Requirement Analysis and Modeling: Nike Revenue Forecasting Project
VerifiedAdded on 2023/06/10
|14
|1538
|318
Project
AI Summary
This assignment presents a comprehensive analysis of Nike's quarterly revenue, employing various forecasting and regression techniques. The project begins with an examination of Nike's revenue in relation to e-commerce sales, utilizing least squares regression to establish a correlation. Furthermore, the document explores time series forecasting methods, including double exponential smoothing, to project future revenue. The project delves into multiple regression analyses, assessing the impact of various economic indicators such as US GDP, China GDP, US employment rates, and consumer price indices on Nike's revenue. The analysis includes detailed regression tables, forecast plots, and accuracy measures to validate the models. The report incorporates data from 2011Q2 to 2018Q2 and provides forecasts for Nike's revenue through Q4 2019, offering insights into the relationship between economic factors and the company's financial performance.
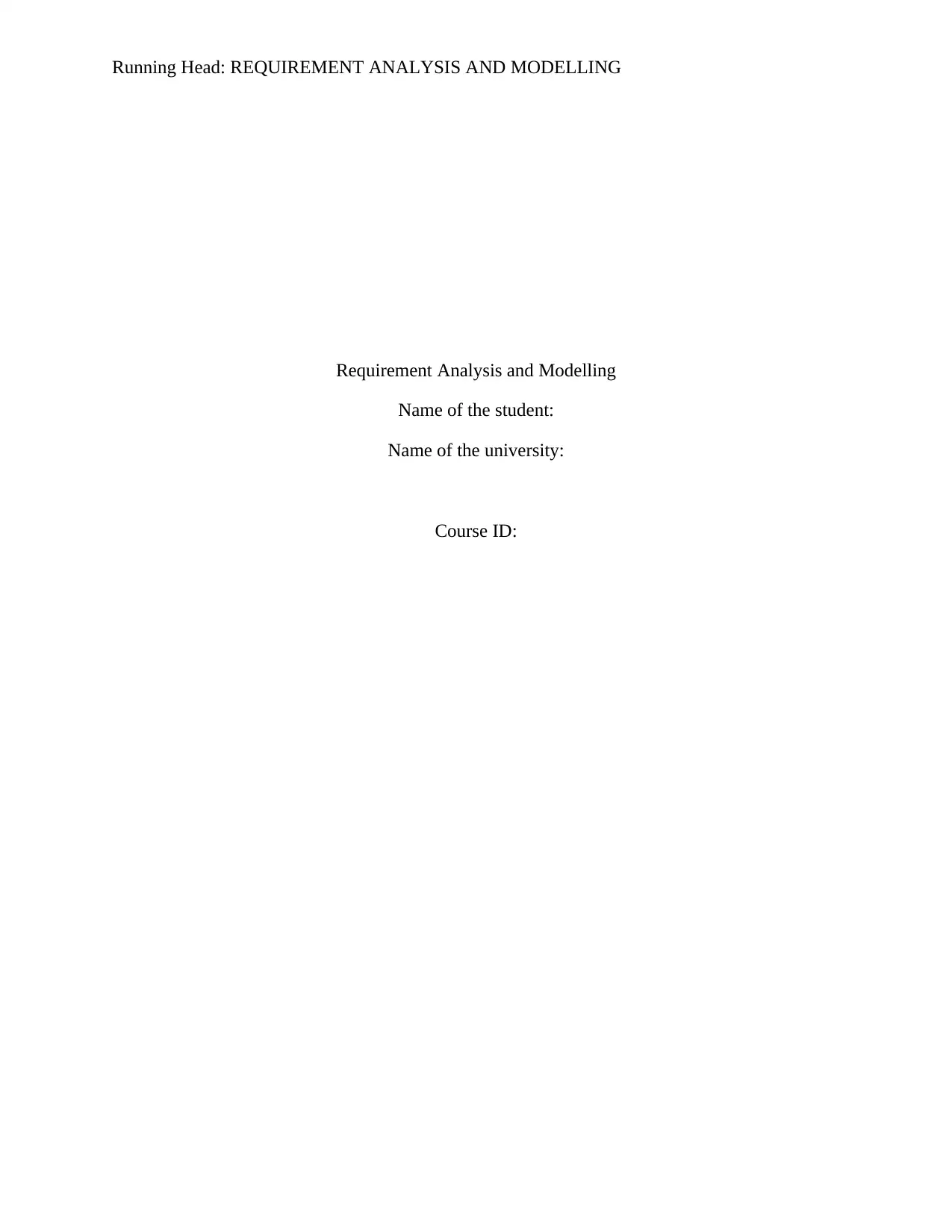
Running Head: REQUIREMENT ANALYSIS AND MODELLING
Requirement Analysis and Modelling
Name of the student:
Name of the university:
Course ID:
Requirement Analysis and Modelling
Name of the student:
Name of the university:
Course ID:
Paraphrase This Document
Need a fresh take? Get an instant paraphrase of this document with our AI Paraphraser
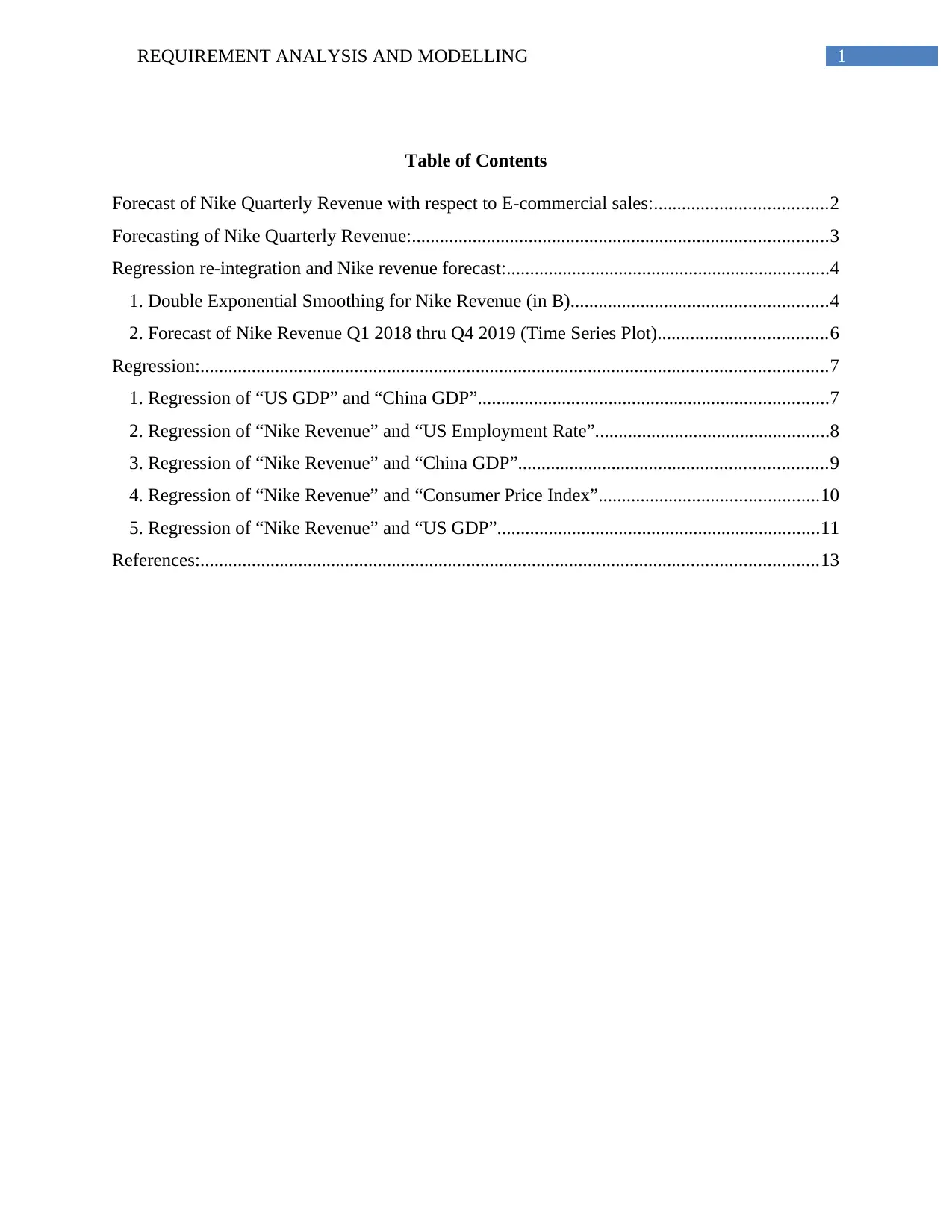
1REQUIREMENT ANALYSIS AND MODELLING
Table of Contents
Forecast of Nike Quarterly Revenue with respect to E-commercial sales:.....................................2
Forecasting of Nike Quarterly Revenue:.........................................................................................3
Regression re-integration and Nike revenue forecast:.....................................................................4
1. Double Exponential Smoothing for Nike Revenue (in B).......................................................4
2. Forecast of Nike Revenue Q1 2018 thru Q4 2019 (Time Series Plot)....................................6
Regression:......................................................................................................................................7
1. Regression of “US GDP” and “China GDP”...........................................................................7
2. Regression of “Nike Revenue” and “US Employment Rate”..................................................8
3. Regression of “Nike Revenue” and “China GDP”..................................................................9
4. Regression of “Nike Revenue” and “Consumer Price Index”...............................................10
5. Regression of “Nike Revenue” and “US GDP”.....................................................................11
References:....................................................................................................................................13
Table of Contents
Forecast of Nike Quarterly Revenue with respect to E-commercial sales:.....................................2
Forecasting of Nike Quarterly Revenue:.........................................................................................3
Regression re-integration and Nike revenue forecast:.....................................................................4
1. Double Exponential Smoothing for Nike Revenue (in B).......................................................4
2. Forecast of Nike Revenue Q1 2018 thru Q4 2019 (Time Series Plot)....................................6
Regression:......................................................................................................................................7
1. Regression of “US GDP” and “China GDP”...........................................................................7
2. Regression of “Nike Revenue” and “US Employment Rate”..................................................8
3. Regression of “Nike Revenue” and “China GDP”..................................................................9
4. Regression of “Nike Revenue” and “Consumer Price Index”...............................................10
5. Regression of “Nike Revenue” and “US GDP”.....................................................................11
References:....................................................................................................................................13
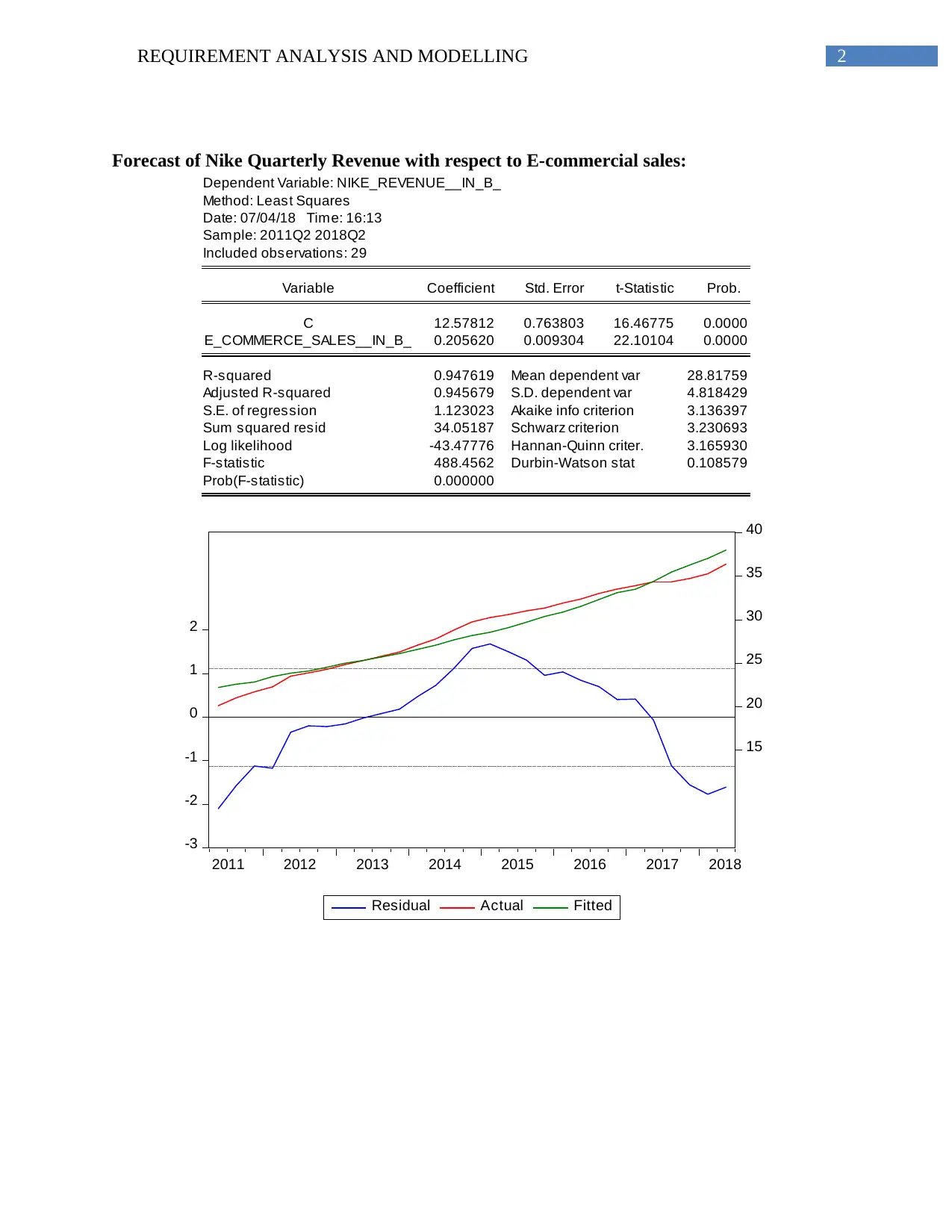
2REQUIREMENT ANALYSIS AND MODELLING
Forecast of Nike Quarterly Revenue with respect to E-commercial sales:
Dependent Variable: NIKE_REVENUE__IN_B_
Method: Least Squares
Date: 07/04/18 Time: 16:13
Sample: 2011Q2 2018Q2
Included observations: 29
Variable Coefficient Std. Error t-Statistic Prob.
C 12.57812 0.763803 16.46775 0.0000
E_COMMERCE_SALES__IN_B_ 0.205620 0.009304 22.10104 0.0000
R-squared 0.947619 Mean dependent var 28.81759
Adjusted R-squared 0.945679 S.D. dependent var 4.818429
S.E. of regression 1.123023 Akaike info criterion 3.136397
Sum squared resid 34.05187 Schwarz criterion 3.230693
Log likelihood -43.47776 Hannan-Quinn criter. 3.165930
F-statistic 488.4562 Durbin-Watson stat 0.108579
Prob(F-statistic) 0.000000
-3
-2
-1
0
1
2
15
20
25
30
35
40
2011 2012 2013 2014 2015 2016 2017 2018
Residual Actual Fitted
Forecast of Nike Quarterly Revenue with respect to E-commercial sales:
Dependent Variable: NIKE_REVENUE__IN_B_
Method: Least Squares
Date: 07/04/18 Time: 16:13
Sample: 2011Q2 2018Q2
Included observations: 29
Variable Coefficient Std. Error t-Statistic Prob.
C 12.57812 0.763803 16.46775 0.0000
E_COMMERCE_SALES__IN_B_ 0.205620 0.009304 22.10104 0.0000
R-squared 0.947619 Mean dependent var 28.81759
Adjusted R-squared 0.945679 S.D. dependent var 4.818429
S.E. of regression 1.123023 Akaike info criterion 3.136397
Sum squared resid 34.05187 Schwarz criterion 3.230693
Log likelihood -43.47776 Hannan-Quinn criter. 3.165930
F-statistic 488.4562 Durbin-Watson stat 0.108579
Prob(F-statistic) 0.000000
-3
-2
-1
0
1
2
15
20
25
30
35
40
2011 2012 2013 2014 2015 2016 2017 2018
Residual Actual Fitted
⊘ This is a preview!⊘
Do you want full access?
Subscribe today to unlock all pages.

Trusted by 1+ million students worldwide
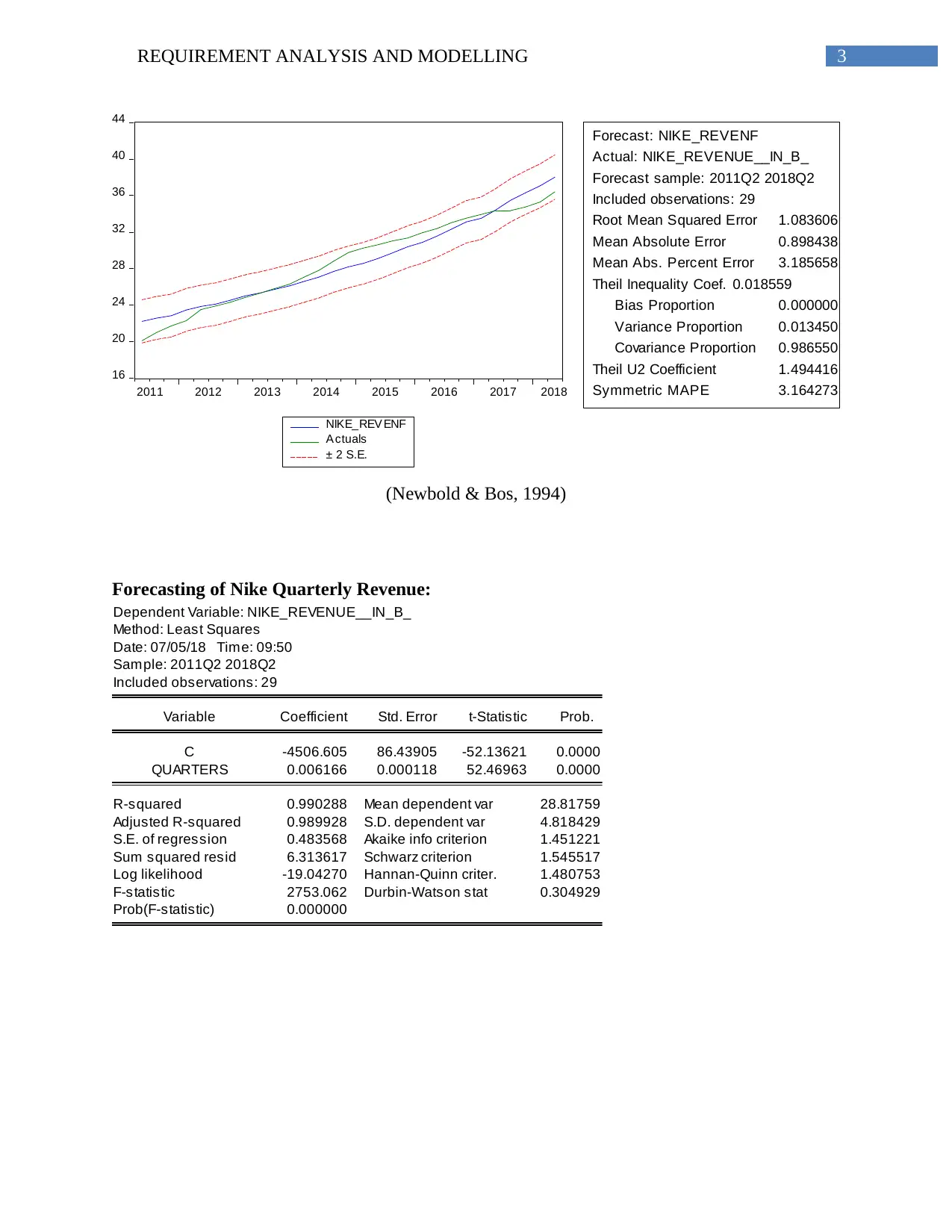
3REQUIREMENT ANALYSIS AND MODELLING
16
20
24
28
32
36
40
44
2011 2012 2013 2014 2015 2016 2017 2018
NIKE_REV ENF
A ctuals
± 2 S.E.
Forecast: NIKE_REVENF
Actual: NIKE_REVENUE__IN_B_
Forecast sample: 2011Q2 2018Q2
Included observations: 29
Root Mean Squared Error 1.083606
Mean Absolute Error 0.898438
Mean Abs. Percent Error 3.185658
Theil Inequality Coef. 0.018559
Bias Proportion 0.000000
Variance Proportion 0.013450
Covariance Proportion 0.986550
Theil U2 Coefficient 1.494416
Symmetric MAPE 3.164273
(Newbold & Bos, 1994)
Forecasting of Nike Quarterly Revenue:
Dependent Variable: NIKE_REVENUE__IN_B_
Method: Least Squares
Date: 07/05/18 Time: 09:50
Sample: 2011Q2 2018Q2
Included observations: 29
Variable Coefficient Std. Error t-Statistic Prob.
C -4506.605 86.43905 -52.13621 0.0000
QUARTERS 0.006166 0.000118 52.46963 0.0000
R-squared 0.990288 Mean dependent var 28.81759
Adjusted R-squared 0.989928 S.D. dependent var 4.818429
S.E. of regression 0.483568 Akaike info criterion 1.451221
Sum squared resid 6.313617 Schwarz criterion 1.545517
Log likelihood -19.04270 Hannan-Quinn criter. 1.480753
F-statistic 2753.062 Durbin-Watson stat 0.304929
Prob(F-statistic) 0.000000
16
20
24
28
32
36
40
44
2011 2012 2013 2014 2015 2016 2017 2018
NIKE_REV ENF
A ctuals
± 2 S.E.
Forecast: NIKE_REVENF
Actual: NIKE_REVENUE__IN_B_
Forecast sample: 2011Q2 2018Q2
Included observations: 29
Root Mean Squared Error 1.083606
Mean Absolute Error 0.898438
Mean Abs. Percent Error 3.185658
Theil Inequality Coef. 0.018559
Bias Proportion 0.000000
Variance Proportion 0.013450
Covariance Proportion 0.986550
Theil U2 Coefficient 1.494416
Symmetric MAPE 3.164273
(Newbold & Bos, 1994)
Forecasting of Nike Quarterly Revenue:
Dependent Variable: NIKE_REVENUE__IN_B_
Method: Least Squares
Date: 07/05/18 Time: 09:50
Sample: 2011Q2 2018Q2
Included observations: 29
Variable Coefficient Std. Error t-Statistic Prob.
C -4506.605 86.43905 -52.13621 0.0000
QUARTERS 0.006166 0.000118 52.46963 0.0000
R-squared 0.990288 Mean dependent var 28.81759
Adjusted R-squared 0.989928 S.D. dependent var 4.818429
S.E. of regression 0.483568 Akaike info criterion 1.451221
Sum squared resid 6.313617 Schwarz criterion 1.545517
Log likelihood -19.04270 Hannan-Quinn criter. 1.480753
F-statistic 2753.062 Durbin-Watson stat 0.304929
Prob(F-statistic) 0.000000
Paraphrase This Document
Need a fresh take? Get an instant paraphrase of this document with our AI Paraphraser
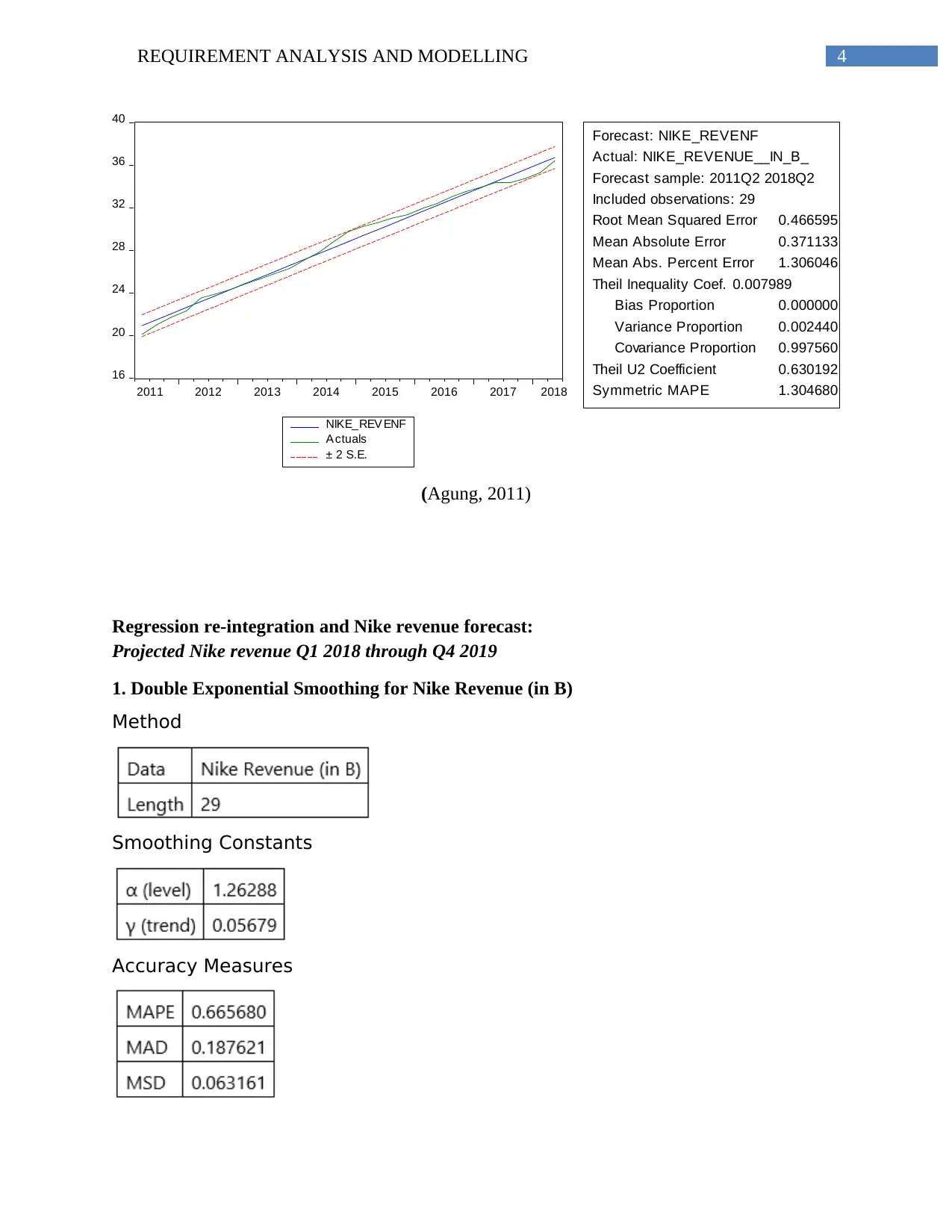
4REQUIREMENT ANALYSIS AND MODELLING
16
20
24
28
32
36
40
2011 2012 2013 2014 2015 2016 2017 2018
NIKE_REV ENF
A ctuals
± 2 S.E.
Forecast: NIKE_REVENF
Actual: NIKE_REVENUE__IN_B_
Forecast sample: 2011Q2 2018Q2
Included observations: 29
Root Mean Squared Error 0.466595
Mean Absolute Error 0.371133
Mean Abs. Percent Error 1.306046
Theil Inequality Coef. 0.007989
Bias Proportion 0.000000
Variance Proportion 0.002440
Covariance Proportion 0.997560
Theil U2 Coefficient 0.630192
Symmetric MAPE 1.304680
(Agung, 2011)
Regression re-integration and Nike revenue forecast:
Projected Nike revenue Q1 2018 through Q4 2019
1. Double Exponential Smoothing for Nike Revenue (in B)
Method
Smoothing Constants
Accuracy Measures
16
20
24
28
32
36
40
2011 2012 2013 2014 2015 2016 2017 2018
NIKE_REV ENF
A ctuals
± 2 S.E.
Forecast: NIKE_REVENF
Actual: NIKE_REVENUE__IN_B_
Forecast sample: 2011Q2 2018Q2
Included observations: 29
Root Mean Squared Error 0.466595
Mean Absolute Error 0.371133
Mean Abs. Percent Error 1.306046
Theil Inequality Coef. 0.007989
Bias Proportion 0.000000
Variance Proportion 0.002440
Covariance Proportion 0.997560
Theil U2 Coefficient 0.630192
Symmetric MAPE 1.304680
(Agung, 2011)
Regression re-integration and Nike revenue forecast:
Projected Nike revenue Q1 2018 through Q4 2019
1. Double Exponential Smoothing for Nike Revenue (in B)
Method
Smoothing Constants
Accuracy Measures
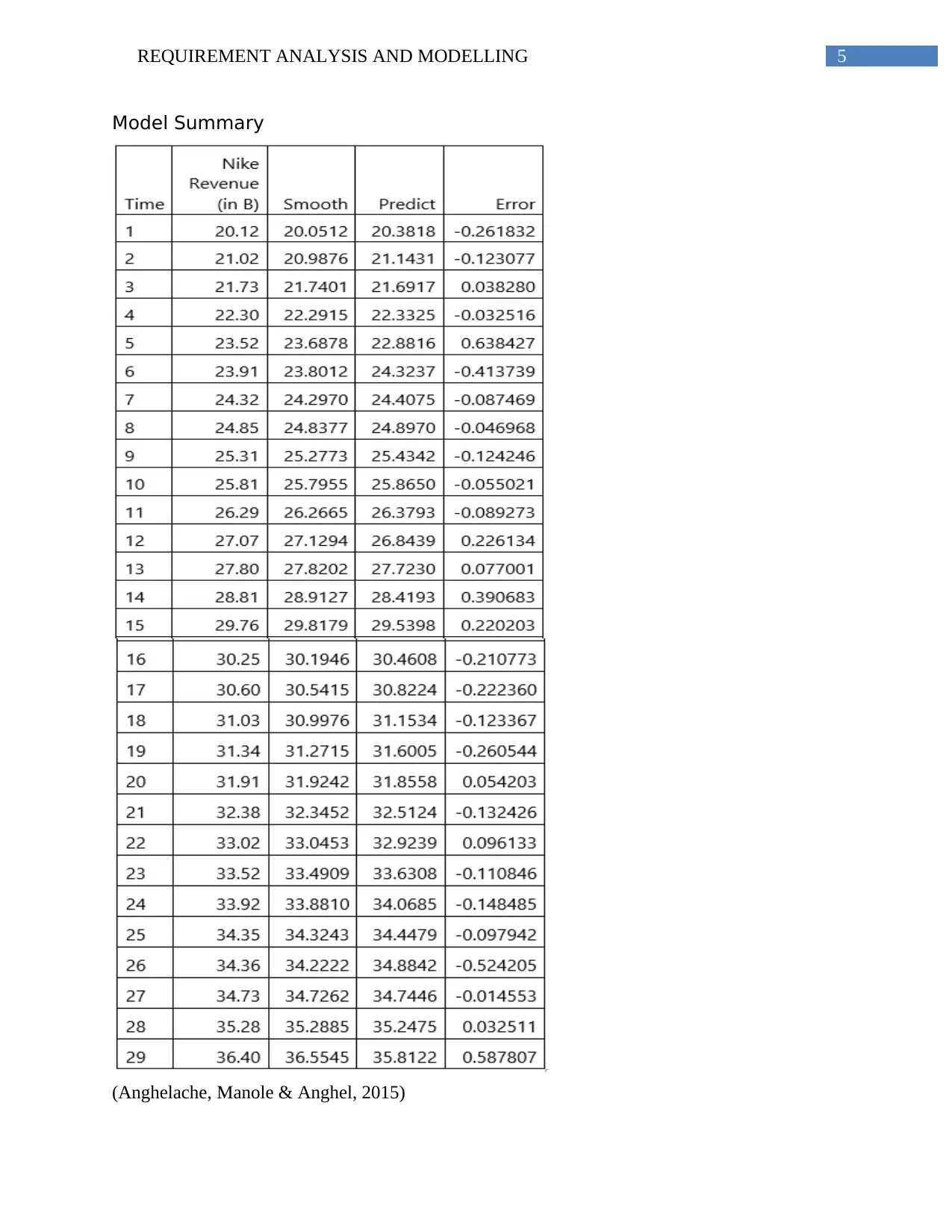
5REQUIREMENT ANALYSIS AND MODELLING
Model Summary
(Anghelache, Manole & Anghel, 2015)
Model Summary
(Anghelache, Manole & Anghel, 2015)
⊘ This is a preview!⊘
Do you want full access?
Subscribe today to unlock all pages.

Trusted by 1+ million students worldwide
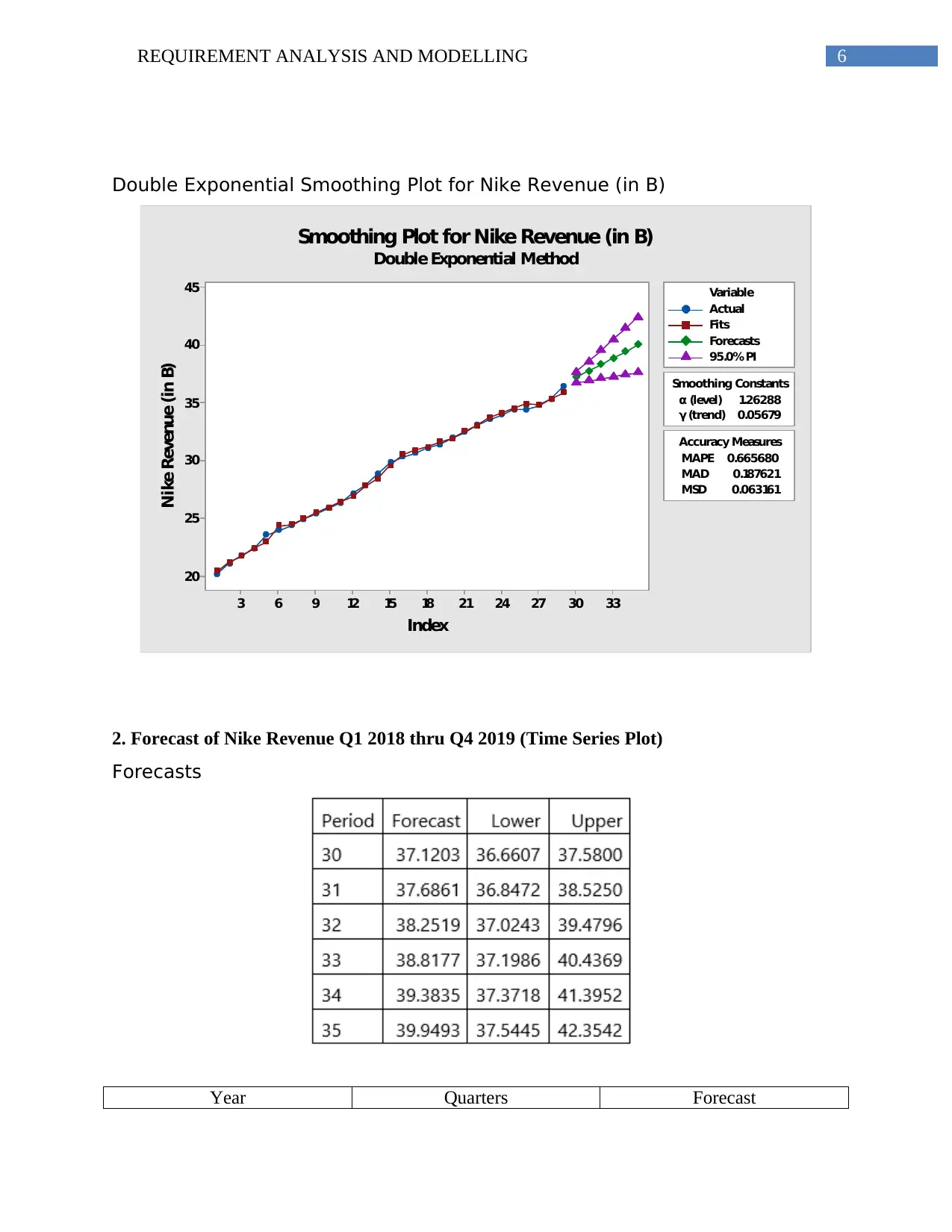
6REQUIREMENT ANALYSIS AND MODELLING
Double Exponential Smoothing Plot for Nike Revenue (in B)
3330272421181512963
45
40
35
30
25
20
α (level) 1.26288
γ (trend) 0.05679
Smoothing Constants
MAPE 0.665680
MAD 0.187621
MSD 0.063161
Accuracy Measures
Index
Nike Revenue (in B)
Actual
Fits
Forecasts
95.0% PI
Variable
Smoothing Plot for Nike Revenue (in B)
Double Exponential Method
2. Forecast of Nike Revenue Q1 2018 thru Q4 2019 (Time Series Plot)
Forecasts
Year Quarters Forecast
Double Exponential Smoothing Plot for Nike Revenue (in B)
3330272421181512963
45
40
35
30
25
20
α (level) 1.26288
γ (trend) 0.05679
Smoothing Constants
MAPE 0.665680
MAD 0.187621
MSD 0.063161
Accuracy Measures
Index
Nike Revenue (in B)
Actual
Fits
Forecasts
95.0% PI
Variable
Smoothing Plot for Nike Revenue (in B)
Double Exponential Method
2. Forecast of Nike Revenue Q1 2018 thru Q4 2019 (Time Series Plot)
Forecasts
Year Quarters Forecast
Paraphrase This Document
Need a fresh take? Get an instant paraphrase of this document with our AI Paraphraser
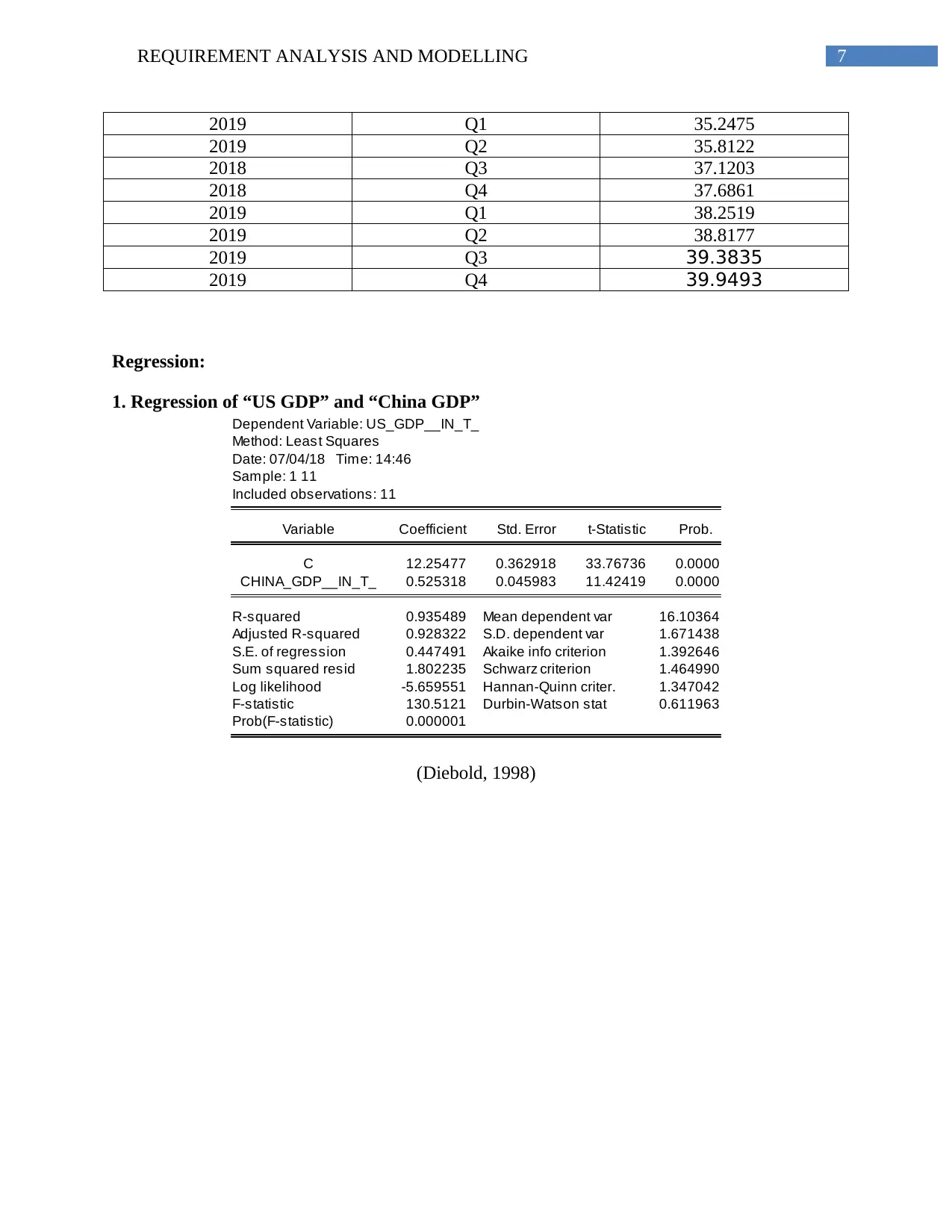
7REQUIREMENT ANALYSIS AND MODELLING
2019 Q1 35.2475
2019 Q2 35.8122
2018 Q3 37.1203
2018 Q4 37.6861
2019 Q1 38.2519
2019 Q2 38.8177
2019 Q3 39.3835
2019 Q4 39.9493
Regression:
1. Regression of “US GDP” and “China GDP”
Dependent Variable: US_GDP__IN_T_
Method: Least Squares
Date: 07/04/18 Time: 14:46
Sample: 1 11
Included observations: 11
Variable Coefficient Std. Error t-Statistic Prob.
C 12.25477 0.362918 33.76736 0.0000
CHINA_GDP__IN_T_ 0.525318 0.045983 11.42419 0.0000
R-squared 0.935489 Mean dependent var 16.10364
Adjusted R-squared 0.928322 S.D. dependent var 1.671438
S.E. of regression 0.447491 Akaike info criterion 1.392646
Sum squared resid 1.802235 Schwarz criterion 1.464990
Log likelihood -5.659551 Hannan-Quinn criter. 1.347042
F-statistic 130.5121 Durbin-Watson stat 0.611963
Prob(F-statistic) 0.000001
(Diebold, 1998)
2019 Q1 35.2475
2019 Q2 35.8122
2018 Q3 37.1203
2018 Q4 37.6861
2019 Q1 38.2519
2019 Q2 38.8177
2019 Q3 39.3835
2019 Q4 39.9493
Regression:
1. Regression of “US GDP” and “China GDP”
Dependent Variable: US_GDP__IN_T_
Method: Least Squares
Date: 07/04/18 Time: 14:46
Sample: 1 11
Included observations: 11
Variable Coefficient Std. Error t-Statistic Prob.
C 12.25477 0.362918 33.76736 0.0000
CHINA_GDP__IN_T_ 0.525318 0.045983 11.42419 0.0000
R-squared 0.935489 Mean dependent var 16.10364
Adjusted R-squared 0.928322 S.D. dependent var 1.671438
S.E. of regression 0.447491 Akaike info criterion 1.392646
Sum squared resid 1.802235 Schwarz criterion 1.464990
Log likelihood -5.659551 Hannan-Quinn criter. 1.347042
F-statistic 130.5121 Durbin-Watson stat 0.611963
Prob(F-statistic) 0.000001
(Diebold, 1998)
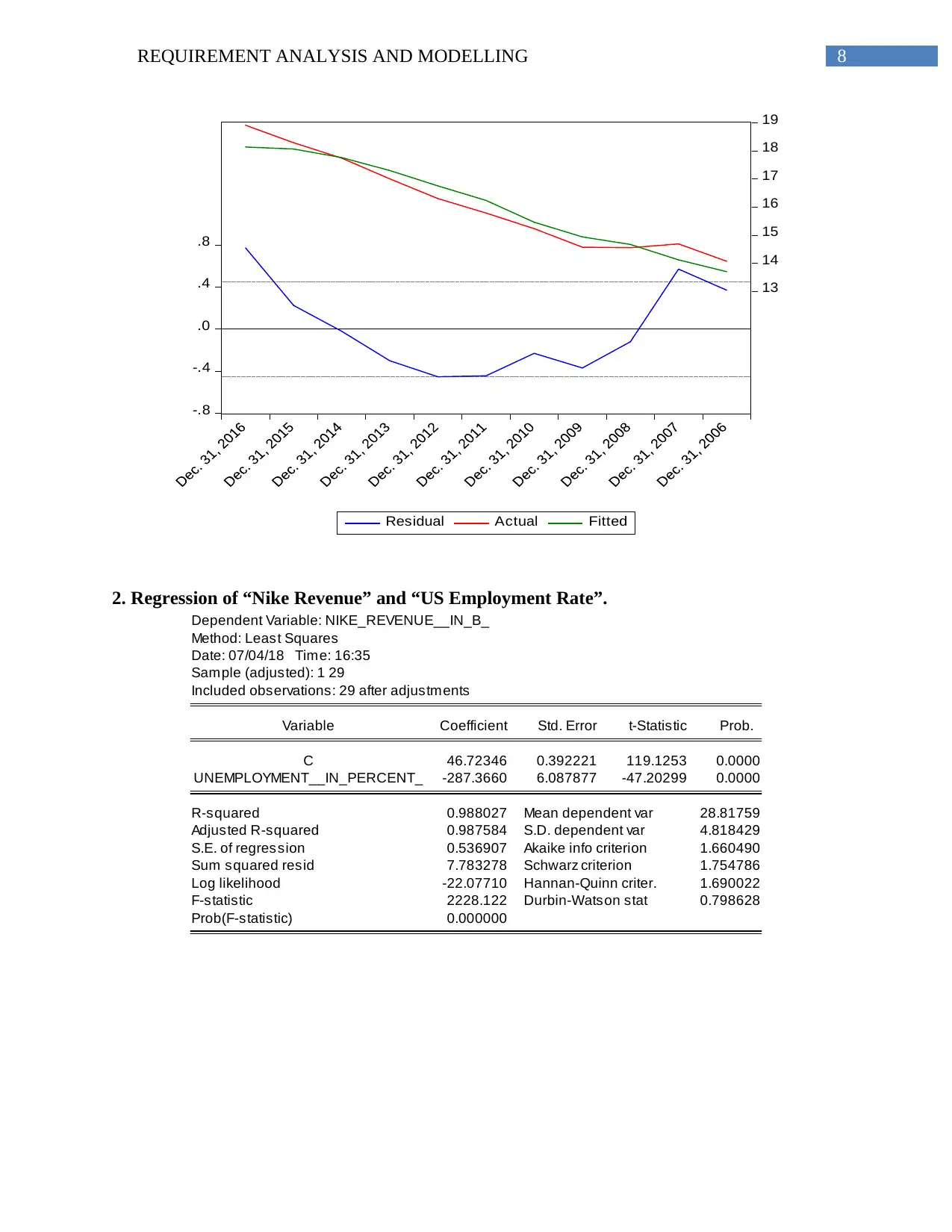
8REQUIREMENT ANALYSIS AND MODELLING
-.8
-.4
.0
.4
.8
13
14
15
16
17
18
19
Residual Actual Fitted
2. Regression of “Nike Revenue” and “US Employment Rate”.
Dependent Variable: NIKE_REVENUE__IN_B_
Method: Least Squares
Date: 07/04/18 Time: 16:35
Sample (adjusted): 1 29
Included observations: 29 after adjustments
Variable Coefficient Std. Error t-Statistic Prob.
C 46.72346 0.392221 119.1253 0.0000
UNEMPLOYMENT__IN_PERCENT_ -287.3660 6.087877 -47.20299 0.0000
R-squared 0.988027 Mean dependent var 28.81759
Adjusted R-squared 0.987584 S.D. dependent var 4.818429
S.E. of regression 0.536907 Akaike info criterion 1.660490
Sum squared resid 7.783278 Schwarz criterion 1.754786
Log likelihood -22.07710 Hannan-Quinn criter. 1.690022
F-statistic 2228.122 Durbin-Watson stat 0.798628
Prob(F-statistic) 0.000000
-.8
-.4
.0
.4
.8
13
14
15
16
17
18
19
Residual Actual Fitted
2. Regression of “Nike Revenue” and “US Employment Rate”.
Dependent Variable: NIKE_REVENUE__IN_B_
Method: Least Squares
Date: 07/04/18 Time: 16:35
Sample (adjusted): 1 29
Included observations: 29 after adjustments
Variable Coefficient Std. Error t-Statistic Prob.
C 46.72346 0.392221 119.1253 0.0000
UNEMPLOYMENT__IN_PERCENT_ -287.3660 6.087877 -47.20299 0.0000
R-squared 0.988027 Mean dependent var 28.81759
Adjusted R-squared 0.987584 S.D. dependent var 4.818429
S.E. of regression 0.536907 Akaike info criterion 1.660490
Sum squared resid 7.783278 Schwarz criterion 1.754786
Log likelihood -22.07710 Hannan-Quinn criter. 1.690022
F-statistic 2228.122 Durbin-Watson stat 0.798628
Prob(F-statistic) 0.000000
⊘ This is a preview!⊘
Do you want full access?
Subscribe today to unlock all pages.

Trusted by 1+ million students worldwide
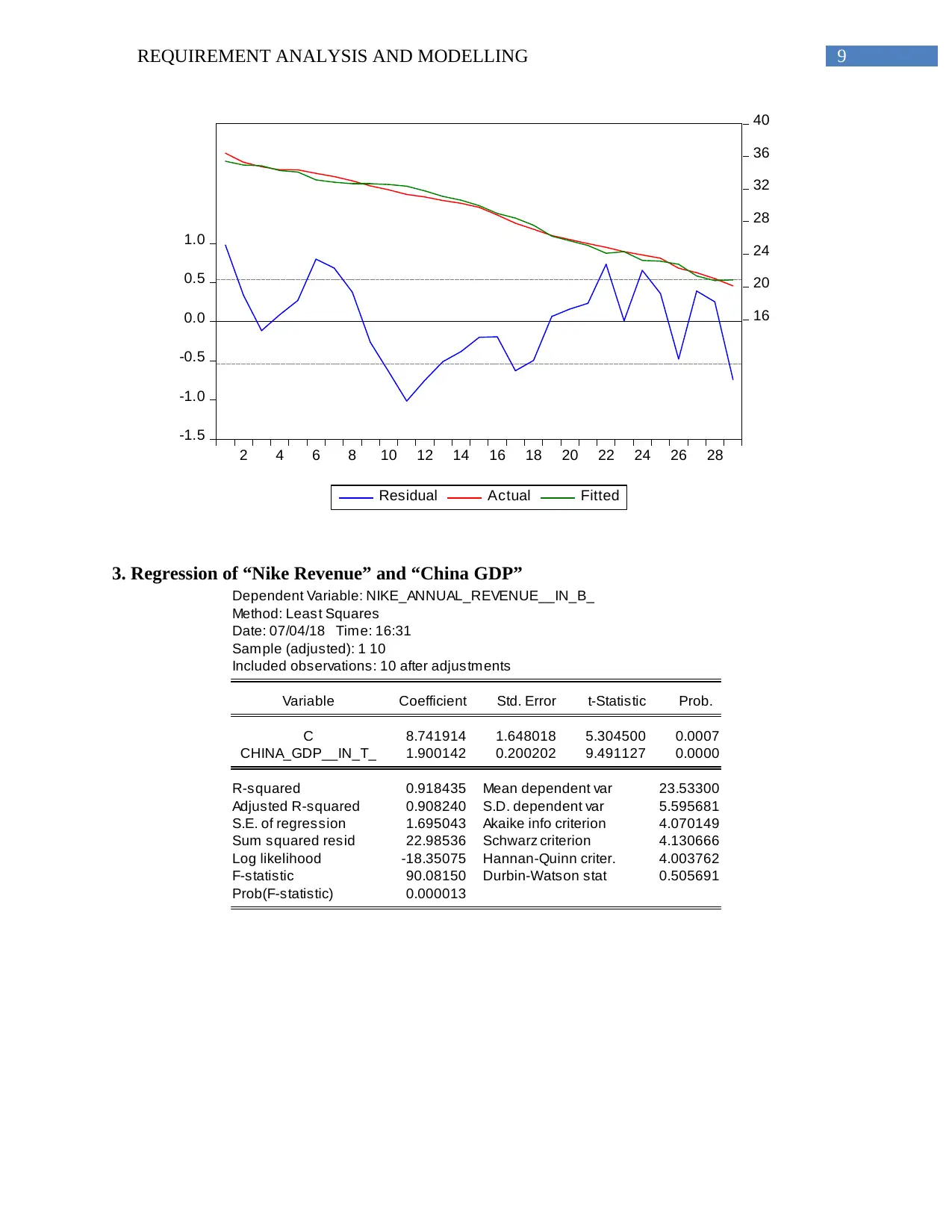
9REQUIREMENT ANALYSIS AND MODELLING
-1.5
-1.0
-0.5
0.0
0.5
1.0
16
20
24
28
32
36
40
2 4 6 8 10 12 14 16 18 20 22 24 26 28
Residual Actual Fitted
3. Regression of “Nike Revenue” and “China GDP”
Dependent Variable: NIKE_ANNUAL_REVENUE__IN_B_
Method: Least Squares
Date: 07/04/18 Time: 16:31
Sample (adjusted): 1 10
Included observations: 10 after adjustments
Variable Coefficient Std. Error t-Statistic Prob.
C 8.741914 1.648018 5.304500 0.0007
CHINA_GDP__IN_T_ 1.900142 0.200202 9.491127 0.0000
R-squared 0.918435 Mean dependent var 23.53300
Adjusted R-squared 0.908240 S.D. dependent var 5.595681
S.E. of regression 1.695043 Akaike info criterion 4.070149
Sum squared resid 22.98536 Schwarz criterion 4.130666
Log likelihood -18.35075 Hannan-Quinn criter. 4.003762
F-statistic 90.08150 Durbin-Watson stat 0.505691
Prob(F-statistic) 0.000013
-1.5
-1.0
-0.5
0.0
0.5
1.0
16
20
24
28
32
36
40
2 4 6 8 10 12 14 16 18 20 22 24 26 28
Residual Actual Fitted
3. Regression of “Nike Revenue” and “China GDP”
Dependent Variable: NIKE_ANNUAL_REVENUE__IN_B_
Method: Least Squares
Date: 07/04/18 Time: 16:31
Sample (adjusted): 1 10
Included observations: 10 after adjustments
Variable Coefficient Std. Error t-Statistic Prob.
C 8.741914 1.648018 5.304500 0.0007
CHINA_GDP__IN_T_ 1.900142 0.200202 9.491127 0.0000
R-squared 0.918435 Mean dependent var 23.53300
Adjusted R-squared 0.908240 S.D. dependent var 5.595681
S.E. of regression 1.695043 Akaike info criterion 4.070149
Sum squared resid 22.98536 Schwarz criterion 4.130666
Log likelihood -18.35075 Hannan-Quinn criter. 4.003762
F-statistic 90.08150 Durbin-Watson stat 0.505691
Prob(F-statistic) 0.000013
Paraphrase This Document
Need a fresh take? Get an instant paraphrase of this document with our AI Paraphraser
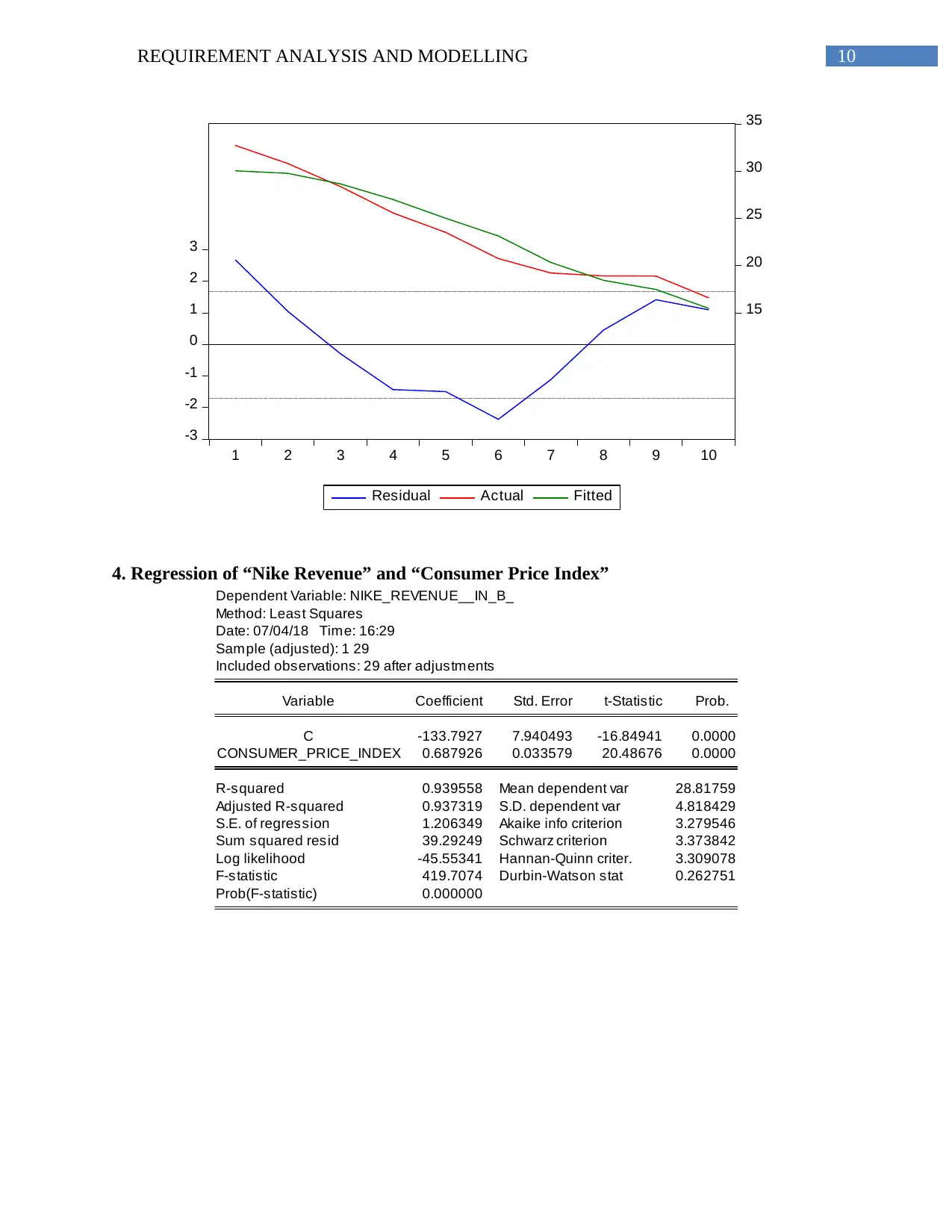
10REQUIREMENT ANALYSIS AND MODELLING
-3
-2
-1
0
1
2
3
15
20
25
30
35
1 2 3 4 5 6 7 8 9 10
Residual Actual Fitted
4. Regression of “Nike Revenue” and “Consumer Price Index”
Dependent Variable: NIKE_REVENUE__IN_B_
Method: Least Squares
Date: 07/04/18 Time: 16:29
Sample (adjusted): 1 29
Included observations: 29 after adjustments
Variable Coefficient Std. Error t-Statistic Prob.
C -133.7927 7.940493 -16.84941 0.0000
CONSUMER_PRICE_INDEX 0.687926 0.033579 20.48676 0.0000
R-squared 0.939558 Mean dependent var 28.81759
Adjusted R-squared 0.937319 S.D. dependent var 4.818429
S.E. of regression 1.206349 Akaike info criterion 3.279546
Sum squared resid 39.29249 Schwarz criterion 3.373842
Log likelihood -45.55341 Hannan-Quinn criter. 3.309078
F-statistic 419.7074 Durbin-Watson stat 0.262751
Prob(F-statistic) 0.000000
-3
-2
-1
0
1
2
3
15
20
25
30
35
1 2 3 4 5 6 7 8 9 10
Residual Actual Fitted
4. Regression of “Nike Revenue” and “Consumer Price Index”
Dependent Variable: NIKE_REVENUE__IN_B_
Method: Least Squares
Date: 07/04/18 Time: 16:29
Sample (adjusted): 1 29
Included observations: 29 after adjustments
Variable Coefficient Std. Error t-Statistic Prob.
C -133.7927 7.940493 -16.84941 0.0000
CONSUMER_PRICE_INDEX 0.687926 0.033579 20.48676 0.0000
R-squared 0.939558 Mean dependent var 28.81759
Adjusted R-squared 0.937319 S.D. dependent var 4.818429
S.E. of regression 1.206349 Akaike info criterion 3.279546
Sum squared resid 39.29249 Schwarz criterion 3.373842
Log likelihood -45.55341 Hannan-Quinn criter. 3.309078
F-statistic 419.7074 Durbin-Watson stat 0.262751
Prob(F-statistic) 0.000000
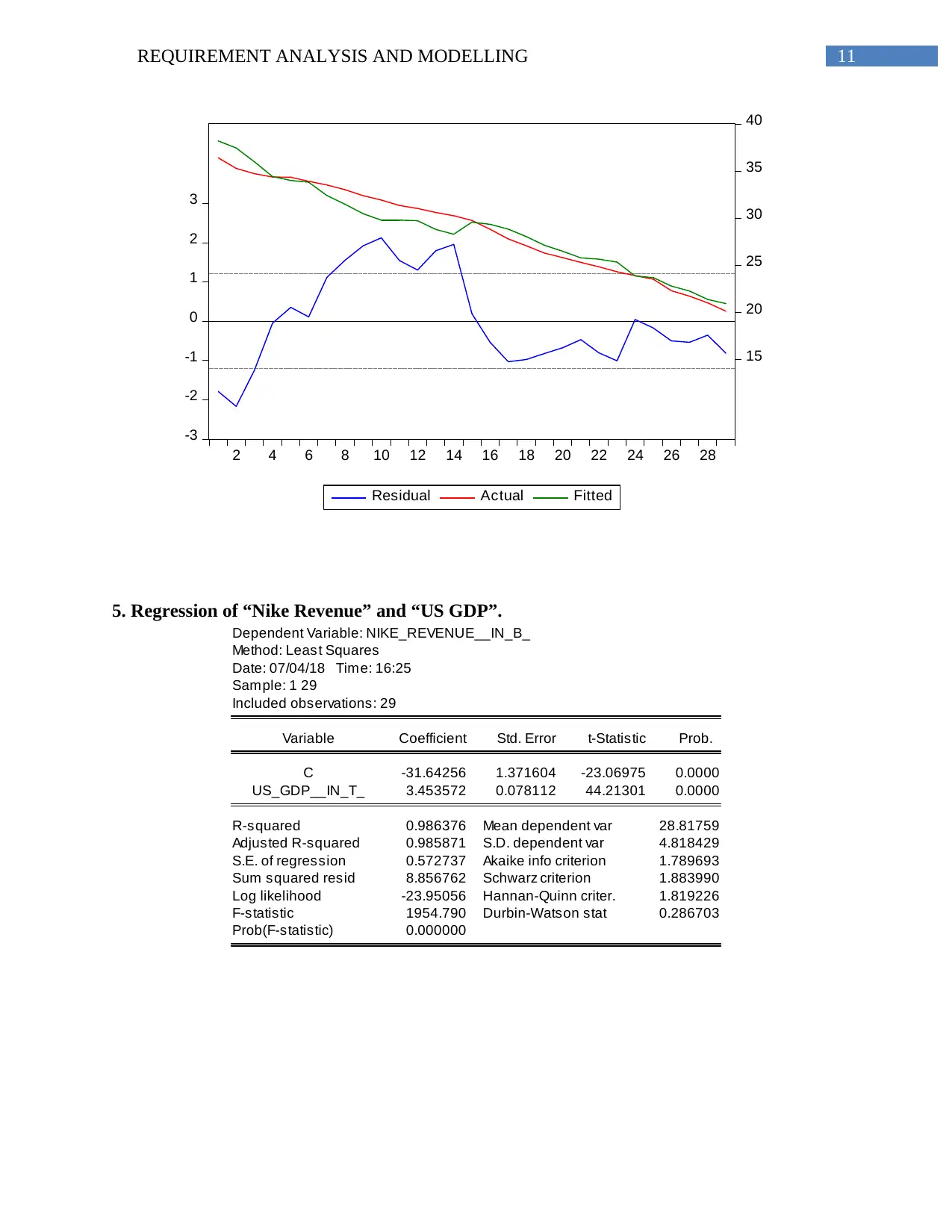
11REQUIREMENT ANALYSIS AND MODELLING
-3
-2
-1
0
1
2
3
15
20
25
30
35
40
2 4 6 8 10 12 14 16 18 20 22 24 26 28
Residual Actual Fitted
5. Regression of “Nike Revenue” and “US GDP”.
Dependent Variable: NIKE_REVENUE__IN_B_
Method: Least Squares
Date: 07/04/18 Time: 16:25
Sample: 1 29
Included observations: 29
Variable Coefficient Std. Error t-Statistic Prob.
C -31.64256 1.371604 -23.06975 0.0000
US_GDP__IN_T_ 3.453572 0.078112 44.21301 0.0000
R-squared 0.986376 Mean dependent var 28.81759
Adjusted R-squared 0.985871 S.D. dependent var 4.818429
S.E. of regression 0.572737 Akaike info criterion 1.789693
Sum squared resid 8.856762 Schwarz criterion 1.883990
Log likelihood -23.95056 Hannan-Quinn criter. 1.819226
F-statistic 1954.790 Durbin-Watson stat 0.286703
Prob(F-statistic) 0.000000
-3
-2
-1
0
1
2
3
15
20
25
30
35
40
2 4 6 8 10 12 14 16 18 20 22 24 26 28
Residual Actual Fitted
5. Regression of “Nike Revenue” and “US GDP”.
Dependent Variable: NIKE_REVENUE__IN_B_
Method: Least Squares
Date: 07/04/18 Time: 16:25
Sample: 1 29
Included observations: 29
Variable Coefficient Std. Error t-Statistic Prob.
C -31.64256 1.371604 -23.06975 0.0000
US_GDP__IN_T_ 3.453572 0.078112 44.21301 0.0000
R-squared 0.986376 Mean dependent var 28.81759
Adjusted R-squared 0.985871 S.D. dependent var 4.818429
S.E. of regression 0.572737 Akaike info criterion 1.789693
Sum squared resid 8.856762 Schwarz criterion 1.883990
Log likelihood -23.95056 Hannan-Quinn criter. 1.819226
F-statistic 1954.790 Durbin-Watson stat 0.286703
Prob(F-statistic) 0.000000
⊘ This is a preview!⊘
Do you want full access?
Subscribe today to unlock all pages.

Trusted by 1+ million students worldwide
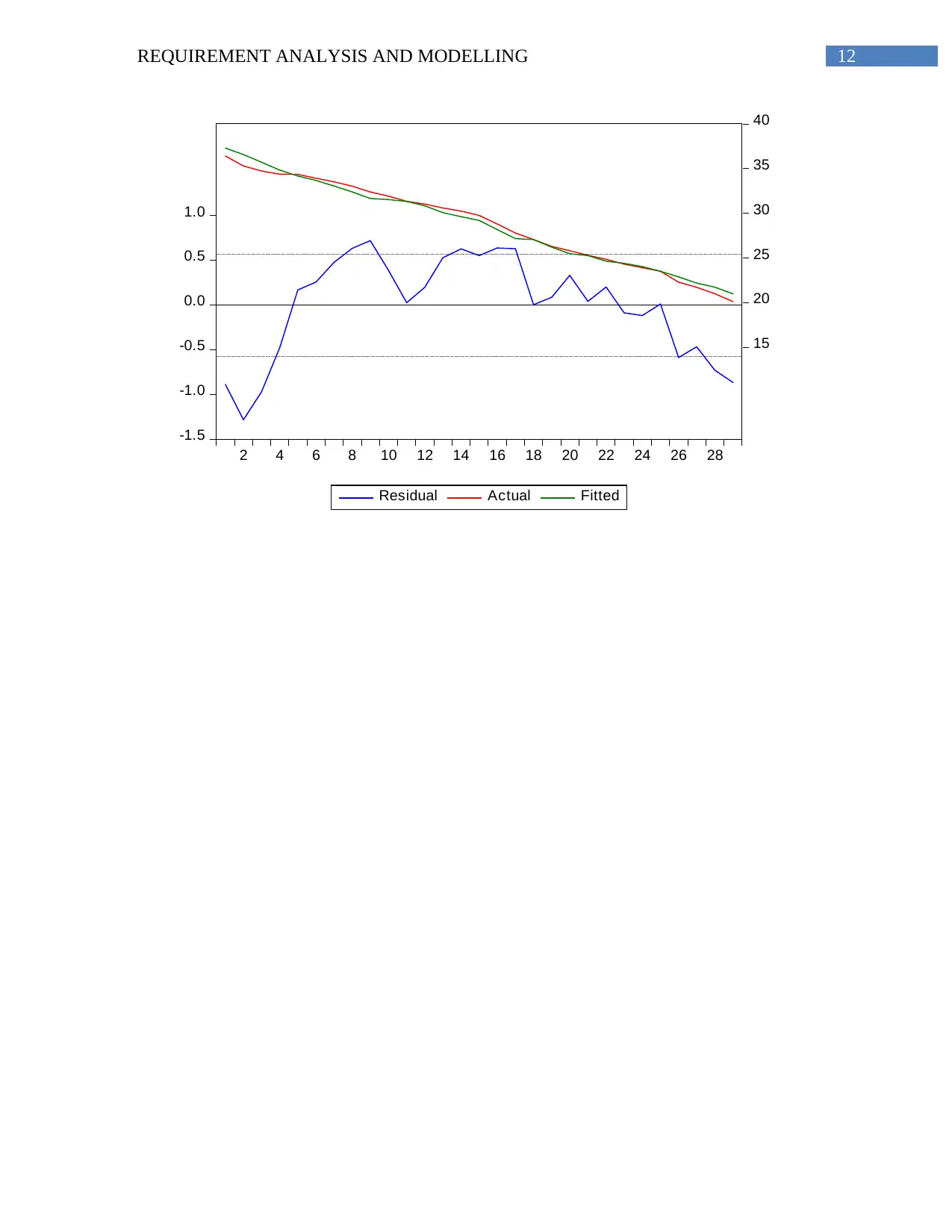
12REQUIREMENT ANALYSIS AND MODELLING
-1.5
-1.0
-0.5
0.0
0.5
1.0
15
20
25
30
35
40
2 4 6 8 10 12 14 16 18 20 22 24 26 28
Residual Actual Fitted
-1.5
-1.0
-0.5
0.0
0.5
1.0
15
20
25
30
35
40
2 4 6 8 10 12 14 16 18 20 22 24 26 28
Residual Actual Fitted
Paraphrase This Document
Need a fresh take? Get an instant paraphrase of this document with our AI Paraphraser
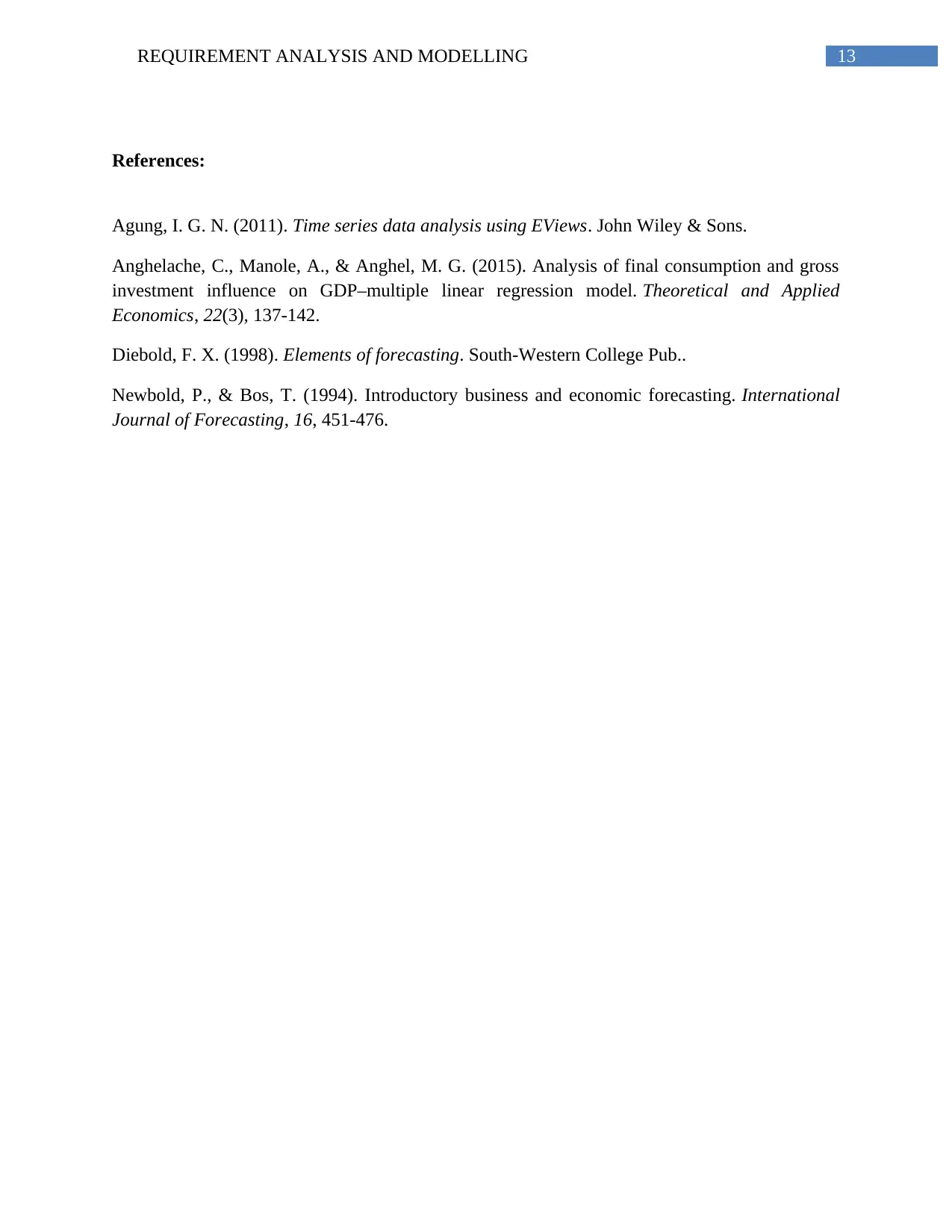
13REQUIREMENT ANALYSIS AND MODELLING
References:
Agung, I. G. N. (2011). Time series data analysis using EViews. John Wiley & Sons.
Anghelache, C., Manole, A., & Anghel, M. G. (2015). Analysis of final consumption and gross
investment influence on GDP–multiple linear regression model. Theoretical and Applied
Economics, 22(3), 137-142.
Diebold, F. X. (1998). Elements of forecasting. South-Western College Pub..
Newbold, P., & Bos, T. (1994). Introductory business and economic forecasting. International
Journal of Forecasting, 16, 451-476.
References:
Agung, I. G. N. (2011). Time series data analysis using EViews. John Wiley & Sons.
Anghelache, C., Manole, A., & Anghel, M. G. (2015). Analysis of final consumption and gross
investment influence on GDP–multiple linear regression model. Theoretical and Applied
Economics, 22(3), 137-142.
Diebold, F. X. (1998). Elements of forecasting. South-Western College Pub..
Newbold, P., & Bos, T. (1994). Introductory business and economic forecasting. International
Journal of Forecasting, 16, 451-476.
1 out of 14
Related Documents

Your All-in-One AI-Powered Toolkit for Academic Success.
+13062052269
info@desklib.com
Available 24*7 on WhatsApp / Email
Unlock your academic potential
© 2024 | Zucol Services PVT LTD | All rights reserved.