Modelling and Analysis of Traffic Light Control Systems - Paper Review
VerifiedAdded on 2023/05/29
|14
|4678
|58
AI Summary
This paper review critiques the article entitled “Modelling and analysis of traffic light control systems” by Huang and Su. The authors developed a traffic light control system using timed coloured Petri nets (TCPN) to improve control and management of traffic congestion at intersections of urban road networks. The article is reliable and relevant to the engineering field.
Contribute Materials
Your contribution can guide someone’s learning journey. Share your
documents today.
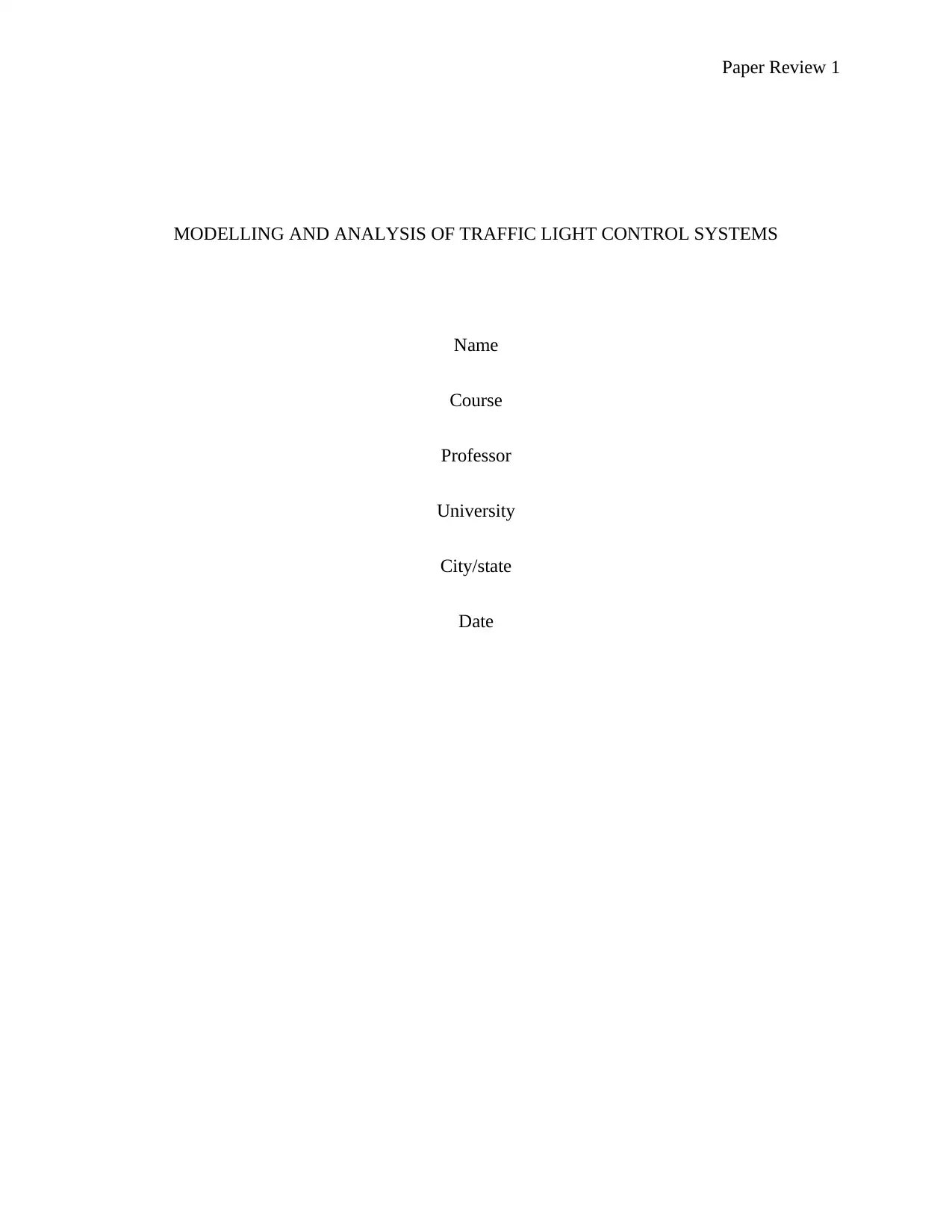
Paper Review 1
MODELLING AND ANALYSIS OF TRAFFIC LIGHT CONTROL SYSTEMS
Name
Course
Professor
University
City/state
Date
MODELLING AND ANALYSIS OF TRAFFIC LIGHT CONTROL SYSTEMS
Name
Course
Professor
University
City/state
Date
Secure Best Marks with AI Grader
Need help grading? Try our AI Grader for instant feedback on your assignments.
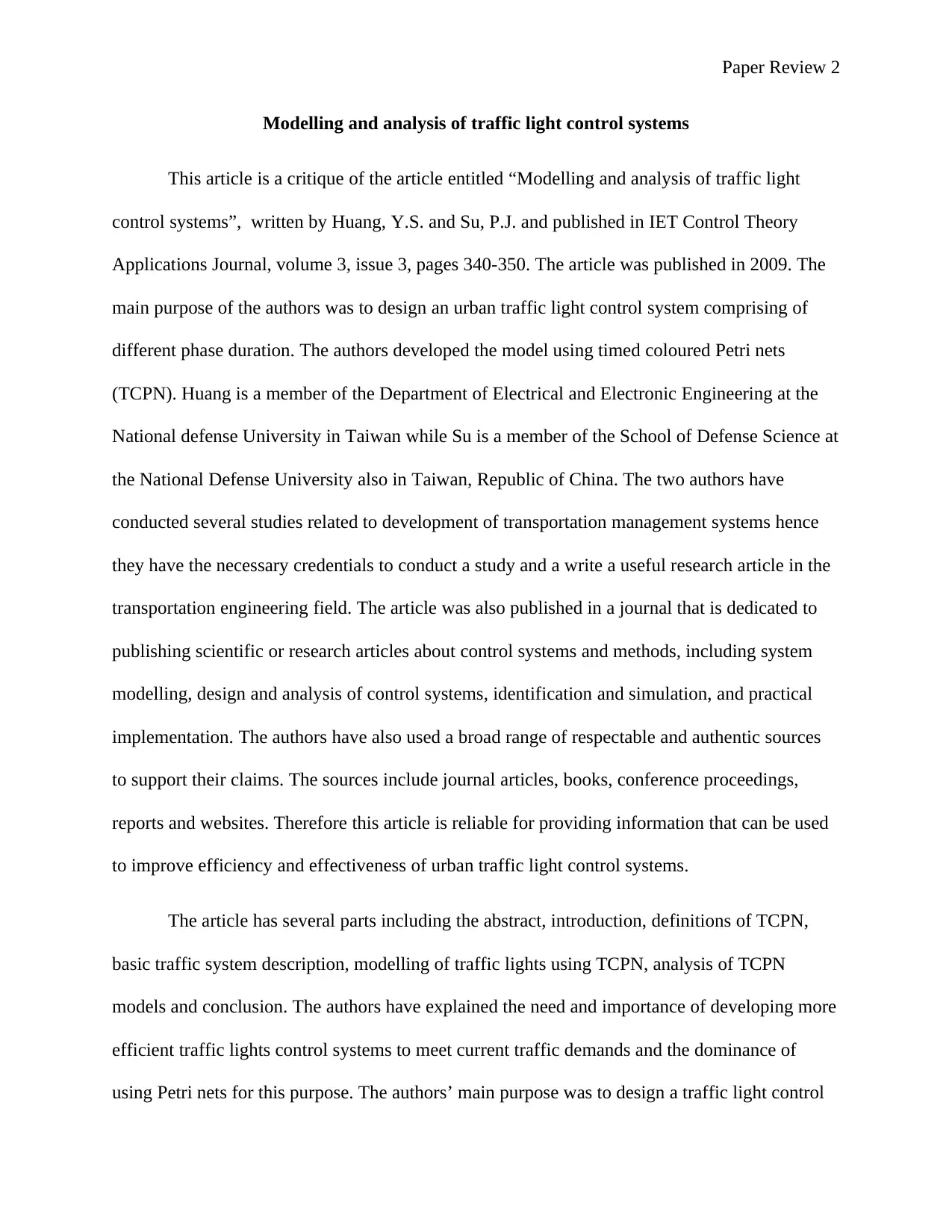
Paper Review 2
Modelling and analysis of traffic light control systems
This article is a critique of the article entitled “Modelling and analysis of traffic light
control systems”, written by Huang, Y.S. and Su, P.J. and published in IET Control Theory
Applications Journal, volume 3, issue 3, pages 340-350. The article was published in 2009. The
main purpose of the authors was to design an urban traffic light control system comprising of
different phase duration. The authors developed the model using timed coloured Petri nets
(TCPN). Huang is a member of the Department of Electrical and Electronic Engineering at the
National defense University in Taiwan while Su is a member of the School of Defense Science at
the National Defense University also in Taiwan, Republic of China. The two authors have
conducted several studies related to development of transportation management systems hence
they have the necessary credentials to conduct a study and a write a useful research article in the
transportation engineering field. The article was also published in a journal that is dedicated to
publishing scientific or research articles about control systems and methods, including system
modelling, design and analysis of control systems, identification and simulation, and practical
implementation. The authors have also used a broad range of respectable and authentic sources
to support their claims. The sources include journal articles, books, conference proceedings,
reports and websites. Therefore this article is reliable for providing information that can be used
to improve efficiency and effectiveness of urban traffic light control systems.
The article has several parts including the abstract, introduction, definitions of TCPN,
basic traffic system description, modelling of traffic lights using TCPN, analysis of TCPN
models and conclusion. The authors have explained the need and importance of developing more
efficient traffic lights control systems to meet current traffic demands and the dominance of
using Petri nets for this purpose. The authors’ main purpose was to design a traffic light control
Modelling and analysis of traffic light control systems
This article is a critique of the article entitled “Modelling and analysis of traffic light
control systems”, written by Huang, Y.S. and Su, P.J. and published in IET Control Theory
Applications Journal, volume 3, issue 3, pages 340-350. The article was published in 2009. The
main purpose of the authors was to design an urban traffic light control system comprising of
different phase duration. The authors developed the model using timed coloured Petri nets
(TCPN). Huang is a member of the Department of Electrical and Electronic Engineering at the
National defense University in Taiwan while Su is a member of the School of Defense Science at
the National Defense University also in Taiwan, Republic of China. The two authors have
conducted several studies related to development of transportation management systems hence
they have the necessary credentials to conduct a study and a write a useful research article in the
transportation engineering field. The article was also published in a journal that is dedicated to
publishing scientific or research articles about control systems and methods, including system
modelling, design and analysis of control systems, identification and simulation, and practical
implementation. The authors have also used a broad range of respectable and authentic sources
to support their claims. The sources include journal articles, books, conference proceedings,
reports and websites. Therefore this article is reliable for providing information that can be used
to improve efficiency and effectiveness of urban traffic light control systems.
The article has several parts including the abstract, introduction, definitions of TCPN,
basic traffic system description, modelling of traffic lights using TCPN, analysis of TCPN
models and conclusion. The authors have explained the need and importance of developing more
efficient traffic lights control systems to meet current traffic demands and the dominance of
using Petri nets for this purpose. The authors’ main purpose was to design a traffic light control
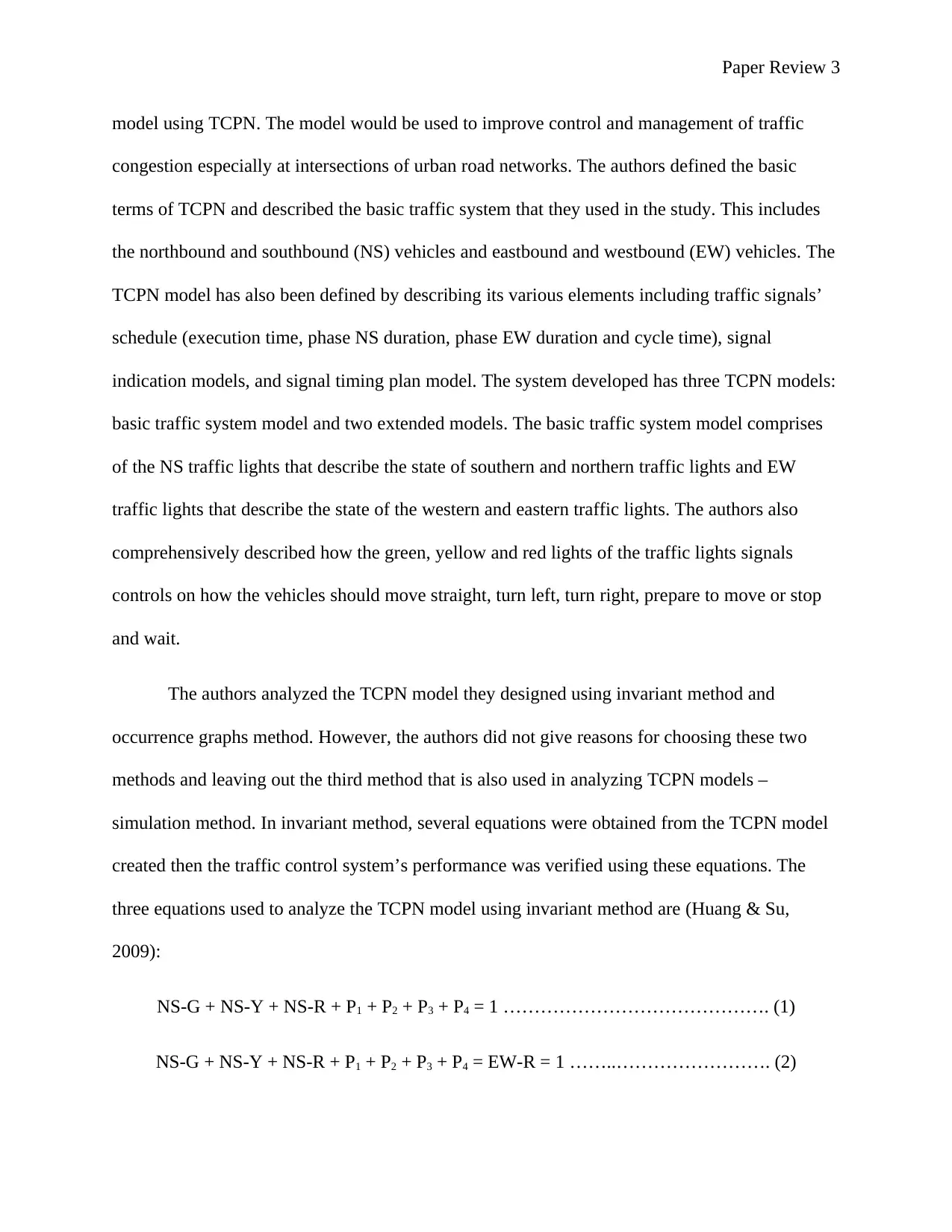
Paper Review 3
model using TCPN. The model would be used to improve control and management of traffic
congestion especially at intersections of urban road networks. The authors defined the basic
terms of TCPN and described the basic traffic system that they used in the study. This includes
the northbound and southbound (NS) vehicles and eastbound and westbound (EW) vehicles. The
TCPN model has also been defined by describing its various elements including traffic signals’
schedule (execution time, phase NS duration, phase EW duration and cycle time), signal
indication models, and signal timing plan model. The system developed has three TCPN models:
basic traffic system model and two extended models. The basic traffic system model comprises
of the NS traffic lights that describe the state of southern and northern traffic lights and EW
traffic lights that describe the state of the western and eastern traffic lights. The authors also
comprehensively described how the green, yellow and red lights of the traffic lights signals
controls on how the vehicles should move straight, turn left, turn right, prepare to move or stop
and wait.
The authors analyzed the TCPN model they designed using invariant method and
occurrence graphs method. However, the authors did not give reasons for choosing these two
methods and leaving out the third method that is also used in analyzing TCPN models –
simulation method. In invariant method, several equations were obtained from the TCPN model
created then the traffic control system’s performance was verified using these equations. The
three equations used to analyze the TCPN model using invariant method are (Huang & Su,
2009):
NS-G + NS-Y + NS-R + P1 + P2 + P3 + P4 = 1 ……………………………………. (1)
NS-G + NS-Y + NS-R + P1 + P2 + P3 + P4 = EW-R = 1 ……..……………………. (2)
model using TCPN. The model would be used to improve control and management of traffic
congestion especially at intersections of urban road networks. The authors defined the basic
terms of TCPN and described the basic traffic system that they used in the study. This includes
the northbound and southbound (NS) vehicles and eastbound and westbound (EW) vehicles. The
TCPN model has also been defined by describing its various elements including traffic signals’
schedule (execution time, phase NS duration, phase EW duration and cycle time), signal
indication models, and signal timing plan model. The system developed has three TCPN models:
basic traffic system model and two extended models. The basic traffic system model comprises
of the NS traffic lights that describe the state of southern and northern traffic lights and EW
traffic lights that describe the state of the western and eastern traffic lights. The authors also
comprehensively described how the green, yellow and red lights of the traffic lights signals
controls on how the vehicles should move straight, turn left, turn right, prepare to move or stop
and wait.
The authors analyzed the TCPN model they designed using invariant method and
occurrence graphs method. However, the authors did not give reasons for choosing these two
methods and leaving out the third method that is also used in analyzing TCPN models –
simulation method. In invariant method, several equations were obtained from the TCPN model
created then the traffic control system’s performance was verified using these equations. The
three equations used to analyze the TCPN model using invariant method are (Huang & Su,
2009):
NS-G + NS-Y + NS-R + P1 + P2 + P3 + P4 = 1 ……………………………………. (1)
NS-G + NS-Y + NS-R + P1 + P2 + P3 + P4 = EW-R = 1 ……..……………………. (2)
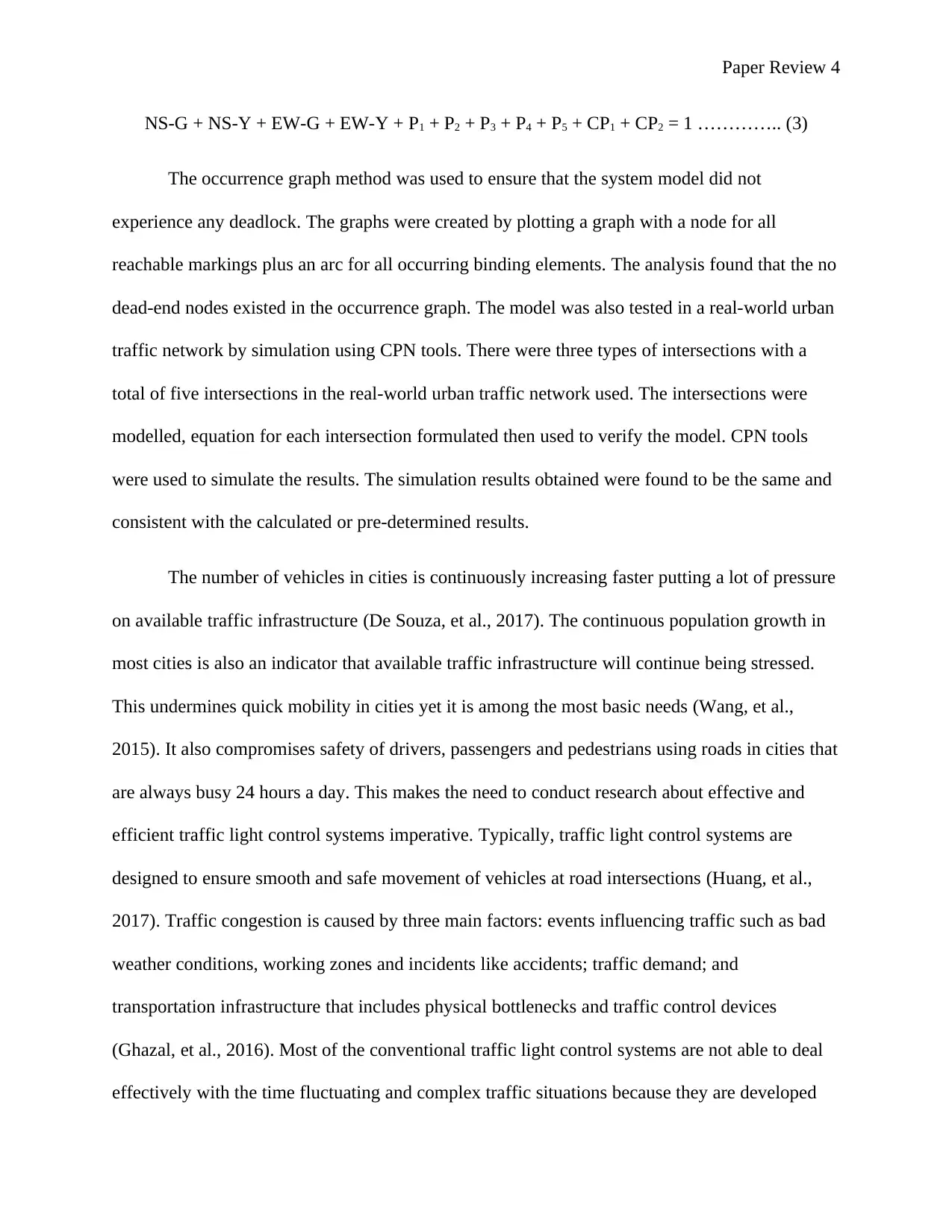
Paper Review 4
NS-G + NS-Y + EW-G + EW-Y + P1 + P2 + P3 + P4 + P5 + CP1 + CP2 = 1 ………….. (3)
The occurrence graph method was used to ensure that the system model did not
experience any deadlock. The graphs were created by plotting a graph with a node for all
reachable markings plus an arc for all occurring binding elements. The analysis found that the no
dead-end nodes existed in the occurrence graph. The model was also tested in a real-world urban
traffic network by simulation using CPN tools. There were three types of intersections with a
total of five intersections in the real-world urban traffic network used. The intersections were
modelled, equation for each intersection formulated then used to verify the model. CPN tools
were used to simulate the results. The simulation results obtained were found to be the same and
consistent with the calculated or pre-determined results.
The number of vehicles in cities is continuously increasing faster putting a lot of pressure
on available traffic infrastructure (De Souza, et al., 2017). The continuous population growth in
most cities is also an indicator that available traffic infrastructure will continue being stressed.
This undermines quick mobility in cities yet it is among the most basic needs (Wang, et al.,
2015). It also compromises safety of drivers, passengers and pedestrians using roads in cities that
are always busy 24 hours a day. This makes the need to conduct research about effective and
efficient traffic light control systems imperative. Typically, traffic light control systems are
designed to ensure smooth and safe movement of vehicles at road intersections (Huang, et al.,
2017). Traffic congestion is caused by three main factors: events influencing traffic such as bad
weather conditions, working zones and incidents like accidents; traffic demand; and
transportation infrastructure that includes physical bottlenecks and traffic control devices
(Ghazal, et al., 2016). Most of the conventional traffic light control systems are not able to deal
effectively with the time fluctuating and complex traffic situations because they are developed
NS-G + NS-Y + EW-G + EW-Y + P1 + P2 + P3 + P4 + P5 + CP1 + CP2 = 1 ………….. (3)
The occurrence graph method was used to ensure that the system model did not
experience any deadlock. The graphs were created by plotting a graph with a node for all
reachable markings plus an arc for all occurring binding elements. The analysis found that the no
dead-end nodes existed in the occurrence graph. The model was also tested in a real-world urban
traffic network by simulation using CPN tools. There were three types of intersections with a
total of five intersections in the real-world urban traffic network used. The intersections were
modelled, equation for each intersection formulated then used to verify the model. CPN tools
were used to simulate the results. The simulation results obtained were found to be the same and
consistent with the calculated or pre-determined results.
The number of vehicles in cities is continuously increasing faster putting a lot of pressure
on available traffic infrastructure (De Souza, et al., 2017). The continuous population growth in
most cities is also an indicator that available traffic infrastructure will continue being stressed.
This undermines quick mobility in cities yet it is among the most basic needs (Wang, et al.,
2015). It also compromises safety of drivers, passengers and pedestrians using roads in cities that
are always busy 24 hours a day. This makes the need to conduct research about effective and
efficient traffic light control systems imperative. Typically, traffic light control systems are
designed to ensure smooth and safe movement of vehicles at road intersections (Huang, et al.,
2017). Traffic congestion is caused by three main factors: events influencing traffic such as bad
weather conditions, working zones and incidents like accidents; traffic demand; and
transportation infrastructure that includes physical bottlenecks and traffic control devices
(Ghazal, et al., 2016). Most of the conventional traffic light control systems are not able to deal
effectively with the time fluctuating and complex traffic situations because they are developed
Secure Best Marks with AI Grader
Need help grading? Try our AI Grader for instant feedback on your assignments.
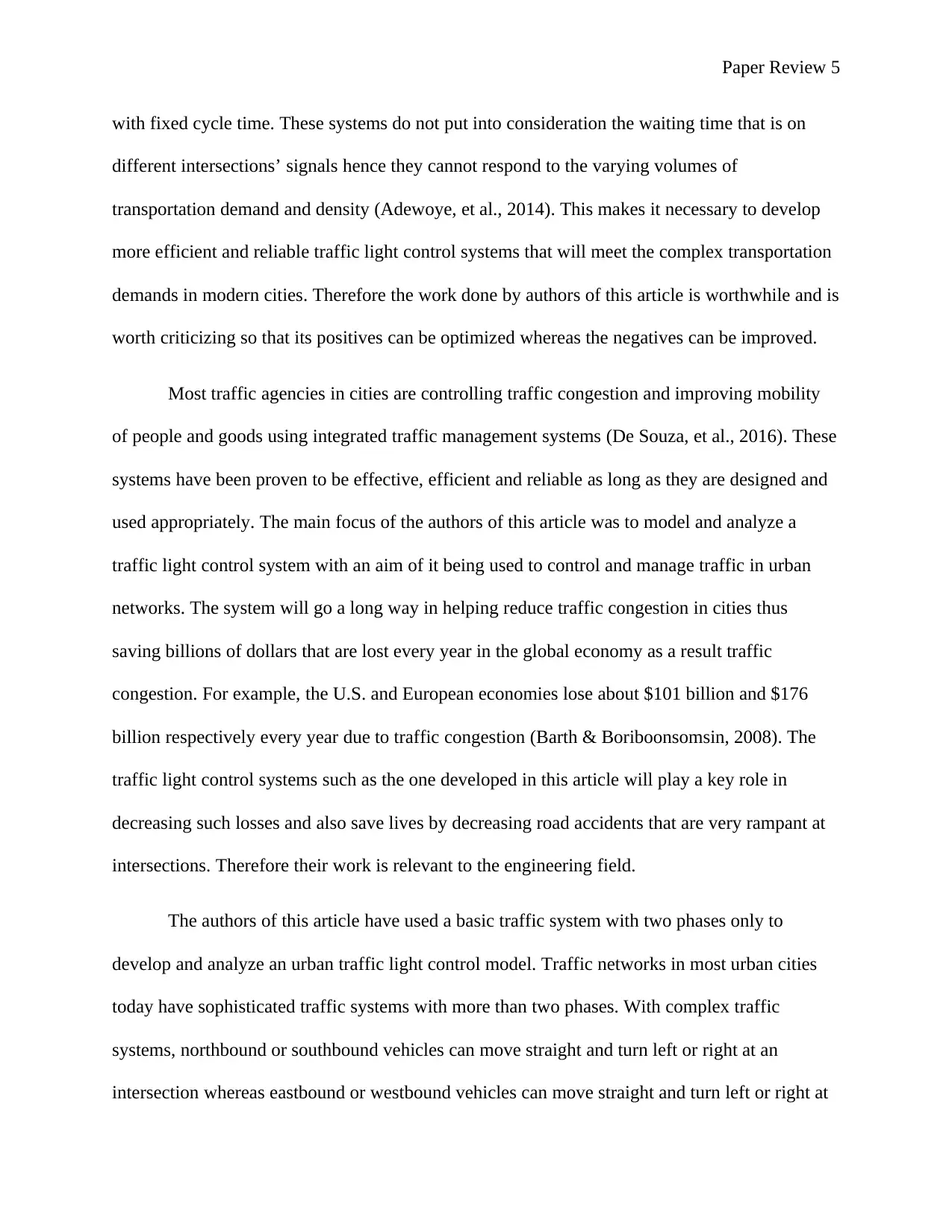
Paper Review 5
with fixed cycle time. These systems do not put into consideration the waiting time that is on
different intersections’ signals hence they cannot respond to the varying volumes of
transportation demand and density (Adewoye, et al., 2014). This makes it necessary to develop
more efficient and reliable traffic light control systems that will meet the complex transportation
demands in modern cities. Therefore the work done by authors of this article is worthwhile and is
worth criticizing so that its positives can be optimized whereas the negatives can be improved.
Most traffic agencies in cities are controlling traffic congestion and improving mobility
of people and goods using integrated traffic management systems (De Souza, et al., 2016). These
systems have been proven to be effective, efficient and reliable as long as they are designed and
used appropriately. The main focus of the authors of this article was to model and analyze a
traffic light control system with an aim of it being used to control and manage traffic in urban
networks. The system will go a long way in helping reduce traffic congestion in cities thus
saving billions of dollars that are lost every year in the global economy as a result traffic
congestion. For example, the U.S. and European economies lose about $101 billion and $176
billion respectively every year due to traffic congestion (Barth & Boriboonsomsin, 2008). The
traffic light control systems such as the one developed in this article will play a key role in
decreasing such losses and also save lives by decreasing road accidents that are very rampant at
intersections. Therefore their work is relevant to the engineering field.
The authors of this article have used a basic traffic system with two phases only to
develop and analyze an urban traffic light control model. Traffic networks in most urban cities
today have sophisticated traffic systems with more than two phases. With complex traffic
systems, northbound or southbound vehicles can move straight and turn left or right at an
intersection whereas eastbound or westbound vehicles can move straight and turn left or right at
with fixed cycle time. These systems do not put into consideration the waiting time that is on
different intersections’ signals hence they cannot respond to the varying volumes of
transportation demand and density (Adewoye, et al., 2014). This makes it necessary to develop
more efficient and reliable traffic light control systems that will meet the complex transportation
demands in modern cities. Therefore the work done by authors of this article is worthwhile and is
worth criticizing so that its positives can be optimized whereas the negatives can be improved.
Most traffic agencies in cities are controlling traffic congestion and improving mobility
of people and goods using integrated traffic management systems (De Souza, et al., 2016). These
systems have been proven to be effective, efficient and reliable as long as they are designed and
used appropriately. The main focus of the authors of this article was to model and analyze a
traffic light control system with an aim of it being used to control and manage traffic in urban
networks. The system will go a long way in helping reduce traffic congestion in cities thus
saving billions of dollars that are lost every year in the global economy as a result traffic
congestion. For example, the U.S. and European economies lose about $101 billion and $176
billion respectively every year due to traffic congestion (Barth & Boriboonsomsin, 2008). The
traffic light control systems such as the one developed in this article will play a key role in
decreasing such losses and also save lives by decreasing road accidents that are very rampant at
intersections. Therefore their work is relevant to the engineering field.
The authors of this article have used a basic traffic system with two phases only to
develop and analyze an urban traffic light control model. Traffic networks in most urban cities
today have sophisticated traffic systems with more than two phases. With complex traffic
systems, northbound or southbound vehicles can move straight and turn left or right at an
intersection whereas eastbound or westbound vehicles can move straight and turn left or right at
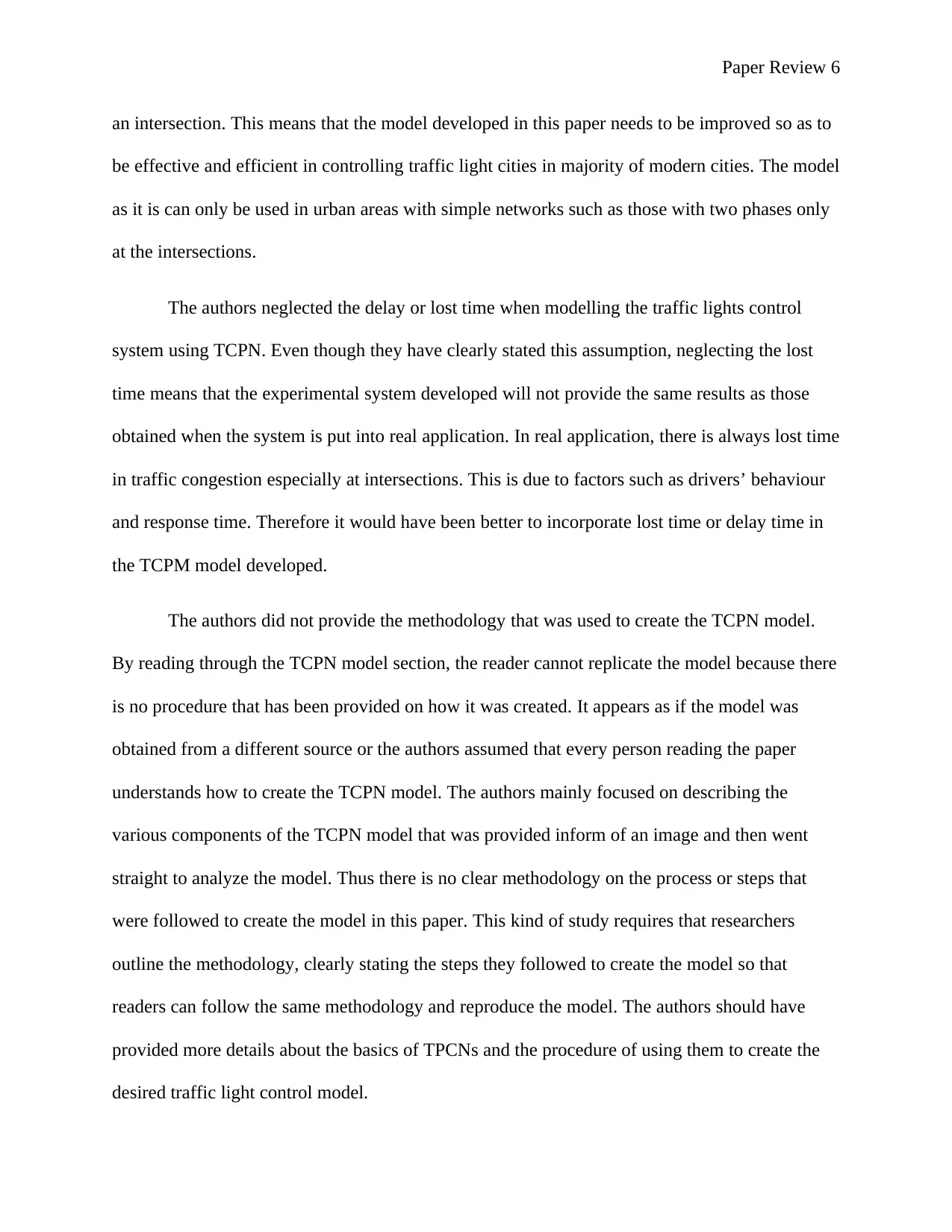
Paper Review 6
an intersection. This means that the model developed in this paper needs to be improved so as to
be effective and efficient in controlling traffic light cities in majority of modern cities. The model
as it is can only be used in urban areas with simple networks such as those with two phases only
at the intersections.
The authors neglected the delay or lost time when modelling the traffic lights control
system using TCPN. Even though they have clearly stated this assumption, neglecting the lost
time means that the experimental system developed will not provide the same results as those
obtained when the system is put into real application. In real application, there is always lost time
in traffic congestion especially at intersections. This is due to factors such as drivers’ behaviour
and response time. Therefore it would have been better to incorporate lost time or delay time in
the TCPM model developed.
The authors did not provide the methodology that was used to create the TCPN model.
By reading through the TCPN model section, the reader cannot replicate the model because there
is no procedure that has been provided on how it was created. It appears as if the model was
obtained from a different source or the authors assumed that every person reading the paper
understands how to create the TCPN model. The authors mainly focused on describing the
various components of the TCPN model that was provided inform of an image and then went
straight to analyze the model. Thus there is no clear methodology on the process or steps that
were followed to create the model in this paper. This kind of study requires that researchers
outline the methodology, clearly stating the steps they followed to create the model so that
readers can follow the same methodology and reproduce the model. The authors should have
provided more details about the basics of TPCNs and the procedure of using them to create the
desired traffic light control model.
an intersection. This means that the model developed in this paper needs to be improved so as to
be effective and efficient in controlling traffic light cities in majority of modern cities. The model
as it is can only be used in urban areas with simple networks such as those with two phases only
at the intersections.
The authors neglected the delay or lost time when modelling the traffic lights control
system using TCPN. Even though they have clearly stated this assumption, neglecting the lost
time means that the experimental system developed will not provide the same results as those
obtained when the system is put into real application. In real application, there is always lost time
in traffic congestion especially at intersections. This is due to factors such as drivers’ behaviour
and response time. Therefore it would have been better to incorporate lost time or delay time in
the TCPM model developed.
The authors did not provide the methodology that was used to create the TCPN model.
By reading through the TCPN model section, the reader cannot replicate the model because there
is no procedure that has been provided on how it was created. It appears as if the model was
obtained from a different source or the authors assumed that every person reading the paper
understands how to create the TCPN model. The authors mainly focused on describing the
various components of the TCPN model that was provided inform of an image and then went
straight to analyze the model. Thus there is no clear methodology on the process or steps that
were followed to create the model in this paper. This kind of study requires that researchers
outline the methodology, clearly stating the steps they followed to create the model so that
readers can follow the same methodology and reproduce the model. The authors should have
provided more details about the basics of TPCNs and the procedure of using them to create the
desired traffic light control model.
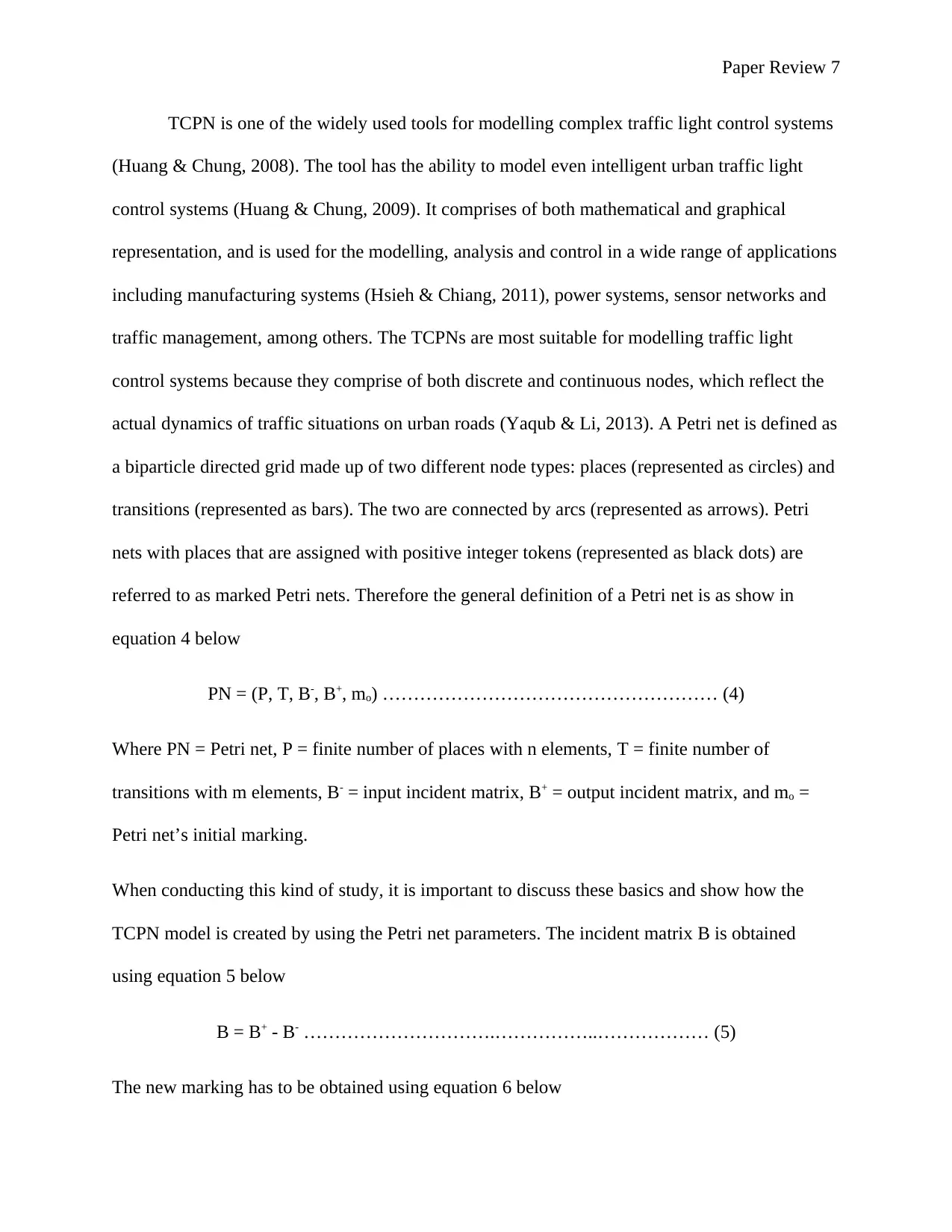
Paper Review 7
TCPN is one of the widely used tools for modelling complex traffic light control systems
(Huang & Chung, 2008). The tool has the ability to model even intelligent urban traffic light
control systems (Huang & Chung, 2009). It comprises of both mathematical and graphical
representation, and is used for the modelling, analysis and control in a wide range of applications
including manufacturing systems (Hsieh & Chiang, 2011), power systems, sensor networks and
traffic management, among others. The TCPNs are most suitable for modelling traffic light
control systems because they comprise of both discrete and continuous nodes, which reflect the
actual dynamics of traffic situations on urban roads (Yaqub & Li, 2013). A Petri net is defined as
a biparticle directed grid made up of two different node types: places (represented as circles) and
transitions (represented as bars). The two are connected by arcs (represented as arrows). Petri
nets with places that are assigned with positive integer tokens (represented as black dots) are
referred to as marked Petri nets. Therefore the general definition of a Petri net is as show in
equation 4 below
PN = (P, T, B-, B+, mo) ……………………………………………… (4)
Where PN = Petri net, P = finite number of places with n elements, T = finite number of
transitions with m elements, B- = input incident matrix, B+ = output incident matrix, and mo =
Petri net’s initial marking.
When conducting this kind of study, it is important to discuss these basics and show how the
TCPN model is created by using the Petri net parameters. The incident matrix B is obtained
using equation 5 below
B = B+ - B- ………………………….……………..……………… (5)
The new marking has to be obtained using equation 6 below
TCPN is one of the widely used tools for modelling complex traffic light control systems
(Huang & Chung, 2008). The tool has the ability to model even intelligent urban traffic light
control systems (Huang & Chung, 2009). It comprises of both mathematical and graphical
representation, and is used for the modelling, analysis and control in a wide range of applications
including manufacturing systems (Hsieh & Chiang, 2011), power systems, sensor networks and
traffic management, among others. The TCPNs are most suitable for modelling traffic light
control systems because they comprise of both discrete and continuous nodes, which reflect the
actual dynamics of traffic situations on urban roads (Yaqub & Li, 2013). A Petri net is defined as
a biparticle directed grid made up of two different node types: places (represented as circles) and
transitions (represented as bars). The two are connected by arcs (represented as arrows). Petri
nets with places that are assigned with positive integer tokens (represented as black dots) are
referred to as marked Petri nets. Therefore the general definition of a Petri net is as show in
equation 4 below
PN = (P, T, B-, B+, mo) ……………………………………………… (4)
Where PN = Petri net, P = finite number of places with n elements, T = finite number of
transitions with m elements, B- = input incident matrix, B+ = output incident matrix, and mo =
Petri net’s initial marking.
When conducting this kind of study, it is important to discuss these basics and show how the
TCPN model is created by using the Petri net parameters. The incident matrix B is obtained
using equation 5 below
B = B+ - B- ………………………….……………..……………… (5)
The new marking has to be obtained using equation 6 below
Paraphrase This Document
Need a fresh take? Get an instant paraphrase of this document with our AI Paraphraser
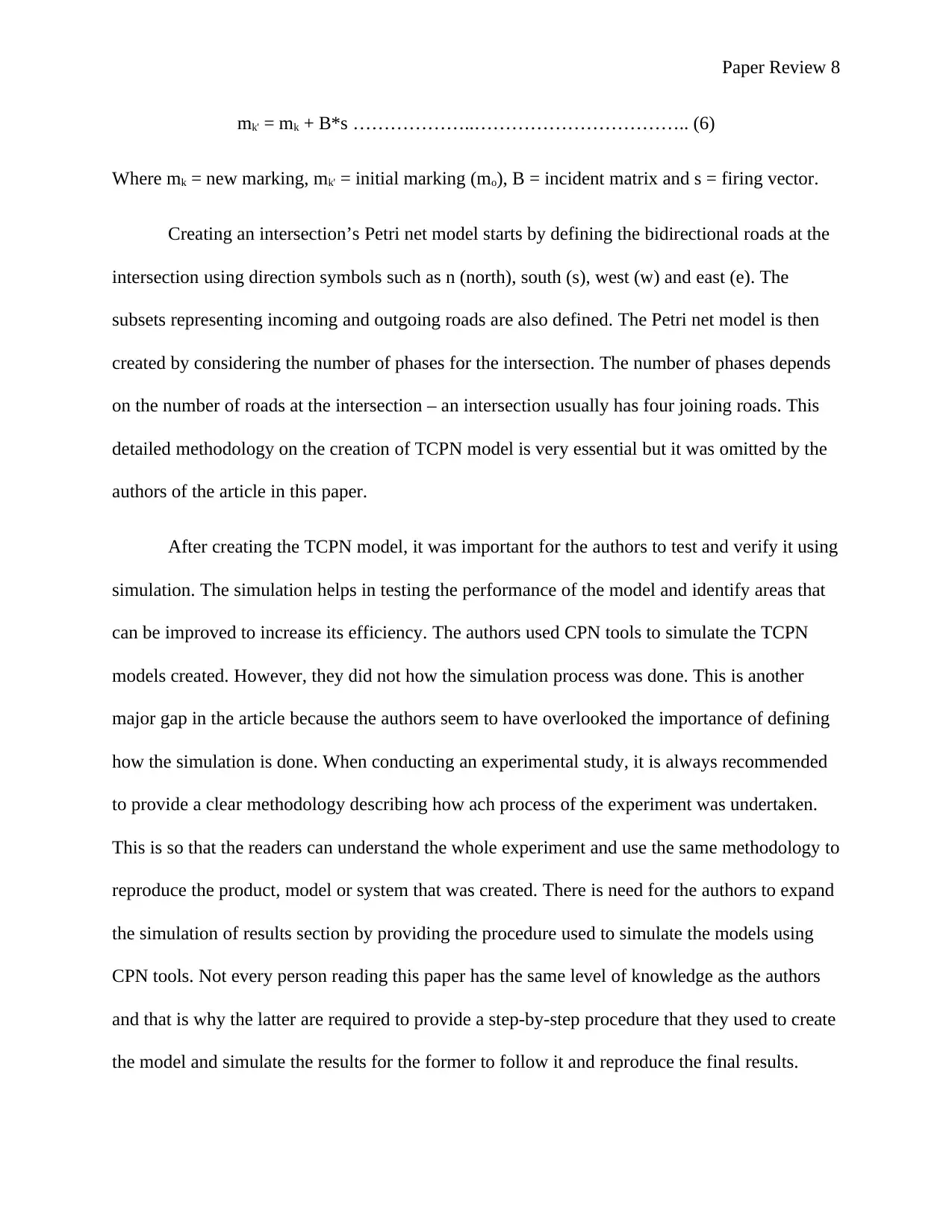
Paper Review 8
mk' = mk + B*s ………………..…………………………….. (6)
Where mk = new marking, mk' = initial marking (mo), B = incident matrix and s = firing vector.
Creating an intersection’s Petri net model starts by defining the bidirectional roads at the
intersection using direction symbols such as n (north), south (s), west (w) and east (e). The
subsets representing incoming and outgoing roads are also defined. The Petri net model is then
created by considering the number of phases for the intersection. The number of phases depends
on the number of roads at the intersection – an intersection usually has four joining roads. This
detailed methodology on the creation of TCPN model is very essential but it was omitted by the
authors of the article in this paper.
After creating the TCPN model, it was important for the authors to test and verify it using
simulation. The simulation helps in testing the performance of the model and identify areas that
can be improved to increase its efficiency. The authors used CPN tools to simulate the TCPN
models created. However, they did not how the simulation process was done. This is another
major gap in the article because the authors seem to have overlooked the importance of defining
how the simulation is done. When conducting an experimental study, it is always recommended
to provide a clear methodology describing how ach process of the experiment was undertaken.
This is so that the readers can understand the whole experiment and use the same methodology to
reproduce the product, model or system that was created. There is need for the authors to expand
the simulation of results section by providing the procedure used to simulate the models using
CPN tools. Not every person reading this paper has the same level of knowledge as the authors
and that is why the latter are required to provide a step-by-step procedure that they used to create
the model and simulate the results for the former to follow it and reproduce the final results.
mk' = mk + B*s ………………..…………………………….. (6)
Where mk = new marking, mk' = initial marking (mo), B = incident matrix and s = firing vector.
Creating an intersection’s Petri net model starts by defining the bidirectional roads at the
intersection using direction symbols such as n (north), south (s), west (w) and east (e). The
subsets representing incoming and outgoing roads are also defined. The Petri net model is then
created by considering the number of phases for the intersection. The number of phases depends
on the number of roads at the intersection – an intersection usually has four joining roads. This
detailed methodology on the creation of TCPN model is very essential but it was omitted by the
authors of the article in this paper.
After creating the TCPN model, it was important for the authors to test and verify it using
simulation. The simulation helps in testing the performance of the model and identify areas that
can be improved to increase its efficiency. The authors used CPN tools to simulate the TCPN
models created. However, they did not how the simulation process was done. This is another
major gap in the article because the authors seem to have overlooked the importance of defining
how the simulation is done. When conducting an experimental study, it is always recommended
to provide a clear methodology describing how ach process of the experiment was undertaken.
This is so that the readers can understand the whole experiment and use the same methodology to
reproduce the product, model or system that was created. There is need for the authors to expand
the simulation of results section by providing the procedure used to simulate the models using
CPN tools. Not every person reading this paper has the same level of knowledge as the authors
and that is why the latter are required to provide a step-by-step procedure that they used to create
the model and simulate the results for the former to follow it and reproduce the final results.
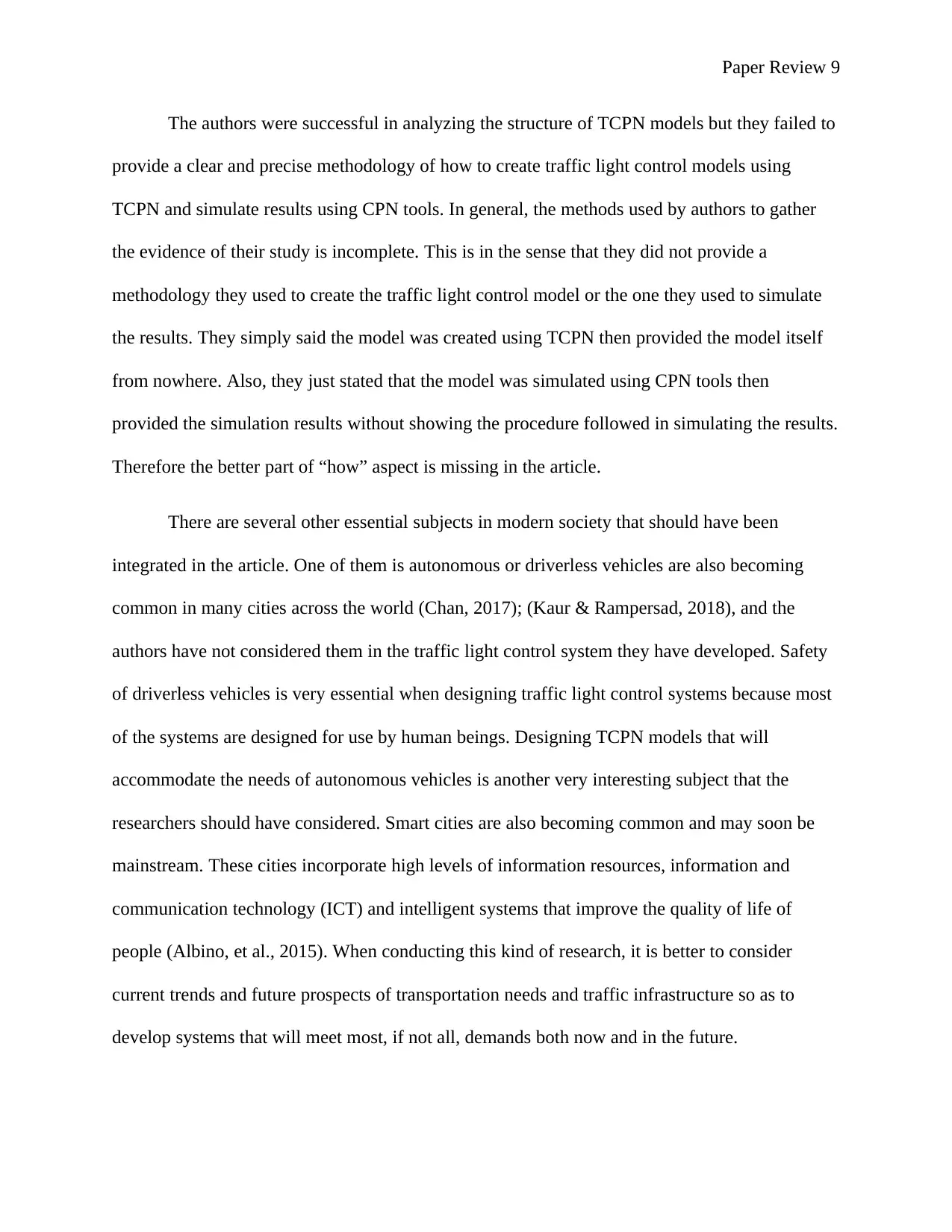
Paper Review 9
The authors were successful in analyzing the structure of TCPN models but they failed to
provide a clear and precise methodology of how to create traffic light control models using
TCPN and simulate results using CPN tools. In general, the methods used by authors to gather
the evidence of their study is incomplete. This is in the sense that they did not provide a
methodology they used to create the traffic light control model or the one they used to simulate
the results. They simply said the model was created using TCPN then provided the model itself
from nowhere. Also, they just stated that the model was simulated using CPN tools then
provided the simulation results without showing the procedure followed in simulating the results.
Therefore the better part of “how” aspect is missing in the article.
There are several other essential subjects in modern society that should have been
integrated in the article. One of them is autonomous or driverless vehicles are also becoming
common in many cities across the world (Chan, 2017); (Kaur & Rampersad, 2018), and the
authors have not considered them in the traffic light control system they have developed. Safety
of driverless vehicles is very essential when designing traffic light control systems because most
of the systems are designed for use by human beings. Designing TCPN models that will
accommodate the needs of autonomous vehicles is another very interesting subject that the
researchers should have considered. Smart cities are also becoming common and may soon be
mainstream. These cities incorporate high levels of information resources, information and
communication technology (ICT) and intelligent systems that improve the quality of life of
people (Albino, et al., 2015). When conducting this kind of research, it is better to consider
current trends and future prospects of transportation needs and traffic infrastructure so as to
develop systems that will meet most, if not all, demands both now and in the future.
The authors were successful in analyzing the structure of TCPN models but they failed to
provide a clear and precise methodology of how to create traffic light control models using
TCPN and simulate results using CPN tools. In general, the methods used by authors to gather
the evidence of their study is incomplete. This is in the sense that they did not provide a
methodology they used to create the traffic light control model or the one they used to simulate
the results. They simply said the model was created using TCPN then provided the model itself
from nowhere. Also, they just stated that the model was simulated using CPN tools then
provided the simulation results without showing the procedure followed in simulating the results.
Therefore the better part of “how” aspect is missing in the article.
There are several other essential subjects in modern society that should have been
integrated in the article. One of them is autonomous or driverless vehicles are also becoming
common in many cities across the world (Chan, 2017); (Kaur & Rampersad, 2018), and the
authors have not considered them in the traffic light control system they have developed. Safety
of driverless vehicles is very essential when designing traffic light control systems because most
of the systems are designed for use by human beings. Designing TCPN models that will
accommodate the needs of autonomous vehicles is another very interesting subject that the
researchers should have considered. Smart cities are also becoming common and may soon be
mainstream. These cities incorporate high levels of information resources, information and
communication technology (ICT) and intelligent systems that improve the quality of life of
people (Albino, et al., 2015). When conducting this kind of research, it is better to consider
current trends and future prospects of transportation needs and traffic infrastructure so as to
develop systems that will meet most, if not all, demands both now and in the future.
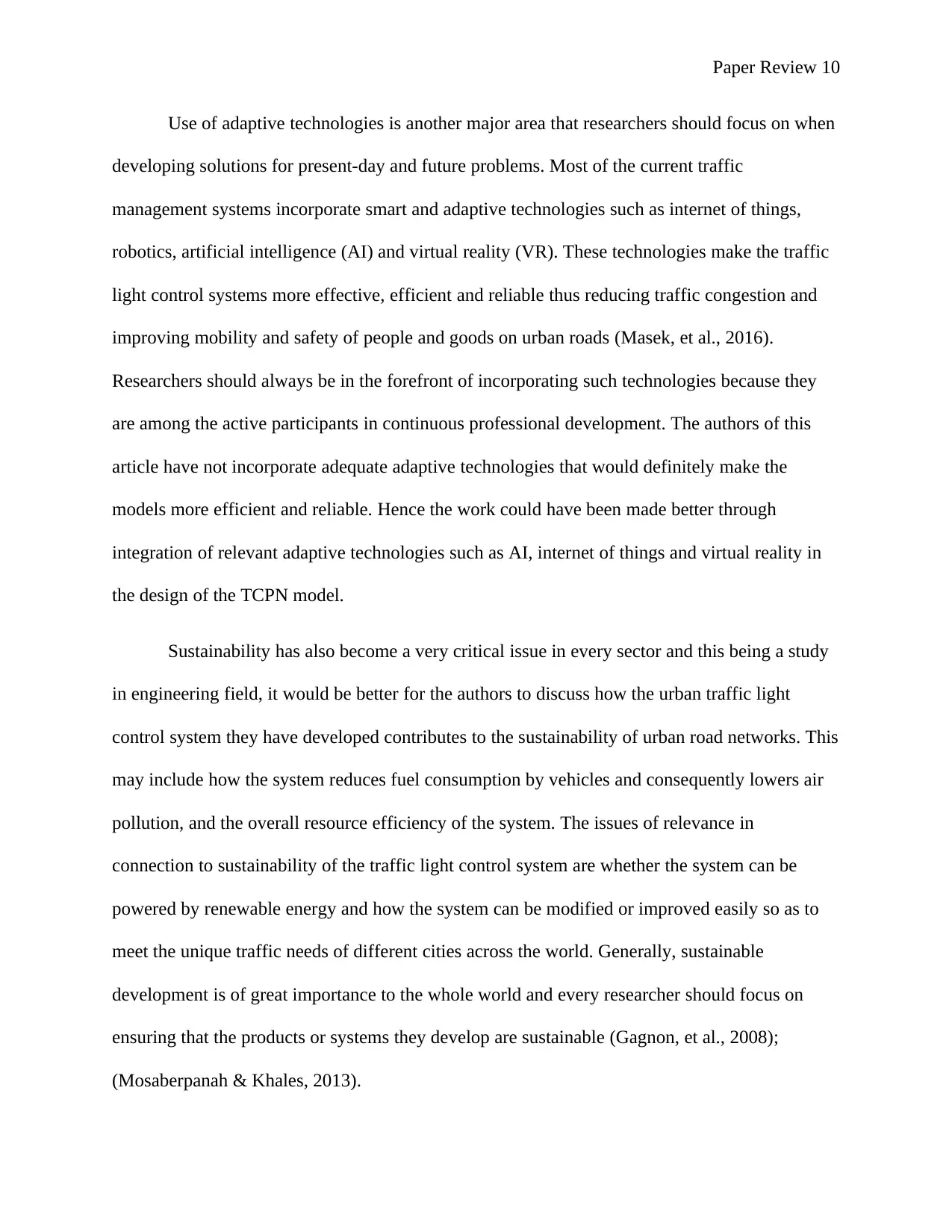
Paper Review 10
Use of adaptive technologies is another major area that researchers should focus on when
developing solutions for present-day and future problems. Most of the current traffic
management systems incorporate smart and adaptive technologies such as internet of things,
robotics, artificial intelligence (AI) and virtual reality (VR). These technologies make the traffic
light control systems more effective, efficient and reliable thus reducing traffic congestion and
improving mobility and safety of people and goods on urban roads (Masek, et al., 2016).
Researchers should always be in the forefront of incorporating such technologies because they
are among the active participants in continuous professional development. The authors of this
article have not incorporate adequate adaptive technologies that would definitely make the
models more efficient and reliable. Hence the work could have been made better through
integration of relevant adaptive technologies such as AI, internet of things and virtual reality in
the design of the TCPN model.
Sustainability has also become a very critical issue in every sector and this being a study
in engineering field, it would be better for the authors to discuss how the urban traffic light
control system they have developed contributes to the sustainability of urban road networks. This
may include how the system reduces fuel consumption by vehicles and consequently lowers air
pollution, and the overall resource efficiency of the system. The issues of relevance in
connection to sustainability of the traffic light control system are whether the system can be
powered by renewable energy and how the system can be modified or improved easily so as to
meet the unique traffic needs of different cities across the world. Generally, sustainable
development is of great importance to the whole world and every researcher should focus on
ensuring that the products or systems they develop are sustainable (Gagnon, et al., 2008);
(Mosaberpanah & Khales, 2013).
Use of adaptive technologies is another major area that researchers should focus on when
developing solutions for present-day and future problems. Most of the current traffic
management systems incorporate smart and adaptive technologies such as internet of things,
robotics, artificial intelligence (AI) and virtual reality (VR). These technologies make the traffic
light control systems more effective, efficient and reliable thus reducing traffic congestion and
improving mobility and safety of people and goods on urban roads (Masek, et al., 2016).
Researchers should always be in the forefront of incorporating such technologies because they
are among the active participants in continuous professional development. The authors of this
article have not incorporate adequate adaptive technologies that would definitely make the
models more efficient and reliable. Hence the work could have been made better through
integration of relevant adaptive technologies such as AI, internet of things and virtual reality in
the design of the TCPN model.
Sustainability has also become a very critical issue in every sector and this being a study
in engineering field, it would be better for the authors to discuss how the urban traffic light
control system they have developed contributes to the sustainability of urban road networks. This
may include how the system reduces fuel consumption by vehicles and consequently lowers air
pollution, and the overall resource efficiency of the system. The issues of relevance in
connection to sustainability of the traffic light control system are whether the system can be
powered by renewable energy and how the system can be modified or improved easily so as to
meet the unique traffic needs of different cities across the world. Generally, sustainable
development is of great importance to the whole world and every researcher should focus on
ensuring that the products or systems they develop are sustainable (Gagnon, et al., 2008);
(Mosaberpanah & Khales, 2013).
Secure Best Marks with AI Grader
Need help grading? Try our AI Grader for instant feedback on your assignments.
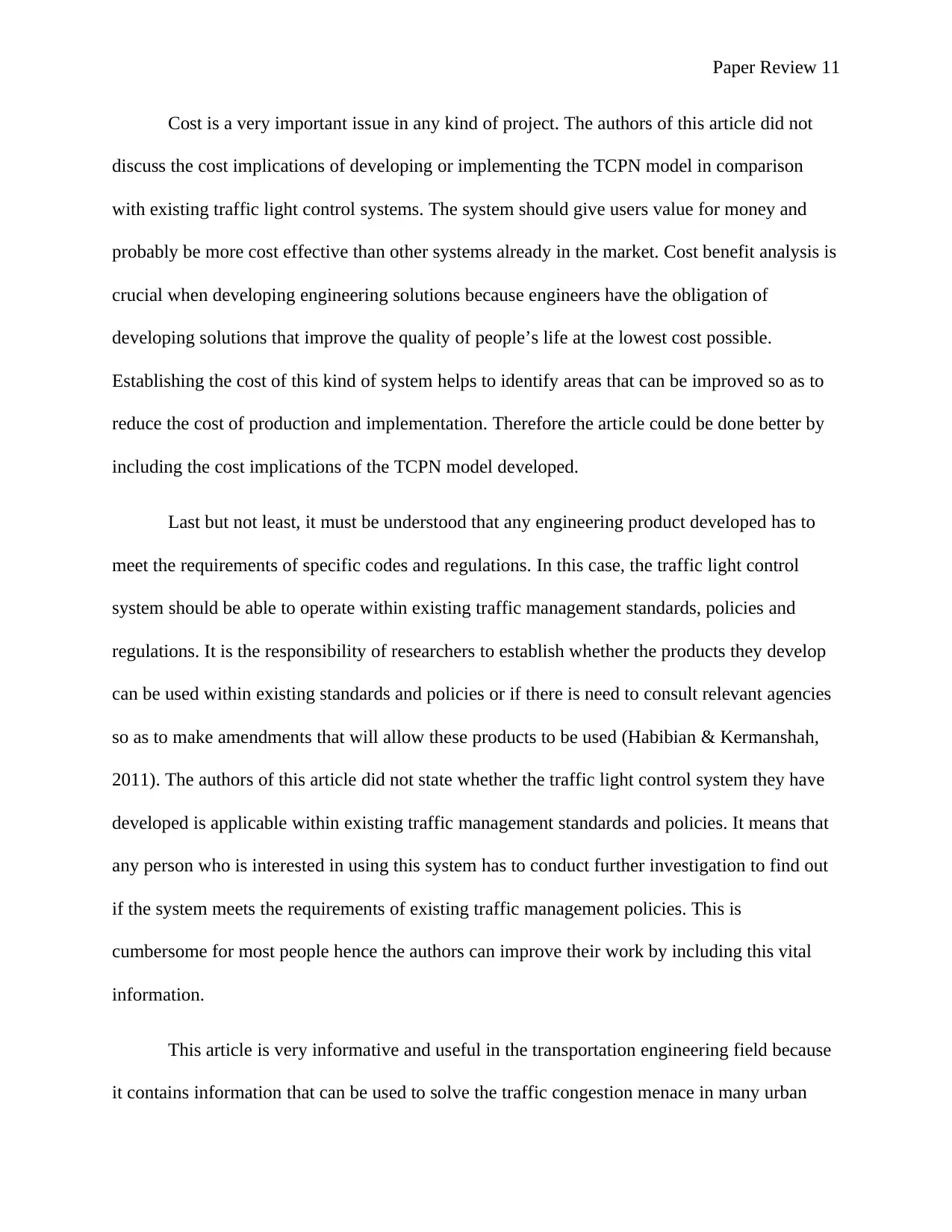
Paper Review 11
Cost is a very important issue in any kind of project. The authors of this article did not
discuss the cost implications of developing or implementing the TCPN model in comparison
with existing traffic light control systems. The system should give users value for money and
probably be more cost effective than other systems already in the market. Cost benefit analysis is
crucial when developing engineering solutions because engineers have the obligation of
developing solutions that improve the quality of people’s life at the lowest cost possible.
Establishing the cost of this kind of system helps to identify areas that can be improved so as to
reduce the cost of production and implementation. Therefore the article could be done better by
including the cost implications of the TCPN model developed.
Last but not least, it must be understood that any engineering product developed has to
meet the requirements of specific codes and regulations. In this case, the traffic light control
system should be able to operate within existing traffic management standards, policies and
regulations. It is the responsibility of researchers to establish whether the products they develop
can be used within existing standards and policies or if there is need to consult relevant agencies
so as to make amendments that will allow these products to be used (Habibian & Kermanshah,
2011). The authors of this article did not state whether the traffic light control system they have
developed is applicable within existing traffic management standards and policies. It means that
any person who is interested in using this system has to conduct further investigation to find out
if the system meets the requirements of existing traffic management policies. This is
cumbersome for most people hence the authors can improve their work by including this vital
information.
This article is very informative and useful in the transportation engineering field because
it contains information that can be used to solve the traffic congestion menace in many urban
Cost is a very important issue in any kind of project. The authors of this article did not
discuss the cost implications of developing or implementing the TCPN model in comparison
with existing traffic light control systems. The system should give users value for money and
probably be more cost effective than other systems already in the market. Cost benefit analysis is
crucial when developing engineering solutions because engineers have the obligation of
developing solutions that improve the quality of people’s life at the lowest cost possible.
Establishing the cost of this kind of system helps to identify areas that can be improved so as to
reduce the cost of production and implementation. Therefore the article could be done better by
including the cost implications of the TCPN model developed.
Last but not least, it must be understood that any engineering product developed has to
meet the requirements of specific codes and regulations. In this case, the traffic light control
system should be able to operate within existing traffic management standards, policies and
regulations. It is the responsibility of researchers to establish whether the products they develop
can be used within existing standards and policies or if there is need to consult relevant agencies
so as to make amendments that will allow these products to be used (Habibian & Kermanshah,
2011). The authors of this article did not state whether the traffic light control system they have
developed is applicable within existing traffic management standards and policies. It means that
any person who is interested in using this system has to conduct further investigation to find out
if the system meets the requirements of existing traffic management policies. This is
cumbersome for most people hence the authors can improve their work by including this vital
information.
This article is very informative and useful in the transportation engineering field because
it contains information that can be used to solve the traffic congestion menace in many urban
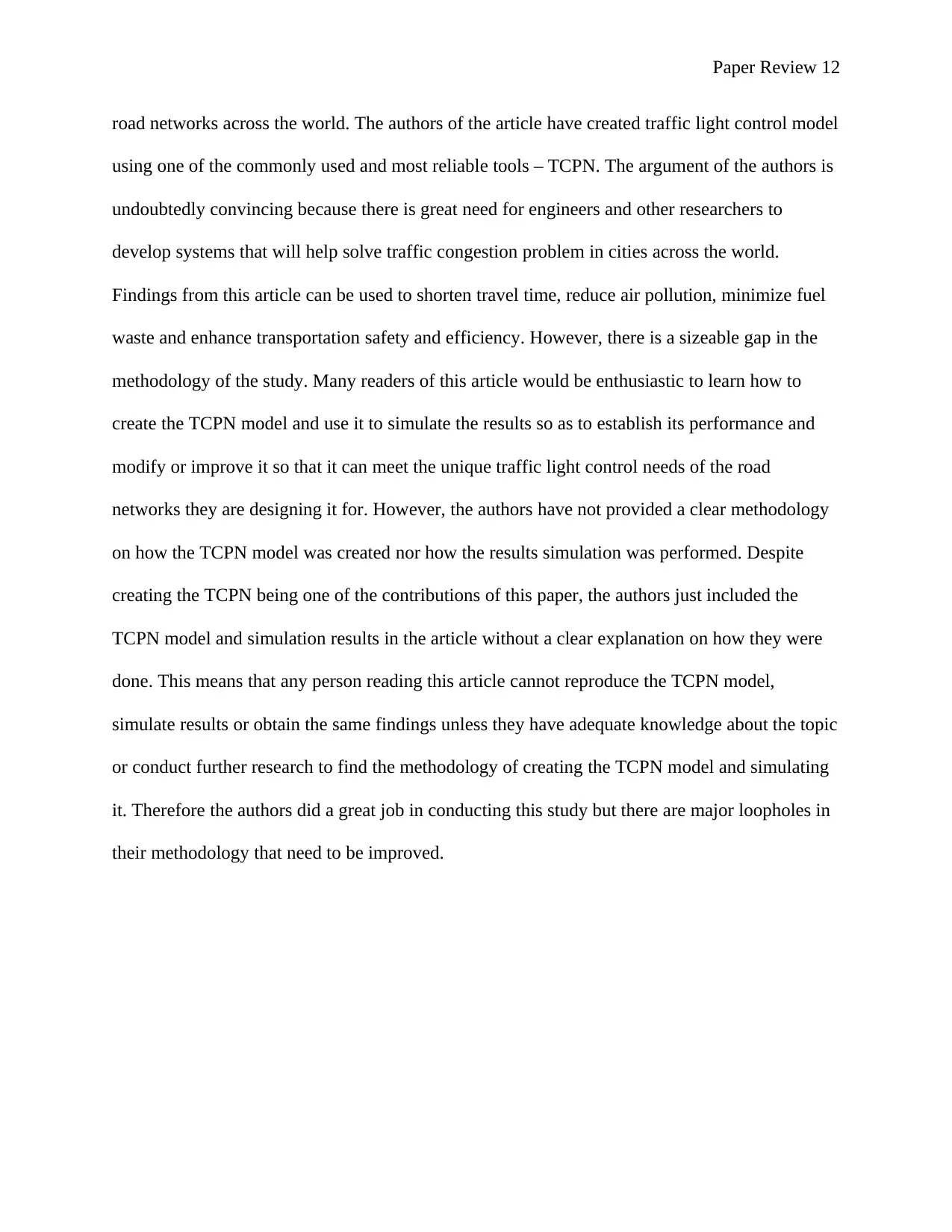
Paper Review 12
road networks across the world. The authors of the article have created traffic light control model
using one of the commonly used and most reliable tools – TCPN. The argument of the authors is
undoubtedly convincing because there is great need for engineers and other researchers to
develop systems that will help solve traffic congestion problem in cities across the world.
Findings from this article can be used to shorten travel time, reduce air pollution, minimize fuel
waste and enhance transportation safety and efficiency. However, there is a sizeable gap in the
methodology of the study. Many readers of this article would be enthusiastic to learn how to
create the TCPN model and use it to simulate the results so as to establish its performance and
modify or improve it so that it can meet the unique traffic light control needs of the road
networks they are designing it for. However, the authors have not provided a clear methodology
on how the TCPN model was created nor how the results simulation was performed. Despite
creating the TCPN being one of the contributions of this paper, the authors just included the
TCPN model and simulation results in the article without a clear explanation on how they were
done. This means that any person reading this article cannot reproduce the TCPN model,
simulate results or obtain the same findings unless they have adequate knowledge about the topic
or conduct further research to find the methodology of creating the TCPN model and simulating
it. Therefore the authors did a great job in conducting this study but there are major loopholes in
their methodology that need to be improved.
road networks across the world. The authors of the article have created traffic light control model
using one of the commonly used and most reliable tools – TCPN. The argument of the authors is
undoubtedly convincing because there is great need for engineers and other researchers to
develop systems that will help solve traffic congestion problem in cities across the world.
Findings from this article can be used to shorten travel time, reduce air pollution, minimize fuel
waste and enhance transportation safety and efficiency. However, there is a sizeable gap in the
methodology of the study. Many readers of this article would be enthusiastic to learn how to
create the TCPN model and use it to simulate the results so as to establish its performance and
modify or improve it so that it can meet the unique traffic light control needs of the road
networks they are designing it for. However, the authors have not provided a clear methodology
on how the TCPN model was created nor how the results simulation was performed. Despite
creating the TCPN being one of the contributions of this paper, the authors just included the
TCPN model and simulation results in the article without a clear explanation on how they were
done. This means that any person reading this article cannot reproduce the TCPN model,
simulate results or obtain the same findings unless they have adequate knowledge about the topic
or conduct further research to find the methodology of creating the TCPN model and simulating
it. Therefore the authors did a great job in conducting this study but there are major loopholes in
their methodology that need to be improved.
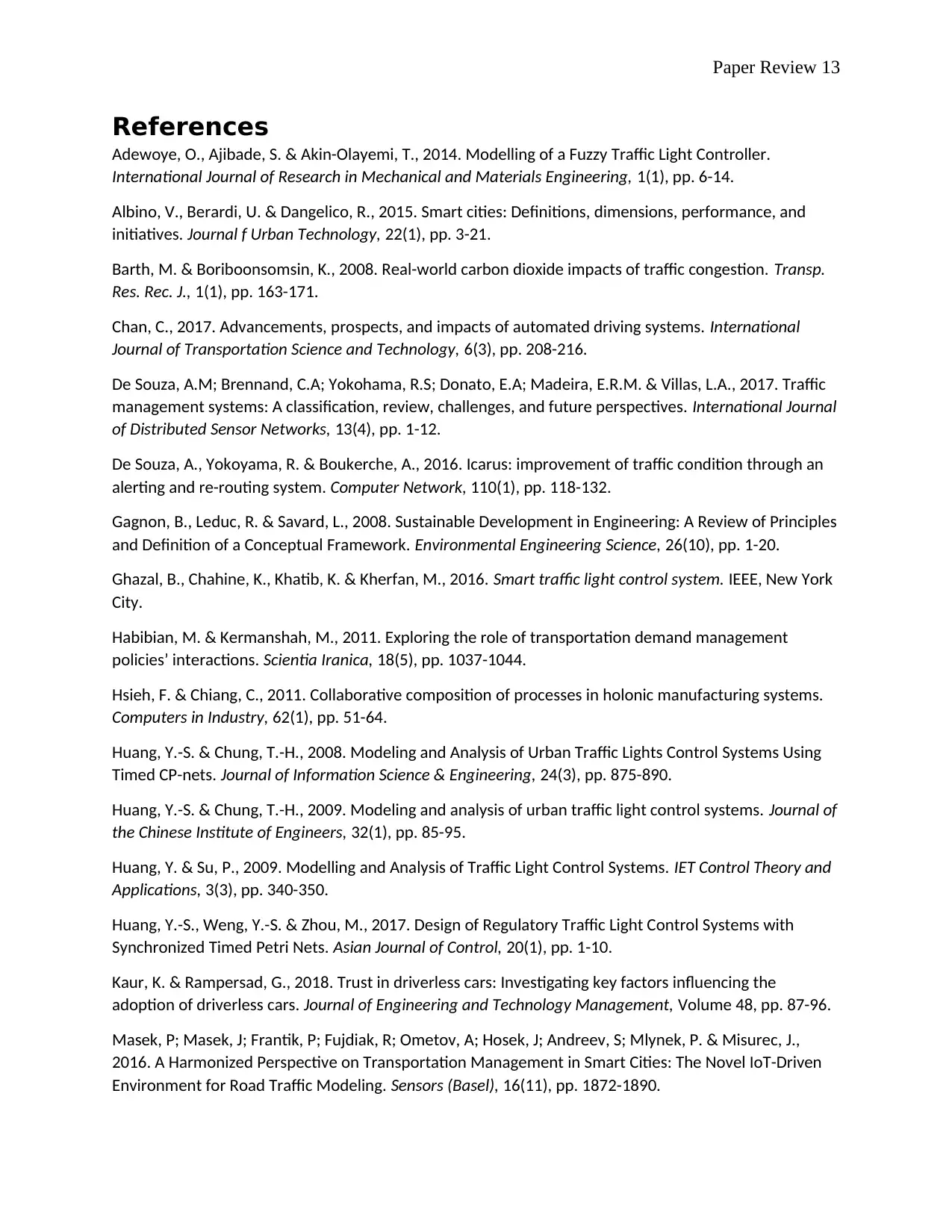
Paper Review 13
References
Adewoye, O., Ajibade, S. & Akin-Olayemi, T., 2014. Modelling of a Fuzzy Traffic Light Controller.
International Journal of Research in Mechanical and Materials Engineering, 1(1), pp. 6-14.
Albino, V., Berardi, U. & Dangelico, R., 2015. Smart cities: Definitions, dimensions, performance, and
initiatives. Journal f Urban Technology, 22(1), pp. 3-21.
Barth, M. & Boriboonsomsin, K., 2008. Real-world carbon dioxide impacts of traffic congestion. Transp.
Res. Rec. J., 1(1), pp. 163-171.
Chan, C., 2017. Advancements, prospects, and impacts of automated driving systems. International
Journal of Transportation Science and Technology, 6(3), pp. 208-216.
De Souza, A.M; Brennand, C.A; Yokohama, R.S; Donato, E.A; Madeira, E.R.M. & Villas, L.A., 2017. Traffic
management systems: A classification, review, challenges, and future perspectives. International Journal
of Distributed Sensor Networks, 13(4), pp. 1-12.
De Souza, A., Yokoyama, R. & Boukerche, A., 2016. Icarus: improvement of traffic condition through an
alerting and re-routing system. Computer Network, 110(1), pp. 118-132.
Gagnon, B., Leduc, R. & Savard, L., 2008. Sustainable Development in Engineering: A Review of Principles
and Definition of a Conceptual Framework. Environmental Engineering Science, 26(10), pp. 1-20.
Ghazal, B., Chahine, K., Khatib, K. & Kherfan, M., 2016. Smart traffic light control system. IEEE, New York
City.
Habibian, M. & Kermanshah, M., 2011. Exploring the role of transportation demand management
policies’ interactions. Scientia Iranica, 18(5), pp. 1037-1044.
Hsieh, F. & Chiang, C., 2011. Collaborative composition of processes in holonic manufacturing systems.
Computers in Industry, 62(1), pp. 51-64.
Huang, Y.-S. & Chung, T.-H., 2008. Modeling and Analysis of Urban Traffic Lights Control Systems Using
Timed CP-nets. Journal of Information Science & Engineering, 24(3), pp. 875-890.
Huang, Y.-S. & Chung, T.-H., 2009. Modeling and analysis of urban traffic light control systems. Journal of
the Chinese Institute of Engineers, 32(1), pp. 85-95.
Huang, Y. & Su, P., 2009. Modelling and Analysis of Traffic Light Control Systems. IET Control Theory and
Applications, 3(3), pp. 340-350.
Huang, Y.-S., Weng, Y.-S. & Zhou, M., 2017. Design of Regulatory Traffic Light Control Systems with
Synchronized Timed Petri Nets. Asian Journal of Control, 20(1), pp. 1-10.
Kaur, K. & Rampersad, G., 2018. Trust in driverless cars: Investigating key factors influencing the
adoption of driverless cars. Journal of Engineering and Technology Management, Volume 48, pp. 87-96.
Masek, P; Masek, J; Frantik, P; Fujdiak, R; Ometov, A; Hosek, J; Andreev, S; Mlynek, P. & Misurec, J.,
2016. A Harmonized Perspective on Transportation Management in Smart Cities: The Novel IoT-Driven
Environment for Road Traffic Modeling. Sensors (Basel), 16(11), pp. 1872-1890.
References
Adewoye, O., Ajibade, S. & Akin-Olayemi, T., 2014. Modelling of a Fuzzy Traffic Light Controller.
International Journal of Research in Mechanical and Materials Engineering, 1(1), pp. 6-14.
Albino, V., Berardi, U. & Dangelico, R., 2015. Smart cities: Definitions, dimensions, performance, and
initiatives. Journal f Urban Technology, 22(1), pp. 3-21.
Barth, M. & Boriboonsomsin, K., 2008. Real-world carbon dioxide impacts of traffic congestion. Transp.
Res. Rec. J., 1(1), pp. 163-171.
Chan, C., 2017. Advancements, prospects, and impacts of automated driving systems. International
Journal of Transportation Science and Technology, 6(3), pp. 208-216.
De Souza, A.M; Brennand, C.A; Yokohama, R.S; Donato, E.A; Madeira, E.R.M. & Villas, L.A., 2017. Traffic
management systems: A classification, review, challenges, and future perspectives. International Journal
of Distributed Sensor Networks, 13(4), pp. 1-12.
De Souza, A., Yokoyama, R. & Boukerche, A., 2016. Icarus: improvement of traffic condition through an
alerting and re-routing system. Computer Network, 110(1), pp. 118-132.
Gagnon, B., Leduc, R. & Savard, L., 2008. Sustainable Development in Engineering: A Review of Principles
and Definition of a Conceptual Framework. Environmental Engineering Science, 26(10), pp. 1-20.
Ghazal, B., Chahine, K., Khatib, K. & Kherfan, M., 2016. Smart traffic light control system. IEEE, New York
City.
Habibian, M. & Kermanshah, M., 2011. Exploring the role of transportation demand management
policies’ interactions. Scientia Iranica, 18(5), pp. 1037-1044.
Hsieh, F. & Chiang, C., 2011. Collaborative composition of processes in holonic manufacturing systems.
Computers in Industry, 62(1), pp. 51-64.
Huang, Y.-S. & Chung, T.-H., 2008. Modeling and Analysis of Urban Traffic Lights Control Systems Using
Timed CP-nets. Journal of Information Science & Engineering, 24(3), pp. 875-890.
Huang, Y.-S. & Chung, T.-H., 2009. Modeling and analysis of urban traffic light control systems. Journal of
the Chinese Institute of Engineers, 32(1), pp. 85-95.
Huang, Y. & Su, P., 2009. Modelling and Analysis of Traffic Light Control Systems. IET Control Theory and
Applications, 3(3), pp. 340-350.
Huang, Y.-S., Weng, Y.-S. & Zhou, M., 2017. Design of Regulatory Traffic Light Control Systems with
Synchronized Timed Petri Nets. Asian Journal of Control, 20(1), pp. 1-10.
Kaur, K. & Rampersad, G., 2018. Trust in driverless cars: Investigating key factors influencing the
adoption of driverless cars. Journal of Engineering and Technology Management, Volume 48, pp. 87-96.
Masek, P; Masek, J; Frantik, P; Fujdiak, R; Ometov, A; Hosek, J; Andreev, S; Mlynek, P. & Misurec, J.,
2016. A Harmonized Perspective on Transportation Management in Smart Cities: The Novel IoT-Driven
Environment for Road Traffic Modeling. Sensors (Basel), 16(11), pp. 1872-1890.
Paraphrase This Document
Need a fresh take? Get an instant paraphrase of this document with our AI Paraphraser
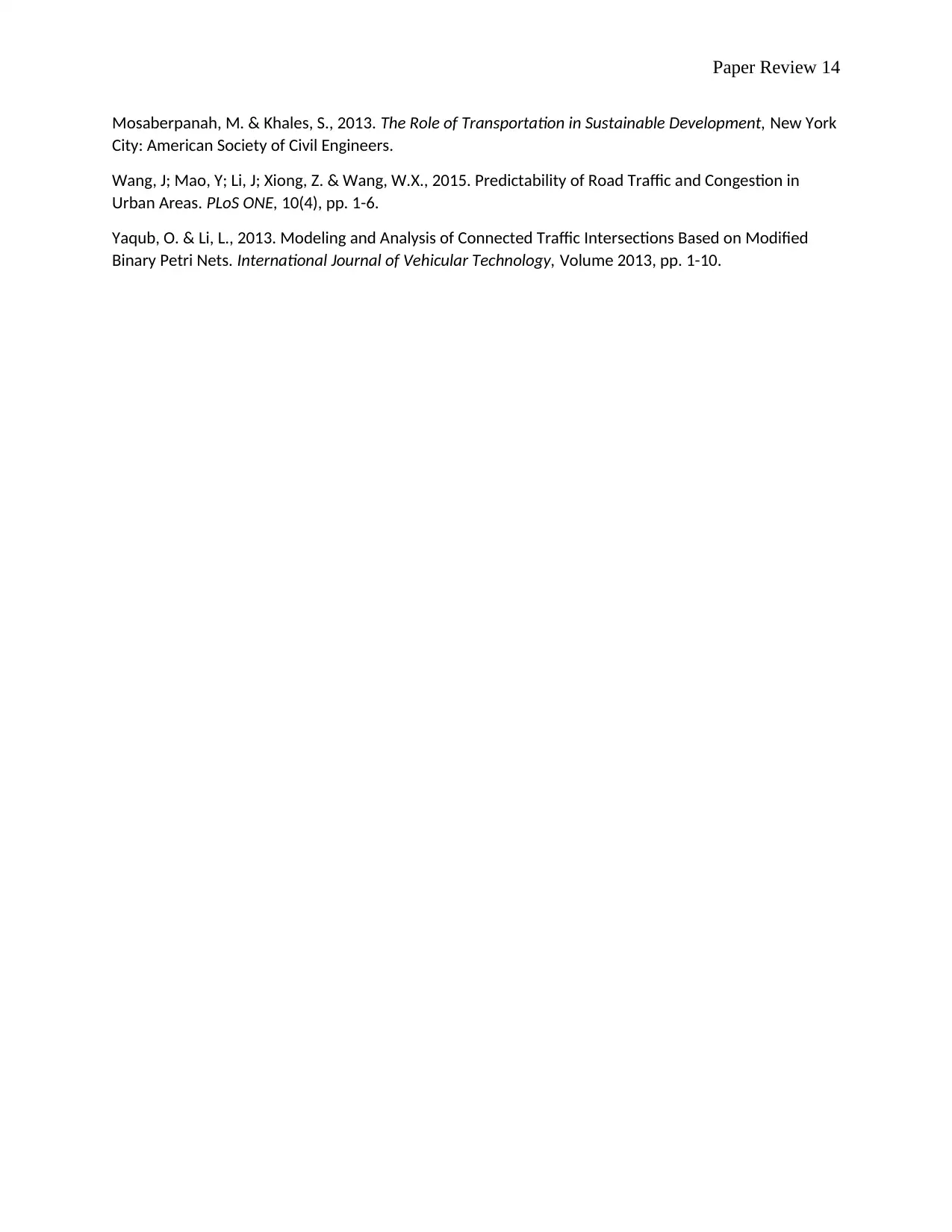
Paper Review 14
Mosaberpanah, M. & Khales, S., 2013. The Role of Transportation in Sustainable Development, New York
City: American Society of Civil Engineers.
Wang, J; Mao, Y; Li, J; Xiong, Z. & Wang, W.X., 2015. Predictability of Road Traffic and Congestion in
Urban Areas. PLoS ONE, 10(4), pp. 1-6.
Yaqub, O. & Li, L., 2013. Modeling and Analysis of Connected Traffic Intersections Based on Modified
Binary Petri Nets. International Journal of Vehicular Technology, Volume 2013, pp. 1-10.
Mosaberpanah, M. & Khales, S., 2013. The Role of Transportation in Sustainable Development, New York
City: American Society of Civil Engineers.
Wang, J; Mao, Y; Li, J; Xiong, Z. & Wang, W.X., 2015. Predictability of Road Traffic and Congestion in
Urban Areas. PLoS ONE, 10(4), pp. 1-6.
Yaqub, O. & Li, L., 2013. Modeling and Analysis of Connected Traffic Intersections Based on Modified
Binary Petri Nets. International Journal of Vehicular Technology, Volume 2013, pp. 1-10.
1 out of 14
![[object Object]](/_next/image/?url=%2F_next%2Fstatic%2Fmedia%2Flogo.6d15ce61.png&w=640&q=75)
Your All-in-One AI-Powered Toolkit for Academic Success.
+13062052269
info@desklib.com
Available 24*7 on WhatsApp / Email
Unlock your academic potential
© 2024 | Zucol Services PVT LTD | All rights reserved.