 from the programming sector.
Another key role of AI is in the Clinical Decision Support (CDS) Coalition guidelines
which require that developers and vendors of clinical support decision-making tools,
especially those powered by machine learning, be transparent about what the product can and
cannot do, its information structures, and future disadvantages if suppliers were to use it. It
helps safeguard clients and earns confidence by teaching healthcare professionals on how an
instrument produces suggestions. The impact of AI that is prominent in Healthcare Data can
change the decision making process in healthcare administration. The AI in healthcare
decision making can be also used as a filtering process of handling incoming patients.
According to Infosys, employee recognition is essential to riding effective AI implementation
by the most promising companies. Also, AI development is believed by the most forward
looking managers to generate more possibilities for staff rather than eliminate them.
Moreover, it has been found that the incorporation of AI into acute care has promoted
more sensitivity and effectiveness of the care plan in clinical decision making and
intervention control area. This provides excellent synergy possibilities between AI systems
and the care-giving human intellect. If AI can provide an efficient problem solving ability,
AI-aided healthcare assistance should be adopted. Genetic algorithm was made a huge
37
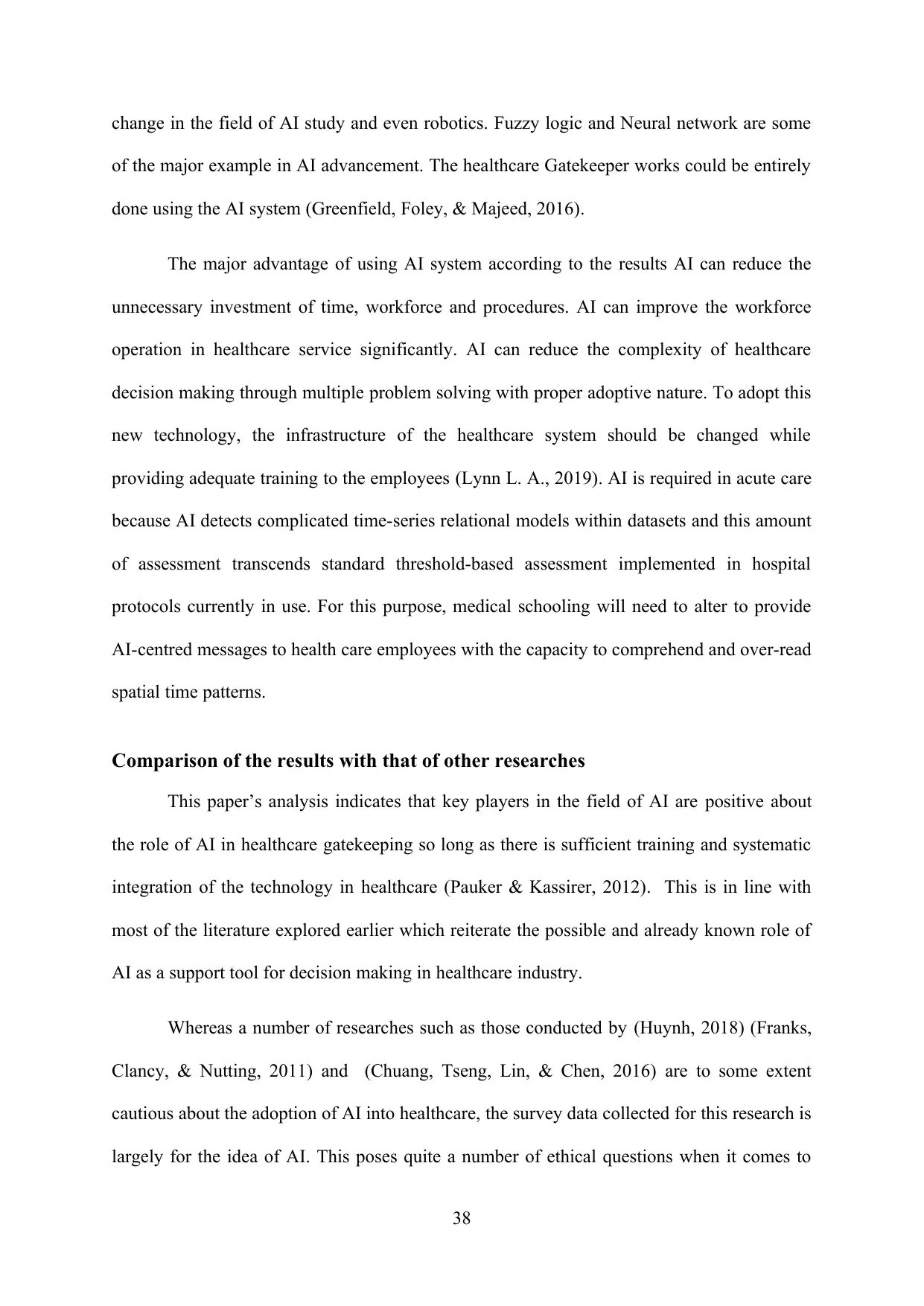
change in the field of AI study and even robotics. Fuzzy logic and Neural network are some
of the major example in AI advancement. The healthcare Gatekeeper works could be entirely
done using the AI system (Greenfield, Foley, & Majeed, 2016).
The major advantage of using AI system according to the results AI can reduce the
unnecessary investment of time, workforce and procedures. AI can improve the workforce
operation in healthcare service significantly. AI can reduce the complexity of healthcare
decision making through multiple problem solving with proper adoptive nature. To adopt this
new technology, the infrastructure of the healthcare system should be changed while
providing adequate training to the employees (Lynn L. A., 2019). AI is required in acute care
because AI detects complicated time-series relational models within datasets and this amount
of assessment transcends standard threshold-based assessment implemented in hospital
protocols currently in use. For this purpose, medical schooling will need to alter to provide
AI-centred messages to health care employees with the capacity to comprehend and over-read
spatial time patterns.
Comparison of the results with that of other researches
This paper’s analysis indicates that key players in the field of AI are positive about
the role of AI in healthcare gatekeeping so long as there is sufficient training and systematic
integration of the technology in healthcare (Pauker & Kassirer, 2012). This is in line with
most of the literature explored earlier which reiterate the possible and already known role of
AI as a support tool for decision making in healthcare industry.
Whereas a number of researches such as those conducted by (Huynh, 2018) (Franks,
Clancy, & Nutting, 2011) and (Chuang, Tseng, Lin, & Chen, 2016) are to some extent
cautious about the adoption of AI into healthcare, the survey data collected for this research is
largely for the idea of AI. This poses quite a number of ethical questions when it comes to
38
of the major example in AI advancement. The healthcare Gatekeeper works could be entirely
done using the AI system (Greenfield, Foley, & Majeed, 2016).
The major advantage of using AI system according to the results AI can reduce the
unnecessary investment of time, workforce and procedures. AI can improve the workforce
operation in healthcare service significantly. AI can reduce the complexity of healthcare
decision making through multiple problem solving with proper adoptive nature. To adopt this
new technology, the infrastructure of the healthcare system should be changed while
providing adequate training to the employees (Lynn L. A., 2019). AI is required in acute care
because AI detects complicated time-series relational models within datasets and this amount
of assessment transcends standard threshold-based assessment implemented in hospital
protocols currently in use. For this purpose, medical schooling will need to alter to provide
AI-centred messages to health care employees with the capacity to comprehend and over-read
spatial time patterns.
Comparison of the results with that of other researches
This paper’s analysis indicates that key players in the field of AI are positive about
the role of AI in healthcare gatekeeping so long as there is sufficient training and systematic
integration of the technology in healthcare (Pauker & Kassirer, 2012). This is in line with
most of the literature explored earlier which reiterate the possible and already known role of
AI as a support tool for decision making in healthcare industry.
Whereas a number of researches such as those conducted by (Huynh, 2018) (Franks,
Clancy, & Nutting, 2011) and (Chuang, Tseng, Lin, & Chen, 2016) are to some extent
cautious about the adoption of AI into healthcare, the survey data collected for this research is
largely for the idea of AI. This poses quite a number of ethical questions when it comes to
38
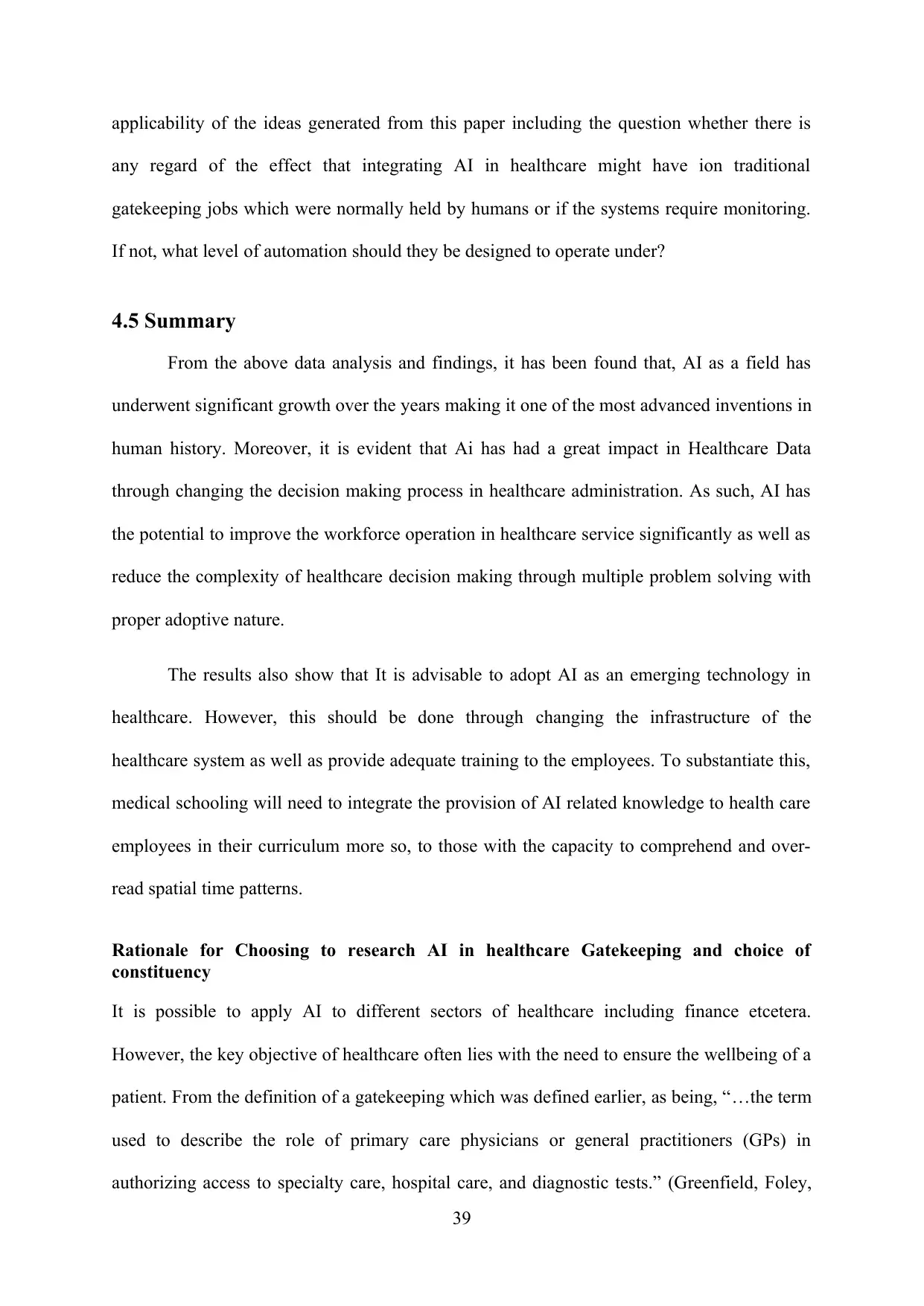
applicability of the ideas generated from this paper including the question whether there is
any regard of the effect that integrating AI in healthcare might have ion traditional
gatekeeping jobs which were normally held by humans or if the systems require monitoring.
If not, what level of automation should they be designed to operate under?
4.5 Summary
From the above data analysis and findings, it has been found that, AI as a field has
underwent significant growth over the years making it one of the most advanced inventions in
human history. Moreover, it is evident that Ai has had a great impact in Healthcare Data
through changing the decision making process in healthcare administration. As such, AI has
the potential to improve the workforce operation in healthcare service significantly as well as
reduce the complexity of healthcare decision making through multiple problem solving with
proper adoptive nature.
The results also show that It is advisable to adopt AI as an emerging technology in
healthcare. However, this should be done through changing the infrastructure of the
healthcare system as well as provide adequate training to the employees. To substantiate this,
medical schooling will need to integrate the provision of AI related knowledge to health care
employees in their curriculum more so, to those with the capacity to comprehend and over-
read spatial time patterns.
Rationale for Choosing to research AI in healthcare Gatekeeping and choice of
constituency
It is possible to apply AI to different sectors of healthcare including finance etcetera.
However, the key objective of healthcare often lies with the need to ensure the wellbeing of a
patient. From the definition of a gatekeeping which was defined earlier, as being, “…the term
used to describe the role of primary care physicians or general practitioners (GPs) in
authorizing access to specialty care, hospital care, and diagnostic tests.” (Greenfield, Foley,
39
any regard of the effect that integrating AI in healthcare might have ion traditional
gatekeeping jobs which were normally held by humans or if the systems require monitoring.
If not, what level of automation should they be designed to operate under?
4.5 Summary
From the above data analysis and findings, it has been found that, AI as a field has
underwent significant growth over the years making it one of the most advanced inventions in
human history. Moreover, it is evident that Ai has had a great impact in Healthcare Data
through changing the decision making process in healthcare administration. As such, AI has
the potential to improve the workforce operation in healthcare service significantly as well as
reduce the complexity of healthcare decision making through multiple problem solving with
proper adoptive nature.
The results also show that It is advisable to adopt AI as an emerging technology in
healthcare. However, this should be done through changing the infrastructure of the
healthcare system as well as provide adequate training to the employees. To substantiate this,
medical schooling will need to integrate the provision of AI related knowledge to health care
employees in their curriculum more so, to those with the capacity to comprehend and over-
read spatial time patterns.
Rationale for Choosing to research AI in healthcare Gatekeeping and choice of
constituency
It is possible to apply AI to different sectors of healthcare including finance etcetera.
However, the key objective of healthcare often lies with the need to ensure the wellbeing of a
patient. From the definition of a gatekeeping which was defined earlier, as being, “…the term
used to describe the role of primary care physicians or general practitioners (GPs) in
authorizing access to specialty care, hospital care, and diagnostic tests.” (Greenfield, Foley,
39
Secure Best Marks with AI Grader
Need help grading? Try our AI Grader for instant feedback on your assignments.
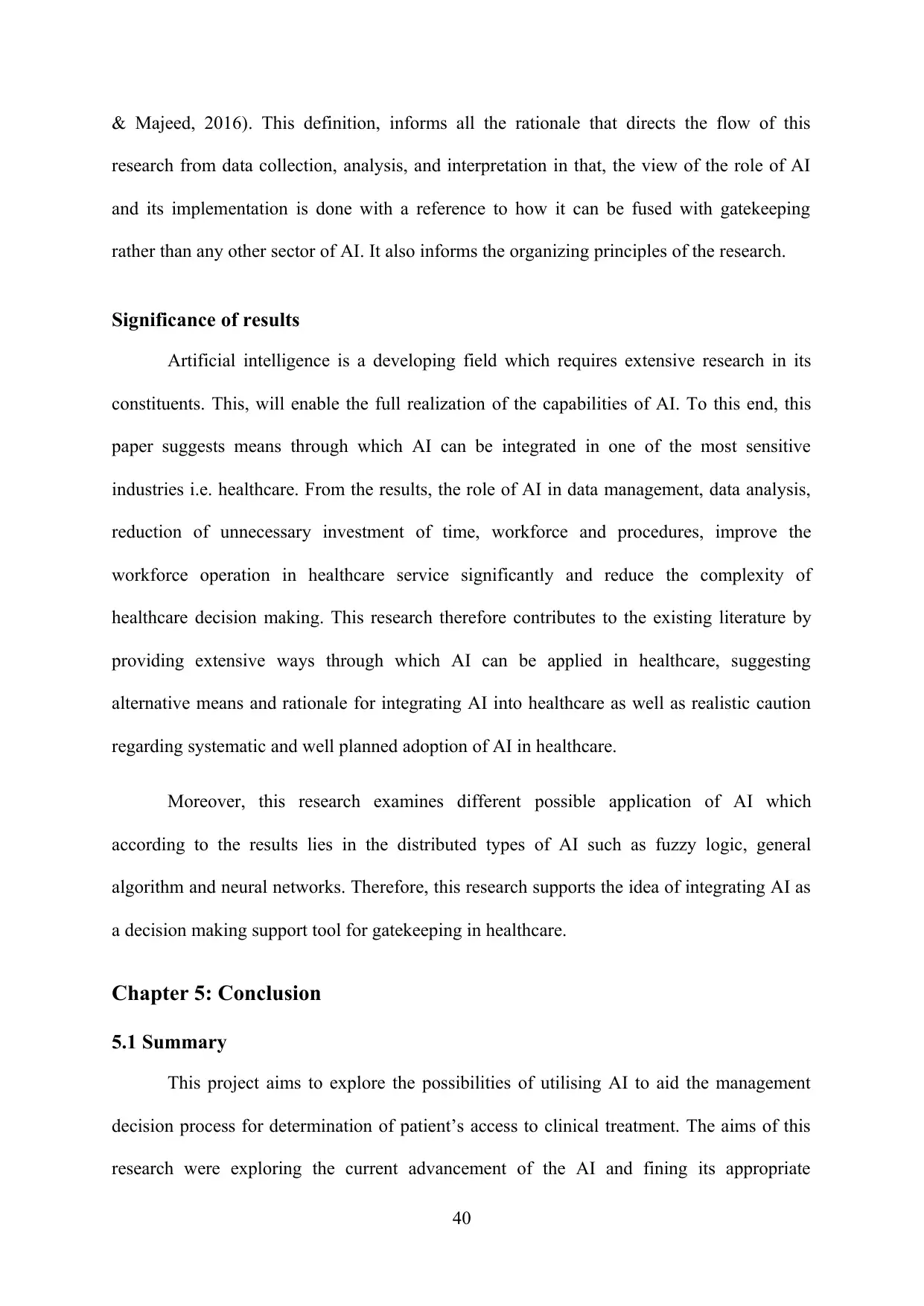
& Majeed, 2016). This definition, informs all the rationale that directs the flow of this
research from data collection, analysis, and interpretation in that, the view of the role of AI
and its implementation is done with a reference to how it can be fused with gatekeeping
rather than any other sector of AI. It also informs the organizing principles of the research.
Significance of results
Artificial intelligence is a developing field which requires extensive research in its
constituents. This, will enable the full realization of the capabilities of AI. To this end, this
paper suggests means through which AI can be integrated in one of the most sensitive
industries i.e. healthcare. From the results, the role of AI in data management, data analysis,
reduction of unnecessary investment of time, workforce and procedures, improve the
workforce operation in healthcare service significantly and reduce the complexity of
healthcare decision making. This research therefore contributes to the existing literature by
providing extensive ways through which AI can be applied in healthcare, suggesting
alternative means and rationale for integrating AI into healthcare as well as realistic caution
regarding systematic and well planned adoption of AI in healthcare.
Moreover, this research examines different possible application of AI which
according to the results lies in the distributed types of AI such as fuzzy logic, general
algorithm and neural networks. Therefore, this research supports the idea of integrating AI as
a decision making support tool for gatekeeping in healthcare.
Chapter 5: Conclusion
5.1 Summary
This project aims to explore the possibilities of utilising AI to aid the management
decision process for determination of patient’s access to clinical treatment. The aims of this
research were exploring the current advancement of the AI and fining its appropriate
40
research from data collection, analysis, and interpretation in that, the view of the role of AI
and its implementation is done with a reference to how it can be fused with gatekeeping
rather than any other sector of AI. It also informs the organizing principles of the research.
Significance of results
Artificial intelligence is a developing field which requires extensive research in its
constituents. This, will enable the full realization of the capabilities of AI. To this end, this
paper suggests means through which AI can be integrated in one of the most sensitive
industries i.e. healthcare. From the results, the role of AI in data management, data analysis,
reduction of unnecessary investment of time, workforce and procedures, improve the
workforce operation in healthcare service significantly and reduce the complexity of
healthcare decision making. This research therefore contributes to the existing literature by
providing extensive ways through which AI can be applied in healthcare, suggesting
alternative means and rationale for integrating AI into healthcare as well as realistic caution
regarding systematic and well planned adoption of AI in healthcare.
Moreover, this research examines different possible application of AI which
according to the results lies in the distributed types of AI such as fuzzy logic, general
algorithm and neural networks. Therefore, this research supports the idea of integrating AI as
a decision making support tool for gatekeeping in healthcare.
Chapter 5: Conclusion
5.1 Summary
This project aims to explore the possibilities of utilising AI to aid the management
decision process for determination of patient’s access to clinical treatment. The aims of this
research were exploring the current advancement of the AI and fining its appropriate
40
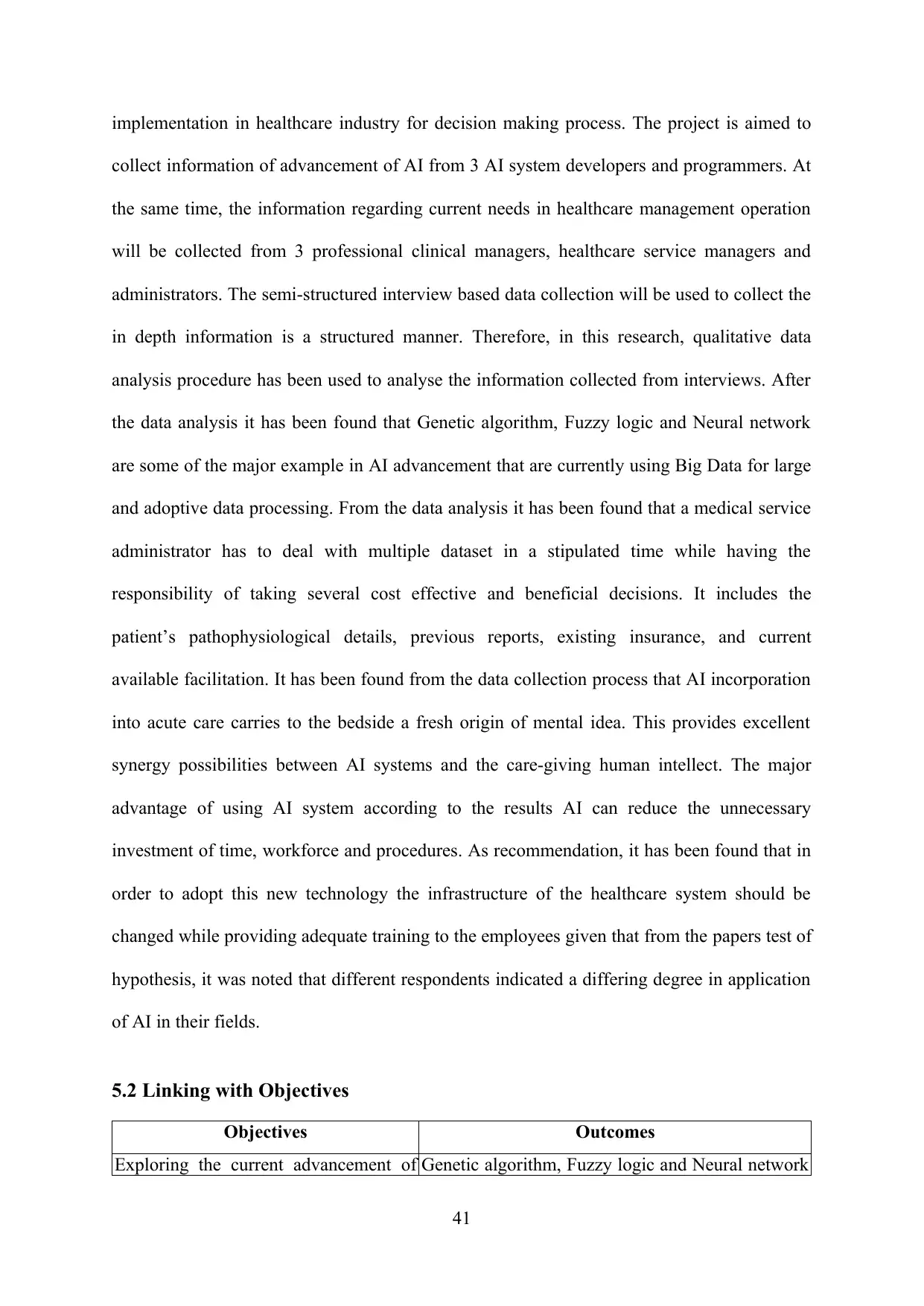
implementation in healthcare industry for decision making process. The project is aimed to
collect information of advancement of AI from 3 AI system developers and programmers. At
the same time, the information regarding current needs in healthcare management operation
will be collected from 3 professional clinical managers, healthcare service managers and
administrators. The semi-structured interview based data collection will be used to collect the
in depth information is a structured manner. Therefore, in this research, qualitative data
analysis procedure has been used to analyse the information collected from interviews. After
the data analysis it has been found that Genetic algorithm, Fuzzy logic and Neural network
are some of the major example in AI advancement that are currently using Big Data for large
and adoptive data processing. From the data analysis it has been found that a medical service
administrator has to deal with multiple dataset in a stipulated time while having the
responsibility of taking several cost effective and beneficial decisions. It includes the
patient’s pathophysiological details, previous reports, existing insurance, and current
available facilitation. It has been found from the data collection process that AI incorporation
into acute care carries to the bedside a fresh origin of mental idea. This provides excellent
synergy possibilities between AI systems and the care-giving human intellect. The major
advantage of using AI system according to the results AI can reduce the unnecessary
investment of time, workforce and procedures. As recommendation, it has been found that in
order to adopt this new technology the infrastructure of the healthcare system should be
changed while providing adequate training to the employees given that from the papers test of
hypothesis, it was noted that different respondents indicated a differing degree in application
of AI in their fields.
5.2 Linking with Objectives
Objectives Outcomes
Exploring the current advancement of Genetic algorithm, Fuzzy logic and Neural network
41
collect information of advancement of AI from 3 AI system developers and programmers. At
the same time, the information regarding current needs in healthcare management operation
will be collected from 3 professional clinical managers, healthcare service managers and
administrators. The semi-structured interview based data collection will be used to collect the
in depth information is a structured manner. Therefore, in this research, qualitative data
analysis procedure has been used to analyse the information collected from interviews. After
the data analysis it has been found that Genetic algorithm, Fuzzy logic and Neural network
are some of the major example in AI advancement that are currently using Big Data for large
and adoptive data processing. From the data analysis it has been found that a medical service
administrator has to deal with multiple dataset in a stipulated time while having the
responsibility of taking several cost effective and beneficial decisions. It includes the
patient’s pathophysiological details, previous reports, existing insurance, and current
available facilitation. It has been found from the data collection process that AI incorporation
into acute care carries to the bedside a fresh origin of mental idea. This provides excellent
synergy possibilities between AI systems and the care-giving human intellect. The major
advantage of using AI system according to the results AI can reduce the unnecessary
investment of time, workforce and procedures. As recommendation, it has been found that in
order to adopt this new technology the infrastructure of the healthcare system should be
changed while providing adequate training to the employees given that from the papers test of
hypothesis, it was noted that different respondents indicated a differing degree in application
of AI in their fields.
5.2 Linking with Objectives
Objectives Outcomes
Exploring the current advancement of Genetic algorithm, Fuzzy logic and Neural network
41
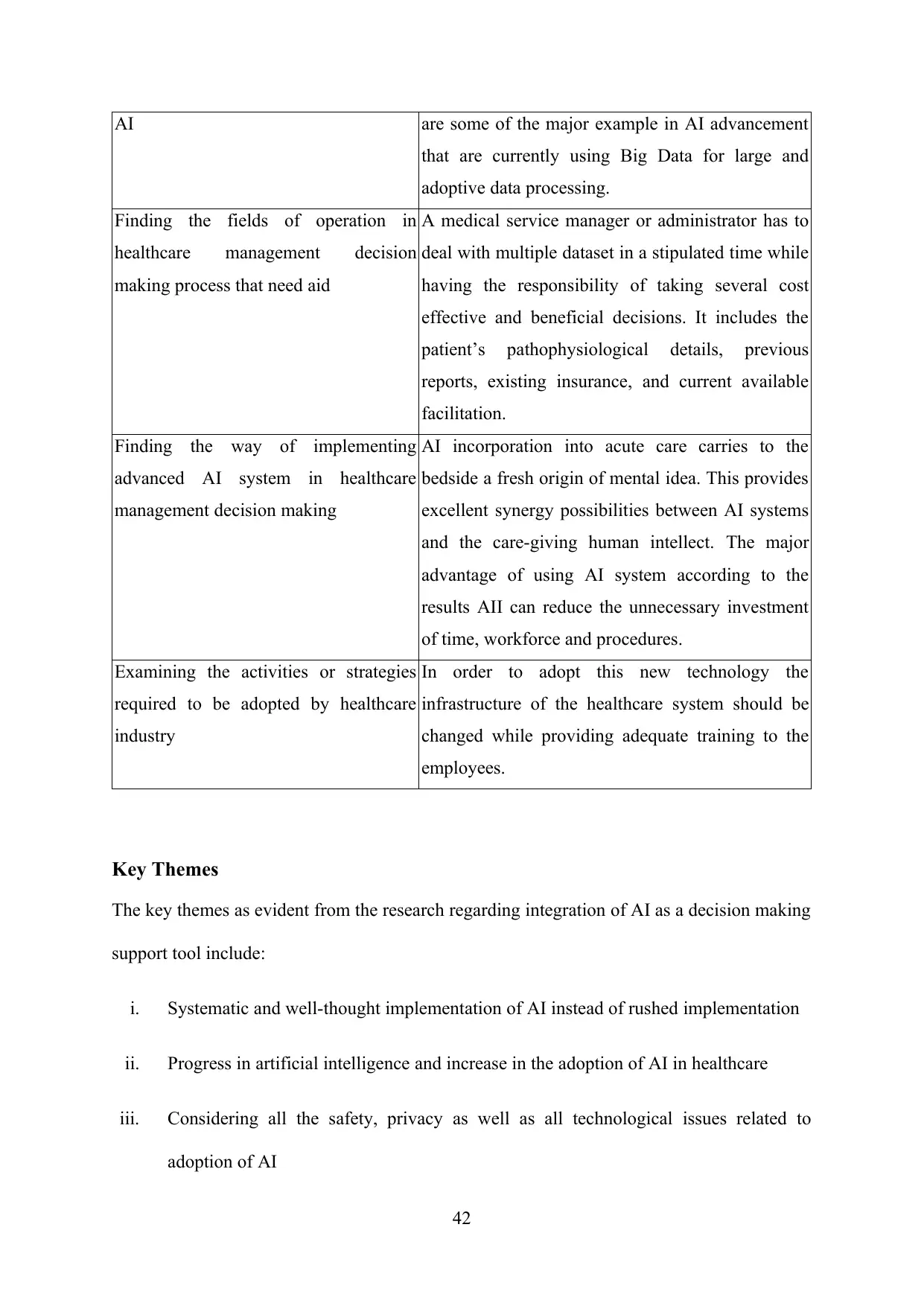
AI are some of the major example in AI advancement
that are currently using Big Data for large and
adoptive data processing.
Finding the fields of operation in
healthcare management decision
making process that need aid
A medical service manager or administrator has to
deal with multiple dataset in a stipulated time while
having the responsibility of taking several cost
effective and beneficial decisions. It includes the
patient’s pathophysiological details, previous
reports, existing insurance, and current available
facilitation.
Finding the way of implementing
advanced AI system in healthcare
management decision making
AI incorporation into acute care carries to the
bedside a fresh origin of mental idea. This provides
excellent synergy possibilities between AI systems
and the care-giving human intellect. The major
advantage of using AI system according to the
results AII can reduce the unnecessary investment
of time, workforce and procedures.
Examining the activities or strategies
required to be adopted by healthcare
industry
In order to adopt this new technology the
infrastructure of the healthcare system should be
changed while providing adequate training to the
employees.
Key Themes
The key themes as evident from the research regarding integration of AI as a decision making
support tool include:
i. Systematic and well-thought implementation of AI instead of rushed implementation
ii. Progress in artificial intelligence and increase in the adoption of AI in healthcare
iii. Considering all the safety, privacy as well as all technological issues related to
adoption of AI
42
that are currently using Big Data for large and
adoptive data processing.
Finding the fields of operation in
healthcare management decision
making process that need aid
A medical service manager or administrator has to
deal with multiple dataset in a stipulated time while
having the responsibility of taking several cost
effective and beneficial decisions. It includes the
patient’s pathophysiological details, previous
reports, existing insurance, and current available
facilitation.
Finding the way of implementing
advanced AI system in healthcare
management decision making
AI incorporation into acute care carries to the
bedside a fresh origin of mental idea. This provides
excellent synergy possibilities between AI systems
and the care-giving human intellect. The major
advantage of using AI system according to the
results AII can reduce the unnecessary investment
of time, workforce and procedures.
Examining the activities or strategies
required to be adopted by healthcare
industry
In order to adopt this new technology the
infrastructure of the healthcare system should be
changed while providing adequate training to the
employees.
Key Themes
The key themes as evident from the research regarding integration of AI as a decision making
support tool include:
i. Systematic and well-thought implementation of AI instead of rushed implementation
ii. Progress in artificial intelligence and increase in the adoption of AI in healthcare
iii. Considering all the safety, privacy as well as all technological issues related to
adoption of AI
42
Paraphrase This Document
Need a fresh take? Get an instant paraphrase of this document with our AI Paraphraser
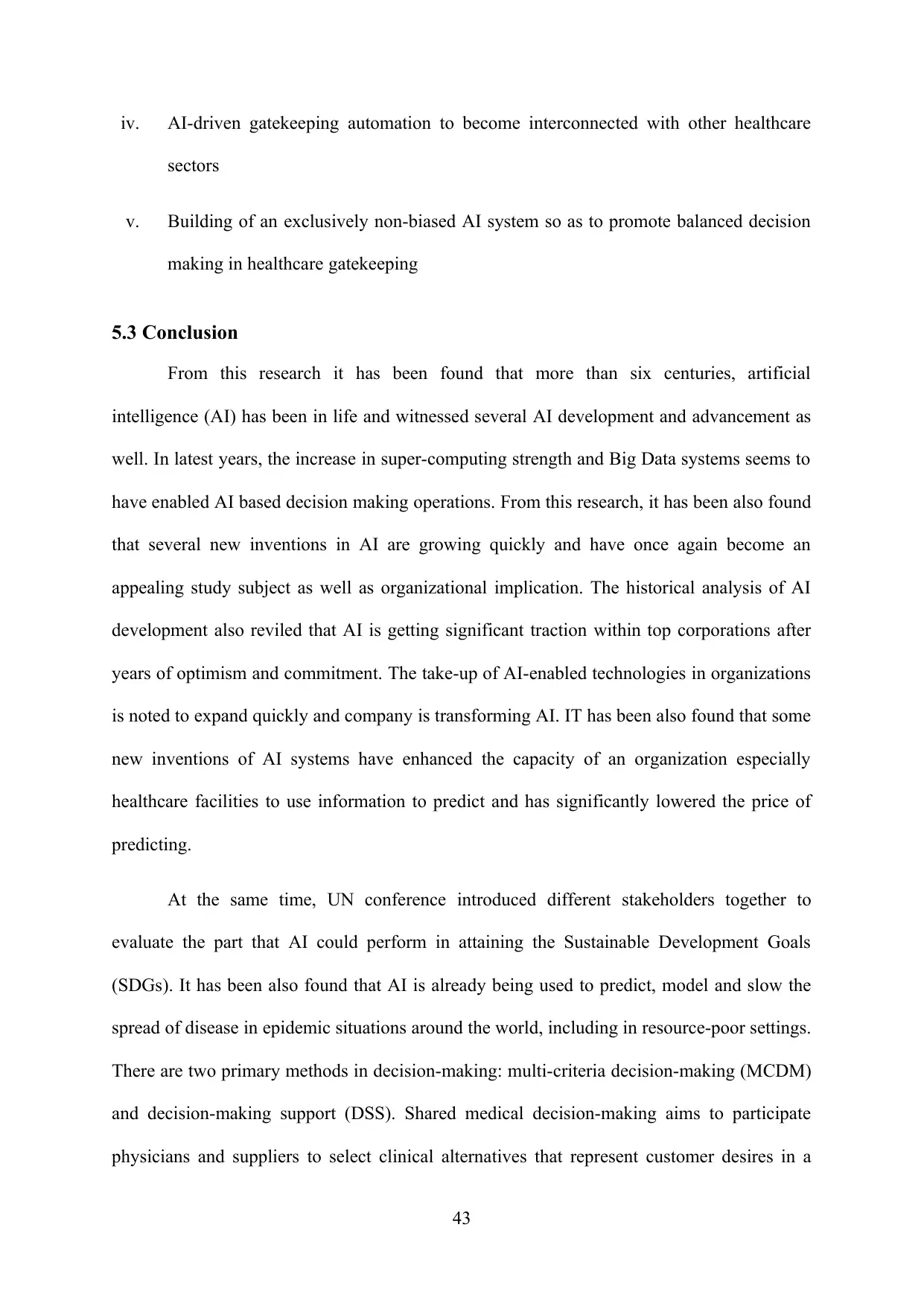
iv. AI-driven gatekeeping automation to become interconnected with other healthcare
sectors
v. Building of an exclusively non-biased AI system so as to promote balanced decision
making in healthcare gatekeeping
5.3 Conclusion
From this research it has been found that more than six centuries, artificial
intelligence (AI) has been in life and witnessed several AI development and advancement as
well. In latest years, the increase in super-computing strength and Big Data systems seems to
have enabled AI based decision making operations. From this research, it has been also found
that several new inventions in AI are growing quickly and have once again become an
appealing study subject as well as organizational implication. The historical analysis of AI
development also reviled that AI is getting significant traction within top corporations after
years of optimism and commitment. The take-up of AI-enabled technologies in organizations
is noted to expand quickly and company is transforming AI. IT has been also found that some
new inventions of AI systems have enhanced the capacity of an organization especially
healthcare facilities to use information to predict and has significantly lowered the price of
predicting.
At the same time, UN conference introduced different stakeholders together to
evaluate the part that AI could perform in attaining the Sustainable Development Goals
(SDGs). It has been also found that AI is already being used to predict, model and slow the
spread of disease in epidemic situations around the world, including in resource-poor settings.
There are two primary methods in decision-making: multi-criteria decision-making (MCDM)
and decision-making support (DSS). Shared medical decision-making aims to participate
physicians and suppliers to select clinical alternatives that represent customer desires in a
43
sectors
v. Building of an exclusively non-biased AI system so as to promote balanced decision
making in healthcare gatekeeping
5.3 Conclusion
From this research it has been found that more than six centuries, artificial
intelligence (AI) has been in life and witnessed several AI development and advancement as
well. In latest years, the increase in super-computing strength and Big Data systems seems to
have enabled AI based decision making operations. From this research, it has been also found
that several new inventions in AI are growing quickly and have once again become an
appealing study subject as well as organizational implication. The historical analysis of AI
development also reviled that AI is getting significant traction within top corporations after
years of optimism and commitment. The take-up of AI-enabled technologies in organizations
is noted to expand quickly and company is transforming AI. IT has been also found that some
new inventions of AI systems have enhanced the capacity of an organization especially
healthcare facilities to use information to predict and has significantly lowered the price of
predicting.
At the same time, UN conference introduced different stakeholders together to
evaluate the part that AI could perform in attaining the Sustainable Development Goals
(SDGs). It has been also found that AI is already being used to predict, model and slow the
spread of disease in epidemic situations around the world, including in resource-poor settings.
There are two primary methods in decision-making: multi-criteria decision-making (MCDM)
and decision-making support (DSS). Shared medical decision-making aims to participate
physicians and suppliers to select clinical alternatives that represent customer desires in a
43
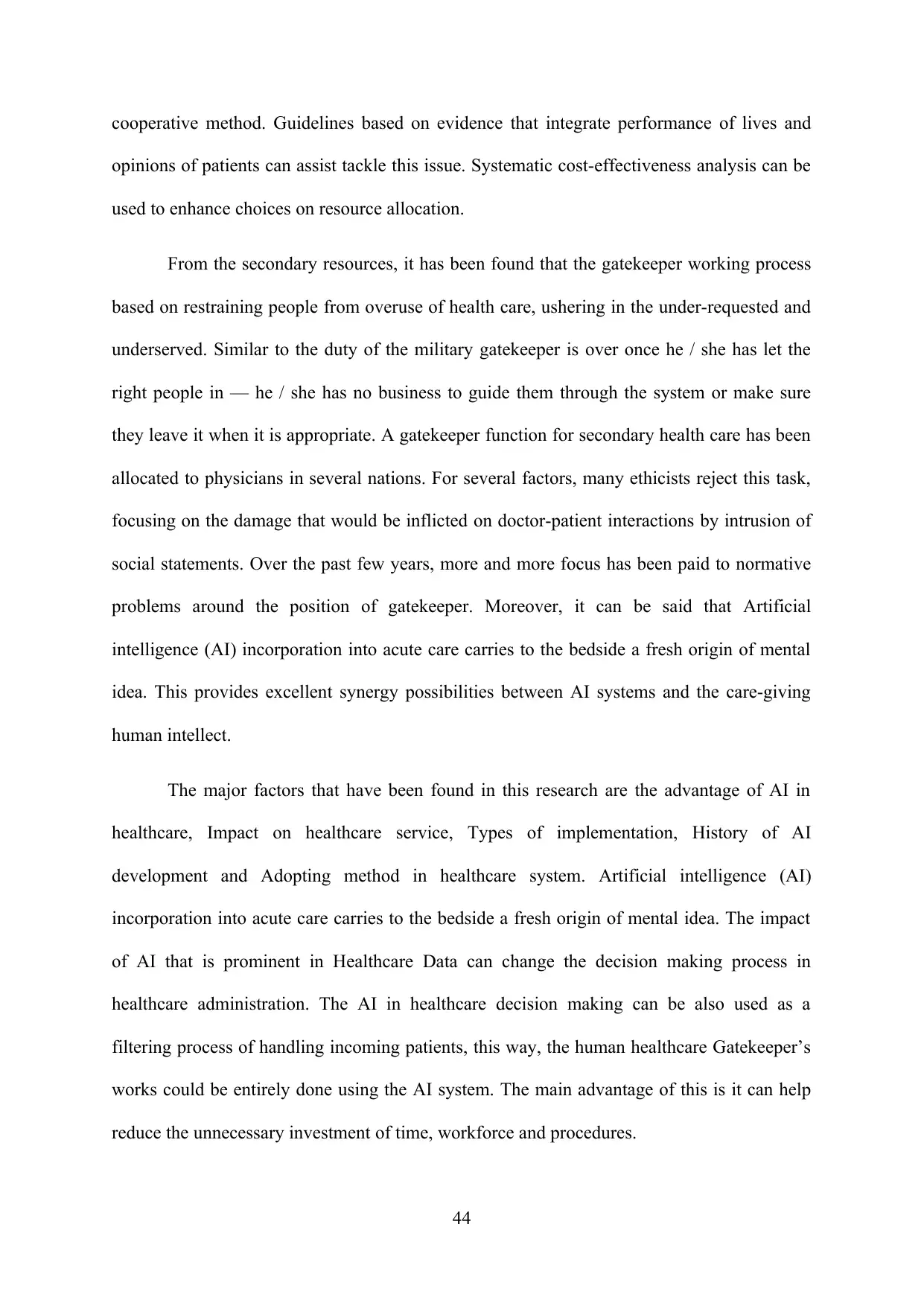
cooperative method. Guidelines based on evidence that integrate performance of lives and
opinions of patients can assist tackle this issue. Systematic cost-effectiveness analysis can be
used to enhance choices on resource allocation.
From the secondary resources, it has been found that the gatekeeper working process
based on restraining people from overuse of health care, ushering in the under-requested and
underserved. Similar to the duty of the military gatekeeper is over once he / she has let the
right people in — he / she has no business to guide them through the system or make sure
they leave it when it is appropriate. A gatekeeper function for secondary health care has been
allocated to physicians in several nations. For several factors, many ethicists reject this task,
focusing on the damage that would be inflicted on doctor-patient interactions by intrusion of
social statements. Over the past few years, more and more focus has been paid to normative
problems around the position of gatekeeper. Moreover, it can be said that Artificial
intelligence (AI) incorporation into acute care carries to the bedside a fresh origin of mental
idea. This provides excellent synergy possibilities between AI systems and the care-giving
human intellect.
The major factors that have been found in this research are the advantage of AI in
healthcare, Impact on healthcare service, Types of implementation, History of AI
development and Adopting method in healthcare system. Artificial intelligence (AI)
incorporation into acute care carries to the bedside a fresh origin of mental idea. The impact
of AI that is prominent in Healthcare Data can change the decision making process in
healthcare administration. The AI in healthcare decision making can be also used as a
filtering process of handling incoming patients, this way, the human healthcare Gatekeeper’s
works could be entirely done using the AI system. The main advantage of this is it can help
reduce the unnecessary investment of time, workforce and procedures.
44
opinions of patients can assist tackle this issue. Systematic cost-effectiveness analysis can be
used to enhance choices on resource allocation.
From the secondary resources, it has been found that the gatekeeper working process
based on restraining people from overuse of health care, ushering in the under-requested and
underserved. Similar to the duty of the military gatekeeper is over once he / she has let the
right people in — he / she has no business to guide them through the system or make sure
they leave it when it is appropriate. A gatekeeper function for secondary health care has been
allocated to physicians in several nations. For several factors, many ethicists reject this task,
focusing on the damage that would be inflicted on doctor-patient interactions by intrusion of
social statements. Over the past few years, more and more focus has been paid to normative
problems around the position of gatekeeper. Moreover, it can be said that Artificial
intelligence (AI) incorporation into acute care carries to the bedside a fresh origin of mental
idea. This provides excellent synergy possibilities between AI systems and the care-giving
human intellect.
The major factors that have been found in this research are the advantage of AI in
healthcare, Impact on healthcare service, Types of implementation, History of AI
development and Adopting method in healthcare system. Artificial intelligence (AI)
incorporation into acute care carries to the bedside a fresh origin of mental idea. The impact
of AI that is prominent in Healthcare Data can change the decision making process in
healthcare administration. The AI in healthcare decision making can be also used as a
filtering process of handling incoming patients, this way, the human healthcare Gatekeeper’s
works could be entirely done using the AI system. The main advantage of this is it can help
reduce the unnecessary investment of time, workforce and procedures.
44
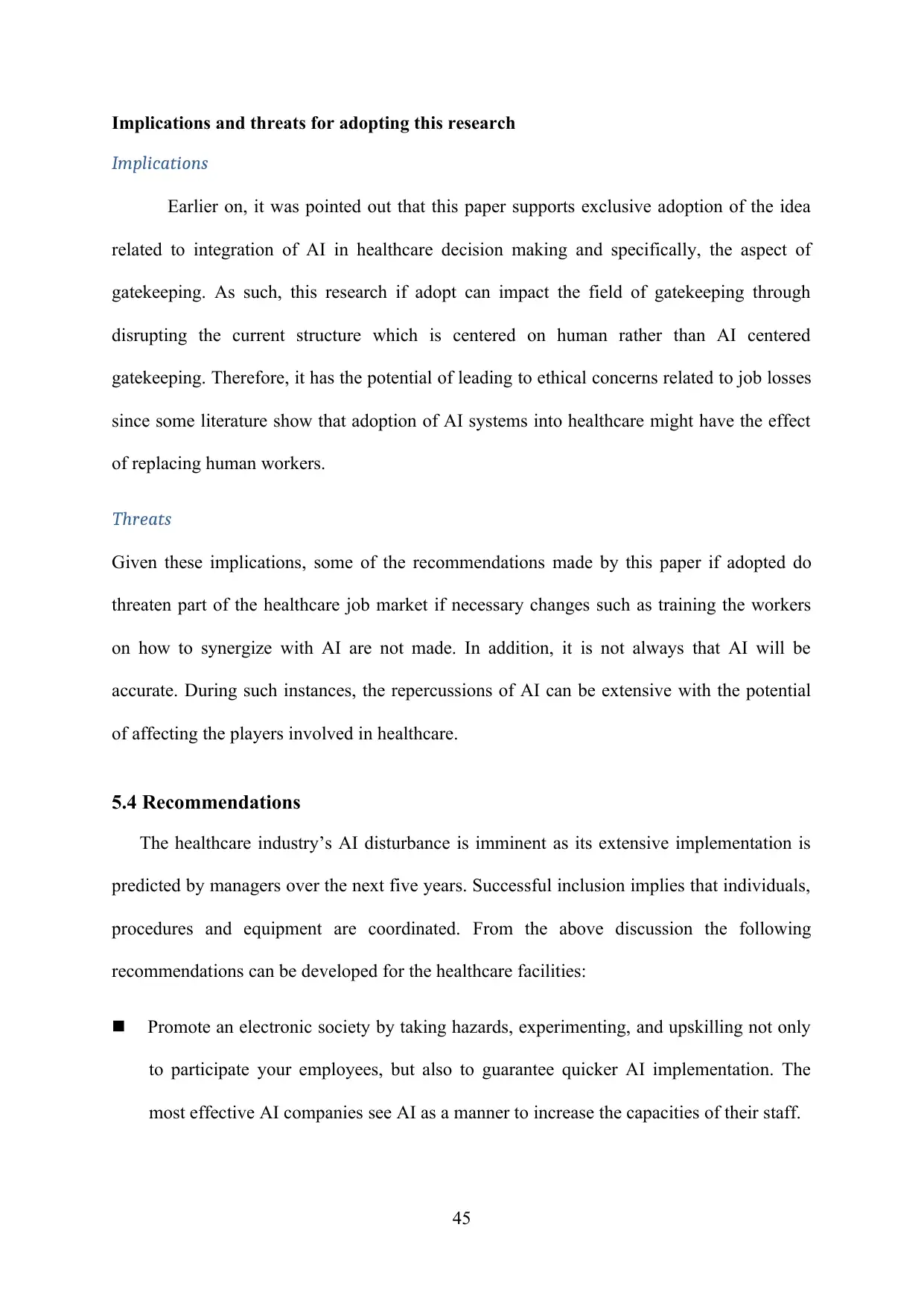
Implications and threats for adopting this research
Implications
Earlier on, it was pointed out that this paper supports exclusive adoption of the idea
related to integration of AI in healthcare decision making and specifically, the aspect of
gatekeeping. As such, this research if adopt can impact the field of gatekeeping through
disrupting the current structure which is centered on human rather than AI centered
gatekeeping. Therefore, it has the potential of leading to ethical concerns related to job losses
since some literature show that adoption of AI systems into healthcare might have the effect
of replacing human workers.
Threats
Given these implications, some of the recommendations made by this paper if adopted do
threaten part of the healthcare job market if necessary changes such as training the workers
on how to synergize with AI are not made. In addition, it is not always that AI will be
accurate. During such instances, the repercussions of AI can be extensive with the potential
of affecting the players involved in healthcare.
5.4 Recommendations
The healthcare industry’s AI disturbance is imminent as its extensive implementation is
predicted by managers over the next five years. Successful inclusion implies that individuals,
procedures and equipment are coordinated. From the above discussion the following
recommendations can be developed for the healthcare facilities:
Promote an electronic society by taking hazards, experimenting, and upskilling not only
to participate your employees, but also to guarantee quicker AI implementation. The
most effective AI companies see AI as a manner to increase the capacities of their staff.
45
Implications
Earlier on, it was pointed out that this paper supports exclusive adoption of the idea
related to integration of AI in healthcare decision making and specifically, the aspect of
gatekeeping. As such, this research if adopt can impact the field of gatekeeping through
disrupting the current structure which is centered on human rather than AI centered
gatekeeping. Therefore, it has the potential of leading to ethical concerns related to job losses
since some literature show that adoption of AI systems into healthcare might have the effect
of replacing human workers.
Threats
Given these implications, some of the recommendations made by this paper if adopted do
threaten part of the healthcare job market if necessary changes such as training the workers
on how to synergize with AI are not made. In addition, it is not always that AI will be
accurate. During such instances, the repercussions of AI can be extensive with the potential
of affecting the players involved in healthcare.
5.4 Recommendations
The healthcare industry’s AI disturbance is imminent as its extensive implementation is
predicted by managers over the next five years. Successful inclusion implies that individuals,
procedures and equipment are coordinated. From the above discussion the following
recommendations can be developed for the healthcare facilities:
Promote an electronic society by taking hazards, experimenting, and upskilling not only
to participate your employees, but also to guarantee quicker AI implementation. The
most effective AI companies see AI as a manner to increase the capacities of their staff.
45
Secure Best Marks with AI Grader
Need help grading? Try our AI Grader for instant feedback on your assignments.
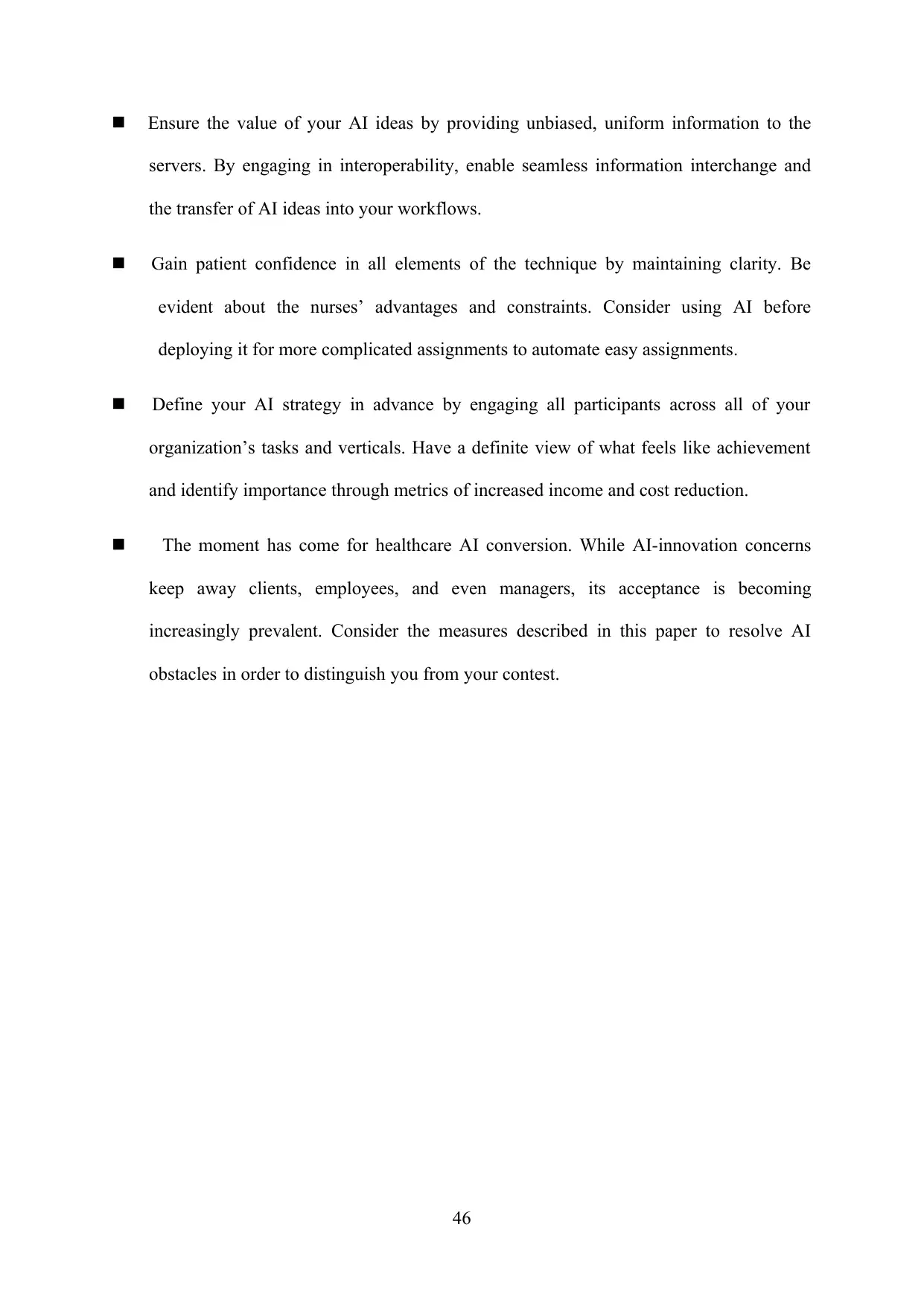
Ensure the value of your AI ideas by providing unbiased, uniform information to the
servers. By engaging in interoperability, enable seamless information interchange and
the transfer of AI ideas into your workflows.
Gain patient confidence in all elements of the technique by maintaining clarity. Be
evident about the nurses’ advantages and constraints. Consider using AI before
deploying it for more complicated assignments to automate easy assignments.
Define your AI strategy in advance by engaging all participants across all of your
organization’s tasks and verticals. Have a definite view of what feels like achievement
and identify importance through metrics of increased income and cost reduction.
The moment has come for healthcare AI conversion. While AI-innovation concerns
keep away clients, employees, and even managers, its acceptance is becoming
increasingly prevalent. Consider the measures described in this paper to resolve AI
obstacles in order to distinguish you from your contest.
46
servers. By engaging in interoperability, enable seamless information interchange and
the transfer of AI ideas into your workflows.
Gain patient confidence in all elements of the technique by maintaining clarity. Be
evident about the nurses’ advantages and constraints. Consider using AI before
deploying it for more complicated assignments to automate easy assignments.
Define your AI strategy in advance by engaging all participants across all of your
organization’s tasks and verticals. Have a definite view of what feels like achievement
and identify importance through metrics of increased income and cost reduction.
The moment has come for healthcare AI conversion. While AI-innovation concerns
keep away clients, employees, and even managers, its acceptance is becoming
increasingly prevalent. Consider the measures described in this paper to resolve AI
obstacles in order to distinguish you from your contest.
46
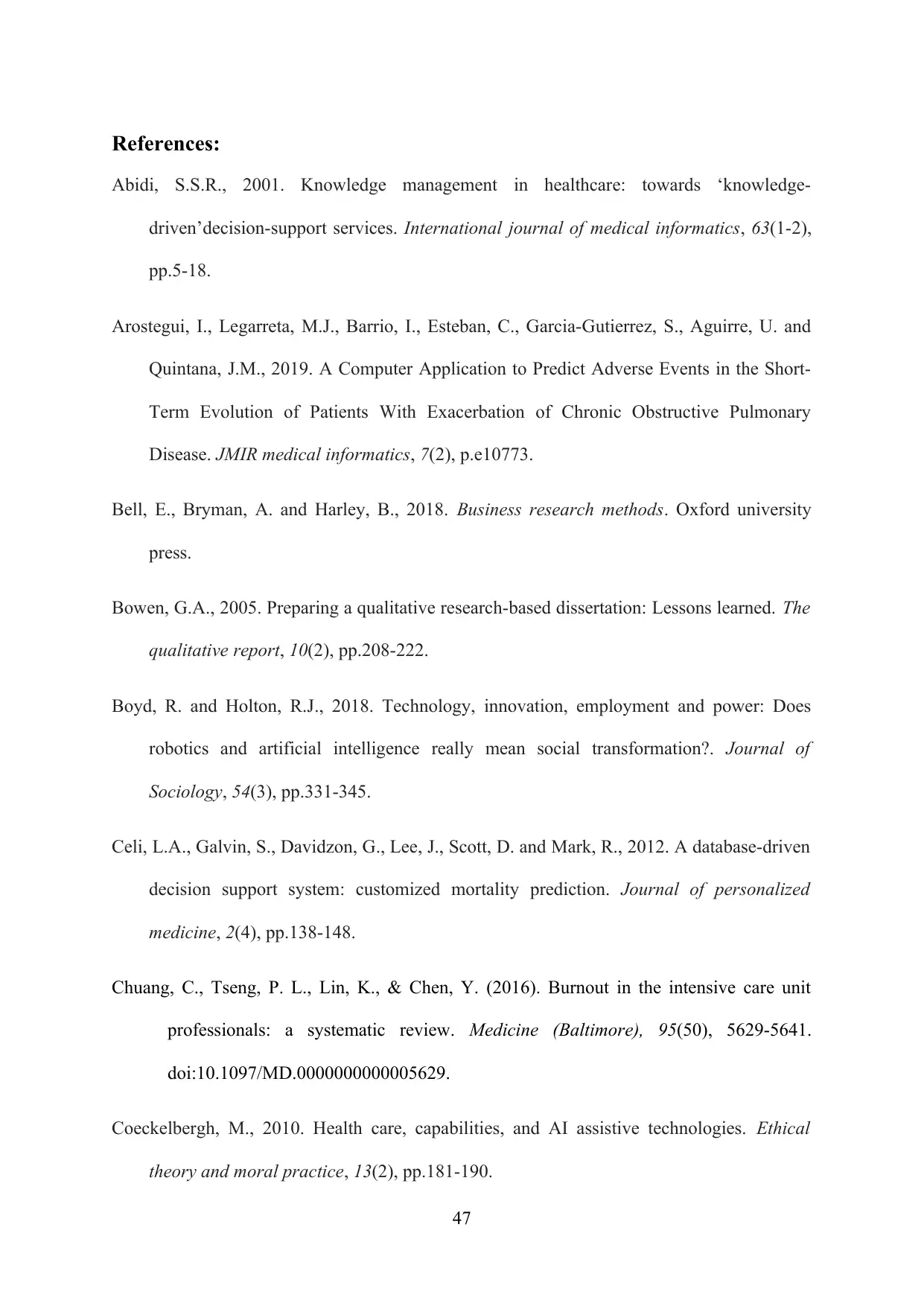
References:
Abidi, S.S.R., 2001. Knowledge management in healthcare: towards ‘knowledge-
driven’decision-support services. International journal of medical informatics, 63(1-2),
pp.5-18.
Arostegui, I., Legarreta, M.J., Barrio, I., Esteban, C., Garcia-Gutierrez, S., Aguirre, U. and
Quintana, J.M., 2019. A Computer Application to Predict Adverse Events in the Short-
Term Evolution of Patients With Exacerbation of Chronic Obstructive Pulmonary
Disease. JMIR medical informatics, 7(2), p.e10773.
Bell, E., Bryman, A. and Harley, B., 2018. Business research methods. Oxford university
press.
Bowen, G.A., 2005. Preparing a qualitative research-based dissertation: Lessons learned. The
qualitative report, 10(2), pp.208-222.
Boyd, R. and Holton, R.J., 2018. Technology, innovation, employment and power: Does
robotics and artificial intelligence really mean social transformation?. Journal of
Sociology, 54(3), pp.331-345.
Celi, L.A., Galvin, S., Davidzon, G., Lee, J., Scott, D. and Mark, R., 2012. A database-driven
decision support system: customized mortality prediction. Journal of personalized
medicine, 2(4), pp.138-148.
Chuang, C., Tseng, P. L., Lin, K., & Chen, Y. (2016). Burnout in the intensive care unit
professionals: a systematic review. Medicine (Baltimore), 95(50), 5629-5641.
doi:10.1097/MD.0000000000005629.
Coeckelbergh, M., 2010. Health care, capabilities, and AI assistive technologies. Ethical
theory and moral practice, 13(2), pp.181-190.
47
Abidi, S.S.R., 2001. Knowledge management in healthcare: towards ‘knowledge-
driven’decision-support services. International journal of medical informatics, 63(1-2),
pp.5-18.
Arostegui, I., Legarreta, M.J., Barrio, I., Esteban, C., Garcia-Gutierrez, S., Aguirre, U. and
Quintana, J.M., 2019. A Computer Application to Predict Adverse Events in the Short-
Term Evolution of Patients With Exacerbation of Chronic Obstructive Pulmonary
Disease. JMIR medical informatics, 7(2), p.e10773.
Bell, E., Bryman, A. and Harley, B., 2018. Business research methods. Oxford university
press.
Bowen, G.A., 2005. Preparing a qualitative research-based dissertation: Lessons learned. The
qualitative report, 10(2), pp.208-222.
Boyd, R. and Holton, R.J., 2018. Technology, innovation, employment and power: Does
robotics and artificial intelligence really mean social transformation?. Journal of
Sociology, 54(3), pp.331-345.
Celi, L.A., Galvin, S., Davidzon, G., Lee, J., Scott, D. and Mark, R., 2012. A database-driven
decision support system: customized mortality prediction. Journal of personalized
medicine, 2(4), pp.138-148.
Chuang, C., Tseng, P. L., Lin, K., & Chen, Y. (2016). Burnout in the intensive care unit
professionals: a systematic review. Medicine (Baltimore), 95(50), 5629-5641.
doi:10.1097/MD.0000000000005629.
Coeckelbergh, M., 2010. Health care, capabilities, and AI assistive technologies. Ethical
theory and moral practice, 13(2), pp.181-190.
47
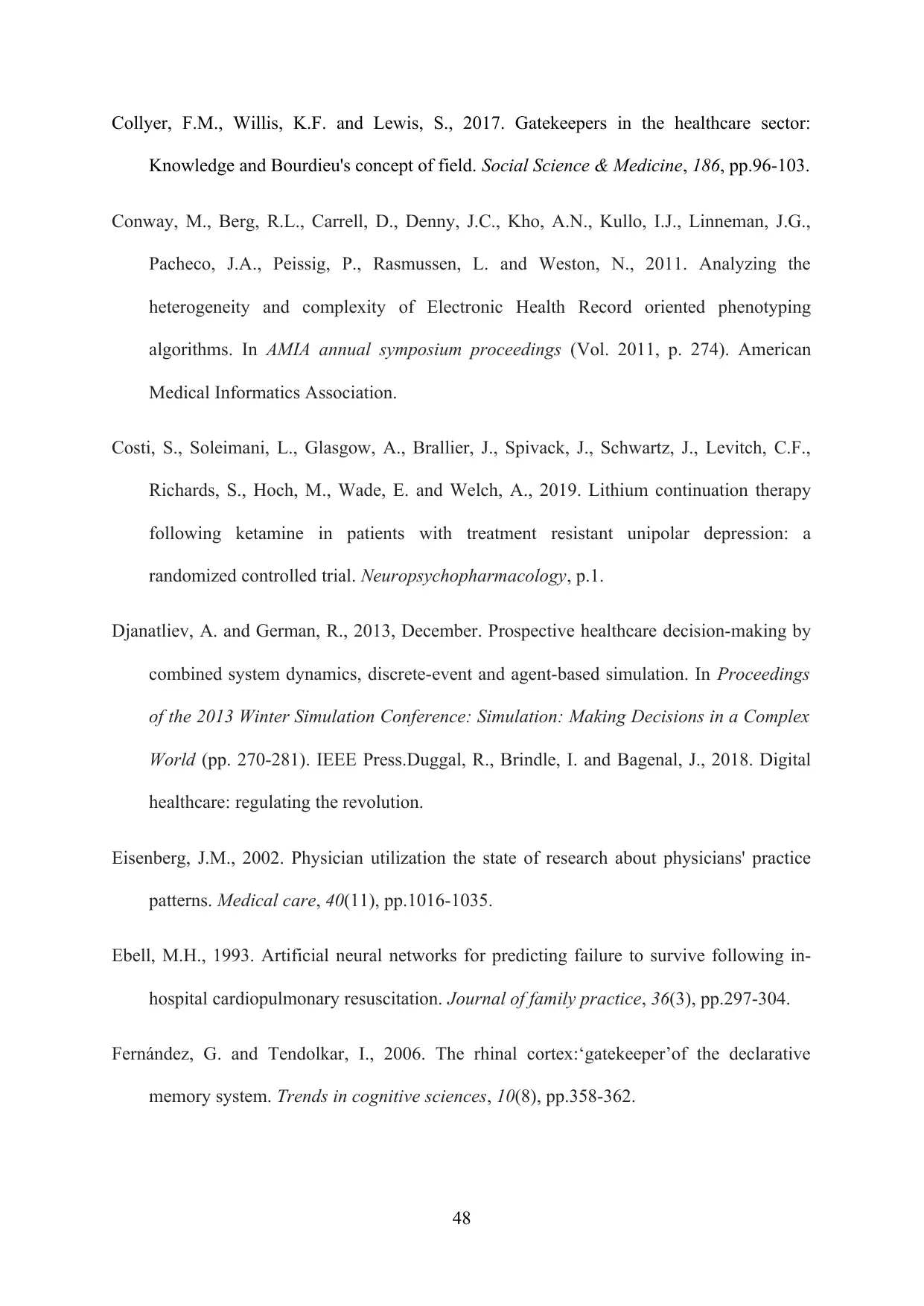
Collyer, F.M., Willis, K.F. and Lewis, S., 2017. Gatekeepers in the healthcare sector:
Knowledge and Bourdieu's concept of field. Social Science & Medicine, 186, pp.96-103.
Conway, M., Berg, R.L., Carrell, D., Denny, J.C., Kho, A.N., Kullo, I.J., Linneman, J.G.,
Pacheco, J.A., Peissig, P., Rasmussen, L. and Weston, N., 2011. Analyzing the
heterogeneity and complexity of Electronic Health Record oriented phenotyping
algorithms. In AMIA annual symposium proceedings (Vol. 2011, p. 274). American
Medical Informatics Association.
Costi, S., Soleimani, L., Glasgow, A., Brallier, J., Spivack, J., Schwartz, J., Levitch, C.F.,
Richards, S., Hoch, M., Wade, E. and Welch, A., 2019. Lithium continuation therapy
following ketamine in patients with treatment resistant unipolar depression: a
randomized controlled trial. Neuropsychopharmacology, p.1.
Djanatliev, A. and German, R., 2013, December. Prospective healthcare decision-making by
combined system dynamics, discrete-event and agent-based simulation. In Proceedings
of the 2013 Winter Simulation Conference: Simulation: Making Decisions in a Complex
World (pp. 270-281). IEEE Press.Duggal, R., Brindle, I. and Bagenal, J., 2018. Digital
healthcare: regulating the revolution.
Eisenberg, J.M., 2002. Physician utilization the state of research about physicians' practice
patterns. Medical care, 40(11), pp.1016-1035.
Ebell, M.H., 1993. Artificial neural networks for predicting failure to survive following in-
hospital cardiopulmonary resuscitation. Journal of family practice, 36(3), pp.297-304.
Fernández, G. and Tendolkar, I., 2006. The rhinal cortex:‘gatekeeper’of the declarative
memory system. Trends in cognitive sciences, 10(8), pp.358-362.
48
Knowledge and Bourdieu's concept of field. Social Science & Medicine, 186, pp.96-103.
Conway, M., Berg, R.L., Carrell, D., Denny, J.C., Kho, A.N., Kullo, I.J., Linneman, J.G.,
Pacheco, J.A., Peissig, P., Rasmussen, L. and Weston, N., 2011. Analyzing the
heterogeneity and complexity of Electronic Health Record oriented phenotyping
algorithms. In AMIA annual symposium proceedings (Vol. 2011, p. 274). American
Medical Informatics Association.
Costi, S., Soleimani, L., Glasgow, A., Brallier, J., Spivack, J., Schwartz, J., Levitch, C.F.,
Richards, S., Hoch, M., Wade, E. and Welch, A., 2019. Lithium continuation therapy
following ketamine in patients with treatment resistant unipolar depression: a
randomized controlled trial. Neuropsychopharmacology, p.1.
Djanatliev, A. and German, R., 2013, December. Prospective healthcare decision-making by
combined system dynamics, discrete-event and agent-based simulation. In Proceedings
of the 2013 Winter Simulation Conference: Simulation: Making Decisions in a Complex
World (pp. 270-281). IEEE Press.Duggal, R., Brindle, I. and Bagenal, J., 2018. Digital
healthcare: regulating the revolution.
Eisenberg, J.M., 2002. Physician utilization the state of research about physicians' practice
patterns. Medical care, 40(11), pp.1016-1035.
Ebell, M.H., 1993. Artificial neural networks for predicting failure to survive following in-
hospital cardiopulmonary resuscitation. Journal of family practice, 36(3), pp.297-304.
Fernández, G. and Tendolkar, I., 2006. The rhinal cortex:‘gatekeeper’of the declarative
memory system. Trends in cognitive sciences, 10(8), pp.358-362.
48
Paraphrase This Document
Need a fresh take? Get an instant paraphrase of this document with our AI Paraphraser
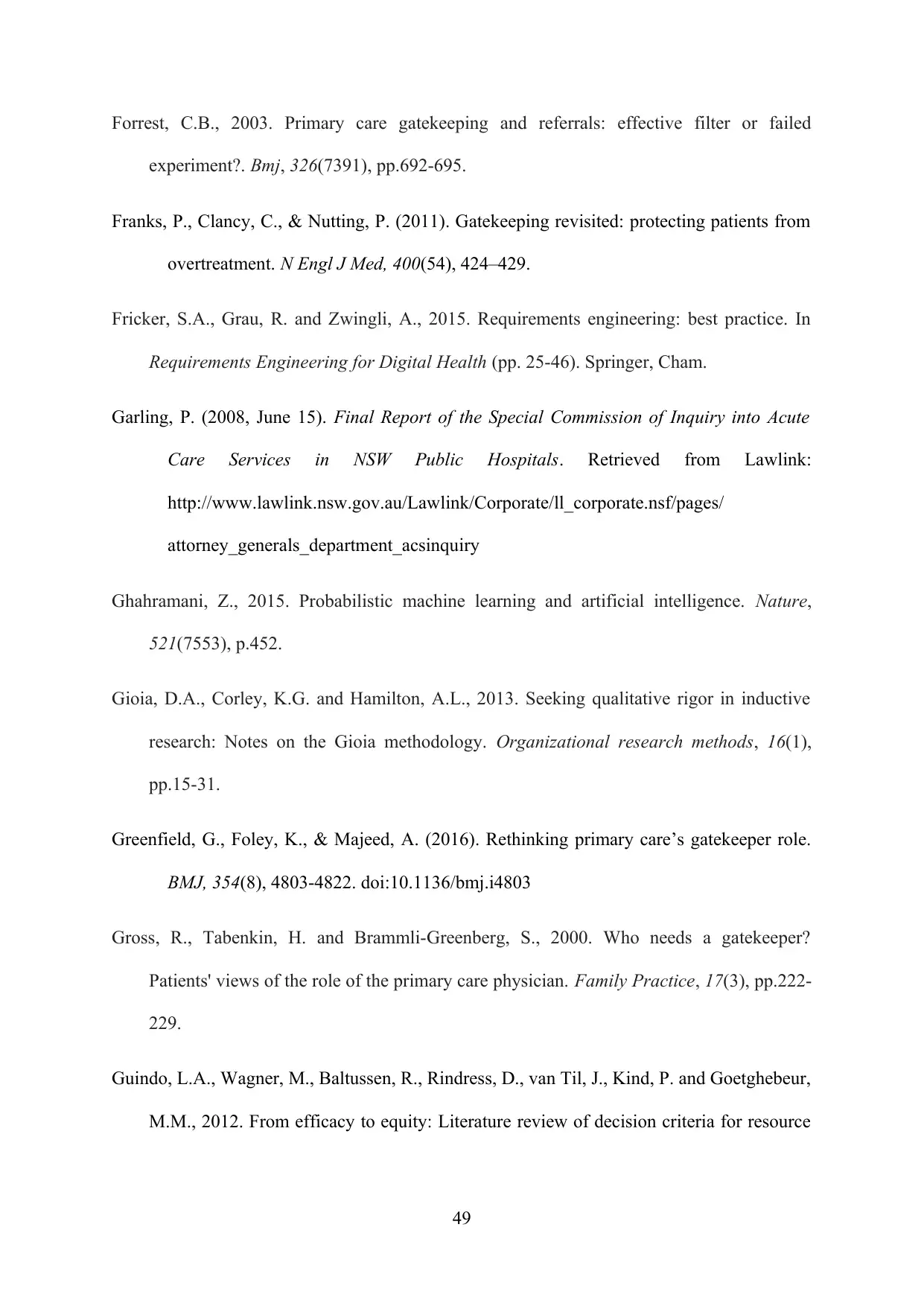
Forrest, C.B., 2003. Primary care gatekeeping and referrals: effective filter or failed
experiment?. Bmj, 326(7391), pp.692-695.
Franks, P., Clancy, C., & Nutting, P. (2011). Gatekeeping revisited: protecting patients from
overtreatment. N Engl J Med, 400(54), 424–429.
Fricker, S.A., Grau, R. and Zwingli, A., 2015. Requirements engineering: best practice. In
Requirements Engineering for Digital Health (pp. 25-46). Springer, Cham.
Garling, P. (2008, June 15). Final Report of the Special Commission of Inquiry into Acute
Care Services in NSW Public Hospitals. Retrieved from Lawlink:
http://www.lawlink.nsw.gov.au/Lawlink/Corporate/ll_corporate.nsf/pages/
attorney_generals_department_acsinquiry
Ghahramani, Z., 2015. Probabilistic machine learning and artificial intelligence. Nature,
521(7553), p.452.
Gioia, D.A., Corley, K.G. and Hamilton, A.L., 2013. Seeking qualitative rigor in inductive
research: Notes on the Gioia methodology. Organizational research methods, 16(1),
pp.15-31.
Greenfield, G., Foley, K., & Majeed, A. (2016). Rethinking primary care’s gatekeeper role.
BMJ, 354(8), 4803-4822. doi:10.1136/bmj.i4803
Gross, R., Tabenkin, H. and Brammli-Greenberg, S., 2000. Who needs a gatekeeper?
Patients' views of the role of the primary care physician. Family Practice, 17(3), pp.222-
229.
Guindo, L.A., Wagner, M., Baltussen, R., Rindress, D., van Til, J., Kind, P. and Goetghebeur,
M.M., 2012. From efficacy to equity: Literature review of decision criteria for resource
49
experiment?. Bmj, 326(7391), pp.692-695.
Franks, P., Clancy, C., & Nutting, P. (2011). Gatekeeping revisited: protecting patients from
overtreatment. N Engl J Med, 400(54), 424–429.
Fricker, S.A., Grau, R. and Zwingli, A., 2015. Requirements engineering: best practice. In
Requirements Engineering for Digital Health (pp. 25-46). Springer, Cham.
Garling, P. (2008, June 15). Final Report of the Special Commission of Inquiry into Acute
Care Services in NSW Public Hospitals. Retrieved from Lawlink:
http://www.lawlink.nsw.gov.au/Lawlink/Corporate/ll_corporate.nsf/pages/
attorney_generals_department_acsinquiry
Ghahramani, Z., 2015. Probabilistic machine learning and artificial intelligence. Nature,
521(7553), p.452.
Gioia, D.A., Corley, K.G. and Hamilton, A.L., 2013. Seeking qualitative rigor in inductive
research: Notes on the Gioia methodology. Organizational research methods, 16(1),
pp.15-31.
Greenfield, G., Foley, K., & Majeed, A. (2016). Rethinking primary care’s gatekeeper role.
BMJ, 354(8), 4803-4822. doi:10.1136/bmj.i4803
Gross, R., Tabenkin, H. and Brammli-Greenberg, S., 2000. Who needs a gatekeeper?
Patients' views of the role of the primary care physician. Family Practice, 17(3), pp.222-
229.
Guindo, L.A., Wagner, M., Baltussen, R., Rindress, D., van Til, J., Kind, P. and Goetghebeur,
M.M., 2012. From efficacy to equity: Literature review of decision criteria for resource
49
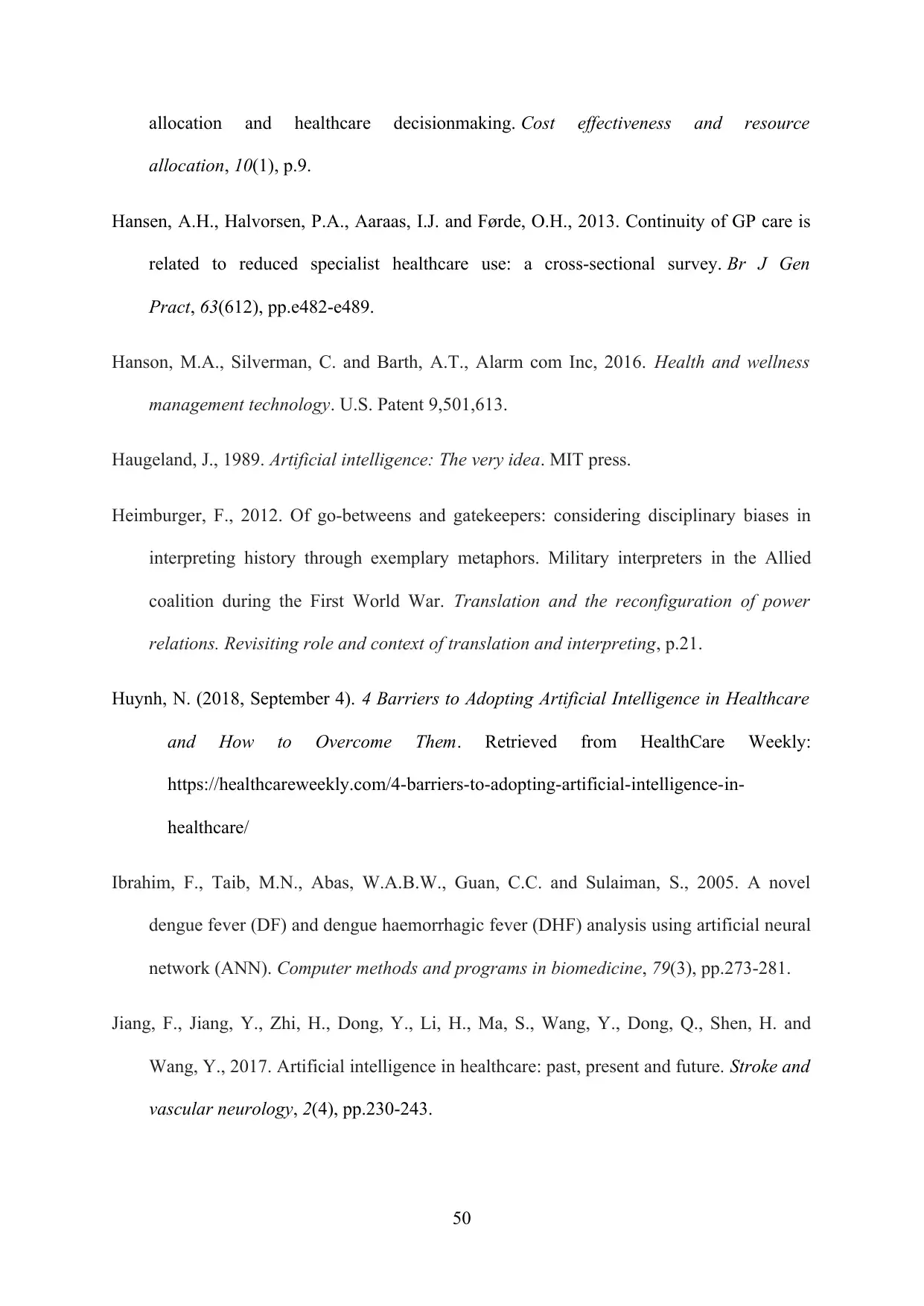
allocation and healthcare decisionmaking. Cost effectiveness and resource
allocation, 10(1), p.9.
Hansen, A.H., Halvorsen, P.A., Aaraas, I.J. and Førde, O.H., 2013. Continuity of GP care is
related to reduced specialist healthcare use: a cross-sectional survey. Br J Gen
Pract, 63(612), pp.e482-e489.
Hanson, M.A., Silverman, C. and Barth, A.T., Alarm com Inc, 2016. Health and wellness
management technology. U.S. Patent 9,501,613.
Haugeland, J., 1989. Artificial intelligence: The very idea. MIT press.
Heimburger, F., 2012. Of go-betweens and gatekeepers: considering disciplinary biases in
interpreting history through exemplary metaphors. Military interpreters in the Allied
coalition during the First World War. Translation and the reconfiguration of power
relations. Revisiting role and context of translation and interpreting, p.21.
Huynh, N. (2018, September 4). 4 Barriers to Adopting Artificial Intelligence in Healthcare
and How to Overcome Them. Retrieved from HealthCare Weekly:
https://healthcareweekly.com/4-barriers-to-adopting-artificial-intelligence-in-
healthcare/
Ibrahim, F., Taib, M.N., Abas, W.A.B.W., Guan, C.C. and Sulaiman, S., 2005. A novel
dengue fever (DF) and dengue haemorrhagic fever (DHF) analysis using artificial neural
network (ANN). Computer methods and programs in biomedicine, 79(3), pp.273-281.
Jiang, F., Jiang, Y., Zhi, H., Dong, Y., Li, H., Ma, S., Wang, Y., Dong, Q., Shen, H. and
Wang, Y., 2017. Artificial intelligence in healthcare: past, present and future. Stroke and
vascular neurology, 2(4), pp.230-243.
50
allocation, 10(1), p.9.
Hansen, A.H., Halvorsen, P.A., Aaraas, I.J. and Førde, O.H., 2013. Continuity of GP care is
related to reduced specialist healthcare use: a cross-sectional survey. Br J Gen
Pract, 63(612), pp.e482-e489.
Hanson, M.A., Silverman, C. and Barth, A.T., Alarm com Inc, 2016. Health and wellness
management technology. U.S. Patent 9,501,613.
Haugeland, J., 1989. Artificial intelligence: The very idea. MIT press.
Heimburger, F., 2012. Of go-betweens and gatekeepers: considering disciplinary biases in
interpreting history through exemplary metaphors. Military interpreters in the Allied
coalition during the First World War. Translation and the reconfiguration of power
relations. Revisiting role and context of translation and interpreting, p.21.
Huynh, N. (2018, September 4). 4 Barriers to Adopting Artificial Intelligence in Healthcare
and How to Overcome Them. Retrieved from HealthCare Weekly:
https://healthcareweekly.com/4-barriers-to-adopting-artificial-intelligence-in-
healthcare/
Ibrahim, F., Taib, M.N., Abas, W.A.B.W., Guan, C.C. and Sulaiman, S., 2005. A novel
dengue fever (DF) and dengue haemorrhagic fever (DHF) analysis using artificial neural
network (ANN). Computer methods and programs in biomedicine, 79(3), pp.273-281.
Jiang, F., Jiang, Y., Zhi, H., Dong, Y., Li, H., Ma, S., Wang, Y., Dong, Q., Shen, H. and
Wang, Y., 2017. Artificial intelligence in healthcare: past, present and future. Stroke and
vascular neurology, 2(4), pp.230-243.
50
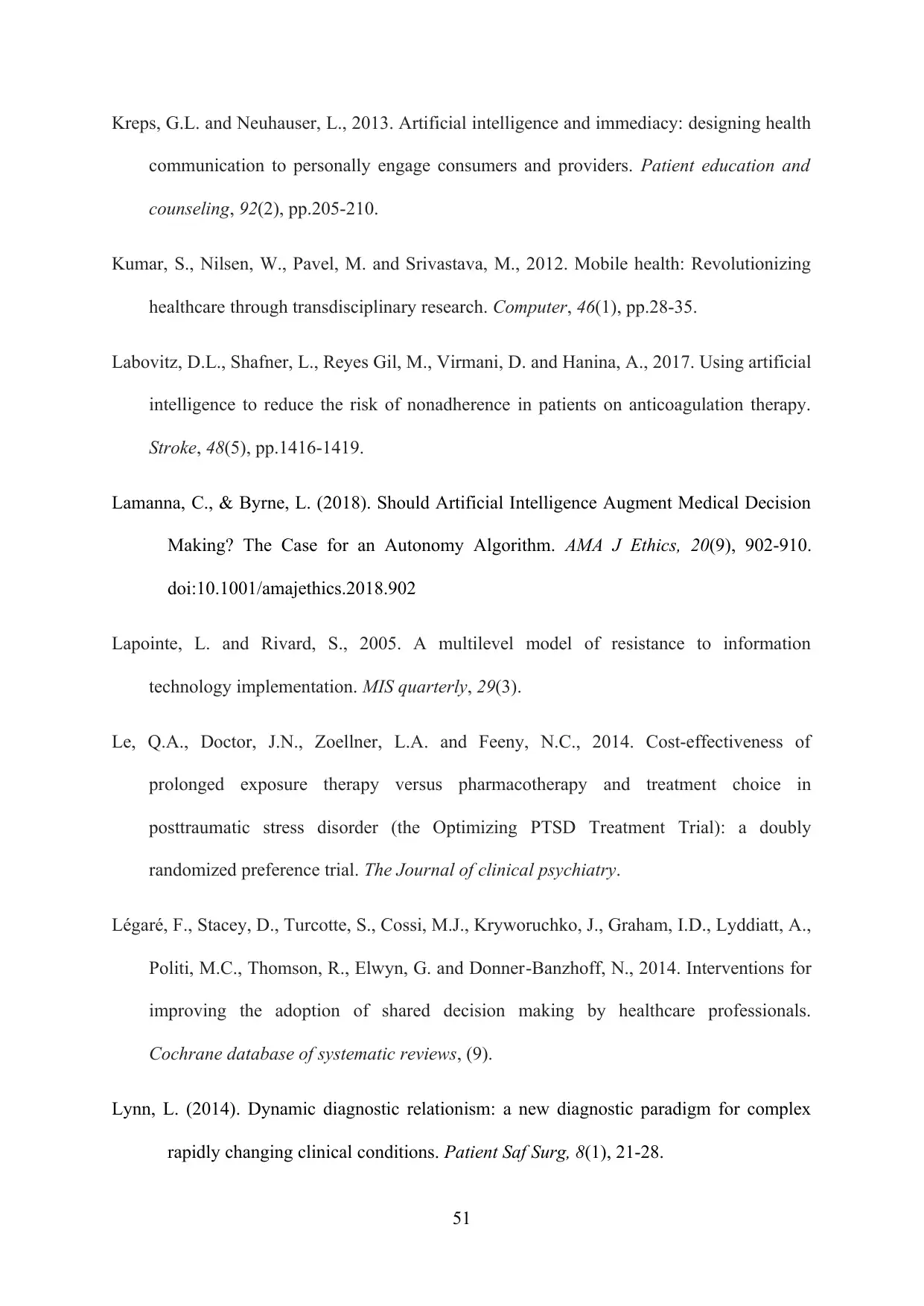
Kreps, G.L. and Neuhauser, L., 2013. Artificial intelligence and immediacy: designing health
communication to personally engage consumers and providers. Patient education and
counseling, 92(2), pp.205-210.
Kumar, S., Nilsen, W., Pavel, M. and Srivastava, M., 2012. Mobile health: Revolutionizing
healthcare through transdisciplinary research. Computer, 46(1), pp.28-35.
Labovitz, D.L., Shafner, L., Reyes Gil, M., Virmani, D. and Hanina, A., 2017. Using artificial
intelligence to reduce the risk of nonadherence in patients on anticoagulation therapy.
Stroke, 48(5), pp.1416-1419.
Lamanna, C., & Byrne, L. (2018). Should Artificial Intelligence Augment Medical Decision
Making? The Case for an Autonomy Algorithm. AMA J Ethics, 20(9), 902-910.
doi:10.1001/amajethics.2018.902
Lapointe, L. and Rivard, S., 2005. A multilevel model of resistance to information
technology implementation. MIS quarterly, 29(3).
Le, Q.A., Doctor, J.N., Zoellner, L.A. and Feeny, N.C., 2014. Cost-effectiveness of
prolonged exposure therapy versus pharmacotherapy and treatment choice in
posttraumatic stress disorder (the Optimizing PTSD Treatment Trial): a doubly
randomized preference trial. The Journal of clinical psychiatry.
Légaré, F., Stacey, D., Turcotte, S., Cossi, M.J., Kryworuchko, J., Graham, I.D., Lyddiatt, A.,
Politi, M.C., Thomson, R., Elwyn, G. and Donner‐Banzhoff, N., 2014. Interventions for
improving the adoption of shared decision making by healthcare professionals.
Cochrane database of systematic reviews, (9).
Lynn, L. (2014). Dynamic diagnostic relationism: a new diagnostic paradigm for complex
rapidly changing clinical conditions. Patient Saf Surg, 8(1), 21-28.
51
communication to personally engage consumers and providers. Patient education and
counseling, 92(2), pp.205-210.
Kumar, S., Nilsen, W., Pavel, M. and Srivastava, M., 2012. Mobile health: Revolutionizing
healthcare through transdisciplinary research. Computer, 46(1), pp.28-35.
Labovitz, D.L., Shafner, L., Reyes Gil, M., Virmani, D. and Hanina, A., 2017. Using artificial
intelligence to reduce the risk of nonadherence in patients on anticoagulation therapy.
Stroke, 48(5), pp.1416-1419.
Lamanna, C., & Byrne, L. (2018). Should Artificial Intelligence Augment Medical Decision
Making? The Case for an Autonomy Algorithm. AMA J Ethics, 20(9), 902-910.
doi:10.1001/amajethics.2018.902
Lapointe, L. and Rivard, S., 2005. A multilevel model of resistance to information
technology implementation. MIS quarterly, 29(3).
Le, Q.A., Doctor, J.N., Zoellner, L.A. and Feeny, N.C., 2014. Cost-effectiveness of
prolonged exposure therapy versus pharmacotherapy and treatment choice in
posttraumatic stress disorder (the Optimizing PTSD Treatment Trial): a doubly
randomized preference trial. The Journal of clinical psychiatry.
Légaré, F., Stacey, D., Turcotte, S., Cossi, M.J., Kryworuchko, J., Graham, I.D., Lyddiatt, A.,
Politi, M.C., Thomson, R., Elwyn, G. and Donner‐Banzhoff, N., 2014. Interventions for
improving the adoption of shared decision making by healthcare professionals.
Cochrane database of systematic reviews, (9).
Lynn, L. (2014). Dynamic diagnostic relationism: a new diagnostic paradigm for complex
rapidly changing clinical conditions. Patient Saf Surg, 8(1), 21-28.
51
Secure Best Marks with AI Grader
Need help grading? Try our AI Grader for instant feedback on your assignments.
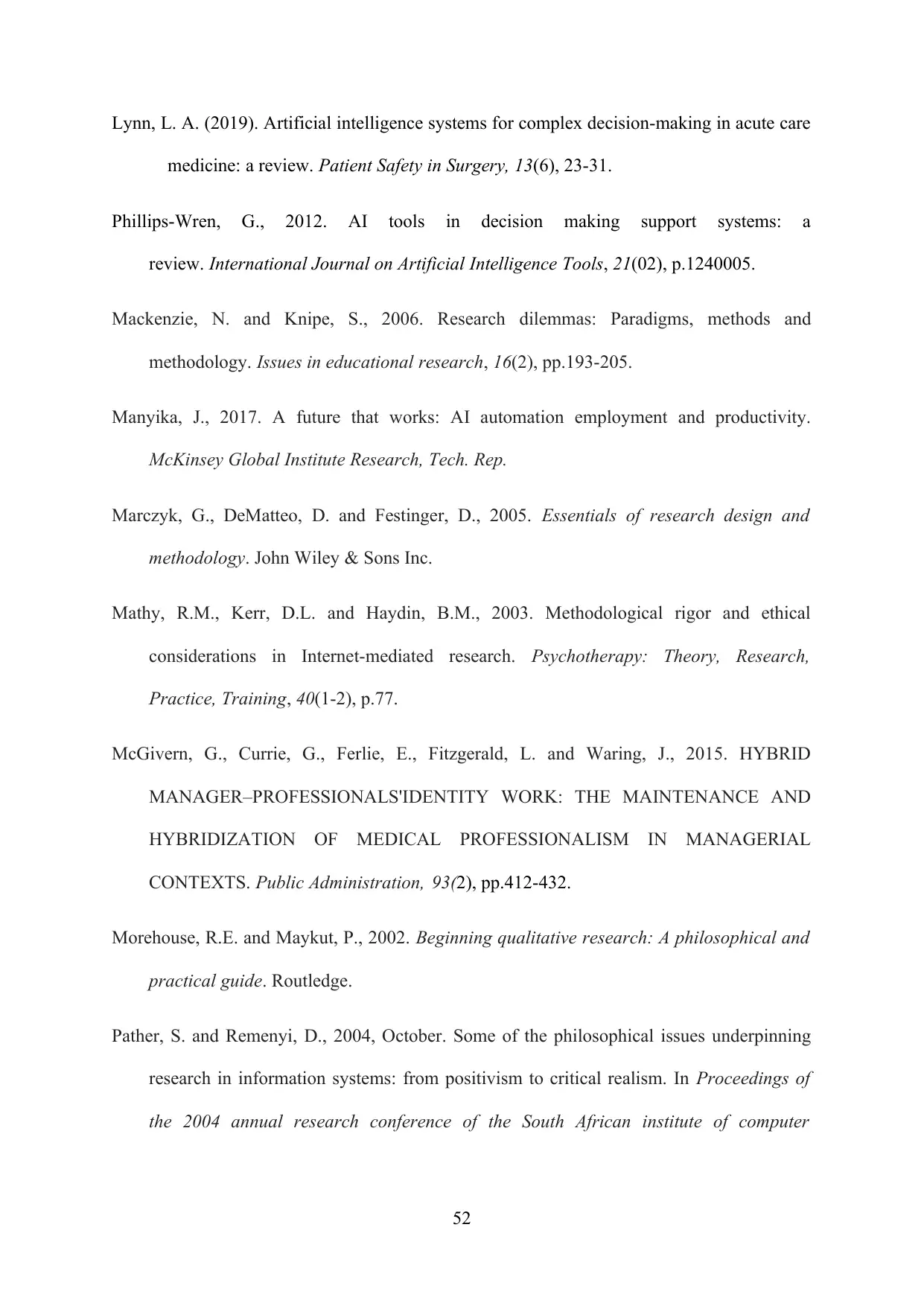
Lynn, L. A. (2019). Artificial intelligence systems for complex decision-making in acute care
medicine: a review. Patient Safety in Surgery, 13(6), 23-31.
Phillips-Wren, G., 2012. AI tools in decision making support systems: a
review. International Journal on Artificial Intelligence Tools, 21(02), p.1240005.
Mackenzie, N. and Knipe, S., 2006. Research dilemmas: Paradigms, methods and
methodology. Issues in educational research, 16(2), pp.193-205.
Manyika, J., 2017. A future that works: AI automation employment and productivity.
McKinsey Global Institute Research, Tech. Rep.
Marczyk, G., DeMatteo, D. and Festinger, D., 2005. Essentials of research design and
methodology. John Wiley & Sons Inc.
Mathy, R.M., Kerr, D.L. and Haydin, B.M., 2003. Methodological rigor and ethical
considerations in Internet-mediated research. Psychotherapy: Theory, Research,
Practice, Training, 40(1-2), p.77.
McGivern, G., Currie, G., Ferlie, E., Fitzgerald, L. and Waring, J., 2015. HYBRID
MANAGER–PROFESSIONALS'IDENTITY WORK: THE MAINTENANCE AND
HYBRIDIZATION OF MEDICAL PROFESSIONALISM IN MANAGERIAL
CONTEXTS. Public Administration, 93(2), pp.412-432.
Morehouse, R.E. and Maykut, P., 2002. Beginning qualitative research: A philosophical and
practical guide. Routledge.
Pather, S. and Remenyi, D., 2004, October. Some of the philosophical issues underpinning
research in information systems: from positivism to critical realism. In Proceedings of
the 2004 annual research conference of the South African institute of computer
52
medicine: a review. Patient Safety in Surgery, 13(6), 23-31.
Phillips-Wren, G., 2012. AI tools in decision making support systems: a
review. International Journal on Artificial Intelligence Tools, 21(02), p.1240005.
Mackenzie, N. and Knipe, S., 2006. Research dilemmas: Paradigms, methods and
methodology. Issues in educational research, 16(2), pp.193-205.
Manyika, J., 2017. A future that works: AI automation employment and productivity.
McKinsey Global Institute Research, Tech. Rep.
Marczyk, G., DeMatteo, D. and Festinger, D., 2005. Essentials of research design and
methodology. John Wiley & Sons Inc.
Mathy, R.M., Kerr, D.L. and Haydin, B.M., 2003. Methodological rigor and ethical
considerations in Internet-mediated research. Psychotherapy: Theory, Research,
Practice, Training, 40(1-2), p.77.
McGivern, G., Currie, G., Ferlie, E., Fitzgerald, L. and Waring, J., 2015. HYBRID
MANAGER–PROFESSIONALS'IDENTITY WORK: THE MAINTENANCE AND
HYBRIDIZATION OF MEDICAL PROFESSIONALISM IN MANAGERIAL
CONTEXTS. Public Administration, 93(2), pp.412-432.
Morehouse, R.E. and Maykut, P., 2002. Beginning qualitative research: A philosophical and
practical guide. Routledge.
Pather, S. and Remenyi, D., 2004, October. Some of the philosophical issues underpinning
research in information systems: from positivism to critical realism. In Proceedings of
the 2004 annual research conference of the South African institute of computer
52
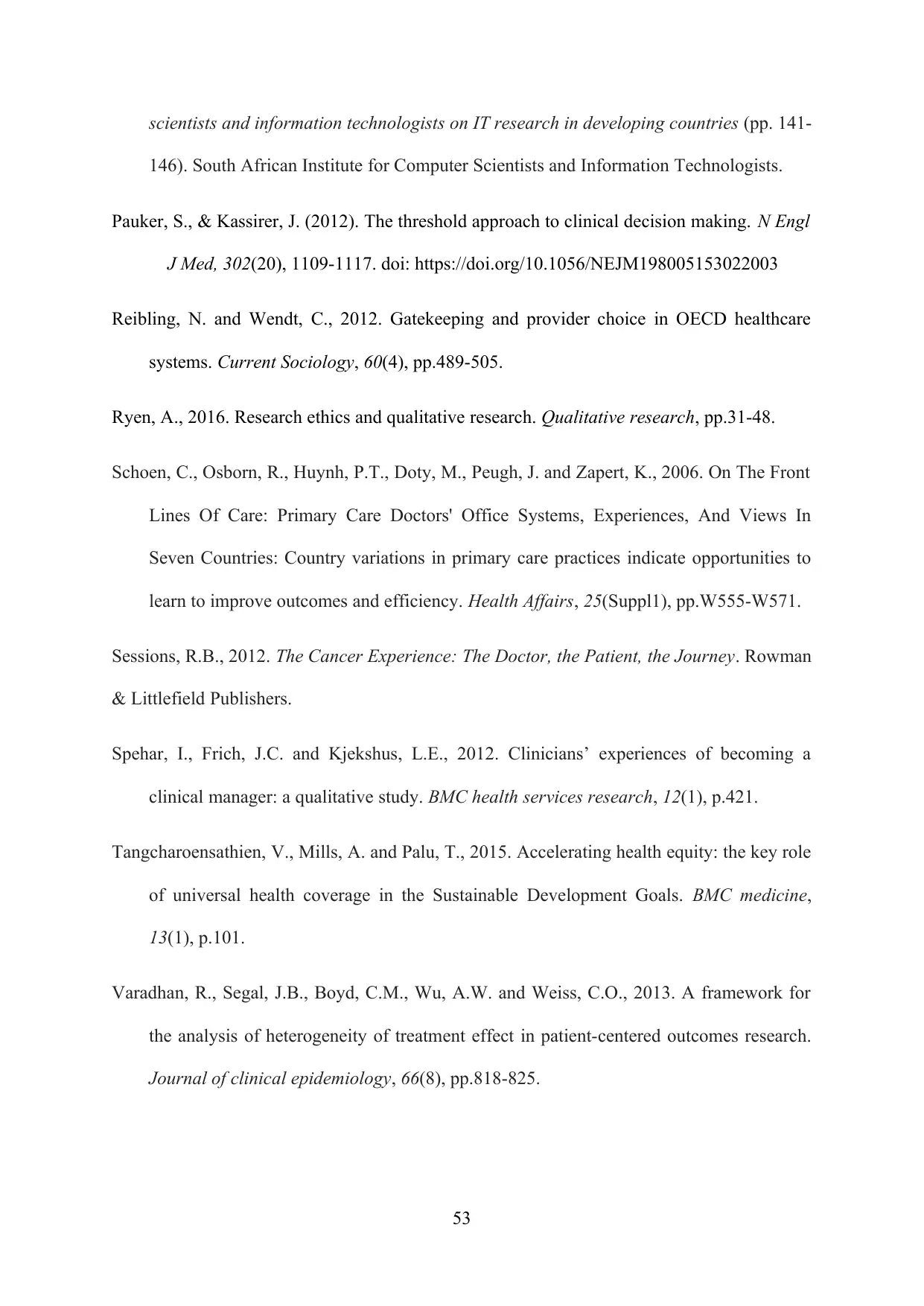
scientists and information technologists on IT research in developing countries (pp. 141-
146). South African Institute for Computer Scientists and Information Technologists.
Pauker, S., & Kassirer, J. (2012). The threshold approach to clinical decision making. N Engl
J Med, 302(20), 1109-1117. doi: https://doi.org/10.1056/NEJM198005153022003
Reibling, N. and Wendt, C., 2012. Gatekeeping and provider choice in OECD healthcare
systems. Current Sociology, 60(4), pp.489-505.
Ryen, A., 2016. Research ethics and qualitative research. Qualitative research, pp.31-48.
Schoen, C., Osborn, R., Huynh, P.T., Doty, M., Peugh, J. and Zapert, K., 2006. On The Front
Lines Of Care: Primary Care Doctors' Office Systems, Experiences, And Views In
Seven Countries: Country variations in primary care practices indicate opportunities to
learn to improve outcomes and efficiency. Health Affairs, 25(Suppl1), pp.W555-W571.
Sessions, R.B., 2012. The Cancer Experience: The Doctor, the Patient, the Journey. Rowman
& Littlefield Publishers.
Spehar, I., Frich, J.C. and Kjekshus, L.E., 2012. Clinicians’ experiences of becoming a
clinical manager: a qualitative study. BMC health services research, 12(1), p.421.
Tangcharoensathien, V., Mills, A. and Palu, T., 2015. Accelerating health equity: the key role
of universal health coverage in the Sustainable Development Goals. BMC medicine,
13(1), p.101.
Varadhan, R., Segal, J.B., Boyd, C.M., Wu, A.W. and Weiss, C.O., 2013. A framework for
the analysis of heterogeneity of treatment effect in patient-centered outcomes research.
Journal of clinical epidemiology, 66(8), pp.818-825.
53
146). South African Institute for Computer Scientists and Information Technologists.
Pauker, S., & Kassirer, J. (2012). The threshold approach to clinical decision making. N Engl
J Med, 302(20), 1109-1117. doi: https://doi.org/10.1056/NEJM198005153022003
Reibling, N. and Wendt, C., 2012. Gatekeeping and provider choice in OECD healthcare
systems. Current Sociology, 60(4), pp.489-505.
Ryen, A., 2016. Research ethics and qualitative research. Qualitative research, pp.31-48.
Schoen, C., Osborn, R., Huynh, P.T., Doty, M., Peugh, J. and Zapert, K., 2006. On The Front
Lines Of Care: Primary Care Doctors' Office Systems, Experiences, And Views In
Seven Countries: Country variations in primary care practices indicate opportunities to
learn to improve outcomes and efficiency. Health Affairs, 25(Suppl1), pp.W555-W571.
Sessions, R.B., 2012. The Cancer Experience: The Doctor, the Patient, the Journey. Rowman
& Littlefield Publishers.
Spehar, I., Frich, J.C. and Kjekshus, L.E., 2012. Clinicians’ experiences of becoming a
clinical manager: a qualitative study. BMC health services research, 12(1), p.421.
Tangcharoensathien, V., Mills, A. and Palu, T., 2015. Accelerating health equity: the key role
of universal health coverage in the Sustainable Development Goals. BMC medicine,
13(1), p.101.
Varadhan, R., Segal, J.B., Boyd, C.M., Wu, A.W. and Weiss, C.O., 2013. A framework for
the analysis of heterogeneity of treatment effect in patient-centered outcomes research.
Journal of clinical epidemiology, 66(8), pp.818-825.
53
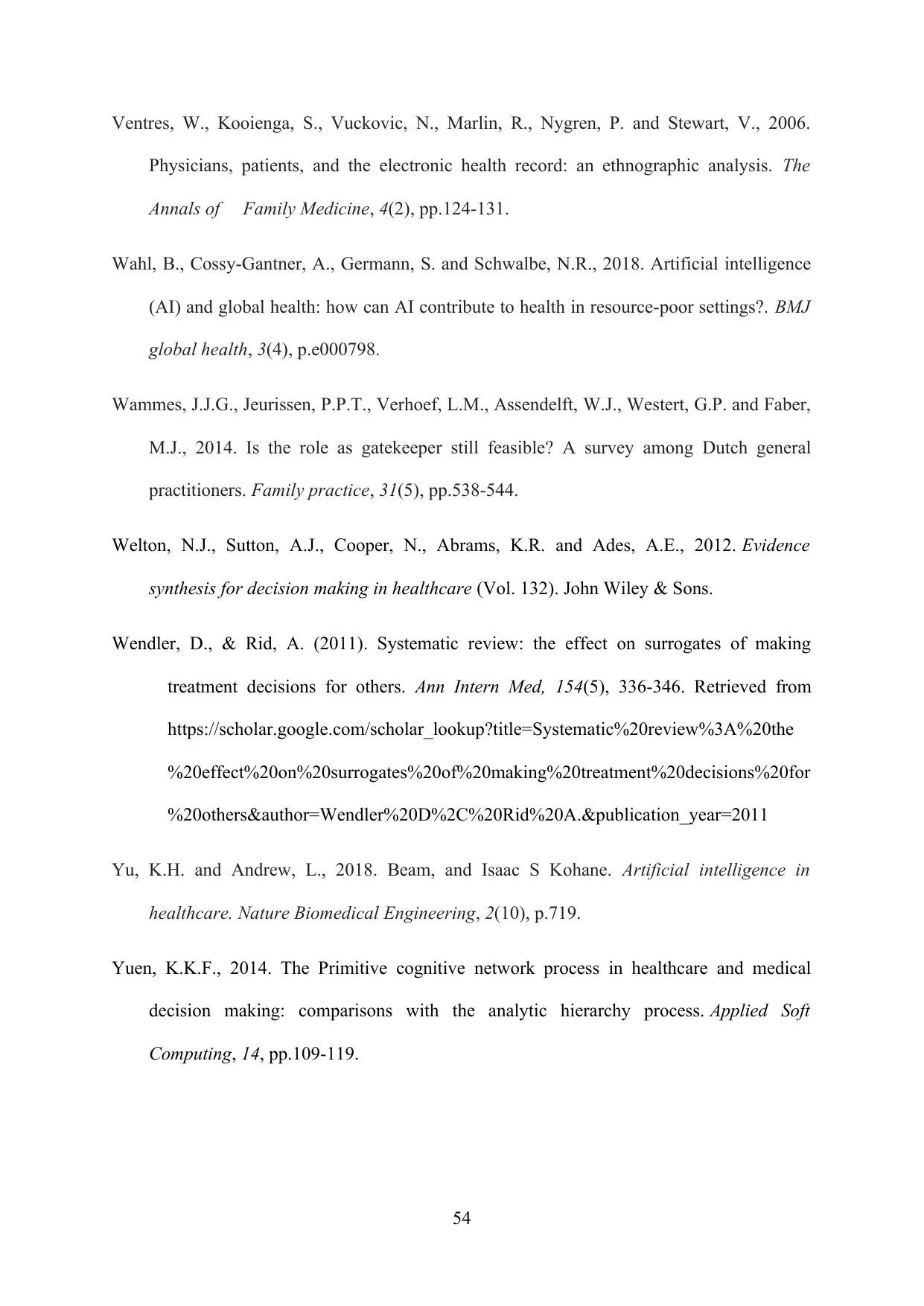
Ventres, W., Kooienga, S., Vuckovic, N., Marlin, R., Nygren, P. and Stewart, V., 2006.
Physicians, patients, and the electronic health record: an ethnographic analysis. The
Annals of Family Medicine, 4(2), pp.124-131.
Wahl, B., Cossy-Gantner, A., Germann, S. and Schwalbe, N.R., 2018. Artificial intelligence
(AI) and global health: how can AI contribute to health in resource-poor settings?. BMJ
global health, 3(4), p.e000798.
Wammes, J.J.G., Jeurissen, P.P.T., Verhoef, L.M., Assendelft, W.J., Westert, G.P. and Faber,
M.J., 2014. Is the role as gatekeeper still feasible? A survey among Dutch general
practitioners. Family practice, 31(5), pp.538-544.
Welton, N.J., Sutton, A.J., Cooper, N., Abrams, K.R. and Ades, A.E., 2012. Evidence
synthesis for decision making in healthcare (Vol. 132). John Wiley & Sons.
Wendler, D., & Rid, A. (2011). Systematic review: the effect on surrogates of making
treatment decisions for others. Ann Intern Med, 154(5), 336-346. Retrieved from
https://scholar.google.com/scholar_lookup?title=Systematic%20review%3A%20the
%20effect%20on%20surrogates%20of%20making%20treatment%20decisions%20for
%20others&author=Wendler%20D%2C%20Rid%20A.&publication_year=2011
Yu, K.H. and Andrew, L., 2018. Beam, and Isaac S Kohane. Artificial intelligence in
healthcare. Nature Biomedical Engineering, 2(10), p.719.
Yuen, K.K.F., 2014. The Primitive cognitive network process in healthcare and medical
decision making: comparisons with the analytic hierarchy process. Applied Soft
Computing, 14, pp.109-119.
54
Physicians, patients, and the electronic health record: an ethnographic analysis. The
Annals of Family Medicine, 4(2), pp.124-131.
Wahl, B., Cossy-Gantner, A., Germann, S. and Schwalbe, N.R., 2018. Artificial intelligence
(AI) and global health: how can AI contribute to health in resource-poor settings?. BMJ
global health, 3(4), p.e000798.
Wammes, J.J.G., Jeurissen, P.P.T., Verhoef, L.M., Assendelft, W.J., Westert, G.P. and Faber,
M.J., 2014. Is the role as gatekeeper still feasible? A survey among Dutch general
practitioners. Family practice, 31(5), pp.538-544.
Welton, N.J., Sutton, A.J., Cooper, N., Abrams, K.R. and Ades, A.E., 2012. Evidence
synthesis for decision making in healthcare (Vol. 132). John Wiley & Sons.
Wendler, D., & Rid, A. (2011). Systematic review: the effect on surrogates of making
treatment decisions for others. Ann Intern Med, 154(5), 336-346. Retrieved from
https://scholar.google.com/scholar_lookup?title=Systematic%20review%3A%20the
%20effect%20on%20surrogates%20of%20making%20treatment%20decisions%20for
%20others&author=Wendler%20D%2C%20Rid%20A.&publication_year=2011
Yu, K.H. and Andrew, L., 2018. Beam, and Isaac S Kohane. Artificial intelligence in
healthcare. Nature Biomedical Engineering, 2(10), p.719.
Yuen, K.K.F., 2014. The Primitive cognitive network process in healthcare and medical
decision making: comparisons with the analytic hierarchy process. Applied Soft
Computing, 14, pp.109-119.
54
Paraphrase This Document
Need a fresh take? Get an instant paraphrase of this document with our AI Paraphraser
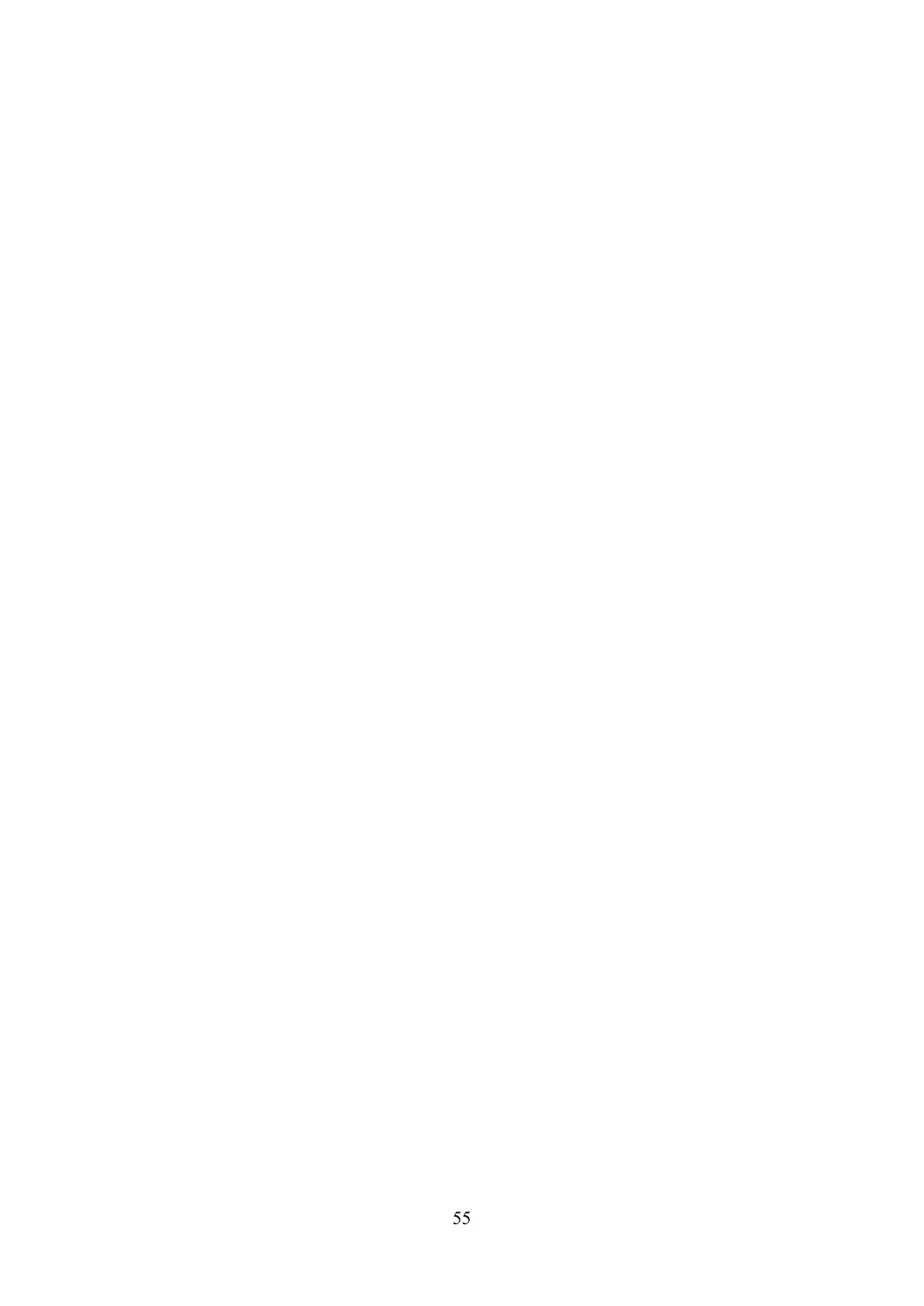
55
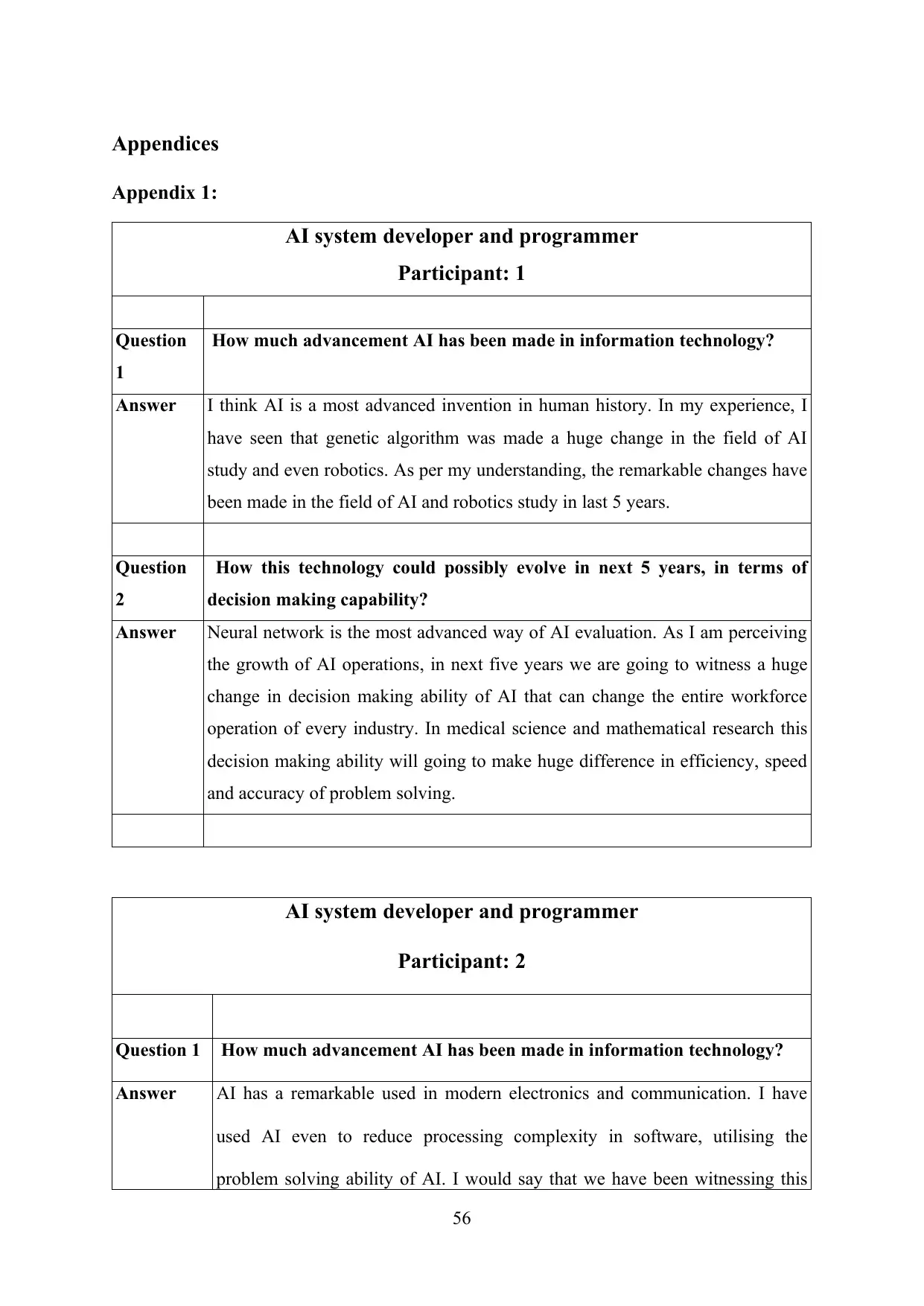
Appendices
Appendix 1:
AI system developer and programmer
Participant: 1
Question
1
How much advancement AI has been made in information technology?
Answer I think AI is a most advanced invention in human history. In my experience, I
have seen that genetic algorithm was made a huge change in the field of AI
study and even robotics. As per my understanding, the remarkable changes have
been made in the field of AI and robotics study in last 5 years.
Question
2
How this technology could possibly evolve in next 5 years, in terms of
decision making capability?
Answer Neural network is the most advanced way of AI evaluation. As I am perceiving
the growth of AI operations, in next five years we are going to witness a huge
change in decision making ability of AI that can change the entire workforce
operation of every industry. In medical science and mathematical research this
decision making ability will going to make huge difference in efficiency, speed
and accuracy of problem solving.
AI system developer and programmer
Participant: 2
Question 1 How much advancement AI has been made in information technology?
Answer AI has a remarkable used in modern electronics and communication. I have
used AI even to reduce processing complexity in software, utilising the
problem solving ability of AI. I would say that we have been witnessing this
56
Appendix 1:
AI system developer and programmer
Participant: 1
Question
1
How much advancement AI has been made in information technology?
Answer I think AI is a most advanced invention in human history. In my experience, I
have seen that genetic algorithm was made a huge change in the field of AI
study and even robotics. As per my understanding, the remarkable changes have
been made in the field of AI and robotics study in last 5 years.
Question
2
How this technology could possibly evolve in next 5 years, in terms of
decision making capability?
Answer Neural network is the most advanced way of AI evaluation. As I am perceiving
the growth of AI operations, in next five years we are going to witness a huge
change in decision making ability of AI that can change the entire workforce
operation of every industry. In medical science and mathematical research this
decision making ability will going to make huge difference in efficiency, speed
and accuracy of problem solving.
AI system developer and programmer
Participant: 2
Question 1 How much advancement AI has been made in information technology?
Answer AI has a remarkable used in modern electronics and communication. I have
used AI even to reduce processing complexity in software, utilising the
problem solving ability of AI. I would say that we have been witnessing this
56
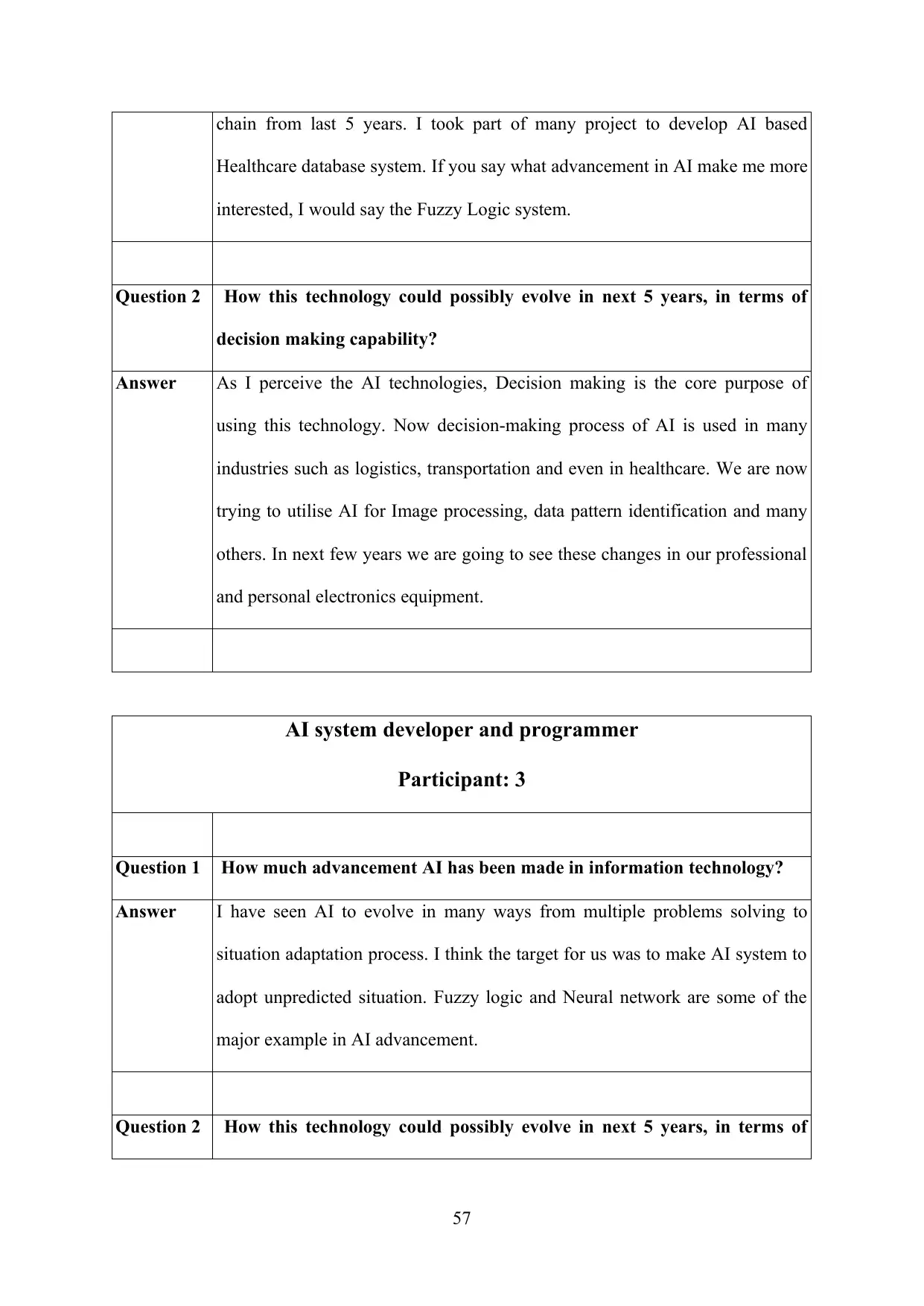
chain from last 5 years. I took part of many project to develop AI based
Healthcare database system. If you say what advancement in AI make me more
interested, I would say the Fuzzy Logic system.
Question 2 How this technology could possibly evolve in next 5 years, in terms of
decision making capability?
Answer As I perceive the AI technologies, Decision making is the core purpose of
using this technology. Now decision-making process of AI is used in many
industries such as logistics, transportation and even in healthcare. We are now
trying to utilise AI for Image processing, data pattern identification and many
others. In next few years we are going to see these changes in our professional
and personal electronics equipment.
AI system developer and programmer
Participant: 3
Question 1 How much advancement AI has been made in information technology?
Answer I have seen AI to evolve in many ways from multiple problems solving to
situation adaptation process. I think the target for us was to make AI system to
adopt unpredicted situation. Fuzzy logic and Neural network are some of the
major example in AI advancement.
Question 2 How this technology could possibly evolve in next 5 years, in terms of
57
Healthcare database system. If you say what advancement in AI make me more
interested, I would say the Fuzzy Logic system.
Question 2 How this technology could possibly evolve in next 5 years, in terms of
decision making capability?
Answer As I perceive the AI technologies, Decision making is the core purpose of
using this technology. Now decision-making process of AI is used in many
industries such as logistics, transportation and even in healthcare. We are now
trying to utilise AI for Image processing, data pattern identification and many
others. In next few years we are going to see these changes in our professional
and personal electronics equipment.
AI system developer and programmer
Participant: 3
Question 1 How much advancement AI has been made in information technology?
Answer I have seen AI to evolve in many ways from multiple problems solving to
situation adaptation process. I think the target for us was to make AI system to
adopt unpredicted situation. Fuzzy logic and Neural network are some of the
major example in AI advancement.
Question 2 How this technology could possibly evolve in next 5 years, in terms of
57
Secure Best Marks with AI Grader
Need help grading? Try our AI Grader for instant feedback on your assignments.
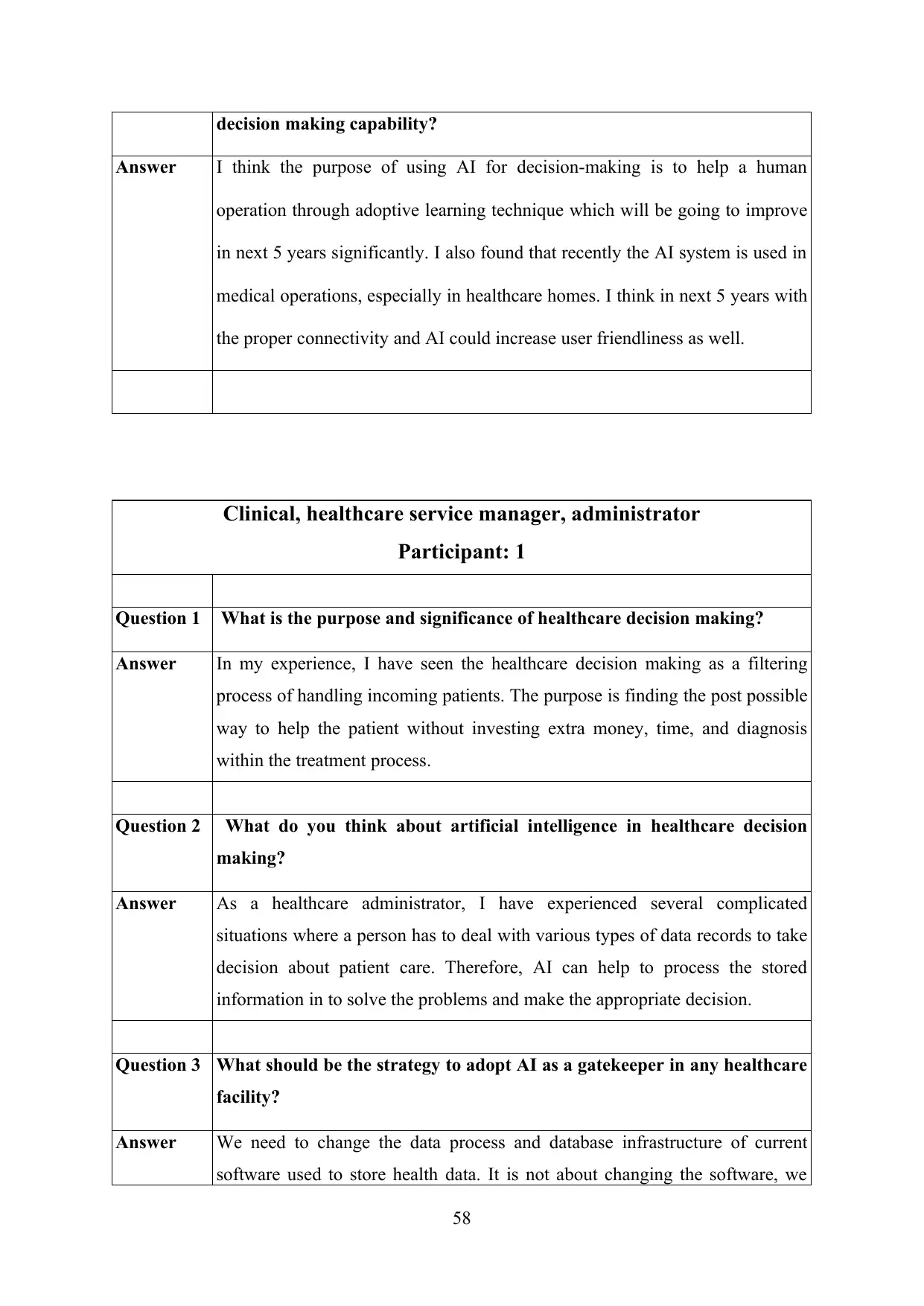
decision making capability?
Answer I think the purpose of using AI for decision-making is to help a human
operation through adoptive learning technique which will be going to improve
in next 5 years significantly. I also found that recently the AI system is used in
medical operations, especially in healthcare homes. I think in next 5 years with
the proper connectivity and AI could increase user friendliness as well.
Clinical, healthcare service manager, administrator
Participant: 1
Question 1 What is the purpose and significance of healthcare decision making?
Answer In my experience, I have seen the healthcare decision making as a filtering
process of handling incoming patients. The purpose is finding the post possible
way to help the patient without investing extra money, time, and diagnosis
within the treatment process.
Question 2 What do you think about artificial intelligence in healthcare decision
making?
Answer As a healthcare administrator, I have experienced several complicated
situations where a person has to deal with various types of data records to take
decision about patient care. Therefore, AI can help to process the stored
information in to solve the problems and make the appropriate decision.
Question 3 What should be the strategy to adopt AI as a gatekeeper in any healthcare
facility?
Answer We need to change the data process and database infrastructure of current
software used to store health data. It is not about changing the software, we
58
Answer I think the purpose of using AI for decision-making is to help a human
operation through adoptive learning technique which will be going to improve
in next 5 years significantly. I also found that recently the AI system is used in
medical operations, especially in healthcare homes. I think in next 5 years with
the proper connectivity and AI could increase user friendliness as well.
Clinical, healthcare service manager, administrator
Participant: 1
Question 1 What is the purpose and significance of healthcare decision making?
Answer In my experience, I have seen the healthcare decision making as a filtering
process of handling incoming patients. The purpose is finding the post possible
way to help the patient without investing extra money, time, and diagnosis
within the treatment process.
Question 2 What do you think about artificial intelligence in healthcare decision
making?
Answer As a healthcare administrator, I have experienced several complicated
situations where a person has to deal with various types of data records to take
decision about patient care. Therefore, AI can help to process the stored
information in to solve the problems and make the appropriate decision.
Question 3 What should be the strategy to adopt AI as a gatekeeper in any healthcare
facility?
Answer We need to change the data process and database infrastructure of current
software used to store health data. It is not about changing the software, we
58
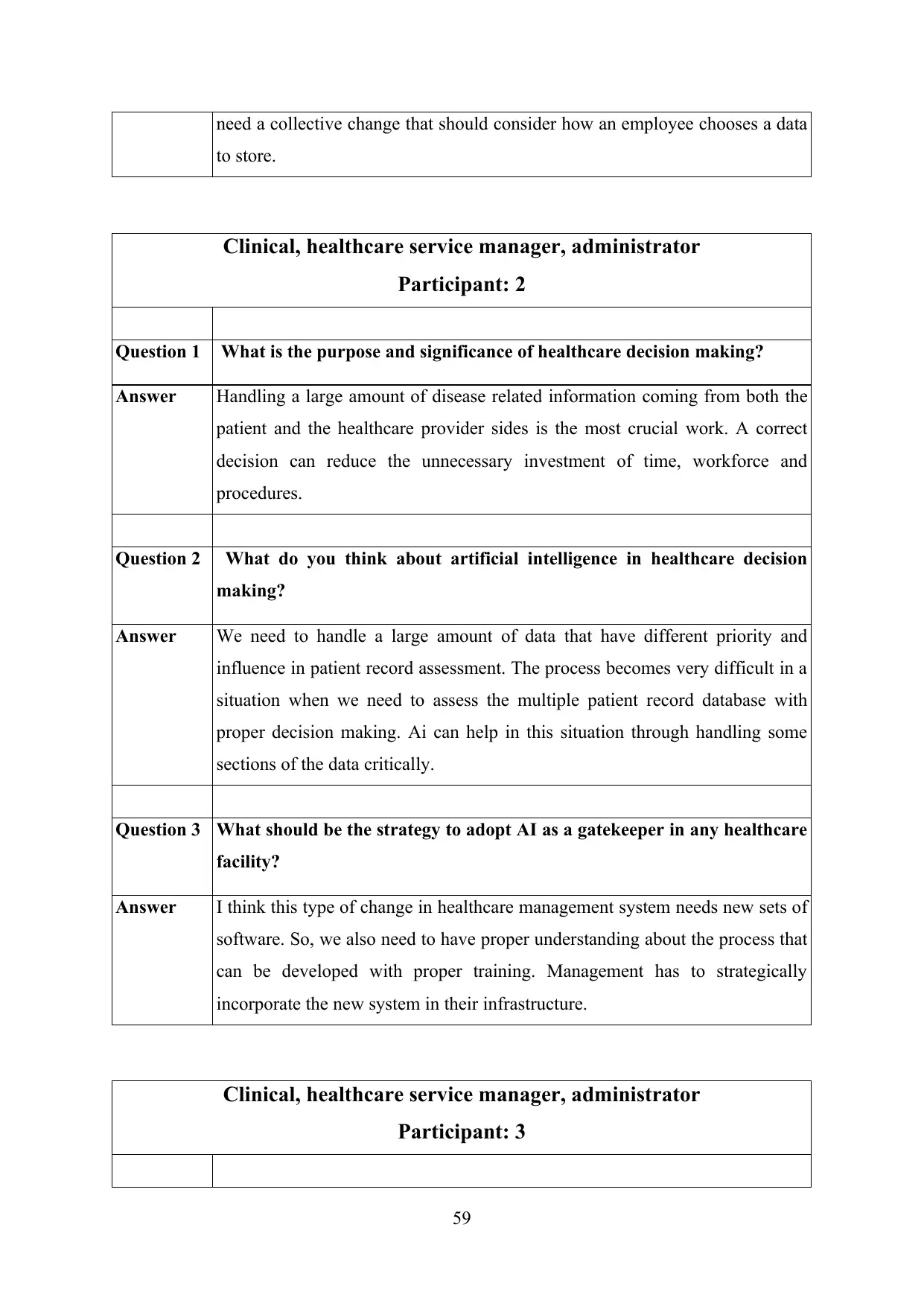
need a collective change that should consider how an employee chooses a data
to store.
Clinical, healthcare service manager, administrator
Participant: 2
Question 1 What is the purpose and significance of healthcare decision making?
Answer Handling a large amount of disease related information coming from both the
patient and the healthcare provider sides is the most crucial work. A correct
decision can reduce the unnecessary investment of time, workforce and
procedures.
Question 2 What do you think about artificial intelligence in healthcare decision
making?
Answer We need to handle a large amount of data that have different priority and
influence in patient record assessment. The process becomes very difficult in a
situation when we need to assess the multiple patient record database with
proper decision making. Ai can help in this situation through handling some
sections of the data critically.
Question 3 What should be the strategy to adopt AI as a gatekeeper in any healthcare
facility?
Answer I think this type of change in healthcare management system needs new sets of
software. So, we also need to have proper understanding about the process that
can be developed with proper training. Management has to strategically
incorporate the new system in their infrastructure.
Clinical, healthcare service manager, administrator
Participant: 3
59
to store.
Clinical, healthcare service manager, administrator
Participant: 2
Question 1 What is the purpose and significance of healthcare decision making?
Answer Handling a large amount of disease related information coming from both the
patient and the healthcare provider sides is the most crucial work. A correct
decision can reduce the unnecessary investment of time, workforce and
procedures.
Question 2 What do you think about artificial intelligence in healthcare decision
making?
Answer We need to handle a large amount of data that have different priority and
influence in patient record assessment. The process becomes very difficult in a
situation when we need to assess the multiple patient record database with
proper decision making. Ai can help in this situation through handling some
sections of the data critically.
Question 3 What should be the strategy to adopt AI as a gatekeeper in any healthcare
facility?
Answer I think this type of change in healthcare management system needs new sets of
software. So, we also need to have proper understanding about the process that
can be developed with proper training. Management has to strategically
incorporate the new system in their infrastructure.
Clinical, healthcare service manager, administrator
Participant: 3
59
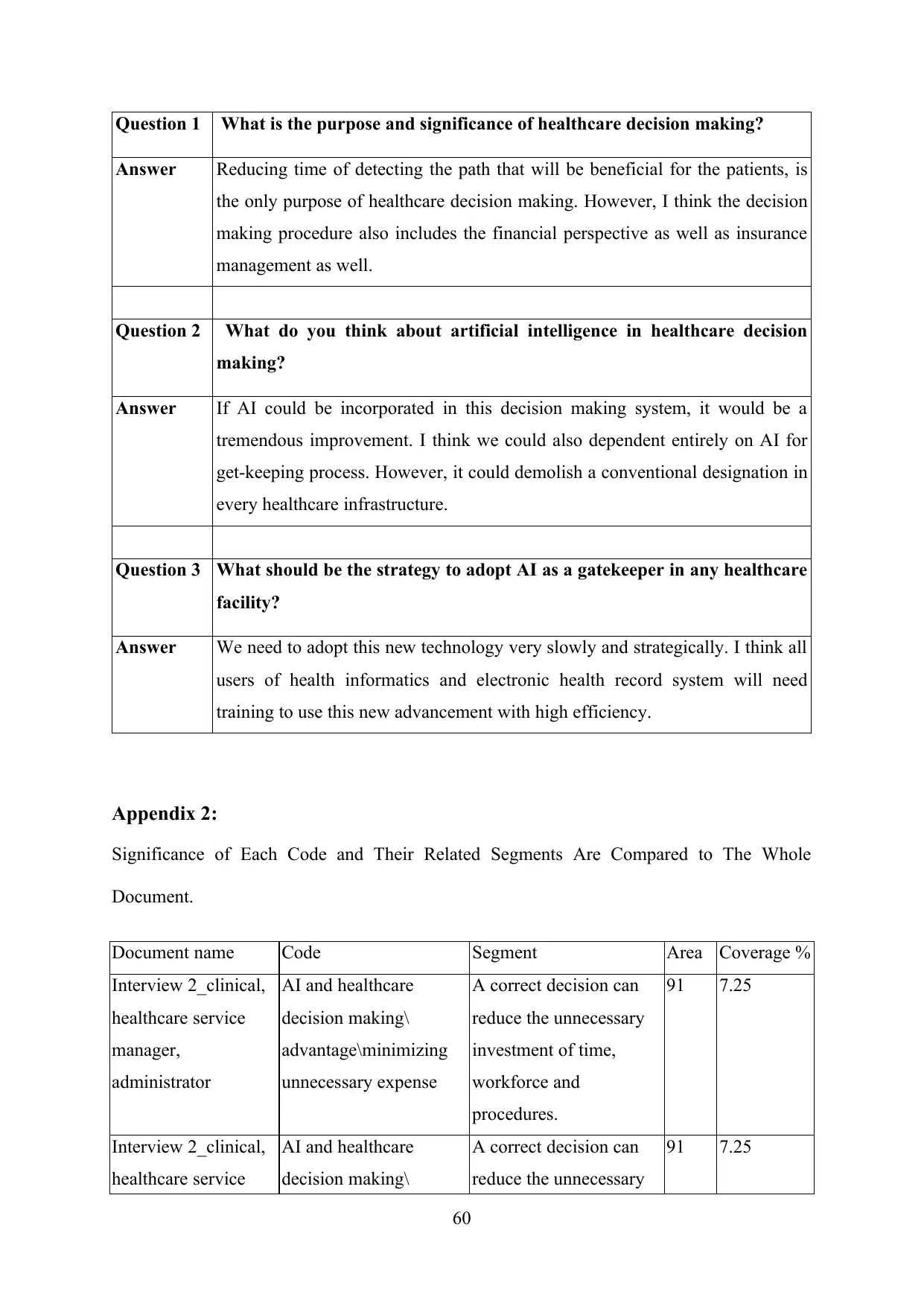
Question 1 What is the purpose and significance of healthcare decision making?
Answer Reducing time of detecting the path that will be beneficial for the patients, is
the only purpose of healthcare decision making. However, I think the decision
making procedure also includes the financial perspective as well as insurance
management as well.
Question 2 What do you think about artificial intelligence in healthcare decision
making?
Answer If AI could be incorporated in this decision making system, it would be a
tremendous improvement. I think we could also dependent entirely on AI for
get-keeping process. However, it could demolish a conventional designation in
every healthcare infrastructure.
Question 3 What should be the strategy to adopt AI as a gatekeeper in any healthcare
facility?
Answer We need to adopt this new technology very slowly and strategically. I think all
users of health informatics and electronic health record system will need
training to use this new advancement with high efficiency.
Appendix 2:
Significance of Each Code and Their Related Segments Are Compared to The Whole
Document.
Document name Code Segment Area Coverage %
Interview 2_clinical,
healthcare service
manager,
administrator
AI and healthcare
decision making\
advantage\minimizing
unnecessary expense
A correct decision can
reduce the unnecessary
investment of time,
workforce and
procedures.
91 7.25
Interview 2_clinical,
healthcare service
AI and healthcare
decision making\
A correct decision can
reduce the unnecessary
91 7.25
60
Answer Reducing time of detecting the path that will be beneficial for the patients, is
the only purpose of healthcare decision making. However, I think the decision
making procedure also includes the financial perspective as well as insurance
management as well.
Question 2 What do you think about artificial intelligence in healthcare decision
making?
Answer If AI could be incorporated in this decision making system, it would be a
tremendous improvement. I think we could also dependent entirely on AI for
get-keeping process. However, it could demolish a conventional designation in
every healthcare infrastructure.
Question 3 What should be the strategy to adopt AI as a gatekeeper in any healthcare
facility?
Answer We need to adopt this new technology very slowly and strategically. I think all
users of health informatics and electronic health record system will need
training to use this new advancement with high efficiency.
Appendix 2:
Significance of Each Code and Their Related Segments Are Compared to The Whole
Document.
Document name Code Segment Area Coverage %
Interview 2_clinical,
healthcare service
manager,
administrator
AI and healthcare
decision making\
advantage\minimizing
unnecessary expense
A correct decision can
reduce the unnecessary
investment of time,
workforce and
procedures.
91 7.25
Interview 2_clinical,
healthcare service
AI and healthcare
decision making\
A correct decision can
reduce the unnecessary
91 7.25
60
Paraphrase This Document
Need a fresh take? Get an instant paraphrase of this document with our AI Paraphraser
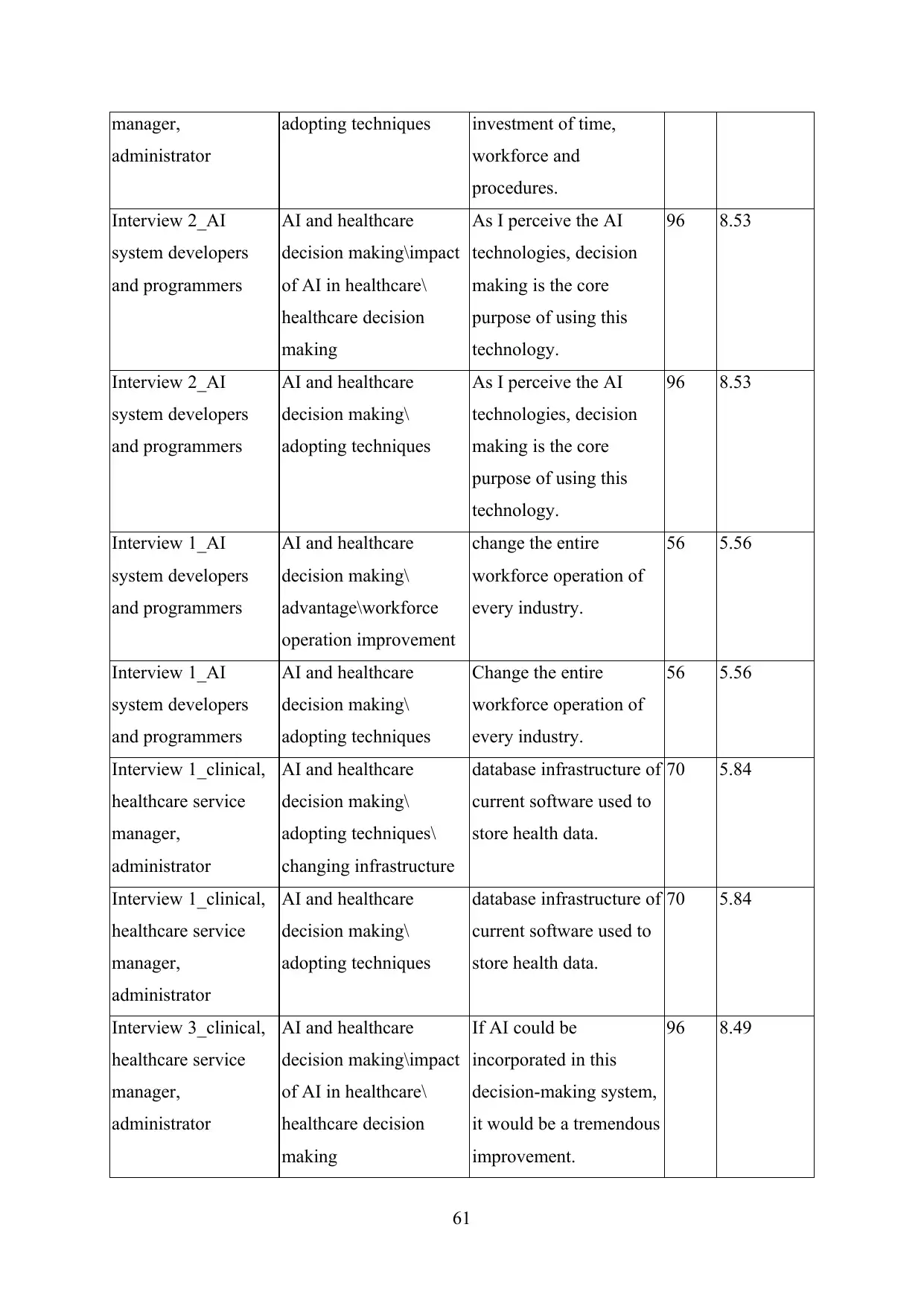
manager,
administrator
adopting techniques investment of time,
workforce and
procedures.
Interview 2_AI
system developers
and programmers
AI and healthcare
decision making\impact
of AI in healthcare\
healthcare decision
making
As I perceive the AI
technologies, decision
making is the core
purpose of using this
technology.
96 8.53
Interview 2_AI
system developers
and programmers
AI and healthcare
decision making\
adopting techniques
As I perceive the AI
technologies, decision
making is the core
purpose of using this
technology.
96 8.53
Interview 1_AI
system developers
and programmers
AI and healthcare
decision making\
advantage\workforce
operation improvement
change the entire
workforce operation of
every industry.
56 5.56
Interview 1_AI
system developers
and programmers
AI and healthcare
decision making\
adopting techniques
Change the entire
workforce operation of
every industry.
56 5.56
Interview 1_clinical,
healthcare service
manager,
administrator
AI and healthcare
decision making\
adopting techniques\
changing infrastructure
database infrastructure of
current software used to
store health data.
70 5.84
Interview 1_clinical,
healthcare service
manager,
administrator
AI and healthcare
decision making\
adopting techniques
database infrastructure of
current software used to
store health data.
70 5.84
Interview 3_clinical,
healthcare service
manager,
administrator
AI and healthcare
decision making\impact
of AI in healthcare\
healthcare decision
making
If AI could be
incorporated in this
decision-making system,
it would be a tremendous
improvement.
96 8.49
61
administrator
adopting techniques investment of time,
workforce and
procedures.
Interview 2_AI
system developers
and programmers
AI and healthcare
decision making\impact
of AI in healthcare\
healthcare decision
making
As I perceive the AI
technologies, decision
making is the core
purpose of using this
technology.
96 8.53
Interview 2_AI
system developers
and programmers
AI and healthcare
decision making\
adopting techniques
As I perceive the AI
technologies, decision
making is the core
purpose of using this
technology.
96 8.53
Interview 1_AI
system developers
and programmers
AI and healthcare
decision making\
advantage\workforce
operation improvement
change the entire
workforce operation of
every industry.
56 5.56
Interview 1_AI
system developers
and programmers
AI and healthcare
decision making\
adopting techniques
Change the entire
workforce operation of
every industry.
56 5.56
Interview 1_clinical,
healthcare service
manager,
administrator
AI and healthcare
decision making\
adopting techniques\
changing infrastructure
database infrastructure of
current software used to
store health data.
70 5.84
Interview 1_clinical,
healthcare service
manager,
administrator
AI and healthcare
decision making\
adopting techniques
database infrastructure of
current software used to
store health data.
70 5.84
Interview 3_clinical,
healthcare service
manager,
administrator
AI and healthcare
decision making\impact
of AI in healthcare\
healthcare decision
making
If AI could be
incorporated in this
decision-making system,
it would be a tremendous
improvement.
96 8.49
61
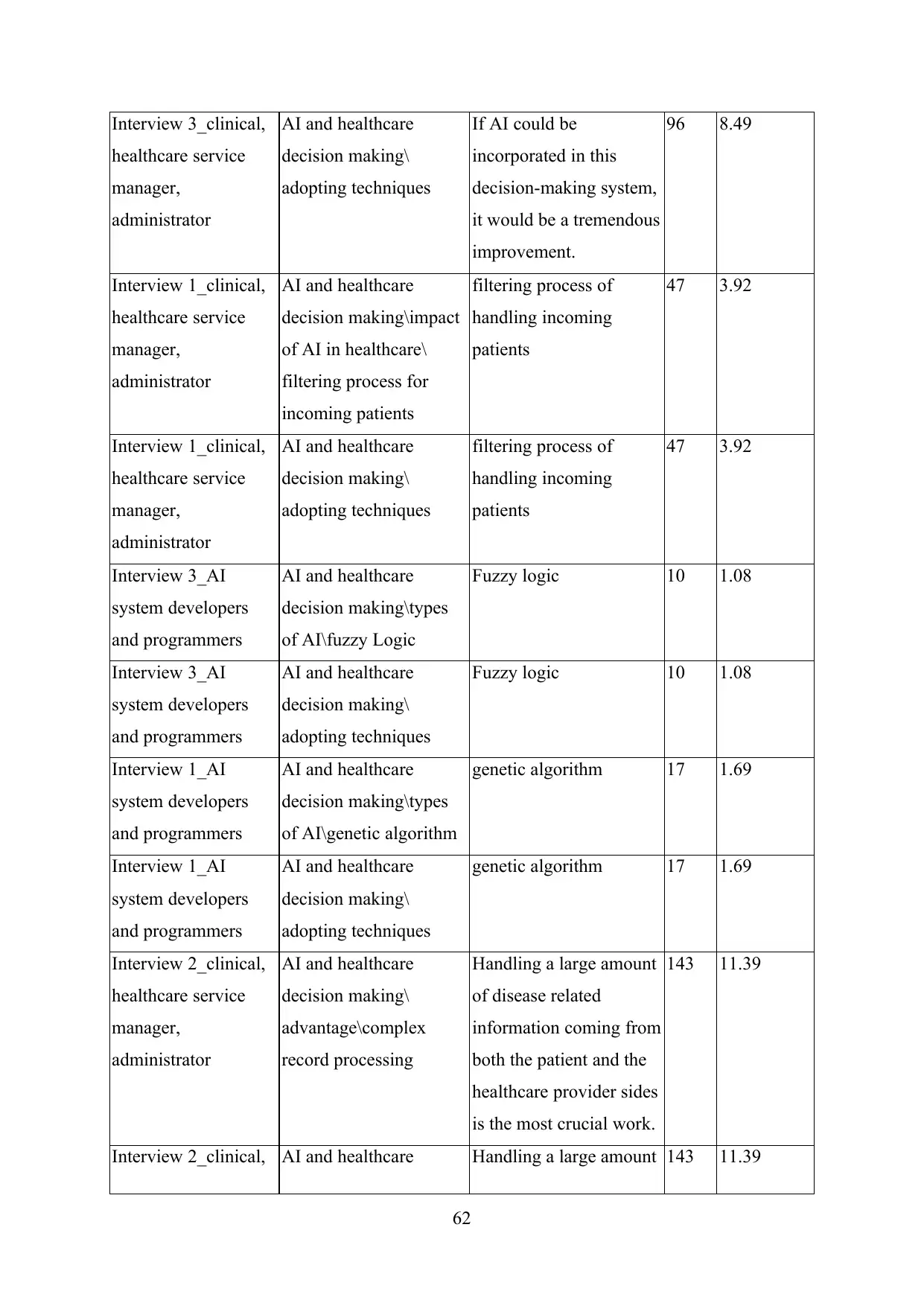
Interview 3_clinical,
healthcare service
manager,
administrator
AI and healthcare
decision making\
adopting techniques
If AI could be
incorporated in this
decision-making system,
it would be a tremendous
improvement.
96 8.49
Interview 1_clinical,
healthcare service
manager,
administrator
AI and healthcare
decision making\impact
of AI in healthcare\
filtering process for
incoming patients
filtering process of
handling incoming
patients
47 3.92
Interview 1_clinical,
healthcare service
manager,
administrator
AI and healthcare
decision making\
adopting techniques
filtering process of
handling incoming
patients
47 3.92
Interview 3_AI
system developers
and programmers
AI and healthcare
decision making\types
of AI\fuzzy Logic
Fuzzy logic 10 1.08
Interview 3_AI
system developers
and programmers
AI and healthcare
decision making\
adopting techniques
Fuzzy logic 10 1.08
Interview 1_AI
system developers
and programmers
AI and healthcare
decision making\types
of AI\genetic algorithm
genetic algorithm 17 1.69
Interview 1_AI
system developers
and programmers
AI and healthcare
decision making\
adopting techniques
genetic algorithm 17 1.69
Interview 2_clinical,
healthcare service
manager,
administrator
AI and healthcare
decision making\
advantage\complex
record processing
Handling a large amount
of disease related
information coming from
both the patient and the
healthcare provider sides
is the most crucial work.
143 11.39
Interview 2_clinical, AI and healthcare Handling a large amount 143 11.39
62
healthcare service
manager,
administrator
AI and healthcare
decision making\
adopting techniques
If AI could be
incorporated in this
decision-making system,
it would be a tremendous
improvement.
96 8.49
Interview 1_clinical,
healthcare service
manager,
administrator
AI and healthcare
decision making\impact
of AI in healthcare\
filtering process for
incoming patients
filtering process of
handling incoming
patients
47 3.92
Interview 1_clinical,
healthcare service
manager,
administrator
AI and healthcare
decision making\
adopting techniques
filtering process of
handling incoming
patients
47 3.92
Interview 3_AI
system developers
and programmers
AI and healthcare
decision making\types
of AI\fuzzy Logic
Fuzzy logic 10 1.08
Interview 3_AI
system developers
and programmers
AI and healthcare
decision making\
adopting techniques
Fuzzy logic 10 1.08
Interview 1_AI
system developers
and programmers
AI and healthcare
decision making\types
of AI\genetic algorithm
genetic algorithm 17 1.69
Interview 1_AI
system developers
and programmers
AI and healthcare
decision making\
adopting techniques
genetic algorithm 17 1.69
Interview 2_clinical,
healthcare service
manager,
administrator
AI and healthcare
decision making\
advantage\complex
record processing
Handling a large amount
of disease related
information coming from
both the patient and the
healthcare provider sides
is the most crucial work.
143 11.39
Interview 2_clinical, AI and healthcare Handling a large amount 143 11.39
62
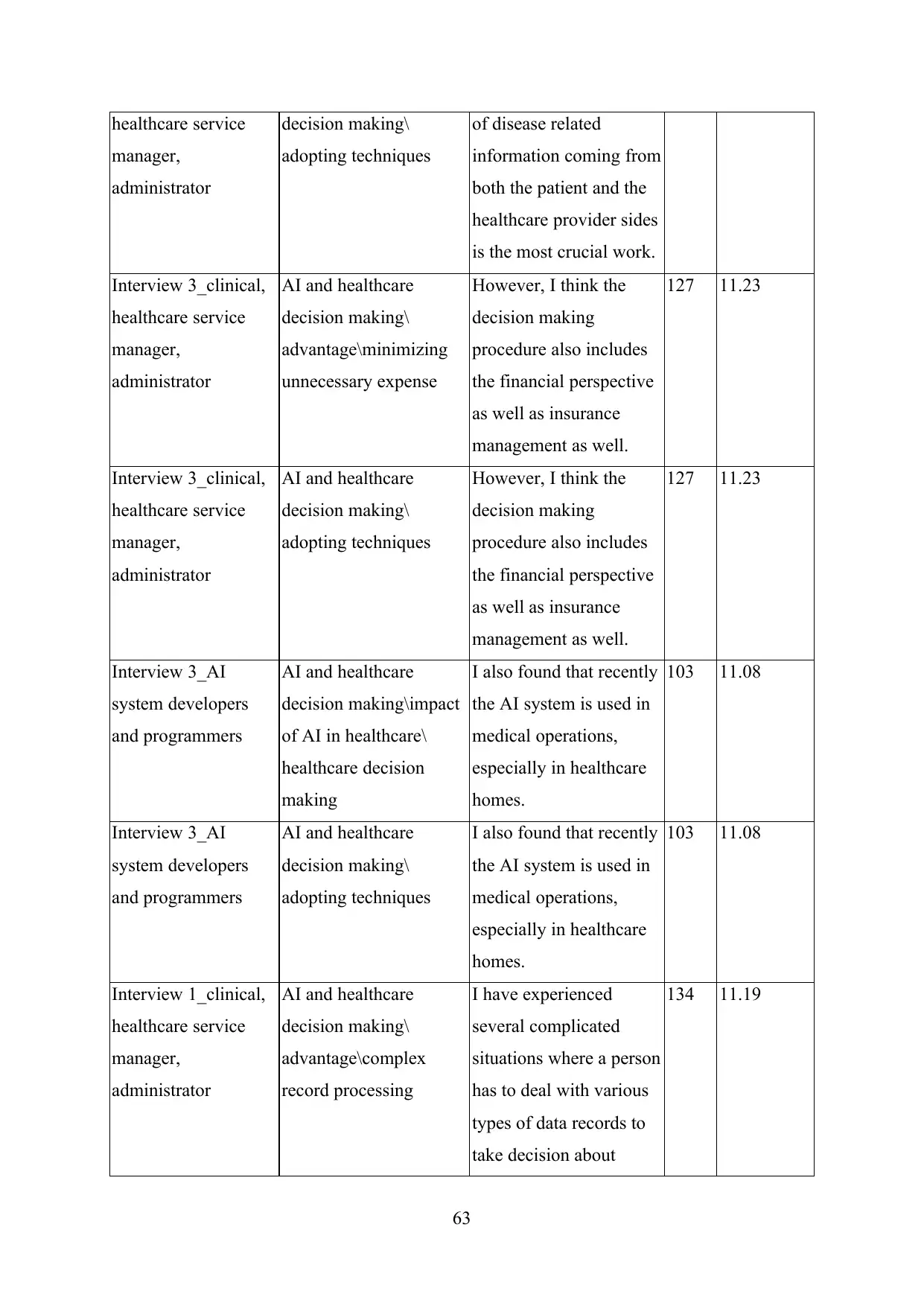
healthcare service
manager,
administrator
decision making\
adopting techniques
of disease related
information coming from
both the patient and the
healthcare provider sides
is the most crucial work.
Interview 3_clinical,
healthcare service
manager,
administrator
AI and healthcare
decision making\
advantage\minimizing
unnecessary expense
However, I think the
decision making
procedure also includes
the financial perspective
as well as insurance
management as well.
127 11.23
Interview 3_clinical,
healthcare service
manager,
administrator
AI and healthcare
decision making\
adopting techniques
However, I think the
decision making
procedure also includes
the financial perspective
as well as insurance
management as well.
127 11.23
Interview 3_AI
system developers
and programmers
AI and healthcare
decision making\impact
of AI in healthcare\
healthcare decision
making
I also found that recently
the AI system is used in
medical operations,
especially in healthcare
homes.
103 11.08
Interview 3_AI
system developers
and programmers
AI and healthcare
decision making\
adopting techniques
I also found that recently
the AI system is used in
medical operations,
especially in healthcare
homes.
103 11.08
Interview 1_clinical,
healthcare service
manager,
administrator
AI and healthcare
decision making\
advantage\complex
record processing
I have experienced
several complicated
situations where a person
has to deal with various
types of data records to
take decision about
134 11.19
63
manager,
administrator
decision making\
adopting techniques
of disease related
information coming from
both the patient and the
healthcare provider sides
is the most crucial work.
Interview 3_clinical,
healthcare service
manager,
administrator
AI and healthcare
decision making\
advantage\minimizing
unnecessary expense
However, I think the
decision making
procedure also includes
the financial perspective
as well as insurance
management as well.
127 11.23
Interview 3_clinical,
healthcare service
manager,
administrator
AI and healthcare
decision making\
adopting techniques
However, I think the
decision making
procedure also includes
the financial perspective
as well as insurance
management as well.
127 11.23
Interview 3_AI
system developers
and programmers
AI and healthcare
decision making\impact
of AI in healthcare\
healthcare decision
making
I also found that recently
the AI system is used in
medical operations,
especially in healthcare
homes.
103 11.08
Interview 3_AI
system developers
and programmers
AI and healthcare
decision making\
adopting techniques
I also found that recently
the AI system is used in
medical operations,
especially in healthcare
homes.
103 11.08
Interview 1_clinical,
healthcare service
manager,
administrator
AI and healthcare
decision making\
advantage\complex
record processing
I have experienced
several complicated
situations where a person
has to deal with various
types of data records to
take decision about
134 11.19
63
Secure Best Marks with AI Grader
Need help grading? Try our AI Grader for instant feedback on your assignments.
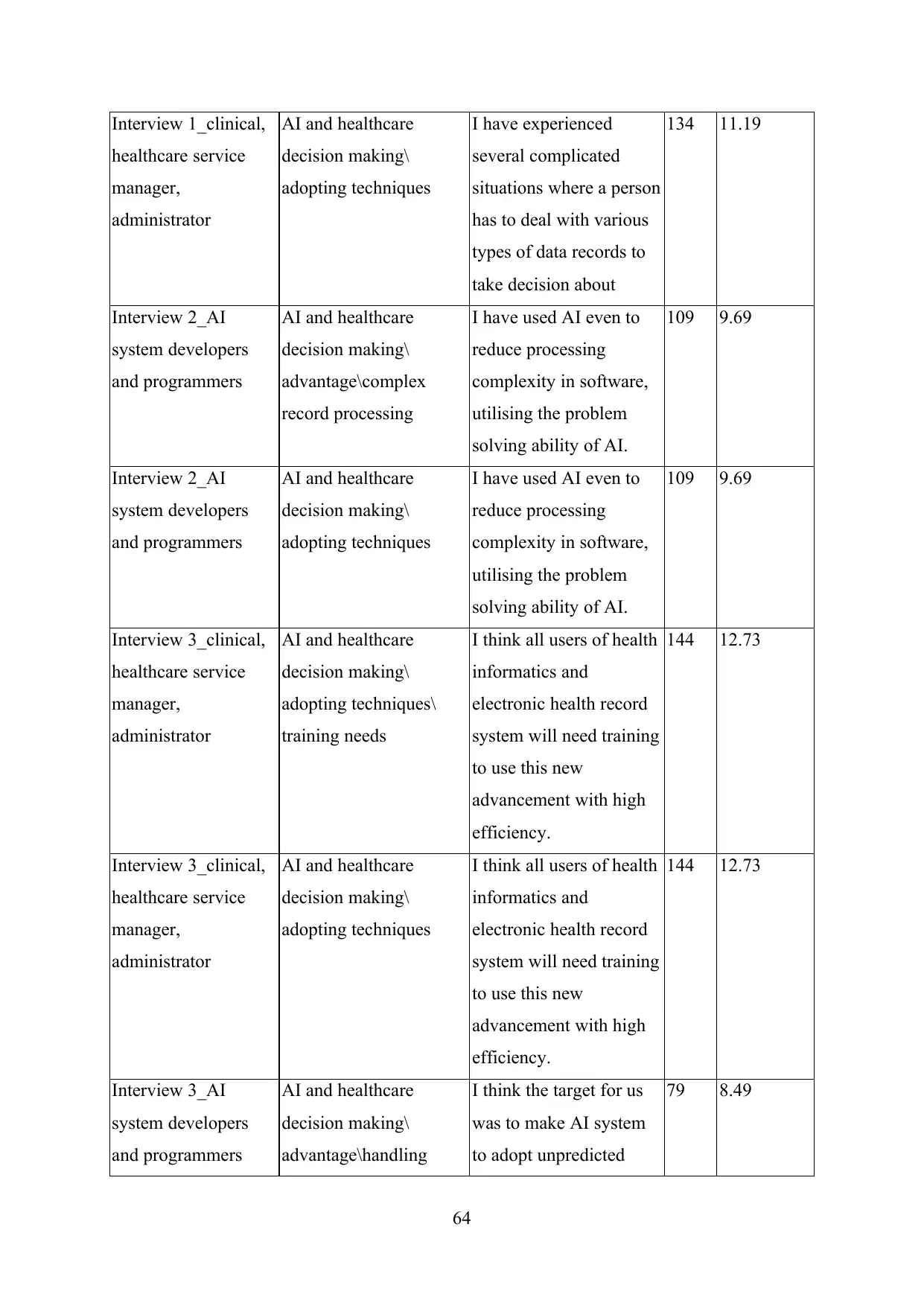
Interview 1_clinical,
healthcare service
manager,
administrator
AI and healthcare
decision making\
adopting techniques
I have experienced
several complicated
situations where a person
has to deal with various
types of data records to
take decision about
134 11.19
Interview 2_AI
system developers
and programmers
AI and healthcare
decision making\
advantage\complex
record processing
I have used AI even to
reduce processing
complexity in software,
utilising the problem
solving ability of AI.
109 9.69
Interview 2_AI
system developers
and programmers
AI and healthcare
decision making\
adopting techniques
I have used AI even to
reduce processing
complexity in software,
utilising the problem
solving ability of AI.
109 9.69
Interview 3_clinical,
healthcare service
manager,
administrator
AI and healthcare
decision making\
adopting techniques\
training needs
I think all users of health
informatics and
electronic health record
system will need training
to use this new
advancement with high
efficiency.
144 12.73
Interview 3_clinical,
healthcare service
manager,
administrator
AI and healthcare
decision making\
adopting techniques
I think all users of health
informatics and
electronic health record
system will need training
to use this new
advancement with high
efficiency.
144 12.73
Interview 3_AI
system developers
and programmers
AI and healthcare
decision making\
advantage\handling
I think the target for us
was to make AI system
to adopt unpredicted
79 8.49
64
healthcare service
manager,
administrator
AI and healthcare
decision making\
adopting techniques
I have experienced
several complicated
situations where a person
has to deal with various
types of data records to
take decision about
134 11.19
Interview 2_AI
system developers
and programmers
AI and healthcare
decision making\
advantage\complex
record processing
I have used AI even to
reduce processing
complexity in software,
utilising the problem
solving ability of AI.
109 9.69
Interview 2_AI
system developers
and programmers
AI and healthcare
decision making\
adopting techniques
I have used AI even to
reduce processing
complexity in software,
utilising the problem
solving ability of AI.
109 9.69
Interview 3_clinical,
healthcare service
manager,
administrator
AI and healthcare
decision making\
adopting techniques\
training needs
I think all users of health
informatics and
electronic health record
system will need training
to use this new
advancement with high
efficiency.
144 12.73
Interview 3_clinical,
healthcare service
manager,
administrator
AI and healthcare
decision making\
adopting techniques
I think all users of health
informatics and
electronic health record
system will need training
to use this new
advancement with high
efficiency.
144 12.73
Interview 3_AI
system developers
and programmers
AI and healthcare
decision making\
advantage\handling
I think the target for us
was to make AI system
to adopt unpredicted
79 8.49
64
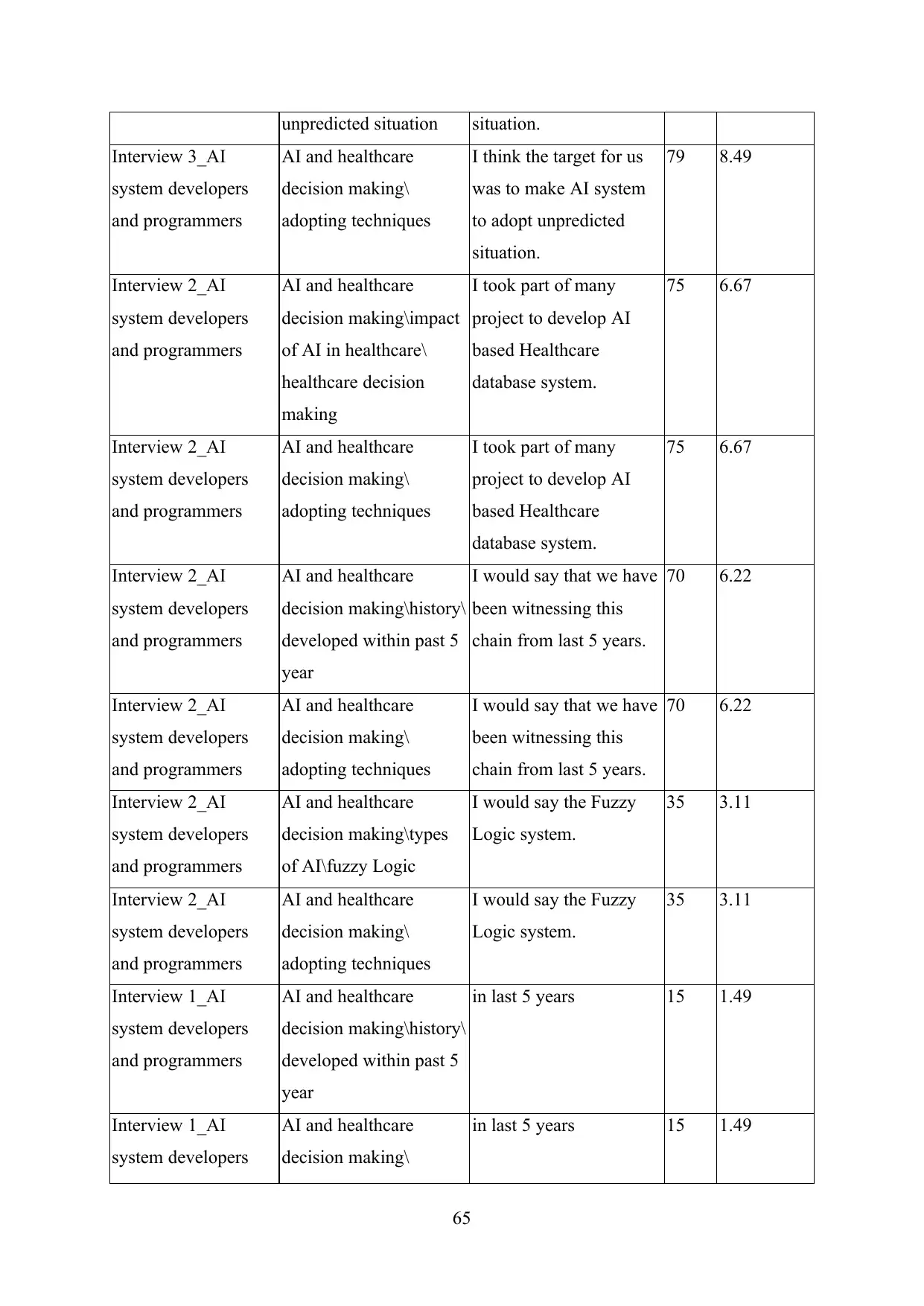
unpredicted situation situation.
Interview 3_AI
system developers
and programmers
AI and healthcare
decision making\
adopting techniques
I think the target for us
was to make AI system
to adopt unpredicted
situation.
79 8.49
Interview 2_AI
system developers
and programmers
AI and healthcare
decision making\impact
of AI in healthcare\
healthcare decision
making
I took part of many
project to develop AI
based Healthcare
database system.
75 6.67
Interview 2_AI
system developers
and programmers
AI and healthcare
decision making\
adopting techniques
I took part of many
project to develop AI
based Healthcare
database system.
75 6.67
Interview 2_AI
system developers
and programmers
AI and healthcare
decision making\history\
developed within past 5
year
I would say that we have
been witnessing this
chain from last 5 years.
70 6.22
Interview 2_AI
system developers
and programmers
AI and healthcare
decision making\
adopting techniques
I would say that we have
been witnessing this
chain from last 5 years.
70 6.22
Interview 2_AI
system developers
and programmers
AI and healthcare
decision making\types
of AI\fuzzy Logic
I would say the Fuzzy
Logic system.
35 3.11
Interview 2_AI
system developers
and programmers
AI and healthcare
decision making\
adopting techniques
I would say the Fuzzy
Logic system.
35 3.11
Interview 1_AI
system developers
and programmers
AI and healthcare
decision making\history\
developed within past 5
year
in last 5 years 15 1.49
Interview 1_AI
system developers
AI and healthcare
decision making\
in last 5 years 15 1.49
65
Interview 3_AI
system developers
and programmers
AI and healthcare
decision making\
adopting techniques
I think the target for us
was to make AI system
to adopt unpredicted
situation.
79 8.49
Interview 2_AI
system developers
and programmers
AI and healthcare
decision making\impact
of AI in healthcare\
healthcare decision
making
I took part of many
project to develop AI
based Healthcare
database system.
75 6.67
Interview 2_AI
system developers
and programmers
AI and healthcare
decision making\
adopting techniques
I took part of many
project to develop AI
based Healthcare
database system.
75 6.67
Interview 2_AI
system developers
and programmers
AI and healthcare
decision making\history\
developed within past 5
year
I would say that we have
been witnessing this
chain from last 5 years.
70 6.22
Interview 2_AI
system developers
and programmers
AI and healthcare
decision making\
adopting techniques
I would say that we have
been witnessing this
chain from last 5 years.
70 6.22
Interview 2_AI
system developers
and programmers
AI and healthcare
decision making\types
of AI\fuzzy Logic
I would say the Fuzzy
Logic system.
35 3.11
Interview 2_AI
system developers
and programmers
AI and healthcare
decision making\
adopting techniques
I would say the Fuzzy
Logic system.
35 3.11
Interview 1_AI
system developers
and programmers
AI and healthcare
decision making\history\
developed within past 5
year
in last 5 years 15 1.49
Interview 1_AI
system developers
AI and healthcare
decision making\
in last 5 years 15 1.49
65
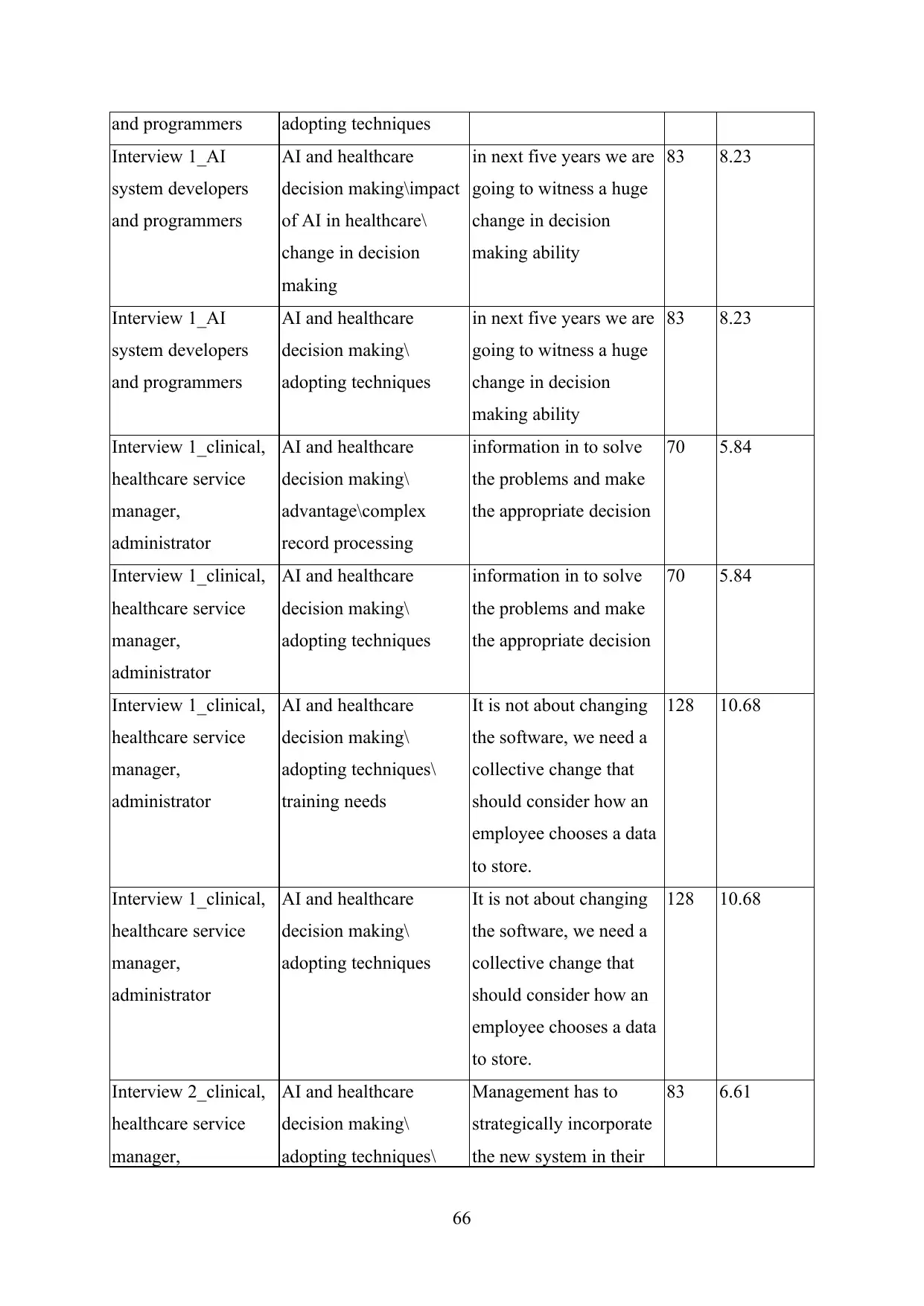
and programmers adopting techniques
Interview 1_AI
system developers
and programmers
AI and healthcare
decision making\impact
of AI in healthcare\
change in decision
making
in next five years we are
going to witness a huge
change in decision
making ability
83 8.23
Interview 1_AI
system developers
and programmers
AI and healthcare
decision making\
adopting techniques
in next five years we are
going to witness a huge
change in decision
making ability
83 8.23
Interview 1_clinical,
healthcare service
manager,
administrator
AI and healthcare
decision making\
advantage\complex
record processing
information in to solve
the problems and make
the appropriate decision
70 5.84
Interview 1_clinical,
healthcare service
manager,
administrator
AI and healthcare
decision making\
adopting techniques
information in to solve
the problems and make
the appropriate decision
70 5.84
Interview 1_clinical,
healthcare service
manager,
administrator
AI and healthcare
decision making\
adopting techniques\
training needs
It is not about changing
the software, we need a
collective change that
should consider how an
employee chooses a data
to store.
128 10.68
Interview 1_clinical,
healthcare service
manager,
administrator
AI and healthcare
decision making\
adopting techniques
It is not about changing
the software, we need a
collective change that
should consider how an
employee chooses a data
to store.
128 10.68
Interview 2_clinical,
healthcare service
manager,
AI and healthcare
decision making\
adopting techniques\
Management has to
strategically incorporate
the new system in their
83 6.61
66
Interview 1_AI
system developers
and programmers
AI and healthcare
decision making\impact
of AI in healthcare\
change in decision
making
in next five years we are
going to witness a huge
change in decision
making ability
83 8.23
Interview 1_AI
system developers
and programmers
AI and healthcare
decision making\
adopting techniques
in next five years we are
going to witness a huge
change in decision
making ability
83 8.23
Interview 1_clinical,
healthcare service
manager,
administrator
AI and healthcare
decision making\
advantage\complex
record processing
information in to solve
the problems and make
the appropriate decision
70 5.84
Interview 1_clinical,
healthcare service
manager,
administrator
AI and healthcare
decision making\
adopting techniques
information in to solve
the problems and make
the appropriate decision
70 5.84
Interview 1_clinical,
healthcare service
manager,
administrator
AI and healthcare
decision making\
adopting techniques\
training needs
It is not about changing
the software, we need a
collective change that
should consider how an
employee chooses a data
to store.
128 10.68
Interview 1_clinical,
healthcare service
manager,
administrator
AI and healthcare
decision making\
adopting techniques
It is not about changing
the software, we need a
collective change that
should consider how an
employee chooses a data
to store.
128 10.68
Interview 2_clinical,
healthcare service
manager,
AI and healthcare
decision making\
adopting techniques\
Management has to
strategically incorporate
the new system in their
83 6.61
66
Paraphrase This Document
Need a fresh take? Get an instant paraphrase of this document with our AI Paraphraser
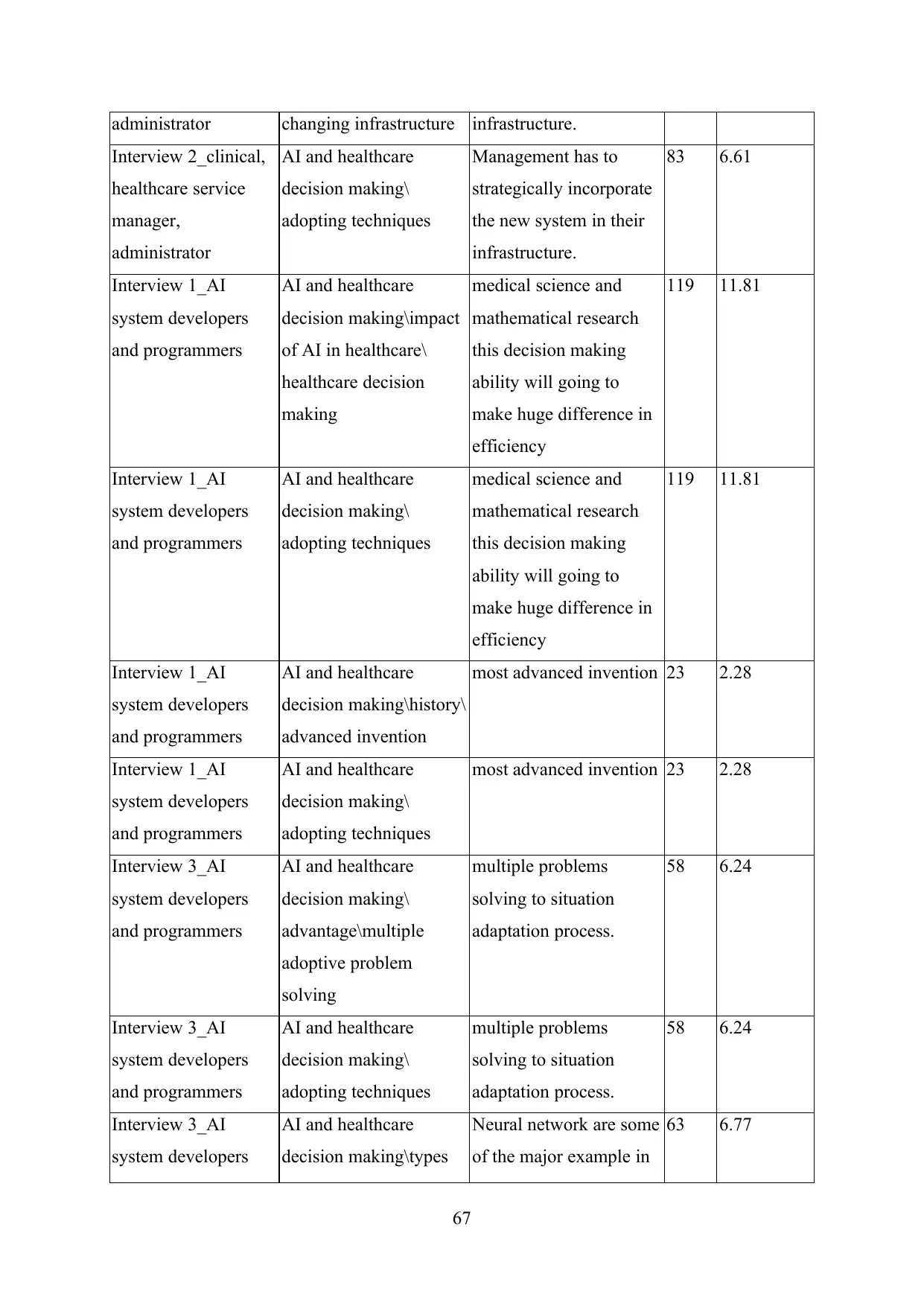
administrator changing infrastructure infrastructure.
Interview 2_clinical,
healthcare service
manager,
administrator
AI and healthcare
decision making\
adopting techniques
Management has to
strategically incorporate
the new system in their
infrastructure.
83 6.61
Interview 1_AI
system developers
and programmers
AI and healthcare
decision making\impact
of AI in healthcare\
healthcare decision
making
medical science and
mathematical research
this decision making
ability will going to
make huge difference in
efficiency
119 11.81
Interview 1_AI
system developers
and programmers
AI and healthcare
decision making\
adopting techniques
medical science and
mathematical research
this decision making
ability will going to
make huge difference in
efficiency
119 11.81
Interview 1_AI
system developers
and programmers
AI and healthcare
decision making\history\
advanced invention
most advanced invention 23 2.28
Interview 1_AI
system developers
and programmers
AI and healthcare
decision making\
adopting techniques
most advanced invention 23 2.28
Interview 3_AI
system developers
and programmers
AI and healthcare
decision making\
advantage\multiple
adoptive problem
solving
multiple problems
solving to situation
adaptation process.
58 6.24
Interview 3_AI
system developers
and programmers
AI and healthcare
decision making\
adopting techniques
multiple problems
solving to situation
adaptation process.
58 6.24
Interview 3_AI
system developers
AI and healthcare
decision making\types
Neural network are some
of the major example in
63 6.77
67
Interview 2_clinical,
healthcare service
manager,
administrator
AI and healthcare
decision making\
adopting techniques
Management has to
strategically incorporate
the new system in their
infrastructure.
83 6.61
Interview 1_AI
system developers
and programmers
AI and healthcare
decision making\impact
of AI in healthcare\
healthcare decision
making
medical science and
mathematical research
this decision making
ability will going to
make huge difference in
efficiency
119 11.81
Interview 1_AI
system developers
and programmers
AI and healthcare
decision making\
adopting techniques
medical science and
mathematical research
this decision making
ability will going to
make huge difference in
efficiency
119 11.81
Interview 1_AI
system developers
and programmers
AI and healthcare
decision making\history\
advanced invention
most advanced invention 23 2.28
Interview 1_AI
system developers
and programmers
AI and healthcare
decision making\
adopting techniques
most advanced invention 23 2.28
Interview 3_AI
system developers
and programmers
AI and healthcare
decision making\
advantage\multiple
adoptive problem
solving
multiple problems
solving to situation
adaptation process.
58 6.24
Interview 3_AI
system developers
and programmers
AI and healthcare
decision making\
adopting techniques
multiple problems
solving to situation
adaptation process.
58 6.24
Interview 3_AI
system developers
AI and healthcare
decision making\types
Neural network are some
of the major example in
63 6.77
67
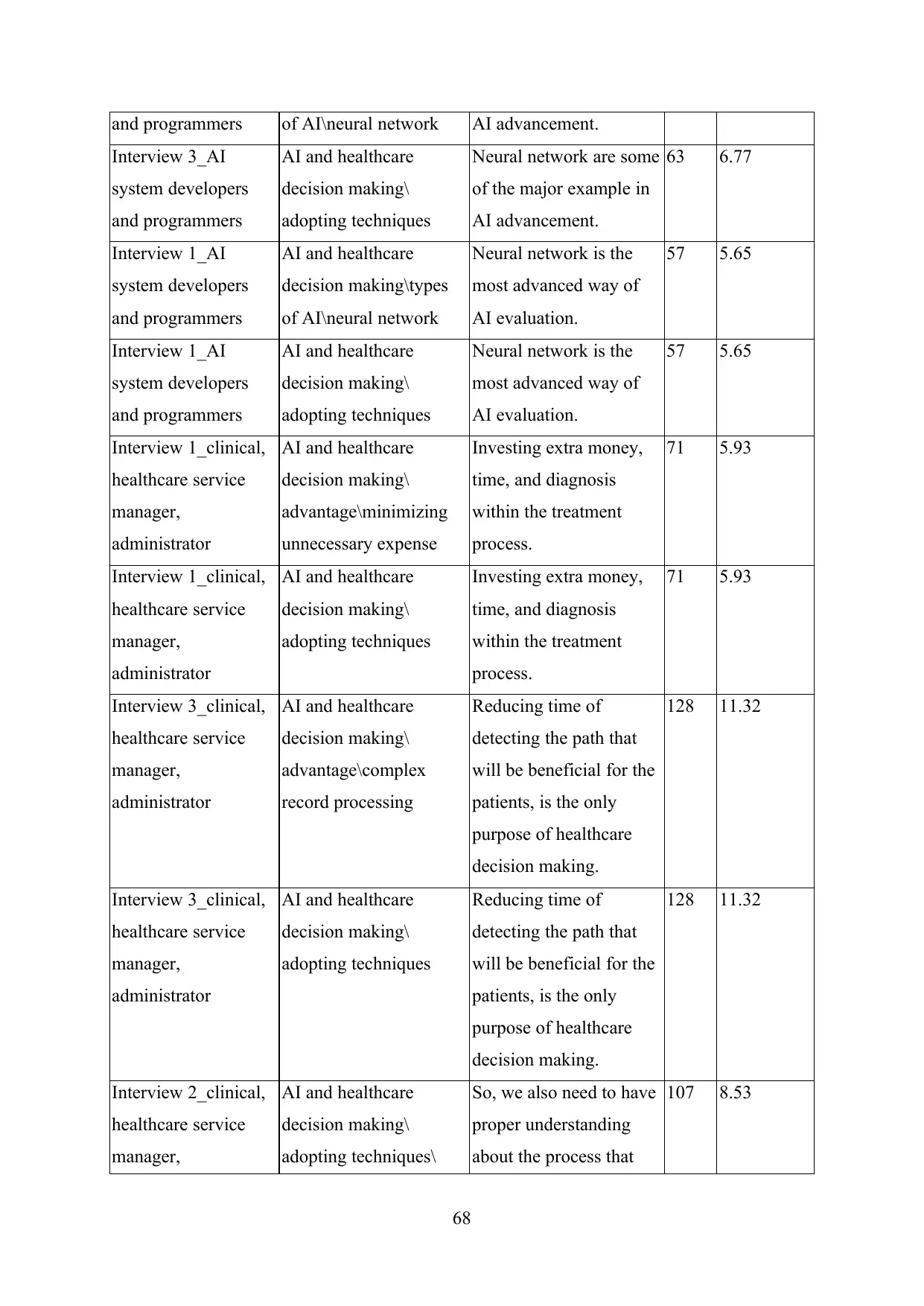
and programmers of AI\neural network AI advancement.
Interview 3_AI
system developers
and programmers
AI and healthcare
decision making\
adopting techniques
Neural network are some
of the major example in
AI advancement.
63 6.77
Interview 1_AI
system developers
and programmers
AI and healthcare
decision making\types
of AI\neural network
Neural network is the
most advanced way of
AI evaluation.
57 5.65
Interview 1_AI
system developers
and programmers
AI and healthcare
decision making\
adopting techniques
Neural network is the
most advanced way of
AI evaluation.
57 5.65
Interview 1_clinical,
healthcare service
manager,
administrator
AI and healthcare
decision making\
advantage\minimizing
unnecessary expense
Investing extra money,
time, and diagnosis
within the treatment
process.
71 5.93
Interview 1_clinical,
healthcare service
manager,
administrator
AI and healthcare
decision making\
adopting techniques
Investing extra money,
time, and diagnosis
within the treatment
process.
71 5.93
Interview 3_clinical,
healthcare service
manager,
administrator
AI and healthcare
decision making\
advantage\complex
record processing
Reducing time of
detecting the path that
will be beneficial for the
patients, is the only
purpose of healthcare
decision making.
128 11.32
Interview 3_clinical,
healthcare service
manager,
administrator
AI and healthcare
decision making\
adopting techniques
Reducing time of
detecting the path that
will be beneficial for the
patients, is the only
purpose of healthcare
decision making.
128 11.32
Interview 2_clinical,
healthcare service
manager,
AI and healthcare
decision making\
adopting techniques\
So, we also need to have
proper understanding
about the process that
107 8.53
68
Interview 3_AI
system developers
and programmers
AI and healthcare
decision making\
adopting techniques
Neural network are some
of the major example in
AI advancement.
63 6.77
Interview 1_AI
system developers
and programmers
AI and healthcare
decision making\types
of AI\neural network
Neural network is the
most advanced way of
AI evaluation.
57 5.65
Interview 1_AI
system developers
and programmers
AI and healthcare
decision making\
adopting techniques
Neural network is the
most advanced way of
AI evaluation.
57 5.65
Interview 1_clinical,
healthcare service
manager,
administrator
AI and healthcare
decision making\
advantage\minimizing
unnecessary expense
Investing extra money,
time, and diagnosis
within the treatment
process.
71 5.93
Interview 1_clinical,
healthcare service
manager,
administrator
AI and healthcare
decision making\
adopting techniques
Investing extra money,
time, and diagnosis
within the treatment
process.
71 5.93
Interview 3_clinical,
healthcare service
manager,
administrator
AI and healthcare
decision making\
advantage\complex
record processing
Reducing time of
detecting the path that
will be beneficial for the
patients, is the only
purpose of healthcare
decision making.
128 11.32
Interview 3_clinical,
healthcare service
manager,
administrator
AI and healthcare
decision making\
adopting techniques
Reducing time of
detecting the path that
will be beneficial for the
patients, is the only
purpose of healthcare
decision making.
128 11.32
Interview 2_clinical,
healthcare service
manager,
AI and healthcare
decision making\
adopting techniques\
So, we also need to have
proper understanding
about the process that
107 8.53
68
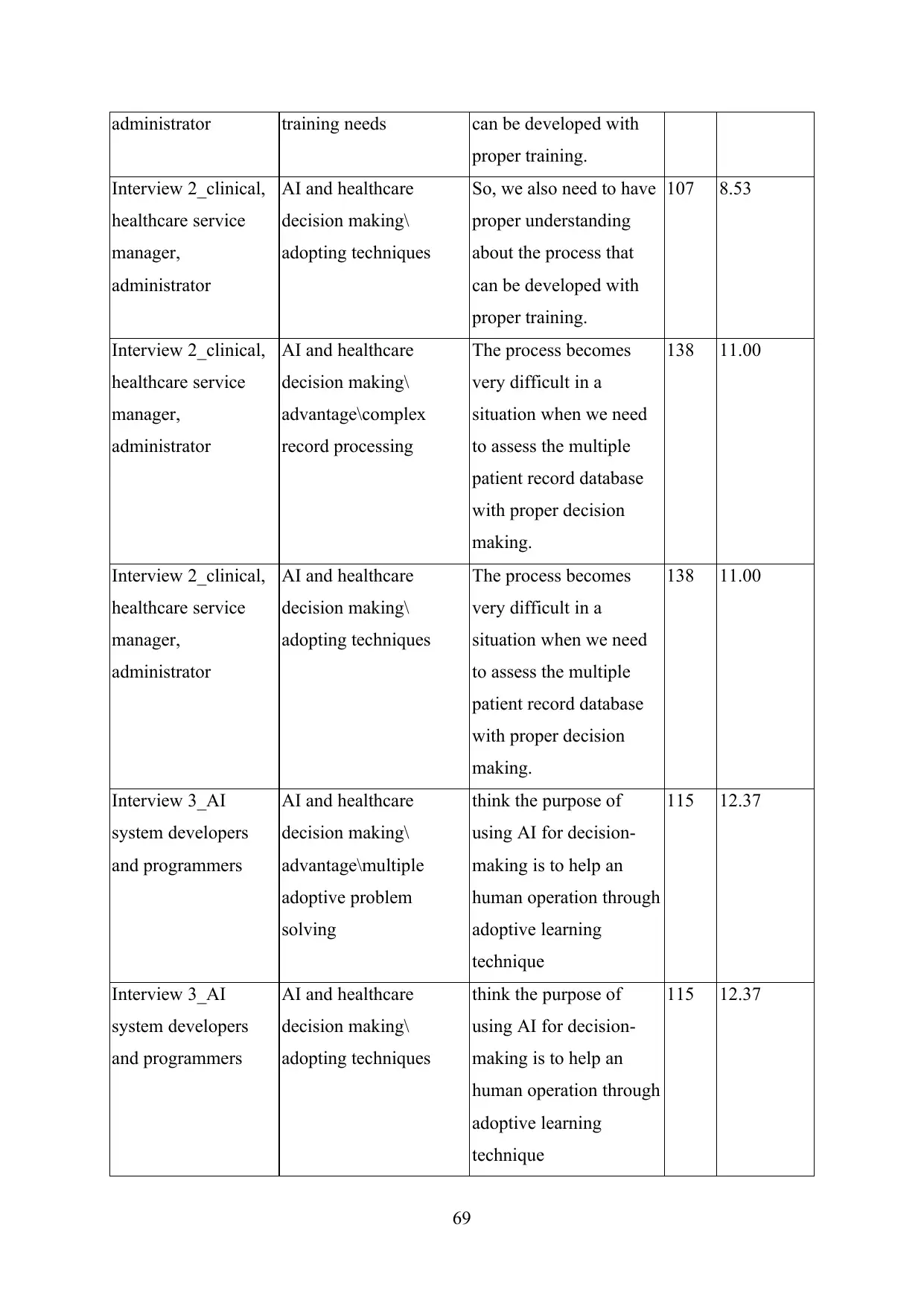
administrator training needs can be developed with
proper training.
Interview 2_clinical,
healthcare service
manager,
administrator
AI and healthcare
decision making\
adopting techniques
So, we also need to have
proper understanding
about the process that
can be developed with
proper training.
107 8.53
Interview 2_clinical,
healthcare service
manager,
administrator
AI and healthcare
decision making\
advantage\complex
record processing
The process becomes
very difficult in a
situation when we need
to assess the multiple
patient record database
with proper decision
making.
138 11.00
Interview 2_clinical,
healthcare service
manager,
administrator
AI and healthcare
decision making\
adopting techniques
The process becomes
very difficult in a
situation when we need
to assess the multiple
patient record database
with proper decision
making.
138 11.00
Interview 3_AI
system developers
and programmers
AI and healthcare
decision making\
advantage\multiple
adoptive problem
solving
think the purpose of
using AI for decision-
making is to help an
human operation through
adoptive learning
technique
115 12.37
Interview 3_AI
system developers
and programmers
AI and healthcare
decision making\
adopting techniques
think the purpose of
using AI for decision-
making is to help an
human operation through
adoptive learning
technique
115 12.37
69
proper training.
Interview 2_clinical,
healthcare service
manager,
administrator
AI and healthcare
decision making\
adopting techniques
So, we also need to have
proper understanding
about the process that
can be developed with
proper training.
107 8.53
Interview 2_clinical,
healthcare service
manager,
administrator
AI and healthcare
decision making\
advantage\complex
record processing
The process becomes
very difficult in a
situation when we need
to assess the multiple
patient record database
with proper decision
making.
138 11.00
Interview 2_clinical,
healthcare service
manager,
administrator
AI and healthcare
decision making\
adopting techniques
The process becomes
very difficult in a
situation when we need
to assess the multiple
patient record database
with proper decision
making.
138 11.00
Interview 3_AI
system developers
and programmers
AI and healthcare
decision making\
advantage\multiple
adoptive problem
solving
think the purpose of
using AI for decision-
making is to help an
human operation through
adoptive learning
technique
115 12.37
Interview 3_AI
system developers
and programmers
AI and healthcare
decision making\
adopting techniques
think the purpose of
using AI for decision-
making is to help an
human operation through
adoptive learning
technique
115 12.37
69
Secure Best Marks with AI Grader
Need help grading? Try our AI Grader for instant feedback on your assignments.
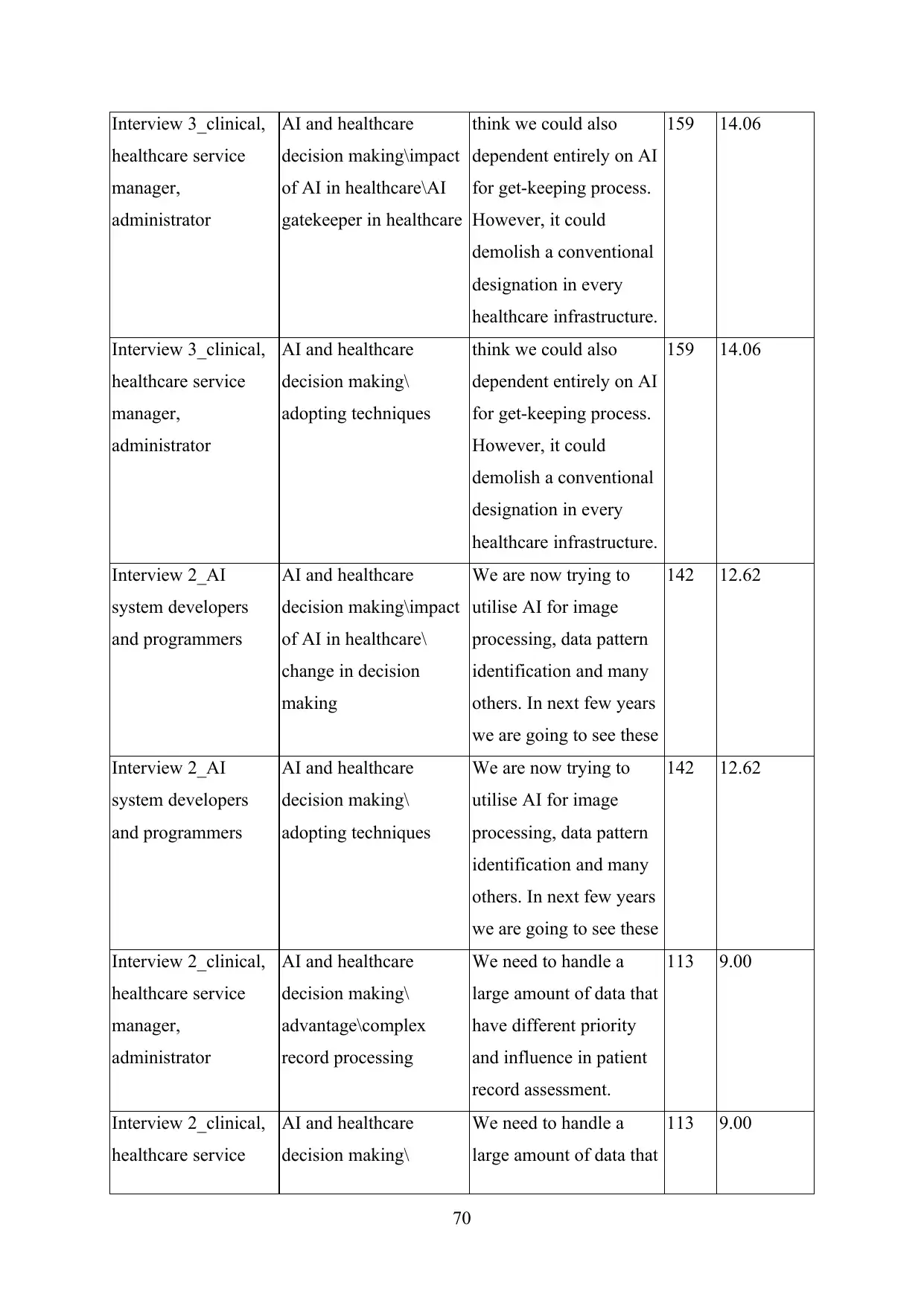
Interview 3_clinical,
healthcare service
manager,
administrator
AI and healthcare
decision making\impact
of AI in healthcare\AI
gatekeeper in healthcare
think we could also
dependent entirely on AI
for get-keeping process.
However, it could
demolish a conventional
designation in every
healthcare infrastructure.
159 14.06
Interview 3_clinical,
healthcare service
manager,
administrator
AI and healthcare
decision making\
adopting techniques
think we could also
dependent entirely on AI
for get-keeping process.
However, it could
demolish a conventional
designation in every
healthcare infrastructure.
159 14.06
Interview 2_AI
system developers
and programmers
AI and healthcare
decision making\impact
of AI in healthcare\
change in decision
making
We are now trying to
utilise AI for image
processing, data pattern
identification and many
others. In next few years
we are going to see these
142 12.62
Interview 2_AI
system developers
and programmers
AI and healthcare
decision making\
adopting techniques
We are now trying to
utilise AI for image
processing, data pattern
identification and many
others. In next few years
we are going to see these
142 12.62
Interview 2_clinical,
healthcare service
manager,
administrator
AI and healthcare
decision making\
advantage\complex
record processing
We need to handle a
large amount of data that
have different priority
and influence in patient
record assessment.
113 9.00
Interview 2_clinical,
healthcare service
AI and healthcare
decision making\
We need to handle a
large amount of data that
113 9.00
70
healthcare service
manager,
administrator
AI and healthcare
decision making\impact
of AI in healthcare\AI
gatekeeper in healthcare
think we could also
dependent entirely on AI
for get-keeping process.
However, it could
demolish a conventional
designation in every
healthcare infrastructure.
159 14.06
Interview 3_clinical,
healthcare service
manager,
administrator
AI and healthcare
decision making\
adopting techniques
think we could also
dependent entirely on AI
for get-keeping process.
However, it could
demolish a conventional
designation in every
healthcare infrastructure.
159 14.06
Interview 2_AI
system developers
and programmers
AI and healthcare
decision making\impact
of AI in healthcare\
change in decision
making
We are now trying to
utilise AI for image
processing, data pattern
identification and many
others. In next few years
we are going to see these
142 12.62
Interview 2_AI
system developers
and programmers
AI and healthcare
decision making\
adopting techniques
We are now trying to
utilise AI for image
processing, data pattern
identification and many
others. In next few years
we are going to see these
142 12.62
Interview 2_clinical,
healthcare service
manager,
administrator
AI and healthcare
decision making\
advantage\complex
record processing
We need to handle a
large amount of data that
have different priority
and influence in patient
record assessment.
113 9.00
Interview 2_clinical,
healthcare service
AI and healthcare
decision making\
We need to handle a
large amount of data that
113 9.00
70
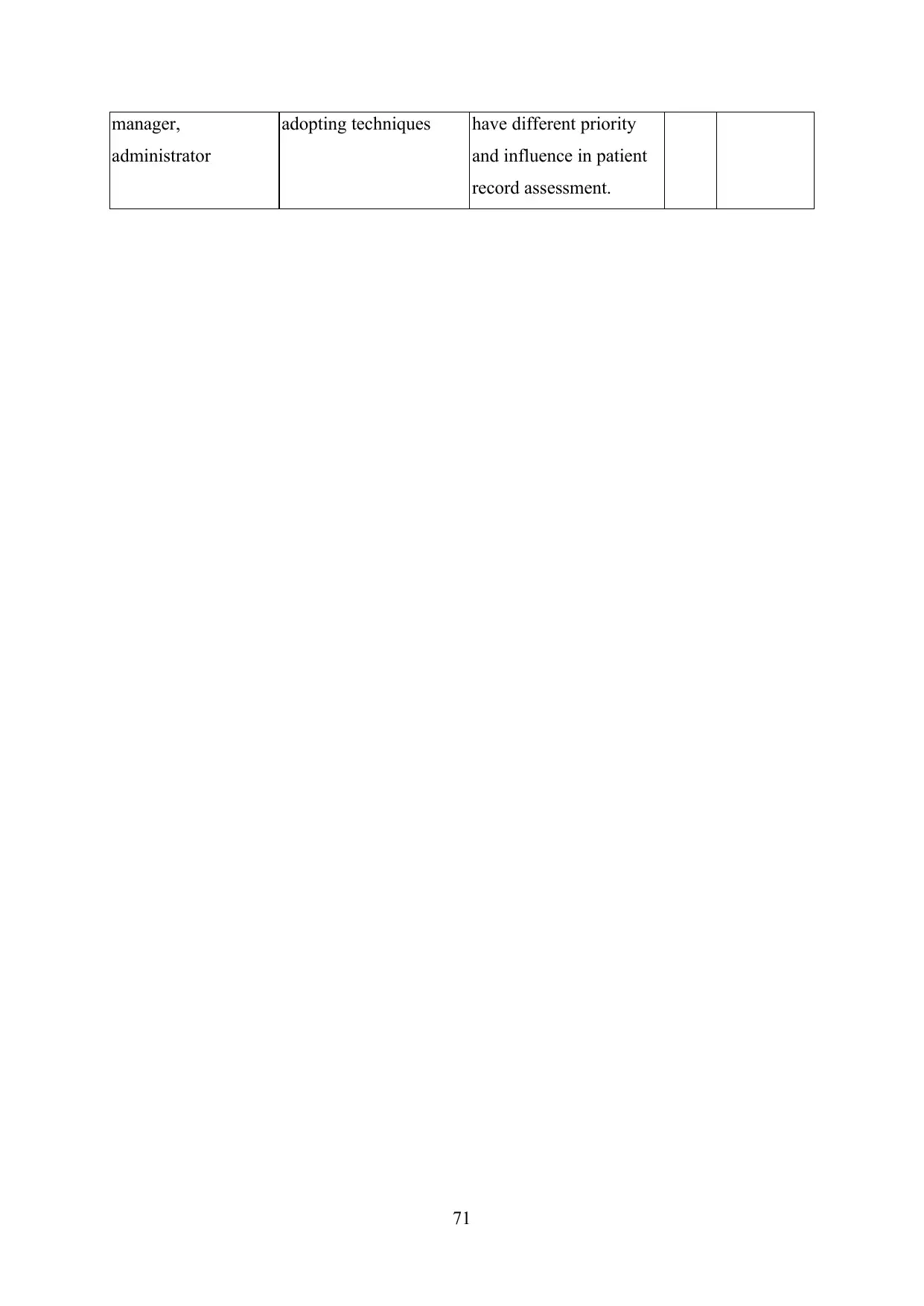
manager,
administrator
adopting techniques have different priority
and influence in patient
record assessment.
71
administrator
adopting techniques have different priority
and influence in patient
record assessment.
71
1 out of 72
Related Documents
![[object Object]](/_next/image/?url=%2F_next%2Fstatic%2Fmedia%2Flogo.6d15ce61.png&w=640&q=75)
Your All-in-One AI-Powered Toolkit for Academic Success.
+13062052269
info@desklib.com
Available 24*7 on WhatsApp / Email
Unlock your academic potential
© 2024 | Zucol Services PVT LTD | All rights reserved.